- 1Magnetic Resonance Research Center (MRRC), Department of Diagnostic Radiology, Yale School of Medicine, New Haven, CT, USA
- 2Department of Psychiatry, Yale School of Medicine, New Haven, CT, USA
- 3Department of Psychology, Yale University, New Haven, CT, USA
- 4Child Study Center, Yale School of Medicine, New Haven, CT, USA
Tailoring treatments to the specific needs and biology of individual patients—personalized medicine—requires delineation of reliable predictors of response. Unfortunately, these have been slow to emerge, especially in neuropsychiatric disorders. We have recently described a real-time functional magnetic resonance imaging (rt-fMRI) neurofeedback protocol that can reduce contamination-related anxiety, a prominent symptom of many cases of obsessive-compulsive disorder (OCD). Individual response to this intervention is variable. Here we used patterns of brain functional connectivity, as measured by baseline resting-state fMRI (rs-fMRI), to predict improvements in contamination anxiety after neurofeedback training. Activity of a region of the orbitofrontal cortex (OFC) and anterior prefrontal cortex, Brodmann area (BA) 10, associated with contamination anxiety in each subject was measured in real time and presented as a neurofeedback signal, permitting subjects to learn to modulate this target brain region. We have previously reported both enhanced OFC/BA 10 control and improved anxiety in a group of subclinically anxious subjects after neurofeedback. Five individuals with contamination-related OCD who underwent the same protocol also showed improved clinical symptomatology. In both groups, these behavioral improvements were strongly correlated with baseline whole-brain connectivity in the OFC/BA 10, computed from rs-fMRI collected several days prior to neurofeedback training. These pilot data suggest that rs-fMRI can be used to identify individuals likely to benefit from rt-fMRI neurofeedback training to control contamination anxiety.
Introduction
Dysregulation of anxiety is a core component of many neuropsychiatric conditions. Obsessive-compulsive disorder (OCD) is characterized by intrusive obsessions, which are often associated with anxiety, and with repetitive compulsions that seek to control that anxiety (Jenike, 2004). One common presentation of OCD is characterized by extreme contamination anxiety, often triggered by thoughts or images of, or contact with, potential contaminates such as dirt, body secretions, or mold (Bloch et al., 2008). Improving control of contamination anxiety is a key step in improving the quality of life for many individuals with OCD.
Treatments for contamination anxiety and for OCD exist, but none are universally effective (Franklin and Foa, 2011). For individuals who do not respond to standard behavioral and pharmacological treatments, interventions that more directly modulate the specific brain regions whose dysfunction is implicated in the disorder may be of benefit. In extreme cases, this modulation is sometimes done through invasive procedures such as deep brain stimulation (Greenberg et al., 2010). Targeted brain modulation using neurofeedback via real-time functional magnetic resonance (rt-fMRI) may prove to be an alternative (Scheinost et al., 2013).
rt-fMRI neurofeedback involves monitoring the blood oxygenation level dependent (BOLD) signal, a measure of brain activity, and providing immediate feedback to the subject showing them how specific brain activity patterns are changing over time. This form of feedback can facilitate learned control over brain activity and associated behaviors (Sulzer et al., 2013; Ruiz et al., 2014). Neurofeedback training has shown promise as a potential treatment in several clinical disorders including addiction (Hanlon et al., 2013; Li et al., 2013), tinnitus (Haller et al., 2010), stroke (Sitaram et al., 2012), depression (Linden et al., 2012; Young et al., 2014), Parkinson’s Disease (Subramanian et al., 2011), and schizophrenia (Ruiz et al., 2013a,b). rt-fMRI neurofeedback can produce changes in brain function (Hampson et al., 2011; Harmelech et al., 2013) and related behaviors (Shibata et al., 2011). In individuals with significant but subclinical contamination anxiety, we have shown that neurofeedback of activity in the orbitofrontal cortex (OFC) and anterior prefrontal cortex Brodmann area (BA) 10 can reorganize functional brain networks associated with anxiety and reduce the anxiety produced by contamination-related stimuli (Scheinost et al., 2013).
Clinically, a trial of an intervention that ultimately proves ineffective carries substantial cost, in both time, resources, and ongoing patient suffering. How best to match an individual to an intervention is therefore a crucially important question. Predictors of response that can help with treatment selection in neuropsychiatric conditions such as OCD would be of enormous clinical value but have been slow to emerge.
Here, we ask whether resting-state fMRI (rs-fMRI) can predict response to neurofeedback training and, thus, potentially guide treatment selection in the future. Previous research suggests that imaging-based biomarkers can be used to predict performance with a brain-computer interface (Halder et al., 2013). rs-fMRI, in particular, provides a great opportunity for identifying biomarkers to aid clinical decisions, given that it can be collected in clinical populations without requiring any task performance and yet provides a wealth of information about brain function (Constable et al., 2013; Lee et al., 2013). To investigate whether brain connectivity at rest can predict reduction in contamination anxiety induced during a neurofeedback intervention, we correlated a voxel-wise measure of functional connectivity, computed from rs-fMRI collected prior to neurofeedback training, with behavioral response to the neurofeedback intervention in a cohort of healthy subjects with subclinical contamination anxiety (Scheinost et al., 2013). We then examined whether a similar relationship existed in a small cohort of patients.
Methods
Data were from studies performed at Yale University School of Medicine, New Haven, CT. All protocols were reviewed and approved by Human Research Protection Program at Yale University. Written informed consent was obtained. All scans were obtained and analyzed at Yale University.
Subjects
Two cohorts of subjects were used in this study. The first cohort has been described previously (Scheinost et al., 2013) and consisted of 10 subjects without any clinical diagnosis of OCD, but with high levels of contamination anxiety. Only the 10 subjects who received true neurofeedback in our previous study—not the 10 who received sham neurofeedback in the control condition—are included in the present analysis. The second cohort consisted of five OCD patients with moderate symptom severity (Table 1) and prominent contamination obsessions.
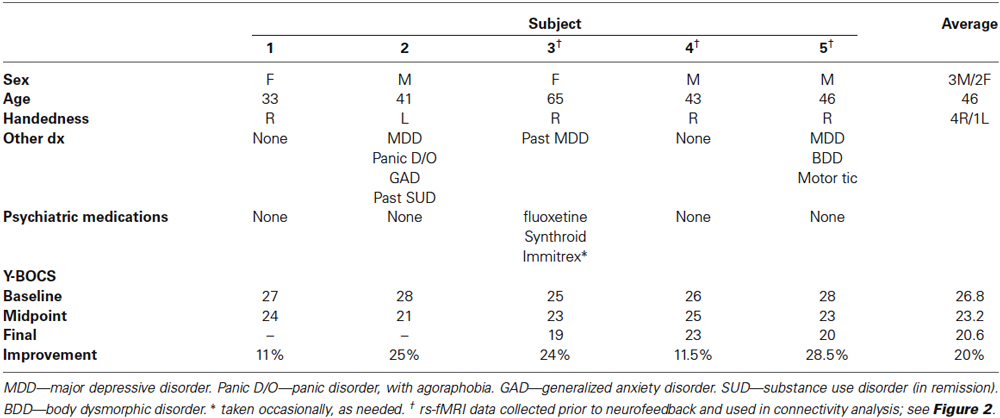
Table 1. Clinical characteristics and symptom improvement in five OCD patients who underwent rt-fMRI biofeedback.
Neurofeedback Training
Healthy subjects and OCD patients received neurofeedback training following a previously detailed protocol (Hampson et al., 2012a). Of the five OCD patients, the first two underwent only a single neurofeedback session, without pre- or post-neurofeedback resting-state scans. All other individuals participated in four separate MRI scanning sessions, spaced several days apart. In the first session, rs-fMRI data were collected and a functional localizer was used to identify the target area of the OFC/BA 10 region to be used for neurofeedback. The second and third sessions involved rt-fMRI neurofeedback training based on the target OFC/BA 10 region. A final session (not of relevance to this work) involved collecting post-intervention rs-fMRI data. The rs-fMRI data were always collected before any other functional scans in a given session to avoid possible effects of previous task on the rs-fMRI data.
The overlap of the target area for feedback for all 15 subjects is shown in Figure 1. Overlap was calculated by (1) smoothing the target region of each individual with a 6 mm Gaussian smoothing kernel to account for differences in functional anatomy and registration errors; (2) warping the target regions to a common reference; and (3) averaging across subjects the likelihood of a voxel being included in the target region.
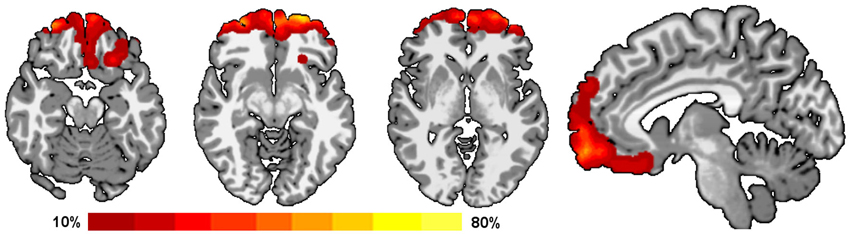
Figure 1. Overlap of target regions for neurofeedback. All subjects received neurofeedback from a region in the OFC/BA 10 (Hampson et al., 2012a). These target regions were determined on individual basis from a functional localizer task allowing for differences in individual functional anatomy. The percent overlap of all these target regions is shown on a template brain using Radiological convention (left is on the right for axial slices). Warmer colors indicate that the voxel was included in a greater number of individual target regions.
Behavioral Measures
Behavioral measures of control over contamination anxiety (for the first cohort) and clinical measures (for the second cohort) were collected before and after neurofeedback training. The pre-intervention assessment data was collected before the first neurofeedback session (second overall imaging session), immediately prior to the start of neurofeedback training. The post-intervention assessment data was collected several days after the completion of neurofeedback training, either in a separate session with no imaging (for the first two OCD patients) or in conjunction with the fourth fMRI session (for all other patients). Finally, midpoint assessment data was collected in between the first and second neurofeedback sessions for the subjects who received two sessions of neurofeedback.
For the healthy subjects, with subclinical contamination anxiety we used a behavioral measure designed to assess the subjects’ ability to control their anxiety. Subjects were instructed to try to control their anxiety while viewing 25 contamination-related images and to indicate their experienced anxiety for each image on a 1–5 scale. A rating of one indicated the least anxiety and a rating of five indicated the most anxiety. The ratings for the 25 contamination-related images were then averaged yielding a single measure of anxiety. Different sets of images were used before and after the intervention, but the sets were designed to induce similar levels of contamination related anxiety and piloted to verify that they were balanced in this respect (Hampson et al., 2012a).
For the patients, we administered a modified version of the Yale–Brown Obsessive Compulsive Scale (Y-BOCS), in which they were instructed to report on their symptoms over the last 3 days, rather than over the past week as in the traditional Y-BOCS (Goodman et al., 1989a,b). The Y-BOCS ranges from 0–40, with higher scores representing more severe symptoms, and measures the frequency, intrusiveness, and distress associated with obsessions and compulsions. Scores in the mid-twenties, as these patients had (Table 1), correspond to moderate to severe disease.
For both groups, change in behavior measures were calculated as score prior to neurofeedback minus score after neurofeedback, such that a positive change indicates an improvement in anxiety.
Imaging Parameters
All imaging was done on a 1.5-T Siemens Sonata scanner (Siemens Medical Systems, Erlangen, Germany). A sequence designed to optimize signal in the OFC was used for all functional data collection (repetition time = 2000 ms, echo time = 30 ms, flip angle = 80, bandwidth = 2604, 200 mm field of view for 3.1 mm isotropic voxels, 31 axial-oblique slices covering almost the whole cerebrum and most of the cerebellum). Two 5 min resting data runs were collected.
Resting-State Connectivity
Images were preprocessed using a previously detailed pipeline (Hampson et al., 2012b). All images were slice time and motion corrected using SPM.1 Unless otherwise specified, all further analysis was performed using BioImage Suite (Joshi et al., 2011). Several covariates of no interest were regressed from the data including linear and quadratic drift, six rigid-body motion parameters, mean cerebrospinal fluid (CSF) signal, mean white-matter signal, and mean global signal. The data were low-pass filtered via temporal smoothing with a 0 mean unit variance Gaussian filter (approximate cutoff frequency = 0.12 Hz). Finally, a gray matter mask was applied to the preprocessed data so that only voxels in the gray matter were used in subsequent calculations. After preprocessing, all resting-state runs were concatenated and the connectivity for each voxel was then calculated in each subject’s individual brain space.
The gray and white matter and CSF masks were defined on a template brain (Holmes et al., 1998), and warped to individual subject space using a series of transformations, described below. The gray matter mask was dilated to ensure full coverage of the gray matter after warping into individual subject space. Regions that were not included in all subjects’ data (for e.g., the bottom of the cerebellum) were excluded from analysis. Likewise, the white matter and CSF masks were eroded to ensure only pure white matter or CSF signal were regressed from the data.
Global functional connectivity of each voxel was measured from rs-fMRI data using the network theory measure degree (Bullmore and Sporns, 2009) as previously described (Martuzzi et al., 2011). The BOLD time course for each voxel was correlated with every other voxel in the gray matter. Two voxels were considered connected if correlation of their timecourses was greater than r = 0.25; the degree of each voxel was defined as the number of such connections. The process was repeated for every voxel in the gray matter. Each subject’s degree map was normalized by subtracting the mean across all voxels and dividing by the standard deviation across all voxels. This normalization has been shown to reduce the impact of confounds related to motion (Yan et al., 2013).
To facilitate comparisons of imaging data, all degree maps were spatially smoothed with a 6 mm Gaussian filter and then warped to a common template space through the concatenation of a series of linear and non-linear registrations, as previously described (Scheinost et al., 2013). All transformations were computed using the intensity-based registration algorithms in BioImage Suite (Papademetris et al., 2004).
Evaluating the Relationship Between Response to Intervention and rs-fMRI Data
To identify which brain regions predicted response to neurofeedback training, we related the rs-fMRI data acquired before any neurofeedback training with changes in the behavioral measure of control over contamination anxiety (for the healthy subjects) and changes in clinical severity (for the patients). For the healthy subjects, we performed a data-driven, whole-brain analysis by correlating the change in control of anxiety with the degree maps in a voxel-wise manner. Significance was assessed at a p < 0.05 level after correcting for multiple comparisons across the gray matter via AFNI’s AlphaSim program. From this voxel-wise analysis, we defined a region of interest (ROI) that showed significant effects in the healthy subjects to explore whether this finding translated to the smaller cohort of OCD patients. For the three OCD patients on whom pre-neurofeedback rs-fMRI was collected, degree averaged over all voxels in this ROI was extracted and related to changes in Y-BOCS scores.
Results
Imaging Predictors of Behavior in Subclinically Anxious Subjects
As reported previously (Scheinost et al., 2013), healthy subjects with subclinical contamination anxiety showed a significant (p < 0.05) increase in control over anxiety after neurofeedback training. Whole-brain connectivity analysis revealed a single significant cluster (p < 0.05 corrected; MNI coordinate of peak voxel: 0, 66, −4, max t-value = 5.84, cluster size = 5857 mm3) in which degree prior to neurofeedback training was significantly correlated with improved control over anxiety (Figure 2A). This cluster was located in the OFC/BA 10 target region. Subjects with the highest connectivity in this region prior to neurofeedback training exhibited the most improvement in post-treatment anxiety. A scatterplot of the average connectivity change in this region vs. the change in control of anxiety is shown in Figure 2B. As the choice of threshold used to consider whether two voxels are connected can impact connectivity results (Scheinost et al., 2012), we repeated this analysis over a range of thresholds (0.10 < r < 0.65). This produced no qualitative change in the findings. Additionally, as motion has been shown to confound functional connectivity results, average frame to frame displacement was calculated for each group (Van Dijk et al., 2012). Motion was not correlated with improved control of anxiety (r = 0.18, p > 0.60) and adding motion as a covariate in the group analysis did not change the presented results.
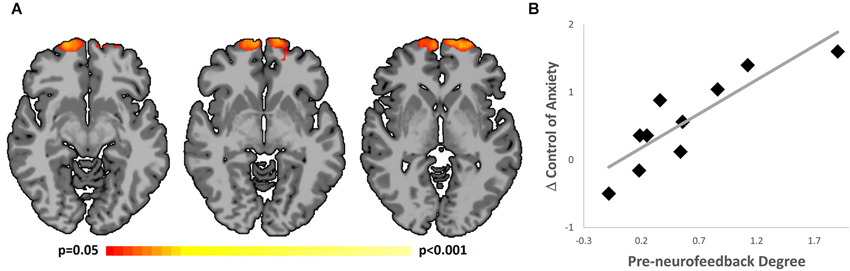
Figure 2. Correlation of improved control over anxiety and rs-fMRI. (A) Subjects with the highest connectivity in the OFC/BA 10 (MNI coordinate of peak voxel: 0, 66, −4) prior to neurofeedback training had the largest improvement in control over anxiety over the course of the intervention. Results shown using Radiological convention at p < 0.05 level, corrected for multiple comparisons. (B) Scatterplot showing improved control over anxiety and pre-neurofeedback rs-fMRI.
Clinical Improvement After Neurofeedback in Subjects with OCD
Five patients with moderate-to-severe OCD and prominent contamination symptoms underwent one or two sessions of neurofeedback (Table 1). All five tolerated the procedure well and exhibited reduced symptoms, as evaluated by the Y-BOCS several days after the last neurofeedback session. Average symptom improvement was 20%. Of the OCD patients, the three with the greatest symptom improvements also had a co-diagnosis or a history of major depressive disorder (MDD). Demographic and clinical details are given in Table 1.
Imaging Predictors of Clinical Improvement
Next, we tested whether a similar relationship between connectivity and behavioral improvements would be found in OCD patients. Pre-neurofeedback rs-fMRI was not measured on the first two subjects; this analysis was therefore performed only on the three subjects who underwent the full two-session neurofeedback protocol. To maximize power in this very limited dataset, we used the OFC/BA 10 region defined in the first cohort as an a priori ROI, Average degree in this ROI prior to neurofeedback training was related to clinical improvement for the three patients. Consistent with the pattern seen in the healthy subjects, a strong linear relationship was observed (r = 0.99). Thus, in both groups, increased connectivity in the OFC/BA 10 measured from rs-fMRI data collected prior to neurofeedback training was associated with greater behavioral improvements.
Discussion
Advances in understanding individual differences motivate a new approach to health care in which treatment is tailored to the specific needs and biology of an individual patient. This “personalized medicine” approach has been endorsed by the National Institute of Mental Health, NIMH,2 but its adoption depends critically on our ability to identify which patients are likely to respond to which interventions. rs-fMRI holds great promise as a tool for providing this information. It is easy to collect, does not require patients to perform any difficult tasks, and yet is a rich source of potentially clinically relevant information about brain function (Constable et al., 2013; Lee et al., 2013).
In a pilot study, we demonstrate, for the first time, that rs-fMRI can be a useful tool to predict response to neurofeedback training via rt-fMRI. After receiving two sessions of neurofeedback training, healthy subjects showed improved control over anxiety and OCD patients showed a reduction in OCD symptom severity. For both groups, these behavioral improvements were strongly correlated with the pre-intervention level of whole-brain connectivity in the anterior prefrontal cortex.
The resting state functional connectivity analysis used in this study was unbiased by a priori expectations regarding regions of interest. Therefore, it is striking that the region that emerged from our whole-brain analysis as most relevant for predicting improvements in contamination anxiety was in our target area of the OFC/BA 10. Taken together with a large body of data highlighting the importance of the OFC and anterior prefrontal cortex in obsessive-compulsive symptoms (Swedo et al., 1992; Chamberlain et al., 2008; Menzies et al., 2008; Harrison et al., 2009; Sakai et al., 2011; Anticevic et al., 2014; Beucke et al., 2013), this gives us confidence that we are targeting a biologically relevant brain area.
Notably, OFC/BA 10 connectivity predicted the response to the intervention in both healthy subjects and OCD patients, suggesting a shared neurobiological mechanism for improved control over contamination anxiety across groups. It is possible that the phenomenon of contamination anxiety is a dimensional construct, differing in a quantitative rather than a qualitative sense in patients when compared to healthy individuals. Supporting this view are previous reports of OFC/BA 10 activations to contamination related imagery in both healthy subjects and OCD patients (Mataix-Cols et al., 2003, 2004).
To the extent that neurobiology of a phenomenon is shared across patients and healthy subjects, interventions developed in the healthy group are likely to translate into the patient population. In this particular intervention, based on our preliminary patient data, translational potential appears high. A variety of other applications of rt-fMRI neurofeedback trainings have been developed in healthy populations (Hampson et al., 2011; Shibata et al., 2011; Chiew et al., 2012; Garrison et al., 2013). It will be interesting to see how well the findings from these studies translate into clinical populations. If the dimensional approach implicit in NIMHs Research Domain Criteria3 is an accurate description of pathological brain dysfunction, many of these studies may successfully translate into the respective patient groups.
An important consideration for predictive validity is the reliability of rs-fMRI. Overall, graph theory measures have been shown to be reliable (Telesford et al., 2010; Braun et al., 2012) and, in particular, voxel-wise degree has shown good test-retest reproducibility across different sites and scanners (Tomasi and Volkow, 2010). While generally reliable, a variety of factors can reduce the predictive power of rs-fMRI. Medications and other drugs such as caffeine can alter connectivity patterns (Rack-Gomer et al., 2009; Martuzzi et al., 2010). Sleep also changes connectivity patterns (Tagliazucchi et al., 2013) which can be an issue if subjects are falling asleep and not reporting it. Finally, factors related to subject comfort such as hunger may reduce data quality and prediction accuracy due to motion artifacts and effects on subject compliance. The degree to which all these variables are controlled is likely to affect the power of future studies to identify clinically relevant biomarkers that predict treatment response.
The major limitation of this pilot study is the small number of subjects, particularly in the patient group, in which we only had three subjects with resting data. Although the finding in the healthy subject group is statistically significant, the finding in the patient group must be considered preliminary. However, the tight correspondence between connectivity and intervention response in our modest clinical sample, and its similarity to the relationship seen in healthy subjects, are promising. Future studies are needed to rigorously examine whether this biomarker is an effective predictor of response in the clinical group. A large study that can examine possible modulating variables would be particularly valuable. For example, the data in our small sample suggest that patients with a current co-diagnosis or a history of MDD show the greatest improvement in clinical symptoms, but we were unable to investigate this given our limited data in the patient group. A study with the power to test that possibility could yield interesting insights.
Conclusion
These pilot data provide evidence that rs-fMRI connectivity can be used to identify individuals likely to benefit from rt-fMRI neurofeedback interventions for training control over contamination anxiety. Specifically, we have identified a biomarker that may be useful in developing personalized treatment programs in patients with OCD. More generally, these findings illustrate the potential utility of rs-fMRI data for identifying biomarkers of treatment response and thereby facilitating a personalized medicine approach to treating mental illness.
Conflict of Interest Statement
The authors declare that the research was conducted in the absence of any commercial or financial relationships that could be construed as a potential conflict of interest.
Acknowledgments
This research was supported by NIH grant R21 MH090384, The Taylor Foundation for Chronic Disease, and this publication was made possible by CTSA Grant Number UL1 TR000142 from the National Center for Advancing Translational Science (NCATS), a component of the National Institutes of Health (NIH). Its contents are solely the responsibility of the authors and do not necessarily represent the official view of NIH. We thank H. Sarofin for her technical assistance and E. Billingslea for her assistance with coordinating OCD patient recruitment, characterization, and logistics.
Footnotes
- ^ www.fil.ion.ucl.ac.uk/spm/
- ^ www.nimh.nih.gov/about/strategic-planning-reports/index.shtml
- ^ www.nimh.nih.gov/research-priorities/rdoc/nimh-research-domain-criteria-rdoc.shtml
References
Anticevic, A., Hu, S., Zhang, S., Savic, A., Billingslea, E., Wasylink, S., et al. (2014). Global resting-state functional magnetic resonance imaging analysis identifies frontal cortex, striatal and cerebellar dysconnectivity in obsessive-compulsive disorder. Biol. Psychiatry 75, 595–605. doi: 10.1016/j.biopsych.2013.10.021
Pubmed Abstract | Pubmed Full Text | CrossRef Full Text | Google Scholar
Beucke, J. C., Sepulcre, J., Talukdar, T., Linnman, C., Zschenderlein, K., Endrass, T., et al. (2013). Abnormally high degree connectivity of the orbitofrontal cortex in obsessive-compulsive disorder. JAMA Psychiatry 70, 619–629. doi: 10.1001/jamapsychiatry.2013.173
Pubmed Abstract | Pubmed Full Text | CrossRef Full Text | Google Scholar
Bloch, M. H., Landeros-Weisenberger, A., Rosario, M. C., Pittenger, C., and Leckman, J. F. (2008). Meta-analysis of the symptom structure of obsessive-compulsive disorder. Am. J. Psychiatry 165, 1532–1542. doi: 10.1176/appi.ajp.2008.08020320
Pubmed Abstract | Pubmed Full Text | CrossRef Full Text | Google Scholar
Braun, U., Plichta, M. M., Esslinger, C., Sauer, C., Haddad, L., Grimm, O., et al. (2012). Test-retest reliability of resting-state connectivity network characteristics using fMRI and graph theoretical measures. Neuroimage 59, 1404–1412. doi: 10.1016/j.neuroimage.2011.08.044
Pubmed Abstract | Pubmed Full Text | CrossRef Full Text | Google Scholar
Bullmore, E., and Sporns, O. (2009). Complex brain networks: graph theoretical analysis of structural and functional systems. Nat. Rev. Neurosci. 10, 186–198. doi: 10.1038/nrn2575
Pubmed Abstract | Pubmed Full Text | CrossRef Full Text | Google Scholar
Chamberlain, S. R., Menzies, L., Hampshire, A., Suckling, J., Fineberg, N. A., del Campo, N., et al. (2008). Orbitofrontal dysfunction in patients with obsessive-compulsive disorder and their unaffected relatives. Science 321, 421–422. doi: 10.1126/science.1154433
Pubmed Abstract | Pubmed Full Text | CrossRef Full Text | Google Scholar
Chiew, M., LaConte, S. M., and Graham, S. J. (2012). Investigation of fMRI neurofeedback of differential primary motor cortex activity using kinesthetic motor imagery. Neuroimage 61, 21–31. doi: 10.1016/j.neuroimage.2012.02.053
Pubmed Abstract | Pubmed Full Text | CrossRef Full Text | Google Scholar
Constable, R. T., Scheinost, D., Finn, E. S., Shen, X., Hampson, M., Winstanley, F. S., et al. (2013). Potential use and challenges of functional connectivity mapping in intractable epilepsy. Front. Neurol. 4:39. doi: 10.3389/fneur.2013.00039
Pubmed Abstract | Pubmed Full Text | CrossRef Full Text | Google Scholar
Franklin, M. E., and Foa, E. B. (2011). Treatment of obsessive compulsive disorder. Annu. Rev. Clin. Psychol. 7, 229–243. doi: 10.1146/annurev-clinpsy-032210-104533
Pubmed Abstract | Pubmed Full Text | CrossRef Full Text | Google Scholar
Garrison, K. A., Scheinost, D., Worhunsky, P. D., Elwafi, H. M., Thornhill, T. A., Thompson, E., et al. (2013). Real-time fMRI links subjective experience with brain activity during focused attention. Neuroimage 81, 110–118. doi: 10.1016/j.neuroimage.2013.05.030
Pubmed Abstract | Pubmed Full Text | CrossRef Full Text | Google Scholar
Goodman, W. K., Price, L. H., Rasmussen, S. A., Mazure, C., Delgado, P., Heninger, G. R., et al. (1989a). The yale-brown obsessive compulsive scale. II. Validity. Arch. Gen. Psychiatry 46, 1012–1016. doi: 10.1001/archpsyc.1989.01810110054008
Pubmed Abstract | Pubmed Full Text | CrossRef Full Text | Google Scholar
Goodman, W. K., Price, L. H., Rasmussen, S. A., Mazure, C., Fleischmann, R. L., Hill, C. L., et al. (1989b). The yale-brown obsessive compulsive scale. I. Development, use and reliability. Arch. Gen. Psychiatry 46, 1006–1011. doi: 10.1001/archpsyc.1989.01810110048007
Pubmed Abstract | Pubmed Full Text | CrossRef Full Text | Google Scholar
Greenberg, B. D., Rauch, S. L., and Haber, S. N. (2010). Invasive circuitry-based neurotherapeutics: stereotactic ablation and deep brain stimulation for OCD. Neuropsychopharmacology 35, 317–336. doi: 10.1038/npp.2009.128
Pubmed Abstract | Pubmed Full Text | CrossRef Full Text | Google Scholar
Halder, S., Varkuti, B., Bogdan, M., Kübler, A., Rosenstiel, W., Sitaram, R., et al. (2013). Prediction of brain-computer interface aptitude from individual brain structure. Front. Hum. Neurosci. 7:105. doi: 10.3389/fnhum.2013.00105
Pubmed Abstract | Pubmed Full Text | CrossRef Full Text | Google Scholar
Haller, S., Birbaumer, N., and Veit, R. (2010). Real-time fMRI feedback training may improve chronic tinnitus. Eur. Radiol. 20, 696–703. doi: 10.1007/s00330-009-1595-z
Pubmed Abstract | Pubmed Full Text | CrossRef Full Text | Google Scholar
Hampson, M., Scheinost, D., Qiu, M., Bhawnani, J., Lacadie, C. M., Leckman, J. F., et al. (2011). Biofeedback of real-time functional magnetic resonance imaging data from the supplementary motor area reduces functional connectivity to subcortical regions. Brain Connect. 1, 91–98. doi: 10.1089/brain.2011.0002
Pubmed Abstract | Pubmed Full Text | CrossRef Full Text | Google Scholar
Hampson, M., Stoica, T., Saksa, J., Scheinost, D., Qiu, M., Bhawnani, J., et al. (2012a). Real-time fMRI biofeedback targeting the orbitofrontal cortex for contamination anxiety. J. Vis. Exp. 3535. doi: 10.3791/3535
Pubmed Abstract | Pubmed Full Text | CrossRef Full Text | Google Scholar
Hampson, M., Tokoglu, F., Shen, X., Scheinost, D., Papademetris, X., and Constable, R. T. (2012b). Intrinsic brain connectivity related to age in young and middle aged adults. PLoS One 7:e44067. doi: 10.1371/journal.pone.0044067
Pubmed Abstract | Pubmed Full Text | CrossRef Full Text | Google Scholar
Hanlon, C. A., Hartwell, K. J., Canterberry, M., Li, X., Owens, M., Lematty, T., et al. (2013). Reduction of cue-induced craving through realtime neurofeedback in nicotine users: the role of region of interest selection and multiple visits. Psychiatry Res. 213, 79–81. doi: 10.1016/j.pscychresns.2013.03.003
Pubmed Abstract | Pubmed Full Text | CrossRef Full Text | Google Scholar
Harmelech, T., Preminger, S., Wertman, E., and Malach, R. (2013). The day-after effect: long term, Hebbian-like restructuring of resting-state fMRI patterns induced by a single epoch of cortical activation. J. Neurosci. 33, 9488–9497. doi: 10.1523/JNEUROSCI.5911-12.2013
Pubmed Abstract | Pubmed Full Text | CrossRef Full Text | Google Scholar
Harrison, B. J., Soriano-Mas, C., Pujol, J., Ortiz, H., López-Solà, M., Hernández-Ribas, R., et al. (2009). Altered corticostriatal functional connectivity in obsessive-compulsive disorder. Arch. Gen. Psychiatry 66, 1189–1200. doi: 10.1001/archgenpsychiatry.2009.152
Pubmed Abstract | Pubmed Full Text | CrossRef Full Text | Google Scholar
Holmes, C. J., Hoge, R., Collins, L., Woods, R., Toga, A. W., and Evans, A. C. (1998). Enhancement of MR images using registration for signal averaging. J. Comput. Assist. Tomogr. 22, 324–333. doi: 10.1097/00004728-199803000-00032
Pubmed Abstract | Pubmed Full Text | CrossRef Full Text | Google Scholar
Jenike, M. A. (2004). Clinical practice. Obsessive-compulsive disorder. N. Engl. J. Med. 350, 259–265. doi: 10.1056/NEJMcp031002
Pubmed Abstract | Pubmed Full Text | CrossRef Full Text | Google Scholar
Joshi, A., Scheinost, D., Okuda, H., Belhachemi, D., Murphy, I., Staib, L. H., et al. (2011). Unified framework for development, deployment and robust testing of neuroimaging algorithms. Neuroinformatics 9, 69–84. doi: 10.1007/s12021-010-9092-8
Pubmed Abstract | Pubmed Full Text | CrossRef Full Text | Google Scholar
Lee, M. H., Smyser, C. D., and Shimony, J. S. (2013). Resting-state fMRI: a review of methods and clinical applications. AJNR Am. J. Neuroradiol. 34, 1866–1872. doi: 10.3174/ajnr.A3263
Pubmed Abstract | Pubmed Full Text | CrossRef Full Text | Google Scholar
Li, X., Hartwell, K. J., Borckardt, J., Prisciandaro, J. J., Saladin, M. E., Morgan, P. S., et al. (2013). Volitional reduction of anterior cingulate cortex activity produces decreased cue craving in smoking cessation: a preliminary real-time fMRI study. Addict. Biol. 18, 739–748. doi: 10.1111/j.1369-1600.2012.00449.x
Pubmed Abstract | Pubmed Full Text | CrossRef Full Text | Google Scholar
Linden, D. E., Habes, I., Johnston, S. J., Linden, S., Tatineni, R., Subramanian, L., et al. (2012). Real-time self-regulation of emotion networks in patients with depression. PLoS One 7:e38115. doi: 10.1371/journal.pone.0038115
Pubmed Abstract | Pubmed Full Text | CrossRef Full Text | Google Scholar
Martuzzi, R., Ramani, R., Qiu, M., Rajeevan, N., and Constable, R. T. (2010). Functional connectivity and alterations in baseline brain state in humans. Neuroimage 49, 823–834. doi: 10.1016/j.neuroimage.2009.07.028
Pubmed Abstract | Pubmed Full Text | CrossRef Full Text | Google Scholar
Martuzzi, R., Ramani, R., Qiu, M., Shen, X., Papademetris, X., and Constable, R. T. (2011). A whole-brain voxel based measure of intrinsic connectivity contrast reveals local changes in tissue connectivity with anesthetic without a priori assumptions on thresholds or regions of interest. Neuroimage 58, 1044–1050. doi: 10.1016/j.neuroimage.2011.06.075
Pubmed Abstract | Pubmed Full Text | CrossRef Full Text | Google Scholar
Mataix-Cols, D., Cullen, S., Lange, K., Zelaya, F., Andrew, C., Amaro, E., et al. (2003). Neural correlates of anxiety associated with obsessive-compulsive symptom dimensions in normal volunteers. Biol. Psychiatry 53, 482–493. doi: 10.1016/s0006-3223(02)01504-4
Pubmed Abstract | Pubmed Full Text | CrossRef Full Text | Google Scholar
Mataix-Cols, D., Wooderson, S., Lawrence, N., Brammer, M. J., Speckens, A., and Phillips, M. L. (2004). Distinct neural correlates of washing, checking and hoarding symptom dimensions in obsessive-compulsive disorder. Arch. Gen. Psychiatry 61, 564–576. doi: 10.1001/archpsyc.61.6.564
Pubmed Abstract | Pubmed Full Text | CrossRef Full Text | Google Scholar
Menzies, L., Williams, G. B., Chamberlain, S. R., Ooi, C., Fineberg, N., Suckling, J., et al. (2008). White matter abnormalities in patients with obsessive-compulsive disorder and their first-degree relatives. Am. J. Psychiatry 165, 1308–1315. doi: 10.1176/appi.ajp.2008.07101677
Pubmed Abstract | Pubmed Full Text | CrossRef Full Text | Google Scholar
Papademetris, X., Jackowski, A., Schultz, R., Staib, L., Duncan, J., Barillot, C., et al. (2004). “Integrated intensity and point-feature nonrigid registration,” in Medical Image Computing and Computer-Assisted Intervention-MICCAI 2004, eds C. Barillot, D. R. Haynor and P. Hellier (Berlin/Heidelberg: Springer), 763–770.
Rack-Gomer, A. L., Liau, J., and Liu, T. T. (2009). Caffeine reduces resting-state BOLD functional connectivity in the motor cortex. Neuroimage 46, 56–63. doi: 10.1016/j.neuroimage.2009.02.001
Pubmed Abstract | Pubmed Full Text | CrossRef Full Text | Google Scholar
Ruiz, S., Birbaumer, N., and Sitaram, R. (2013a). Abnormal neural connectivity in schizophrenia and fMRI-brain-computer interface as a potential therapeutic approach. Front. Psychiatry 4:17. doi: 10.3389/fpsyt.2013.00017
Pubmed Abstract | Pubmed Full Text | CrossRef Full Text | Google Scholar
Ruiz, S., Buyukturkoglu, K., Rana, M., Birbaumer, N., and Sitaram, R. (2014). Real-time fMRI brain computer interfaces: self-regulation of single brain regions to networks. Biol. Psychol. 95, 4–20. doi: 10.1016/j.biopsycho.2013.04.010
Pubmed Abstract | Pubmed Full Text | CrossRef Full Text | Google Scholar
Ruiz, S., Lee, S., Soekadar, S. R., Caria, A., Veit, R., Kircher, T., et al. (2013b). Acquired self-control of insula cortex modulates emotion recognition and brain network connectivity in schizophrenia. Hum. Brain Mapp. 34, 200–212. doi: 10.1002/hbm.21427
Pubmed Abstract | Pubmed Full Text | CrossRef Full Text | Google Scholar
Sakai, Y., Narumoto, J., Nishida, S., Nakamae, T., Yamada, K., Nishimura, T., et al. (2011). Corticostriatal functional connectivity in non-medicated patients with obsessive-compulsive disorder. Eur. Psychiatry 26, 463–469. doi: 10.1016/j.eurpsy.2010.09.005
Pubmed Abstract | Pubmed Full Text | CrossRef Full Text | Google Scholar
Scheinost, D., Benjamin, J., Lacadie, C. M., Vohr, B., Schneider, K. C., Ment, L. R., et al. (2012). The intrinsic connectivity distribution: a novel contrast measure reflecting voxel level functional connectivity. Neuroimage 62, 1510–1519. doi: 10.1016/j.neuroimage.2012.05.073
Pubmed Abstract | Pubmed Full Text | CrossRef Full Text | Google Scholar
Scheinost, D., Stoica, T., Saksa, J., Papademetris, X., Constable, R. T., Pittenger, C., et al. (2013). Orbitofrontal cortex neurofeedback produces lasting changes in contamination anxiety and resting-state connectivity. Transl. Psychiatry 3:e250. doi: 10.1038/tp.2013.24
Pubmed Abstract | Pubmed Full Text | CrossRef Full Text | Google Scholar
Shibata, K., Watanabe, T., Sasaki, Y., and Kawato, M. (2011). Perceptual learning incepted by decoded fMRI neurofeedback without stimulus presentation. Science 334, 1413–1415. doi: 10.1126/science.1212003
Pubmed Abstract | Pubmed Full Text | CrossRef Full Text | Google Scholar
Sitaram, R., Veit, R., Stevens, B., Caria, A., Gerloff, C., Birbaumer, N., et al. (2012). Acquired control of ventral premotor cortex activity by feedback training: an exploratory real-time FMRI and TMS study. Neurorehabil. Neural Repair 26, 256–265. doi: 10.1177/1545968311418345
Pubmed Abstract | Pubmed Full Text | CrossRef Full Text | Google Scholar
Subramanian, L., Hindle, J. V., Johnston, S., Roberts, M. V., Husain, M., Goebel, R., et al. (2011). Real-time functional magnetic resonance imaging neurofeedback for treatment of Parkinson’s disease. J. Neurosci. 31, 16309–16317. doi: 10.1523/JNEUROSCI.3498-11.2011
Pubmed Abstract | Pubmed Full Text | CrossRef Full Text | Google Scholar
Sulzer, J., Haller, S., Scharnowski, F., Weiskopf, N., Birbaumer, N., Blefari, M. L., et al. (2013). Real-time fMRI neurofeedback: progress and challenges. Neuroimage 76, 386–399. doi: 10.1016/j.neuroimage.2013.03.033
Pubmed Abstract | Pubmed Full Text | CrossRef Full Text | Google Scholar
Swedo, S. E., Leonard, H. L., Kruesi, M. J., Rettew, D. C., Listwak, S. J., Berrettini, W., et al. (1992). Cerebrospinal fluid neurochemistry in children and adolescents with obsessive-compulsive disorder. Arch. Gen. Psychiatry 49, 29–36. doi: 10.1001/archpsyc.1992.01820010029004
Pubmed Abstract | Pubmed Full Text | CrossRef Full Text | Google Scholar
Tagliazucchi, E., von Wegner, F., Morzelewski, A., Brodbeck, V., Jahnke, K., and Laufs, H. (2013). Breakdown of long-range temporal dependence in default mode and attention networks during deep sleep. Proc. Natl. Acad. Sci. U S A 110, 15419–15424. doi: 10.1073/pnas.1312848110
Pubmed Abstract | Pubmed Full Text | CrossRef Full Text | Google Scholar
Telesford, Q. K., Morgan, A. R., Hayasaka, S., Simpson, S. L., Barret, W., Kraft, R. A., et al. (2010). Reproducibility of graph metrics in FMRI networks. Front. Neuroinform. 4:117. doi: 10.3389/fninf.2010.00117
Pubmed Abstract | Pubmed Full Text | CrossRef Full Text | Google Scholar
Tomasi, D., and Volkow, N. D. (2010). Functional connectivity density mapping. Proc. Natl. Acad. Sci. U S A 107, 9885–9890. doi: 10.1073/pnas.1001414107
Pubmed Abstract | Pubmed Full Text | CrossRef Full Text | Google Scholar
Van Dijk, K. R., Sabuncu, M. R., and Buckner, R. L. (2012). The influence of head motion on intrinsic functional connectivity MRI. Neuroimage 59, 431–438. doi: 10.1016/j.neuroimage.2011.07.044
Pubmed Abstract | Pubmed Full Text | CrossRef Full Text | Google Scholar
Yan, C. G., Cheung, B., Kelly, C., Colcombe, S., Craddock, R. C., Di Martino, A., et al. (2013). A comprehensive assessment of regional variation in the impact of head micromovements on functional connectomics. Neuroimage 76, 183–201. doi: 10.1016/j.neuroimage.2013.03.004
Pubmed Abstract | Pubmed Full Text | CrossRef Full Text | Google Scholar
Young, K. D., Zotev, V., Phillips, R., Misaki, M., Yuan, H., Drevets, W. C., et al. (2014). Real-time FMRI neurofeedback training of amygdala activity in patients with major depressive disorder. PLoS One 9:e88785. doi: 10.1371/journal.pone.0088785
Pubmed Abstract | Pubmed Full Text | CrossRef Full Text | Google Scholar
Keywords: neurofeedback, real-time fMRI, resting state connectivity, obsessive-compulsive disorder, orbitofrontal cortex
Citation: Scheinost D, Stoica T, Wasylink S, Gruner P, Saksa J, Pittenger C and Hampson M (2014) Resting state functional connectivity predicts neurofeedback response. Front. Behav. Neurosci. 8:338. doi: 10.3389/fnbeh.2014.00338
Received: 23 April 2014; Accepted: 08 September 2014;
Published online: 24 September 2014.
Edited by:
Sergio Ruiz, Pontificia Universidad Catolica de Chile, ChileReviewed by:
Gillipsie Minhas, Post Graduate Institute of Medical Education and Research, India (in collaboration with Akshay Anand)Akshay Anand, Post Graduate Institute of Medical Education and Research, India
Alicia Izquierdo, University of California, Los Angeles, USA
Ralf Veit, Eberhard Karls-University, Germany
Copyright © 2014 Scheinost, Stoica, Wasylink, Gruner, Saksa, Pittenger and Hampson. This is an open-access article distributed under the terms of the Creative Commons Attribution License (CC BY). The use, distribution and reproduction in other forums is permitted, provided the original author(s) or licensor are credited and that the original publication in this journal is cited, in accordance with accepted academic practice. No use, distribution or reproduction is permitted which does not comply with these terms.
*Correspondence: Michelle Hampson, Magnetic Resonance Research Center (MRRC), Department of Diagnostic Radiology, Yale School of Medicine, The Anlyan Center, N121, 300 Cedar Street, New Haven, CT 06520-8043, USA e-mail:bWljaGVsbGUuaGFtcHNvbkB5YWxlLmVkdQ==