- 1Department of Molecular Medicine, Advanced Technologies Faculty, Golestan University of Medical Science, Gorgan, Iran
- 2Department of Animal Physiology and Immunology, TUM School of Life Sciences, Technical University of Munich, Munich, Germany
- 3Cellular and Molecular Research Center, Golestan University of Medical Science, Gorgan, Iran
Introduction: MicroRNAs have a significant role in the regulation of the transcriptome. Several miRNAs have been proposed as potential biomarkers in different malignancies. However, contradictory results have been reported on the capability of miRNA biomarkers in cancer detection. The human biological clock involves molecular mechanisms that regulate several genes over time. Therefore, the sampling time becomes one of the significant factors in gene expression studies.
Method: In the present study, we have tried to find miRNAs with minimum fluctuation in expression levels at different time points that could be more accurate candidates as diagnostic biomarkers. The small RNA-seq raw data of ten healthy individuals across nine-time points were analyzed to identify miRNAs with stable expression.
Results: We have found five oscillation patterns. The stable miRNAs were investigated in 779 small-RNA-seq datasets of eleven cancer types. All miRNAs with the highest differential expression were selected for further analysis. The selected miRNAs were explored for functional pathways. The predominantly enriched pathways were miRNA in cancer and the P53-signaling pathway. Finally, we have found seven miRNAs, including miR-142-3p, miR-199a-5p, miR-223-5p, let-7d-5p, miR-148b-3p, miR-340-5p, and miR-421. These miRNAs showed minimum fluctuation in healthy blood and were dysregulated in the blood of eleven cancer types.
Conclusion: We have found a signature of seven stable miRNAs which dysregulate in several cancer types and may serve as potential pan-cancer biomarkers.
Introduction
The discovery of microRNAs (miRNA) as the new player of the transcriptome has changed the field of molecular biology. miRNAs are single-stranded small non-coding RNAs composed of 18–22 nucleotides (Sarshar et al., 2020). The binding of miRNA to the target genes, especially to the 3′ untranslated region (UTR), induces post-transcriptional gene regulation (Huntzinger and Izaurralde, 2011; Ipsaro and Joshua-Tor, 2015). A single miRNA could potentially target several mRNAs. Therefore, dysregulation of miRNAs profoundly affects the expression of numerous genes that may lead to human diseases such as cancer (Dharap et al., 2013; Peng and Croce, 2016; Zhang et al., 2018). Many studies have confirmed that miRNAs play an essential role in various cancer-associated biological processes such as apoptosis, proliferation, metabolism, invasion, differentiation, immune response, and metastasis (Kabekkodu et al., 2018; Abkhooie et al., 2021; O'Brien et al., 2018; He et al., 2019).
miRNAs have been found in various biological samples, including peripheral blood, which is readily obtainable in significant amounts (El-Mogy et al., 2018; Hermann et al., 2019). Studies have shown that miRNAs are the most significant regulating RNA components existing in peripheral blood and could be applied as biomarkers with high specificity and sensitivity for detecting cancers (Buschmann et al., 2016; Bhome et al., 2017; Carter et al., 2017; Dumache, 2017; Yan et al., 2017). However, the application of miRNAs as diagnostic biomarkers has some significant challenges. Several factors such as data preprocessing and optimization, interpersonal differences, the interaction of miRNAs with serum molecules, and the retention time of samples have been reported as the causes of controversies regarding the use of miRNAs as diagnostic biomarkers (Witwer, 2015; Monzo et al., 2017; Xu et al., 2017; Zhou et al., 2017; Grätz et al., 2022). The circadian clock system is based on a transcription-translation feedback loop (Jennifer et al., 2012). The circadian cycle organizes the regulation of the daily timing of transcriptome (including miRNA) (Du et al., 2014; Zhou et al., 2021). Therefore, in gene expression studies, the sampling time becomes one of the significant factors affecting the gene expression results. In this study, we tried to find miRNAs in the blood with minimum fluctuation at several time points. We proposed that these miRNAs could be a better candidate for cancer detection. We present here a panel of seven miRNAs with the slightest oscillation in healthy peripheral blood, which showed a significant dysregulation in 11 types of cancers.
Materials and methods
Patients and samples
We conducted a detailed search in the Gene Expression Omnibus (GEO) database to find appropriate small RNA sequencing raw data, using the keywords “small-RNA seq”, “whole blood derived”, and “blood-derived cancer” resulting in 779 patient raw data sets from 11 cancer types (Table 1 and Table 2). Our input criteria only include datasets that obtained blood. The RNA seq raw data for healthy individuals (n = 90) were obtained from the study of (Haberberger et al., 2018; Mussack et al., 2021), including whole blood samples of ten healthy male individuals at nine time-points (0 h, 3 h, 6 h, 24 h, 48 h, 72 h, Day7, Day30, and day60). A table of clinical information of 10 healthy individuals was provided in Supplementary Table S1 (Supplementary File S1).
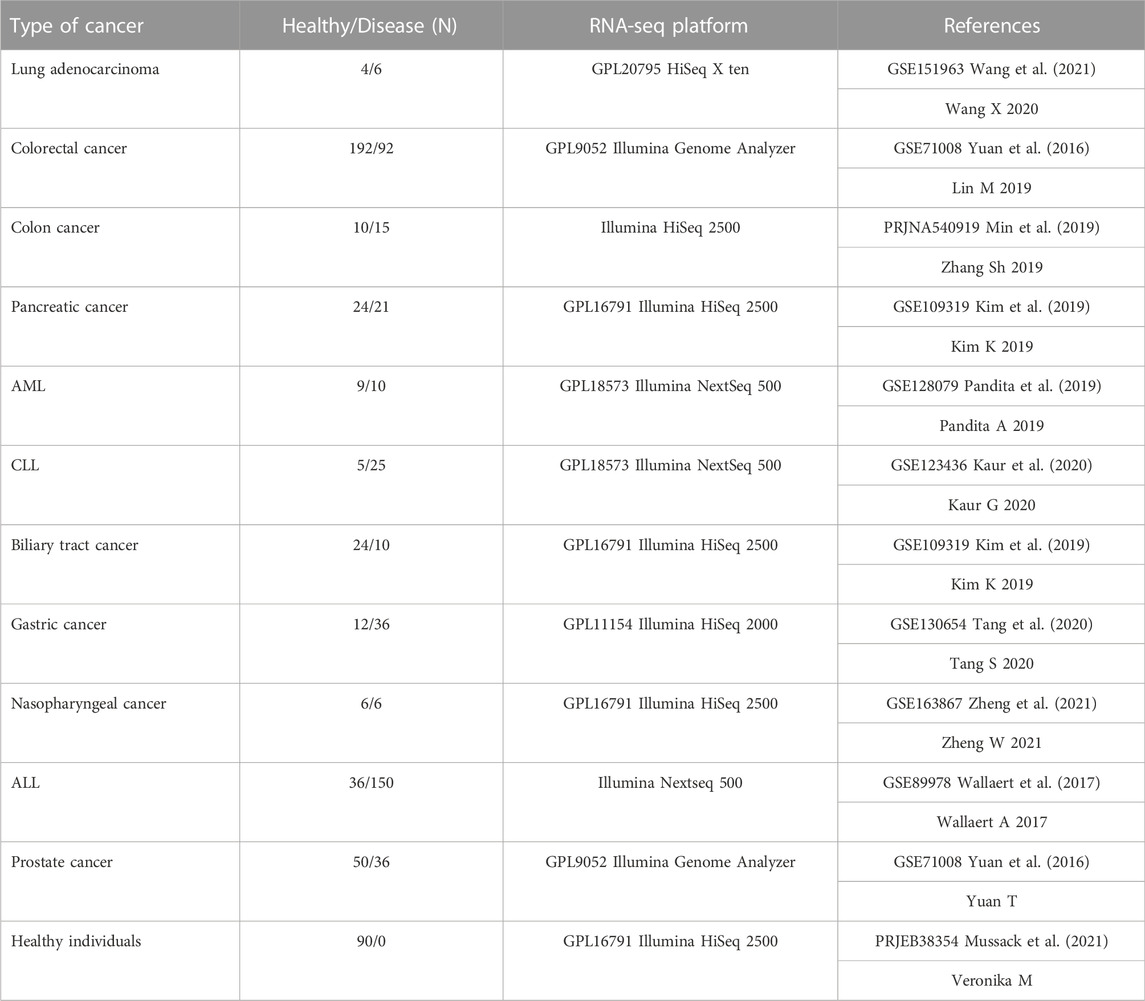
TABLE 1. Cancer small RNA-seq raw datasets. Eleven different cancer datasets with their specific controls in nine different tissues. One time series dataset obtained from ten healthy individuals including 90 samples (Mussack et al., 2021). All the samples were obtained from blood in each cancer. All datasets are available in Gene Expression Omnibus (GEO) database (c). AML: acute myelogenous leukemia, CLL: chronic lymphocytic leukemia, ALL: acute lymphocytic leukemia.
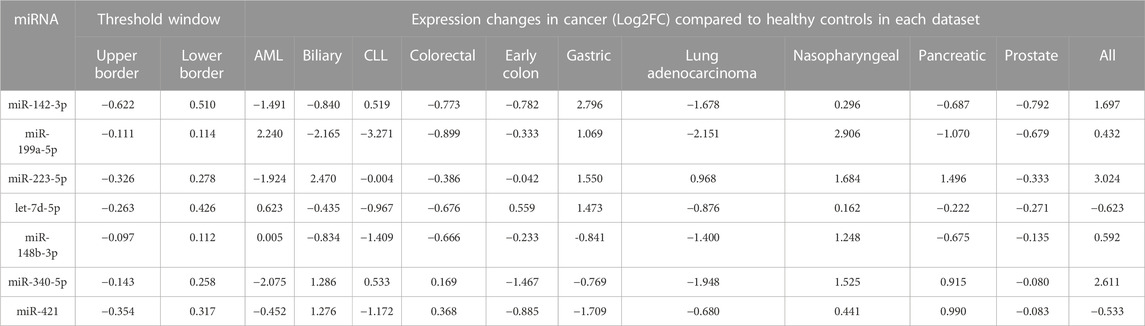
TABLE 2. The foldchange of 37 selected DEMs in 11 cancer datasets compared to control in respective datasets. The miRNAs with expression fold change levels inside the threshold window were discarded. Seven DEMs with the minimum oscillation (MAD) in healthy controls showed the highest dysregulation in 11 cancers cancer datasets. All miRNAs indicated MAD score <0.2. AML: acute myeloid leukemia, CLL: chronic lymphoid leukemia. ALL: acute lymphoid leukemia. MAD: median absolute fold change.
Study design
This study was conducted in two steps (Figure 1). At first, to determine the miRNAs with minimum oscillation in different time points, each time point’s Deseq2-normalized count is compared to the mean of Deseq2-normalized count in all nine time points separately. miRNAs that showed aberrant expression across time points were therefore removed from the analysis. Median absolute deviation (MAD) was applied to measure miRNA variability fold changes during nine time points and define the cutoff for expression change (Rousseeuw and Croux, 1993; Leys et al., 2013). The value of MAD for each miRNA was calculated as follows, where b is constant (b = 1.4826) (Leys et al., 2013) and Xn is the expression value Log2(FC) for a single miRNA of each time point of healthy samples compared to the overall mean expression:
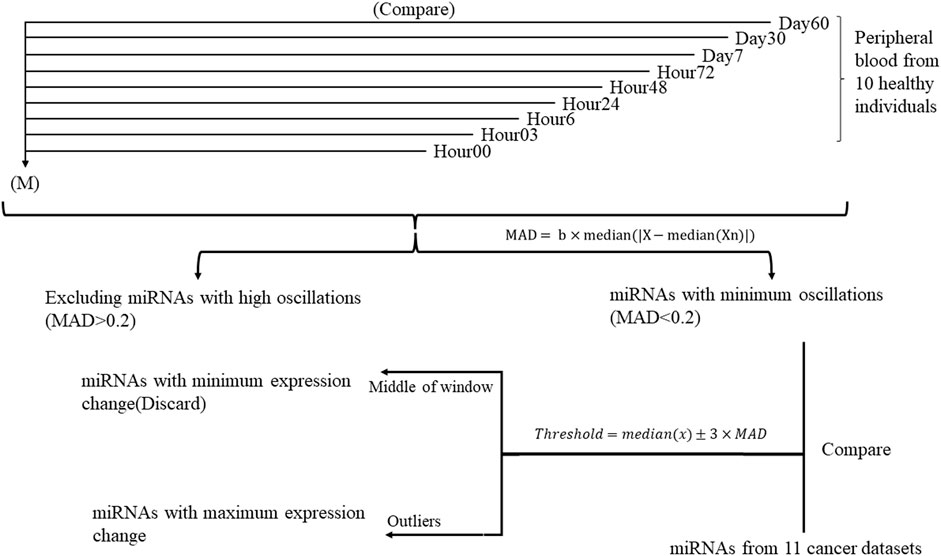
FIGURE 1. Study design flowchart performed on ten healthy individuals throughout nine time points. Each time point is compared to the mean of expression of 90 samples during nine timepoints (M) separately. miRNAs with similar expression in all time points were selected for further analysis.
To specify whether the miRNA was diverted throughout the timepoints, thresholds were defined as where x stands for each miRNA in all time points:
In the second step, miRNAs with MAD <0.2 and fold changes out of the threshold range (outlier) compared to cancer datasets and their specific control samples were selected for further analysis. COSINOR analysis was used to confirm the absence of oscillation in selected miRNAs.
Data processing and differential expression analysis
Small RNA-seq data analysis was performed on the GALAXY platform (Afgan et al., 2016). The quality of the small RNA libraries was assessed using FASTQC software. Sequence quality was checked per base sequence quality, and all reads with a mean quality score under 30 were excluded. Trim Galore was used to discard adapter sequences and sequences less than 16 bases to ignore false positive mapping. Then sequences were aligned to the human reference genome (Hg38). The annotation file was used from miRbase v22.1 (Ambros et al., 2003), which contains all known human miRNAs. Alignment and quantification were performed using Bowtie 1.2.0 and FeatureCount 1.6.4 (Bates et al., 2014; Mascher et al., 2017). Differential expression analysis of miRNAs was performed using DESeq2 version 2.11.40.6 with the Benjamini–Hochberg procedure, which controls the false discovery rate (FDR) (Love et al., 2016). miRNAs with DESeq2-normalized mean read counts under 20 were discarded to avoid false-positive results.
Cluster analysis of gene expression
TimeClust software (Magni et al., 2008) was used to cluster the miRNA’s expression foldchanges during nine time points according to their temporal expression profile. The random walk models for Bayesian clustering were used as the clustering algorithm.
Principal component analysis (PCA) was performed using GraphPad Prism version 9.0.0 (GraphPad Software, San Diego, California United States, www.graphpad.com) to specify clustering in healthy versus cancer samples.
ROC curve analysis
The Deseq2-normalized read counts of all overlapping differentially expressed miRNAs(DEMs) were used to assess the sensitivity and specificity of each DEM to evaluate its detection capability in cancer datasets versus the control group using receiver-operating characteristic (ROC) curves and the area under curve (AUC).
The sensitivity and specificity of all miRNAs as a prognostic signature for prediction were evaluated by AUC of the receiver operation characteristic with R program software using the Combiroc package (Bombaci and Rossi, 2019).
Functional enrichment analysis
MIENTURNET (Licursi et al., 2019) was used to find the interaction network of miRNAs with their target genes using Targetscan (McGeary et al., 2019) and miRTarbase (Huang et al., 2022) database and exploring the Kyoto encyclopedia of genes and genomes (KEGG) database (Kanehisa and Goto, 2000). Also, we used ShinyGo as a powerful functional enrichment analyzer tool to specify the KEGG pathway and Gene ontology of targeted genes (Ge et al., 2020).
miRNA-protein interaction network
The MiRNET platform was used to explore the miRNA target genes and the miRNA-gene interaction network (Chang et al., 2020). Moreover, we explored other miRNA target prediction analyzing tools, including miRDIP(Tokar et al., 2018), miRDB (Chen and Wang, 2020), TargetScan, and miRTarBase by a Venn diagram to find shared miRNAs. Each miRNA and target gene with interaction number <3 (Degree filter) were discarded to reduce the false positive targets.
Validation
The expression of candidate miRNAs was explored in three different studies. Array express and TCGA datasets was assessed and samples obtained from whole blood of patients with different types of cancer with no treatment was selected. Three different study were explored and analyzed. miRNA expression level from whole blood of patients detected by lung cancer and nasopharyngeal cancer including 1052 healthy individuals and 1438 cancer patients were assessed (Patnaik et al., 2012; Wen, 2018; Fehlmann et al., 2020). The mean expression levels of the seven candidate miRNAs in the blood of cancer patients was compared to the mean expression levels in blood of healthy individuals. The expression intensity of candidate miRNAs in the blood of cancer patients demonstrated a significant difference with expression levels in healthy blood (Figure 8) (Supplementary File S1). All datasets were analyzed by one-way ANOVA followed by Dunnett’s multiple comparisons tests using GraphPad Prism version 9.0.0 for Windows, GraphPad Software, San Diego, California United States, www.graphpad.com.
Results
miRNAs have different oscillation patterns
The common miRNAs through nine time points were analyzed to find the miRNAs with minimum oscillation patterns. One hundred ninety-five miRNAs were found that were expressed in all time points (Supplementary File S1). Five different oscillation patterns were detected (Figure 2). Cluster E comprised most miRNAs (61), and Cluster A the least (17). Cluster C (red line) comprised 37 miRNAs with the lowest MAD score (MAD <0.2) among other clusters and showed the most consistent behavior. Hence cluster C represented a group of miRNAs with minimum fluctuation in expression levels across all time points (Supplementary File S1).
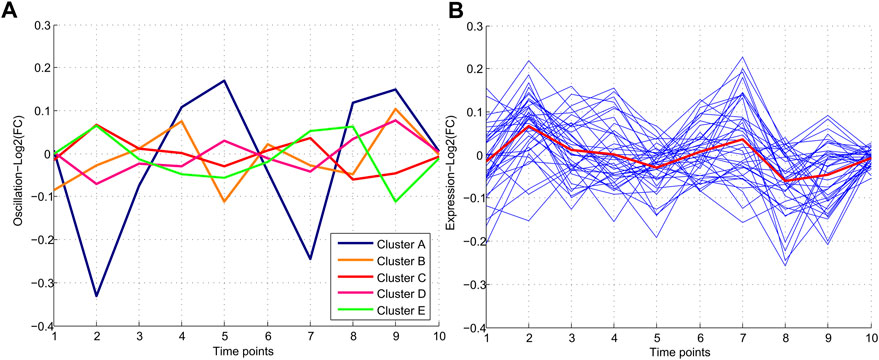
FIGURE 2. Patterns of miRNA expression during nine different time points. (A) Five clusters were detected in clustering analysis. Pattern C which includes 37 miRNAs, demonstrates the minimum and Pattern A demonstrated the maximum oscillation among the other patterns. (B) Pattern C consisting of 37 miRNAs with a similar oscillation pattern which demonstrated the lowest oscillations.
miRNAs with minimum oscillation patterns show dysregulation in cancers
To evaluate the potential dysregulation regarding miRNAs in cluster C (Figure 2A), we explored the total number of differentially expressed miRNAs (DEM) in the blood of 11 cancers in comparison to their study-specific controls (Figure 3A). The range of DEMs varied from 401 in ALL (Acute lymphocyte leukemia) to 74 in CLL (Chronic lymphocyte leukemia). The fold changes of 37 candidate miRNAs (cluster C) in all cancers indicated that 17 miRNAs with positive and 20 miRNAs with a negative average of fold changes throughout all 11 cancer datasets. The miR-223-5p demonstrated the highest (1.5), and miR-19b-3p showed the lowest (0.01) average of fold changes compared to study-specific controls (Figure 3B).
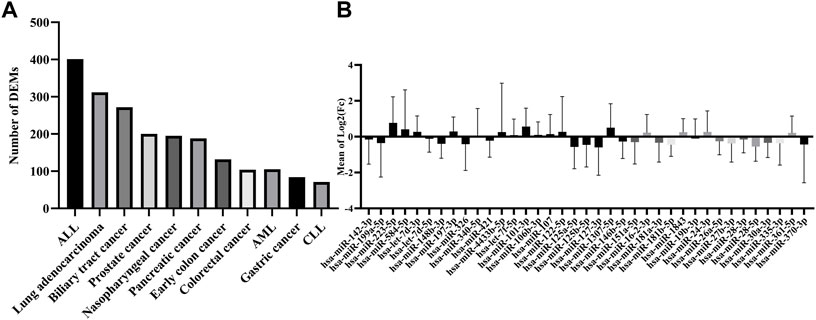
FIGURE 3. Expression and number of miRNAs in all cancer datasets. (A) The absolute number of miRNAs were differentially expressed in each cancer dataset. (B) The range of expression levels of each miRNA in all cancer datasets.
Finally, the presence (miRNA with Adj p-value <0.05) and dysregulation level of the 37 candidate miRNAs were checked in all types of cancer by ranking miRNAs according to their distance from the threshold window. The top seven DEMs were selected based on their distance from the threshold (outliers) [2], including miR-142-3p, miR-199a-5p, and miR-223-5p showed the most significant distance to the cutoff border. Seven miRNAs with significantly distinct fold change in comparison to cancer datasets and study-specific controls and also distance from the threshold window in more than 80% of cancers were selected as top dysregulated miRNAs.
In order to find the miRNAs responsible for variance in expression of the cancer datasets from healthy and cancer samples, we performed principal component analysis (PCA) with the first principal component (PC1) and second principal component (PC2) for seven miRNAs. The PC1 showed 54%, and PC2 showed a 17% (Figure 4A) variance between all samples. A loading plot was created utilizing the PC axis to distinguish between the miRNAs responsible for these clusters. On the PC2, let-7d-5p, miR-148b-3p, miR-223-5p, and miR-340-5p all exhibited a negative value. miR-148b-3p and miR-421 were small contributors to the PC1. The PC2 contained the highest contribution levels for miR-148b-3p and miR-421 (Figure 4B). The panel of miRNAs demonstrated the best discrimination power in lung, Biliary tract and nasopharyngeal cancer.
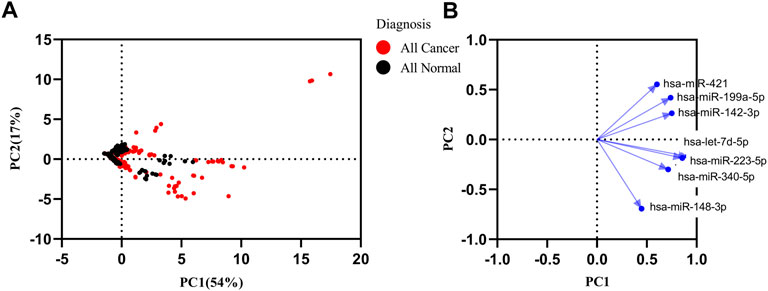
FIGURE 4. Principal component analysis (PCA) of healthy and all types of cancer. (A) PCA matrix was used to discover cancer and control group. 71% variance was discovered in PC1 and PC2. (B) Loading plot of seven miRNAs.
Moreover, we conducted a literature review to compare the reported miRNAs in other cancer studies with the present candidate miRNAs result (Supplementary File S2). The most important miRNAs reported numerously as biomarkers in the blood of cancers were miR-142-3p and miR-223-5p. These miRNAs and other reported miRNAs demonstrated significant dysregulation in different cancer types.
Diagnostic values of DEMs
In order to evaluate the diagnostic values of DEMs in discriminating cancer from healthy controls, ROC curve analysis was performed on Deseq2-normalized read counts of seven miRNAs with minimum oscillation. The seven miRNAs displayed promising results in discriminating the two groups with a specificity and sensitivity greater than 80% and a p-value <0.05 (Supplementary File S1). The best model performance was observed in AML, pancreatic, and colon cancers (AUC> 90%). Gastric cancer and lung adenocarcinoma showed lower performance (AUC>80%) than other cancers. Seven miRNAs, including miR-142-3p, miR-199a-5p, miR-223-5p, let-7d-5p, miR-148b-3p, miR-340-5p, and miR-421 demonstrated the significant signatures as a panel for distinguishing cancer from healthy samples (Figure 5).
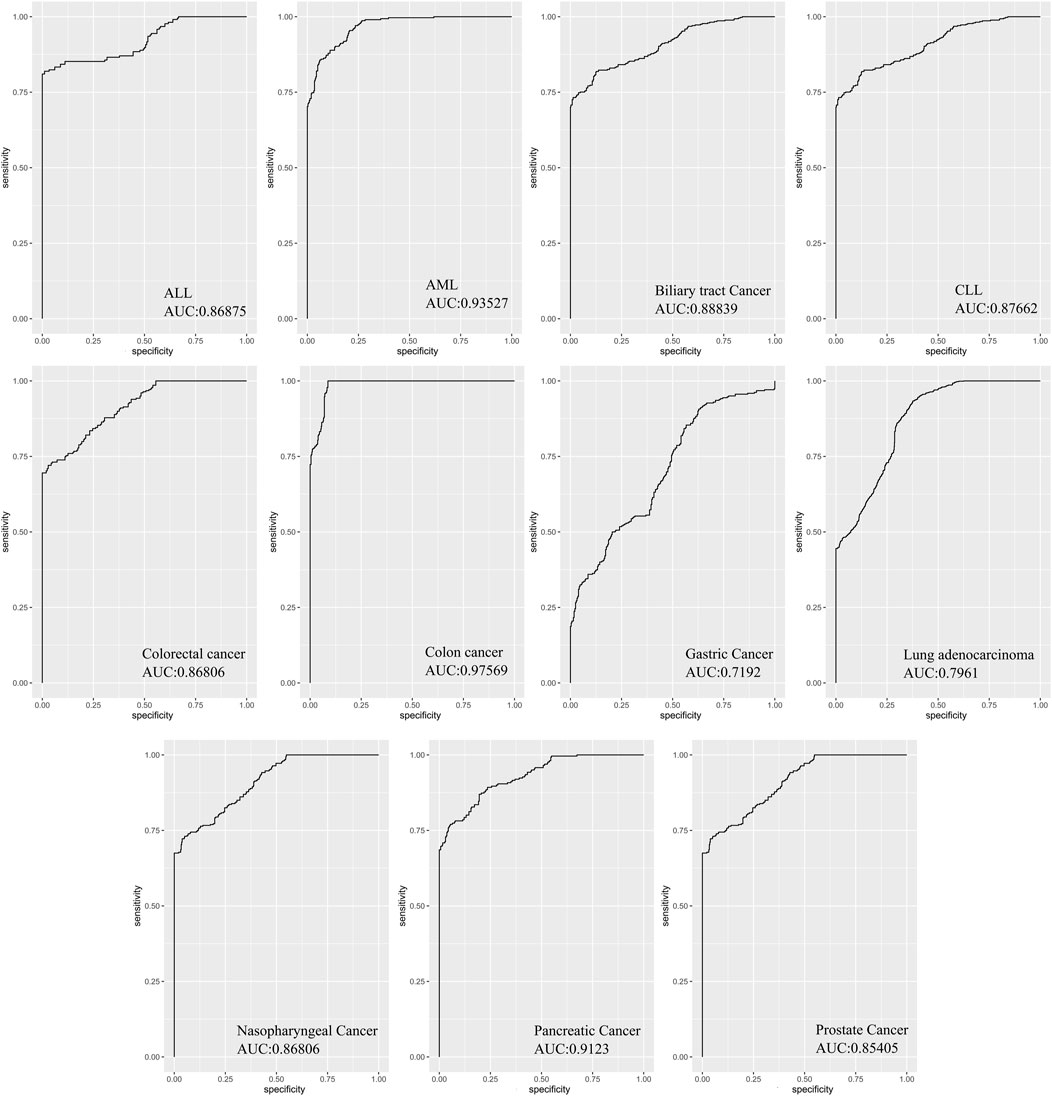
FIGURE 5. The area under curves analysis for seven DEMs as a signature in eleven cancers. AUC analysis of all seven miRNAs as a signature panel checked with sensitivity and specificity >80% and p-value <0.05. The best model performance was demonstrated in Colon cancer.
miRNA-protein interaction network and functional analysis
The miRNA interaction network of seven candidate miRNAs had 2212 edges (interactions) and 1971 nodes (target genes) (Figure 6A). Several miRNA target predictions analyzing were used to find the best target genes for each miRNA. A module with 26 nodes and 79 edges was extracted with the most significant interaction scores (degree score ≥3), including seven miRNAs and 26 target genes (Figure 6B). The list of best fitted genes is available in Supplementary File S1 and Supplementary Table S5. The miR-340-5p and miR-142-3p demonstrated the best interaction by having the most connectivity with target genes (Figure 6C). The KEGG pathway enrichment analysis of candidate miRNAs showed that microRNAs in cancer and P53 signaling pathway are the most important pathways (Figure 6D).
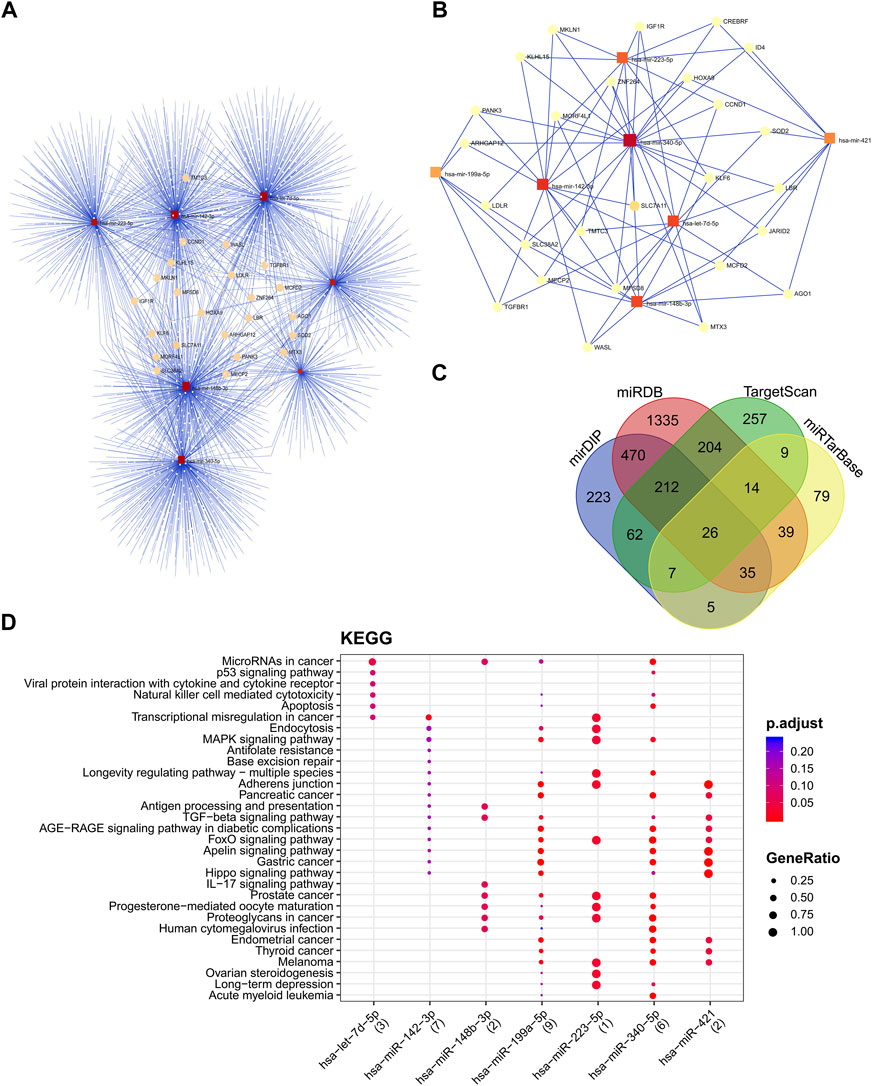
FIGURE 6. The miRNA-protein interaction network and cluster analysis of target genes and the interactions between selected miRNAs and target genes. (A) miRNA-mRNA interaction network of all seven candidate miRNAs. (B)The best miRNA candidate association with target genes squares represented miRNAs, and circles represented target genes. (C) miRNA target prediction tools were explored and found 26 best-fitted target genes common in all platforms. (D) KEGG enrichment analysis of DEMs. KEGG pathway analysis showed candidate miRNAs significantly implicated with the cancer-related disease.
The KEGG pathway enrichment and gene ontology analysis on 26 best-fitted target genes were performed using the ShinyGO tool. GO analysis in biological process term (BP) demonstrated that the target genes were associated with nucleobase-containing compound metabolite process and regulation of gene expression (Figure 7A). In addition, the endomembrane system was the cellular components (CC) most involved in related targeted genes (Figure 7B). In molecular function (MF) categories, target genes are associated with sequence-specific DNA binding and transcription regulatory activity. miRNA prediction tools were explored to find the overlap (Figure 7C). On the other hand, we checked the KEGG enrichment results based on their weight scores to cluster results based on the minimum subset of target genes that cover all the genes from enrichment sets. FOXO signaling pathway, signaling pathways regulating pluripotency of stem cells, and longevity regulation pathway were demonstrated with a greater fold enrichment ratio and significant FDR <0.05 (Figure 7D).
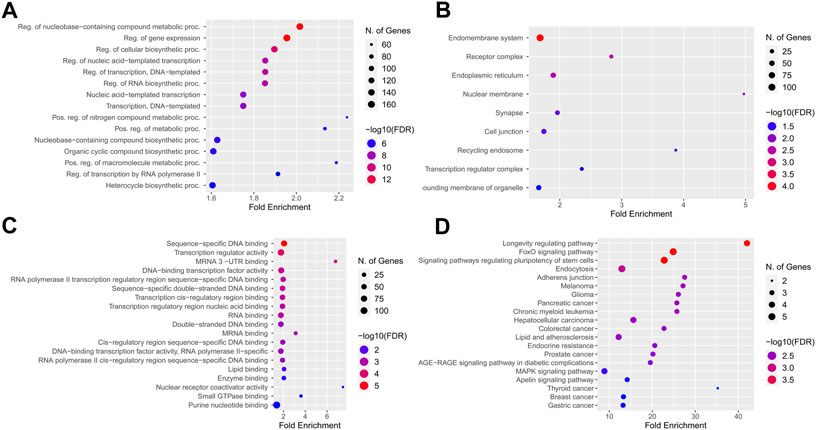
FIGURE 7. KEGG pathway enrichment and Gene ontology analysis best-fitted target genes. (A) Biological process term (BP) association with nucleobase containing compound metabolite process and regulation of gene expression. (B) Endomembrane system of cells were the cellular components (CC) most involved regarding targeted genes. (C) In molecular function (MF) categories, target genes are associated with sequence-specific DNA binding, and transcription regulatory activity. (D) Longevity regulation pathway, FOXO signaling pathway and signaling pathways regulation pluripotency of stem cells had best enrichment ratio and the more significant number of identified targets in KEGG pathway analysis.
Validation
The expression of candidate miRNAs was explored in total seven studies Including three studies from different cancer types obtained from whole blood. The expression intensity of candidate miRNAs in the whole blood (Figure 8) of cancer patients demonstrated a significant difference in compare the healthy controls. Statistical analysis provided as supplementary file (Supplementary File S1 and Supplementary Table S6). All clinical data including sex and age of control and patient samples were provided in supplementary file (Supplementary File S1 and Supplementary Table S7).
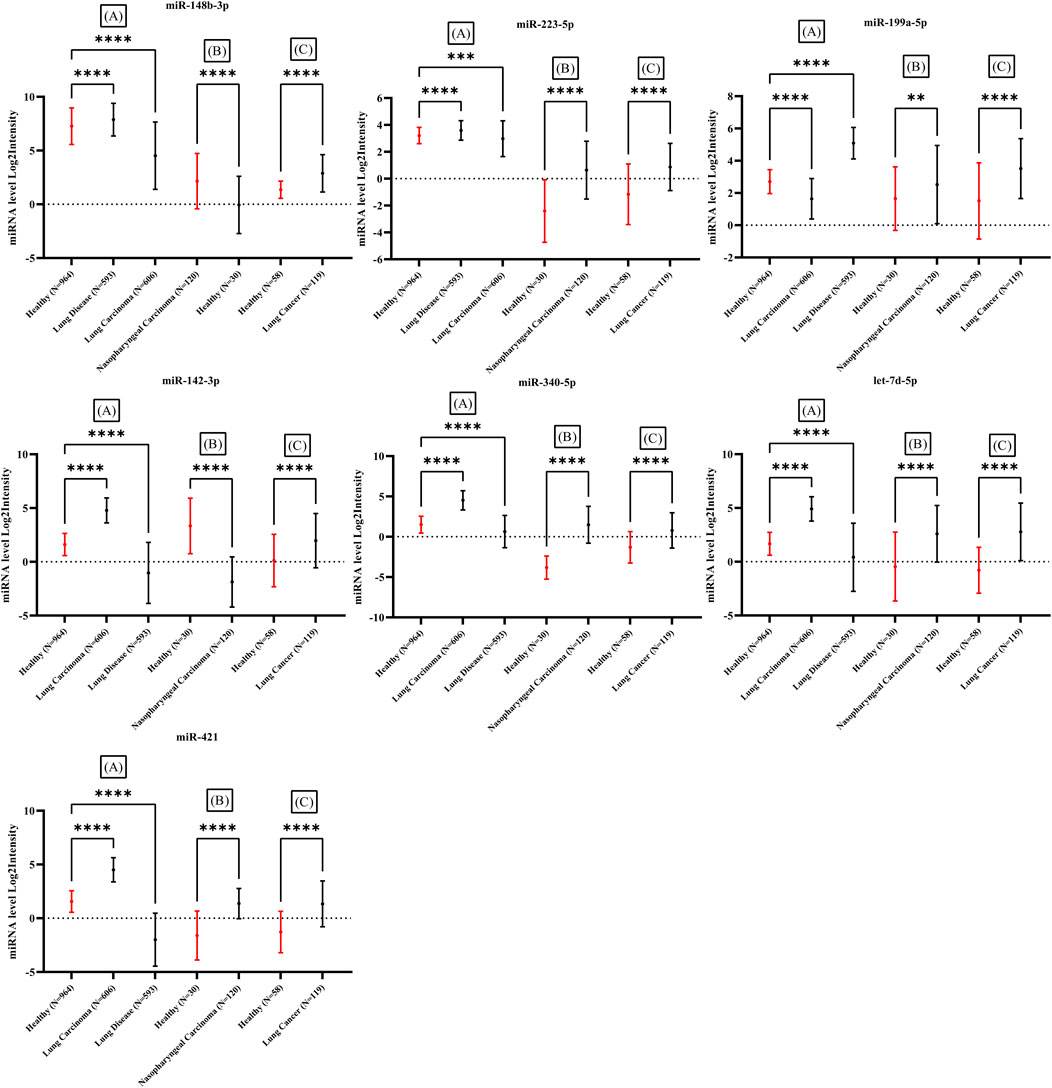
FIGURE 8. Expression difference in seven miRNAs in comparison between healthy individuals and several types of cancer in three studies. All samples obtained from whole blood of cancer patients. Significant difference demonstrated with p-value <0.05 in all three studies. Three studies including (A): (Fehlmann et al., 2020), (B): (Wen, 2018) and (C): (Patnaik et al., 2012).
Discussion
The molecular mechanisms that control a range of genes across time are part of the human biological clock, which influences many molecular processes (Takahashi et al., 2008). The regulation of the daily timing of the transcriptome, including miRNA, is organized by the circadian cycle (Du et al., 2014; Zhou et al., 2021). As a result, one of the key variables influencing the results of gene expression investigations is the sampling time. In the present study, we have tried to find miRNAs in the peripheral blood with minimum fluctuation at different time points. Then, the candidate miRNAs expression was assessed in eleven cancers.
The blood miRNAs in healthy controls showed different oscillation patterns. We have found a cluster of 37 miRNAs with the most stable behavior across all time points. It can be assumed that a change in the expression of these miRNAs may indicate an abnormal or pathological event (Farrell et al., 2015; Bertoli et al., 2016). Therefore, we explored the expression level of these stable miRNAs in the peripheral blood of different types of cancer. We have found that seven miRNAs, including miR-142-3p, miR-199a-5p, miR-223-5p, let-7d-5p, miR-148b-3p, miR-340-5p, and miR-421 were significantly deregulated in the blood of cancer patients. The sensitivity and specificity of these miRNAs were higher than 80%. Several studies have been reported the deregulation of miR-142-3p (Lv et al., 2012; Hu et al., 2013; Wang et al., 2018; Gao et al., 2019; Liu et al., 2021), miR-199a-5p (Zhou et al., 2015; Zhang et al., 2021), miR-223-5p (Gilicze et al., 2014; Zhu et al., 2019; Deng et al., 2021), let-7d-5p (De Santis and Götte, 2021; Li et al., 2021), miR-148b-3p (Mollazadeh et al., 2019; Yuan et al., 2019; Shan et al., 2021), miR-340-5p (Guo et al., 2021; Huang et al., 2021; Tan et al., 2021), and miR-421 (Chen et al., 2019; Mo et al., 2020) in the blood of different cancer types. The KEGG pathway analysis showed that the microRNAs in cancer and P53 signaling pathway were the most enriched pathways. These pathways are important routes of cancer development and progression.
The critical question is how changes in the expression of miRNAs in the blood may be associated with cancer in other tissues. A standard answer to this question is that the leakage of miRNAs from cancerous tissues into the blood changes the level of these molecules in the blood of cancer patients (Fichtlscherer et al., 2011; Keller et al., 2011; Creemers et al., 2012). However, blood cells are the primary producer of circulating miRNA (Pritchard et al., 2012; Sohel, 2016). Moreover, comparisons of blood miRNAs with cancerous tissues have shown that miRNAs in blood are not just a byproduct of cancerous tissue but are a part of the body’s defense against cancer (Coombs et al., 2017).
In a contradictory hypothesis (a version of clonal hematopoiesis) (Jamieson, 2017), it can be assumed that deregulation of circulating miRNAs due to somatic mutation in hematopoietic stem cells may lead to an increased risk of cancer in other tissues. It has been shown that miRNAs can be transferred to cancer cells and regulate cellular processes and signaling pathways (Turchinovich et al., 2011; Ramachandran and Palanisamy, 2012; Turchinovich and Burwinkel, 2012; Turchinovich et al., 2013; Chevillet et al., 2014; Turchinovich et al., 2015; Anfossi et al., 2018; Sun et al., 2018; Reshke et al., 2020). We have found seven miRNAs that are highly stable under normal conditions, which may dysregulate in mutated hematopoietic cells with age (Coombs et al., 2017; Jamieson, 2017; Fabre et al., 2022; Jeon et al., 2022). In addition, this panel of seven miRNA may have use in surgical treatment response. There are numerous studies reporting the use of circulating miRNAs in surgical treatment response. Recent studies have shown that the expression of some circulating miRNAs in patients diagnosed with cancer was back to the level of expression in healthy individuals after surgical treatment (Heneghan et al., 2010; van Schooneveld et al., 2012; Chen et al., 2016). Although the present study results are promising for cancer detection, there is a limitation due to using samples in different stages. Therefore, it is not clear whether these miRNAs could detect cancer in its early stages. Furthermore, due to the lack of time series datasets in healthy individual’s females, the results may include sex bias expression. Also, Deeper verification is required for our findings due to poor overlap among the other reports.
In summary, we have found a pan-cancer signature of seven miRNAs that may have the potential for cancer detection. Also, this approach could be used as a survey for identifying biomarkers for other pathological conditions. However, further investigation is needed to validate our results and examine the miRNA’s pan-cancer role.
Data availability statement
The original contributions presented in the study are included in the article/Supplementary Material, further inquiries can be directed to the corresponding author.
Author contributions
AS: conceived the study and carried out the experiment; MK, MB, and VM: collected the data and edited the manuscript; BK: verified the analytical methods; MP: contributed to the interpretation of the results; MG: designed and supervised the study. All authors read and approved the final manuscript.
Funding
This work was funded by a grant from Golestan University of Medical Sciences (Grant number 111373).
Acknowledgments
The authors acknowledge the support of the Freiburg Galaxy Team: Björn Grüning, Bioinformatics, University of Freiburg (Germany) funded by the Collaborative Research Centre 992 Medical Epigenetics (DFG grant SFB 992/1 2012) and the German Federal Ministry of Education and Research BMBF grant 031 A538A de.NBI-RBC. The results here are in whole or part based upon data generated by the TCGA Research Network: https://www.cancer.gov/tcga.
Conflict of interest
The authors declare that the research was conducted in the absence of any commercial or financial relationships that could be construed as a potential conflict of interest.
Publisher’s note
All claims expressed in this article are solely those of the authors and do not necessarily represent those of their affiliated organizations, or those of the publisher, the editors and the reviewers. Any product that may be evaluated in this article, or claim that may be made by its manufacturer, is not guaranteed or endorsed by the publisher.
Supplementary material
The Supplementary Material for this article can be found online at: https://www.frontiersin.org/articles/10.3389/fmolb.2022.1030749/full#supplementary-material
References
Abkhooie, L., Sarabi, M. M., Kahroba, H., Eyvazi, S., Montazersaheb, S., Tarhriz, V., et al. (2021). Potential roles of MyomiRs in cardiac development and related diseases. Curr. Cardiol. Rev. 17, e010621188335–e010621188379. doi:10.2174/1573403X16999201124201021
Afgan, E., Baker, D., Van Den Beek, M., Blankenberg, D., Bouvier, D., Čech, M., et al. (2016). The Galaxy platform for accessible, reproducible and collaborative biomedical analyses: 2016 update. Nucleic Acids Res. 44, W3–W10. doi:10.1093/nar/gkw343
Ambros, V., Bartel, B., Bartel, D. P., Burge, C. B., Carrington, J. C., Chen, X., et al. (2003). A uniform system for microRNA annotation. Rna 9, 277–279. doi:10.1261/rna.2183803
Anfossi, S., Babayan, A., Pantel, K., and Calin, G. A. (2018). Clinical utility of circulating non-coding RNAs—An update. Nat. Rev. Clin. Oncol. 15, 541–563. doi:10.1038/s41571-018-0035-x
Bates, D., Mächler, M., Bolker, B., and Walker, S. (2014). Fitting linear mixed-effects models using lme4. arXiv Prepr. arXiv 1406.5823.
Bertoli, G., Cava, C., and Castiglioni, I. (2016). MicroRNAs as biomarkers for diagnosis, prognosis and theranostics in prostate cancer. Int. J. Mol. Sci. 17, 421. doi:10.3390/ijms17030421
Bhome, R., Goh, R. W., Bullock, M. D., Pillar, N., Thirdborough, S. M., Mellone, M., et al. (2017). Exosomal microRNAs derived from colorectal cancer-associated fibroblasts: Role in driving cancer progression. Aging (Albany NY) 9, 2666–2694. doi:10.18632/aging.101355
Bombaci, M., and Rossi, R. L. (2019). Computation and selection of optimal biomarker combinations by integrative ROC analysis using CombiROC Proteomics for Biomarker Discovery. Springer.
Buschmann, D., Haberberger, A., Kirchner, B., Spornraft, M., Riedmaier, I., Schelling, G., et al. (2016). Toward reliable biomarker signatures in the age of liquid biopsies-how to standardize the small RNA-Seq workflow. Nucleic Acids Res. 44, 5995–6018. doi:10.1093/nar/gkw545
Carter, J. V., Galbraith, N. J., Yang, D., Burton, J. F., Walker, S. P., and Galandiuk, S. (2017). Blood-based microRNAs as biomarkers for the diagnosis of colorectal cancer: A systematic review and meta-analysis. Br. J. Cancer 116, 762–774. doi:10.1038/bjc.2017.12
Chang, L., Zhou, G., Soufan, O., and Xia, J. (2020). miRNet 2.0: network-based visual analytics for miRNA functional analysis and systems biology. Nucleic Acids Res. 48, W244–W251. doi:10.1093/nar/gkaa467
Chen, J., Wu, L., Sun, Y., Yin, Q., Chen, X., Liang, S., et al. (2019). Mir-421 in plasma as a potential diagnostic biomarker for precancerous gastric lesions and early gastric cancer. PeerJ 7, e7002. doi:10.7717/peerj.7002
Chen, X., Liu, X., Liu, H., Lu, Y., and Li, Y. (2016). Reduced expression of serum miR-204 predicts poor prognosis of gastric cancer. Genet. Mol. Res. 15, 15027702. doi:10.4238/gmr.15027702
Chen, Y., and Wang, X. (2020). miRDB: an online database for prediction of functional microRNA targets. Nucleic Acids Res. 48, D127–D131. doi:10.1093/nar/gkz757
Chevillet, J. R., Kang, Q., Ruf, I. K., Briggs, H. A., Vojtech, L. N., Hughes, S. M., et al. (2014). Quantitative and stoichiometric analysis of the microRNA content of exosomes. Proc. Natl. Acad. Sci. U. S. A. 111, 14888–14893. doi:10.1073/pnas.1408301111
Coombs, C. C., Zehir, A., Devlin, S. M., Kishtagari, A., Syed, A., Jonsson, P., et al. (2017). Therapy-related clonal hematopoiesis in patients with non-hematologic cancers is common and associated with adverse clinical outcomes. Cell stem Cell 21, 374–382.e4. e4. doi:10.1016/j.stem.2017.07.010
Creemers, E. E., Tijsen, A. J., and Pinto, Y. M. (2012). Circulating microRNAs: Novel biomarkers and extracellular communicators in cardiovascular disease? Circ. Res. 110, 483–495. doi:10.1161/CIRCRESAHA.111.247452
de Santis, C., and Götte, M. (2021). The role of microRNA let-7d in female malignancies and diseases of the female reproductive tract. Int. J. Mol. Sci. 22, 7359. doi:10.3390/ijms22147359
Deng, Y., Tong, J., Shi, W., Tian, Z., Yu, B., and Tang, J. (2021). Thromboangiitis obliterans plasma-derived exosomal miR-223-5p inhibits cell viability and promotes cell apoptosis of human vascular smooth muscle cells by targeting VCAM1. Ann. Med. 53, 1129–1141. doi:10.1080/07853890.2021.1949487
Dharap, A., Pokrzywa, C., Murali, S., Pandi, G., and Vemuganti, R. (2013). MicroRNA miR-324-3p induces promoter-mediated expression of RelA gene. PLoS one 8, e79467. doi:10.1371/journal.pone.0079467
Du, N.-H., Arpat, A. B., De Matos, M., and Gatfield, D. (2014). MicroRNAs shape circadian hepatic gene expression on a transcriptome-wide scale. Elife 3, e02510. doi:10.7554/eLife.02510
Dumache, R. (2017). Early diagnosis of oral squamous cell carcinoma by salivary microRNAs. Clin. Lab. 63, 1771–1776. doi:10.7754/Clin.Lab.2017.170607
El-Mogy, M., Lam, B., Haj-Ahmad, T. A., Mcgowan, S., Yu, D., Nosal, L., et al. (2018). Diversity and signature of small RNA in different bodily fluids using next generation sequencing. BMC genomics 19, 408–424. doi:10.1186/s12864-018-4785-8
Fabre, M. A., De Almeida, J. G., Fiorillo, E., Mitchell, E., Damaskou, A., Rak, J., et al. (2022). The longitudinal dynamics and natural history of clonal haematopoiesis. Nature 606, 335–342. doi:10.1038/s41586-022-04785-z
Farrell, D., Shaughnessy, R. G., Britton, L., Machugh, D. E., Markey, B., and Gordon, S. V. (2015). The identification of circulating MiRNA in bovine serum and their potential as novel biomarkers of early Mycobacterium avium subsp paratuberculosis infection. PloS one 10, e0134310. doi:10.1371/journal.pone.0134310
Fehlmann, T., Kahraman, M., Ludwig, N., Backes, C., Galata, V., Keller, V., et al. (2020). Evaluating the use of circulating microRNA profiles for lung cancer detection in symptomatic patients. JAMA Oncol. 6, 714–723. doi:10.1001/jamaoncol.2020.0001
Fichtlscherer, S., Zeiher, A. M., and Dimmeler, S. (2011). Circulating microRNAs: Biomarkers or mediators of cardiovascular diseases? Arterioscler. Thromb. Vasc. Biol. 31, 2383–2390. doi:10.1161/ATVBAHA.111.226696
Gao, W., Pang, D., and Yu, S. (2019). Serum level of miR-142-3p predicts prognostic outcome for colorectal cancer following curative resection. J. Int. Med. Res. 47, 2116–2125. doi:10.1177/0300060519834815
Ge, S. X., Jung, D., and Yao, R. (2020). ShinyGO: A graphical gene-set enrichment tool for animals and plants. Bioinformatics 36, 2628–2629. doi:10.1093/bioinformatics/btz931
Gilicze, A. B., Wiener, Z., Tóth, S., Buzás, E., Pállinger, É., Falcone, F. H., et al. (2014). Myeloid-derived microRNAs, miR-223, miR27a, and miR-652, are dominant players in myeloid regulation. Biomed. Res. Int. 2014, 870267. doi:10.1155/2014/870267
Grätz, C., Bui, M. L., Thaqi, G., Kirchner, B., Loewe, R. P., and Pfaffl, M. W. (2022). Obtaining reliable RT-qPCR results in molecular diagnostics—MIQE goals and pitfalls for transcriptional biomarker discovery. Life 12, 386. doi:10.3390/life12030386
Guo, S., Qin, H., Liu, K., Wang, H., Bai, S., Liu, S., et al. (2021). Blood small extracellular vesicles derived miRNAs to differentiate pancreatic ductal adenocarcinoma from chronic pancreatitis. Clin. Transl. Med. 11, e520. doi:10.1002/ctm2.520
Haberberger, A., Kirchner, B., Riedmaier, I., Henschler, R., Wichmann, C., Buhmann, R., et al. (2018). Changes in the microRNA expression profile during blood storage. BMJ Open Sport Exerc. Med. 4, e000354. doi:10.1136/bmjsem-2018-000354
He, W., Xu, J., Huang, Z., Zhang, J., and Dong, L. (2019). MiRNAs in cancer therapy: Focusing on their bi-directional roles. ExRNA 1, 7–6. doi:10.1186/s41544-019-0005-1
Heneghan, H. M., Miller, N., Lowery, A. J., Sweeney, K. J., Newell, J., and Kerin, M. J. (2010). Circulating microRNAs as novel minimally invasive biomarkers for breast cancer. Ann. Surg. 251, 499–505. doi:10.1097/SLA.0b013e3181cc939f
Hermann, S., Buschmann, D., Kirchner, B., Borrmann, M., Brandes, F., Kotschote, S., et al. (2019). Transcriptomic profiling of cell-free and vesicular microRNAs from matched arterial and venous sera. J. Extracell. Vesicles 8, 1670935. doi:10.1080/20013078.2019.1670935
Hu, W., Ye, Y., Zhang, W., Wang, J., Chen, A., and Guo, F. (2013). miR-142-3p promotes osteoblast differentiation by modulating Wnt signaling. Mol. Med. Rep. 7, 689–693. doi:10.3892/mmr.2012.1207
Huang, H.-Y., Lin, Y.-C.-D., Cui, S., Huang, Y., Tang, Y., Xu, J., et al. (2022). miRTarBase update 2022: an informative resource for experimentally validated miRNA–target interactions. Nucleic Acids Res. 50, D222–D230. doi:10.1093/nar/gkab1079
Huang, Z., Xu, Y., Wan, M., Zeng, X., and Wu, J. (2021). miR-340: A multifunctional role in human malignant diseases. Int. J. Biol. Sci. 17, 236–246. doi:10.7150/ijbs.51123
Huntzinger, E., and Izaurralde, E. (2011). Gene silencing by microRNAs: Contributions of translational repression and mRNA decay. Nat. Rev. Genet. 12, 99–110. doi:10.1038/nrg2936
Ipsaro, J. J., and Joshua-Tor, L. (2015). From guide to target: Molecular insights into eukaryotic RNA-interference machinery. Nat. Struct. Mol. Biol. 22, 20–28. doi:10.1038/nsmb.2931
Jamieson, C. (2017). Cancer: Bad blood promotes tumour progression. Nature 549, 465–466. doi:10.1038/549465a
Jennifer, A., Carla, B., and Joseph, S. (2012). Central and peripheral circadian clocks in mammal. Annu. Rev. Neurosci. 35, 445–462.
Jeon, O. H., Mehdipour, M., Gil, T.-H., Kang, M., Aguirre, N. W., Robinson, Z. R., et al. (2022). Systemic induction of senescence in young mice after single heterochronic blood exchange. Nat. Metab. 4, 995–1006. doi:10.1038/s42255-022-00609-6
Kabekkodu, S. P., Shukla, V., Varghese, V. K., D'Souza, J., Chakrabarty, S., and Satyamoorthy, K. (2018). Clustered miRNAs and their role in biological functions and diseases. Biol. Rev. Camb. Philos. Soc. 93, 1955–1986. doi:10.1111/brv.12428
Kanehisa, M., and Goto, S. (2000). Kegg: Kyoto encyclopedia of genes and genomes. Nucleic Acids Res. 28, 27–30. doi:10.1093/nar/28.1.27
Kaur, G., Ruhela, V., Rani, L., Gupta, A., Sriram, K., Gogia, A., et al. (2020). RNA-Seq profiling of deregulated miRs in CLL and their impact on clinical outcome. Blood Cancer J. 10, 6–9. doi:10.1038/s41408-019-0272-y
Keller, A., Leidinger, P., Bauer, A., Elsharawy, A., Haas, J., Backes, C., et al. (2011). Toward the blood-borne miRNome of human diseases. Nat. Methods 8, 841–843. doi:10.1038/nmeth.1682
Kim, K., Yoo, D., Lee, H. S., Lee, K. J., Park, S. B., Kim, C., et al. (2019). Identification of potential biomarkers for diagnosis of pancreatic and biliary tract cancers by sequencing of serum microRNAs. BMC Med. Genomics 12, 62–11. doi:10.1186/s12920-019-0521-8
Leys, C., Ley, C., Klein, O., Bernard, P., and Licata, L. (2013). Detecting outliers: Do not use standard deviation around the mean, use absolute deviation around the median. J. Exp. Soc. Psychol. 49, 764–766. doi:10.1016/j.jesp.2013.03.013
Li, X., Chen, Y., Wang, J., Jiang, C., and Huang, Y. (2021). Lung cancer cell-derived exosomal let-7d-5p down-regulates OPRM1 to promote cancer-induced bone pain. Front. Cell Dev. Biol. 9, 666857. doi:10.3389/fcell.2021.666857
Licursi, V., Conte, F., Fiscon, G., and Paci, P. (2019). Mienturnet: An interactive web tool for microRNA-target enrichment and network-based analysis. BMC Bioinforma. 20, 545–554. doi:10.1186/s12859-019-3105-x
Liu, P., Cao, F., Sui, J., Hong, Y., Liu, Q., Gao, X., et al. (2021). MicroRNA-142-3p inhibits tumorigenesis of colorectal cancer via suppressing the activation of wnt signaling by directly targeting to β-Catenin. Front. Oncol. 3339, 552944. doi:10.3389/fonc.2020.552944
Lv, M., Zhang, X., Jia, H., Li, D., Zhang, B., Zhang, H., et al. (2012). An oncogenic role of miR-142-3p in human T-cell acute lymphoblastic leukemia (T-ALL) by targeting glucocorticoid receptor-α and cAMP/PKA pathways. Leukemia 26, 769–777. doi:10.1038/leu.2011.273
Magni, P., Ferrazzi, F., Sacchi, L., and Bellazzi, R. (2008). TimeClust: A clustering tool for gene expression time series. Bioinformatics 24, 430–432. doi:10.1093/bioinformatics/btm605
Mascher, M., Gundlach, H., Himmelbach, A., Beier, S., Twardziok, S. O., Wicker, T., et al. (2017). A chromosome conformation capture ordered sequence of the barley genome. Nature 544, 427–433. doi:10.1038/nature22043
Mcgeary, S. E., Lin, K. S., Shi, C. Y., Pham, T. M., Bisaria, N., Kelley, G. M., et al. (2019). The biochemical basis of microRNA targeting efficacy. Science 366, eaav1741. doi:10.1126/science.aav1741
Min, L., Zhu, S., Chen, L., Liu, X., Wei, R., Zhao, L., et al. (2019). Evaluation of circulating small extracellular vesicles derived miRNAs as biomarkers of early colon cancer: A comparison with plasma total miRNAs. J. Extracell. Vesicles 8, 1643670. doi:10.1080/20013078.2019.1643670
Mo, X.-M., Qin, P.-F., Wang, B., Liu, F.-H., and Li, H.-H. (2020). miR-421 promotes the viability of A549 lung cancer cells by targeting forkhead box O1. Oncol. Lett. 20, 306–312. doi:10.3892/ol.2020.12169
Mollazadeh, S., Fazly Bazzaz, B. S., Neshati, V., De Vries, A. A., Naderi-Meshkin, H., Mojarad, M., et al. (2019). Overexpression of MicroRNA-148b-3p stimulates osteogenesis of human bone marrow-derived mesenchymal stem cells: The role of MicroRNA-148b-3p in osteogenesis. BMC Med. Genet. 20, 117–126. doi:10.1186/s12881-019-0854-3
Monzo, M., Santasusagna, S., Moreno, I., Martinez, F., Hernáez, R., Muñoz, C., et al. (2017). Exosomal microRNAs isolated from plasma of mesenteric veins linked to liver metastases in resected patients with colon cancer. Oncotarget 8, 30859–30869. doi:10.18632/oncotarget.16103
Mussack, V., Wittmann, G., and Pfaffl, M. W. (2021). On the trail of blood doping—microRNA fingerprints to monitor autologous blood transfusions in vivo. Am. J. Hematol. 96, 338–353. doi:10.1002/ajh.26078
O'brien, J., Hayder, H., Zayed, Y., and Peng, C. (2018). Overview of microRNA biogenesis, mechanisms of actions, and circulation. Front. Endocrinol. 9, 402. doi:10.3389/fendo.2018.00402
Pandita, A., Ramadas, P., Poudel, A., Saad, N., Anand, A., Basnet, A., et al. (2019). Differential expression of miRNAs in acute myeloid leukemia quantified by Nextgen sequencing of whole blood samples. Plos one 14, e0213078. doi:10.1371/journal.pone.0213078
Patnaik, S. K., Yendamuri, S., Kannisto, E., Kucharczuk, J. C., Singhal, S., and Vachani, A. (2012). MicroRNA expression profiles of whole blood in lung adenocarcinoma.
Peng, Y., and Croce, C. M. (2016). The role of MicroRNAs in human cancer. Signal Transduct. Target. Ther. 1, 15004–15009. doi:10.1038/sigtrans.2015.4
Pritchard, C. C., Kroh, E., Wood, B., Arroyo, J. D., Dougherty, K. J., Miyaji, M. M., et al. (2012). Blood cell origin of circulating microRNAs: A cautionary note for cancer biomarker studies. Cancer Prev. Res. 5, 492–497. doi:10.1158/1940-6207.CAPR-11-0370
Ramachandran, S., and Palanisamy, V. (2012). Horizontal transfer of RNAs: Exosomes as mediators of intercellular communication. Wiley Interdiscip. Rev. RNA 3, 286–293. doi:10.1002/wrna.115
Reshke, R., Taylor, J. A., Savard, A., Guo, H., Rhym, L. H., Kowalski, P. S., et al. (2020). Reduction of the therapeutic dose of silencing RNA by packaging it in extracellular vesicles via a pre-microRNA backbone. Nat. Biomed. Eng. 4, 52–68. doi:10.1038/s41551-019-0502-4
Rousseeuw, P. J., and Croux, C. (1993). Alternatives to the median absolute deviation. J. Am. Stat. Assoc. 88, 1273–1283. doi:10.1080/01621459.1993.10476408
Sarshar, M., Scribano, D., Ambrosi, C., Palamara, A. T., and Masotti, A. (2020). Fecal microRNAs as innovative biomarkers of intestinal diseases and effective players in host-microbiome interactions. Cancers 12, 2174. doi:10.3390/cancers12082174
Shan, G., Zhou, X., Gu, J., Zhou, D., Cheng, W., Wu, H., et al. (2021). Downregulated exosomal microRNA-148b-3p in cancer associated fibroblasts enhance chemosensitivity of bladder cancer cells by downregulating the Wnt/β-catenin pathway and upregulating PTEN. Cell. Oncol. 44, 45–59. doi:10.1007/s13402-020-00500-0
Sohel, M. H. (2016). Extracellular/circulating microRNAs: Release mechanisms, functions and challenges. Achiev. Life Sci. 10, 175–186. doi:10.1016/j.als.2016.11.007
Sun, Z., Shi, K., Yang, S., Liu, J., Zhou, Q., Wang, G., et al. (2018). Effect of exosomal miRNA on cancer biology and clinical applications. Mol. Cancer 17, 147–165. doi:10.1186/s12943-018-0897-7
Takahashi, J. S., Hong, H.-K., Ko, C. H., and Mcdearmon, E. L. (2008). The genetics of mammalian circadian order and disorder: Implications for physiology and disease. Nat. Rev. Genet. 9, 764–775. doi:10.1038/nrg2430
Tan, Y., Zhu, L., Cui, L., and Guan, Y. (2021). Differential expression of miRNA in the peripheral blood mononuclear cells in myasthenia gravis with muscle-specific receptor tyrosine kinase antibodies. Crit. Rev. Eukaryot. Gene Expr. 31, 1–15. doi:10.1615/CritRevEukaryotGeneExpr.2021037369
Tang, S., Cheng, J., Yao, Y., Lou, C., Wang, L., Huang, X., et al. (2020). Combination of four serum Exosomal MiRNAs as novel diagnostic biomarkers for early-stage gastric Cancer. Front. Genet. 11, 237. doi:10.3389/fgene.2020.00237
Tokar, T., Pastrello, C., Rossos, A. E., Abovsky, M., Hauschild, A.-C., Tsay, M., et al. (2018). mirDIP 4.1—integrative database of human microRNA target predictions. Nucleic Acids Res. 46, D360–D370. doi:10.1093/nar/gkx1144
Turchinovich, A., and Burwinkel, B. (2012). Distinct AGO1 and AGO2 associated miRNA profiles in human cells and blood plasma. RNA Biol. 9, 1066–1075. doi:10.4161/rna.21083
Turchinovich, A., Samatov, T. R., Tonevitsky, A. G., and Burwinkel, B. (2013). Circulating miRNAs: Cell–cell communication function? Front. Genet. 4, 119. doi:10.3389/fgene.2013.00119
Turchinovich, A., Tonevitsky, A. G., Cho, W. C., and Burwinkel, B. (2015). Check and mate to exosomal extracellular miRNA: New lesson from a new approach. Front. Mol. Biosci. 2, 11. doi:10.3389/fmolb.2015.00011
Turchinovich, A., Weiz, L., Langheinz, A., and Burwinkel, B. (2011). Characterization of extracellular circulating microRNA. Nucleic Acids Res. 39, 7223–7233. doi:10.1093/nar/gkr254
Van Schooneveld, E., Wouters, M. C., Auwera, IVD., Peeters, D. J., Wildiers, H., Van Dam, P. A., et al. (2012). Expression profiling of cancerous and normal breast tissues identifies microRNAs that are differentially expressed in serum from patients with (metastatic) breast cancer and healthy volunteers. Breast Cancer Res. 14, R34–R16. doi:10.1186/bcr3127
Wallaert, A., Van Loocke, W., Hernandez, L., Taghon, T., Speleman, F., and Van Vlierberghe, P. (2017). Comprehensive miRNA expression profiling in human T-cell acute lymphoblastic leukemia by small RNA-sequencing. Sci. Rep. 7, 7901–7908. doi:10.1038/s41598-017-08148-x
Wang, X.-J., Gao, J., Wang, Z., and Yu, Q. (2021). Identification of a potentially functional microRNA–mRNA regulatory network in lung adenocarcinoma using a Bioinformatics analysis. Front. Cell Dev. Biol. 9, 641840. doi:10.3389/fcell.2021.641840
Wang, Y., Cao, Z., Wang, L., Liu, S., and Cai, J. (2018). Downregulation of microRNA-142-3p and its tumor suppressor role in gastric cancer. Oncol. Lett. 15, 8172–8180. doi:10.3892/ol.2018.8330
Wen, W. (2018). Two miRNA signatures for identifying nasopharyngeal carcinoma from head-neck tumors and the normal. Ann. Oncol. 29, ix99–ix100. doi:10.1093/annonc/mdy438.021
Witwer, K. W. (2015). Circulating microRNA biomarker studies: Pitfalls and potential solutions. Clin. Chem. 61, 56–63. doi:10.1373/clinchem.2014.221341
Xu, W., Zhou, Y., Xu, G., Geng, B., and Cui, Q. (2017). Transcriptome analysis reveals non-identical microRNA profiles between arterial and venous plasma. Oncotarget 8, 28471–28480. doi:10.18632/oncotarget.15310
Yan, S., Han, B., Gao, S., Wang, X., Wang, Z., Wang, F., et al. (2017). Exosome-encapsulated microRNAs as circulating biomarkers for colorectal cancer. Oncotarget 8, 60149–60158. doi:10.18632/oncotarget.18557
Yuan, L., Liu, Y., Qu, Y., Liu, L., and Li, H. (2019). Exosomes derived from MicroRNA-148b-3p-overexpressing human umbilical cord mesenchymal stem cells restrain breast cancer progression. Front. Oncol. 9, 1076. doi:10.3389/fonc.2019.01076
Yuan, T., Huang, X., Woodcock, M., Du, M., Dittmar, R., Wang, Y., et al. (2016). Plasma extracellular RNA profiles in healthy and cancer patients. Sci. Rep. 6, 19413–19423. doi:10.1038/srep19413
Zhang, J., Zhou, W., Liu, Y., Liu, T., Li, C., and Wang, L. (2018). Oncogenic role of microRNA-532-5p in human colorectal cancer via targeting of the 5'UTR of RUNX3. Oncol. Lett. 15, 7215–7220. doi:10.3892/ol.2018.8217
Zhang, L., Cao, H., Gu, G., Hou, D., You, Y., Li, X., et al. (2021). Exosomal miR-199a-5p inhibits tumorigenesis and angiogenesis by targeting VEGFA in osteosarcoma.
Zheng, W., Ye, W., Wu, Z., Huang, X., Xu, Y., Chen, Q., et al. (2021). Identification of potential plasma biomarkers in early-stage nasopharyngeal carcinoma-derived exosomes based on RNA sequencing. Cancer Cell Int. 21, 185–197. doi:10.1186/s12935-021-01881-4
Zhou, G., Lu, M., Chen, J., Li, C., Zhang, J., Chen, J., et al. (2015). Identification of miR-199a-5p in serum as noninvasive biomarkers for detecting and monitoring osteosarcoma. Tumour Biol. 36, 8845–8852. doi:10.1007/s13277-015-3421-3
Zhou, L., Miller, C., Miraglia, L. J., Romero, A., Mure, L. S., Panda, S., et al. (2021). A genome-wide microRNA screen identifies the microRNA-183/96/182 cluster as a modulator of circadian rhythms. Proc. Natl. Acad. Sci. U. S. A. 118. doi:10.1073/pnas.2020454118
Zhou, X., Wen, W., Shan, X., Zhu, W., Xu, J., Guo, R., et al. (2017). A six-microRNA panel in plasma was identified as a potential biomarker for lung adenocarcinoma diagnosis. Oncotarget 8, 6513–6525. doi:10.18632/oncotarget.14311
Keywords: miRNA, biomarker, cancer, small RNA-seq, stable gene expression
Citation: Sabbaghian A, Mussack V, Kirchner B, Bui MLU, Kalani MR, Pfaffl MW and Golalipour M (2022) A panel of blood-derived miRNAs with a stable expression pattern as a potential pan-cancer detection signature. Front. Mol. Biosci. 9:1030749. doi: 10.3389/fmolb.2022.1030749
Received: 29 August 2022; Accepted: 30 November 2022;
Published: 15 December 2022.
Edited by:
Andrey Turchinovich, German Cancer Research Center (DKFZ), GermanyReviewed by:
Wubin Ding, Children’s Hospital of Philadelphia, United StatesCristiana Tanase, Victor Babes National Institute of Pathology (INCDVB), Romania
Copyright © 2022 Sabbaghian, Mussack, Kirchner, Bui, Kalani, Pfaffl and Golalipour. This is an open-access article distributed under the terms of the Creative Commons Attribution License (CC BY). The use, distribution or reproduction in other forums is permitted, provided the original author(s) and the copyright owner(s) are credited and that the original publication in this journal is cited, in accordance with accepted academic practice. No use, distribution or reproduction is permitted which does not comply with these terms.
*Correspondence: Masoud Golalipour, Z2FwbWFzb29kQGdvdW1zLmFjLmly
†ORCID: Veronika Mussack, orcid.org/0000-0002-4539-9313; Benedikt Kirchner, orcid.org/0000-0003-3878-0148; Maria L. U. Bui, orcid.org/0000-0002-4448-6642; Michael W. Pfaffl, orcid.org/0000-0002-3192-1019; Masoud Golalipour, orcid.org/0000-0002-3429-5519