- 1College of Life Science, Jilin Agricultural University, Changchun, China
- 2Jilin Provincial Key Laboratory of Animal Resource Conservation and Utilization, Northeast Normal University, Changchun, China
High-frequency hearing is regarded as one of the most functionally important traits in laryngeally echolocating bats. Abundant candidate hearing-related genes have been identified to be the important genetic bases underlying high-frequency hearing for laryngeally echolocating bats, however, extensive metabolites presented in the cochleae have not been studied. In this study, we identified 4,717 annotated metabolites in the cochleae of two typical laryngeally echolocating bats using the liquid chromatography–mass spectroscopy technology, metabolites classified as amino acids, peptides, and fatty acid esters were identified as the most abundant in the cochleae of these two echolocating bat species, Rhinolophus sinicus and Vespertilio sinensis. Furthermore, 357 metabolites were identified as significant differentially accumulated (adjusted p-value <0.05) in the cochleae of these two bat species with distinct echolocating dominant frequencies. Downstream KEGG enrichment analyses indicated that multiple biological processes, including signaling pathways, nervous system, and metabolic process, were putatively different in the cochleae of R. sinicus and V. sinensis. For the first time, this study investigated the extensive metabolites and associated biological pathways in the cochleae of two laryngeal echolocating bats and expanded our knowledge of the metabolic molecular bases underlying high-frequency hearing in the cochleae of echolocating bats.
1 Introduction
Echolocation is a remarkable and perceptive behavior that is well evolved in bats, which is usually used for orientation, obstacle avoidance, and hunting (Jones, 2005; Jones and Teeling, 2006). Although echolocation is found in several mammalian lineages (He et al., 2021), laryngeally echolocating bats are renowned for their sophisticated echolocation (Simmons et al., 1979; Jones and Teeling, 2006). High-frequency hearing is an important component of echolocation and is essential for echolocating bats to perceive ultrasonic signals (Wohlgemuth et al., 2016; Moss, 2018). The molecular bases underlying echolocation accompanied by high-frequency hearing have attracted increasing attentions (Cao et al., 2022).
The majority of echolocating bats are usually referred to as laryngeally echolocating bats who can emit ultrasonic vocalizations through their larynxes (Waters and Vollrath, 2003; Yovel et al., 2010), including constant-frequency (CF) bats and frequency-modulated (FM) bats. Therefore, both CF and FM bats have been identified as laryngeally echolocating bats in the true sense of the term with high-frequency hearing. The realization of high-frequency hearing involves many organs and physiological processes, among them, the cochlea is a most important organ of the auditory system (Adams and Pedersen, 2013; Moss, 2018). It is a snail-shaped inner ear structure that plays important roles in sound perception, signal processing, and transmission to the brain (Davies et al., 2013; Moss, 2018). Previous studies have demonstrated that the cochleae of laryngeally echolocating bats have possessed special structural, physiological and genetic adaptations for detecting high-frequency acoustic signals (Ulanovsky and Moss, 2008; Schnitzler and Denzinger, 2011; Vater and Kössl, 2011; Davies et al., 2013).
Transcriptomic approaches have been usually used to uncover candidate genes and biological pathways underlying the genetic bases of adaptations for high-frequency hearing in echolocating bats (Dong et al., 2013; Wang et al., 2018; Ma et al., 2020). A study involving comparative inner ear transcriptomic analysis between M. ricketti (FM echolocating bat) and Cynopterus sphinx (non-echolocating bat) demonstrated that the genes upregulated in Myotis ricketti were particularly associated with cochlear morphogenesis, inner ear morphogenesis, and sensory perception of sound categories, which are consistent with the morphological and physiological differentiation of the inner ear between these two species (Dong et al., 2013). In addition, comparative cochlear transcriptomic analyses of four different bat species have demonstrated variations of gene expression among the bats and different nervous system activities during auditory perception in the cochlea particularly in CF bats (Wang et al., 2018). Besides, numerous efforts to identify high-frequency hearing-related genes have examined differences in coding sequences between echolocating and non-echolocating mammalian species including bats and even whales (Li et al., 2008; Liu et al., 2010; Davies et al., 2012; Wang et al., 2020; Liu et al., 2022). Genome-wide screening has also revealed that multiple hearing-related genes show molecular adaptation in lineages of echolocators (Parker et al., 2013; Zou and Zhang, 2015; Liu et al., 2018; Liu et al., 2022).
In recent years, an accumulating body of researches have indicated that abundant metabolites may be particularly important for specific phenotype (Keurentjes, 2009; Zampieri and Sauer, 2017; Han et al., 2021). Metabolomics is a new branch of “-omics” science in the postgenomic era that has high potential due to its close relationship with phenotype (Fraga-Corral et al., 2022). In addition to the hearing-related genes, cochlear metabolites may also play important roles in the realization of high-frequency hearing for echolocating bats (Meng et al., 2020; Wörheide et al., 2021). However, cochlear metabolites and associated metabolic pathways in laryngeally echolocating bats have been less clearly understood.
Therefore, in this study, we explored the utility of liquid chromatography–mass spectroscopy (LC-MS) to take an insight into the cochlear metabolites of two typical laryngeally echolocating bats, R. sinicus and V. sinensis. R. sinicus, belonging to the Rhinolophidae, is a typical CF bat species, whose dominant frequency is around 83.15 kHz (Xie et al., 2017; Wang et al., 2018). Meanwhile, Vespertilio sinensis is a typical FM bat and belongs to the Vespertilionidae, with a dominant frequency around 24.2 kHz (Fukui et al., 2004). For the first time, we here aim to detect the metabolites responsible for the cochlear function of laryngeally echolocating bats. We also aim to uncover the differences of metabolites and related biological processes in the cochleae between these two bat species. This study is expected to provide a new perspective for the studies of echolocation and high-frequency hearing in bats.
2 Materials and Methods
2.1 Sample collection
Six biological repeats for each bat species were included for the metabolomic analyses. Therefore, six adults of R. sinicus and V. sinensis were captured during July, respectively. To avoid any influence of sex-related differences, only females were selected for inclusion in the study. All individuals were euthanized by cervical dislocation, and a pair of cochleae from each individual were collected and immediately flash-frozen in liquid nitrogen in the field, before transfer to a −80°C freezer.
2.2 Metabolite extraction and sequencing sample preparation
Equal amounts of the cochlear samples from the two bat species (30 mg, n = 12) were transferred to a 2 ml centrifuge tube, supplemented with 600 µl of precooled 50% methanol (stored at −20°C) containing 2-amino-3-(2-chlorophenyl)-propionic acid (4 ppm), and vortexed for 1 min. Subsequently, the samples were precooled at −20°C for 2 min, supplemented with 100 mg of glass beads, and placed in a tissue grinder for 2 min at 60 Hz. Room temperature ultrasonic extraction was then applied for 15 min, followed by storage at −20°C for 30 min. After centrifugation at 13,000 g for 10 min at 4°C, the supernatant was transferred to a new tube. The extraction solution was vacuum-dried and resuspended in 50% methanol (vortexing for 30 s and ultrasonic extraction for 3 min), followed by storage at −20°C for 2 h. Finally, centrifugation was applied at 13,000 g for 10 min at 4°C, and the obtained supernatant was transferred into a detection bottle. Meanwhile, pooled quality control (QC) samples were prepared by mixing an equal volume of each extraction sample. All the samples were stored at −80°C prior to the LC-MS analysis. The experimental process mainly refers to the reagent supplies manual instruction and references (Zhou et al., 2012; Cao et al., 2020).
2.3 LC-MS-based metabolomic analysis
All samples were analyzed using an ACQUITY UPLC I-Class system (Waters Corporation, Milford, MA, USA) coupled with a VION IMS QTOF mass spectrometer (Waters Corporation, Milford, MA, United States) for metabolic profiling in both ESI positive and ESI negative ion modes. An ACQUITY UPLC BEH C18 column (1.8 μm, 2.1 × 100 mm) was employed in both positive and negative modes. Water and acetonitrile/methanol 2/3 (v/v), both containing 0.1% formic acid, were used as mobile phases A and B, respectively. The following linear gradient was applied: 0.01 min, 5% B; 4 min, 30% B; 8 min, 50% B; 10 min, 80% B; 14 min, 100% B; 15 min, 100% B; 15.1 min, 5% B; and 16 min, 5% B. The flow rate was 0.35 mL/min and the column temperature was maintained at 45°C. All samples were kept at 4°C during the analysis. The injection volume was 2 μL. The mass range was from m/z 100 to 1,200. The resolution was set at 70,000 for the full MS scans and 17,500 for the HCD MS/MS scans. The collision energy was set at 10, 20, and 40 eV. The mass spectrometer was operated as follows: spray voltage, 3,800 V (+) for the positive ion mode and 3,200 V (−) for the negative ion mode; sheath gas flow rate, 40 arbitrary units; auxiliary gas flow rate, 8 arbitrary units; capillary temperature, 320°C; probe heater temperature, 350°C; and S-lens RF level, 50. The QC samples (created by pooling all of the samples) were injected at regular intervals throughout the analytical run to provide a set of data from which repeatability could be assessed (Zhou et al., 2012; Cao et al., 2020).
2.4 Metabolomic data processing
The raw LC-MS data were processed using the software Progenesis QI V2.3 (Non-linear Dynamics, Newcastle, United Kingdom) for baseline filtering, peak identification, integrity, retention time correction, peak alignment, and normalization. The main parameters of 5 ppm precursor tolerance, 10 ppm product tolerance, and 5% product ion threshold were applied. Compound identification was based on the precise mass-to-charge ratio (m/z), secondary fragments, and isotopic distribution using the Human Metabolome Database (HMDB, http://www.hmdb.ca), The Kyoto Encyclopedia of Genes and Genomes (KEGG, http://www.kegg.com/), Lipidmaps (V2.3), Metlin, EMDB, PMDB, and custom-made databases to perform qualitative analysis. The extracted data were then further processed by removing any peaks with a missing value (ion intensity = 0) in more than 50% in groups, and by screening according to the qualitative results of the compound. Compounds with resulting scores below 36 points were also deemed to be inaccurate and removed (the full score is 60 and the pass mark is 36). A data matrix was combined from the positive ion and negative ion data. The matrix was imported into R to carry out principal component analysis (PCA) to observe the overall distribution among the samples and the stability of the whole analytical process. Orthogonal partial least-squares discriminant analysis (OPLS-DA) and partial least-squares discriminant analysis (PLS-DA) were used to distinguish the metabolites that differ between groups. To prevent overfitting, sevenfold cross-validation and 200 response permutation testing (RPT) were used to evaluate the quality of the model. Variable importance of projection (VIP) values obtained from the OPLS-DA model were used to rank the overall contribution of each variable to group discrimination. Two-tailed Student’s t-test was further used to verify that the metabolites differing between groups were significant. Differential metabolites with VIP values greater than 1.0 and p-values less than 0.05 were selected.
2.5 Differentially accumulated metabolites (DAMs) identified and bioinformatic analysis
To reveal the differences between the cochleae of R. sinicus and V. sinensis, a series of criteria were used to identify the DAMs among annotated metabolites: VIP >1, |fold change| > 1.5, and adjusted p-value <0.05. Visualization of the DAMs in the two bat species was achieved by creating heatmaps and a volcano plot using the OmicShare tools (http://www.omicshare.com/tools), a free online platform for data analysis. Among the DAMs, the predominantly accumulated metabolites in the cochleae of R. sinicus and V. sinensis were submitted to the OmicShare tools (http://www.omicshare.com/tools) to identify the representative KEGG pathways for further elucidation of the functional properties.
3 Results
3.1 Global metabolites detected in the cochlea of two laryngeally echolocating bat species
To explore the global metabolites in the cochleae of the two laryngeally echolocating bats, R. sinicus and V. sinensis, untargeted metabolomic analyses were performed, which identified 4,717 annotated metabolites from 10,958 positive ion and negative ion features (Table 1; Supplementary Table S1). The overlapping total ion chromatograms (TIC) of positive mode and negative mode of all samples and the QC samples demonstrated that all samples obtained in the positive ion and negative ion modes had a good overlap, which indicated that this model was stable, reproducible, and consistent for all of the samples (Supplementary Figure S1).
3.2 Multivariate statistical analysis of sequencing samples
PCA was used to determine the sample separation and aggregation between R. sinicus and V. sinensis (Figure 1A). Each point on the PCA score graph represents a single sample. Aggregation of points indicates that the observed variables are highly similar, while discrete points represent significant differences in the observed variables. The PCA scores illustrated that PC1 and PC2 were responsible for 54.4% and 9.6% of the variation, respectively, indicating a clear separation between these two bat species. The results demonstrated that R. sinicus and V. sinensis had different cochlear metabolic characteristics. In addition, PLS-DA, which is a supervised discriminant profiling statistical method, was used to identify more specific differences between the groups (Figure 1B). Accordingly, higher values for PLS-DA model parameters denote greater reliability for the PLS-DA model. R2 of the PLS-DA model was 0.998 and Q2 was 0.967, which denoted greater reliability for the PLS-DA model. According to the PLS-DA model parameters, this model was reasonable for interpreting the differences between the two bat species. In addition, the OPLS-DA score plot demonstrated a clearer separation of R. sinicus and V. sinensis and the parameters were as follows: R2X = 0.774, R2Y = 0.998, and Q2 = 0.974, indicating that the current OPLS-DA model is more reliable and that consistent modeling and predictability were achieved (Figures 1C, D). Therefore, these data were used for subsequent analyses.
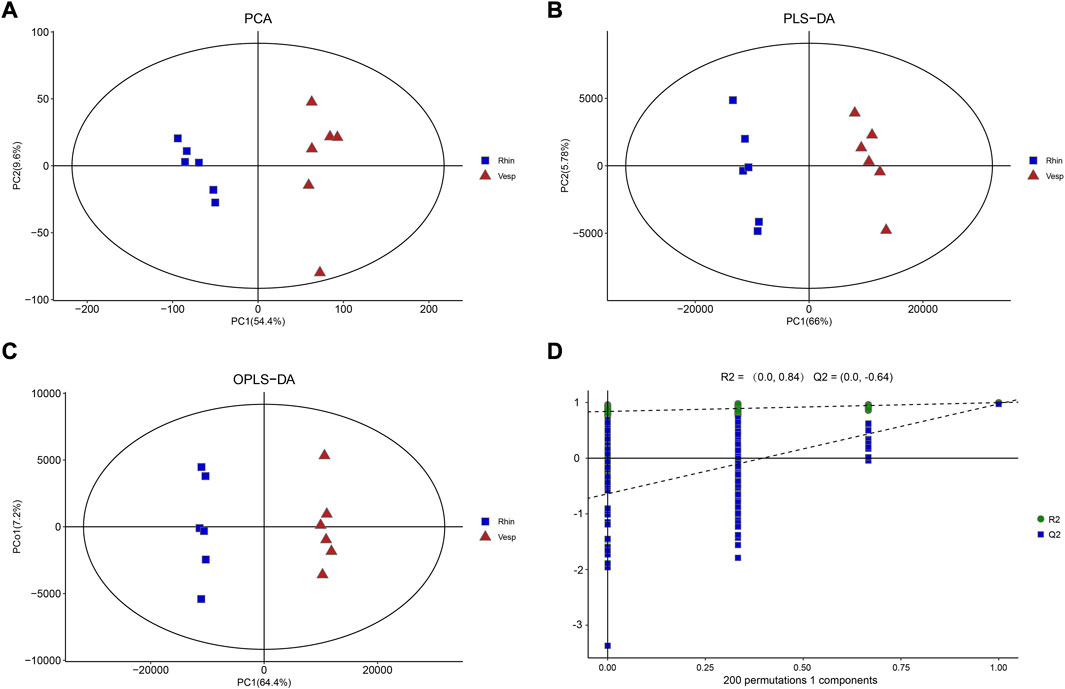
FIGURE 1. Results of multivariate analysis of cochlear samples from the R. sinicus, and V. sinensis. (A) PCA score plot, (B) OPLS-DA score plot, (C) PLS-DA score plot, (D) permutation plot. There is a clear separation of R. sinicus, and V. sinensis.
3.3 Classification of metabolites
In addition, heatmaps of the 4,717 annotated metabolites identified in the cochleae of the two echolocating bats are presented in Figure 2, which illustrates that metabolites varied greatly between R. sinicus and V. sinensis according to the total metabolites and both sets of metabolites detected based on positive ion and negative ion analyses.
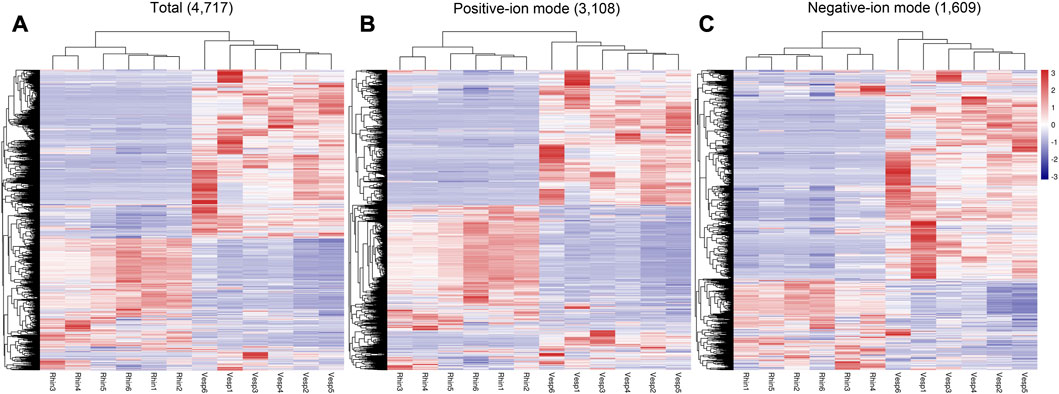
FIGURE 2. Heatmaps of the 4,717 annotated metabolites identified in the cochlea of R. sinicus and V. sinensis. (A) Total ions, (B) Positive ion mode, (C) Negative ion mode. Rhin and Vesp stand R. sinicus and V. sinensis, respectively, as also used elsewhere in this paper.
Based on the annotations of the 4,717 metabolites, most metabolites were assigned to at least one metabolic category and various different types of metabolites were detected in the cochleae of the two echolocating bats (Supplementary Table S2). Among these 4,717 annotated metabolites, 3,410 metabolites, 3,405 metabolites, and 3,177 metabolites were identified to be Super-class, Class, and Sub-class metabolic categories (Figure 3; Supplementary Table S2). At the Super-class level, the three largest metabolic categories were Lipids and lipid-like molecules (1,987 metabolites), Organic acids and derivatives (446 metabolites), Organoheterocyclic compounds (315 metabolites) (Figure 3A). Similarly, metabolites detected at the Class and the Sub-class levels were illustrated by Figures 3B, C, respectively.
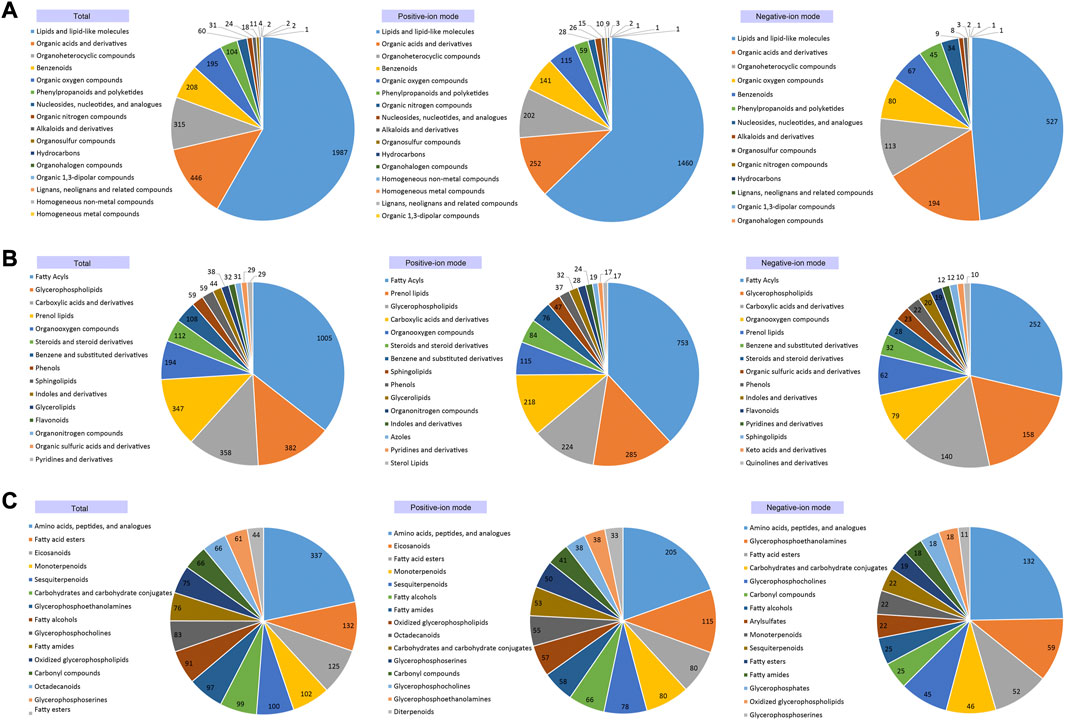
FIGURE 3. Annotated cochlear metabolites detected for Super-classification (A), Classification (B) and Sub-classification (C), respectively.
Various metabolic categories were identified for the metabolites detected in the cochleae of the two echolocating bats. Figure 4 has showed the KEGG classes of all identified metabolites, indicating that several metabolism related processes were the most abundant, including Amino acid metabolism, Lipid metabolism, and Carbohydrate metabolism. Further analysis of the third hierarchical levels of KEGG pathways is listed in Supplementary Table S3. The top 5 largest KEGG pathways were Metabolic pathways (416), Arachidonic acid metabolism (46), Glycerophospholipid metabolism (39), Biosynthesis of amino acids (39), and ABC transporters (39).
3.4 DAMs detected in the cochlea between R. sinicus and V. sinensis
Accordingly, 4,717 high-quality metabolites were used to screen the significant DAMs using the criteria referred to in the Materials and Methods. A total of 357 DAMs were identified to be significantly differentially accumulated (adjusted p-value <0.05) between R. sinicus and V. sinensis, including 129 and 228 metabolites significantly abundant in the cochleae of R. sinicus and V. sinensis, respectively. A heatmap was used to exhibit the 357 DAMs with different levels in the cochleae of R. sinicus and V. sinensis (Figure 5A), which suggested that significantly different metabolites were accumulated in the cochleae of the two bat species. A volcano plot indicated the results of analyzing the significance of the DAMs between R. sinicus and V. sinensis (Figure 5B). Concretely, the DAMs were further assigned to the Super-class (Supplementary Figure S2), Class (Supplementary Figure S3), and Sub-class categories (Supplementary Figure S4) indicating variations of cochlear metabolites between R. sinicus and V. sinensis.
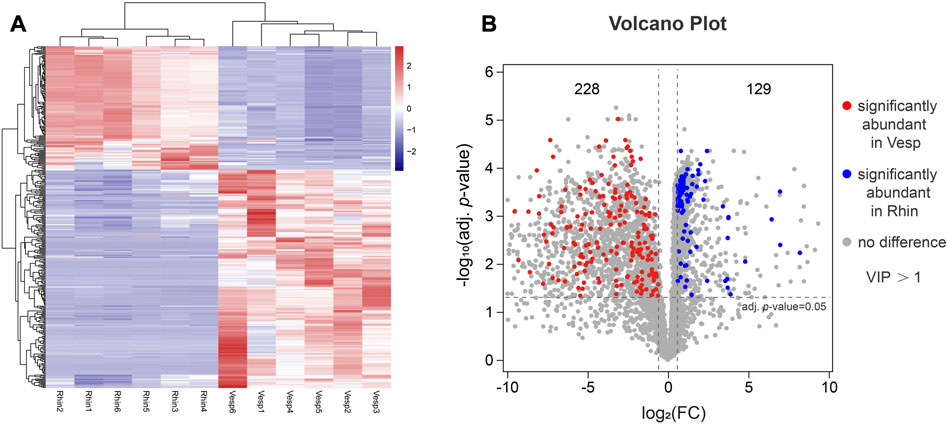
FIGURE 5. Visualization of DAMs present at significantly different levels in R. sinicus and V. sinensis. (A) Heatmaps of DAMs with the abundance levels in all cochlear samples. Red indicates an increase, blue indicates a decrease, rows indicate different metabolites, and columns indicate different samples. (B) Volcano plot of DAMs.
3.5 Differences of related biological processes between R. sinicus and V. sinensis revealed by DAMs
Numerous KEGG pathways were significantly associated with the DAMs from the R. sinicus versus V. sinensis comparison (Figure 6). In detail, 39 pathways were significantly associated with the DAMs predominantly accumulated in R. sinicus, while 10 pathways were for the DAMs predominantly accumulated in V. sinensis. According to the order of p-values, the top 10 pathways are represented in a bubble plot (Figures 6B, D), which indicates that different biological processes were activated in the cochleae of R. sinicus and V. sinensis. In particular, two nervous system-related pathways, Neurotrophin signaling pathway (ko04722) and Cholinergic synapse (ko04725), were significantly associated with the DAMs predominantly accumulated in R. sinicus, both of which are closely associated with the process of auditory perception. Several signal transduction-related pathways were also found to be important in the cochlea of R. sinicus, such as the MAPK signaling pathway (ko04010), ErbB signaling pathway (ko04012), Ras signaling pathway (ko04014), Rap1 signaling pathway (ko04015), and Calcium signaling pathway (ko04020).
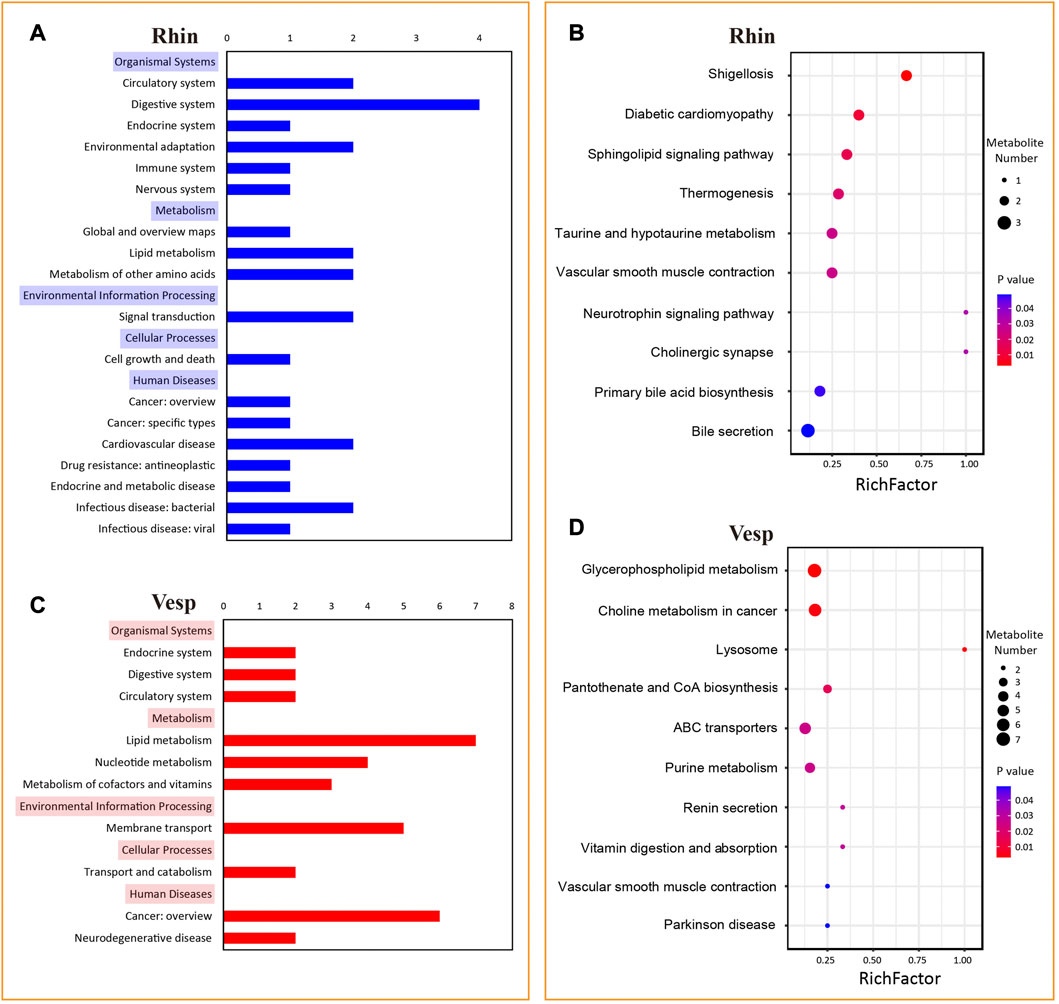
FIGURE 6. KEGG pathways significantly associated with 129 and 228 DAMs with significantly abundant levels in the cochleae of R. sinicus and V. sinensis, respectively. (A) and (C) KEGG A and B categories significantly associated with DAMs for R. sinicus and V. sinensis. (B) and (D) The top 10 KEGG pathways significantly associated with DAMs for R. sinicus and V. sinensis.
Furthermore, network analysis for significantly enriched KEGG pathways was performed to obtain insights into the relationships of the metabolites differently accumulated in R. sinicus and V. sinensis. The network for R. sinicus as shown in Figure 7A indicated that the MAPK signaling pathway (ko04010) was the most important core pathway, which communicated with multiple other signaling pathways, such as the NF-kappa B signaling pathway (ko04064) and Calcium signaling pathway (ko04020). At the same time, it also connected with various biological systems, such as Nervous system, Immune system, and Endocrine system. Meanwhile, specific metabolism-related pathways were potentially more active in the cochlea of V. sinensis, as revealed by the network analysis of significantly enriched KEGG pathways (Figure 7B), including Glycerophospholipid metabolism (ko00564), Pantothenate and CoA biosynthesis (ko00770), and Purine metabolism (ko00230), which closely interacted with each other.
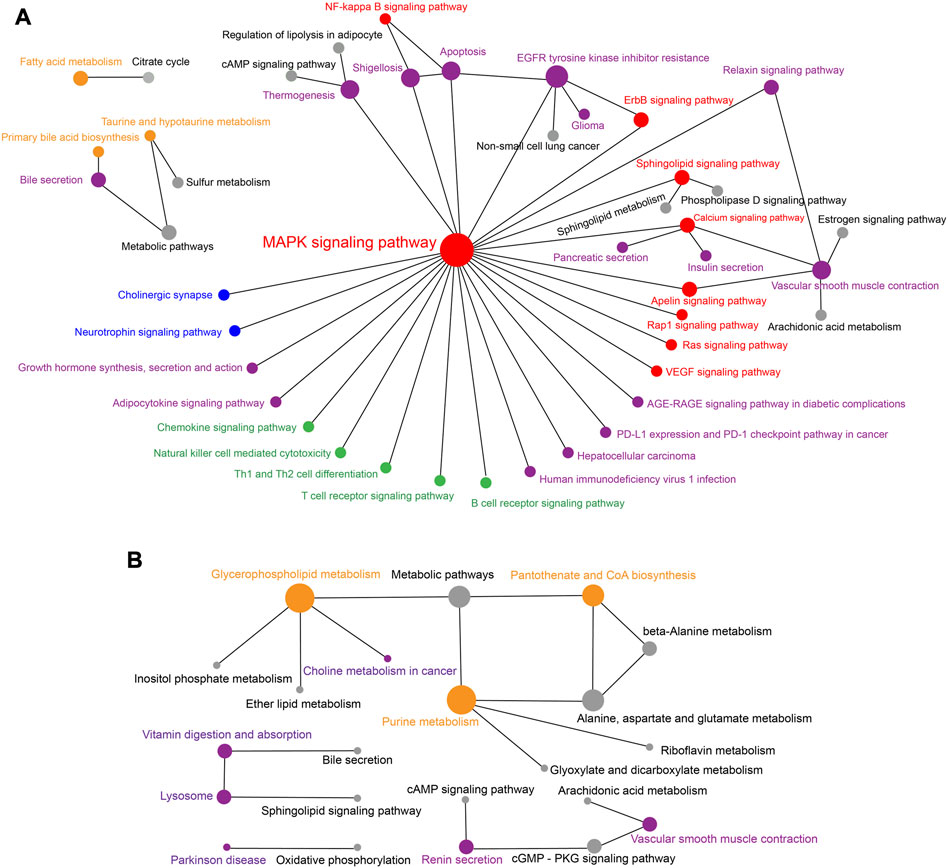
FIGURE 7. Network relationships of the KEGG pathways significantly associated with the significantly abundant metabolites in the cochlea of R. sinicus (A) and V. sinensis (B), respectively. The size of the dot indicates the level of connectivity of a specific pathway interacting with others. Significantly enriched pathways are indicated by colored dots rather than gray ones. Dot and font colors are as follows: signal transduction-related pathways (red), metabolism-related pathways (orange), nervous system-related pathways (blue), immune system-related pathways (green), and others (purple).
4 Discussion
Echolocating bats have attracted much attentions for their remarkable high-frequency hearing ability (Moss, 2018; Cao et al., 2022). However, there seems to be a lack of further understanding of the extensive metabolites in the key auditory organs. Among multiple omics approaches, metabolomics has gradually become the most direct and efficient method to explore complex biological traits (Fiehn, 2022). It is very necessary to identify the cochlear metabolites and to provide further scientific clues for understanding the molecular mechanisms of high-frequency hearing in echolocating bats. Therefore, untargeted metabolomics were performed here to obtain an insight into the various metabolites and biological processes in the cochleae of echolocating bats for the first time, and also to reveal the differences of metabolites and related physiological processes in the cochleae of the two bat species.
The cochleae of R. sinicus and V. sinensis were characterized by abundant metabolites that reflect specific biochemical pathways involved in the process of auditory perception. Metabolites classified as amino acids, peptides, and analogues were the most abundant in the cochleae of echolocating bats, while similar abundance levels were also detected in the inner ear fluid of guinea pig (Fujita et al., 2015; Pirttilä et al., 2019). Besides, amino acid metabolites were also detected in the inner ear of mice (Ji et al., 2019). In addition to amino acids, various metabolites, including hydroxy acids, carbohydrates, alcohols and polyols, homogeneous non-metal compounds, carboxylic acids, fatty acids, and purines and purine derivatives were detected in the inner ear fluid of guinea pig cochlea by gas chromatography–mass spectrometry (GC-MS) (Fujita et al., 2015), which were also detected in the cochleae of the two echolocating bats in this study by LC-MS. However, 4,717 annotated metabolites were detected in the cochlea of bats, which was a greater number than the 77 kinds of metabolites detected in the inner ear fluid of guinea pig cochlea (Fujita et al., 2015). This is reasonable given that the cochlea contains more microscopic structures and more abundant cells than the inner ear fluid of the cochlea, so the metabolites in the cochlea could be more abundant than those only detected in the inner ear fluid of the cochlea. Besides, various metabolites across the major metabolic pathways in central carbon metabolism, including amino acids, nucleotides, cytosine, L-methionine, L-arginine, glutamate, xanthurenic acid, aspartate, phenylalanine, tyrosine, aromatic amino acids, adenosine, oxidized glutathione, methionine, and tryptophan, were detected in the inner ear of mice, which may be involved in responses to noise trauma (Ji et al., 2019); those metabolites were also identified in the cochlea of our two echolocating bat species, which may also constitute the molecular basis to response to noise stimulation, for bats were more usually expose to high intensity noise environment.
In addition, more abundant metabolites were found in the cochlea of laryngeally echolocating bats, which are involved in various aspects of the physiological function of the cochlea, including metabolic processes of amino acids, carbohydrates, lipids, cofactors and vitamins, and nucleotides, as well as the immune system, nervous system, sensory system, membrane transport, and signal transduction. Previous studies demonstrated that hearing acquisition relies on the functional maturation and appropriate organization of the cochlea that couples the transfer of signaling, ions, and nutrients (Kelly and Chen, 2009; Ceriani and Mammano, 2012). Therefore, metabolites detected in the cochleae of bats that participated in the pathways related to various signal transductions, the nervous system, membrane transport, and transport and catabolism, among others, potentially provide comprehensive references for future studies on cochlear function.
Significant metabolic differences were detected between the cochleae of R. sinicus and V. sinensis. Compared with the levels in V. sinensis, 129 metabolites were identified to be significantly abundant in the cochlea of R. sinicus. Multiple pathways were shown to be influenced by these metabolites, including those involving signal transduction, the nervous system, endocrine system, lipid metabolism, and environmental adaptation. This indicates that these biological processes were different and specific in the cochlea of R. sinicus. Neurotrophin signaling pathway (ko04722) and Cholinergic synapse (ko04725) were the two most important pathways, which may play important roles in the auditory perception in R. sinicus. Neurotrophins have been identified as a key factor in the maintenance of spiral ganglion health, playing important roles in the normal function of cochleae (Shew et al., 2021). Previous studies identified several key features of cholinergic synapses in the cochleae of mammals, especially the efferent cholinergic synaptic transmission in the vestibular periphery (Poppi et al., 2020). Our findings suggest that the metabolites participating in the neurotrophin signaling pathway and cholinergic synapses are potentially crucial metabolic bases for the process of auditory perception in R. sinicus. As a representative species of CF bats, R. sinicus possessed unique and high-frequency CF component in their echolocation calls compared with V. sinensis, this regarded as one of the possible reasons for the more abundant neurotrophin signaling pathway and cholinergic synapses in the cochleae of R. sinicus. Besides, CF bat have developed special cochlear adaptations, such as auditory fovea and neurons with extraordinarily sharp frequency tuning, these may consist of other potential reasons for the differences between R. sinicus and V. sinensis revealed by DAMs and related pathways. Besides, it has been reported that SK2 calcium-activated potassium channel is required for cholinergic function in mouse cochlear hair cells (Kong et al., 2008; Jordan et al., 2013) and also for the long-term maintenance of efferent synapses on-to mammalian cochlear hair cells (Murthy et al., 2009). A previous study demonstrated that the SK2 gene, as one of the most important hearing-related genes, has undergone more rapid evolution in echolocating mammals than in non-echolocating mammals and may be involved in the high-frequency hearing of echolocating mammals (Wang et al., 2021). Therefore, adaptive evolutionary changes detected in the cochlea of R. sinicus may be revealed by analyses of both metabolites and genes, and even complex networks of interactions between them, which have adapted in the process of auditory perception.
Notably, compared with the findings in FM bat, different nervous system activities were also demonstrated in the cochlea of R. sinicus (CF bat), as revealed by the gene expression data obtained using RNA-Seq (Wang et al., 2018). Genes overexpressed in R. sinicus (CF bat) compared with the levels in Taphozous melanopogon (FM bat) were shown to be significantly associated with various nervous system components, such as Cholinergic synapse (ko04725), Glutamatergic synapse (ko04724), and Dopaminergic synapse (ko04728). Similarly, at the metabolic level here, two nervous system-related pathways, Cholinergic synapse (ko04725) and Neurotrophin signaling pathway (ko04722) were significantly associated with the DAMs predominantly accumulated in R. sinicus. The Cholinergic synapse (ko04725) was the same pathway that significantly enriched by overexpressed genes and high-accumulated metabolites detected in the cochleae of R. sinicus. Taken together, both expressed genes and metabolites detected in the cochlea of R. sinicus indicated that Cholinergic synapse (ko04725) is an important physiological basis underlying the auditory function. However, more studies are needed to conduct to verify if these clues were suitable for other CF bats.
In addition, the activity of multiple signal transduction pathways was also detected in the cochlea of R. sinicus compared with the findings for V. sinensis, which suggested more active signal transduction in the former. Importantly, the MAPK signaling pathway (ko04010) was identified as the most central signal transduction pathway. Previously, metabolomic and bioinformatic analyses indicated that this is the major pathway in various types of hearing loss (Alagramam et al., 2014; Muurling and Stankovic, 2014; Liu et al., 2021). Numerous studies related to hearing damage have also shown that noise exposure immediately activates the cochlear MAPK signaling pathway, which plays important roles in maintaining normal physiological function of the cochlea (Maeda et al., 2013; Alagramam et al., 2014; Kurabi et al., 2017). For nocturnal echolocating bats, which are highly dependent on acoustic signals and are continually exposed to ultrasonic signals in daily life, the MAPK signaling pathway could be particularly important in regulating the development and survival of auditory hair cells. Compared with V. sinensis (around 24.2 kHz), R. sinicus (around 83.15 kHz) has developed a much higher- and constant-frequency component in its acoustic signals (Fukui et al., 2004; Xie et al., 2017; Wang et al., 2018), so the metabolites significantly accumulated in the MAPK signaling pathway may constitute an important auditory basis in the cochlea of R. sinicus. The molecular interactions of the MAPK signaling pathway and other signaling pathways play an important role in the survival of hair cells and the normal function of cochlea. Pathways such as the calcium signaling pathway play numerous fundamental roles in the inner ear (including in neurotransmitter release and synaptic transmission) (Ceriani and Mammano, 2012; Liu et al., 2021), along with multiple signaling pathways that interact with the MAPK signaling pathway in the cochlea of R. sinicus. Similarly, among the pathways significantly associated with the overexpressed genes detected in the cochlea of R. sinicus rather than in T. melanopogon, signal transduction pathways were almost the most represented, second only to nervous system pathways (Wang et al., 2018). Taken together, evidence from comparative cochlear transcriptomic and metabolomic analyses consistently indicated that signal transduction might be more active in the cochlea R. sinicus compared with FM bats.
More abundant metabolites in the cochlea of V. sinensis when compared with the levels in R. sinicus were significantly associated with metabolic pathways including Glycerophospholipid metabolism (ko00564), Pantothenate and CoA biosynthesis (ko00770), and Purine metabolism (ko00230), which have generally been demonstrated to be involved with exposure to noise. A recent animal experimental study on the brain of rats found that purine metabolism was markedly altered by acoustic trauma (He et al., 2017). Furthermore, in mice, purinergic signaling has been suggested to protect against noise trauma and contribute to cochlear adaptation to elevated sound levels (Muñoz et al., 1999; Housley et al., 2013). It was also identified that metabolites that showed a significant difference in accumulation between microtia and healthy ear cartilage were associated with Pantothenate and CoA biosynthesis, so these may have an association with the development of microtia ear cartilage (Yang and He, 2020). Finally, with regard to Glycerophospholipid metabolism, this has potential protective roles in the cochlea, which was reported to be significantly related to noise-induced hearing loss (Xie et al., 2021). Taken together, our results indicated that different metabolic processes presenting in the cochleae of R. sinicus and V. sinensis. The specific metabolic pathways identified in V. sinensis could be related to cochlear development and protective mechanisms in response to echolocation calls and echoes. Moreover, in this study, it should be noted that the V. sinensis individuals were collected from a large colony roosting under a bridge, containing about 10,000 individuals living together from early June to late September each year. These bats are thus exposed to high-intensity noise from their peers at night and from traffic in the day (Song et al., 2020). The specific metabolic pathways detected in the cochlea of V. sinensis could also be related to cochlear development and protective mechanisms in response to inhabiting a noisy environment. However, more evidences are needed in future to uncover the functions of these metabolic pathways in the cochlea of V. sinensis.
To better understand the molecular bases underlying high-frequency hearing of bats, our study provided an overview of the cochlear metabolites and associated biological processes of two typical laryngeally echolocating bat species. Our study takes new insights into the molecular mechanisms of auditory perception of echolocating bats. In consideration of the limitations of single omics, future work should focus on a comprehensively study to fully uncover the molecular mechanisms and key network relationships combing the transcriptome, proteome, and metabolome in echolocating bats. Unfortunately, no sufficient bat cochlear samples were left here for the experimental validation which needs to be further studied. Therefore, comparative cochlear metabolic and transcriptomic sequencing of more representative bat species from CF, FM and even non-echolocating bat groups will be further performed in future along with the experimental validations to reveal the genetic bases of high-frequency hearing and also the different molecular bases and biological mechanisms underlying the auditory processes of bats with distinct hearing traits.
Data availability statement
The datasets presented in this study can be found in the article/Supplementary Material.
Ethics statement
The animal study was reviewed and approved by Laboratory Animal Welfare and Ethics Committee of Jilin Agricultural University (approval code: 20210607001).
Author contributions
HW and JF designed the project. RS, NX, AL, and JL prepared the materials. XW, MB, and XL performed the experiments. HW analyzed the data and wrote the manuscript. All authors read and approved the final manuscript.
Funding
This research was funded by the National Natural Science Foundation of China (grant number 32001089 to HW and grant number 32071492 to JF).
Acknowledgments
We are grateful to the faculty and students of the Key Laboratory of Jilin Province for the conservation and utilization of animal resources for their invaluable assistance in the field. We thank Liwen Bianji (Edanz) (www.liwenbianji.cn) for editing the language of a draft of this manuscript.
Conflict of interest
The authors declare that the research was conducted in the absence of any commercial or financial relationships that could be construed as a potential conflict of interest.
Publisher’s note
All claims expressed in this article are solely those of the authors and do not necessarily represent those of their affiliated organizations, or those of the publisher, the editors and the reviewers. Any product that may be evaluated in this article, or claim that may be made by its manufacturer, is not guaranteed or endorsed by the publisher.
Supplementary material
The Supplementary Material for this article can be found online at: https://www.frontiersin.org/articles/10.3389/fmolb.2023.1171366/full#supplementary-material
References
Adams, R. A., and Pedersen, S. C. (2013). Bat evolution, ecology, and conservation. New York: Springer.
Alagramam, K. N., Stepanyan, R., Jamesdaniel, S., Chen, D. H-C., and Davis, R. R. (2014). Noise exposure immediately activates cochlear mitogen-activated protein kinase signaling. Noise Health 16, 400–409. doi:10.4103/1463-1741.144418
Cao, M., Li, C., Liu, Y., Cai, K., Chen, L., Yuan, C., et al. (2020). Assessing urinary metabolomics in giant pandas using chromatography/mass spectrometry: Pregnancy-related changes in the metabolome. Front. Endocrinol. 11, 215. doi:10.3389/fendo.2020.00215
Cao, R., Li, J., and Koyabu, D. (2022). A bibliometric analysis of research trends in bat echolocation studies between 1970 and 2021. Ecol. Inf. 69, 101654. doi:10.1016/j.ecoinf.2022.101654
Ceriani, F., and Mammano, F. (2012). Calcium signaling in the cochlea–molecular mechanisms and physiopathological implications. Cell Commun. Signal. 10, 20–12. doi:10.1186/1478-811X-10-20
Davies, K., Cotton, J., Kirwan, J., Teeling, E., and Rossiter, S. (2012). Parallel signatures of sequence evolution among hearing genes in echolocating mammals: An emerging model of genetic convergence. Heredity 108, 480–489. doi:10.1038/hdy.2011.119
Davies, K. T., Maryanto, I., and Rossiter, S. J. (2013). Evolutionary origins of ultrasonic hearing and laryngeal echolocation in bats inferred from morphological analyses of the inner ear. Front. Zool. 10, 2–15. doi:10.1186/1742-9994-10-2
Dong, D., Lei, M., Liu, Y., and Zhang, S. (2013). Comparative inner ear transcriptome analysis between the Rickett’s big-footed bats (Myotis ricketti) and the greater short-nosed fruit bats (Cynopterus sphinx). BMC Genomics 14, 916–1010. doi:10.1186/1471-2164-14-916
Fiehn, O. (2022). Metabolmics-the link between genotypes and phenotypes. Plant Mol. bio. 48, 155–171. doi:10.1023/a:1013713905833
Fraga-Corral, M., Carpena, M., Garcia-Oliveira, P., Pereira, A., Prieto, M., and Simal-Gandara, J. (2022). Analytical metabolomics and applications in health, environmental and food science. Crit. Rev. Anal. Chem. 52, 712–734. doi:10.1080/10408347.2020.1823811
Fujita, T., Yamashita, D., Irino, Y., Kitamoto, J., Fukuda, Y., Inokuchi, G., et al. (2015). Metabolomic profiling in inner ear fluid by gas chromatography/mass spectrometry in Guinea pig cochlea. Neurosci. Lett. 606, 188–193. doi:10.1016/j.neulet.2015.09.001
Fukui, D., Agetsuma, N., and Hill, D. A. (2004). Acoustic identification of eight species of bat (mammalia: Chiroptera) inhabiting forests of southern hokkaido, Japan: Potential for conservation monitoring. Zool. Sci. 21, 947–955. doi:10.2108/zsj.21.947
Han, S., Van-Treuren, W., Fischer, C. R., Merrill, B. D., DeFelice, B. C., Sanchez, J. M., et al. (2021). A metabolomics pipeline for the mechanistic interrogation of the gut microbiome. Nature 595, 415–420. doi:10.1038/s41586-021-03707-9
He, J., Zhu, Y., Aa, J., Smith, P. F., De-Ridder, D., Wang, G., et al. (2017). Brain metabolic changes in rats following acoustic trauma. Front. Neurosci. 11, 1–13. doi:10.3389/fnins.2017.00148
He, K., Liu, Q., Xu, D. M., Qi, F. Y., Bai, J., He, S. W., et al. (2021). Echolocation in soft-furred tree mice. Science 372, eaay1513. doi:10.1126/science.aay1513
Housley, G. D., Morton-Jones, R., Vlajkovic, S. M., Telang, R. S., Paramananthasivam, V., Tadros, S. F., et al. (2013). ATP-gated ion channels mediate adaptation to elevated sound levels. P. Natl. Acad. Sci. U. S. A. 110, 7494–7499. doi:10.1073/pnas.1222295110
Ji, L., Lee, H. J., Wan, G., Wang, G. P., Zhang, L., Sajjakulnukit, P., et al. (2019). Auditory metabolomics, an approach to identify acute molecular effects of noise trauma. Sci. Rep. 9, 9273–9279. doi:10.1038/s41598-019-45385-8
Jones, G., and Teeling, E. C. (2006). The evolution of echolocation in bats. Trends Ecol. Evol. 21, 149–156. doi:10.1016/j.tree.2006.01.001
Jordan, P. M., Parks, X. X., Contini, D., and Holt, J. C. (2013). A review of synaptic mechanisms of vestibular efferent signaling in turtles: Extrapolation to efferent actions in mammals. J. Vestibul. Res. 23, 161–175. doi:10.3233/VES-130492
Kelly, M. C., and Chen, P. (2009). Development of form and function in the mammalian cochlea. Curr. Opin. Neurobiol. 19, 395–401. doi:10.1016/j.conb.2009.07.010
Keurentjes, J. J. (2009). Genetical metabolomics: Closing in on phenotypes. Curr. Opin. Plant Biol. 12, 223–230. doi:10.1016/j.pbi.2008.12.003
Kong, J. H., Adelman, J. P., and Fuchs, P. A. (2008). Expression of the SK2 calcium-activated potassium channel is required for cholinergic function in mouse cochlear hair cells. J. Physiol. 586, 5471–5485. doi:10.1113/jphysiol.2008.160077
Kurabi, A., Keithley, E. M., Housley, G. D., Ryan, A. F., and Wong, A. C-Y. (2017). Cellular mechanisms of noise-induced hearing loss. Hear. Res. 349, 129–137. doi:10.1016/j.heares.2016.11.013
Li, G., Wang, J., Rossiter, S. J., Jones, G., Cotton, J. A., and Zhang, S. (2008). The hearing gene Prestin reunites echolocating bats. P. Natl. Acad. Sci. U. S. A. 105, 13959–13964. doi:10.1073/pnas.0802097105
Liu, Y., Cotton, J. A., Shen, B., Han, X., Rossiter, S. J., and Zhang, S. (2010). Convergent sequence evolution between echolocating bats and dolphins. Curr. Biol. 20, R53–R54. doi:10.1016/j.cub.2009.11.058
Liu, Y., Wei, M., Mao, X., Chen, T., Lin, P., and Wang, W. (2021). Key signaling pathways regulate the development and survival of auditory hair cells. Neural Plast. 2021, 5522717–5522812. doi:10.1155/2021/5522717
Liu, Z., Chen, P., Xu, D. M., Qi, F. Y., Guo, Y. T., Liu, Q., et al. (2022). Molecular convergence and transgenic evidence suggest a single origin of laryngeal echolocation in bats. Iscience 25, 104114. doi:10.1016/j.isci.2022.104114
Liu, Z., Qi, F. Y., Xu, D. M., Zhou, X., and Shi, P. (2018). Genomic and functional evidence reveals molecular insights into the origin of echolocation in whales. Sci. Adv. 4, eaat8821. doi:10.1126/sciadv.aat8821
Ma, L., Sun, H., and Mao, X. (2020). Transcriptome sequencing of cochleae from constant-frequency and frequency-modulated echolocating bats. Sci. Data 7, 341–345. doi:10.1038/s41597-020-00686-w
Maeda, Y., Fukushima, K., Omichi, R., Kariya, S., and Nishizaki, K. (2013). Time courses of changes in phospho-and total-MAP kinases in the cochlea after intense noise exposure. PloS One 8, e58775. doi:10.1371/journal.pone.0058775
Meng, X., Li, G., Gu, L., Sun, Y., Li, Z., Liu, J., et al. (2020). Comparative metabolomic and transcriptome analysis reveal distinct flavonoid biosynthesis regulation between petals of white and purple phalaenopsis amabilis. Phalaenopsis amabilis J. Plant Growth Regul. 39, 823–840. doi:10.1007/s00344-019-10025-y
Moss, C. F. (2018). “Auditory mechanisms of echolocation in bats,” in Oxford research Encyclopedia of neuroscience. Editor S. M. Sherman (Oxford University Press).
Muñoz, D. J., Thorne, P. R., and Housley, G. D. (1999). P2X receptor-mediated changes in cochlear potentials arising from exogenous adenosine 5′-triphosphate in endolymph. Hear. Res. 138, 56–64. doi:10.1016/s0378-5955(99)00151-3
Murthy, V., Maison, S. F., Taranda, J., Haque, N., Bond, C. T., Elgoyhen, A. B., et al. (2009). SK2 channels are required for function and long-term survival of efferent synapses on mammalian outer hair cells. Mol. Cell. Neurosci. 40, 39–49. doi:10.1016/j.mcn.2008.08.011
Muurling, T., and Stankovic, K. M. (2014). Metabolomic and network analysis of pharmacotherapies for sensorineural hearing loss. Otol. Neurotol. 35, 1–6. doi:10.1097/MAO.0000000000000254
Parker, J., Tsagkogeorga, G., Cotton, J. A., Liu, Y., Provero, P., Stupka, E., et al. (2013). Genome-wide signatures of convergent evolution in echolocating mammals. Nature 502, 228–231. doi:10.1038/nature12511
Pirttilä, K., Videhult-Pierre, P., Haglöf, J., Engskog, M., Hedeland, M., Laurell, G., et al. (2019). An LCMS-based untargeted metabolomics protocol for cochlear perilymph: Highlighting metabolic effects of hydrogen gas on the inner ear of noise exposed Guinea pigs. Metabolomics 15, 138–212. doi:10.1007/s11306-019-1595-1
Poppi, L. A., Holt, J. C., Lim, R., and Brichta, A. M. (2020). A review of efferent cholinergic synaptic transmission in the vestibular periphery and its functional implications. J. Neurophysiol. 123, 608–629. doi:10.1152/jn.00053.2019
Schnitzler, H. U., and Denzinger, A. (2011). Auditory fovea and Doppler shift compensation: Adaptations for flutter detection in echolocating bats using CF-FM signals. J. Comp. Physiol. A 197, 541–559. doi:10.1007/s00359-010-0569-6
Shew, M., Wichova, H., Warnecke, A., Lenarz, T., and Staecker, H. (2021). Evaluating neurotrophin signaling using microRNA perilymph profiling in cochlear implant patients with and without residual hearing. Otol. Neurotol. 42, e1125–e1133. doi:10.1097/MAO.0000000000003182
Simmons, J. A., Fenton, M. B., and O'Farrell, M. J. (1979). Echolocation and pursuit of prey by bats. Science 203, 16–21. doi:10.1126/science.758674
Song, S., Chang, Y., Wang, D., Jiang, T., Feng, J., and Lin, A. (2020). Chronic traffic noise increases food intake and alters gene expression associated with metabolism and disease in bats. J. Appl. Ecol. 57, 1915–1925. doi:10.1111/1365-2664.13710
Ulanovsky, N., and Moss, C. F. (2008). What the bat's voice tells the bat's brain. P. Natl. Acad. Sci. U. S. A. 105, 8491–8498. doi:10.1073/pnas.0703550105
Vater, M., and Kössl, M. (2011). Comparative aspects of cochlear functional organization in mammals. Hear. Res. 273, 89–99. doi:10.1016/j.heares.2010.05.018
Wang, H., Zhao, H., Chu, Y., Feng, J., and Sun, K. (2021). Assessing evidence for adaptive evolution in two hearing-related genes important for high-frequency hearing in echolocating mammals. G3-Genes Genom. Genet. 11, jkab069. doi:10.1093/g3journal/jkab069
Wang, H., Zhao, H., Huang, X., Sun, K., and Feng, J. (2018). Comparative cochlear transcriptomics of echolocating bats provides new insights into different nervous activities of CF bat species. Sci. Rep. 8, 15934–16012. doi:10.1038/s41598-018-34333-7
Wang, H., Zhao, H., Sun, K., Huang, X., Jin, L., and Feng, J. (2020). Evolutionary basis of high-frequency hearing in the cochleae of echolocators revealed by comparative genomics. Genome Biol. Evol. 12, 3740–3753. doi:10.1093/gbe/evz250
Waters, D. A., and Vollrath, C. (2003). Echolocation performance and call structure in the megachiropteran fruit-bat Rousettus aegyptiacus. Acta Chiropterol 5, 209–219.
Wohlgemuth, M. J., Luo, J., and Moss, C. F. (2016). Three-dimensional auditory localization in the echolocating bat. Curr. Opin. Neurobiol. 41, 78–86. doi:10.1016/j.conb.2016.08.002
Wörheide, M. A., Krumsiek, J., Kastenmüller, G., and Arnold, M. (2021). Multi-omics integration in biomedical research–A metabolomics-centric review. Anal. Chim. Acta 1141, 144–162. doi:10.1016/j.aca.2020.10.038
Xie, C., Niu, Y., Ping, J., Zhang, Q., Zhang, Z., Wang, Y., et al. (2021). Transcriptome-wide association study identifies novel susceptibility genes contributing to hearing impairment. Res. Sq. Prepr., 1–19.
Xie, L., Sun, K., Jiang, T., Liu, S., Lu, G., Jin, L., et al. (2017). The effects of cultural drift on geographic variation in echolocation calls of the Chinese rufous horseshoe bat (Rhinolophus sinicus). Ethology 123, 532–541. doi:10.1111/eth.12627
Yang, J., and He, L. (2020). Mass spectrometric characterization of metabolites in ear cartilage: Congenital microtia and normal auricle. Ann. Plas. Surg. 85, 76–82. doi:10.1097/SAP.0000000000002127
Yovel, Y., Falk, B., Moss, C. F., and Ulanovsky, N. (2010). Optimal localization by pointing off axis. Science 327, 701–704. doi:10.1126/science.1183310
Zampieri, M., and Sauer, U. (2017). Metabolomics-driven understanding of genotype-phenotype relations in model organisms. Curr. Opin. Syst. Biol. 6, 28–36. doi:10.1016/j.coisb.2017.08.007
Zhou, B., Xiao, J. F., Tuli, L., and Ressom, H. W. (2012). LC-MS-based metabolomics. Mol. Biosyst. 8, 470–481. doi:10.1039/c1mb05350g
Keywords: bats, cochlea, echolocation, high-frequency hearing, untargeted metabolomics
Citation: Wang H, Sun R, Xu N, Wang X, Bao M, Li X, Li J, Lin A and Feng J (2023) Untargeted metabolomics of the cochleae from two laryngeally echolocating bats. Front. Mol. Biosci. 10:1171366. doi: 10.3389/fmolb.2023.1171366
Received: 22 February 2023; Accepted: 06 April 2023;
Published: 19 April 2023.
Edited by:
Elda Dervishi, University of Alberta, CanadaReviewed by:
Nagarjun Vijay, Indian Institute of Science Education and Research, IndiaYazhi Xing, Shanghai Jiao Tong University, China
Copyright © 2023 Wang, Sun, Xu, Wang, Bao, Li, Li, Lin and Feng. This is an open-access article distributed under the terms of the Creative Commons Attribution License (CC BY). The use, distribution or reproduction in other forums is permitted, provided the original author(s) and the copyright owner(s) are credited and that the original publication in this journal is cited, in accordance with accepted academic practice. No use, distribution or reproduction is permitted which does not comply with these terms.
*Correspondence: Hui Wang, wangh681@nenu.edu.cn; Jiang Feng, fengj@nenu.edu.cn