- 1Wicking Dementia Research and Education Centre, University of Tasmania, Hobart, TAS, Australia
- 2Royal Hobart Hospital, Hobart, TAS, Australia
- 3Roche Diagnostics International Ltd, Rotkreuz, Switzerland
- 4Roche Diagnostics GmbH, Penzberg, Germany
- 5Department of Radiology and Nuclear Medicine, University of Amsterdam, De Boelelaan, Amsterdam, Netherlands
Introduction: Modifiable risk factors account for a substantial proportion of Alzheimer’s disease (AD) cases and we currently have a discrete AT(N) biomarker profile for AD biomarkers: amyloid (A), p-tau (T), and neurodegeneration (N). Here, we investigated how modifiable risk factors relate to the three hallmark AT(N) biomarkers of AD.
Methods: Participants from the European Prevention of Alzheimer’s Dementia (EPAD) study underwent clinical assessments, brain magnetic resonance imaging, and cerebrospinal fluid collection and analysis. Generalized additive models (GAMs) with penalized regression splines were modeled in the AD Workbench on the NTKApp.
Results: A total of 1,434 participants were included (56% women, 39% APOE ε4+) with an average age of 65.5 (± 7.2) years. We found that modifiable risk factors of less education (t = 3.9, p < 0.001), less exercise (t = 2.1, p = 0.034), traumatic brain injury (t = −2.1, p = 0.036), and higher body mass index (t = −4.5, p < 0.001) were all significantly associated with higher AD biomarker burden.
Discussion: This cross-sectional study provides further support for modifiable risk factors displaying neuroprotective associations with the characteristic AT(N) biomarkers of AD.
Introduction
Dementia remains one of the greatest global health, social, and economic challenges of the 21st century (Livingston et al., 2017), yet evidence suggests up to 40% of dementia risk is modifiable (Livingston et al., 2020). Modifiable risk factors for dementia are education, hearing loss, traumatic brain injury (TBI), hypertension, alcohol consumption, obesity, smoking, depression, social isolation, physical inactivity, air pollution, and diabetes (Livingston et al., 2020). With research indicating that many dementia cases could be avoided by changing lifestyle behaviors (Livingston et al., 2020), addressing modifiable risk factors could prevent or delay over 40 million cases worldwide by 2050 (Moore et al., 2015). To our knowledge, modifiable risk factors contribute a significant proportion to dementia risk (Livingston et al., 2020), are highly prevalent in the community (Yaffe, 2018), and are exacerbated by low socioeconomic status (Kezios et al., 2022); however, modifiable risk factors are often overlooked as potential therapeutic targets for risk reduction.
Targeting modifiable risk factors entails a suite of positive impacts not only on dementia but also on the prevention of other chronic conditions such as cardiovascular disease and cancer, which share similar risk factors (Edwards et al., 2019). Intervening earlier in the disease course is likely to bring about greater benefits for individuals, where effective interventions for blood pressure reduction, smoking cessation, and prevention of diabetes deliver the greatest efficacy in significantly reducing health care expenditure, reducing dementia prevalence, and producing quality-adjusted life-year gains (Mukadam et al., 2020). There is also evidence for clustering incremental risk from modifiable risk factors. Modifiable risk factors tend to co-occur (Griffin et al., 2014; Morris et al., 2016), where clusters typically consist of smoking, excess alcohol consumption, and physical inactivity (Peters et al., 2019).
Alzheimer’s disease (AD) has a prolonged period of ‘silent’ degeneration, a preclinical phase that lasts up to decades prior to clinical symptom onset (Sperling et al., 2011). During this phase, pathophysiological processes of beta-amyloid (Aβ) accumulation, phosphorylated-tau (p-tau) aggregation, and neurodegeneration emerge, with subtle cognitive deficits developing alongside (Sperling et al., 2011). Under this framework, the National Institute on Aging—Alzheimer’s Association (NIA-AA) has established discrete biomarker profiles to discriminate Alzheimer’s pathological change from non-Alzheimer’s pathological change (Jack et al., 2018). The NIA-AA’s AT(N) profiling system classifies individuals based on the presence of one or more hallmark biomarkers of AD: amyloid β (A), p-tau (T), and neurodegeneration (N). This window of biologically burdened, yet cognitively unimpaired, pathological change presents an ideal opportunity to intervene with modifiable risk factors that can impact dementia risk. By the time clinical symptoms emerge, the underlying AD pathology has likely entered an advanced stage, limiting the impact of interventions (Reiman et al., 2011).
We have strong evidence from around the world that targeting modifiable risk factors can maintain and improve cognitive function (Ngandu et al., 2015), as well as reduce the risk for AD and other forms of dementia (Kivipelto et al., 2018), which is evidenced by findings from a systematic review (Coley et al., 2022) recommending age-, sex-, and factor-specific lifestyle modifications. Whether an intervention targets physical activity and hypertension or is multidomain in nature, risk factor reduction efforts for dementia will likely overlap with preventative efforts for other chronic conditions. This aligns with a global effort to improve the quality of life throughout aging, accounting for the shared pathways and biological mechanisms of age-related chronic conditions. Furthermore, recent advancements have come alongside calls for biomarker results to be disclosed to research participants (Grill and Karlawish, 2022). We currently have the tools to safely disclose biomarker insights in order to foster positive lifestyle modification and create supportive environments for individuals to reduce their risk (Frisoni et al., 2023).
Despite strong evidence for a biologically defined AD continuum (Jack et al., 2018) and modifiable risk amelioration (Livingston et al., 2020), there are very few studies investigating modifiable risk categories against the biologically driven AT(N) biomarkers. Addressing this gap is important as it opens earlier periods of life to intervention. In this study, we aimed to investigate how modifiable risk factors are related to the three hallmark AD biomarkers: amyloid β (A), p-tau (T), and neurodegeneration (N). We hypothesized that we would observe associations between adherence to modifiable risk factors and increased AD biomarker burden measured via AT(N) criteria and that these associations would be of use to clinicians, researchers, and caregivers in assessing the biological and modifiable risk profile of individuals.
Materials and methods
Participants
Participants were drawn from the European Prevention of Alzheimer’s Dementia (EPAD) Longitudinal Cohort Study (LCS) (Ritchie et al., 2020). A full protocol has been published previously (Solomon et al., 2018). Briefly, EPAD is a prospective, multicenter, pan-European longitudinal cohort study. Participants were recruited across 21 different European sites and were eligible if they were at least 50 years of age, had completed at least 5 years of formal education, and did not have a dementia diagnosis at baseline. Participants underwent clinical and neurological assessments including a mini-mental state examination (MMSE), brain magnetic resonance imaging (MRI), lumbar puncture for cerebrospinal fluid (CSF), and comprehensive neuropsychological assessment. For the purposes of this study, we used only EPAD LCS Visit 1 (V1) baseline data (EPAD LCS-v.IMI V1 [n = 2,737, 55.8% women]) as we intended to investigate the clinical utility of a biomarker + modifiable risk factor panel to foster early detection and intervention in healthy populations. For the neurodegeneration analysis, there was a small fraction (n = 17, 1.3%) of participants returning extremely low values for L and R hippocampal volume; therefore, we excluded those participants with a value of less than 1,000 mm3 for total hippocampal volume (THV). Hearing loss was removed from all models due to missing data and insufficient levels for GAM comparison. We did not apply any other exclusion criteria to the study population. Baseline data (V1) from 1,474 participants were included, with sample size numbers varying according to the availability of neuroimaging and lumbar puncture data. The study was approved by the ethical committees of all participating EPAD centers. All study participants provided written informed consent prior to the collection of any study data. All procedures were conducted in accordance with the Declaration of Helsinki.
Non-modifiable risk factors
Non-modifiable criteria consisted of age, sex, and presence of the apolipoprotein epsilon 4 allele (APOE ε4). Age at baseline (in years) was calculated from age (years) and age (months) reported at the time of assessment. Sex at birth was also collected in demographic assessments. APOE genetic analysis was carried out on blood samples collected during baseline assessments. APOE allelic carriage was stratified by two ε alleles for six different combinations: ε2/ε2, ε2/ε3, ε3/ε3, ε3/ε4, ε4/ ε4, and ε4/ε2. We also stratified based on ε4 presence as ε4+ (ε3/ε4, ε4/ε4, or ε4/ε2) and ε4- (ε2/ε2, ε2/ε3, or ε3/ε3).
Modifiable risk factors
Participants completed a variety of demographic, clinical, medical history, and lifestyle surveys at EPAD assessments. Survey collection was standardized across all European sites to ensure consistency of measurement. Data collection protocols were harmonized and were in accordance with the International Conference on Harmonization (ICH). Ten modifiable risk criteria from Livingston et al.’s Lancet Commission were collected (Livingston et al., 2020). We were unable to account for social isolation and air pollution due to data availability. Education (in years) data were collected in demographics. Medical questionnaires asked about the history of diabetes (type 1 or 2), obesity, hypertension, TBI, depression, and hearing loss. Lifestyle factors collected were smoking (never/past/current), alcohol consumption (units/week), and frequency of physical activity, defined as leisure-time physical activity that lasted at least 20 min, and caused breathlessness and sweating (daily, 2–3 times a week, once a week, 2–3 times a month, a few times a year, or not at all) (Rovio et al., 2005). We dichotomized adherence to modifiable risk factors based on existing evidence (Livingston et al., 2020) and stratified the cohort based on fulfilling the following criteria: medical history (yes for diabetes, hypertension, TBI, depression, obesity, and hearing loss); lifestyle (physical inactivity: not at all/a few times a year; smoking: past/current; and alcohol: 2–6 units per day); and education (≤12 years).
Cerebrospinal fluid
CSF was obtained at baseline assessment using a pre-analytical protocol harmonized across study sites. Analyses were performed using the Roche ELECSYS® immunoassays (Roche Diagnostics International Ltd., Rotkreuz, Switzerland) at the University of Gothenburg (Solomon et al., 2018). Concentrations of Aβ1-42 and p-tau 181 were determined according to the manufacturer’s instructions.
Magnetic resonance imaging
Brain MRI scans were performed with standardized acquisition protocols. Images were centrally evaluated by experienced raters and blinded to neuropsychological and clinical data. Scans were visually assessed for white matter hyperintensities, perivascular spaces, microbleeds, medial temporal lobe atrophy (MTA), and posterior cortical atrophy. Regional measures for white matter volume, gray matter volume, and hippocampal volume were determined using a segmentation process based on atlas propagation with the Learning Embeddings for Atlas Propagation framework (Jack et al., 2017). THV is the sum of left hippocampal volume (LHV) and right hippocampal volume (RHV), all expressed in mm3.
AT(N) biomarkers
AT(N) biomarker profiling and classification of EPAD participants was based on published research (Jack et al., 2018; Ebenau et al., 2020; Ingala et al., 2021). Eligible EPAD participants had their AT(N) biomarkers measured in CSF (CSF Aβ1-42 [pg/mL] and CSF p-tau 181 [pg/mL]) and MRI (THV [mm3]). Central laboratories conducted a harmonized protocol to ensure measurement consistency and interpretation: CSF at the University of Gothenburg, genetics at the University of Edinburgh, and neuroimaging at the Amsterdam University Medical Center (Solomon et al., 2018). Participants were classified into presence (+) or absence (−) of abnormal CSF Aβ1-42 (“A”), CSF p-tau 181 (“T”), and neurodegeneration (“N”). For A+/−, participants were split using a cut-off of CSF Aβ1-42: < 1,000 pg./mL classified as A+ and ≥ 1,000 pg./mL classified as A-. For T+/−, CSF p-tau 181: > 27 pg./mL was classified as T+ and ≤ 27 pg./mL was classified as T-. For N+/−, participants’ age and MTA average (L/R) were used: participants were classified as N+ if their age was <65 years and their MTA average (L/R) was ≥1 or their age was ≥65 years and their MTA average (L/R) was ≥1.5; all other participants were classified as N-. AT(N) criteria were further classified based on A+/−, T+/−, and N+/−, stratifying participants into 8 groups: A−/T-/N-, A−/T-/N+; A−/T+/N-, A−/T+/N+, A+/T-/N-, A+/T-/N+, A+/T+/N-, and A+/T+/N+.
Statistical methods
Data were accessed via the NeuroToolKit (NTK) Application (NTKApp, BetaVersion, 2022) on the AD Workbench, a powerful cloud-based data-sharing platform designed by the Alzheimer’s Disease Data Initiative (ADDI). All analyses were performed using R code on the NTK’s Analysis module. Demographic characteristics were expressed as frequencies (percentage) and mean ± standard deviation (SD). T-test for continuous variables and chi-squared test for categorical variables were used to test the difference between men and women and discrete AT(N) groups. Generalized additive models (GAMs) with regression splines were used to model the associations between modifiable risk factor adherence and AT(N) biomarkers. Two GAMs were fit against each individual AT(N) biomarker as the dependent variable. The goodness of fit was determined by interpreting Akaike’s Information Criterion (AIC). Age (in years) and total MMSE (out of 30) were smoothed due to being non-linearly associated with AT(N) biomarkers. Since AD is a disease of aging, an appropriate adjustment of the age that acknowledges this non-linearity was made to more accurately estimate the effects of modifiable risk factors independent of age. Education (in years) and BMI (weight in kg * height in m2) were included in GAMs due to their better fit than binary variables (less education/obesity). Following an inspection of Q-Q plots, AT(N) biomarkers were log-transformed to approximate the normal distribution of residuals; however, untransformed values were used for visualization. Modifiable risk factor independent variables were tested against the individual AT(N) dependent variables in both individual and multiple independent variable models; however, the results were similar and thus multiple independent variables are shown in Model 1. Model 1 was adjusted for all modifiable criteria (education, TBI, hearing, hypertension, alcohol, BMI, smoking, depression, exercise, and diabetes), all unmodifiable criteria (age, sex, and APOE ε4 presence), and cognition (MMSE). Model 2 was adjusted for all significant variables in Model 1 together with unmodifiable covariates. In all GAMs, Model 1 (with all unmodifiable and modifiable criteria) displayed the best fit, with the lowest relative AIC. Figures were produced using the ggplot2 package for R in NTK Analysis. Reproducible NTK Analysis code and data are both available from the AD Workbench.
Data availability
All EPAD data are available via the NTKApp on the AD Workbench, designed by ADDI. NTKApp is accessible at: https://www.alzheimersdata.org/ntk. R code is available upon request to the corresponding author, Dr. Eddy Roccati (ZWRkeS5yb2NjYXRpQHV0YXMuZWR1LmF1).
The EPAD Longitudinal Cohort Study brings together participants from 21 sites across Europe. For a full list of collaborators contributing to open-access data, please visit: https://ep-ad.org/open-access-data/overview/.
Results
Baseline demographic statistics are displayed in Table 1. Participants were predominantly women (56%, N = 821), with an average age of 14.4 (SD 3.7) years of education and the highest genotypic prevalence for APOE being ε3 homozygotes (52%, N = 714). The overall penetrance for APOE ε4 allele was 39.0% in both women and men. Men were significantly older, more educated, and had a higher prevalence of alcohol misuse, smoking, and physical inactivity. Women demonstrated significantly higher rates of depression, higher CSF Aβ1-42, and lower THV than men. The most commonly reported risk factors were obesity (59.3%), smoking (53.9%), less education (34.4%), physical inactivity (21.5%), and alcohol consumption (12.4%). A total of 338 (22.9%) participants reported adhering to zero risk factors, 340 (23.1%) of them reported one, 440 (29.9%) of them reported two, 272 (18.5%) of them reported three, 79 (5.4%) of them reported four, and 5 (0.3%) of them reported five.
AT(N) comparison
Summary statistics stratified by amyloid classification are displayed in Supplementary Table 1. A+ participants (33%, N = 406) were significantly older, had higher APOE ε4 penetrance, higher CSF p-tau 181, lower THV, and lower MMSE scores than A- participants (67%, N = 810). For modifiable risk factors, A+ participants had a significantly higher prevalence of alcohol misuse and obesity. All other modifiable risk factors were non-significant.
Summary statistics stratified by tau classification are displayed in Supplementary Table 2. T+ participants (18%, N = 214) were significantly older, less educated, and had higher penetrance for APOE ε4, lower THV, and lower MMSE scores than T- participants (82%, N = 1,001). Compared with T–, T+ participants had significantly higher rates of smoking and obesity.
Summary statistics stratified by neurodegeneration classification are displayed in Supplementary Table 3. N+ participants (15%, N = 195) were significantly older and displayed higher CSF Aβ1-42 and lower MMSE scores than N- participants (85%, N = 1,105). In terms of modifiable risk factors, N+ participants only had a significantly higher prevalence of smoking than N- participants.
AT(N) generalized additive models
For A in Model 1, APOE ε4 presence and TBI were significantly negatively associated with CSF Aβ1-42 (Table 2; Figure 1). In Model 2, these results remained significant after removing non-significant modifiable risk factors. Smoothed age and MMSE were also significantly associated with CSF Aβ1-42.
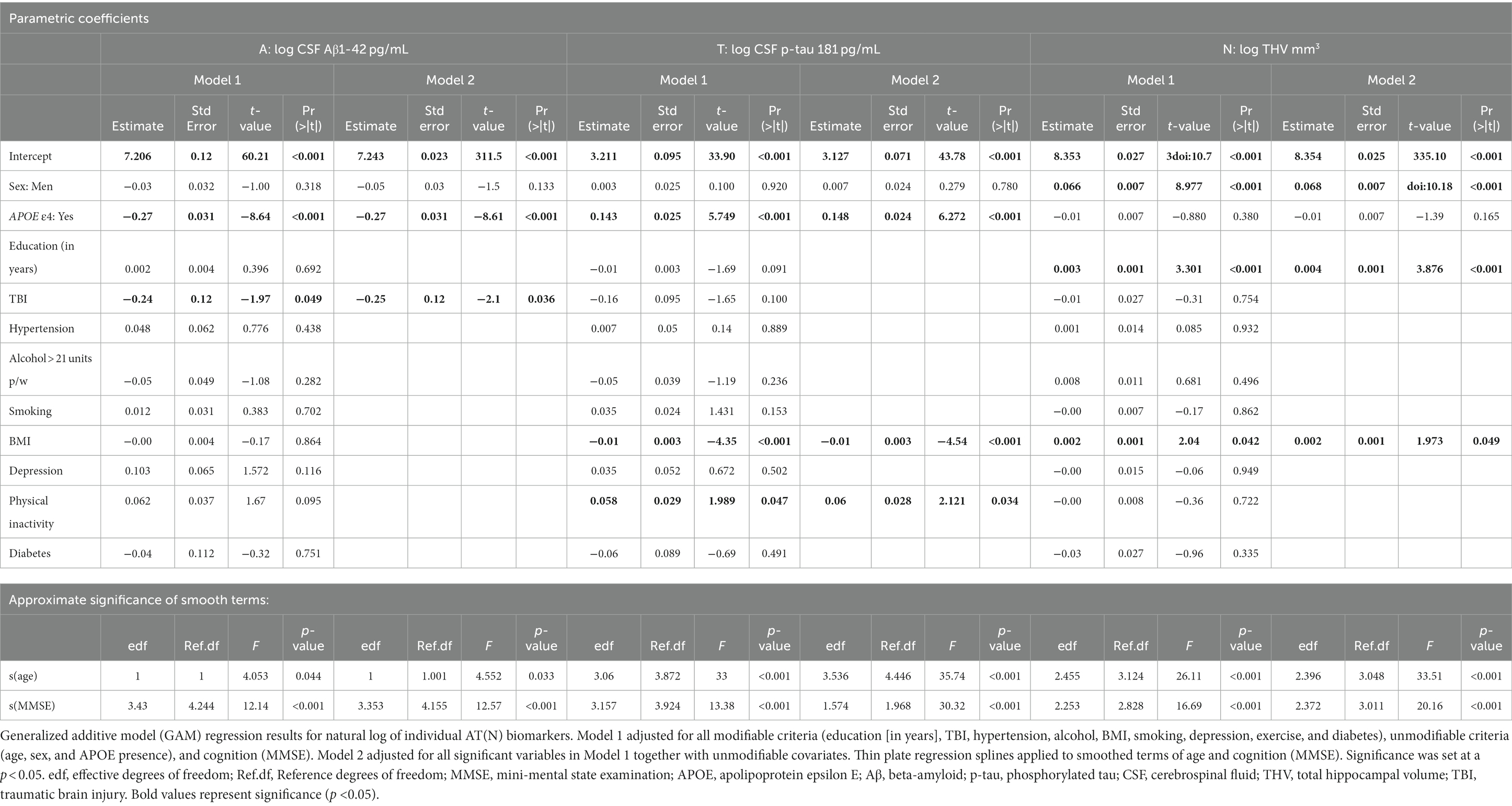
Table 2. Detailed summary table of generalized additive model (GAM) regression results for AT(N) individual biomarkers.
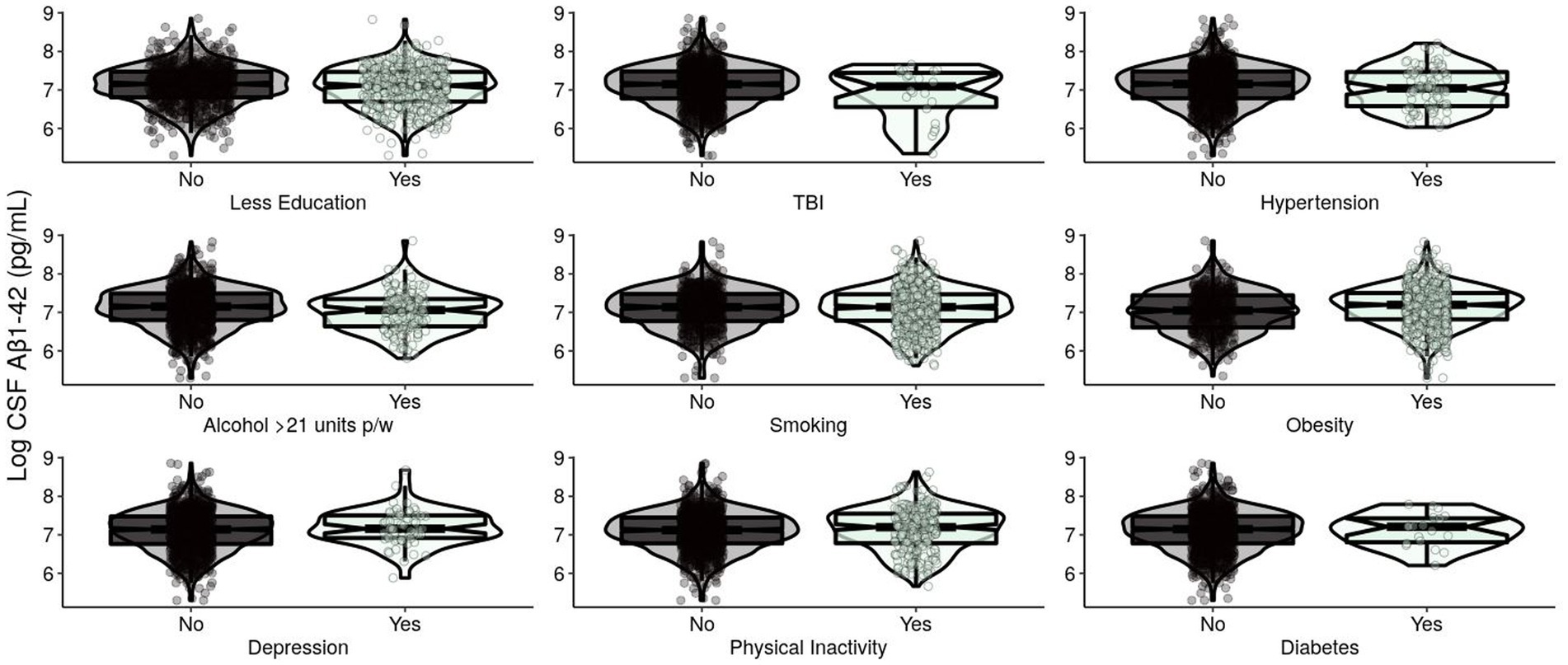
Figure 1. Levels of log-transformed CSF Aβ1-42 (pg/mL) of EPAD participants grouped by adherence to modifiable risk factors. Log-transformed levels of CSF beta-amyloid 1–42 (pg/mL) are presented. Raw amyloid +/− was classified using a cut-off of CSF Aβ1-42: < 1,000 pg./mL classified as A+ and ≥ 1,000 pg./mL classified as A−. CSF, cerebrospinal fluid; Aβ, beta-amyloid; EPAD, European Prevention of Alzheimer’s Dementia; TBI, traumatic brain injury.
For T in Model 1, APOE ε4 and BMI were significantly positively associated with CSF p-tau 181 (Table 2; Figure 2). In Model 2, these results remained significant. BMI was significantly negatively associated with CSF p-tau 181. Smoothed age and MMSE were significantly associated with CSF p-tau 181.
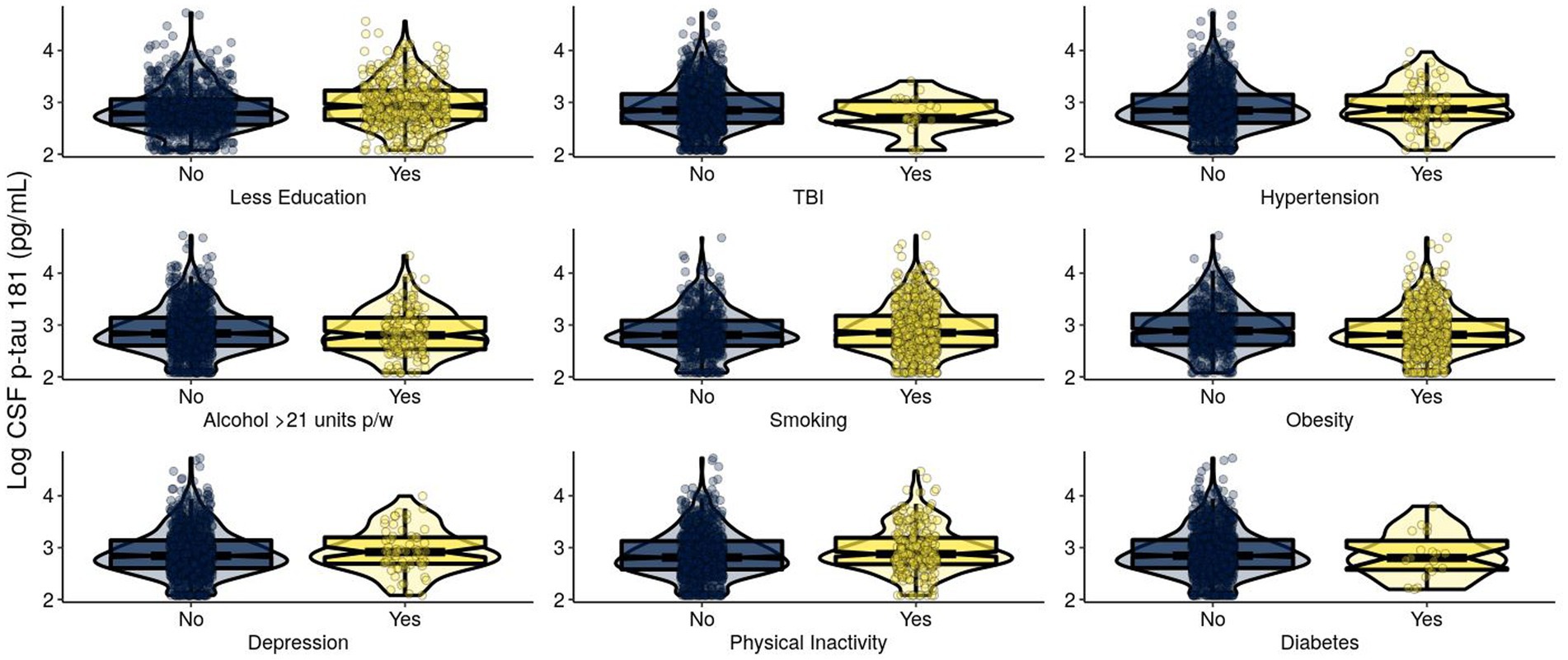
Figure 2. Levels of log-transformed CSF p-tau 181 (pg/mL) of EPAD participants grouped by adherence to modifiable risk factors. Log-transformed levels of CSF p-tau 181 (pg/mL) are presented. Raw tau +/− was classified using a cutoff of CSF p-tau 181: > 27 pg./mL classified as T+ and ≤ 27 pg./mL classified as T−. CSF, cerebrospinal fluid; p-tau, phosphorylated tau; EPAD, European Prevention of Alzheimer’s Dementia; TBI, traumatic brain injury.
For N in Model 1, sex, education, and BMI were significantly positively associated with THV (Table 2; Figure 3). In Model 2, these results remained significant. Smoothed age and MMSE were significantly associated with THV.
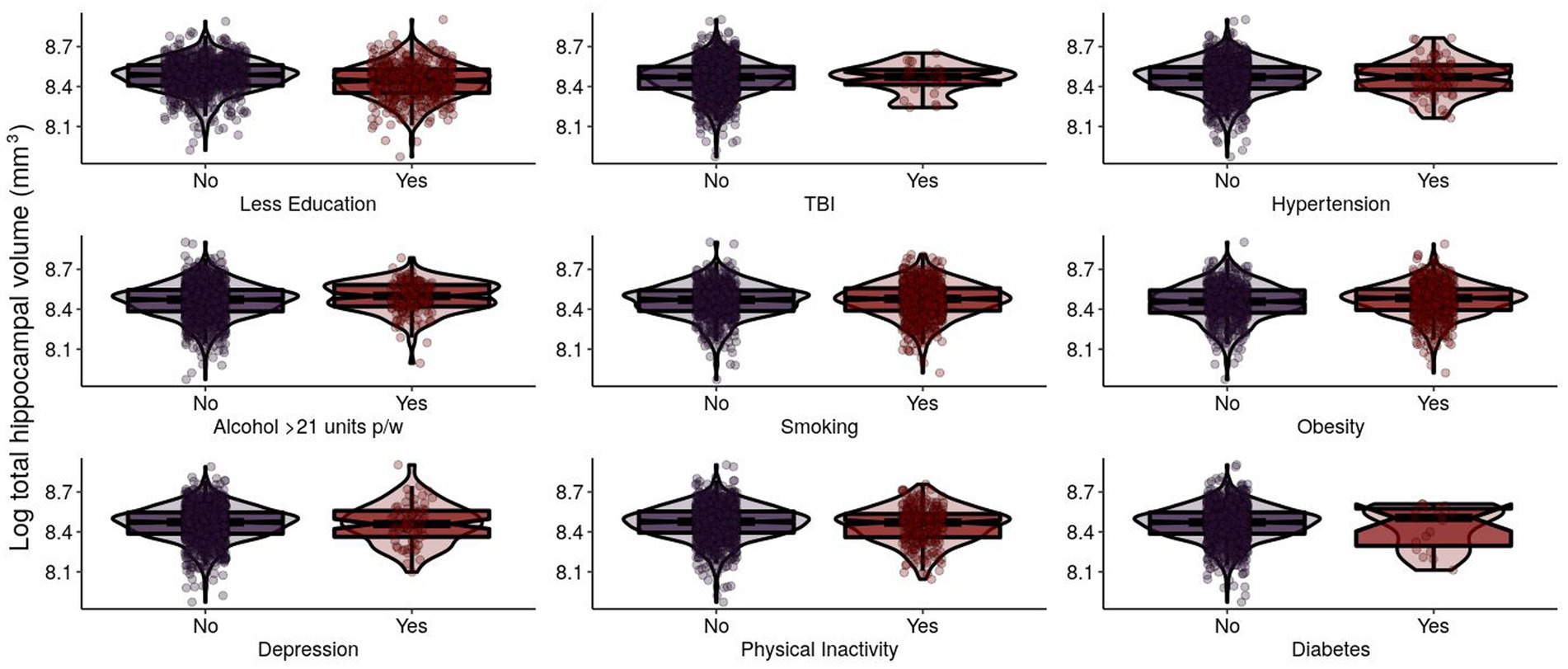
Figure 3. Log-transformed total hippocampal volume (mm3) of EPAD participants grouped by adherence to modifiable risk factors. Log-transformed levels of total hippocampal volume (mm3) are presented. Total hippocampal volume (THV) is the sum of left hippocampal volume (LHV) and right hippocampal volume (RHV). EPAD, European Prevention of Alzheimer’s Dementia; TBI, traumatic brain injury.
Discussion
In a large-scale community cohort of cognitively healthy participants from the EPAD study, we found significant relationships between modifiable risk factors for dementia and the hallmark AT(N) biomarkers that precede clinical symptoms of AD and other forms of dementia. Self-reported history of TBI was significantly associated with lower levels of Aβ1-42 levels in CSF; physical inactivity and lower BMI were significantly associated with higher levels of p-tau 181 in CSF; and higher education and higher BMI were both significantly associated with higher THV.
We found that the history of TBI was significantly associated with lower CSF Aβ1-42. Even after adjusting for age, sex, APOE ε4 presence, and cognition, the relationship remained, which is concordant with previous research into post-mortem Aβ plaque pathology following survivors of TBI (Johnson et al., 2012) and acute ventricular CSF Aβ1-42 elevations in the initial week following severe TBI (Olsson et al., 2004). Lower CSF Aβ1-42 could indicate reduced clearance of Aβ1-42, which has been hypothesized to be a result of amyloid accumulation in the brain (Tarasoff-Conway et al., 2015). Therefore, TBI events may be causing acute increases in amyloid production. However, several studies did not find an association between TBI and CSF (Neselius et al., 2012; Alosco et al., 2018; Weiner et al., 2022) and cerebral (Hicks et al., 2022) Aβ. Following a TBI event, axonal injury results in the accumulation of amyloid precursor protein, leading to intra-axonal Aβ aggregation and potentially Aβ aggregation and plaque formation. For this reason, CSF Aβ has been suggested as a potential biomarker for TBI (Tsitsopoulos and Marklund, 2013). There may also be other biological mechanisms at play in this pathway; for example, hypoperfusion, vascular dysfunction, and ischemia post-TBI may all contribute to Aβ deposition (Ramos-Cejudo et al., 2018). We found that age, APOE ε4, and cognition were all significantly associated with CSF Aβ1-42. Furthermore, the prevalence of TBI did not significantly differ between men (2.5%) and women (1.9%), indicating that the biological pathway operates independently of sex; yet, it may be mediated by a genetic predisposition for AD via APOE ε4 pathways (Jellinger et al., 2001).
Physical inactivity and lower BMI were both significantly associated with higher levels of p-tau 181 in CSF. Evidence suggests that exercise plays a critical role in reducing the accumulation of tau pathology and may be most beneficial in the preclinical phases of AD (Brown et al., 2019). In a study of cognitively normal participants, higher levels of self-reported physical activity predicted lower levels of CSF p-tau (Baker et al., 2012). Other research has found similar results with physical activity measured via actigraphy, where more time spent in moderate physical activity was significantly associated with lower levels of CSF p-tau (Law et al., 2018). On the other hand, several studies found different results: either the results attenuated after adjusting for covariates (Liang et al., 2010) or a lack of an association was observed in cognitively normal participants (Stojanovic et al., 2020; Roccati et al., 2023), pre-symptomatic autosomal-dominant AD (ADAD) (Brown et al., 2017), or AD patients via a 16-week moderate–high intense physical activity intervention (Steen Jensen et al., 2016). In our study, we found physical inactivity was significantly associated with higher levels of CSF p-tau. This relationship was significant even when adjusting for age, sex, cognition, and presence of APOE ε4. There are several potential mechanisms to explain this relationship. Physical activity has been shown to elicit a number of positive impacts on the brain, including an increase in levels of growth factors such as brain-derived neurotrophic factor (BDNF), altered inflammation, neurogenesis, and increased energy supply (Chen and Nakagawa, 2023). The relationship between these improvements and tau phosphorylation is less clear but may be due in part to increased clearance or altered production pathways. In this regard, the normal role of tau phosphorylation at specific sites, which occurs through a number of different kinase pathways such as glycogen synthase kinase 3 (GSK3) and cyclin-dependent kinase 5 (CDK5), is neither fully understood nor are the alterations in function that occur in disease states. APOE ε4 is a mediator of numerous pathological processes related to AD risk. APOE increases tau hyperphosphorylation, yet APOE ε4 carriers and non-carriers show similar benefits to brain health as a result of engagement in physical activity (Pearce et al., 2022). Given physical activity engagement entails a suite of biological processes, it is likely that many of these molecular targets align with AD neuropathology and therefore require further investigation (de Frutos Lucas et al., 2023). We also found lower BMI was associated with higher levels of p-tau 181 in CSF, where the prevailing evidence seems to indicate an association (Mathys et al., 2017; Bos et al., 2019; Zhang et al., 2022) rather than a lack of association (Pegueroles et al., 2020; Sun et al., 2020). There is considerable literature concerning an age-related risk matrix (Vidoni et al., 2011; Besser et al., 2016; Gottesman et al., 2017; Müller et al., 2017; Bos et al., 2019), a so-called “obesity paradox”, where lower BMI in midlife is associated with decreased AD risk while lower BMI in later life is associated with an increased risk. This paradox pattern appears to continue into the preclinical AD stage as indicated by biomarkers, where lower BMI has been associated with higher levels of CSF p-tau 181 in midlife (Mathys et al., 2017), and later life obesity has been linked with lower levels of CSF p-tau (Zhang et al., 2022).
We found that having more education and a higher BMI were both significantly associated with higher THV. Systematic review and meta-analysis from 45 observational, cross-sectional, epidemiological studies have demonstrated a clear association between higher BMI and lower brain volume (Han et al., 2021). However, there is some longitudinal evidence that changes in body weight are not related to hippocampal volume in later-life participants (Giudici et al., 2019). In our EPAD participants (mean age 66 years at baseline), higher BMI was associated with higher THV, adjusting for age and cognition, which were both significant. Previous research has found that midlife obesity (BMI > 30) was associated with an increased rate of hippocampal atrophy and global brain atrophy in cognitively normal individuals (Debette et al., 2011); however, in patients with AD, a negative correlation was observed (Ho et al., 2011). Given these findings and ours, it may be that the relationship between BMI and neurodegeneration is particularly important in midlife, where a higher BMI is associated with lower brain volume. This relationship is less important, however, in later life when changes in body weight do not seem to impact longitudinal changes in hippocampal volume.
In this study, we have shown how several key modifiable risk factors are associated with the AD hallmark AT(N) biomarkers. This study has substantial implications in clinical settings, where routine screening tests could use modifiable dementia risk factor profiles to assess risk, severity, and potential therapeutic interventions. Given modifiable risk factors often cluster together, addressing them in designated memory clinics could be a one-stop-shop for lifestyle modification, where we know precision medicine has the potential to catalyze positive behavior change (Freling et al., 2020). There are several strengths of our study of note. To the best of our knowledge, this is the first comprehensive investigation of modifiable dementia risk factors and AT(N) biomarkers. We used an open-source data repository from a large-scale, highly characterized, epidemiological cohort study, and our data and code are freely accessible and available to be reproduced. There are also several limitations. This was an observational study on existing longitudinal epidemiological data, and as such, no causal relationship can be construed. All participants were cognitively healthy at baseline; therefore, we were unable to include clinical staging on AT(N) instead of opting to adjust for cognition (MMSE) and age as proxies. Most self-reported measures were dichotomous, and hearing loss was omitted due to insufficient values for GAM comparison. Furthermore, the self-reported nature of medical history and risk factor adherence may have imparted bias to null, especially for participants who have experienced a TBI (McKinlay et al., 2016), where we were unable to account for injury severity. However, for the application of biomarker and risk factor interpretation in designated memory clinics or routine clinical practice, self-reported measures are commonplace and concordant with objective measures of risk. We were also unable to account for racial status as, in the EPAD LCS cohort, the participants were predominantly white Caucasian of European descent and not necessarily representative of the general population, which may limit the generalizability of our findings to other racial groups. Cognition was accounted for in our GAMs by adjusted for MMSE scores, which is a brief cognitive screening tool and not necessarily sensitive to precisely detecting early cognitive decline and individuals at risk (Gallegos et al., 2022). Finally, we acknowledge how modifiable risk factors have a tendency to cluster (Peters et al., 2019); however, this was outside the scope of our investigation. There is growing evidence that individual risk factors tend to interact, potentially leading to an underestimation of population attributable fraction (Welberry et al., 2023). Further research is necessary to elucidate the role of clustering risk factors and their impact on AT(N) biomarkers.
In conclusion, this study found that TBI, physical inactivity, lower BMI, and lower education were all significantly associated with increased burden of individual AT(N) biomarkers. Lifestyle modification offers an accessible, cost-effective, non-invasive, and easily targeted avenue for risk reduction. Given the strong evidence for modifiable risk factors being associated with AD incidence and prevalence, here we have shown significant relationships between several key modifiable dementia risk factors and the hallmark biomarkers of AD.
Data availability statement
Publicly available datasets were analyzed in this study. This data can be found at: All EPAD data is available via the NTKApp on the AD Workbench, designed by ADDI. NTKApp is accessible at: https://www.alzheimersdata.org/ntk. R code is available upon request to the corresponding author, ER (ZWRkeS5yb2NjYXRpQHV0YXMuZWR1LmF1).
Ethics statement
The studies involving humans were approved by the ethics committees of all participating EPAD centers. All procedures were conducted in accordance with the Declaration of Helsinki. The studies were conducted in accordance with the local legislation and institutional requirements. The participants provided their written informed consent to participate in this study.
Author contributions
ER: Conceptualization, Formal analysis, Investigation, Methodology, Resources, Visualization, Writing – original draft, Writing – review & editing. AB: Conceptualization, Formal analysis, Investigation, Methodology, Visualization, Writing – original draft, Writing – review & editing. JC: Formal analysis, Investigation, Writing – review & editing. JE: Formal analysis, Investigation, Writing – review & editing. JB: Formal analysis, Investigation, Writing – review & editing. JA: Writing – review & editing. AK: Writing – review & editing. JV: Supervision, Writing – review & editing. MC: Writing – review & editing. CL: Writing – review & editing.
Funding
The author(s) declare financial support was received for the research, authorship, and/or publication of this article. EPAD has received support from the EU/EFPIA Innovative Medicines Initiative Joint Undertaking EPAD grant agreement number 115736.
Acknowledgments
The authors would like to sincerely thank the EPAD participants for their contribution. This manuscript is the result of an international collaboration stemming from the ADDI NTK Hackathon held on July 2022, with winners announced at the Alzheimer’s Association International Conference (AAIC), August 2022. Team Wicking is made up of authors ER, AB, JB, and JE, won the Patient Value and Clinical Impact category, and would like to thank ADDI for the opportunity to compete in this initiative. The authors would also like to thank the sponsors of this event, ADDI, Roche, Nagarro, and Aridhia DRE.
Conflict of interest
MC is a full-time employee of Roche Diagnostics International Ltd, Rotkreuz, Switzerland and holds shares in F. Hoffman-La Roche. CL is a full-time employee of Roche Diagnostics GmbH, Penzberg, Germany. ELECSYS is a trademark of Roche.
The remaining authors declare that the research was conducted in the absence of any commercial or financial relationships that could be construed as a potential conflict of interest.
The author(s) declared that they were an editorial board member of Frontiers, at the time of submission. This had no impact on the peer review process and the final decision.
Publisher’s note
All claims expressed in this article are solely those of the authors and do not necessarily represent those of their affiliated organizations, or those of the publisher, the editors and the reviewers. Any product that may be evaluated in this article, or claim that may be made by its manufacturer, is not guaranteed or endorsed by the publisher.
Supplementary material
The Supplementary material for this article can be found online at: https://www.frontiersin.org/articles/10.3389/fnagi.2024.1346214/full#supplementary-material
References
Alosco, M. L., Tripodis, Y., Fritts, N. G., Heslegrave, A., Baugh, C. M., Conneely, S., et al. (2018). Cerebrospinal fluid tau, Aβ, and sTREM2 in former National Football League Players: modeling the relationship between repetitive head impacts, microglial activation, and neurodegeneration. Alzheimers Dement. 14, 1159–1170. doi: 10.1016/j.jalz.2018.05.004
Alzheimer’s Disease Neuroimaging InitiativeSun, Z., Wang, Z. T., Sun, F. R., Shen, X. N., Xu, W., et al. (2020). Late-life obesity is a protective factor for prodromal Alzheimer’s disease: a longitudinal study. Aging (Albany NY) 12, 2005–2017. doi: 10.18632/aging.102738
Baker, L. D., Bayer-Carter, J. L., Skinner, J., Montine, T. J., Cholerton, B. A., Callaghan, M., et al. (2012). High-intensity physical activity modulates diet effects on cerebrospinal amyloid-β levels in normal aging and mild cognitive impairment. J. Alzheimers Dis. 28, 137–146. doi: 10.3233/JAD-2011-111076
Besser, L. M., Alosco, M. L., Ramirez Gomez, L., Zhou, X. H., McKee, A. C., Stern, R. A., et al. (2016). Late-life vascular risk factors and Alzheimer disease neuropathology in individuals with normal cognition. J. Neuropathol. Exp. Neurol. 75, 955–962. doi: 10.1093/jnen/nlw072
Bos, I., Vos, S. J. B., Schindler, S. E., Hassenstab, J., Xiong, C., Grant, E., et al. (2019). Vascular risk factors are associated with longitudinal changes in cerebrospinal fluid tau markers and cognition in preclinical Alzheimer's disease. Alzheimers Dement. 15, 1149–1159. doi: 10.1016/j.jalz.2019.04.015
Brown, B. M., Peiffer, J., and Rainey-Smith, S. R. (2019). Exploring the relationship between physical activity, beta-amyloid and tau: a narrative review. Ageing Res. Rev. 50, 9–18. doi: 10.1016/j.arr.2019.01.003
Brown, B. M., Sohrabi, H. R., Taddei, K., Gardener, S. L., Rainey-Smith, S. R., Peiffer, J. J., et al. (2017). Habitual exercise levels are associated with cerebral amyloid load in presymptomatic autosomal dominant Alzheimer's disease. Alzheimers Dement. 13, 1197–1206. doi: 10.1016/j.jalz.2017.03.008
Chen, C., and Nakagawa, S. (2023). Physical activity for cognitive health promotion: an overview of the underlying neurobiological mechanisms. Ageing Res. Rev. 86:101868. doi: 10.1016/j.arr.2023.101868
Coley, N., Giulioli, C., Aisen, P. S., Vellas, B., and Andrieu, S. (2022). Randomised controlled trials for the prevention of cognitive decline or dementia: a systematic review. Ageing Res. Rev. 82:101777. doi: 10.1016/j.arr.2022.101777
Debette, S., Seshadri, S., Beiser, A., Au, R., Himali, J. J., Palumbo, C., et al. (2011). Midlife vascular risk factor exposure accelerates structural brain aging and cognitive decline. Neurology 77, 461–468. doi: 10.1212/WNL.0b013e318227b227
de Frutos Lucas, J., Sewell, K. R., and García-Colomo, A., Markovic, S., Erickson, K. I., and Brown, B. M. (2023). How does apolipoprotein E genotype influence the relationship between physical activity and Alzheimer’s disease risk? A novel integrative model. Alzheimers Res. Ther. 15:22. doi: 10.1186/s13195-023-01170-4
Ebenau, J. L., Timmers, T., Wesselman, L. M. P., Verberk, I. M. W., Verfaillie, S. C. J., Slot, R. E. R., et al. (2020). ATN classification and clinical progression in subjective cognitive decline: the SCIENCe project. Neurology 95, e46–e58. doi: 10.1212/WNL.0000000000009724
Edwards, G. A., Gamez, N., Escobedo, G., Calderon, O., and Moreno-Gonzalez, I. (2019). Modifiable risk factors for Alzheimer’s disease. Front. Aging Neurosci. 11:146. doi: 10.3389/fnagi.2019.00146
Freling, T. H., Yang, Z., Saini, R., Itani, O. S., and Rashad Abualsamh, R. (2020). When poignant stories outweigh cold hard facts: a meta-analysis of the anecdotal bias. Organ. Behav. Hum. Decis. Process. 160, 51–67. doi: 10.1016/j.obhdp.2020.01.006
Frisoni, G. B., Altomare, D., Ribaldi, F., Villain, N., Brayne, C., Mukadam, N., et al. (2023). Dementia prevention in memory clinics: recommendations from the European task force for brain health services. Lancet Region Health–Europe 26:100576. doi: 10.1016/j.lanepe.2022.100576
Gallegos, M., Morgan, M. L., Cervigni, M., Martino, P., Murray, J., Calandra, M., et al. (2022). 45 years of the mini-mental state examination (MMSE): a perspective from ibero-america. Dement. Neuropsychol. 16, 384–387. doi: 10.1590/1980-5764-dn-2021-0097
Giudici, K. V., Guyonnet, S., Rolland, Y., Vellas, B., de Souto Barreto, P., Nourhashemi, F., et al. (2019). Body weight variation patterns as predictors of cognitive decline over a 5 year follow-up among community-dwelling elderly (MAPT study). Nutrients 11:ARTN 1371:1371. doi: 10.3390/nu11061371
Gottesman, R. F., Schneider, A. L. C., Zhou, Y., Coresh, J., Green, E., Gupta, N., et al. (2017). Association between midlife vascular risk factors and estimated brain amyloid deposition. JAMA 317, 1443–1450. doi: 10.1001/jama.2017.3090
Griffin, B., Sherman, K. A., Jones, M., and Bayl-Smith, P. (2014). The clustering of health behaviours in older Australians and its association with physical and psychological status, and sociodemographic indicators. Ann. Behav. Med. 48, 205–214. doi: 10.1007/s12160-014-9589-8
Grill, J. D., and Karlawish, J. (2022). Disclosing Alzheimer disease biomarker results to research participants. JAMA Neurol. 79, 645–646. doi: 10.1001/jamaneurol.2022.1307
Han, Y.-P., Tang, X., Han, M., Yang, J., Cardoso, M. A., Zhou, J., et al. (2021). Relationship between obesity and structural brain abnormality: accumulated evidence from observational studies. Ageing Res. Rev. 71:101445. ARTN 101445. doi: 10.1016/j.arr.2021.101445
Hicks, A. J., Ponsford, J. L., Spitz, G., Dore, V., Krishnadas, N., Roberts, C., et al. (2022). β-Amyloid and tau imaging in chronic traumatic brain injury: a cross-sectional study. Neurology 99, e1131–e1141. doi: 10.1212/WNL.0000000000200857
Ho, A. J., Raji, C. A., Saharan, P., DeGiorgio, A., Madsen, S. K., Hibar, D. P., et al. (2011). Hippocampal volume is related to body mass index in Alzheimer’s disease. Neuroreport 22, 10–14. doi: 10.1097/WNR.0b013e3283412868
Ingala, S., de Boer, C., Masselink, L. A., Vergari, I., Lorenzini, L., Blennow, K., et al. (2021). Application of the ATN classification scheme in a population without dementia: findings from the EPAD cohort. Alzheimers Dement. 17, 1189–1204. doi: 10.1002/alz.12292
Jack, C. R. Jr., Bennett, D. A., Blennow, K., Carrillo, M. C., Dunn, B., Haeberlein, S. B., et al. (2018). NIA-AA research framework: toward a biological definition of Alzheimer's disease. Alzheimers Dement. 14, 535–562. doi: 10.1016/j.jalz.2018.02.018
Jack, C. R. Jr., Wiste, H. J., Weigand, S. D., Therneau, T. M., Lowe, V. J., Knopman, D. S., et al. (2017). Defining imaging biomarker cut points for brain aging and Alzheimer's disease. Alzheimers Dement. 13, 205–216. doi: 10.1016/j.jalz.2016.08.005
Jellinger, K. A., Paulus, W., Wrocklage, C., and Litvan, I. (2001). Traumatic brain injury as a risk factor for Alzheimer disease. Comparison of two retrospective autopsy cohorts with evaluation of ApoE genotype. BMC Neurol. 1, 1–4. doi: 10.1186/1471-2377-1-3
Johnson, V. E., Stewart, W., and Smith, D. H. (2012). Widespread tau and amyloid-beta pathology many years after a single traumatic brain injury in humans. Brain Pathol. 22, 142–149. doi: 10.1111/j.1750-3639.2011.00513.x
Kezios, K. L., Zhang, A., Kim, S., Lu, P., Glymour, M. M., Elfassy, T., et al. (2022). Association of low Hourly Wages in middle age with faster memory decline in older age: evidence from the health and retirement study. Am. J. Epidemiol. 191, 2051–2062. doi: 10.1093/aje/kwac166
Kivipelto, M., Mangialasche, F., and Ngandu, T. (2018). Lifestyle interventions to prevent cognitive impairment, dementia and Alzheimer disease. Nat. Rev. Neurol. 14, 653–666. doi: 10.1038/s41582-018-0070-3
Law, L. L., Rol, R. N., Schultz, S. A., Dougherty, R. J., Edwards, D. F., Koscik, R. L., et al. (2018). Moderate intensity physical activity associates with CSF biomarkers in a cohort at risk for Alzheimer's disease. Alzheim. Dement. Diagn. Assess. Dis. Monit. 10, 188–195. doi: 10.1016/j.dadm.2018.01.001
Liang, K. Y., Mintun, M. A., Fagan, A. M., Goate, A. M., Bugg, J. M., Holtzman, D. M., et al. (2010). Exercise and Alzheimer's disease biomarkers in cognitively normal older adults. Ann. Neurol. 68, 311–318. doi: 10.1002/ana.22096
Livingston, G., Huntley, J., Sommerlad, A., Ames, D., Ballard, C., Banerjee, S., et al. (2020). Dementia prevention, intervention, and care: 2020 report of the lancet commission. Lancet 396, 413–446. doi: 10.1016/s0140-6736(20)30367-6
Livingston, G., Sommerlad, A., Orgeta, V., Costafreda, S. G., Huntley, J., Ames, D., et al. (2017). Dementia prevention, intervention, and care. Lancet 390, 2673–2734. doi: 10.1016/s0140-6736(17)31363-6
Mathys, J., Gholamrezaee, M., Henry, H., von Gunten, A., and Popp, J. (2017). Decreasing body mass index is associated with cerebrospinal fluid markers of Alzheimer's pathology in MCI and mild dementia. Exp. Gerontol. 100, 45–53. doi: 10.1016/j.exger.2017
McKinlay, A., Horwood, L. J., and Fergusson, D. M. (2016). Accuracy of self-report as a method of screening for lifetime occurrence of traumatic brain injury events that resulted in hospitalization. J. Int. Neuropsychol. Soc. 22, 717–723. doi: 10.1017/S1355617716000497
Moore, B., McDonald, M., Carlon, L., and O'Rourke, K. (2015). Reducing the prevalence of Alzheimer's disease: modifiable risk factors or social determinants of health. Sydney Alzheim. Aust. 30, ii102–ii115. doi: 10.1093/heapro/dav031
Morris, L. J., D'Este, C., Sargent-Cox, K., and Anstey, K. J. (2016). Concurrent lifestyle risk factors: clusters and determinants in an Australian sample. Prev. Med. 84, 1–5. doi: 10.1016/j.ypmed.2015.12.009
Mukadam, N., Anderson, R., Knapp, M., Wittenberg, R., Karagiannidou, M., Costafreda, S. G., et al. (2020). Effective interventions for potentially modifiable risk factors for late-onset dementia: a costs and cost-effectiveness modelling study. Lancet Healthy Longevity 1, e13–e20. doi: 10.1016/S2666-7568(20)30004-0
Müller, S., Preische, O., Sohrabi, H. R., Gräber, S., Jucker, M., Dietzsch, J., et al. (2017). Decreased body mass index in the preclinical stage of autosomal dominant Alzheimer’s disease. Sci. Rep. 7:1225. doi: 10.1038/s41598-017-01327-w
Neselius, S., Brisby, H., Theodorsson, A., Blennow, K., Zetterberg, H., and Marcusson, J. (2012). CSF-biomarkers in Olympic boxing: diagnosis and effects of repetitive head trauma. PLoS One 7:e33606. doi: 10.1371/journal.pone.0033606
Ngandu, T., Lehtisalo, J., Solomon, A., Levälahti, E., Ahtiluoto, S., Antikainen, R., et al. (2015). A 2 year multidomain intervention of diet, exercise, cognitive training, and vascular risk monitoring versus control to prevent cognitive decline in at-risk elderly people (FINGER): a randomised controlled trial. Lancet 385, 2255–2263. doi: 10.1016/S0140-6736(15)60461-5
Olsson, A., Csajbok, L., Ost, M., Höglund, K., Nylén, K., Rosengren, L., et al. (2004). Marked increase of β-amyloid (1–42) and amyloid precursor protein in ventricular cerebrospinal fluid after severe traumatic brain injury. J. Neurol. 251, 870–876. doi: 10.1007/s00415-004-0451-y
Pearce, A., Dewar, M., Marr, C., and Gow, A. J. (2022). Apolipoprotein E genotype moderation of the association between physical activity and brain health. A systematic review and Meta-analysis. Front. Aging Neurosci. 13:815439. doi: 10.3389/fnagi.2021.815439
Pegueroles, J., Pané, A., Vilaplana, E., Montal, V., Bejanin, A., Videla, L., et al. (2020). Obesity impacts brain metabolism and structure independently of amyloid and tau pathology in healthy elderly. Alzheim. Dement. Diagn. Assess. Dis. Monit. 12:e12052. doi: 10.1002/dad2.12052
Peters, R., Booth, A., Rockwood, K., Peters, J., D’Este, C., and Anstey, K. J. (2019). Combining modifiable risk factors and risk of dementia: a systematic review and meta-analysis. BMJ Open 9:e022846. doi: 10.1136/bmjopen-2018-022846
Ramos-Cejudo, J., Wisniewski, T., Marmar, C., Zetterberg, H., Blennow, K., de Leon, M. J., et al. (2018). Traumatic brain injury and Alzheimer's disease: the cerebrovascular link. EBioMedicine 28, 21–30. doi: 10.1016/j.ebiom.2018.01.021
Reiman, E. M., McKhann, G. M., Albert, M. S., Sperling, R. A., Petersen, R. C., and Blacker, D. (2011). Alzheimer's disease: implications of the updated diagnostic and research criteria. J. Clin. Psychiatry 72, 1190–1196. doi: 10.4088/JCP.10087co1c
Ritchie, C. W., Muniz-Terrera, G., Kivipelto, M., Solomon, A., Tom, B., and Molinuevo, J. L. (2020). The European prevention of Alzheimer’s dementia (EPAD) longitudinal cohort study: baseline data release V500. 0. J. Prev Alzheimers Dis. 7, 8–13. doi: 10.14283/jpad.2019.46
Roccati, E., Collins, J. M., Bindoff, A. D., Alty, J. E., Bartlett, L., King, A. E., et al. (2023). Modifiable risk factors for dementia, cognition, and plasma phosphorylated tau 181 in a large-scale cohort of Australian older adults. Neurobiol. Aging 131, 106–114. doi: 10.1016/j.neurobiolaging.2023.06.018
Rovio, S., Kåreholt, I., Helkala, E. L., Viitanen, M., Winblad, B., Tuomilehto, J., et al. (2005). Leisure-time physical activity at midlife and the risk of dementia and Alzheimer's disease. Lancet Neurol. 4, 705–711. doi: 10.1016/S1474-4422(05)70198-8
Solomon, A., Kivipelto, M., Molinuevo, J. L., Tom, B., and Ritchie, C. W.EPAD Consortium (2018). European prevention of Alzheimer’s dementia longitudinal cohort study (EPAD LCS): study protocol. BMJ Open 8:e021017. doi: 10.1136/bmjopen-2017-021017
Sperling, R. A., Aisen, P. S., Beckett, L. A., Bennett, D. A., Craft, S., Fagan, A. M., et al. (2011). Toward defining the preclinical stages of Alzheimer's disease: recommendations from the National Institute on Aging-Alzheimer's Association workgroups on diagnostic guidelines for Alzheimer's disease. Alzheimers Dement. 7, 280–292. doi: 10.1016/j.jalz.2011.03.003
Steen Jensen, C., Portelius, E., Siersma, V., Høgh, P., Wermuth, L., Blennow, K., et al. (2016). Cerebrospinal fluid amyloid beta and tau concentrations are not modulated by 16 weeks of moderate-to high-intensity physical exercise in patients with Alzheimer disease. Dement. Geriatr. Cogn. Disord. 42, 146–158. doi: 10.1159/000449408
Stojanovic, M., Jin, Y., Fagan, A. M., Benzinger, T. L., Hassenstab, J., Cruchaga, C., et al. (2020). Physical exercise and longitudinal trajectories in Alzheimer disease biomarkers and cognitive functioning. Alzheimer Dis. Assoc. Disord. 34, 212–219. doi: 10.1097/WAD.0000000000000385
Tarasoff-Conway, J. M., Carare, R. O., Osorio, R. S., Glodzik, L., Butler, T., Fieremans, E., et al. (2015). Clearance systems in the brain—implications for Alzheimer disease. Nat. Rev. Neurol. 11, 457–470. doi: 10.1038/nrneurol.2015.119
Tsitsopoulos, P. P., and Marklund, N. (2013). Amyloid-β peptides and tau protein as biomarkers in cerebrospinal and interstitial fluid following traumatic brain injury: a review of experimental and clinical studies. Front. Neurol. 4:79. doi: 10.3389/fneur.2013.00079
Vidoni, E., Townley, R. A., Honea, R. A., and Burns, J. M.For the Alzheimer's Disease Neuroimaging Initiative (2011). Alzheimer disease biomarkers are associated with body mass index. Neurology 77, 1913–1920. doi: 10.1212/WNL.0b013e318238eec1
Weiner, M. W., Harvey, D., Landau, S. M., Veitch, D. P., Neylan, T. C., Grafman, J. H., et al. (2022). Traumatic brain injury and post-traumatic stress disorder are not associated with Alzheimer's disease pathology measured with biomarkers. Alzheimers Dement. doi: 10.1002/alz.12712
Welberry, H. J., Tisdell, C. C., Huque, M. H., and Jorm, L. R. (2023). Have we been underestimating modifiable dementia risk? An alternative approach for calculating the combined population attributable fraction for modifiable dementia risk factors. Am. J. Epidemiol. 192, 1763–1771. doi: 10.1093/aje/kwad138
Yaffe, K. (2018). Modifiable risk factors and prevention of dementia: what is the latest evidence? JAMA Intern. Med. 178, 281–282. doi: 10.1001/jamainternmed.2017.7299
Keywords: Alzheimer’s disease, amyloid-beta, tau, neurodegeneration, modifiable dementia risk, biomarkers
Citation: Roccati E, Bindoff AD, Collins JM, Eastgate J, Borchard J, Alty J, King AE, Vickers JC, Carboni M and Logan C EPAD Consortium (2024) Modifiable dementia risk factors and AT(N) biomarkers: findings from the EPAD cohort. Front. Aging Neurosci. 16:1346214. doi: 10.3389/fnagi.2024.1346214
Edited by:
Teresa Wu, Arizona State University, United StatesReviewed by:
Hyunsoo Yoon, Yonsei University, Republic of KoreaJay Shah, Arizona State University, United States
Copyright © 2024 Roccati, Bindoff, Collins, Eastgate, Borchard, Alty, King, Vickers, Carboni and Logan. This is an open-access article distributed under the terms of the Creative Commons Attribution License (CC BY). The use, distribution or reproduction in other forums is permitted, provided the original author(s) and the copyright owner(s) are credited and that the original publication in this journal is cited, in accordance with accepted academic practice. No use, distribution or reproduction is permitted which does not comply with these terms.
*Correspondence: Eddy Roccati, ZWRkeS5yb2NjYXRpQHV0YXMuZWR1LmF1