- Yusuf Hamied Department of Chemistry, University of Cambridge, Cambridge, United Kingdom
The energy landscape perspective is outlined with particular reference to biomolecules that perform multiple functions. We associate these multifunctional molecules with multifunnel energy landscapes, illustrated by some selected examples, where understanding the organisation of the landscape has provided new insight into function. Conformational selection and induced fit may provide alternative routes to realisation of multifunctionality, exploiting the possibility of environmental control and distinct binding modes.
1 Introduction
Since the first work on protein folding over 50 years ago (Levinthal, 1966; Levinthal, 1968; Anfinsen, 1972; Anfinsen, 1973), the advance of new experimental and computational techniques has led to a greatly improved understanding of proteins and nucleic acids. In addition to insights into the structural properties of biomolecules in their functional forms, this work has revealed alternative functional roles. Not only has this research provided a better understanding of the folding process (Karplus, 2011; Wolynes et al., 2012; Wolynes, 2015), but the importance of folding and misfolding in disease has been analysed (Chiti and Dobson, 2017). This improved understanding has enabled the design of new functional biomolecules [for example (Dou et al. 2018), facilitated by the emergence of design principles based on the fundamental principles governing protein folding (Huang et al., 2016; Baker, 2019). While this progress has occurred over a relatively long time scale, the immediate impact was put into focus during the last 2 years, when these techniques were applied to the SARS-CoV2 virus and its molecular constituents to understand structure and function [for example (Bai and Warshel, 2020; Sikora et al., 2021)], to identify potential drugs [for example Shoemark et al. (2021)], and in combination with bioinformatics tools (Waman et al., 2021).
Given the large number of experimental and computational methods commonly used to investigate biomolecules (Röder et al., 2019), it is not surprising that the corresponding results can sometimes seem contradictory and difficult to reconcile. These issues can arise for a variety of reasons. For example, differences in environmental conditions, as well as non-equilibrium effects due to the long timescales associated with biomolecular motions impact both experiment and simulation (Wales and Salamon, 2014). Not only do these effects combine to provide challenges in understanding molecular biological assemblies and their functionality, but they also complicate the design and development of de novo biomolecules. Consequently, a viewpoint that allows us to unify and interpret a range of experimental and computational findings is highly desirable.
One key organisational principle emerged from efforts to resolve Levinthal’s paradox, namely the existence of an underlying, funnelled energy landscape (Leopold et al., 1992; Onuchic and Wolynes, 2004; Bryngelson et al., 1995). Starting from the potential energy function, this landscape can be analysed from first principles and contains all the information necessary to describe kinetic, thermodynamic and structural properties of a given molecular system (Wales, 2003). Any method, whether experiment or simulation, samples this energy landscape, but the sampling is often implicit.
The focus of this Perspective involves direct exploration of the potential energy landscape. Here we exploit the natural coarse-grained representation in terms of local minima and the transition states that connect them, which are characterised using geometry optimisation. This framework provides a general description applicable to any molecular system (Wales, 2003). State-of-the-art algorithms allow us to explore such landscapes in the context of a specific set of environmental conditions for a given protein or nucleic acid sequence, and for their complexes. The theoretical insight obtained from simulations can be directly translated into experimental observables; mechanistic explanations for these properties can readily be obtained. This approach elucidates the underlying structural causes for the behaviour of molecular systems, and emergent observable properties, providing both a conceptual and a computational framework (Joseph et al., 2017; Röder et al., 2019). The methodology is briefly summarised in §2, followed by an overview of some recent applications. In particular, we highlight recent analysis of multifunnel biomolecular energy landscapes including intrinsically disordered proteins.
2 The Potential Energy Landscape Framework
Our exploration of potential energy landscapes is based on geometry optimisation techniques, and we extract observable thermodynamic and kinetic properties using standard tools of statistical mechanics and unimolecular rate theory (Forst, 1973; Laidler, 1987). There are three distinct but complementary aspects to the calculations. Basin-hopping global optimisation is employed to locate the global minimum and other low-lying structures on the landscape (Li and Scheraga, 1987; Wales and Doye, 1997; Wales and Scheraga, 1999). Here, steps are taken between local minima based on the potential or free energy (Sutherland-Cash et al., 2015) difference and a parameter with dimensions of energy, which determines the probability of accepting an uphill move. Many variants of basin-hopping exist, featuring alternative move sets, accept/reject criteria (Leary and Doye, 1999; Shang and Wales, 2014), and ensembles (Calvo et al., 2016). The common feature that accelerates the exploration is the local minimisation that focuses sampling on a discrete set of structures.
Exploration of the low-lying minima via basin-hopping can be coupled with a method such as parallel tempering (Swendsen and Wang, 1986; Geyer, 1991; Hukushima and Nemoto, 1996) that can sample high energy parts of landscape, where the barriers are generally small. This combination provides methods capable of overcoming broken ergodicity problems, such as basin-sampling (Wales, 2013).
2.1 Characterising Kinetic Properties Based on Energy Landscape Explorations
Characterising molecular rearrangements and the associated kinetics requires computation of transition states, here defined geometrically as stationary points with a single imaginary normal mode frequency (Murrell and Laidler, 1968). The corresponding algorithms for computing transition states and pathways are relatively mature, and details are available elsewhere (Wales, 2003; Joseph et al., 2017; Wales, 2018; Röder et al., 2019). We refer to pathways defined by local minima and intervening transition states as discrete paths. Having obtained an initial discrete path between target product and reactant states we can expand the resulting database of minima and transition states in various ways to converge the rates of interest (Joseph et al., 2017; Wales, 2018; Röder et al., 2019). The database constitutes a kinetic transition network (Rao and Caflisch, 2004; Noé and Fischer, 2008; Prada-Gracia et al., 2009; Wales, 2010), and we refer to the harvesting of discrete paths as discrete path sampling (DPS) (Wales, 2002; Wales, 2004) by analogy to transition path sampling methods based on explicit dynamics (Bolhuis et al., 2002; Dellago and Bolhuis, 2009). The discrete and dynamical approaches are complementary; the geometry optimisation techniques on which DPS is based are largely agnostic to barrier heights and enable rare events to be analysed on experimental time scales, subject to well-defined approximations.
Post-processing analysis of kinetic transition networks provides rates via mean first passage times, along with a variety of pathway characteristics. The landscapes of interest generally feature highly metastable states corresponding to relatively slow rare events. Extracting observable dynamical properties requires methods based on graph transformation (Trygubenko and Wales, 2006; Wales, 2009; Stevenson and Wales, 2014; MacKay and Robinson, 2018; Swinburne and Wales, 2020), which overcome the numerical problems associated with linear algebra approaches. The first passage time (FPT) distribution can also be analysed, using methods such as kinetic path sampling Athènes and Bulatov, 2014; Athènes et al., 2019), which also exploits graph transformation. This distribution contains far more information than the mean value (Swinburne et al., 2020). For example, we have applied kinetic path sampling to analyse the FPT for tryptophan zipper peptide tryptophan zipper1 (TZ1) (Cochran et al., 2001), revealing a bimodal distribution, which can be directly interpreted in terms of the underlying energy landscape (Sharpe and Wales, 2020). Additional properties such as committor probabilities, reactive visitation probabilities, and productive paths can also be obtained (Sharpe and Wales, 2021a; Sharpe and Wales, 2021b), providing a clear resolution of dynamical bottlenecks.
3 The Importance of the Energy Landscape Topography
A key advantage of exploring the energy landscape explicitly is that the organisation is obtained directly. Energy landscapes for proteins and nucleic acids that have evolved to perform a single function are expected to be funnelled (Bryngelson and Wolynes, 1987, Bryngelson and Wolynes, 1989; Leopold et al., 1992; Onuchic et al., 1997; Morcos et al., 2014), allowing fast and reliable folding, and overcoming Levinthal’s paradox (Levinthal (1969)). According to the principle of minimal frustration, the native state is characterised by formation of all native contacts required by the sequence, leading to a single funnel in the potential energy landscape (Bryngelson and Wolynes, 1987; Leopold et al., 1992). However, competing structures might be stabilised in multiple funnels, leading to multifunnel energy landscapes, which are observed for many biomolecules [for example Cragnolini et al., 2017; Röder and Wales, 2018a; Joseph et al., 2019)].
3.1 Structural Heterogeneity and Multiple Functions
It is probably not surprising that multifunnelled energy landscapes are commonly observed, since many biomolecules adopt different configurations for alternative functions. Distinct structures require stabilisation to guarantee a sufficient population, since unpopulated structures cannot function. Because such stabilisation needs to be competitive to facilitate control, it leads to separation into distinct ensembles and funnels. Thus, the energy landscape will be multifunnelled. This association between multiple funnels and multiple functions extends the principle of minimal frustration. We expect the energy landscape to support a number of funnels associated with the distinct functions (Röder and Wales, 2018c).
3.1.1 Nucleic Acid Landscapes
The energy landscape picture described for proteins is also applicable to nucleic acid folding (Thirumalai and Woodson, 1996; Thirumalai, 1998; Chen and Dill, 2000). However, the structural plasticity is even more pronounced in nucleic acids (Tinoco and Bustamante, 1999; Li et al., 2008; Solomatin et al., 2010), and represents a key challenge in the description of RNA structures (Schroeder, 2018). Nonetheless, the explicit exploration of energy landscapes for nucleic acids is possible, and provides important insight into the structural variation compatible with a given sequence (Cragnolini et al., 2017; Xiao et al., 2019; Röder et al., 2020).
3.1.2 Defining Structural Ensembles in Multifunnel Energy Landscapes
While the organisation is revealed by energy landscape explorations, the question remains how to study and analyse the associated, distinct structural ensembles. While energy landscapes are sometimes represented using low-dimensional projections, our approach preserves all degrees of freedom, thus avoiding possible projection errors. The best way to visualise the landscape organisation is using disconnectivity graphs (Becker and Karplus, 1998; Wales et al., 1998), which allow us to identify the funnels on the energy landscape directly. This intrinsic separation of the structures into funnels can also serve as the definition of structural ensembles, where each ensemble is a subgraph of the tree graph representation of the energy landscape. This approach allows us to identify conserved structural motifs within each ensemble, providing a robust definition and analysis of different configurations (Röder et al. 2020).
4 Examples
The computational energy landscape framework has been applied for a wide range of biomolecules. This research includes commonly studied systems such as ubiquitin (Röder and Wales, 2018a), key transformations of nucleic acids (Cragnolini et al., 2017; Chakraborty and Wales, 2018; Xiao et al., 2019), smaller disordered peptides (Joseph and Wales, 2018), and biomolecular interactions and binding (Röder et al., 2020). Some of these case studies have been highlighted in previous reviews (Joseph et al., 2017; Röder et al., 2020]. Here, we present two recent examples; the first showing how the methodology can be applied to larger and more complex molecular rearrangements, and the second examining phosphorylation of a protein, and how these chemical modifications modify the landscape.
4.1 Influenza a Hemagglutinin Fusion Pathway
Infection by Influenza A is associated with binding of the trimeric hemagglutinin (HA) surface glycoprotein to sialic acid at the termini of glycans in a host cell. First, the HA protein is cleaved into two chains, HA1 and HA2. A key event in the infection pathway is the dissociation of the N-terminal region of HA2 from a helical stem and formation of an extended helix in a “spring-loading” mechanism (Carr and Kim, 1993). The landscape for this process has been characterised to connect pre-fusion (Lin et al., 2012) and post-fusion coiled-coil structures, using a minimal model based on amino acids 33–172 of HA (Burke et al., 2020).
The predicted pathway involves a two-stage process for conversion of the B-loop into a helical conformation, while the highest barriers are associated with rearrangements that result in reorientation of helical sequences (Burke et al., 2020). The corresponding landscape exhibits a significant range of substructures on various energy and length scales. At the most coarse-grained level there are two principal funnels, associated with the pre- and post-fusion structures, separated by a high barrier (Figure 1). This overall structure is consistent with a switch, with an essentially irreversible transformation on the relevant experimental time scale.
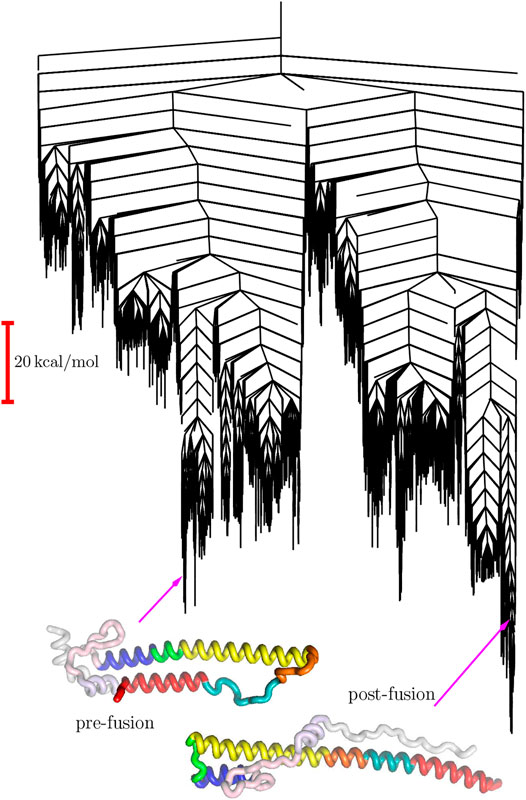
FIGURE 1. Disconnectivity graph for the HA2 system marking the locations of the pre-fusion and post-fusion minima (Burke et al., 2020).
4.2 A Phosphorylated Protein
Phosphorylation can induce significant changes in the relative stabilities of alternative protein conformations, and hence change the organisation of the energy landscape. Such changes have been seen for a variety of intrinsically disordered proteins, suggesting that reorganisation may be associated with specific stabilisation of marginally metastable states and the associated funnels in the energy landscape. We have investigated one particular example, namely eukaryotic translation initiation factor 4E (eIF4E) binding protein 2 (4E-BP2), a 120-residue system that exhibits local secondary structure (Fletcher et al., 1998). In the unphosphorylated state, 4E-BP2 competes with eukaryotic translation initiation factor 4G (eIF4G) for binding to eIF4E, and the resulting complex exhibits α-helical structure (Fukuyo et al., 2011). Phosphorylation can occur at up to five sites, and stabilises a four-stranded β-sheet structure, with low affinity for eIF4E (Bah et al., 2015). The balance of stability is shifted to the β-sheet upon phosphorylation at two sites, but in this state the protein retains some affinity for eIF4E. This balance is also strongly affected by mutations.
To understand these effects we explored the energy landscapes of the doubly phosphorylated wild type (pWT) protein and two mutants, p(D33K) and p(Y54A/L59A) (Kang et al., 2020). All the potential and free energy landscapes exhibit multifunnel organisation, with four states competing with the folded conformation in pWT. Differences in relative stability can be interpreted in terms of missing hydrogen-bonds, and the p(D33K) landscape exhibits the most frustration, with four states of similar stability separated by high barriers. Some of the states with minority equilibrium populations in pWT include α helical structure in the binding motif, which would explain the residual affinity for eIF4E, and could account for NOE signals that do not arise from the dominant folded conformations. The minority states also feature stabilisation from hydrogen-bonds to the two phosphate groups. Hence phosphorylation causes reorganisation of the multifunnel landscape, which appears to be associated with control of binding affinity functionality. This changes is reminiscent of the reorganisation caused by mutations (Röder and Wales, 2018c), and can also be observed in nucleic acids (Sharpe et al., 2020); Röder et al., 2021).
5 Intrinsically Disordered Systems
While globular proteins associated with a single function are expected to exhibit a single funnel landscape, a multifunnel organisation seems necessary to support additional structural ensembles, and hence funnels, required to fulfil distinct functions. In contrast to the energy landscapes characterised for structural glass-formers (de Souza and Wales, 2008; Niblett et al., 2016; de Souza and Wales, 2016), we expect to see a relatively small number of well-defined funnels in such cases. The question then arises: where on this continuous spectrum of energy landscape topographies do we find intrinsically disordered proteins? Descriptions in terms of glassy landscapes may arise from the observation of structural heterogeneity and kinetic trapping in experiments. Due to those features, the experimental and computational study of such systems is more complicated (Baker and Best, 2014; Bhattacharya and Lin, 2019); Strodel, 2021). It is noteworthy that the same observations may be made about non-coding, but functional, RNAs, which also exhibit structural heterogeneity and kinetic trapping. However, these system are rarely described as disordered.
5.1 Distinct Energy Landscape Topographies Exist for IDPs
Further questions arise when we look closer at energy landscapes for intrinsically disordered systems. A NMR study of the Nuclear Coactivator Binding Domain, an intrinsically disordered system, revealed the existence of two distinct states, resembling fold-switch proteins (Kjaergaard et al., 2013). In contrast, the free energy landscape of the pKID region of the cAMP response element-binding (CREB) protein exhibits a shallow funnel, allowing for structural heterogeneity, while upon binding the funnel becomes steeper, leading to well defined structure (Chong and Ham, 2019). Systems described as intrinsically disordered proteins and peptides therefore fall into at least two categories: namely molecules that exhibit a number of competing states, where no state is thermodynamically preferred, and those exhibiting a shallow energy landscape, with no or little topographic bias.
This observation has a number of important consequences. Firstly, in both cases the energy landscape might be altered through external influences, promoting a subset of dominant structures. Secondly, the existence of multifunnel energy landscapes, which are associated with controlled biological function, might hint at an evolutionary development towards specific sequences. Which type of energy landscape is exhibited is dependent on the sequence and the resulting interactions, and can be discussed in these terms (Uversky, 2019). Importantly, these structural preferences lead to differences in binding behaviour, as observed in experiment (Arai et al., 2015). The two binding mechanisms described in this context are induced fit and conformational selection. It is straightforward to correlate these mechanisms with the organisation of the energy landscape. Induced fit relies on flexibility and adaptation of the structure to external drivers, i.e. the structure of the binding partner, and is likely associated with the shallow energy landscapes. The induced fit then restores the topographical bias through the alteration of the folding funnel (Arai et al., 2015; Chong and Ham, 2019). In contrast, multifunnel energy landscapes seem likely to be associated with conformational selection, with specific interactions stabilising the alternative structural ensembles. Schematic representations of these two ideas are shown in Figure 2.
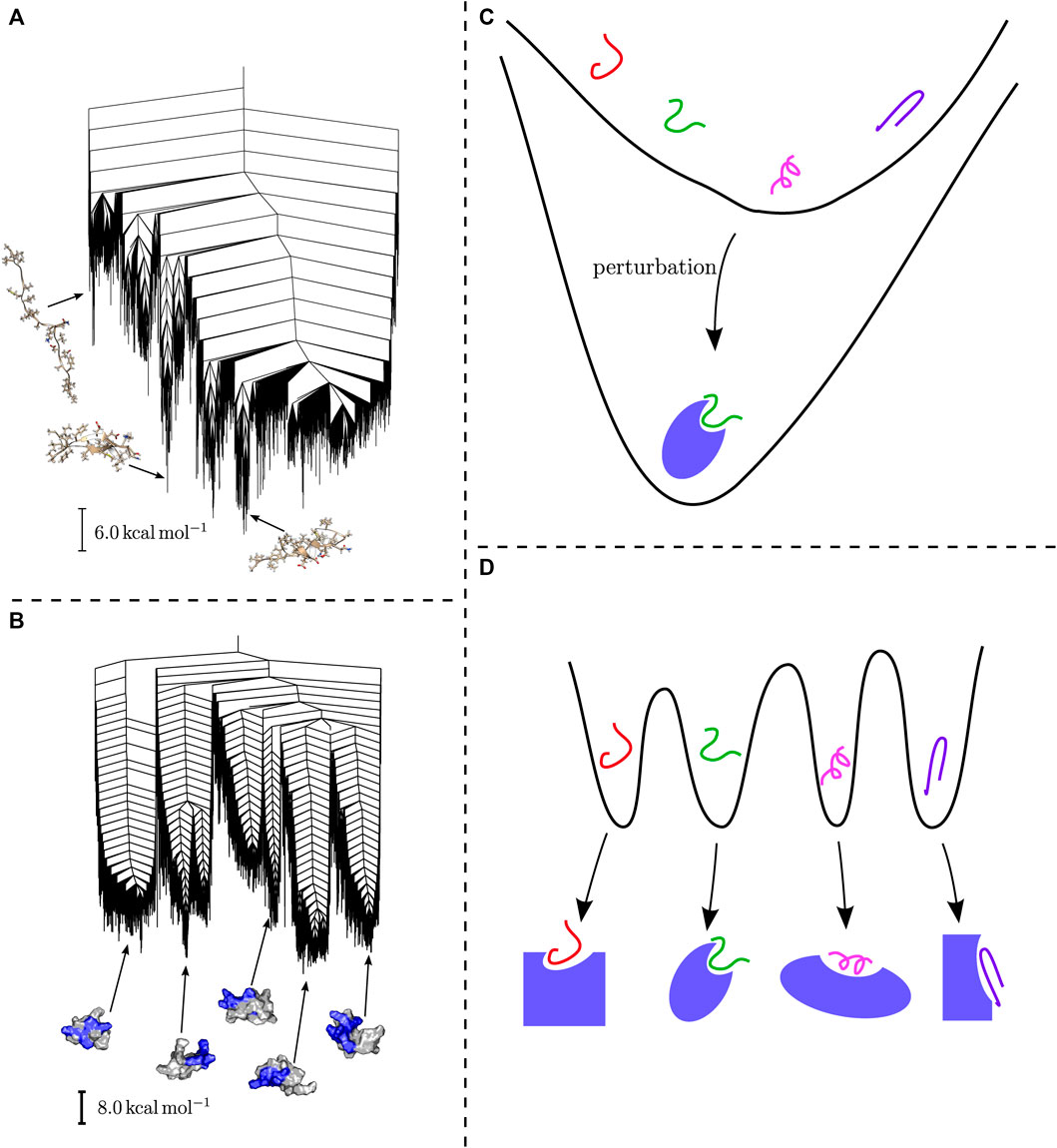
FIGURE 2. (A) The disconnectivity graph for the energy landscape of an amyloid-β monomer adapted from (Röder and Wales, 2018b) exhibits a shallow landscape with small subfunnels, while (B) the landscape for the H4 histone tail, adapted from (Röder, 2021), supports multiple funnels with distinct structural ensembles. (C) Schematic representation of how binding may affect shallow energy landscapes. (D) Conformational selection based on a multifunnel energy landscape allows binding to alternative partners for a single peptide or protein.
This picture is supported by results from the explicit exploration of energy landscapes, which yield important insight into the corresponding organisation and structural ensembles (Chebaro et al., 2015); Joseph and Wales, 2018). For example, the amyloid-β monomer exhibits a shallow folding funnel, with some minor substructure, and significant structural heterogeneity (Röder and Wales, 2018b). In contrast, the energy landscape for the H4 histone tail exhibits multiple funnels, with essentially identical energies, but widely varying associated structural ensembles (Röder, 2021). Disconnectivity graphs for both examples are shown in Figure 2.
Aggregates of misfolded proteins are likely to be associated with high energy barriers and a more frustrated energy landscape (Strodel, 2021; Röder and Wales, 2018b). This observation is perhaps not surprising, given the large number of possible interactions for even two or three peptides with structural heterogeneity. However, this class of energy landscape should be distinguished from the structure expected for IDPs, which arise from different considerations. This distinction is important considering the association of multiple funnels and multiple functions. Amyloid aggregation is a process that probably has not been challenged by evolution, as it only occurs late in life.
6 Machine Learning and Energy Landscape Exploration
Here we briefly consider the future potential of machine learning methods for exploration of energy landscapes and hence contributing to this perspective. Structure prediction based on knowledge-based approaches can now locate low energy conformations efficiently given appropriate data (Tunyasuvunakool et al., 2021); some protein and peptide targets, especially disordered systems, are not yet accessible (Strodel, 2021). Predicting a single structure is not sufficient for a survey of the landscape, as many biomolecules exhibit multiple structural ensembles, which define multiple functionality. However, identifying the structures that underpin multifunnel landscapes may be a realistic target for data driven methods, and would provide input for pathway analysis employing geometry optimisation techniques, as described above. Machine learning also has the potential to accelerate some of this geometry optimisation directly (Garrido Torres et al., 2019); Yang et al., 2021). The prediction of nucleic acid structures represents a further ongoing challenge.
Important advances have also been made in the application of ML-based methods throughout the field of protein simulations (Noé et al. (2020)). Examples include efficient and more accurate potentials [for example Smith et al. (2017)], design of coarse-grained potentials (Wang et al. (2019)), and work on general frameworks to represent atoms and molecules based on the underlying physics (Schütt et al., 2018; Musil et al., 2021). In addition, subsequent analysis steps, for example the calculation of kinetic properties, can also benefit from ML-based frameworks (Mardt et al., 2018; Olsson and Noé, 2019). Such advances should provide more accurate physically motivated models. The bottleneck for most current simulations, especially those that aim to explore large parts of the energy landscape, is the high computational cost. Faster and more accurate methods will find widespread applications for enhanced sampling (Ribeiro et al., 2018; Noé et al., 2019) and treatment of high-dimensional systems (Olsson and Noé, 2019), with new physical insight beyond structure prediction.
Data Availability Statement
The original contributions presented in the study are included in the article/Supplementary Material, further inquiries can be directed to the corresponding author.
Author Contributions
All authors listed have made a substantial, direct, and intellectual contribution to the work and approved it for publication.
Funding
KR is funded by the Cambridge Philosophical Society. DJW gratefully acknowledges funding from EPSRC Grant EP/N035003/1.
Conflict of Interest
The authors declare that the research was conducted in the absence of any commercial or financial relationships that could be construed as a potential conflict of interest.
Publisher’s Note
All claims expressed in this article are solely those of the authors and do not necessarily represent those of their affiliated organizations, or those of the publisher, the editors and the reviewers. Any product that may be evaluated in this article, or claim that may be made by its manufacturer, is not guaranteed or endorsed by the publisher.
References
Anfinsen, C. B. (1973). Principles that Govern the Folding of Protein Chains. Science 181, 223–230. doi:10.1126/science.181.4096.223
Anfinsen, C. B. (1972). The Formation and Stabilization of Protein Structure. Biochem. J. 128, 737–749. doi:10.1042/bj1280737
Arai, M., Sugase, K., Dyson, H. J., and Wright, P. E. (2015). Conformational Propensities of Intrinsically Disordered Proteins Influence the Mechanism of Binding and Folding. Proc. Natl. Acad. Sci. USA 112, 9614–9619. doi:10.1073/pnas.1512799112
Athènes, M., and Bulatov, V. V. (2014). Path Factorization Approach to Stochastic Simulations. Phys. Rev. Lett. 113, 230601. doi:10.1103/physrevlett.113.230601
Athènes, M., Kaur, S., Adjanor, G., Vanacker, T., and Jourdan, T. (2019). Elastodiffusion and Cluster Mobilities Using Kinetic Monte Carlo Simulations: Fast First-Passage Algorithms for Reversible Diffusion Processes. Phys. Rev. Mater. 3, 103802. doi:10.1103/physrevmaterials.3.103802
Bah, A., Vernon, R. M., Siddiqui, Z., Krzeminski, M., Muhandiram, R., Zhao, C., et al. (2015). Folding of an Intrinsically Disordered Protein by Phosphorylation as a Regulatory Switch. Nature 519, 106–109. doi:10.1038/nature13999
Bai, C., and Warshel, A. (2020). Critical Differences between the Binding Features of the Spike Proteins of Sars-Cov-2 and Sars-Cov. J. Phys. Chem. B 124, 5907–5912. doi:10.1021/acs.jpcb.0c04317
Baker, C., and Best, R. (2014). Insights into the Binding of Intrinsically Disordered Proteins from Molecular Dynamics Simulation. Wires Comput. Mol. Sci. 4, 182–198. doi:10.1002/wcms.1167
Baker, D. (2019). What Has De Novo Protein Design Taught Us about Protein Folding and Biophysics? Protein Sci. 28, 678–683. doi:10.1002/pro.3588
Becker, O. M., and Karplus, M. (1998). The Topology of Multidimensional Potential Energy Surfaces: Theory and Application to Peptide Structure and Kinetics. J. Chem. Phys. 106, 1495–1517. doi:10.1063/1.473299
Bhattacharya, S., and Lin, X. (2019). Recent Advances in Computational Protocols Addressing Intrinsically Disordered Proteins. Biomolecules 9, 146. doi:10.3390/biom9040146
Bolhuis, P. G., Chandler, D., Dellago, C., and Geissler, P. L. (2002). Transition Path Sampling : Throwing Ropes over Rough Mountain Passes, in the Dark. Annu. Rev. Phys. Chem. 53, 291–318. doi:10.1146/annurev.physchem.53.082301.113146
Bryngelson, J. D., Onuchic, J. N., Socci, N. D., and Wolynes, P. G. (1995). Funnels, Pathways, and the Energy Landscape of Protein Folding: A Synthesis. Proteins 21, 167–195. doi:10.1002/prot.340210302
Bryngelson, J. D., and Wolynes, P. G. (1989). Intermediates and Barrier Crossing in a Random Energy Model (With Applications to Protein Folding). J. Phys. Chem. 93, 6902–6915. doi:10.1021/j100356a007
Bryngelson, J. D., and Wolynes, P. G. (1987). Spin Glasses and the Statistical Mechanics of Protein Folding. Proc. Natl. Acad. Sci. USA 84, 7524–7528. doi:10.1073/pnas.84.21.7524
Burke, D. F., Mantell, R. G., Pitt, C. E., and Wales, D. J. (2020). Energy Landscape for the Membrane Fusion Pathway in Influenza a Hemagglutinin from Discrete Path Sampling. Front. Chem. 8, 869. doi:10.3389/fchem.2020.575195
Calvo, F., Schebarchov, D., and Wales, D. J. (2016). Grand and Semigrand Canonical basin-hopping. J. Chem. Theor. Comput. 12, 902–909. doi:10.1021/acs.jctc.5b00962
Carr, C. M., and Kim, P. S. (1993). A spring-loaded Mechanism for the Conformational Change of Influenza Hemagglutinin. Cell 73, 823–832. doi:10.1016/0092-8674(93)90260-w
Chakraborty, D., and Wales, D. J. (2018). Energy Landscape and Pathways for Transitions between Watson–Crick and Hoogsteen Base Pairing in Dna. J. Phys. Chem. Lett. 9, 229–241. doi:10.1021/acs.jpclett.7b01933
Chebaro, Y., Ballard, A. J., Chakraborty, D., and Wales, D. J. (2015). Intrinsically Disordered Energy Landscapes. Sci. Rep. 5, 10386. doi:10.1038/srep10386
Chen, S. J., and Dill, K. A. (2000). Rna Folding Energy Landscapes. Proc. Natl. Acad. Sci. USA 97, 646–651. doi:10.1073/pnas.97.2.646
Chiti, F., and Dobson, C. M. (2017). Protein Misfolding, Amyloid Formation, and Human Disease: A Summary of Progress over the Last Decade. Annu. Rev. Biochem. 86, 27–68. doi:10.1146/annurev-biochem-061516-045115
Chong, S.-H., and Ham, S. (2019). Folding Free Energy Landscape of Ordered and Intrinsically Disordered Proteins. Sci. Rep. 9, 14927. doi:10.1038/s41598-019-50825-6
Cochran, A. G., Skelton, N. J., and Starovasnik, M. A. (2001). Tryptophan Zippers: Stable, Monomeric β-hairpins. Proc. Natl. Acad. Sci. U.S.A. 98, 5578–5583. doi:10.1073/pnas.091100898
Cragnolini, T., Chakraborty, D., Šponer, J., Derreumaux, P., Pasquali, S., and Wales, D. (2017). Multifunctional Energy Landscape for a Dna G-Quadruplex: An Evolved Molecular Switch. J. Chem. Phys. 147, 152715. doi:10.1063/1.4997377
de Souza, V. K., and Wales, D. J. (2008). Energy Landscapes for Diffusion: Analysis of Cage-Breaking Processes (13 Pages). J. Chem. Phys. 129, 164507. doi:10.1063/1.2992128
de Souza, V. K., and Wales, D. J. (2016). The Potential Energy Landscape for Crystallisation of a Lennard-jones Fluid. J. Stat. Mech. 2016, 074001. doi:10.1088/1742-5468/2016/07/074001
Dellago, C., and Bolhuis, P. G. (2009). Transition Path Sampling and Other Advanced Simulation Techniques for Rare Events. Adv. Polym. Sci. 221, 167–233.
Dou, J., Vorobieva, A. A., Sheffler, W., Doyle, L. A., Park, H., Bick, M. J., et al. (2018). De Novo design of a Fluorescence-Activating β-barrel. Nature 561, 485–491. doi:10.1038/s41586-018-0509-0
Fletcher, C. M., McGuire, A. M., Gingras, A.-C., Li, H., Matsuo, H., Sonenberg, N., et al. (1998). 4e binding proteins inhibit the translation factor eif4e without folded structure. Biochemistry 37, 9–15. doi:10.1021/bi972494r
Fukuyo, A., In, Y., Ishida, T., and Tomoo, K. (2011). Structural scaffold for eif4e binding selectivity of 4e-bp isoforms: crystal structure of eif4e binding region of 4e-bp2 and its comparison with that of 4e-bp1. J. Pept. Sci. 17, 650–657. doi:10.1002/psc.1384
Garrido Torres, J. A., Jennings, P. C., Hansen, M. H., Boes, J. R., and Bligaard, T. (2019). Low-scaling Algorithm for Nudged Elastic Band Calculations Using a Surrogate Machine Learning Model. Phys. Rev. Lett. 122, 156001. doi:10.1103/PhysRevLett.122.156001
Geyer, G. (1991). “Markov Chain Monte Carlo Maximum Likelihood,” in Computing Science and Statistics: Proceedings of the 23rd Symposium on the Interface, Seattle, Washington, April 21-24, 1991. Editor E. K. Keramidas (Virginia, US: Interface Foundation of North America), 156.
Huang, P.-S., Boyken, S. E., and Baker, D. (2016). The Coming of Age of De Novo Protein Design. Nature 537, 320–327. doi:10.1038/nature19946
Hukushima, K., and Nemoto, K. (1996). Exchange Monte Carlo Method and Application to Spin Glass Simulations. J. Phys. Soc. Jpn. 65, 1604. doi:10.1143/jpsj.65.1604
Joseph, J. A., Chakraborty, D., and Wales, D. J. (2019). Energy Landscape for Fold-Switching in Regulatory Protein Rfah. J. Chem. Theor. Comput. 15, 731–742. doi:10.1021/acs.jctc.8b00912
Joseph, J. A., Röder, K., Chakraborty, D., Mantell, R. G., and Wales, D. J. (2017). Exploring Biomolecular Energy Landscapes. Chem. Commun. 53, 6974–6988. doi:10.1039/c7cc02413d
Joseph, J. A., and Wales, D. J. (2018). Intrinsically Disordered Landscapes for Human Cd4 Receptor Peptide. J. Phys. Chem. B 122, 11906–11921. doi:10.1021/acs.jpcb.8b08371
Kang, W., Jiang, F., Wu, Y.-D., and Wales, D. J. (2020). Multifunnel Energy Landscapes for Phosphorylated Translation Repressor 4e-Bp2 and its Mutants. J. Chem. Theor. Comput. 16, 800–810. doi:10.1021/acs.jctc.9b01042
Karplus, M. (2011). Behind the Folding Funnel Diagram. Nat. Chem. Biol. 7, 401–404. doi:10.1038/nchembio.565
Kjaergaard, M., Andersen, L., Nielsen, L., and Teilum, K. (2013). A Folded State of Ligand-free Nuclear Coactivator Binding Domain (Ncbd) Underlies Plasticity in Ligand Recognition. Biochemistry 52, 1686–1693. doi:10.1021/bi4001062
Leary, R. H., and Doye, J. P. K. (1999). New Tetrahedral Global Minimum for the 98-atom Lennard-jones Cluster. Phys. Rev. E 60, R6320–R6322. doi:10.1103/physreve.60.r6320
Leopold, P. E., Montal, M., and Onuchic, J. N. (1992). Protein Folding Funnels: a Kinetic Approach to the Sequence-Structure Relationship. Proc. Natl. Acad. Sci. USA 89, 8721–8725. doi:10.1073/pnas.89.18.8721
Levinthal, C. (1968). Are There Pathways for Protein Folding? J. Chim. Phys. Pcb. 65, 44. doi:10.1051/jcp/1968650044
Levinthal, C. (1969). “How to Fold Graciously,” in Mossbauer Spectroscopy in Biological Systems, Proceedings of a Meeting held at Allerton House, Monticello, Illinois, March 17 and 18. Editors P. Debrunner, J. Tsibris, and E. Munck (Urbana: University of Illinois Press), 22.
Levinthal, C. (1966). Molecular Model-Building by Computer. Sci. Am. 214, 42–53. doi:10.1038/scientificamerican0666-42
Li, P. T. X., Vieregg, J., and Tinoco, I. (2008). How Rna Unfolds and Refolds. Annu. Rev. Biochem. 77, 77–100. doi:10.1146/annurev.biochem.77.061206.174353
Li, Z., and Scheraga, H. A. (1987). Monte Carlo-Minimization Approach to the Multiple-Minima Problem in Protein Folding. Proc. Natl. Acad. Sci. USA 84, 6611–6615. doi:10.1073/pnas.84.19.6611
Lin, Y. P., Xiong, X., Wharton, S. A., Martin, S. R., Coombs, P. J., Vachieri, S. G., et al. (2012). Evolution of the Receptor Binding Properties of the Influenza A(h3n2) Hemagglutinin. Proc. Natl. Acad. Sci. USA 109, 21474–21479. doi:10.1073/pnas.1218841110
MacKay, R. S., and Robinson, J. D. (2018). Aggregation of Markov Flows I: Theory. Philos. T. Roy. Soc. A. 376. doi:10.1098/rsta.2017.0232
Mardt, A., Pasquali, L., Wu, H., and Noé, F. (2018). Vampnets for Deep Learning of Molecular Kinetics. Nat. Commun. 9, 5. doi:10.1038/s41467-017-02388-1
Morcos, F., Schafer, N. P., Cheng, R. R., Onuchic, J. N., and Wolynes, P. G. (2014). Coevolutionary Information, Protein Folding Landscapes, and the Thermodynamics of Natural Selection. Proc. Natl. Acad. Sci. USA 111, 12408–12413. doi:10.1073/pnas.1413575111
Murrell, J. N., and Laidler, K. J. (1968). Symmetries of Activated Complexes. Trans. Faraday. Soc. 64, 371–377. doi:10.1039/tf9686400371
Musil, F., Grisafi, A., Bartók, A. P., Ortner, C., Csányi, G., and Ceriotti, M. (2021). Physics-inspired Structural Representations for Molecules and Materials. Chem. Rev. 121, 9759–9815. doi:10.1021/acs.chemrev.1c00021
Niblett, S. P., de Souza, V. K., Stevenson, J. D., and Wales, D. J. (2016). Dynamics of a Molecular Glass Former: Energy Landscapes for Diffusion in Ortho-Terphenyl. J. Chem. Phys. 145, 024505. doi:10.1063/1.4954324
Noé, F., de Fabritiis, G., and Clementi, C. (2020). Machine Learning for Protein Folding and Dynamics. Curr. Opin. Struct. Biol. 60, 77–84. doi:10.1016/j.sbi.2019.12.005
Noé, F., and Fischer, S. (2008). Transition Networks for Modeling the Kinetics of Conformational Change in Macromolecules. Curr. Opin. Struc. Biol. 18, 154–162. doi:10.1016/j.sbi.2008.01.008
Noé, F., Olsson, S., Köhler, J., and Wu, H. (2019). Boltzmann Generators: Sampling Equilibrium States of many-body Systems with Deep Learning. Science 365, eaaw1147. doi:10.1126/science.aaw1147
Olsson, S., and Noé, F. (2019). Dynamic Graphical Models of Molecular Kinetics. Proc. Natl. Acad. Sci. 116, 15001–15006. doi:10.1073/pnas.1901692116
Onuchic, J. N., Luthey-Schulten, Z., and Wolynes, P. G. (1997). Theory of Protein Folding: the Energy Landscape Perspective. Annu. Rev. Phys. Chem. 48, 545–600. doi:10.1146/annurev.physchem.48.1.545
Onuchic, J. N., and Wolynes, P. G. (2004). Theory of Protein Folding. Curr. Opin. Struct. Biol. 14, 70–75. doi:10.1016/j.sbi.2004.01.009
Prada-Gracia, D., Gómez-Gardenes, J., Echenique, P., and Falo, F. (2009). Exploring the Free Energy Landscape: From Dynamics to Networks and Back. Plos Comput. Biol. 5, e1000415. doi:10.1371/journal.pcbi.1000415
Rao, F., and Caflisch, A. (2004). The Protein Folding Network. J. Mol. Biol. 342, 299–306. doi:10.1016/j.jmb.2004.06.063
Ribeiro, J. M. L., Bravo, P., Wang, Y., and Tiwary, P. (2018). Reweighted Autoencoded Variational Bayes for Enhanced Sampling (Rave). J. Chem. Phys. 149, 072301. doi:10.1063/1.5025487
Röder, K., Barker, A. M., Whitehouse, A., and Pasquali, S. (2021). Investigating the Structural Changes Due to Adenosine Methylation of the Kaposi’s Sarcoma-Associated Herpes Virus Orf50 Transcript. bioRxiv. doi:10.1101/2019.12.11.123456
Röder, K. (2021). Is the H4 Histone Tail Intrinsically Disordered or Intrinsically Multifunctional? Phys. Chem. Chem. Phys. 23, 5134–5142. doi:10.1039/d0cp05405d
Röder, K., Joseph, J. A., Husic, B. E., and Wales, D. J. (2019). Energy Landscapes for Proteins: From Single Funnels to Multifunctional Systems. Adv. Theor. Simul. 2, 1800175. doi:10.1002/adts.201800175
Röder, K., Stirnemann, G., Dock-Bregeon, A.-C., Wales, D. J., and Pasquali, S. (2020). Structural Transitions in the Rna 7sk 5′ Hairpin and Their Effect on Hexim Binding. Nucleic Acids Res. 48, 373–389. doi:10.1093/nar/gkz1071
Röder, K., and Wales, D. J. (2018a). Analysis of the Ub to Ub-Cr Transition in Ubiquitin. Biochemistry 57, 6180–6186. doi:10.1021/acs.biochem.8b00770
Röder, K., and Wales, D. J. (2018b). Energy Landscapes for the Aggregation of Aβ17−42. J. Am. Chem. Soc. 140, 4018–4027. doi:10.1021/jacs.7b12896
Röder, K., and Wales, D. J. (2018c). Evolved Minimal Frustration in Multifunctional Biomolecules. J. Phys. Chem. B 122, 10989–10995. doi:10.1021/acs.jpcb.8b03632
Schroeder, S. J. (2018). Challenges and Approaches to Predicting Rna with Multiple Functional Structures. RNA 24, 1615–1624. doi:10.1261/rna.067827.118
Schütt, K. T., Sauceda, H. E., Kindermans, P.-J., Tkatchenko, A., and Müller, K.-R. (2018). Schnet – a Deep Learning Architecture for Molecules and Materials. J. Chem. Phys. 148, 241722. doi:10.1063/1.5019779
Shang, C., and Wales, D. J. (2014). Communication: Optimal Parameters for basin-hopping Global Optimization Based on Tsallis Statistics. J. Chem. Phys. 141, 071101. doi:10.1063/1.4893344
Sharpe, D. J., Röder, K., and Wales, D. J. (2020). Energy Landscapes of Deoxyxylo- and Xylo-Nucleic Acid Octamers. J. Phys. Chem. B 124, 4062–4068. doi:10.1021/acs.jpcb.0c01420
Sharpe, D. J., and Wales, D. J. (2020). Efficient and Exact Sampling of Transition Path Ensembles on Markovian Networks. J. Chem. Phys. 153, 024121. doi:10.1063/5.0012128
Sharpe, D. J., and Wales, D. J. (2021a). Numerical Analysis of First-Passage Processes in Finite Markov Chains Exhibiting Metastability. Phys. Rev. E 104, 015301. doi:10.1103/PhysRevE.104.015301
Sharpe, D. J., and Wales, D. J. (2021b). Perspective: Nearly Reducible Finite Markov Chains: Theory and Algorithms. J. Chem. Phys. 155, 140901. doi:10.1063/5.0060978
Shoemark, D. K., Colenso, C. K., Toelzer, C., Gupta, K., Sessions, R. B., Davidson, A. D., et al. (2021). Molecular Simulations Suggest Vitamins, Retinoids and Steroids as Ligands of the Free Fatty Acid Pocket of the Sars-Cov-2 Spike Protein. Angew. Chem. Int. Edit. 60, 7098–7110. doi:10.1002/anie.202015639
Sikora, M., von Bülow, S., Blanc, F. E. C., Gecht, M., Covino, R., and Hummer, G. (2021). Computational Epitope Map of Sars-Cov-2 Spike Protein. PLOS Comput. Biol. 17, e1008790. doi:10.1371/journal.pcbi.1008790
Smith, J. S., Isayev, O., and Roitberg, A. E. (2017). Ani-1: an Extensible Neural Network Potential with Dft Accuracy at Force Field Computational Cost. Chem. Sci. 8, 3192–3203. doi:10.1039/c6sc05720a
Solomatin, S. V., Greenfeld, M., Chu, S., and Herschlag, D. (2010). Multiple Native States Reveal Persistent Ruggedness of an Rna Folding Landscape. Nature 463, 681–684. doi:10.1038/nature08717
Stevenson, J. D., and Wales, D. J. (2014). Communication: Analysing Kinetic Transition Networks for Rare Events. J. Chem. Phys. 141, 041104. doi:10.1063/1.4891356
Strodel, B. (2021). Energy Landscapes of Protein Aggregation and Conformation Switching in Intrinsically Disordered Proteins. J. Mol. Biol. 433, 167182. doi:10.1016/j.jmb.2021.167182
Sutherland-Cash, K., Wales, D., and Chakrabarti, D. (2015). Free Energy basin-hopping. Chem. Phys. Lett. 625, 1–4. doi:10.1016/j.cplett.2015.02.015
Swendsen, R. H., and Wang, J.-S. (1986). Replica Monte Carlo Simulation of Spin-Glasses. Phys. Rev. Lett. 57, 2607. doi:10.1103/physrevlett.57.2607
Swinburne, T. D., Kannan, D., Sharpe, D. J., and Wales, D. J. (2020). Rare Events and First Passage Time Statistics from the Energy Landscape. J. Chem. Phys. 153, 134115. doi:10.1063/5.0016244
Swinburne, T. D., and Wales, D. J. (2020). Defining, Calculating, and Converging Observables of a Kinetic Transition Network. J. Chem. Theor. Comput. 16, 2661–2679. doi:10.1021/acs.jctc.9b01211
Thirumalai, D. (1998). Native Secondary Structure Formation in Rna May Be a Slave to Tertiary Folding. Proc. Natl. Acad. Sci. USA 95, 11506–11508. doi:10.1073/pnas.95.20.11506
Thirumalai, D., and Woodson, S. A. (1996). Kinetics of Folding of Proteins and Rna. Acc. Chem. Res. 29, 433–439. doi:10.1021/ar9500933
Tinoco, I., and Bustamante, C. (1999). How Rna Folds. J. Mol. Biol. 293, 271–281. doi:10.1006/jmbi.1999.3001
Trygubenko, S. A., and Wales, D. J. (2006). Graph Transformation Method for Calculating Waiting Times in Markov Chains. J. Chem. Phys. 124, 234110. doi:10.1063/1.2198806
Tunyasuvunakool, K., Adler, J., Wu, Z., Green, T., Zielinski, M., Žídek, A., et al. (2021). Highly Accurate Protein Structure Prediction for the Human Proteome. Nature 596, 590–596. doi:10.1038/s41586-021-03828-1
Uversky, V. N. (2019). Intrinsically Disordered Proteins and Their “Mysterious” (Meta)physics. Front. Phys. 7, 10. doi:10.3389/fphy.2019.00010
Wales, D. J. (2009). Calculating Rate Constants and Committor Probabilities for Transition Networks by Graph Transformation. J. Chem. Phys. 130, 204111. doi:10.1063/1.3133782
Wales, D. J. (2002). Discrete Path Sampling. Mol. Phys. 100, 3285–3305. doi:10.1080/00268970210162691
Wales, D. J., and Doye, J. P. K. (1997). Global Optimization by basin-hopping and the Lowest Energy Structures of Lennard-jones Clusters Containing up to 110 Atoms. J. Phys. Chem. A. 101, 5111–5116. doi:10.1021/jp970984n
Wales, D. J. (2010). Energy Landscapes: Some New Horizons. Curr. Opin. Struc. Biol. 20, 3–10. doi:10.1016/j.sbi.2009.12.011
Wales, D. J. (2018). Exploring Energy Landscapes. Ann. Rev. Phys. Chem. 69, 401–425. doi:10.1146/annurev-physchem-050317-021219
Wales, D. J., Miller, M. A., and Walsh, T. R. (1998). Archetypal Energy Landscapes. Nature 394, 758–760. doi:10.1038/29487
Wales, D. J., and Salamon, P. (2014). Observation Time Scale, Free-Energy Landscapes, and Molecular Symmetry. P. Natl. Acad. Sci. USA 111, 617–622. doi:10.1073/pnas.1319599111
Wales, D. J., and Scheraga, H. A. (1999). Global Optimization of Clusters, Crystals and Biomolecules. Science 285, 1368–1372. doi:10.1126/science.285.5432.1368
Wales, D. J. (2004). Some Further Applications of Discrete Path Sampling to Cluster Isomerization. Mol. Phys. 102, 891–908. doi:10.1080/00268970410001703363
Wales, D. J. (2013). Surveying a Complex Potential Energy Landscape: Overcoming Broken Ergodicity Using basin-sampling. Chem. Phys. Lett. 584, 1–9. doi:10.1016/j.cplett.2013.07.066
Waman, V. P., Sen, N., Varadi, M., Daina, A., Wodak, S. J., Zoete, V., et al. (2021). The Impact of Structural Bioinformatics Tools and Resources on Sars-Cov-2 Research and Therapeutic Strategies. Brief. Bioinform. 22, 742–768. doi:10.1093/bib/bbaa362
Wang, J., Olsson, S., Wehmeyer, C., Pérez, A., Charron, N. E., de Fabritiis, G., et al. (2019). Machine Learning of Coarse-Grained Molecular Dynamics Force fields. ACS Cent. Sci. 5, 755–767. doi:10.1021/acscentsci.8b00913
Wolynes, P. G., Eaton, W. A., and Fersht, A. R. (2012). Chemical Physics of Protein Folding. Proc. Natl. Acad. Sci. USA 109, 17770–17771. doi:10.1073/pnas.1215733109
Wolynes, P. G. (2015). Evolution, Energy Landscapes and the Paradoxes of Protein Folding. Biochimie 119, 218–230. doi:10.1016/j.biochi.2014.12.007
Xiao, S., Sharpe, D. J., Chakraborty, D., and Wales, D. J. (2019). Energy Landscapes and Hybridization Pathways for Dna Hexamer Duplexes. J. Phys. Chem. Lett. 10, 6771–6779. doi:10.1021/acs.jpclett.9b02356
Keywords: energy landscapes, multiple functions, biomolecules, folding, disorder, structural heterogeneity
Citation: Röder K and Wales DJ (2022) The Energy Landscape Perspective: Encoding Structure and Function for Biomolecules. Front. Mol. Biosci. 9:820792. doi: 10.3389/fmolb.2022.820792
Received: 23 November 2021; Accepted: 07 January 2022;
Published: 27 January 2022.
Edited by:
Naoto Hori, University of Nottingham, United KingdomReviewed by:
Simon Olsson, Chalmers University of Technology, SwedenCopyright © 2022 Röder and Wales. This is an open-access article distributed under the terms of the Creative Commons Attribution License (CC BY). The use, distribution or reproduction in other forums is permitted, provided the original author(s) and the copyright owner(s) are credited and that the original publication in this journal is cited, in accordance with accepted academic practice. No use, distribution or reproduction is permitted which does not comply with these terms.
*Correspondence: David J. Wales, ZHczNEBjYW0uYWMudWs=