- 1Department of Biochemistry and Cellular and Molecular Biology, University of Tennessee, Knoxville, TN, United States
- 2UT-ORNL Graduate School of Genome Science and Technology, Knoxville, TN, United States
The receptor RORγ belongs to the nuclear receptor superfamily that senses small signaling molecules and regulates at the gene transcription level. Since RORγ has a high basal activity and plays an important role in immune responses, inhibitors targeting this receptor have been a focus for many studies. The receptor-ligand interaction is complex, and often subtle differences in ligand structure can determine its role as an inverse agonist or an agonist. We examined more than 130 existing RORγ crystal structures that have the same receptor complexed with different ligands. We reported the features of receptor-ligand interaction patterns and the differences between agonist and inverse agonist binding. Specific changes in the contact interaction map are identified to distinguish active and inactive conformations. Further statistical analysis of the contact interaction patterns using principal component analysis reveals a dominant mode which separates allosteric binding vs. canonical binding and a second mode which may indicate active vs. inactive structures. We also studied the nature of constitutive activity by performing a 100-ns computer simulation of apo RORγ. Using constitutively active nuclear receptor CAR as a comparison, we identified a group of conserved contacts that have similar contact strength between the two receptors. These conserved contact interactions, especially a couple key contacts in H11–H12 interaction, can be considered essential to the constitutive activity of RORγ. These protein-ligand and internal protein contact interactions can be useful in the development of new drugs that direct receptor activity.
Introduction
The nuclear receptor (NR) superfamily is a group of important transcription factors that detect the presence of specific compounds using their ligand-binding domain (LBD) and respond by modulating gene transcription, which is directed through interaction between specific DNA response elements and the DNA-binding domain (DBD) and interaction between co-activators and the LBD (Helsen et al., 2012; Weikum et al., 2018). Well-known examples of the NR superfamily include estrogen receptor, androgen receptor, glucocorticoid receptor, vitamin D receptor, peroxisome proliferator-activated receptor, retinoid receptor, thyroid hormone receptors, and many others. While the structures of the DBD and the LBD are highly conserved, there is a highly varied and largely unstructured N-terminal domain (NTD) that also plays an important role in the function of these transcription factors (Kumar and Thompson, 2003; Simons et al., 2014).
One of the important NRs is called RAR-related orphan receptor (ROR), since initially ROR was discovered as an orphan receptor that is related to retinoid acid receptor (RAR) (Solt and Burris, 2012; Zhang et al., 2015). ROR was found to play important roles in regulating immune responses and circadian rhythm (Takeda et al., 2012; Cook et al., 2015). Furthermore, ROR was also found to be one of the few NRs that are constitutively active, meaning the receptor exhibits high basal activity (active without ligand). Since a hyperactive ROR can be tied to autoimmune diseases such as multiple sclerosis and rheumatoid arthritis, identifying potent inverse agonists to regulate ROR is of interest (Zhang et al., 2015). One essential question arises as to how one can efficiently obtain details of the protein-ligand interaction and predict how ligands affect the protein conformation, i.e., turn on or off ROR activity. As detailed below, this remains a puzzle as the ligand-protein pairing for NRs is highly sensitive.
Various structural biology and chemical biology studies have focused on ROR-ligand interactions. Among the three subtypes of ROR (RORα, RORβ, and RORγ), RORγ appears to be very important with the most structural data available, and thus we focus on examining the LBD of the γ-subtype in this study. Indeed, there have been more than 100 X-ray crystallography structures reported in the Protein Data Bank (PDB), all of which are in the monomer form having the identical protein sequence while the only differences are the unique identity of ligand(s) that forms a complex with RORγ. In many previous reports, a set of similar ligands was used to probe the cellular activity and/or biophysical properties of ROR induced by ligand binding. Similar to other NRs, binding of an agonist to the LBD leads to a conformational change that facilitates a more favorable interaction with the co-activators at the activation function 2 (AF2) region of the LBD (Weikum et al., 2018). Alternatively, when an inverse agonist binds to the LBD, co-activator binding becomes inhibited due to (at least in part) the structural changes in helices H10, H11, and/or H12 (Li et al., 2017; Noguchi et al., 2017; Gong et al., 2018). Two mechanisms of inverse agonism that have been observed include: 1) a disorder of helix H12, which would otherwise form part of the binding pocket, reduces available agonist interaction sites and 2) the formation of a “kink” between helices H10 and H11 consequently obstructs the co-activator binding site formed by helix H12, as shown in Figure 1.
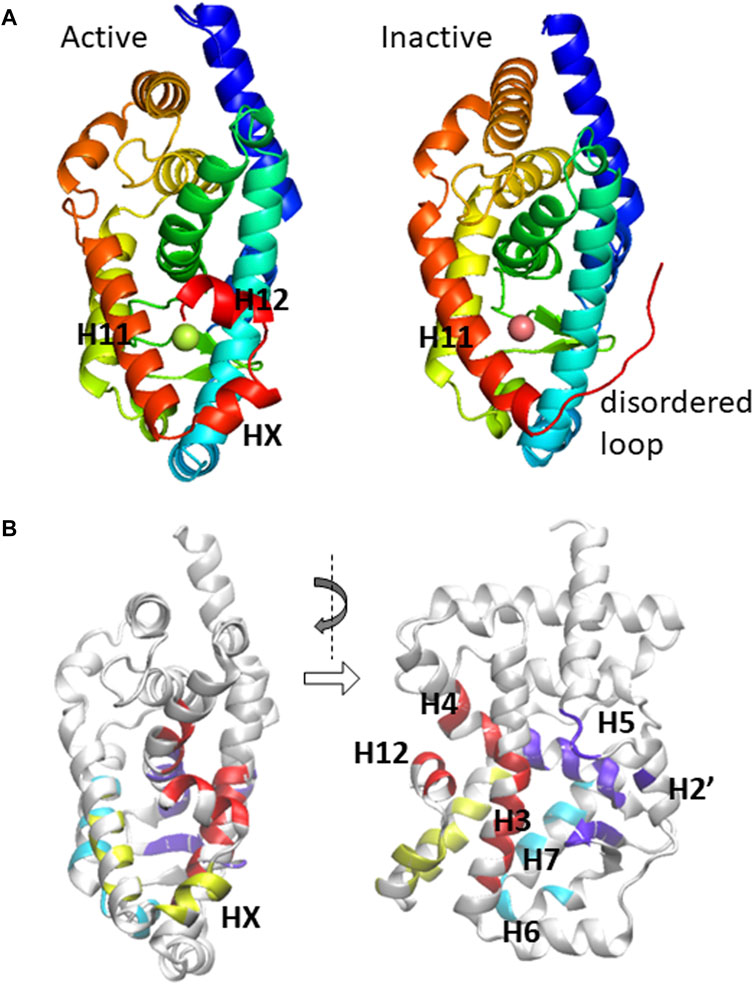
FIGURE 1. (A) The ligand-binding domain of RORγ is displayed in active (PDB: 3L0L) and inactive (PDB: 4QM0) conformations. The important secondary structural elements that undergo conformational change are located at the C-terminal region and are explicitly labeled. (B) The residues that potentially form direct contact with ligands are shown in a canonical front view and a side view.
Often, researchers found that whether specific ligands can turn ROR on or off is quite sensitive. For example, several studies showed that a slight modification of a known agonist or inverse agonist can switch its properties. Specifically, there were reported pairs of ligands (obtained from tertiary sulfonamides, biaryl amides, tertiary amines, benzoxazinones, and other families) that bind at the same binding site; however, the shorter of the two is an agonist while the longer ligand is an inverse agonist or vice versa, exemplified by PDB pairs: 4WPF/4WQP, 5IZ0/5IXK, 6NWU/6NWS, and 6R7K/6R7J (agonist/inverse agonist-bound structures) (Yang et al., 2014; René et al., 2015; Wang et al., 2015a; Marcotte et al., 2016; Gong et al., 2018; Wang et al., 2018; Strutzenberg et al., 2019; von Berg et al., 2019). Using 5IZ0/5IXK as an example, M358 was reported to interact with an inverse agonist ligand Bio399 (synthetic benzoxazinone) and consequently, it affects residue F506 and changes the protein conformation into an inactive form (PDB: 5IXK). In contrast, a similar ligand Bio592 has nearly identical contact interactions with the rest of the binding pocket while lacking the contact with M358, which in turn keeps RORγ in an active conformation (PDB: 5IZ0) (Marcotte et al., 2016). Meanwhile, another intriguing study found that lengthening or shortening modifications of a specific agonist (biphenyl-ethylsulfonyl-phenyl-acetamide) leads to inverse agonism (Wang et al., 2018). As different studies reported different local trigger spots for inverse agonists, one may want to consolidate ROR-ligand interactions and rethink the canonical view that the ligand-directed action comes from a fixed chemical group of the compound with a specific residue of the binding pocket. Instead, the ligands examined in these studies are diverse and distinct from one study to another. It appears that a specific chemical group is not enough to determine the effect of a molecule, and yet the ligand identity clearly affects the interactions with the binding pocket and subsequently the protein activities. For nuclear receptors, there is a challenge on how to connect the ligand identity with directed structural changes and subsequent activities.
We think that a statistical analysis of extensive structural information where one collectively examines protein-ligand contact interaction patterns may provide insight into this challenge. For this work, we studied 136 RORγ structures: 132 with one ligand (or ligand fragments) bound (100+ distinct ligands) from X-ray crystallography experiments (Jin et al., 2010; Fujita-Sato et al., 2011; Fauber et al., 2013; Fauber et al., 2014; van Niel et al., 2014; Yang et al., 2014; Chao et al., 2015; Muegge et al., 2015; René et al., 2015; Santori et al., 2015; Scheepstra et al., 2015; Wang et al., 2015b; Wang et al., 2015c; Enyedy et al., 2016; Hirata et al., 2016; Hintermann et al., 2016; Marcotte et al., 2016; Olsson et al., 2016; Ouvry et al., 2016; Xue et al., 2016; Kallen et al., 2017; Kummer et al., 2017; Li et al., 2017; Noguchi et al., 2017; Carcache et al., 2018; Fukase et al., 2018; Gege et al., 2018; Gong et al., 2018; Kono et al., 2018; Narjes et al., 2018; Noguchi et al., 2018; Sasaki et al., 2018; Schnute et al., 2018; Shirai et al., 2018; Wang et al., 2018; Amaudrut et al., 2019; Duan et al., 2019; Hoegenauer et al., 2019; Kotoku et al., 2019; Lu et al., 2019; Marcoux et al., 2019; Sato et al., 2019; Strutzenberg et al., 2019; Tanis et al., 2019; von Berg et al., 2019; Yukawa et al., 2019; Zhang et al., 2019; Cherney et al., 2020; Duan et al., 2020; Gege et al., 2020; Harikrishnan et al., 2020; Jiang et al., 2020; Liu et al., 2020; Meijer et al., 2020; Nakajima et al., 2020; Shi et al., 2020; Vries et al., 2020; Zhang et al., 2020; Lugar et al., 2021; Meijer et al., 2021; Nakajima et al., 2021; Ruan et al., 2021; Yang et al., 2021). Additionally, there was also a report of 12 structures (with two ligands bound) (de Vries et al., 2021). The full list of PDBs and their associated properties can be found in the Supplementary Data File S1. We did not include the double-liganded structures in the analysis since almost all the ligands in those structures have been crystalized with RORγ previously, and thus these ligand-protein contacts have already been included in our analysis. There might be new RORγ structures deposited in the PDB since the time of our structural bioinformatics research, and any newly reported RORγ structures after that time would not be included in the current analysis. However, we expect that the results of our statistical analyses and the conclusions drawn should still hold.
The current work has two main focuses. The first one is the statistical analyses of the protein-ligand interactions, obtained from previous experimental studies in which each examined ligand or multiple ligands interact with the binding pocket. The comparison across all ligands will provide a more comprehensive picture of the molecular interactions that differentiate between agonists and inverse agonists, and potentially illustrate the mechanism (structural change) by which each ligand imposes its effect. The second focus is the nature of NR constitutive activity. The RORs have a high basal activity and thus they are considered to be active without any ligands. Such constitutive activity of receptors, including many prominent examples from the NR and GPCR families, are difficult to study experimentally at times. Often, receptors, including RORγ, do not have structures resolved experimentally in the absence of ligand. Computational study of an apo conformation may provide clues on how they function (Pham et al., 2019a; Rosenberg et al., 2019). Within the nuclear receptor superfamily, only a few wild-type receptors display constitutive activity. Constitutive androstane receptor (CAR) is also deemed to be constitutively active as suggested by its name (Dussault et al., 2002; Xu et al., 2004). The CAR protein functions as a xenobiotic sensor, which detects foreign substances such as drug molecules and metabolizes them primarily in the liver (Xie et al., 2003; Wang et al., 2012). Additionally, a couple other NRs were also suggested to have a high basal activity, such as ERR and SF-1/LRH-1 (Schimmer and White, 2010; Huss et al., 2015). Often, they have a relatively small binding pocket. A previous computational study performed on CAR has shown some essential protein contacts contributing to the constitutive stability of the unliganded CAR (Pham et al., 2019a). By comparing CAR with RORγ, we may gain insights into the important protein interactions that help facilitate the constitutive activity of nuclear receptors in general.
Methods and Systems
Crystal Structure Ensemble of RORs With Various Ligands Bound
The statistical analysis includes a total of 136 X-ray crystal structures. Only four of the structures (PDB: 5K38, 5VB3, 5X8U, and 5X8W) are absent of a ligand and the other 132 structures contain a single ligand at the ligand-binding pocket. The binding pocket mentioned refers to either a canonical, largely enclosed ligand-binding pocket or an adjacent, more exposed allosteric binding site. We did not include the 12 structures with double ligands (one each at canonical and allosteric binding sites).
For the protein component of the complex, all 136 structures contain a single chain of LBD of RORγ. Note that we also include RORγt, an isoform of RORγ that is selectively expressed in the thymus. Although the sequence of RORγt is 21-residues shorter than RORγ at the N-terminal domain (NTD) due to alternative splicing, both RORγt and RORγ have an identical LBD. Among 136 structures, only a few of them (PDBs: 4NB6, 6O98, 6XFV, and 7JH2) were reported using RORγt indices for their residues while the rest used RORγ. Six of them are from gibbon ROR, which only contains a double substitution (K469A/R473A) from human UNP P51449. Another 34 PDBs are single point mutants at C455 (mostly C455S, occasionally C–H or C–E mutations were reported). By visual inspection, these mutations or substitutions are far from the ligand binding pocket, e.g., C455 is at helix H9, thus none of them are directly involved with the protein-ligand contact interaction. Therefore, we do not treat them separately from the wild-type RORγt.
For the 132 structures containing only one ligand, there are a total of 125 distinct ligands. Notably, four PDB pairs (4YPQ and 5C4O, 5K3M and 5X8S, 5NI5 and 5NU1, 5APJ and 5APK) share the same ligands and four additional PDBs (4NB6, 5EJV, 5K3L, and 5NTQ) all share the same synthetic ligand T0901317 (also an agonist for LXR). Even though these pairs and groups may share the same ligand, the protein conformations are not necessarily the same. For example, despite sharing the same inverse agonist, 5APJ is active (due to a fused coactivator) while 5APK is in an inactive conformation (Olsson et al., 2016). The structures of 4YPQ and 5C4O are in different space groups (Scheepstra et al., 2015), whereas 5NI5 and 5NU1 are bound to different coactivators. A unique case, 5G44, contains three ligand fragments in the binding pocket as it was obtained from a cosolvent engineering study (Xue et al., 2016). We treated this three-ligand “cocktail” as a single ligand. One of the 116 PDBs, 6W9J, was removed from the structure database and replaced by 6XAE after we started our study. Since 6W9J has an identical ligand as the one from another structure already in this database, 6W9H, we have included 6XAE in the below analysis and excluded 6W9J. As mentioned in the Introduction section, a few additional structures of ROR-ligand complexes were reported after our search but we did not include them in the study.
Structural Ensemble of Apo ROR From MD Simulation
To construct the initial conformation of the unliganded ROR system, we used a crystal structure of a coactivator-fused ROR (PDB: 5VB3) that is absent of any ligands (Li et al., 2017). The structure is deemed to be in an active conformation, and it was selected from a set of four apo crystal structures (PDB: 5K38, 5VB3, 5X8U, and 5X8W) which are void of agonist ligand binding (Li et al., 2017; Noguchi et al., 2017). It is worth noting that three structures (PDB: 5VB3, 5X8U, and 5X8W) within that set are not fully unliganded since they are either bound to or fused with the coactivator peptide (CoA), while the other structure (PDB: 5K38) without CoA binding has an incomplete C-terminus. As a fused protein complex of the ligand-binding domain of ROR and CoA, the CoA component is believed to assist ROR in remaining in the active conformation. Interestingly, the CoA effect is so strong that the inverse agonist-bound form of this fused protein is still in the active conformation as the PDB 4YMQ shows (Muegge et al., 2015). To simulate our fully apo system, we removed the fused CoA segment from the ROR protein of the crystal structure. The protein contains 243 residues with internal indices (1–243) corresponding to the standard RORγ (UniProt: P51449) A265–S507.
The AMBER14SB forcefield was used for the protein molecules of the simulation, whereas the TIP3P model was used to solvate the system with 11,622 water molecules in a rectangular box. The protonation status of the residues was determined by H++ (Anandakrishnan et al., 2012) at pH of 7.0 and assigned accordingly: Asp and Glu are deprotonated, Arg and Lys are protonated, and all His are singly protonated at the ε position, except His452 and His479 which are protonated at the δ position. Two Cl− counterions were added to neutralize the system.
After the initial setup of the system, we conducted minimization, heating, and production runs using NAMD. NPT simulations were used for the system with T = 300 K and p = 1 atm. The production run time was 100 ns after an initial 5 ns equilibration. The time step was 2 fs, and snapshots were collected every 1 ps.
Statistical Analysis
Contact matrices are calculated to render the structure information at residue-residue contact resolution (Johnson et al., 2015; Clark et al., 2016; Johnson et al., 2018). For the calculation of residue-residue and residue-ligand contacts of these 136 PDBs, hydrogen atoms are excluded from all but the 10 ligands for which they were explicitly reported. Since the hydrogen atoms of proteins were not explicitly reported either, we remove hydrogens from all of the PDBs to obtain a uniform resolution (heavy atom only) of the protein complex systems. A contact
Several analysis methods are used to further render the information contained in the contact matrix ensemble. Besides the principal component analysis (PCA) (Jolliffe, 2002; Brunton and Kutz, 2019) of contacts, statistical analyses such as hierarchical clustering and construction of a dendrogram can be used to classify the protein structures (contact maps), the residues of binding pocket, and the ligands. The distance score between two protein contact matrices
For protein-ligand interactions, we used an I-PCA style of contact statistical analysis (Lindsay et al., 2018). The I-PCA method was initially developed to reveal internal domain structures of semi-structured biopolymers, from large-scale chromosome structures to intrinsically disordered proteins (Das et al., 2020; Lindsay et al., 2021). In those cases, each row (or column) of the mean contact map of the structure ensemble is treated as a linear set of contact variables (the number of rows is the number of monomer units of the system) that symbolize the contact interaction with other unlabeled monomers. Here, we generalize this idea to protein-ligand contacts, i.e., protein residues have a contact variable
Results and Discussion
Conformations With Various Ligand Binding Status Expressed by Contact Matrices
We first use contact interaction matrices to compare the conformations of RORγ structures reported in the PDB database. As mentioned in the previous section, a distance (dissimilarity score
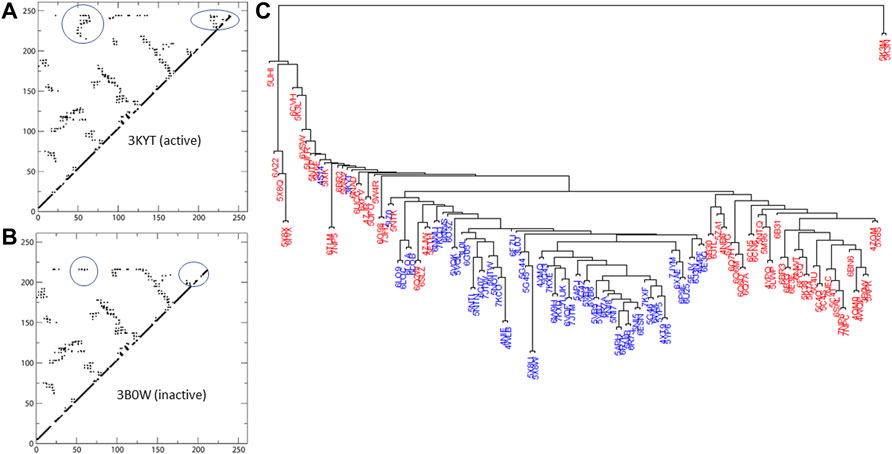
FIGURE 2. Active and inactive structures of RORγ are rendered as contact maps in (A,B), respectively. Based on the similarity matrix, a dendrogram of the structures (active labeled in blue, inactive in red) at the contact level is displayed in (C).
With the exception of some isolated structures that branch off earlier, the bulk of the structures form two main branches in the dendrogram. As seen in Figure 2, the active structures form the majority of one branch, the active branch, whereas the inactive ones concentrate in the other branch. Note that none of the nine structures complexed with allosteric inhibitors (PDBs: 4YPQ, 5C4O, 5C4S, 5C4T, 5C4U, 5LWP, 6SAL, 6TLM, and 6UCG) is in the active branch and most of them are in the inactive branch. Two structure outliers (5K3M and 5K3N) branch off the earliest, since they have distinct conformations compared to the rest of the structures with less contacts being formed. In addition, three of the four apo structures can be found within the “active branch” of the cluster tree and the fourth is found within the “inactive branch.”
We use color labeling to demonstrate the conformation being active (blue) or inactive (red) in Figure 2. Active structures are largely located in one branch of the cluster tree. Note that our definition of active conformation is based on the features of contact matrices as stated below. We could not solely rely on the self-reported status from literature associated with the PDBs, because not all ligands were self-reported as an agonist or an inverse agonist. Additionally, the active or inactive conformation is not always linked to the ligand being reported as an agonist or an inverse agonist. In certain cases, such as a coactivator-fused ROR or due to ROR-coactivator interaction, a known inverse agonist can be “trapped” within the active conformation of the protein (e.g., PDB: 5APJ). However, by visual inspection of the contact interactions, one can clearly observe two distinct patterns of contact maps being formed. The first group of contact maps is predominantly associated with self-identified active conformations and features two regions of contacts that are absent from the second (inactive) group. One of the two regions represents contacts between H3-H4 and HX-H12, whereas the other region contains contacts between HX and H12. Examples of the active and inactive contact maps are displayed in Figure 2.
In practice, we summed the total number of contacts from two regions on the contact maps where Region one is defined as any contacts between residues
Ligand Binding Patterns Revealed by Statistical Analysis of Protein-Ligand Contacts
In general, the ligand binding pockets of NR can be quite large and complex. For most cases, ligands are considered to be completely enclosed inside the LBD of the receptor. A unique aspect of ROR is that it contains an allosteric binding site besides the canonical (orthosteric) binding site (Scheepstra et al., 2015). It was reported further that both sites can be occupied by ligands and exhibit a degree of communication between them (de Vries et al., 2021). Specifically, even when an agonist ligand binds to the canonical site and stabilizes the binding pocket structure, the presence of an allosteric inverse agonist can negate the agonistic effect and turn off the receptor activity (de Vries et al., 2021). Although such complex multivalent interaction is interesting to study and can have deep implications on controlling how the protein functions via allostery (Pham et al., 2019b), our study is limited to only single ligand-protein interaction.
A basic property of ligands which we can investigate is their size and its relationship with the ligand identity as an agonist or an inverse agonist. Here, we chose to characterize each ligand by its total number of atoms. The mean ligand size is
With the same noise filtering cutoff, we define the binding-pocket residues as those forming contacts with ligands in at least 10 out of 132 ligand-bound structures. As a result, we found a total of 55 residues forming the binding pocket. For comparison, a slightly more relaxed, alternative cutoff of 8 hits yields a total of 56 residues.
As shown in Figure 3A, the total number of ligands
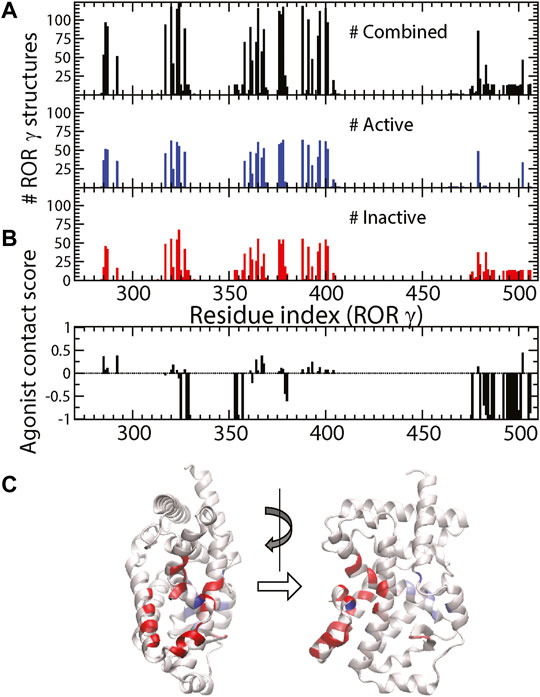
FIGURE 3. (A) The total number of ligand-protein contacts made by a specific residue (black), the number of ligand-protein contacts in active and inactive structures (blue and red). (B) The agonist contact score is displayed as a function of residue. (C) The corresponding values from (B) are color-labeled onto the 3D structure of RORγ (front and side views).
It might be useful to point out that the residues with strongest negative agonist contact scores (highlighted in red in Figure 3C) are located at five specific spots (near residues 325, 328–329, 353–354, 357, and 379–380) and the C-terminus (476–506). Note that three of those spots belong to the allosteric binding pocket and the other two spots (residues 357 and 379–380) reside in the boundary between the allosteric and canonical binding pockets. These inverse agonist “hot spots” can be important for ligand design and some of them have been reported as the “trigger” for inducing inactive conformations of RORγ. For example, one of the most potent inverse agonists from isoxazole family was reported to have contact interaction with Q329, L353, and K354 (Meijer et al., 2020). Another example on the boundary is the M358 trigger (Marcotte et al., 2016) mentioned in the Introduction. Combined with molecular docking (Trott and Olson, 2010), this contact score (Figure 3B) can be further developed and applied to high throughput screening for selecting new inverse agonist ligands for RORγ. This statistical approach may also be generalized to study ligand recognition by other receptors.
Besides obtaining independent statistics on the ligand contact tendency of each residue, we further investigated the concerted pattern of the residue-ligand contacts, i.e., whether residues i and j form protein-ligand contacts in sync. Various statistical analyses can be used to achieve this correlation analysis, and we use contact PCA as described in the Method section. The contact PCA on the covariance matrix of residue-ligand contacts provides the dominant patterns of residue-ligand interaction. The top eigenvectors PC1 and PC2 were presented in Figures 4A,B. We also analyzed the PC projection for PC1 and PC2, which is shown in Figure 4C. Each PC mode indicates a specific binding pattern: all residues with positive values form contacts with the ligand (i.e., not necessarily the same ligand) in sync and the same goes for residues with all negative values. Additionally, there is an anti-correlation between positive residues and negative residues. One can see that the dominant mode, PC1, largely divides residues into two groups. As ligand contacts from conformations of PDB structures (4YPQ, 5C4O, 5C4S, 5C4T, 5C4U, 5LWP, 6SAL, 6TLM, and 6UCG) mostly come from the positive group, they show up as positive PC projections, whereas the remaining conformations comprise the negative group. Overall, we found that PC1 distinguishes two binding modes: allosteric binding for the positive group and canonical binding for the negative one. The position of the allosteric binding pocket is distal to the traditional canonical binding pocket, and the ligands that interact with the allosteric binding pocket have been found to be a class of inverse agonists (Meijer et al., 2020; Vries et al., 2020; Zhang et al., 2020; de Vries et al., 2021; Meijer et al., 2021). Function-wise, these allosteric inverse agonists induce another orientation of helix H12 such that it prevents the binding of a coactivator. The second dominant interaction pattern, PC2, shows another prominent binding feature, which seems to weakly separate agonist vs. inverse agonist binding. It is interesting to point out that most conformations with an extreme positive PC2 projection are inactive conformations (red) and vice versa, an extreme negative PC2 for active conformations (black).
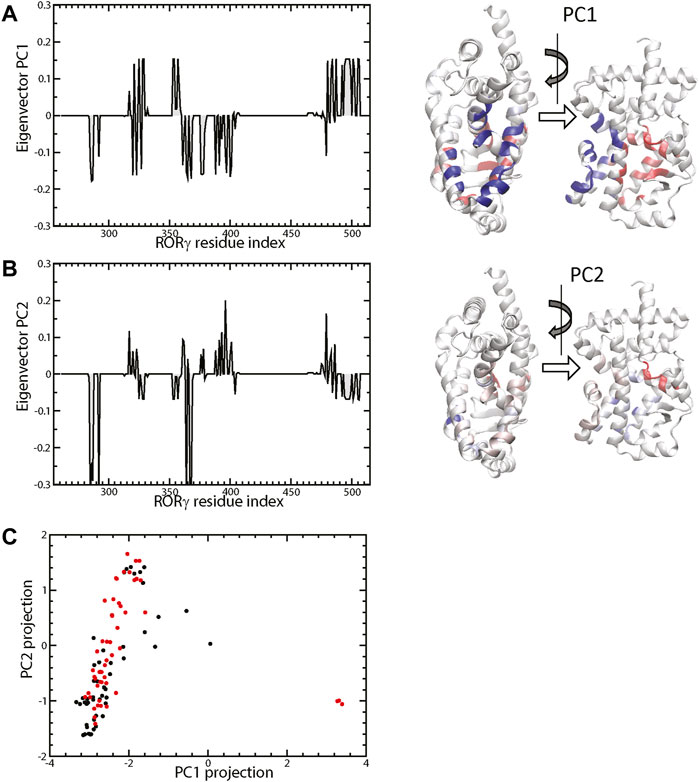
FIGURE 4. The top two eigenvectors PC1 and PC2 are shown in (A,B), respectively. The 3D representations are colored by the elements of the corresponding eigenvectors (blue+ and red−). The projection of protein-ligand interaction from the top two PCs of the 132 structures (active labeled in black and inactive in red) is shown in (C).
Essential Contacts for Constitutive Activity Revealed by Molecular Dynamics Simulation
As mentioned in the Introduction, the high basal activity of certain NRs makes finding the mechanism of constitutive activity and inhibitors to these NRs important. Since the apo structure of NRs in general is difficult to obtain and most existing NR structures are complexed with ligand(s), we use computer simulation to sample the apo structural ensemble. Furthermore, we use statistics of residue-residue contacts to characterize the mechanism of high basal activity at the residue-residue interaction resolution.
We performed a 100-ns simulation on the unliganded RORγ and analyzed the snapshots using contact analysis, which focuses on residue-residue contact interaction during the simulation. We then examined the contact interactions within the RORγ receptor and focused on identifying contacts with high interaction strength in the apo ensemble. The mean contact matrix of apo RORγ is shown in Figure 5A (upper triangle). Each element of the mean contact matrix (also termed contact frequency) is displayed on a contact map using spectrum color-labeling, ranging from rarely formed with contact ratio at 0.1–0.2 (red) to nearly always formed at 0.9–1.0 (dark gray), which largely reflects the contact interaction strength during the simulation.
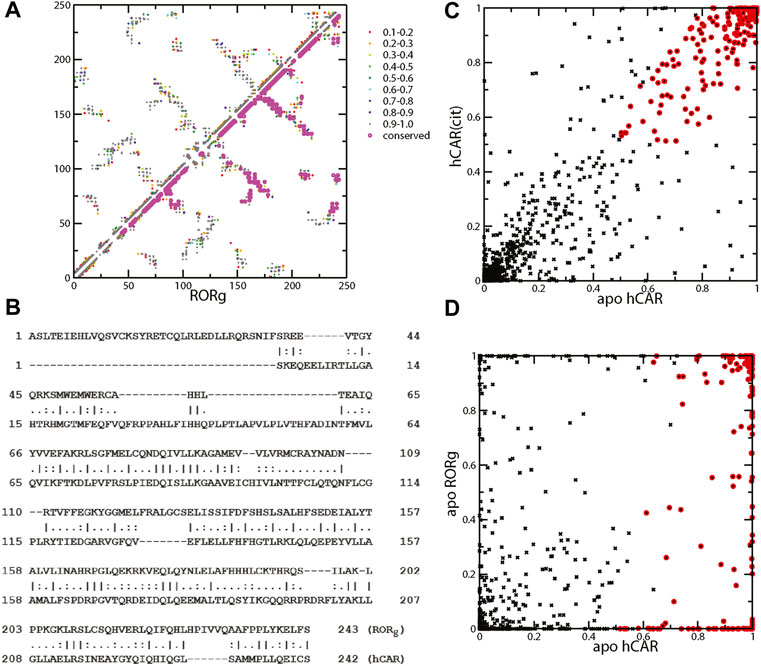
FIGURE 5. (A) Mean contact interaction of unliganded RORγ during simulation (upper-left triangle). The contact strength values are color-coded, and the conserved contacts are highlighted in magenta. (B) Sequence alignment between RORγ (UniProt: P51449) and hCAR (UniProt: Q14994) for a direct comparison between contacts. The listed index can be converted to the standard index by +264 and +106 for RORγ and hCAR, respectively, i.e., the first Ala is A265 for RORγ and the first Ser is S107 for hCAR. (C) The scatter plot of contact interaction between unliganded hCAR and hCAR with the agonist CITCO. The conserved contacts are labeled using red circles. (D) The scatter plot of contact interaction between unliganded hCAR and unliganded RORγ.
There are many ways of selecting essential contact interactions that are responsible for high basal activity. Here, we focus on two aspects of conserved highly-formed contact interactions. One aspect is the conservation across different nuclear receptors and the other is the conservation between ligand-bound and apo forms. Thus, we emphasize that the essential contacts are the contacts that not only consistently show up regardless of the ligand binding status but also persist across different NRs. To address how constitutive activity can be conserved across nuclear receptors, we compare the essential contact interactions of RORγ with those of a prominent constitutively active receptor, CAR. The LBDs of both receptors are similar in size and structure. The LBD of RORγ contains 243 residues compared to 242 for CAR. Structure-wise, these two LBDs share a similar fold and both display a short helix, HX, which is unique among the LBDs of nuclear receptors. The presence of helix HX in CAR has been suggested to stabilize the active conformation of the apo form leading to the constitutive activity of CAR (Pham et al., 2019a). The sequence alignment on RORγ and CAR shows a good alignment and conserved residues in Figure 5B, especially after the first 50 residues. The sequences of the two receptors share 59 identical residues (∼20%). This alignment facilitates our comparison of residues between RORγ and CAR and the comparison of residue-residue contact interactions between the two receptors.
Before we locate the conserved contacts between NRs, we first identify the contacts that are conserved between apo and agonist ligand-bound forms. Since we only performed the apo RORγ simulation and we have previously obtained both ligand-bound and apo simulations for CAR, we use the CAR system to select the contacts between apo and agonist ligand-bound forms. Specifically, we use an ad hoc selection criterion of contact formation that is larger than 50% for both forms to identify the conserved contacts between apo and ligand-bound forms, and these conserved contacts are annotated with red circles as seen in Figure 5C. Furthermore, to locate the essential contacts that are conserved between different receptors, we directly compared the contact interactions of RORγ with the CAR receptor, as shown in Figure 5D. Again, the red circles are annotated for the conserved contacts selected (based on the high contact conservation between apo and ligand-bound) from Figure 5C. Finally, a subset of annotated contacts, which are the conserved contacts across NRs (defined as larger than 50% for both apo forms), is highlighted in magenta in Figure 5A (lower triangle). The conservation between CAR and RORγ contacts is quite extensive especially at the C-terminal half of the LBD, which leads to the conclusion that the mechanism of constitutive activity is similar between them. It may be of interest to investigate whether we can apply the inverse agonist ligand design of ROR to another system, e.g., to explore a potential allosteric binding site of CAR.
Based on the mean contact strength (Figure 5A) and the sequence alignment (Figure 5B), we found that the contacts between helices H11 and H12 are preserved for the two apo receptors. Specifically, the contact pairs H479-Y502 (H11–H12) and Y502-F506 (H12) of RORγ have a high contact strength and they are similar to Y326-L343 and L343-C347 in CAR. These three residues H479-Y502-F506 are collectively known as the HYF triplet, which forms a contact interaction network that is important for RORγ activity (Li et al., 2017; Ma et al., 2021). For example, inverse agonist may function by interacting with residue M358 and further disrupting contact interaction involving F506 (Marcotte et al., 2016). Previously, Y326-L343 in CAR was found to be critical to the agonist activity in the active conformation for CAR. In both CAR and RORγ, the His-Tyr lock stabilizes the position of helix H12 and contributes to the formation of the AF2 region. The disruption of H479-Y502 (H11–H12) through mutagenesis can prevent the coactivator from binding, thus reducing RORγ transcriptional activity (Kurebayashi et al., 2004). This is also supported by a high number of ligands forming contacts with both residues His479 and Tyr502 in the active conformation of RORγ in Figure 3A. In a previous study, the equivalent contact to His479-Tyr502 in CAR (Tyr326-Leu343) has been shown to be present in the apo conformation and strengthened by the binding of an agonist ligand (Pham et al., 2019a). Analogous to CAR, the His-Tyr lock is also present in our apo RORγ simulation with the average contact strength of 96.2%.
Concluding Remarks
We studied the existing crystal structures of nuclear receptor RORγ, where various ligands (100+) interact with the binding pocket differently and result in an active or inactive conformation. By characterizing the protein conformation and protein-ligand interaction using residue contact interactions, we further performed a statistical analysis on these contact patterns. We identified the important residues at the binding pocket(s) that may be essential for interacting with potential inverse agonists. Besides studying the experimental data on a protein-ligand complex, we also used simulation to examine the apo structure ensemble and compared the high basal activity between RORγ and CAR. We found that the mechanism of constitutive activity is highly similar between them. These efforts lead to the understanding of the structure ensemble and protein-ligand interaction from a contact viewpoint, and they may facilitate future designs of inverse agonists for nuclear receptors.
Data Availability Statement
The original contributions presented in the study are included in the article/Supplementary Material, further inquiries can be directed to the corresponding author.
Author Contributions
BP, RL, DF, and TS conceptualized and designed the research. All authors involved extracting data from PDB files and calculated contact interactions. BP set up the computer simulation and performed computer simulation. BP and TS performed simulation data analysis and wrote the initial draft. All authors participated in the revision.
Funding
This work was also supported in parts by NIH R15 GM123469.
Conflict of Interest
The authors declare that the research was conducted in the absence of any commercial or financial relationships that could be construed as a potential conflict of interest.
Publisher’s Note
All claims expressed in this article are solely those of the authors and do not necessarily represent those of their affiliated organizations, or those of the publisher, the editors and the reviewers. Any product that may be evaluated in this article, or claim that may be made by its manufacturer, is not guaranteed or endorsed by the publisher.
Acknowledgments
We thank Dr. E. J. Fernandez for the helpful discussion on NR constitutive activity. We also acknowledge the computational support provided by the allocations of advanced computing resources XSEDE (STAMPEDE2 at TACC) on the simulation of unliganded ROR.
Supplementary Material
The Supplementary Material for this article can be found online at: https://www.frontiersin.org/articles/10.3389/fmolb.2022.904445/full#supplementary-material
References
Amaudrut, J., Argiriadi, M. A., Barth, M., Breinlinger, E. C., Bressac, D., Broqua, P., et al. (2019). Discovery of Novel Quinoline Sulphonamide Derivatives as Potent, Selective and Orally Active RORγ Inverse Agonists. Bioorg. Med. Chem. Lett. 29, 1799–1806. doi:10.1016/j.bmcl.2019.05.015
Anandakrishnan, R., Aguilar, B., and Onufriev, A. V. (2012). H++ 3.0: Automating pK Prediction and the Preparation of Biomolecular Structures for Atomistic Molecular Modeling and Simulations. Nucleic Acids Res. 40, W537–W541. doi:10.1093/nar/gks375
Brunton, S. L., and Kutz, J. N. (2019). Data-Driven Science and Engineering: Machine Learning, Dynamical Systems, and Control. Cambridge: Cambridge University Press.
Carcache, D. A., Vulpetti, A., Kallen, J., Mattes, H., Orain, D., Stringer, R., et al. (2018). Optimizing a Weakly Binding Fragment into a Potent RORγt Inverse Agonist with Efficacy in an In Vivo Inflammation Model. J. Med. Chem. 61, 6724–6735. doi:10.1021/acs.jmedchem.8b00529
Chao, J., Enyedy, I., Van Vloten, K., Marcotte, D., Guertin, K., Hutchings, R., et al. (2015). Discovery of Biaryl Carboxylamides as Potent RORγ Inverse Agonists. Bioorg. Med. Chem. Lett. 25, 2991–2997. doi:10.1016/j.bmcl.2015.05.026
Cherney, R. J., Cornelius, L. A. M., Srivastava, A., Weigelt, C. A., Marcoux, D., Duan, J. J.-W., et al. (2020). Discovery of BMS-986251: A Clinically Viable, Potent, and Selective RORγt Inverse Agonist. ACS Med. Chem. Lett. 11, 1221–1227. doi:10.1021/acsmedchemlett.0c00063
Clark, A. K., Wilder, J. H., Grayson, A. W., Johnson, Q. R., Lindsay, R. J., Nellas, R. B., et al. (2016). The Promiscuity of Allosteric Regulation of Nuclear Receptors by Retinoid X Receptor. J. Phys. Chem. B 120, 8338–8345. doi:10.1021/acs.jpcb.6b02057
Cook, D. N., Kang, H. S., and Jetten, A. M. (2015). Retinoic Acid-Related Orphan Receptors (RORs): Regulatory Functions in Immunity, Development, Circadian Rhythm, and Metabolism. Nucl. Recept. Res. 2, 101185. doi:10.11131/2015/101185
Das, P., Golloshi, R., McCord, R. P., and Shen, T. (2020). Using Contact Statistics to Characterize Structure Transformation of Biopolymer Ensembles. Phys. Rev. E 101, 012419. doi:10.1103/PhysRevE.101.012419
de Vries, R. M. J. M., Meijer, F. A., Doveston, R. G., Leijten-van de Gevel, I. A., and Brunsveld, L. (2021). Cooperativity Between the Orthosteric and Allosteric Ligand Binding Sites of RORγt. Proc. Natl. Acad. Sci. 118, e2021287118. doi:10.1073/pnas.2021287118
Duan, J. J.-W., Jiang, B., Lu, Z., Stachura, S., Weigelt, C. A., Sack, J. S., et al. (2020). Discovery of 2,6-difluorobenzyl Ether Series of Phenyl ((R)-3-phenylpyrrolidin-3-yl)sulfones as Surprisingly Potent, Selective and Orally Bioavailable RORγt Inverse Agonists. Bioorg. Med. Chem. Lett. 30, 127441. doi:10.1016/j.bmcl.2020.127441
Duan, J. J.-W., Lu, Z., Jiang, B., Stachura, S., Weigelt, C. A., Sack, J. S., et al. (2019). Structure-based Discovery of Phenyl (3-Phenylpyrrolidin-3-Yl) Sulfones as Selective, Orally Active RORγt Inverse Agonists. ACS Med. Chem. Lett. 10, 367–373. doi:10.1021/acsmedchemlett.9b00010
Dussault, I., Lin, M., Hollister, K., Fan, M., Termini, J., Sherman, M. A., et al. (2002). A Structural Model of the Constitutive Androstane Receptor Defines Novel Interactions that Mediate Ligand-independent Activity. Mol. Cell Biol. 22, 5270–5280. doi:10.1128/mcb.22.15.5270-5280.2002
Enyedy, I. J., Powell, N. A., Caravella, J., van Vloten, K., Chao, J., Banerjee, D., et al. (2016). Discovery of Biaryls as RORγ Inverse Agonists by Using Structure-Based Design. Bioorg. Med. Chem. Lett. 26, 2459–2463. doi:10.1016/j.bmcl.2016.03.109
Fauber, B. P., de Leon Boenig, G., Burton, B., Eidenschenk, C., Everett, C., Gobbi, A., et al. (2013). Structure-based Design of Substituted Hexafluoroisopropanol-Arylsulfonamides as Modulators of RORc. Bioorg. Med. Chem. Lett. 23, 6604–6609. doi:10.1016/j.bmcl.2013.10.054
Fauber, B. P., René, O., de Leon Boenig, G., Burton, B., Deng, Y., Eidenschenk, C., et al. (2014). Reduction in Lipophilicity Improved the Solubility, Plasma-Protein Binding, and Permeability of Tertiary Sulfonamide RORc Inverse Agonists. Bioorg. Med. Chem. Lett. 24, 3891–3897. doi:10.1016/j.bmcl.2014.06.048
Fujita-Sato, S., Ito, S., Isobe, T., Ohyama, T., Wakabayashi, K., Morishita, K., et al. (2011). Structural Basis of Digoxin that Antagonizes RORγt Receptor Activity and Suppresses Th17 Cell Differentiation and Interleukin (IL)-17 Production. J. Biol. Chem. 286, 31409–31417. doi:10.1074/jbc.m111.254003
Fukase, Y., Sato, A., Tomata, Y., Ochida, A., Kono, M., Yonemori, K., et al. (2018). Identification of Novel Quinazolinedione Derivatives as RORγt Inverse Agonist. Bioorg. Med. Chem. 26, 721–736. doi:10.1016/j.bmc.2017.12.039
Gege, C., Albers, M., Kinzel, O., Kleymann, G., Schlüter, T., Steeneck, C., et al. (2020). Optimization and Biological Evaluation of Thiazole-Bis-Amide Inverse Agonists of RORγt. Bioorg. Med. Chem. Lett. 30, 127205. doi:10.1016/j.bmcl.2020.127205
Gege, C., Cummings, M. D., Albers, M., Kinzel, O., Kleymann, G., Schlüter, T., et al. (2018). Identification and Biological Evaluation of Thiazole-Based Inverse Agonists of RORγt. Bioorg. Med. Chem. Lett. 28, 1446–1455. doi:10.1016/j.bmcl.2018.03.093
Gong, H., Weinstein, D. S., Lu, Z., Duan, J. J.-W., Stachura, S., Haque, L., et al. (2018). Identification of Bicyclic Hexafluoroisopropyl Alcohol Sulfonamides as Retinoic Acid Receptor-Related Orphan Receptor Gamma (RORγ/RORc) Inverse Agonists. Employing Structure-Based Drug Design to Improve Pregnane X Receptor (PXR) Selectivity. Bioorg. Med. Chem. Lett. 28, 85–93. doi:10.1016/j.bmcl.2017.12.006
Harikrishnan, L. S., Gill, P., Kamau, M. G., Qin, L.-Y., Ruan, Z., O'Malley, D., et al. (2020). Substituted Benzyloxytricyclic Compounds as Retinoic Acid-Related Orphan Receptor Gamma T (RORγt) Agonists. Bioorg. Med. Chem. Lett. 30, 127204. doi:10.1016/j.bmcl.2020.127204
Helsen, C., Kerkhofs, S., Clinckemalie, L., Spans, L., Laurent, M., Boonen, S., et al. (2012). Structural Basis for Nuclear Hormone Receptor DNA Binding. Mol. Cell. Endocrinol. 348, 411–417. doi:10.1016/j.mce.2011.07.025
Hintermann, S., Guntermann, C., Mattes, H., Carcache, D. A., Wagner, J., Vulpetti, A., et al. (2016). Synthesis and Biological Evaluation of New Triazolo- and Imidazolopyridine RORγt Inverse Agonists. ChemMedChem 11, 2640–2648. doi:10.1002/cmdc.201600500
Hirata, K., Kotoku, M., Seki, N., Maeba, T., Maeda, K., Hirashima, S., et al. (2016). SAR Exploration Guided by LE and Fsp3: Discovery of a Selective and Orally Efficacious RORγ Inhibitor. ACS Med. Chem. Lett. 7, 23–27. doi:10.1021/acsmedchemlett.5b00253
Hoegenauer, K., Kallen, J., Jiménez-Núñez, E., Strang, R., Ertl, P., Cooke, N. G., et al. (2019). Structure-Based and Property-Driven Optimization of N-Aryl Imidazoles toward Potent and Selective Oral RORγt Inhibitors. J. Med. Chem. 62, 10816–10832. doi:10.1021/acs.jmedchem.9b01291
Huss, J. M., Garbacz, W. G., and Xie, W. (2015). Constitutive Activities of Estrogen-Related Receptors: Transcriptional Regulation of Metabolism by the ERR Pathways in Health and Disease. Biochimica Biophysica Acta (BBA) - Mol. Basis Dis. 1852, 1912–1927. doi:10.1016/j.bbadis.2015.06.016
Jiang, B., Duan, J. J.-W., Stachura, S., Karmakar, A., Hemagiri, H., Raut, D. K., et al. (2020). Discovery of (3S,4S)-3-Methyl-3-(4-Fluorophenyl)-4-(4-(1,1,1,3,3,3-Hexafluoro-2-Hydroxyprop-2-Yl)phenyl) Pyrrolidines as Novel RORγt Inverse Agonists. Bioorg. Med. Chem. Lett. 30, 127392. doi:10.1016/j.bmcl.2020.127392
Jin, L., Martynowski, D., Zheng, S., Wada, T., Xie, W., and Li, Y. (2010). Structural Basis for Hydroxycholesterols as Natural Ligands of Orphan Nuclear Receptor RORγ. Mol. Endocrinol. 24, 923–929. doi:10.1210/me.2009-0507
Johnson, Q. R., Lindsay, R. J., Nellas, R. B., Fernandez, E. J., and Shen, T. (2015). Mapping Allostery through Computational Glycine Scanning and Correlation Analysis of Residue-Residue Contacts. Biochemistry 54, 1534–1541. doi:10.1021/bi501152d
Johnson, Q. R., Lindsay, R. J., and Shen, T. (2018). CAMERRA: An Analysis Tool for the Computation of Conformational Dynamics by Evaluating Residue-Residue Associations. J. Comput. Chem. 39, 1568–1578. doi:10.1002/jcc.25192
Kallen, J., Izaac, A., Be, C., Arista, L., Orain, D., Kaupmann, K., et al. (2017). Structural States of RORγt: X-Ray Elucidation of Molecular Mechanisms and Binding Interactions for Natural and Synthetic Compounds. ChemMedChem 12, 1014–1021. doi:10.1002/cmdc.201700278
Kono, M., Ochida, A., Oda, T., Imada, T., Banno, Y., Taya, N., et al. (2018). Discovery of [cis-3-({(5r)-5-[(7-Fluoro-1,1-Dimethyl-2,3-Dihydro-1h-Inden-5-Yl)carbamoyl]-2-Methoxy-7,8-Dihydro-1,6-Naphthyridin-6(5h)-Yl} Carbonyl)cyclobutyl] Acetic Acid (TAK-828F) as a Potent, Selective, and Orally Available Novel Retinoic Acid Receptor-Related Orphan Receptor γt Inverse Agonist. J. Med. Chem. 61, 2973–2988. doi:10.1021/acs.jmedchem.8b00061
Kotoku, M., Maeba, T., Fujioka, S., Yokota, M., Seki, N., Ito, K., et al. (2019). Discovery of Second Generation RORγ Inhibitors Composed of an Azole Scaffold. J. Med. Chem. 62, 2837–2842. doi:10.1021/acs.jmedchem.8b01567
Kumar, R., and Thompson, E. B. (2003). Transactivation Functions of the N-Terminal Domains of Nuclear Hormone Receptors: Protein Folding and Coactivator Interactions. Mol. Endocrinol. 17, 1–10. doi:10.1210/me.2002-0258
Kummer, D. A., Cummings, M. D., Abad, M., Barbay, J., Castro, G., Wolin, R., et al. (2017). Identification and Structure Activity Relationships of Quinoline Tertiary Alcohol Modulators of RORγt. Bioorg. Med. Chem. Lett. 27, 2047–2057. doi:10.1016/j.bmcl.2017.02.044
Kurebayashi, S., Nakajima, T., Kim, S.-C., Chang, C.-Y., McDonnell, D. P., Renaud, J.-P., et al. (2004). Selective LXXLL Peptides Antagonize Transcriptional Activation by the Retinoid-Related Orphan Receptor RORγ. Biochem. Biophysical Res. Commun. 315, 919–927. doi:10.1016/j.bbrc.2004.01.131
Li, X., Anderson, M., Collin, D., Muegge, I., Wan, J., Brennan, D., et al. (2017). Structural Studies Unravel the Active Conformation of Apo RORγt Nuclear Receptor and a Common Inverse Agonism of Two Diverse Classes of RORγt Inhibitors. J. Biol. Chem. 292, 11618–11630. doi:10.1074/jbc.m117.789024
Lindsay, R. J., Mansbach, R. A., Gnanakaran, S., and Shen, T. (2021). Effects of pH on an IDP Conformational Ensemble Explored by Molecular Dynamics Simulation. Biophys. Chem. 271, 106552. doi:10.1016/j.bpc.2021.106552
Lindsay, R. J., Pham, B., Shen, T., and McCord, R. P. (2018). Characterizing the 3D Structure and Dynamics of Chromosomes and Proteins in a Common Contact Matrix Framework. Nucleic Acids Res. 46, 8143–8152. doi:10.1093/nar/gky604
Liu, Q., Batt, D. G., Weigelt, C. A., Yip, S., Wu, D.-R., Ruzanov, M., et al. (2020). Novel Tricyclic Pyroglutamide Derivatives as Potent RORγt Inverse Agonists Identified Using a Virtual Screening Approach. ACS Med. Chem. Lett. 11, 2510–2518. doi:10.1021/acsmedchemlett.0c00496
Lu, Z., Duan, J. J.-W., Xiao, H., Neels, J., Wu, D.-R., Weigelt, C. A., et al. (2019). Identification of Potent, Selective and Orally Bioavailable Phenyl ((R)-3-phenylpyrrolidin-3-yl) Sulfone Analogues as RORγt Inverse Agonists. Bioorg. Med. Chem. Lett. 29, 2265–2269. doi:10.1016/j.bmcl.2019.06.036
Lugar, C. W., Clarke, C. A., Morphy, R., Rudyk, H., Sapmaz, S., Stites, R. E., et al. (2021). Defining Target Engagement Required for Efficacy In Vivo at the Retinoic Acid Receptor-Related Orphan Receptor C2 (RORγt). J. Med. Chem. 64, 5470–5484. doi:10.1021/acs.jmedchem.0c01918
Ma, X., Sun, N., Li, X., and Fu, W. (2021). Discovery of Novel N-Sulfonamide-Tetrahydroisoquinolines as Potent Retinoic Acid Receptor-Related Orphan Receptor γt Agonists. Eur. J. Med. Chem. 222, 113585. doi:10.1016/j.ejmech.2021.113585
Marcotte, D. J., Liu, Y., Little, K., Jones, J. H., Powell, N. A., Wildes, C. P., et al. (2016). Structural Determinant for Inducing RORgamma Specific Inverse Agonism Triggered by a Synthetic Benzoxazinone Ligand. BMC Struct. Biol. 16, 7. doi:10.1186/s12900-016-0059-3
Marcoux, D., Duan, J. J.-W., Shi, Q., Cherney, R. J., Srivastava, A. S., Cornelius, L., et al. (2019). Rationally Designed, Conformationally Constrained Inverse Agonists of RORγt-Identification of a Potent, Selective Series with Biologic-like In Vivo Efficacy. J. Med. Chem. 62, 9931–9946. doi:10.1021/acs.jmedchem.9b01369
Meijer, F. A., Doveston, R. G., de Vries, R. M. J. M., Vos, G. M., Vos, A. A. A., Leysen, S., et al. (2020). Ligand-Based Design of Allosteric Retinoic Acid Receptor-Related Orphan Receptor γt (RORγt) Inverse Agonists. J. Med. Chem. 63, 241–259. doi:10.1021/acs.jmedchem.9b01372
Meijer, F. A., Saris, A. O. W. M., Doveston, R. G., Oerlemans, G. J. M., de Vries, R. M. J. M., Somsen, B. A., et al. (2021). Structure-Activity Relationship Studies of Trisubstituted Isoxazoles as Selective Allosteric Ligands for the Retinoic-Acid-Receptor-Related Orphan Receptor γt. J. Med. Chem. 64, 9238–9258. doi:10.1021/acs.jmedchem.1c00475
Muegge, I., Collin, D., Cook, B., Hill-Drzewi, M., Horan, J., Kugler, S., et al. (2015). Discovery of 1,3-Dihydro-2,1,3-Benzothiadiazole 2,2-Dioxide Analogs as New RORC Modulators. Bioorg. Med. Chem. Lett. 25, 1892–1895. doi:10.1016/j.bmcl.2015.03.042
Nakajima, R., Oono, H., Kumazawa, K., Ida, T., Hirata, J., White, R. D., et al. (2021). Discovery of 6-Oxo-4-Phenyl-Hexanoic Acid Derivatives as RORγt Inverse Agonists Showing Favorable ADME Profile. Bioorg. Med. Chem. Lett. 36, 127786. doi:10.1016/j.bmcl.2021.127786
Nakajima, R., Oono, H., Sugiyama, S., Matsueda, Y., Ida, T., Kakuda, S., et al. (2020). Discovery of [1,2,4] Triazolo[1,5-A] Pyridine Derivatives as Potent and Orally Bioavailable RORγt Inverse Agonists. ACS Med. Chem. Lett. 11, 528–534. doi:10.1021/acsmedchemlett.9b00649
Narjes, F., Xue, Y., von Berg, S., Malmberg, J., Llinas, A., Olsson, R. I., et al. (2018). Potent and Orally Bioavailable Inverse Agonists of RORγt Resulting from Structure-Based Design. J. Med. Chem. 61, 7796–7813. doi:10.1021/acs.jmedchem.8b00783
Noguchi, M., Nomura, A., Doi, S., Yamaguchi, K., Hirata, K., Shiozaki, M., et al. (2018). Ternary Crystal Structure of Human RORγ Ligand-Binding-Domain, an Inhibitor and Corepressor Peptide Provides a New Insight into Corepressor Interaction. Sci. Rep. 8, 17374. doi:10.1038/s41598-018-35783-9
Noguchi, M., Nomura, A., Murase, K., Doi, S., Yamaguchi, K., Hirata, K., et al. (2017). Ternary Complex of Human RORγ Ligand-Binding Domain, Inverse Agonist and SMRT Peptide Shows a Unique Mechanism of Corepressor Recruitment. Genes cells. 22, 535–551. doi:10.1111/gtc.12494
Olsson, R. I., Xue, Y., von Berg, S., Aagaard, A., McPheat, J., Hansson, E. L., et al. (2016). Benzoxazepines Achieve Potent Suppression of IL-17 Release in Human T-Helper 17 (TH17) Cells Through an Induced-Fit Binding Mode to the Nuclear Receptor RORγ. ChemMedChem 11, 207–216. doi:10.1002/cmdc.201500432
Ouvry, G., Bouix-Peter, C., Ciesielski, F., Chantalat, L., Christin, O., Comino, C., et al. (2016). Discovery of Phenoxyindazoles and Phenylthioindazoles as RORγ Inverse Agonists. Bioorg. Med. Chem. Lett. 26, 5802–5808. doi:10.1016/j.bmcl.2016.10.023
Pham, B., Arons, A. B., Vincent, J. G., Fernandez, E. J., and Shen, T. (2019). Regulatory Mechanics of Constitutive Androstane Receptors: Basal and Ligand-Directed Actions. J. Chem. Inf. Model. 59, 5174–5182. doi:10.1021/acs.jcim.9b00695
Pham, B., Lindsay, R. J., and Shen, T. (2019). Effector-Binding-Directed Dimerization and Dynamic Communication Between Allosteric Sites of Ribonucleotide Reductase. Biochemistry 58, 697–705. doi:10.1021/acs.biochem.8b01131
René, O., Fauber, B. P., Boenig, G. L., Burton, B., Eidenschenk, C., Everett, C., et al. (2015). Minor Structural Change to Tertiary Sulfonamide RORc Ligands Led to Opposite Mechanisms of Action. ACS Med. Chem. Lett. 6, 276–281. doi:10.1021/ml500420y
Rosenberg, E. M., Harrison, R. E. S., Tsou, L. K., Drucker, N., Humphries, B., Rajasekaran, D., et al. (2019). Characterization, Dynamics, and Mechanism of CXCR4 Antagonists on a Constitutively Active Mutant. Cell Chem. Biol. 26, 662–673. doi:10.1016/j.chembiol.2019.01.012
Ruan, Z., Park, P. K., Wei, D., Purandare, A., Wan, H., O'Malley, D., et al. (2021). Substituted Diaryl Ether Compounds as Retinoic Acid-Related Orphan Receptor-Γt (RORγt) Agonists. Bioorg. Med. Chem. Lett. 35, 127778. doi:10.1016/j.bmcl.2021.127778
Santori, F. R., Huang, P., van de Pavert, S. A., Douglass, E. F., Leaver, D. J., Haubrich, B. A., et al. (2015). Identification of Natural RORγ Ligands that Regulate the Development of Lymphoid Cells. Cell metab. 21, 286–298. doi:10.1016/j.cmet.2015.01.004
Sasaki, Y., Odan, M., Yamamoto, S., Kida, S., Ueyama, A., Shimizu, M., et al. (2018). Discovery of a Potent Orally Bioavailable Retinoic Acid Receptor-Related Orphan Receptor-Gamma-T (RORγt) Inhibitor, S18-000003. Bioorg. Med. Chem. Lett. 28, 3549–3553. doi:10.1016/j.bmcl.2018.09.032
Sato, A., Fukase, Y., Kono, M., Ochida, A., Oda, T., Sasaki, Y., et al. (2019). Design and Synthesis of Conformationally Constrained RORγt Inverse Agonists. ChemMedChem 14, 1917–1932. doi:10.1002/cmdc.201900416
Scheepstra, M., Leysen, S., van Almen, G. C., Miller, J. R., Piesvaux, J., Kutilek, V., et al. (2015). Identification of an Allosteric Binding Site for RORγt Inhibition. Nat. Commun. 6, 8833. doi:10.1038/ncomms9833
Schimmer, B. P., and White, P. C. (2010). Minireview: Steroidogenic Factor 1: Its Roles in Differentiation, Development, and Disease. Mol. Endocrinol. 24, 1322–1337. doi:10.1210/me.2009-0519
Schnute, M. E., Wennerstål, M., Alley, J., Bengtsson, M., Blinn, J. R., Bolten, C. W., et al. (2018). Discovery of 3-Cyano- N-(3-(1-Isobutyrylpiperidin-4-yl)-1-methyl-4-(Trifluoromethyl)-1 H-Pyrrolo[2,3- B]pyridin-5-Yl) Benzamide: A Potent, Selective, and Orally Bioavailable Retinoic Acid Receptor-Related Orphan Receptor C2 Inverse Agonist. J. Med. Chem. 61, 10415–10439. doi:10.1021/acs.jmedchem.8b00392
Shi, Q., Xiao, Z., Yang, M. G., Marcoux, D., Cherney, R. J., Yip, S., et al. (2020). Tricyclic Sulfones as Potent, Selective and Efficacious RORγt Inverse Agonists - Exploring C6 and C8 SAR Using Late-Stage Functionalization. Bioorg. Med. Chem. Lett. 30, 127521. doi:10.1016/j.bmcl.2020.127521
Shirai, J., Tomata, Y., Kono, M., Ochida, A., Fukase, Y., Sato, A., et al. (2018). Discovery of Orally Efficacious RORγt Inverse Agonists, Part 1: Identification of Novel Phenylglycinamides as Lead Scaffolds. Bioorg. Med. Chem. 26, 483–500. doi:10.1016/j.bmc.2017.12.006
Simons, S. S., Edwards, D. P., and Kumar, R. (2014). Minireview: Dynamic Structures of Nuclear Hormone Receptors: New Promises and Challenges. Mol. Endocrinol. 28, 173–182. doi:10.1210/me.2013-1334
Solt, L. A., and Burris, T. P. (2012). Action of RORs and Their Ligands in (Patho)physiology. Trends Endocrinol. Metabolism 23, 619–627. doi:10.1016/j.tem.2012.05.012
Strutzenberg, T. S., Garcia-Ordonez, R. D., Novick, S. J., Park, H., Chang, M. R., Doebellin, C., et al. (2019). HDX-MS Reveals Structural Determinants for RORγ Hyperactivation by Synthetic Agonists. eLife 8, 47172. doi:10.7554/eLife.47172
Takeda, Y., Jothi, R., Birault, V., and Jetten, A. M. (2012). RORγ Directly Regulates the Circadian Expression of Clock Genes and Downstream Targets In Vivo. Nucleic Acids Res. 40, 8519–8535. doi:10.1093/nar/gks630
Tanis, V. M., Venkatesan, H., Cummings, M. D., Albers, M., Kent Barbay, J., Herman, K., et al. (2019). 3-Substituted Quinolines as RORγt Inverse Agonists. Bioorg. Med. Chem. Lett. 29, 1463–1470. doi:10.1016/j.bmcl.2019.04.021
Trott, O., and Olson, A. J. (2010). Auto Dock Vina: Improving the Speed and Accuracy of Docking with a New Scoring Function, Efficient Optimization, and Multithreading. J. Comput. Chem. 31, 455–461. doi:10.1002/jcc.21334
van Niel, M. B., Fauber, B. P., Cartwright, M., Gaines, S., Killen, J. C., René, O., et al. (2014). A Reversed Sulfonamide Series of Selective RORc Inverse Agonists. Bioorg. Med. Chem. Lett. 24, 5769–5776. doi:10.1016/j.bmcl.2014.10.037
von Berg, S., Xue, Y., Collins, M., Llinas, A., Olsson, R. I., Halvarsson, T., et al. (2019). Discovery of Potent and Orally Bioavailable Inverse Agonists of the Retinoic Acid Receptor-Related Orphan Receptor C2. ACS Med. Chem. Lett. 10, 972–977. doi:10.1021/acsmedchemlett.9b00158
Vries, R. M. J. M., Doveston, R. G., Meijer, F. A., and Brunsveld, L. (2020). Elucidation of an Allosteric Mode of Action for a Thienopyrazole RORγt Inverse Agonist. ChemMedChem 15, 561–565. doi:10.1002/cmdc.202000044
Wang, T., Banerjee, D., Bohnert, T., Chao, J., Enyedy, I., Fontenot, J., et al. (2015). Discovery of Novel Pyrazole-Containing Benzamides as Potent RORγ Inverse Agonists. Bioorg. Med. Chem. Lett. 25, 2985–2990. doi:10.1016/j.bmcl.2015.05.028
Wang, Y.-M., Ong, S. S., Chai, S. C., and Chen, T. (2012). Role of CAR and PXR in Xenobiotic Sensing and Metabolism. Expert Opin. Drug Metabolism Toxicol. 8, 803–817. doi:10.1517/17425255.2012.685237
Wang, Y., Cai, W., Cheng, Y., Yang, T., Liu, Q., Zhang, G., et al. (2015). Discovery of Biaryl Amides as Potent, Orally Bioavailable, and CNS Penetrant RORγt Inhibitors. ACS Med. Chem. Lett. 6, 787–792. doi:10.1021/acsmedchemlett.5b00122
Wang, Y., Cai, W., Tang, T., Liu, Q., Yang, T., Yang, L., et al. (2018). From RORγt Agonist to Two Types of RORγt Inverse Agonists. ACS Med. Chem. Lett. 9, 120–124. doi:10.1021/acsmedchemlett.7b00476
Wang, Y., Yang, T., Liu, Q., Ma, Y., Yang, L., Zhou, L., et al. (2015). Discovery of N -(4-Aryl-5-Aryloxy-Thiazol-2-Yl)-Amides as Potent RORγt Inverse Agonists. Bioorg. Med. Chem. 23, 5293–5302. doi:10.1016/j.bmc.2015.07.068
Weikum, E. R., Liu, X., and Ortlund, E. A. (2018). The Nuclear Receptor Superfamily: A Structural Perspective. Protein Sci. 27, 1876–1892. doi:10.1002/pro.3496
Xie, W., Yeuh, M.-F., Radominska-Pandya, A., Saini, S. P. S., Negishi, Y., Bottroff, B. S., et al. (2003). Control of Steroid, Heme, and Carcinogen Metabolism by Nuclear Pregnane X Receptor and Constitutive Androstane Receptor. Proc. Natl. Acad. Sci. U.S.A. 100, 4150–4155. doi:10.1073/pnas.0438010100
Xu, R. X., Lambert, M. H., Wisely, B. B., Warren, E. N., Weinert, E. E., Waitt, G. M., et al. (2004). A Structural Basis for Constitutive Activity in the Human CAR/RXRα Heterodimer. Mol. Cell 16, 919–928. doi:10.1016/j.molcel.2004.11.042
Xue, Y., Guo, H., and Hillertz, P. (2016). Fragment Screening of RORγt Using Cocktail Crystallography: Identification of Simultaneous Binding of Multiple Fragments. ChemMedChem 11, 1881–1885. doi:10.1002/cmdc.201600242
Yang, M. G., Beaudoin-Bertrand, M., Xiao, Z., Marcoux, D., Weigelt, C. A., Yip, S., et al. (2021). Tricyclic-Carbocyclic RORγt Inverse Agonists-Discovery of BMS-986313. J. Med. Chem. 64, 2714–2724. doi:10.1021/acs.jmedchem.0c01992
Yang, T., Liu, Q., Cheng, Y., Cai, W., Ma, Y., Yang, L., et al. (2014). Discovery of Tertiary Amine and Indole Derivatives as Potent RORγt Inverse Agonists. ACS Med. Chem. Lett. 5, 65–68. doi:10.1021/ml4003875
Yukawa, T., Nara, Y., Kono, M., Sato, A., Oda, T., Takagi, T., et al. (2019). Design, Synthesis, and Biological Evaluation of Retinoic Acid-Related Orphan Receptor γt (RORγt) Agonist Structure-Based Functionality Switching Approach from in House RORγt Inverse Agonist to RORγt Agonist. J. Med. Chem. 62, 1167–1179. doi:10.1021/acs.jmedchem.8b01181
Zhang, H., Lapointe, B. T., Anthony, N., Azevedo, R., Cals, J., Correll, C. C., et al. (2020). Discovery of N-(Indazol-3-yl) Piperidine-4-carboxylic Acids as RORγt Allosteric Inhibitors for Autoimmune Diseases. ACS Med. Chem. Lett. 11, 114–119. doi:10.1021/acsmedchemlett.9b00431
Zhang, Y., Luo, X.-y., Wu, D.-h., and Xu, Y. (2015). ROR Nuclear Receptors: Structures, Related Diseases, and Drug Discovery. Acta Pharmacol. Sin. 36, 71–87. doi:10.1038/aps.2014.120
Keywords: protein-ligand interaction, statistical analysis, nuclear receptor, constitutive activity, inverse agonist
Citation: Pham B, Cheng Z, Lopez D, Lindsay RJ, Foutch D, Majors RT and Shen T (2022) Statistical Analysis of Protein-Ligand Interaction Patterns in Nuclear Receptor RORγ. Front. Mol. Biosci. 9:904445. doi: 10.3389/fmolb.2022.904445
Received: 25 March 2022; Accepted: 16 May 2022;
Published: 15 June 2022.
Edited by:
Weiliang Zhu, Shanghai Institute of Materia Medica (CAS), ChinaReviewed by:
Ho Leung Ng, Atomwise Inc., United StatesJian Zhang, Shanghai Jiao Tong University, China
Copyright © 2022 Pham, Cheng, Lopez, Lindsay, Foutch, Majors and Shen. This is an open-access article distributed under the terms of the Creative Commons Attribution License (CC BY). The use, distribution or reproduction in other forums is permitted, provided the original author(s) and the copyright owner(s) are credited and that the original publication in this journal is cited, in accordance with accepted academic practice. No use, distribution or reproduction is permitted which does not comply with these terms.
*Correspondence: Tongye Shen, dHNoZW5AdXRrLmVkdQ==