- 1Imaging Physics, Delft University of Technology, Delft, Netherlands
- 2Department of Biomedical Sciences of Cells and Systems, University Groningen, University Medical Center Groningen, Groningen, Netherlands
Volume electron microscopy (EM) of biological systems has grown exponentially in recent years due to innovative large-scale imaging approaches. As a standalone imaging method, however, large-scale EM typically has two major limitations: slow rates of acquisition and the difficulty to provide targeted biological information. We developed a 3D image acquisition and reconstruction pipeline that overcomes both of these limitations by using a widefield fluorescence microscope integrated inside of a scanning electron microscope. The workflow consists of acquiring large field of view fluorescence microscopy (FM) images, which guide to regions of interest for successive EM (integrated correlative light and electron microscopy). High precision EM-FM overlay is achieved using cathodoluminescent markers. We conduct a proof-of-concept of our integrated workflow on immunolabelled serial sections of tissues. Acquisitions are limited to regions containing biological targets, expediting total acquisition times and reducing the burden of excess data by tens or hundreds of GBs.
Introduction
A central objective within neuroscience and cell biology is to produce high-resolution (1–10 nm), three-dimensional reconstructions of biological specimen. Volume electron microscopy (EM) is the preferred imaging method in this arena because of its unique ability to resolve features across a wide spectrum of spatial scales (Peddie and Collinson, 2014; Kornfeld and Denk, 2018). While EM provides highly relevant structural information and precise localization of targets, immunogold labeling can only be visualized at high resolution, and in Tokuyasu labeling section areas are typically limited to 0.01 mm2 for analysis (Liou et al., 1996; Van Rijnsoever et al., 2008). Fluorescence microscopy (FM) provides biologically relevant information by tagging specific biomolecules with fluorescent labels at large scale (Giepmans et al., 2006). Regions of interest (ROI) can in this way be quickly and reliably identified for subsequent high magnification EM imaging. The information from these two imaging modalities are combined in correlative light and electron microscopy (CLEM). ROI retrieval across different microscopes is, however, nontrivial at large scales, particularly when spread across multiple sections (Polishchuk et al., 2000; Bishop et al., 2011; Karreman et al., 2014; Collinson et al., 2017; Booth et al., 2019). Other challenges associated with CLEM include the reliance on fiducial markers and intermediate sample preparation (de Boer et al., 2015; Karreman et al., 2016). One means of combating these challenges is by merging these separate imaging systems into a single, integrated fluorescence and electron microscope (Liv et al., 2013). By detecting fluorescence expression in-situ, it can further be decided in an automated fashion which areas to scan at high magnification and which areas to omit for the sake of higher throughput (Delpiano et al., 2018). For array tomography applications, ROIs can be targeted with increasing magnification through a sequence of feedback loops (Gabarre et al., 2021). Similarly, strategies for rapidly screening sections have been developed for sequential CLEM to limit volume acquisitions to select ROI (Burel et al., 2018; Ronchi et al., 2021).
Despite these potential benefits, an integrated microscope presents new challenges. In conventional array tomography sample preparation, the sample is eluted and restained between imaging methods (Micheva and Smith, 2007). Hence, there is no need to preserve fluorescence labelling, which allows for post-staining to enhance EM contrast (Watson, 1958; Tapia et al., 2012). The traditional way to compensate for diminished contrast is to boost the EM signal by increasing the dwell time per pixel, but this comes at the expense of throughput. An additional complication in integrated CLEM is electron-beam-induced quenching of the fluorescence (Srinivasa Raja et al., 2021). This imposes the constraint that the fluorescence in a given area must be acquired prior to exposure from the electron beam, which prohibits uniformly pre-irradiating the sample with the electron beam to enhance and stabilize contrast (Kuipers et al., 2015). Conversely, in conventional serial-section EM, there are scarce constraints regarding the number of times a particular sample may be scanned, making possible approaches such as that by Hildebrand et al. (Hildebrand et al., 2017).
Our goal is to establish a workflow capable of quickly and efficiently rendering three-dimensional CLEM volumes from serial sections in such a way as to overcome these challenges. Three key initiatives steered the design of our integrated correlative array tomography (iCAT) procedure. First, to prevent damaging or quenching of the fluorescence signal via electron-beam irradiation, each FM field of view must be acquired prior to EM exposure. Second, to compensate for the reduced application of contrast agents, backscattered electron (BSE) collection efficiency is enhanced via a negative stage bias, allowing for higher throughput (Bouwer et al., 2016; Lane et al., 2021). Finally, a high precision EM-FM overlay is facilitated by the use of cathodoluminescent (CL) points, which eliminates the need for artificial fiducial markers (Haring et al., 2017). An alignment method was then developed to reconstruct the correlative image stack. Islets of Langerhans from both rat and zebrafish pancreas tissue were chosen to prototype the imaging and reconstruction workflows. By offering a more holistic visualization of tissue, our integrated approach to 3D CLEM could lead to greater insights in (patho)biology (de Boer et al., 2020).
Materials and Methods
Tissue and Sample Preparation
Rat pancreas was prepared as follows: fresh pancreas was cut from an 83 day old rat into small pieces and fixed in 4% paraformaldehyde (PFA; Merck) + 0.1% glutaraldehyde (GA; Polysciences) as described in Ravelli et al. (Ravelli et al., 2013). A complete zebrafish larva (120hpf) was fixed in 2% PFA +2% GA. Both samples were post-fixed in 1% osmium tetroxide and 1.5% potassium ferricyanide in 0.1 M cacodylate buffer, dehydrated through ethanol and embedded in EPON (Serva). 100nm serial sections were cut and placed onto formvar-covered ITO-coated glass coverslips (Optics Balzers). Immunolabeling was performed as described previously (Kuipers et al., 2015). Samples were etched with 1% periodic acid for 10 min, followed by a 30 min blocking step: 1% bovine serum albumin (BSA; Sanquin, Netherlands) in tris-buffered saline (TBS), pH 7.4. Next, anti-insulin was incubated for 2 h (guinea pig; 1:50, Invitrogen, PA1-26938, RRID: AB_794668, for rat pancreas and anti-insulin; 1:100, Abcam, ab210560, for zebrafish pancreas), followed by washing and subsequent incubation for 1 h with biotinylated secondary antibody (donkey-anti-guinea pig; 1:400, Jackson Immunoresearch, for rat pancreas and goat-anti-rabbit; 1:400, Dako, for zebrafish pancreas) followed by washing steps. Finally, streptavidin conjugated AF594 (1:100, Jackson Immunoresearch, for rat pancreas) and streptavidin conjugated TRITC (1:100, Jackson Immunoresearch, for zebrafish pancreas) were added for 1 h followed by washing.
Digital Light Microscopy
The sections, after being placed on the ITO-coated glass slide, are imaged at 30X magnification (∼7 μm px−1) using a VHX-6000 digital light microscope (Keyence) operating in reflection mode. To capture every section on the 22 mm × 22 mm ITO-coated glass slide, a 3 × 3 grid of RGB images is acquired and automatically stitched together.
Integrated Microscopy
The integrated microscope is a widefield SECOM fluorescence microscope (Delmic B.V.) retrofitted into the vacuum chamber of a Verios 460 SEM (Thermo Fisher Scientific) (Liv et al., 2013; Zonnevylle et al., 2013). The microscopes share a common optical axis, translation stage, and control software. FM images are obtained with 10s exposures, recorded by a Zyla 4.2 sCMOS camera (Andor—Oxford Instruments). Excitation wavelengths of 405 and 555 nm are used to excite Hoechst and AF594. The SECOM is equipped with a CFI S Plan Fluor ELWD 60XC (0.70 NA) objective (Nikon), which allows for long working distance imaging (1.8–2.6 mm), to prevent electrical breakdown in vacuum, which must be accounted for due to the presence of high electric fields induced by the stage bias (Vos et al., 2021).
SEM imaging is conducted in two rounds: 1) low-magnification (38 nm px−1) scans accompanying each fluorescent acquisition; 2) high-magnification (5 nm px−1) acquisitions on ROI identified by fluorescence expression. Both low and high magnification imaging are performed at 2.5 keV primary beam energy with a −1 kV bias potential applied to the sample stage such that the landing energy is 1.5 keV, which proved optimal for ∼100 nm sections. The negative potential bias enhances the backscattered electron (BSE) signal, which is collected by the insertable concentric backscattered detector (Thermo Fisher Scientific) (Lane et al., 2021).
Alignment and Reconstruction Software
Image data from the integrated microscope is uploaded to a local storage server running an instance of render-ws1, a collection of open-source web services for rendering transformed image tiles. The tiles and their respective metadata are organized into stacks, configured as MongoDB databases. The alignment routines are arranged in a series of Jupyter notebooks2, which parse the image metadata for the EM-FM overlay as well as make calls to render-ws via a python wrapper (render-python3). EM image stitching and volume alignment are based on the scale-invariant feature transform (SIFT)—an algorithm designed to detect and match local features in corresponding images (Lowe, 1999). SIFT features are stored in render-ws databases where they can be processed by BigFeta4, a linear least squares solver for scalable 2D and 3D image alignment based on point correspondences. CLEM datasets are ultimately exported to CATMAID (Saalfeld et al., 2009) for google-maps-like visualization. 3D visualizations are done in Fiji (Schindelin et al., 2012) using the Volume Viewer plugin5.
Results
Section Detection and In-Situ Navigation
Simple navigation between serial sections within the integrated microscope is crucial. Following joint EM-FM sample preparation (Figure 1A), the sections are imaged by a digital light microscope (DLM) (Figure 1B). To facilitate navigation within the integrated microscope (Figure 1C), the resulting overview image is used for detecting the boundaries of each section on the ITO-coated glass substrate via a segmentation routine6 (Figure 1D). The overview image (inset 1) is first contrast enhanced and converted to grayscale (inset 2). Intensity-based thresholding is used to create a binary mask image (inset 3), which is then applied to the grayscale image. To retrieve outlines of the section boundaries, the gradient is computed (inset 4). Watershed segmentation is then implemented by flooding the gradient image with a number of markers equal to the number of serial sections in the image (inset 5). The resulting labelled image (inset 6) then serves as input for navigation using a plugin within Odemis7, the open-source software that controls the microscope (Figure 1E).
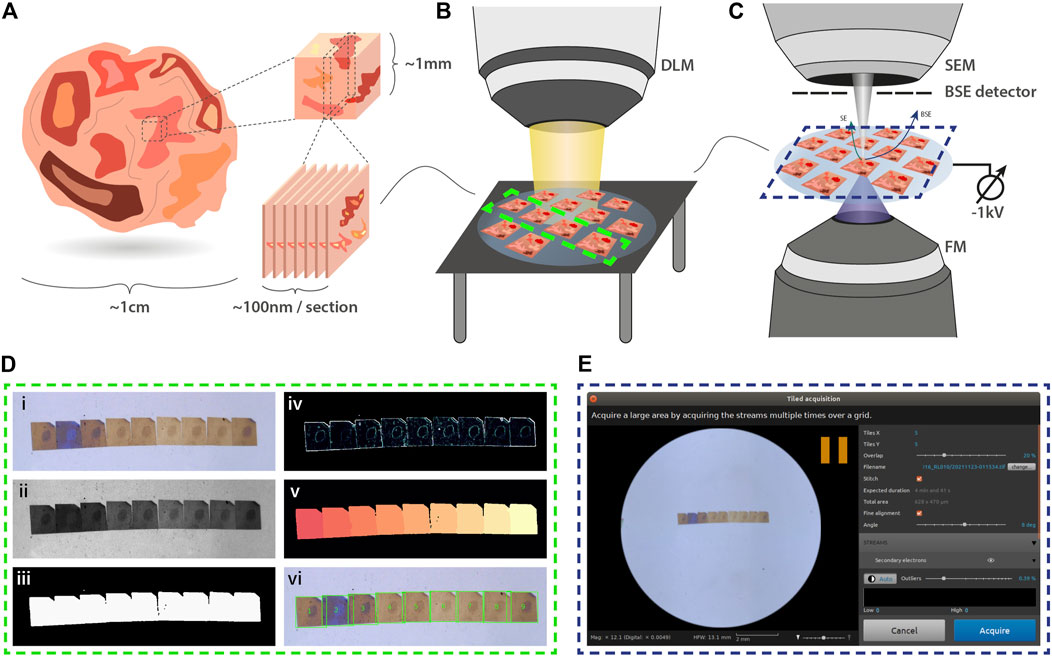
FIGURE 1. Integrated array tomography. (A) Ultrathin sections are prepared for simultaneous FM and EM imaging. (B) An overview image of the sections on the ITO-coated glass slide is then acquired by a digital light microscope (DLM). (C) Next, the sample is transferred to the integrated microscope for CLEM imaging. SEM imaging is performed using a −1kV bias potential applied to the sample stage to enhance BSE collection from the non-post-stained sections. (D) The overview image obtained by the DLM is used for instance segmentation of the serial sections. Descriptions of the image processing steps (i-vi) is provided in the main text. (E) The section overview image and bounding box coordinates of each serial section are fed to the microscope software to facilitate navigation.
Targeted Correlative Acquisition of an Individual Region of Interest
To identify ROI in the integrated microscope for subsequent EM acquisition, the correlative imaging scheme is engineered to obtain fluorescence overviews of each section, undamaged by the electron beam. The workflow starts by acquiring a FM and low-magnification EM image tile (Figure 2A). The FM tile is acquired prior to the EM tile to preserve the fluorescence signal. An automated registration routine guided by cathodoluminescent (CL) spots is then run to register the image pair (Haring et al., 2017). This sequence of correlative imaging is automatically repeated in a grid-like pattern, encompassing the entire section. FM image tiles are acquired with a 20% overlap such that they can be stitched together to allow for fluorescence-based ROI detection within each section (Figure 2B). The field width of the EM tile (∼140 μm) is chosen such that it spans the maximum extent possible without entering the overlap region of the neighboring FM image tiles,
where
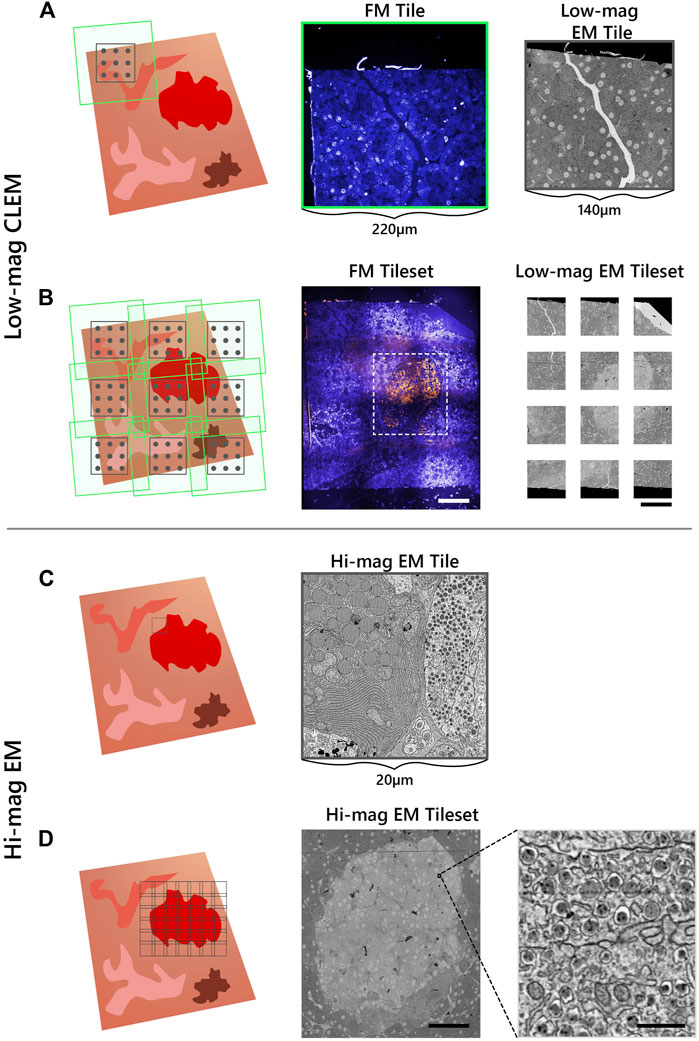
FIGURE 2. Integrated array tomography provides efficient, high-precision EM-FM imaging without bleaching of the fluorescence. (A) Acquisition of correlative FM (green outline) and low-magnification EM (black outline) images, followed by a registration procedure involving CL spots (grey circles) to register the image pair. (B) The stage is translated in a grid-like fashion such that there is sufficient overlap between neighboring FM image tiles—leaving a gap between adjacent EM tiles. (C) The fluorescence signal is used to identify targets for subsequent EM imaging (black outline). (D) The target ROI is captured by an automated tileset of high magnification EM tiles. Scalebars: (B) 100μm; (D) 50 μm (inset, 0.5 μm).
Fluorescence expression is then used to target areas for additional EM imaging at higher magnification (5 nm px−1) (Figure 2C). The ROI is manually navigated to via stage translation, whereby an automated tileset acquisition is initiated (Figure 2D). The tiles are spaced with a 10–15% overlap such that they can be stitched during post-processing. The correlative imaging pipeline is then repeated on the remaining serial sections.
2D Stitching and Correlation
Overlaying the fluorescence onto the high-magnification EM requires correlating the datasets across different modalities and spatial scales. Each FM tile is first overlaid onto the corresponding low-magnification EM tile using the metadata generated by the CL registration procedure. A grid of CL spots is recorded with the camera of the fluorescence microscope in the absence of excitation light (Figure 3A). The appropriate affine transformation is calculated by localizing each CL spot and matching it with the known position of the electron beam (“cross-modal” registration) (Haring et al., 2017). The stage coordinates are extracted to then correlate and position each image pair in the tileset (Figure 3A).
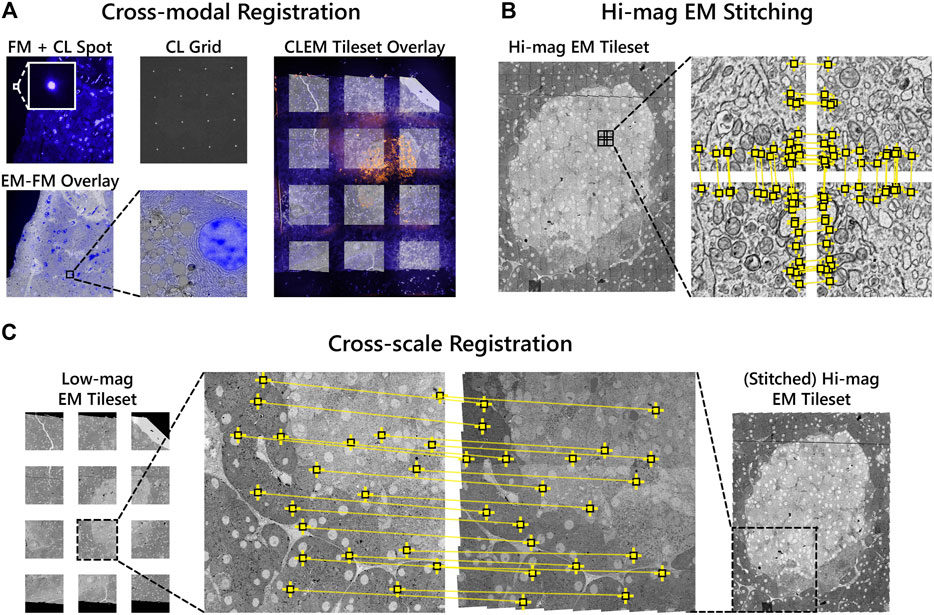
FIGURE 3. Correlative alignment routine registers tilesets across modalities and scales. (A) Automated registration procedure for registering FM and low-magnification EM image pairs using CL spots. FM tiles are then overlaid onto the low-magnification EM tiles of each section. (B) SIFT features (yellow squares) are extracted and used to stitch together neighboring high-magnification EM tiles within each section. (C) Low-magnification EM images are registered to the corresponding area of the stitched together high-magnification EM tileset. The low-magnification tiles thereby serve as a reference to ultimately overlay the fluorescence onto the high-magnification EM.
The high-magnification EM tileset is stitched independently of both the FM and low-magnification EM tiles (Figure 3B). Stage coordinates are used to first establish a set of potential neighboring tiles. For each tile, SIFT features are extracted and matched between the candidate neighbors. Affine transformation parameters for each tile are then estimated by minimizing the squared distance between corresponding features (Saalfeld et al., 2012; Khairy et al., 2018).
Next, the low-magnification image tiles are registered to the corresponding area of the stitched high-magnification EM tileset (“cross-spatial” registration, Figure 3C). Stage coordinates are used to determine the set of high-magnification tiles that overlap with each low-magnification tile. A composite image of the overlapping tiles is rendered, processed with SIFT, and matched with the features in the low-magnification tile. The affine transformation computed from the feature matching is then propagated to each of the FM tiles such that they are overlaid onto the high-magnification EM tileset. In this way, the low-magnification EM serves as a proxy to correlate the fluorescence to the high-magnification EM. The overlay accuracy is reduced in the areas between low-magnification tiles where the transformation is extrapolated (Supplementary Figure S1A—F). This can be corrected for via (manual) landmark registration by e.g. aligning the Hoechst signal to nuclei recognized in the EM using software such as ec-CLEM (Paul-Gilloteaux et al., 2017), which is routinely used for image registration in sequential CLEM experiments (Franke et al., 2019; Tuijtel et al., 2019; Lee et al., 2020). In general, the overlay accuracy cannot be expected to be below the pixel size of the low magnification EM.
Correlative 3D Reconstruction
A robust and scalable solution is required for volume alignment of the high-magnification EM stack, the “backbone” of the multimodal dataset. The stitched sections are downsampled and roughly aligned in
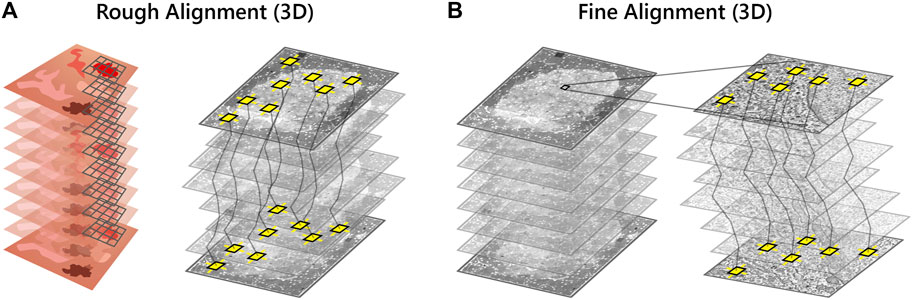
FIGURE 4. Volume reconstruction of the high-magnification EM stack. (A) SIFT features (yellow squares) are used to roughly align the high-magnification EM stack in z. A downsampled image of each section is rendered as the full resolution EM tileset is too large (several GB) for feature extraction. (B) The EM stack alignment is refined by least squares optimization of the displacement between matched features.
The 2D correlative alignment procedure (Figure 3) is then run on each section, mapping the fluorescence onto the high-magnification EM volume. The nine serial sections of rat pancreas were thereby used to realize a proof-of-concept of the iCAT workflow (Figure 5A). An islet of Langerhans was identified from anti-insulin immunofluorescence of AF594 and chosen for subsequent, high-magnification EM imaging (Figure 5B). The fluorescence data clearly delineates the endocrine region from the surrounding exocrine tissue, which is characterized by dense endoplasmic reticulum (ER) and the absence of insulin labeling (Figure 5C). Although it was chosen as a nuclear marker, Hoechst also binds to the RNA present in the ER. The endocrine region, in contrast, is characterized by an abundance of insulin-secreting beta cells with distinct nuclei. The high EM-FM registration accuracy afforded by iCAT enables a clear distinction between different types of granules present in the endocrine tissue (Figure 5D). Discerning insulin from other hormone granules is nontrivial as all are roughly 100 nm in diameter. Making this differentiation from EM data alone requires expert-level interpretation.
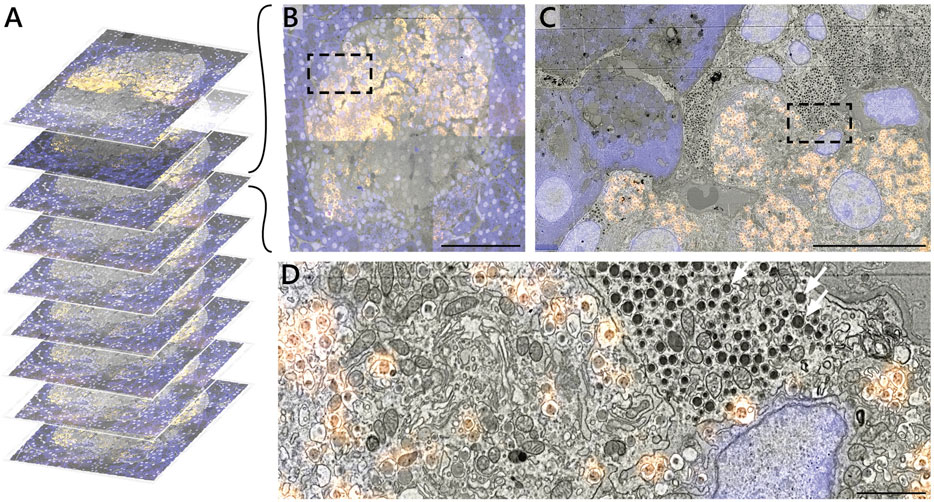
FIGURE 5. Correlative reconstruction of nine sections of pancreas. (A) 2D and 3D alignment routines are combined to yield a CLEM stack of nine serial sections of rat pancreas tissue. (B–D) CLEM imaging of the islet of Langerhans at varying spatial resolution. Hoechst (blue) and AF594 (orange) fluorescence signals are superimposed onto the EM ultrastructure. The AF594 signal, in particular, facilitates recognition of insulin granules from e.g. non-fluorescent glucagon granules (white arrows, D). Scale bars: (B) 50μm; (C) 5μm; (D) 1 μm. Raw data at full resolution is available via Nanotomy.
By limiting high-magnification EM to only the islet, the total imaging volume is reduced by a factor ∼10 with respect to the full section volume (0.03 mm2 per islet vs 0.4 mm2 per section). Similar reductions are realized in the total dataset size (0.1 vs ∼1 TB) easing data management requirements. This initial proof of concept was designed around only a limited number of serial sections to more efficiently optimize each procedure in the workflow.
Proof of Concept on Zebrafish Pancreas Tissue
To demonstrate the scalability of the workflow, we applied it to a larger volume of larval zebrafish (Figure 6). The Hoechst signal was useful in identifying the exocrine region of the pancreas (Figure 6B) as the insulin immunofluorescence from TRITC was weak. TRITC was chosen for its stronger fluorescence in vacuum compared to Alexa dyes (manuscript in preparation); potential causes for the weak immunofluorescence in the zebrafish pancreas are still under investigation. The exocrine region, encompassing an islet of Langerhans, together with the underlying muscle tissue was selected for high magnification EM. The reconstruction of the CLEM volume was cropped to remove the background fluorescence in the swim bladder (Figure 6C). Sub-stacks within the correlative volume were then extracted for further analysis (Figures 6D,G). Note that the ultrastructure is better preserved in most tissues than in the islet (Figures 6H,I), a phenomenon previously seen in other species (unpublished results).
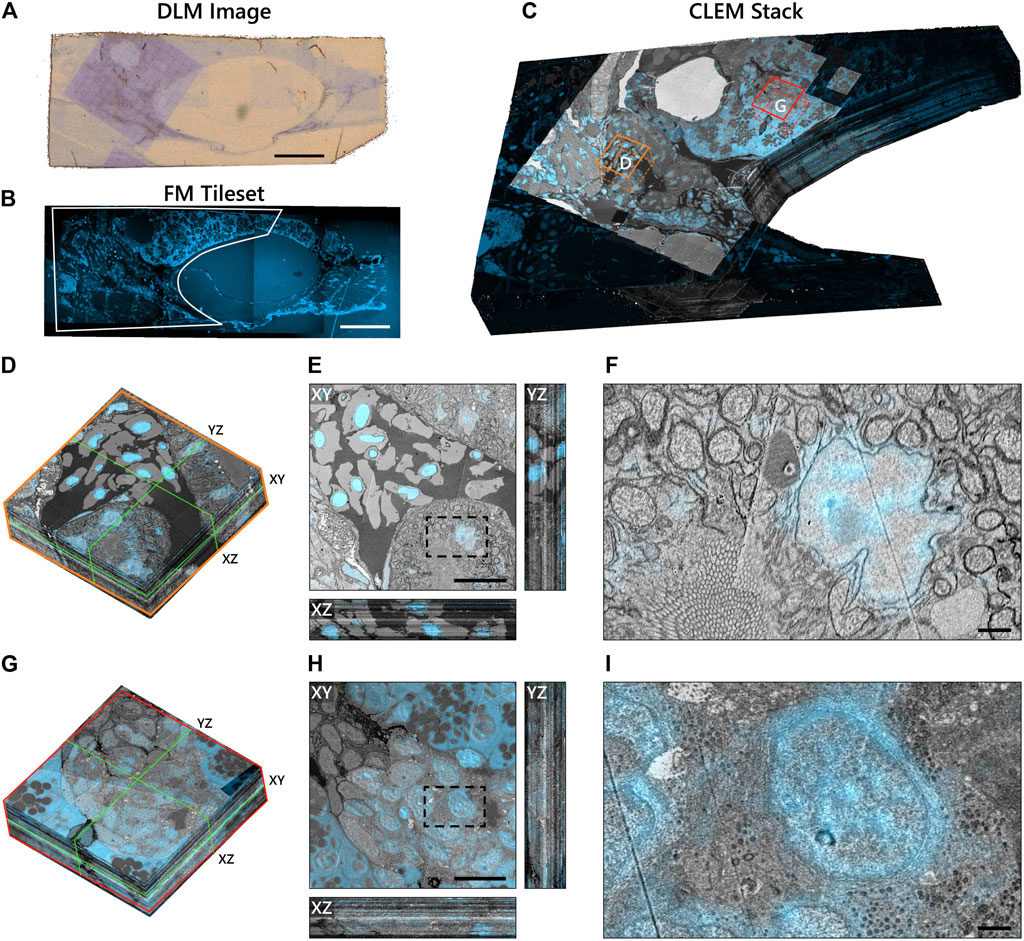
FIGURE 6. Integrated correlative array tomography applied to 63 serial sections of zebrafish pancreas. (A) Contrast-enhanced optical image of an individual serial section obtained by the DLM. The section was re-acquired post-EM imaging, revealing the region of interest irradiated by the electron beam. The biological material can be seen in pale blue in contrast with the bare EPON (brown background). (B) Hoechst signal of an individual serial section, outlining (white) the portion of the tissue shown in (C). (C) CLEM volume of the zebrafish tissue cropped to the ROI selected for high-magnification EM imaging—plus a portion of the surrounding fluorescence signal. Sub-stacks for inspection are denoted by orange (D–F) and red (G–I) boxes. (D) 3D sub-stack of muscle tissue within the zebrafish pancreas. Green lines indicate orthoslices of the XY, XZ, and YZ planes shown in (E). (F) Zoomed-in region of the XY plane showing high-precision FM overlay of Hoechst onto a cell nucleus. (G–I) Same as in (D–F), but for an islet of Langerhans. Ill-defined cell and organelle membranes in (H) and (I) indicate suboptimal preservation of the ultrastructure in this region of the pancreas. Scale bars: (A,B) 100μm; (E,H) 10μm; (F,I) 1 μm. Raw data at full resolution is available via Nanotomy.
We generally observe high EM-FM overlay precision as evidenced by the Hoechst signal confined to the nuclear envelope in the muscle tissue (Figures 6E,F). The registration accuracy does, however, exhibit variability in several sections (Movies A.1 and A.2)—more so than is seen in the rat pancreas tissue where it appears limited to the gaps between low-magnification EM tiles. In these instances, the inaccuracy stems from a malfunction in the CL registration procedure itself (Supplementary Figure S1G—N). Variations in the EM image intensity, particularly in the islet, can also be observed for a number of sections (Figure 6H: XZ and YZ cross-sections). We attribute these artifacts primarily to ultrastructure preservation as they do not appear to be as prevalent in the muscle tissue. While the SEM imaging parameters and detection settings were held constant throughout the acquisition, day-to-day changes in the environment (e.g. temperature, humidity levels) may have varied.
In total, 66 sections were prepared, of which three (z = 9, 10, 34) were discarded due to excess surface debris. The omission of consecutive sections was mitigated by extending the SIFT feature depth search from 2 to 3 such that sections z = 8 and z = 11 could be registered. Total acquisition times for low-magnification CLEM and high-magnification EM were 7.2 and 71 h respectively, versus 335 h for full section imaging at high-magnification.
Discussion
A new workflow for integrated array tomography for the semi-automated acquisition and reconstruction of volume CLEM data is presented. High-resolution EM is limited to select ROI by targeting areas based on fluorescence expression. This not only expedites acquisition time, but eases the burden on data management requirements. Interpretation of EM data is in turn facilitated by the addition of fluorescent labels. The workflow demonstrated here extends the work of Liv et al. (Liv et al., 2013), which introduced the integrated microscope, and Haring et al. (Haring et al., 2017), which presented the fiducial-free CL registration procedure, to targeted correlative imaging of serial sections. Gabarre et al. (Gabarre et al., 2021) presented an alternative method for integrated array tomography in which light microscopy and EM are combined to localize structures through a series of feedback loops. Our approach differs in several ways. First, fluorescence imaging is done in-vacuo as opposed to transmitted light microscopy done at ambient pressure. This allows for more automated EM-FM (or EM-LM) overlay, as the CL registration procedure can only be done in high vacuum (Haring et al., 2017). Additionally, the multi-modal alignment methodology conceived here offers a more scalable solution for generating volumetric CLEM data. Integrated array tomography was inspired in part by the multi-scale approach of Hildebrand et al. (Hildebrand et al., 2017), in which full brain EM imaging of a larval zebrafish was conducted by selecting ROI for subsequent acquisition based on inspection between imaging rounds. In this work, conversely, ROI are identified by in-situ fluorescence, bypassing the need for post-processing and alignment between magnification scales.
On-section immunofluorescence and fluorescent staining constitute viable options for FM imaging of resin-embedded sections in high vacuum. Pancreas tissue in particular is well-suited for immunofluorescence due to the prevalence of insulin epitopes. While in both nature and technique development, immunolabeling approaches are always dependent on the capacity for antibodies and epitopes to interact, this is typically inefficient for most antibodies, and particularly so for EPON-embedded sections. We find that approximately 1 in 10 antibodies tested in our lab are applicable for EPON labeling. While acrylic resins (e.g. Lowicryl, LR White) have been shown to be more compatible with immunolabeling, a trade-off must be made between the strength of the fluorescence signal and the quality of the ultrastructure (Watanabe et al., 2011; Paez-Segala et al., 2015). Complications with serial sectioning and ultrastructure preservation (beyond that shown in the zebrafish pancreas) arose when experimenting with Lowicryl; hence EPON was selected as the embedding medium for this study.
Probes typically used for live FM, such as fluorescent proteins, are likewise incompatible with conventional EM sample preparation techniques (de Boer et al., 2015). Although protocols have been developed for retaining fluorescence post-embedding (Kukulski et al., 2011; Watanabe et al., 2011; Peddie et al., 2014; Fu et al., 2020), the same compromises exist between fluorescence retention and ultrastructure preservation. Fluorescent proteins have the additional limitation that the specimen must be genetically modified, rendering them unsuitable for use in native animals and humans. In-resin fluorescence preservation thus remains a challenge—only made more difficult by imposing high vacuum conditions, which may lower fluorescence intensities for biological probes typically optimized for use in aqueous environments (Peddie et al., 2014). We are nevertheless confident that future developments in fluorescent proteins and embedding media will present compelling opportunities to apply integrated array tomography to a variety of biological questions.
We foresee that the multimodal datasets obtained using this method will be instrumental in forthcoming machine learning applications (Eckstein et al., 2020; Liu et al., 2020; Heinrich et al., 2021). Thus far, applications of registered EM-FM datasets appear to be limited to facilitating registration of sequential CLEM data using artificial predictions for the fluorescence signal (Ounkomol et al., 2018; Seifert et al., 2020). Volume EM datasets, particularly in connectomics, are now routinely segmented via deep convolutional neural networks (Buhmann et al., 2021; Heinrich et al., 2021). Acquisition rates and manual annotation of datasets, however, both serve as bottlenecks for reconstructing dense networks of cells and organelles (Kornfeld and Denk, 2018). Given its ability to provide labeled biological information as well as reduce imaging volumes to select regions, integrated array tomography is poised to deliver significant gains in this arena.
Future work will be directed towards further refinement and automation. The CL registration procedure could be made more robust by illuminating the sample with a greater number of CL spots or by increasing the camera integration time. Updates to the alignment software could furthermore allow for the distortion field correction used in Haring et al. (Haring et al., 2017) to achieve sub-5nm overlay precision. Cutting sections manually remains a significant bottleneck for throughput, as it is prone to error and requires expert training (Wanner et al., 2015). We expanded from a single section to nine, to 63, and have now placed more than 100 serial sections onto ITO-coated coverslips. Increasing beyond ∼10 μm of biological material, however, is cumbersome without more sophisticated sectioning techniques such as automated tape-collecting ultramicrotome (ATUM) (Hayworth et al., 2014) or magnetic collection (Templier, 2019). These may introduce their respective complications; ATUM, for example, is designed to collect sections on (opaque) Kapton tape. More extensive automation strategies can alternatively be applied to the correlative imaging pipeline. Delpiano et al. (Delpiano et al., 2018) devised a way to automatically detect fluorescent cells using an integrated light and electron microscope. We envision a workflow for fully automated integrated array tomography in which fluorescent ROIs are automatically recognized, navigated to, and acquired, rendering three-dimensional CLEM datasets tailored to answer the specific biological research question.
Data Availability Statement
The datasets presented in this study are available from the Nanotomy repository (http://www.nanotomy.org/OA/Lane2022FiCD/).
Ethics Statement
The animals used in this study were fed ad libitum with food pellets conducted according to the guidelines for Ethics Committee and Animal Experimentation, University of Groningen.
Author Contributions
RL designed and performed the experiments, created the figures, drafted and edited the manuscript. AW prepared the samples for integrated array tomography. BG and JH provided guidance, conceived the study, and edited the manuscript.
Funding
This research is financially supported by the Dutch Research Council (NWO) through a Building Blocks of Life grant (737.016.010), NWO Microscopy Valley 12718, Netherlands Electron Microscopy Infrastructure (NEMI), NWO National Roadmap for Large-Scale Research Infrastructure of the Dutch Research Council (NWO 184.034.014), and a ZonMW DTL hotel grant (435005035).
Conflict of Interest
RL, AHGW, and BNGG declare that the research was conducted in the absence of any commercial or financial relationships that could be construed as a potential conflict of interest. The integrated microscope used in this study is a product of Delmic B.V. of which JPH is a co-founder and shareholder.
Publisher’s Note
All claims expressed in this article are solely those of the authors and do not necessarily represent those of their affiliated organizations, or those of the publisher, the editors, and the reviewers. Any product that may be evaluated in this article, or claim that may be made by its manufacturer, is not guaranteed or endorsed by the publisher.
Acknowledgments
We would like to thank Eric Piel, Philip Winkler, and Andries Effting for technical support and software development related to the SECOM; Isabel Postmes and Daan Gieles for assisting in the development of the image processing routine for section detection; and Elizabeth Carroll for guidance on zebrafish anatomy.
Supplementary Material
The Supplementary Material for this article can be found online at: https://www.frontiersin.org/articles/10.3389/fmolb.2021.822232/full#supplementary-material
Footnotes
1https://github.com/saalfeldlab/render.
2https://github.com/hoogenboom-group/iCAT-workflow.
3https://github.com/AllenInstitute/render-python.
4https://github.com/AllenInstitute/BigFeta.
5https://imagej.net/plugins/volume-viewer.
6https://github.com/hoogenboom-group/secdetect.
7https://github.com/delmic/odemis.
References
Bishop, D., Nikić, I., Brinkoetter, M., Knecht, S., Potz, S., Kerschensteiner, M., et al. (2011). Near-infrared Branding Efficiently Correlates Light and Electron Microscopy. Nat. Methods 8, 568–570. doi:10.1038/nmeth.1622
Booth, D. G., Beckett, A. J., Prior, I. A., and Meijer, D. (2019). SuperCLEM: an Accessible Correlative Light and Electron Microscopy Approach for Investigation of Neurons and Glia In Vitro. Biol. Open 8 (5), bio042085. doi:10.1242/bio.042085
Bouwer, J. C., Deerinck, T. J., Bushong, E., Astakhov, V., Ramachandra, R., Peltier, S. T., et al. (2016). Deceleration of Probe Beam by Stage Bias Potential Improves Resolution of Serial Block-Face Scanning Electron Microscopic Images. Adv. Struct. Chem. Imaging 2 (1), 11–13. doi:10.1186/s40679-016-0025-y
Buhmann, J., Sheridan, A., Malin-Mayor, C., Schlegel, P., Gerhard, S., Kazimiers, T., et al. (2021). Automatic Detection of Synaptic Partners in a Whole-Brain Drosophila Electron Microscopy Data Set. Nat. Methods 18, 771–774. doi:10.1038/s41592-021-01183-7
Burel, A., Lavault, M. T., Chevalier, C., Gnaegi, H., Prigent, S., Mucciolo, A., et al. (2018). A Targeted 3D EM and Correlative Microscopy Method Using SEM Array Tomography. Development 145 (12), dev160879. doi:10.1242/dev.160879
Collinson, L. M., Carroll, E. C., and Hoogenboom, J. P. (2017). Correlating 3D Light to 3D Electron Microscopy for Systems Biology. Curr. Opin. Biomed. Eng. 3, 49–55. doi:10.1016/j.cobme.2017.10.006
de Boer, P., Hoogenboom, J. P., and Giepmans, B. N. G. (2015). Correlated Light and Electron Microscopy: Ultrastructure Lights up!. Nat. Methods 12, 503–513. doi:10.1038/nmeth.3400
de Boer, P., Pirozzi, N. M., Wolters, A. H. G., Kuipers, J., Kusmartseva, I., Atkinson, M. A., et al. (2020). Large-scale Electron Microscopy Database for Human Type 1 Diabetes. Nat. Commun. 11 (1), 2475–2479. doi:10.1038/s41467-020-16287-5
Delpiano, J., Pizarro, L., Peddie, C. J., Jones, M. L., Griffin, L. D., and Collinson, L. M. (2018). Automated Detection of Fluorescent Cells in In-Resin Fluorescence Sections for Integrated Light and Electron Microscopy. J. Microsc. 271 (1), 109–119. doi:10.1111/jmi.12700
Eckstein, N., Buhmann, J., Cook, M., and Funke, J. (2020). “Microtubule Tracking in Electron Microscopy Volumes,” in International Conference on Medical Image Computing and Computer-Assisted Intervention, Lima, Peru, October 4–8, 2020 (Springer), 99–108. doi:10.1007/978-3-030-59722-1_10
Franke, C., Repnik, U., Segeletz, S., Brouilly, N., Kalaidzidis, Y., Verbavatz, J. M., et al. (2019). Correlative Single‐molecule Localization Microscopy and Electron Tomography Reveals Endosome Nanoscale Domains. Traffic 20 (8), 601–617. doi:10.1111/tra.12671
Fu, Z., Peng, D., Zhang, M., Xue, F., Zhang, R., He, W., et al. (2020). mEosEM Withstands Osmium Staining and Epon Embedding for Super-resolution CLEM. Nat. Methods 17 (1), 55–58. doi:10.1038/s41592-019-0613-6
Gabarre, S., Vernaillen, F., Baatsen, P., Vints, K., Cawthorne, C., Boeynaems, S., et al. (2021). A Workflow for Streamlined Acquisition and Correlation of Serial Regions of Interest in Array Tomography. BMC Biol. 19 (1), 1–15. doi:10.1186/s12915-021-01072-7
Giepmans, B. N., Adams, S. R., Ellisman, M. H., and Tsien, R. Y. (2006). The Fluorescent Toolbox for Assessing Protein Location and Function. Science 312 (5771), 217–224. doi:10.1126/science.1124618
Haring, M. T., Liv, N., Zonnevylle, A. C., Narvaez, A. C., Voortman, L. M., Kruit, P., et al. (2017). Automated Sub-5 Nm Image Registration in Integrated Correlative Fluorescence and Electron Microscopy Using Cathodoluminescence Pointers. Sci. Rep. 7, 436211–436219. doi:10.1038/srep43621
Hayworth, K. J., Morgan, J. L., Schalek, R., Berger, D. R., Hildebrand, D. G. C., and Lichtman, J. W. (2014). Imaging ATUM Ultrathin Section Libraries with WaferMapper: a Multi-Scale Approach to EM Reconstruction of Neural Circuits. Front. Neural Circuits 8, 68. doi:10.3389/fncir.2014.00068
Heinrich, L., Bennett, D., Ackerman, D., Park, W., Bogovic, J., Eckstein, N., et al. (2021). Whole-cell Organelle Segmentation in Volume Electron Microscopy. Nature 599, 141–146. doi:10.1038/s41586-021-03977-3
Hildebrand, D. G. C., Cicconet, M., Torres, R. M., Choi, W., Quan, T. M., Moon, J., et al. (2017). Whole-brain Serial-Section Electron Microscopy in Larval Zebrafish. Nature 545, 7654345–7654349. doi:10.1038/nature22356
Karreman, M. A., Mercier, L., Schieber, N. L., Shibue, T., Schwab, Y., and Goetz, J. G. (2014). Correlating Intravital Multi-Photon Microscopy to 3D Electron Microscopy of Invading Tumor Cells Using Anatomical Reference Points. PLoS One 9, e114448. doi:10.1371/journal.pone.0114448
Karreman, M. A., Hyenne, V., Schwab, Y., and Goetz, J. G. (2016). Intravital Correlative Microscopy: Imaging Life at the Nanoscale. Trends Cel Biol. 26 (11), 848–863. doi:10.1016/j.tcb.2016.07.003
Khairy, K., Denisov, G., and Saalfeld, S. (2018). Joint Deformable Registration of Large EM Image Volumes: A Matrix Solver Approach. arXiv preprint arXiv:1804.10019. arXiv.
Kornfeld, J., and Denk, W. (2018). Progress and Remaining Challenges in High-Throughput Volume Electron Microscopy. Curr. Opin. Neurobiol. 50, 261–267. doi:10.1016/j.conb.2018.04.030
Kuipers, J., de Boer, P., and Giepmans, B. N. G. (2015). Scanning EM of Non-heavy Metal Stained Biosamples: Large-Field of View, High Contrast and Highly Efficient Immunolabeling. Exp. Cel Res. 337 (2), 202–207. doi:10.1016/j.yexcr.2015.07.012
Kukulski, W., Schorb, M., Welsch, S., Picco, A., Kaksonen, M., and Briggs, J. A. G. (2011). Correlated Fluorescence and 3D Electron Microscopy with High Sensitivity and Spatial Precision. J. Cel Biol. 192 (1), 111–119. doi:10.1083/jcb.201009037
Lane, R., Vos, Y., Wolters, A. H. G., van Kessel, L., Chen, S. E., Liv, N., et al. (2021). Optimization of Negative Stage Bias Potential for Faster Imaging in Large-Scale Electron Microscopy. J. Struct. Biol. X 5, 100046. doi:10.1016/j.yjsbx.2021.100046
Lee, C.-W., Wilfling, F., Ronchi, P., Allegretti, M., Mosalaganti, S., Jentsch, S., et al. (2020). Selective Autophagy Degrades Nuclear Pore Complexes. Nat. Cel Biol. 22 (2), 159–166. doi:10.1038/s41556-019-0459-2
Liou, W., Geuze, H. J., and Slot, J. W. (1996). Improving Structural Integrity of Cryosections for Immunogold Labeling. Histochem. Cel Biol. 106 (1), 41–58. doi:10.1007/bf02473201
Liu, J., Li, L., Yang, Y., Hong, B., Chen, X., Xie, Q., et al. (2020). Automatic Reconstruction of Mitochondria and Endoplasmic Reticulum in Electron Microscopy Volumes by Deep Learning. Front. Neurosci. 14, 599. doi:10.3389/fnins.2020.00599
Liv, N., Zonnevylle, A. C., Narvaez, A. C., Effting, A. P. J., Voorneveld, P. W., Lucas, M. S., et al. (2013). Simultaneous Correlative Scanning Electron and High-NA Fluorescence Microscopy. PloS One 8 (2), e55707. doi:10.1371/journal.pone.0055707
Lowe, D. G. (1999). “Object Recognition from Local Scale-Invariant Features,” in Proceedings of the Seventh IEEE International Conference on Computer Vision, Kerkyra, Greece, 20-27 September, 1999 (IEEE), 1150–1157. doi:10.1109/iccv.1999.790410
Micheva, K. D., and Smith, S. J. (2007). Array Tomography: a New Tool for Imaging the Molecular Architecture and Ultrastructure of Neural Circuits. Neuron 55 (1), 25–36. doi:10.1016/j.neuron.2007.06.014
Ounkomol, C., Seshamani, S., Maleckar, M. M., Collman, F., and Johnson, G. R. (2018). Label-free Prediction of Three-Dimensional Fluorescence Images from Transmitted-Light Microscopy. Nat. Methods 15 (11), 917–920. doi:10.1038/s41592-018-0111-2
Paez-Segala, M. G., Sun, M. G., Shtengel, G., Viswanathan, S., Baird, M. A., Macklin, J. J., et al. (2015). Fixation-resistant Photoactivatable Fluorescent Proteins for CLEM. Nat. Methods 12 (3), 215–218. doi:10.1038/nmeth.3225
Paul-Gilloteaux, P., Heiligenstein, X., Belle, M., Domart, M.-C., Larijani, B., Collinson, L., et al. (2017). eC-CLEM: Flexible Multidimensional Registration Software for Correlative Microscopies. Nat. Methods 14 (2), 102–103. doi:10.1038/nmeth.4170
Peddie, C. J., and Collinson, L. M. (2014). Exploring the Third Dimension: Volume Electron Microscopy Comes of Age. Micron 61, 9–19. doi:10.1016/j.micron.2014.01.009
Peddie, C. J., Blight, K., Wilson, E., Melia, C., Marrison, J., Carzaniga, R., et al. (2014). Correlative and Integrated Light and Electron Microscopy of In-Resin GFP Fluorescence, Used to Localise Diacylglycerol in Mammalian Cells. Ultramicroscopy 143, 3–14. doi:10.1016/j.ultramic.2014.02.001
Polishchuk, R. S., Polishchuk, E. V., Marra, P., Alberti, S., Buccione, R., Luini, A., et al. (2000). Correlative Light-Electron Microscopy Reveals the Tubular-Saccular Ultrastructure of Carriers Operating between Golgi Apparatus and Plasma Membrane. J. Cel Biol. 148, 45–58. doi:10.1083/jcb.148.1.45
Ravelli, R. B., Kalicharan, R. D., Avramut, M. C., Sjollema, K. A., Pronk, J. W., Dijk, F., et al. (2013). Destruction of Tissue, Cells and Organelles in Type 1 Diabetic Rats Presented at Macromolecular Resolution. Sci. Rep. 3 (1), 1804–1806. doi:10.1038/srep01804
Ronchi, P., Mizzon, G., Machado, P., D’Imprima, E., Best, B. T., Cassella, L., et al. (2021). High-Precision Targeting Workflow for Volume Electron Microscopy. J. Cell Biol. 220 (9), e202104069.
Saalfeld, S., Cardona, A., Hartenstein, V., and Tomancak, P. (2009). CATMAID: Collaborative Annotation Toolkit for Massive Amounts of Image Data. Bioinformatics 25 (15), 1984–1986. doi:10.1093/bioinformatics/btp266
Saalfeld, S., Fetter, R., Cardona, A., and Tomancak, P. (2012). Elastic Volume Reconstruction from Series of Ultra-thin Microscopy Sections. Nat. Methods 9 (7), 717–720. doi:10.1038/nmeth.2072
Schindelin, J., Arganda-Carreras, I., Frise, E., Kaynig, V., Longair, M., Pietzsch, T., et al. (2012). Fiji: an Open-Source Platform for Biological-Image Analysis. Nat. Methods 9 (7), 676–682. doi:10.1038/nmeth.2019
Seifert, R., Markert, S. M., Britz, S., Perschin, V., Erbacher, C., Stigloher, C., et al. (2020). DeepCLEM: Automated Registration for Correlative Light and Electron Microscopy Using Deep Learning. F1000Res 9, 1275. doi:10.12688/f1000research.27158.1
Srinivasa Raja, A., Boer, P., Giepmans, B. N. G., and Hoogenboom, J. P. (2021). Electron‐Beam Induced Luminescence and Bleaching in Polymer Resins and Embedded Biomaterial. Macromol. Biosci. 21, 2100192. doi:10.1002/mabi.202100192
Tapia, J. C., Kasthuri, N., Hayworth, K. J., Schalek, R., Lichtman, J. W., Smith, S. J., et al. (2012). High-contrast en bloc staining of neuronal tissue for field emission scanning electron microscopy. Nat. Protoc. 7 (2), 193–206. doi:10.1038/nprot.2011.439
Templier, T. (2019). MagC, Magnetic Collection of Ultrathin Sections for Volumetric Correlative Light and Electron Microscopy. Elife 8, e45696. doi:10.7554/eLife.45696
Tuijtel, M. W., Koster, A. J., Jakobs, S., Faas, F. G. A., and Sharp, T. H. (2019). Correlative Cryo Super-resolution Light and Electron Microscopy on Mammalian Cells Using Fluorescent Proteins. Sci. Rep. 9 (1), 1369–1411. doi:10.1038/s41598-018-37728-8
Van Rijnsoever, C., Oorschot, V., and Klumperman, J. (2008). Correlative Light-Electron Microscopy (CLEM) Combining Live-Cell Imaging and Immunolabeling of Ultrathin Cryosections. Nat. Methods 5, 973–980. doi:10.1038/nmeth.1263
Vos, Y., Lane, R. I., Peddie, C. J., Wolters, A. H. G., and Hoogenboom, J. P. (2021). Retarding Field Integrated Fluorescence and Electron Microscope. Microsc. Microanal. 27 (1), 109–120. doi:10.1017/s1431927620024745
Wanner, A. A., Kirschmann, M. A., and Genoud, C. (2015). Challenges of Microtome-Based Serial Block-Face Scanning Electron Microscopy in Neuroscience. J. Microsc. 259 (2), 137–142. doi:10.1111/jmi.12244
Watanabe, S., Punge, A., Hollopeter, G., Willig, K. I., Hobson, R. J., Davis, M. W., et al. (2011). Protein Localization in Electron Micrographs Using Fluorescence Nanoscopy. Nat. Methods 8 (1), 80–84. doi:10.1038/nmeth.1537
Watson, M. L. (1958). Staining of Tissue Sections for Electron Microscopy with Heavy Metals. J. Cel Biol. 4, 475–478. doi:10.1083/jcb.4.4.475
Keywords: correlative light and electron microscopy, volume electron microscopy, integrated microscopy, array tomography, serial section electron microscopy, scanning electron microscopy
Citation: Lane R, Wolters AHG, Giepmans BNG and Hoogenboom JP (2022) Integrated Array Tomography for 3D Correlative Light and Electron Microscopy. Front. Mol. Biosci. 8:822232. doi: 10.3389/fmolb.2021.822232
Received: 25 November 2021; Accepted: 15 December 2021;
Published: 19 January 2022.
Edited by:
Christopher Guerin, Vlaams Instituut voor Biotechnologie, BelgiumReviewed by:
Kristina D Micheva, Stanford University, United StatesPaolo Ronchi, European Molecular Biology Laboratory Heidelberg, Germany
Copyright © 2022 Lane, Wolters, Giepmans and Hoogenboom. This is an open-access article distributed under the terms of the Creative Commons Attribution License (CC BY). The use, distribution or reproduction in other forums is permitted, provided the original author(s) and the copyright owner(s) are credited and that the original publication in this journal is cited, in accordance with accepted academic practice. No use, distribution or reproduction is permitted which does not comply with these terms.
*Correspondence: Ryan Lane, ci5pLmxhbmVAdHVkZWxmdC5ubA==