Prolonged Bat Call Exposure Induces a Broad Transcriptional Response in the Male Fall Armyworm (Spodoptera frugiperda; Lepidoptera: Noctuidae) Brain
- 1Illinois Natural History Survey, Prairie Research Institute, University of Illinois at Urbana-Champaign, Champaign, IL, United States
- 2Insect Evolution, Behavior, and Genomics Lab, Florida Museum of Natural History, University of Florida, Gainesville, FL, United States
- 3Colorado College, Colorado Springs, CO, United States
Predation risk induces broad behavioral and physiological responses that have traditionally been considered acute and transitory. However, prolonged or frequent exposure to predators and the sensory cues of their presence they broadcast to the environment impact long-term prey physiology and demographics. Though several studies have assessed acute and chronic stress responses in varied taxa, these attempts have often involved a priori expectations of the molecular pathways involved in physiological responses, such as glucocorticoid pathways and neurohormone production in vertebrates. While relatively little is known about physiological and molecular predator-induced stress in insects, many dramatic insect defensive behaviors have evolved to combat selection by predators. For instance, several moth families, such as Noctuidae, include members equipped with tympanic organs that allow the perception of ultrasonic bat calls and facilitate predation avoidance by eliciting evasive aerial flight maneuvers. In this study, we exposed adult male fall armyworm (Spodoptera frugiperda) moths to recorded ultrasonic bat foraging and attack calls for a prolonged period and constructed a de novo transcriptome based on brain tissue from predator cue-exposed relative to control moths kept in silence. Differential expression analysis revealed that 290 transcripts were highly up- or down-regulated among treatment tissues, with many annotating to noteworthy proteins, including a heat shock protein and an antioxidant enzyme involved in cellular stress. Though nearly 50% of differentially expressed transcripts were unannotated, those that were are implied in a broad range of cellular functions within the insect brain, including neurotransmitter metabolism, ionotropic receptor expression, mitochondrial metabolism, heat shock protein activity, antioxidant enzyme activity, actin cytoskeleton dynamics, chromatin binding, methylation, axonal guidance, cilia development, and several signaling pathways. The five most significantly overrepresented Gene Ontology terms included chromatin binding, macromolecular complex binding, glutamate synthase activity, glutamate metabolic process, and glutamate biosynthetic process. As a first assessment of transcriptional responses to ecologically relevant auditory predator cues in the brain of moth prey, this study lays the foundation for examining the influence of these differentially expressed transcripts on insect behavior, physiology, and life history within the framework of predation risk, as observed in ultrasound-sensitive Lepidoptera and other ‘eared’ insects.
Introduction
Predator-induced stress has long fascinated biologists for its integrated, scalable effects on prey physiology, behavior (Slos and Stoks, 2008), and even spatiotemporal population demographics (Clinchy et al., 2013). Though a mechanistic understanding of the physiological responses that are induced by predation related stress in vertebrates has been known for some time, researchers interested in similar responses in invertebrate taxa, such as insects, now seek a similar descriptive model. The study of invertebrate stress responses has a rich history, yet the diversity of molecular components induced by various stressors has thus far stymied most attempts at holistic understanding. Recently, however, Adamo (2010, 2017a) demonstrated that the early stages of stress responses in insects are homologous, and likely anciently related, to vertebrate neurotransmitter signaling and downstream neurohormonal activation. The challenge remains, then, in describing the varied taxon- and tissue-specific responses seen in insects and elucidating the mechanisms responsible for inducing them.
Often before a predator has even localized its prey, a suite of adaptive behavioral and physiological responses which improve the chances of survival (Endler, 1991) are induced in prey organisms which may be eavesdropping on mechanical, auditory, visual, and chemosensory predation cues (Adamo et al., 2013). For instance, moths and butterflies that are sensitive to ultrasound display startle responses when exposed to synthetic broad frequency ultrasound (Roeder, 1966; Ratcliffe et al., 2008, 2011; ter Hofstede et al., 2011) and recorded bat calls (Acharya and McNeil, 1998; Rydell et al., 2003; Ratcliffe and Fullard, 2005), such as changing the course of flight, ceasing flight, accelerating, performing evasive flight maneuvers (Yack, 2004; Yack et al., 2007; Pfuhl et al., 2015), and/or calling back with jamming ultrasound themselves (Corcoran et al., 2009). Upon exposure to ultrasound, non-flying noctuid moths cease movement while many aerial noctuids exhibit evasive flight maneuvers, such as erratic changes in direction, loops, increases in flight velocity, and even falling to the ground (Surlykke and Miller, 1982). Moreover, when exposed to bat calls, many female and male tympanate moths alter their mating behavior by stopping pheromone release or ceasing flight, respectively (Acharya and McNeil, 1998). These behavioral responses, especially when borne out for an extended period of time, may contribute to patterns of stressor-induced gene regulation in insects that may contribute to reports of moths that display modified fecundity and life history patterns following prolonged exposure to recorded and synthetic bat ultrasound in a laboratory setting (Huang et al., 2003; Zha et al., 2008, 2013). For instance, Plodia interpunctella (Lepidoptera: Pyralidae) exposed to short bursts of ultrasound near their hearing range (approximately 50 kHz) respond by modifying mating behavior (Trematerra and Pavan, 1995) and long-term exposure even affects spermatophore quality and larval numbers by up to 75% (Kirkpatrick and Harein, 1965; Huang et al., 2003) while simultaneously reducing F1 larval weight and growth rates (Huang et al., 2003; Huang and Subramanyam, 2004). Conversely, long-term exposure to broadband ultrasound in Helicoverpa armigera (Lepidoptera: Noctuidae) significantly increased whole-body acetylcholinesterase activity (Zha et al., 2008), the number of spermatophores per female, and the number of eggs laid (Zha et al., 2013).
In order to maintain internal homeostasis during stressful periods, whether osmotically, metabolically, or otherwise, insects and most other forms of life evolved biomolecular signaling cascades, both intra- and extra-cellularly, that often regulate the expression of stress-related genes (Pauwels et al., 2005; Aruda et al., 2011; Yamaguchi et al., 2012; Roszkowski et al., 2016) and resulting behaviors, including vigilance (Lima, 1990; Kight and Swaddle, 2011) and modified activity patterns (Abramsky et al., 2014). Further, these responses mediate cellular metabolism and the degradative effects of prolonged and persistent stressor exposure, including oxidative damage (Slos and Stoks, 2008; Clinchy et al., 2013), protein misfolding (Fleshner et al., 2004; Even et al., 2012), and organelle turnover (Salvetti et al., 2000; Gesi et al., 2002). However, individual cells can respond to stressful conditions by activating transcriptional pathways that usually produce one or more damage-mitigating antioxidant enzymes or protein folding chaperones, such as the heat shock proteins (Hsps). Though these molecular defenses promote physiological homeostasis in the short-term, prolonged periods of stress clearly influence the life history and fitness of many species. Even though biologists have long recognized the importance of stress hormone signaling for initiating behavioral and physiological defenses to predation, the cellular- and tissue-level mechanisms by which long-term acclimation to predation risk can influence the life history and fitness of prey species remains unclear, particularly among insects.
In this study, we exposed adult male fall armyworm moths to recorded ultrasonic foraging and attack calls of three insectivorous bat species over an 8-h period to test the influence of an ecologically relevant auditory cue of predation on the cellular physiology of the noctuid brain. The fall armyworm, though a non-model species itself, is in the same family as the corn earworm (Helicoverpa zea), whose annotated reference genome was recently published (Pearce et al., 2017) and whose old world sister species, H. armigera, has long been a prominent subject in insect auditory neuroethology studies for its dramatic neurobehavioral responses to ultrasound. The fall armyworm, and many other tympanic moths, thus make prime candidates for describing the biochemical and cellular responses that have evolved to cope with prolonged predation risk in insects. We hypothesized that a broader transcriptomic response would be induced in the brains of cue-exposed relative to unexposed individuals. Further, we predicted this response might involve transcripts pertaining to the following physiological functions: (1) intracellular secondary messenger systems, (2) antioxidant and Hsp activity, and (3) gene regulation.
Materials and Methods
Fall armyworm larvae were purchased from Frontier Agricultural Sciences (Newark, DE, United States) under USDA APHIS PPQ 526 permit (P526P-04080) and were shipped over-night as second and third instar larvae. Upon arrival at the Illinois Natural History Survey, Prairie Research Institute, University of Illinois at Urbana-Champaign in Champaign, IL, United States, larvae were transferred to individual 59 mL (2 oz.) plastic cups filled with 10–15 mL of standard lepidopteran diet and reared in an environmental chamber (Percival Scientific, Perry, IA, United States) at 30 ± 1°C and 75 ± 5% RH, with a photoperiod of 16 h light/8 h dark. Larvae fed ad libitum on a modified standard larval lepidopteran diet (Sims, 1998; Cohen, 2001; Elvira et al., 2010) prepared every 2 weeks. This diet consisted of 13 g agar, 770 mL distilled water, 31.5 g vitamin-free casein, 24 g sucrose, 27 g wheatgerm, 9 g Wesson’s salt mix, 10 g alphacel, 5 mL 4 M potassium hydroxide, 18 g Vanderzant’s vitamins, 1.6 g sorbic acid, 1.6 g methyl paraben, 3.2 g ascorbic acid, 0.12 g streptomycin salt, 4 mL wheatgerm oil, and 2 mL 10% formaldehyde. We blended the casein, sucrose, wheatgerm, Wesson’s salt mix, alphacel, 220 mL distilled water, and potassium hydroxide on high for 5 min, to which we added 550 mL of mildly boiling distilled, deionized water mixed with agar. We then blended the mixture for another 5 min and allowed it to cool to 60°C before we added Vanderzant’s vitamins, sorbic acid, methyl paraben, ascorbic acid, streptomycin, wheatgerm oil, and formaldehyde and blended for a final 5 min. We poured 10–15 mL of the cooled diet into each 2 oz. rearing cup and allowed them to solidify in a cold-room for at least 30 min.
We then placed a larva into each filled cup and secured a lid in which two holes had been punched using a No. 1 insect pin. Once a larva cleared its gut before pupation, we transferred it to a shallow Tupperware container (29.4 cm × 15.1 cm × 10.5 cm) filled with 3.5 cm of loose potting soil (SunGro Horticulture, Vancouver, BC, Canada). Once per day, this soil was sifted gently by hand to extract any pupae, which were placed in a separate 30.48 cm3 mesh cage (BioQuip Products, Inc., Compton, CA, United States) with a mesh-size of 51.15 holes/cm2 within the environmental chamber until emergence.
Upon emergence, adults were transferred to a similar mesh cage and allowed to mate. Twice daily, we saturated the sides of the mesh cage with a 10% sucrose solution to allow feeding. To avoid the possible confounding effects of shipment and the change in diet undergone by the generation of larvae received from Frontier Agricultural Sciences, F1 eggs were collected daily from within this cage and placed in small plastic containers within the rearing chamber. Once hatched, we reared F1 larvae as above until emergence as adults.
Predator Cue Exposure
A random sample of four control and four experimental F1 adult males (sex determined by visual inspection of terminal pupal abdominal segment) were selected for use in trials 24–48 h post-eclosion. Females were not used, as female noctuid moths broadcasting pheromones are often sedentary (Stelinski et al., 2014) and may be preyed upon less frequently by aerial-hawking insectivorous bats. Three individual recordings were sampled at 480 kHz, 16-bit format and concatenated with 10 s of silence between each call. The calls consisted of (1) a 4.27 s Molossus molossus (Chiroptera: Molossidae) attack call, (2) a 1.51 s Myotis nigricans (Chiroptera: Vespertilionidae) foraging call, and (3) a 2.92 Saccopteryx bilineata (Chiroptera: Emballonuridae) foraging call. These three neotropical bat species were selected specifically because the neurophysiological response of S. frugiperda auditory neurons to these species’ calls have been explicitly described (Mora et al., 2014), they each represent a ubiquitous species throughout much of S. frugiperda’s range in the Americas (Mora et al., 2004; Jung et al., 2007; Surlykke and Kalko, 2008), and they likely represent novel predators for the lab-reared, United States-based S. frugiperda colony used in this study. Further, these species produce calls of varying amplitudes and frequencies that together span the known response curve of the S. frugiperda tympanum (Mora et al., 2004, 2014). Specifically, M. molossus, M. nigricans, and S. bilineata broadcast at 20–50 (Mora et al., 2004), 50–85, and 45–55 (Jung et al., 2007) kHz, respectively, whereas S. frugiperda responds optimally to sounds within 20–50 kHz (Mora et al., 2014). The individual sound files were processed in Audacity v. 2.1.0. to reduce background ultrasound by applying a 20-dB noise reduction filter to frequencies lower than 30 kHz with moderate sensitivity (10.0) and re-sampled each file at 195.3125 kHz to meet the limitations of our playback system. This down-sampling attenuated frequencies greater than 75 kHz (Tucker-Davis Technologies, personal communication), but reproduced the bat calls faithfully within the 20–50 kHz optimal hearing range reported for noctuid moths (Fullard, 1988; Norman and Jones, 2000). The resulting 38 s file was then broadcast on a loop for the 8-h duration of each experimental trial while control trials consisted of an identical setup with no sound played whatsoever. Calls were broadcast via a Tucker-Davis Technologies (TDT; Alachua, FL, United States) System 3 amplifier powering an ES1 electrostatic free-field speaker (TDT) that was situated 30 cm from the center of the cage in a soundproof, anechoic chamber at the Beckman Institute, University of Illinois at Urbana-Champaign in Urbana, IL, United States. The RPvdsEx software suite v. 80 (TDT) was used to process and playback the audio file via the TDT RP2.1 processor, ED1 Electrostatic Speaker Driver, and SA1 Stereo Amplifier tandem setup. Each of the four, 8-h replicate exposure and control trials took place on alternating nights in September 2017 from 22:00 to 05:00.
Sample Preparation and Sequencing
Post-exposure, each moth was placed into a 2 mL vial and immediately immersed in liquid nitrogen. After 30 s, the moth was removed from the vial and transferred quickly to a Petri dish on dry ice. After the head was removed, we immersed it in RNAlater stabilization solution (Life Technologies). Upon immersion, scales on the head capsule were removed by scraping with scalpel, and a 1 mm × 1 mm section of cuticle was cut to expose the brain tissue directly to RNAlater. We then dissected the brain from the head capsule, rinsed it with fresh RNAlater solution, placed it in a 2 mL microtube of fresh RNAlater solution, and stored it at 2°C until all samples had been collected.
RNA was extracted from each brain using a PicoPure RNA Isolation Kit (Arcturus Bioscience). RNA was eluted in 30 μL of RNase-free water and stored at -80°C until further analysis. Before freezing, 3.5 μL aliquots were removed from each extract and used for RNA quantification via a NanoDrop (Thermo Fisher Scientific) spectrophotometer and a Qubit fluorometer (Life Technologies) using a Qubit RNA HS Assay Kit (Life Technologies). After a 1:10 or 1:15 dilution based on each sample’s concentration, we submitted these subsamples to the Functional Genomics Unit of the University of Illinois at Urbana-Champaign’s (UIUC) Roy J. Carver Biotechnology Center to confirm RNA quality with a Bioanalyzer RNA 6000 Pico chip (Agilent).
We then submitted each RNA extract to the UIUC Roy J. Carver Biotechnology Center’s High-Throughput Sequencing and Genotyping Unit for library preparation and sequencing. Strand-specific cDNA libraries were prepared using an Illumina TruSeq Stranded mRNA Sample Prep Kit (dUTP based) according to manufacturer specifications and quantified by quantitative polymerase chain reaction (qPCR). The eight samples were multiplexed on a single lane of an Illumina 2500 sequencer and the RNA fragments were sequenced using Illumina’s HiSeq SBS Sequencing Kit v4 for 101 cycles with a 100 nt paired-end read length.
Raw mRNA Read Preprocessing
Sequence files were demultiplexed with Illumina’s bcl2fstq v. 217.1.14 conversion software. To ascertain raw read quality, we used FastQC v. 0.11.2 (Andrews, 2010) with default settings on each set of reads. We then preprocessed the raw reads by performing adapter trimming, quality filtering, and in silico normalization. Adapter trimming and quality filtering was achieved using Trimmomatic v. 0.33 (Bolger et al., 2014) in palindrome mode to search for and remove adapter sequences and low quality bases. To remove redundant reads and improve transcriptome assembly performance, the remaining reads were then digitally normalized to a coverage depth of 50× via the Trinity transcriptome assembly suite v. 2.1.1 (Grabherr et al., 2011; Haas et al., 2013).
De novo Transcriptome Assembly, Annotation, and Quality Assessment
To our knowledge, there is no publicly available annotated reference genome for Spodoptera frugiperda; therefore, we chose to build a de novo transcriptome assembly with the pre-processed reads using the Trinity assembler v. 2.1.1 (Grabherr et al., 2011). We designated the sequence-specific strand orientation to ‘reverse-forward’ (RF) when possible. The quality of the resulting transcriptome was then assessed using TransRate v. 1.0.1 (Smith-Unna et al., 2016) and BUSCO v. 3 (Simão et al., 2015). We then utilized the Annocript v. 2.0 automated transcriptome annotation algorithm (Musacchia et al., 2015) to complete sequence-similarity searches on each assembled transcript against the National Center for Biotechnology Information (NCBI)’s non-redundant nucleotide database using BLAST+ v. 2.2.30 (Camacho et al., 2009). We selected the UniRef90 protein database (Boutet et al., 2016) to screen for computationally derived protein annotations. Annocript first downloaded the UniRef90 database, stored it in a MySQL v. 7.3 (Oracle Corporation, Redwood City, CA, United States) database, and indexed it for faster searches (Camacho et al., 2009). Annocript carried out BLASTX searches against the UniRef90 database and reported those hits with an e-value < 1e-5. Annocript output a tab-delimited feature map file containing the collated annotation information for each putative assembled transcript.
Read Alignment, Abundance, and Differential Expression Analysis
Following annotation, we indexed the transcriptome in Kallisto (Bray et al., 2016) using the ‘kallisto index’ command before aligning each sample’s reads against the index using the ‘kallisto quant’ command to select 250 bootstrap replicates each. In R v. 3.5.1 (R Core Team, 2014), we utilized the packages ‘edgeR’ v. 3.12.1 (Robinson et al., 2009) and ‘limma’ v. 3.26.9 (Ritchie et al., 2015) to import the estimated read counts and perform DE statistical analyses. First, we used the trimmed mean of M-values (TMM) normalization method (Robinson and Oshlack, 2010) to account for small biases in each sample’s overall read library size. To filter out transcripts with low or no expression estimates in one or more grouped replicates (Rau et al., 2013), we calculated the counts per million (CPM) mapped reads for each transcript and removed those with a CPM < 1.
We then visually assessed the presence of batch effects in our data by performing principal components analysis (PCA) on log-transformed CPM expression values across each sample using the ‘affycoretools’ v. 1.42.0 (MacDonald, 2008) package in R. To account for a large amount of expression variation observed between replicate samples (Figure 1A), we used the ‘sva’ v. 3.18.0 (Leek et al., 2012, 2010) package to explicitly model three identified surrogate variables as covariates. After adding these covariates to our dataset, we log-transformed all CPM estimates to prepare for linear modeling. We then used the ‘limma’ package and its ‘voom’ function (Law et al., 2014) to fit a negative binomial linear model and proceeded to compute pairwise t-statistics, F-statistics, and log-odds of differential expression for each transcript according to exposure type using empirical Bayes (Smyth, 2004). The resulting differentially expressed transcripts were filtered by selecting only those with false discovery rate (FDR)-adjusted p-values < 0.05 and a fold-change > 2 to account for multiple testing bias on p-value significance (Benjamini and Hochberg, 1995; Benjamini and Heller, 2007).
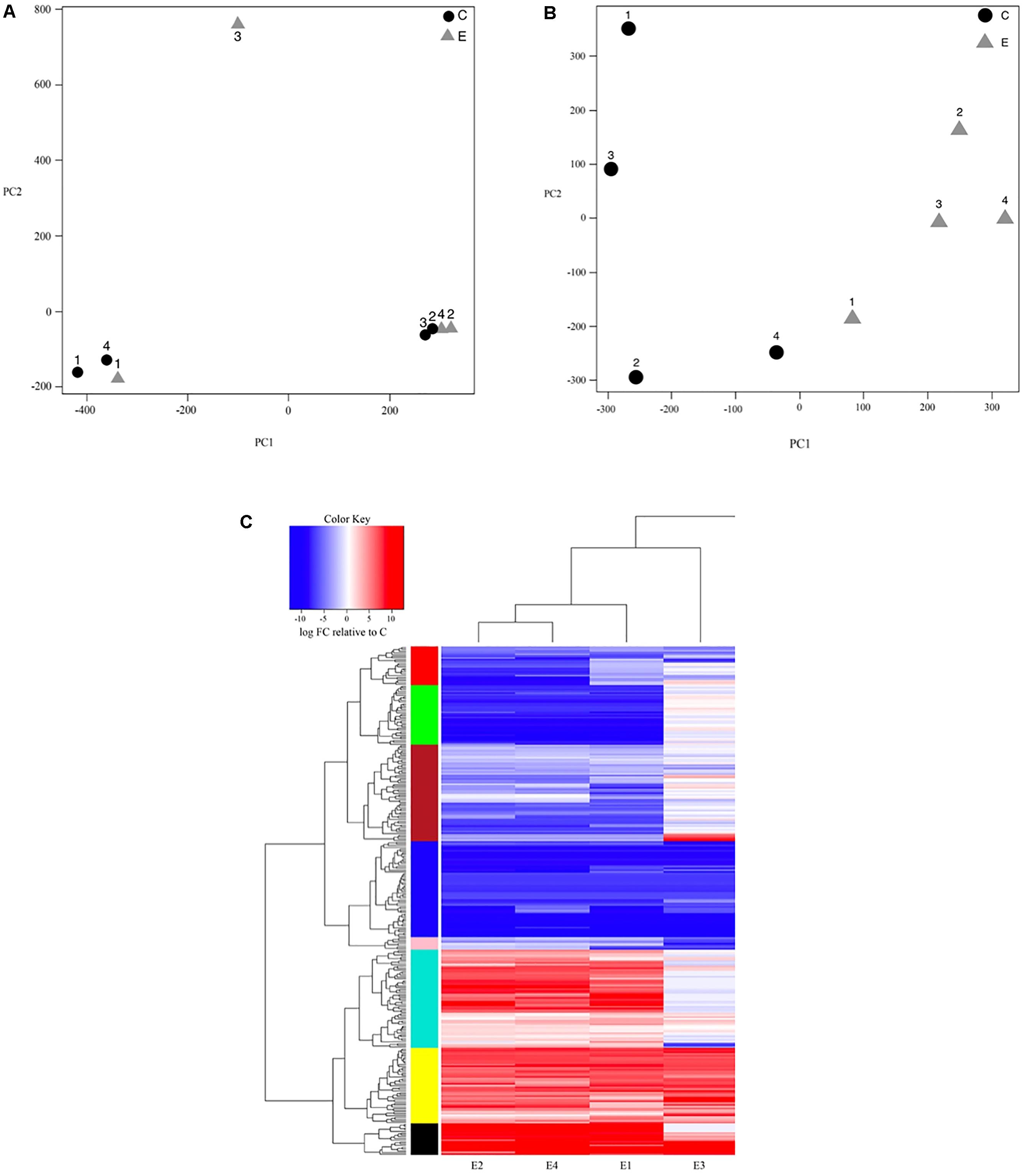
Figure 1. (A) Principal components plot showing sample clustering based on the first two principal components of variation in log-based counts per million read estimates for both control (C; black circle) and bat-ultrasound exposed (E; gray triangle) Spodoptera frugiperda moths; numbers (1–4) represent replicate samples from each of the control and exposure groups. (B) Principal components plot after surrogate variable analysis was performed to account for unexpected batch effects showing sample clustering based on the first two principal components of variation in log-based counts per million read estimates for both control (C; black circle) and bat-ultrasound exposed (E; gray triangle) adult male Spodoptera frugiperda moths; numbers (1–4) represent replicate samples from each of the control and exposure groups. (C) Transcript expression heatmap detailing the up- (red) and down- (blue) regulation (log2FC) of each transcript relative to the mean expression of the control group across bat-ultrasound exposed (E) adult male Spodoptera frugiperda moths; samples (horizontal axis) and transcripts (vertical axis) are clustered according to expression similarity (stacked multicolored bars).
To produce a heatmap of gene expression across the samples, we scaled each transcript’s associated fold-change to the mean fold-change observed in all transcripts from the control group. To assess similarity in expression between samples, we used a hierarchical clustering method based on a distance matrix compiled by taking the maximal distance between any two expression values in each sample via the ‘fastcluster’ package v. 1.1.20 (Müllner, 2013) in R. The resultant base dendrogram of similarity between individual transcripts was then used to identify the most appropriate level at which to cluster our transcripts using the R package ‘dynamicTreeCut’ v. 1.63-1 (Langfelder et al., 2008). We chose to use the ‘hybrid’ method to first identify large, base clusters following four criteria: (1) each cluster must contain ≥2 transcripts; (2) transcripts that are too distant from a cluster are excluded, even if they occur on the same branch; (3) each preliminary cluster must be distinct from those clusters near to it; and (4) the tips of each preliminary cluster must be tightly connected. Once these clusters were identified, any transcripts not previously assigned were placed in the closest neighboring cluster. Using ‘cdbfasta’ v. 0.991, we then retrieved the sequences and Gene Ontology (GO) terms associated with these differentially expressed (DE) transcripts from our annotated transcriptome for downstream functional GO enrichment analysis. Figures were constructed using the R packages ‘graphics’ v. 3.2.4, ‘grDevices’ v. 3.2.4, ‘rgl’ v. 0.95.1441, and ‘gplots’ v. 2.17.0.
Functional Gene Ontology Term Enrichment and KEGG Pathway Analyses
To obtain a broader perspective on the function of our DE transcripts and how they may be related, we tested their associated annotated GO terms for statistically significant over- and under-representation via GO term enrichment analysis. The background set of transcripts we used to test our DE set against included all GO annotations from base transcriptome. Using the ‘Biological Networks Gene Ontology’ (BiNGO) plugin v. 3.0.3 (Maere et al., 2005) in the Cytoscape platform v. 3.3.0 (Shannon et al., 2003), we tested for both over- and under-representation using a hypergeometric test at an FDR-adjusted p-value < 0.05. Each differentially expressed transcript was also annotated using the automated BlastKOALA (Kanehisa et al., 2016) KEGG pathway webserver and analyzed manually for functional relevance.
Data Availability
The raw sequence reads have been uploaded to the NCBI Sequence Read Archive (SRA) database (accessions: SRR3406020, SRR3406031, SRR3406036, SRR3406052, SRR3406053, SRR3406054, SRR3406055, SRR3406059) and are also available through the BioProject accession PRJNA3188192. The transcriptome has been archived to NCBI’s Transcriptome Shotgun Assembly database under accession GESP00000000; the version used here is GESP00000000.1. A repository containing R scripts and output files from all analyses downstream of assembly is also hosted on GitHub3.
Results
RNA Extraction, Library Preparation, and Read Processing
Each RNA extract was found to produce satisfactory yields, and these were subsequently used in downstream analyses. Total RNA concentration in each sample was generally consistent between NanoDrop and Qubit estimates, the absorbance ratios signified little if any contamination (A260/280 > 2) and the bioanalyzer assay revealed each sample consisted of high-quality RNA with negligible signs of degradation (RIN > 8; Table 1). Our cDNA fragment lengths after library preparation ranged from 80 to 700 bp, with an average of 300 bp. Each sample produced similar numbers of reads, ranging between 28.3 million and 31.1 million. The average quality scores for each base in each sample were ≥33 (phred-33 scaling), allowing us to proceed without sequencing error correction. Preprocessing steps led to less than 0.12% of reads being removed in each sample, and the GC content of the samples ranged from 42 to 45% post-trimming.
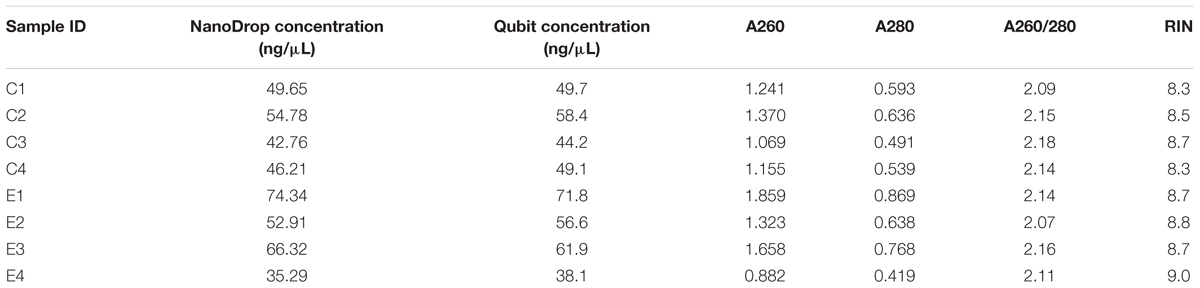
Table 1. Total RNA concentration, absorbance values, absorbance ratios, and RNA Integrity Number (RIN) for each brain tissue RNA extraction from control (C) and bat ultrasound-exposed (E) adult male Spodoptera frugiperda moths.
De novo Transcriptome Assembly Statistics
Our transcriptome contained a total of 27,734 putative transcript contigs in total, ranging in length from 124 to 38,522 bp with an average of 1,399.9 bp. The contig N50 of our assembly was 2,933 bp and 40.9% of the contigs exceeded 1,000 bp in length. Out of 303 eukaryotic orthologs used as a reference in BUSCO, we identified 290 (95.7%) complete matches, with 234 single-copy and 56 duplicate hits, along with three fragmented and ten missing orthologs. Annocript annotated 10,367 (37.38%) contigs with reliable protein annotations from significant (e-value < 10-5) BLASTX hits using the UniRef90 database. Of these hits, 97.0% and 93.6% were annotated to species of Insecta and Lepidoptera, respectively. Mapping GO annotations to these hits resulted in 6,476 GO annotations present in the transcriptome, with 4,075 gene products attributed to biological processes, 815 to cellular components, and 1,586 to molecular function. The top GO terms attributed to the largest numbers of transcript contigs included ‘integral to membrane’ (GO:0016021), ‘nucleic acid binding’ (GO:0003676), ‘ATP binding’ (GO:0005524), ‘nucleus’ (GO:0005634), and ‘zinc ion binding’ (GO:0008270).
Read Alignment and Abundance Quantification
On average, 36.59% ± 0.573% (95% CI) of reads from each sample mapped to the transcriptome. TMM normalization resulted in normalization factors ranging from 0.915 to 1.104, which we then multiplied by our actual library sizes to find our final effective library sizes. After filtering low and no expression transcripts with <1 CPM, 17,558 out of 27,734 (63.3%) were retained for DE analysis.
Differential Transcript Expression Analysis
Our initial PCA indicated strong, unexpected clustering of samples along the first two principal axes (Figure 1A), leading us to use surrogate variable analysis in effort to remove potential unaccounted batch effects. We found three significant surrogate variables that we included in our negative binomial regression model as covariates, resulting in clear clustering of samples by experimental group (Figure 1B). Further, we improved our detection of significant DE transcripts at a FDR < 0.05 with ≥2-fold change in expression from 75 to 290 transcripts after including the covariates (Figure 1C). Of the 290 DE transcripts, 146 (50.3%) had significant BLASTX hits (e-value < 1e-5), though 44 (15.2%) had uncharacterized functions (Tables 2, 3). The top 11 organisms with the highest number of hits to DE transcripts were all also lepidopteran taxa, with most pertaining to Amyelois transitella (Lepidoptera: Pyralidae). Of the 290 DE transcripts, 117 were upregulated while 173 were downregulated.
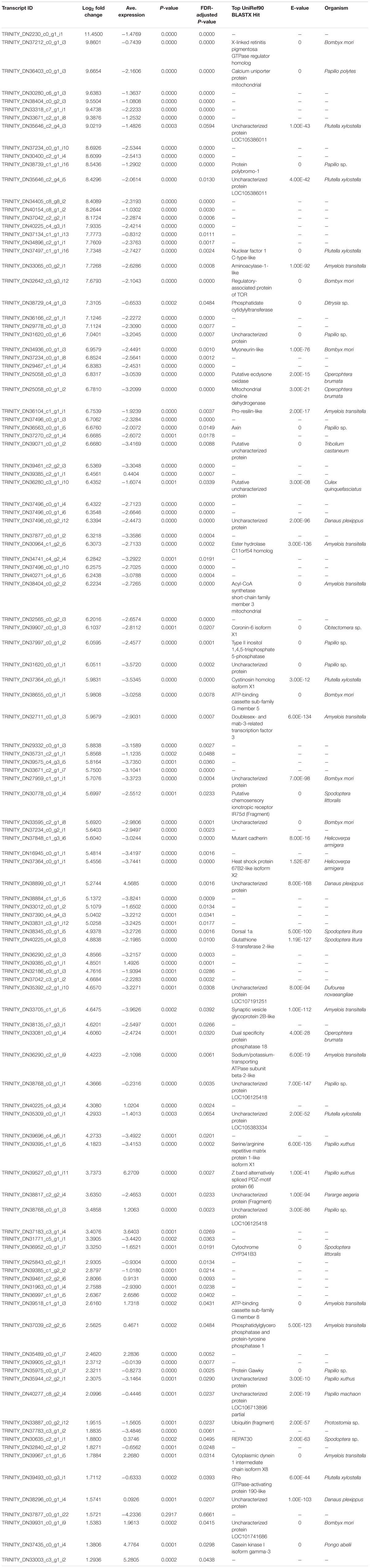
Table 2. List of differentially upregulated (log2-transformed fold change) transcripts recovered from brain tissue mRNA extractions in bat call-exposed Spodoptera frugiperda adult male moths relative to controls, including the most significant (e-value < 1e-5) BLASTX protein annotation from the UniRef90 database and the organism from which the annotation is derived.

Table 3. List of downregulated (log2-transformed fold change) transcripts recovered from brain tissue RNA extractions in bat call-exposed Spodoptera frugiperda adult male moths relative to controls, including the most significant (e-value < 1e-5) BLASTX protein annotation from the UniRef90 database and the organism from which the annotation is derived.
Upregulated Genes
Among the top 10 most highly upregulated genes were a X-linked retinitis pigmentosa GTPase regulator (RPGR) homolog and a mitochondrial calcium uniporter protein, though seven genes were unannotated, including the most highly upregulated transcript, with the remaining transcripts annotated by uncharacterized proteins. Genes also had highly variable absolute log2-transformed fold changes (log2FC) ranging from 1.29 to 11.45. Additional upregulated genes of interest include the regulatory-associated protein of TOR, axin, inositol 1,4 5-triphosphate 5-phosphatase, Hsp 67B2-like isoform X2, glutathione (GSH) S-transferase 2-like, and the rho GTPase-activating protein.
Downregulated Genes
The top 10 most downregulated genes included three with annotations, a 27 kDa hemolymph protein, an equilibrative nucleoside transporter, and protein polybromo-1 (Pb-1), while the remaining seven failed to be annotated. Again, absolute fold-change expression varied broadly (1.48–10.55 log2FC) though several other annotated and functionally relevant genes were downregulated. In particular, voltage-gated ion channels, DNA N6-methyl adenine (6mA) demethylase-like isoform, histone-lysine N-methyltransferase, phosphatidylinositol 5-phosphae 4-kinase, two different cytochrome P450s, glutamate synthase, integrin beta, mitoferrin-1, ankyrin repeat domain-containing protein 17, arrestin, and several zinc finger proteins.
Gene Ontology Enrichment Analysis and KEGG Pathway Reconstruction
Of the 146 DE annotated transcripts, 102 (69.8%) displayed GO term sequence identity (Figure 2A). GO term enrichment analysis identified 15 overrepresented and 0 underrepresented GO categories in our exposed samples (FDR-adjusted p-value < 0.05; Table 4). Six of these overrepresented GO terms pertained to glutamate metabolism, biosynthesis, and synthase activity, while dicarboxylic acid biosynthesis and metabolism corresponded to two terms, and oxidoreductase, aminoacylase, flavin mononucleotide binding, chromatin binding, and macromolecular complex binding corresponded to one term each. Notably, 14 of these 15 overrepresented GO terms annotated a downregulated transcript while only a single term pertained to an upregulated transcript. Of note is that the majority of transcripts mapping to significantly enriched GO terms occurred as very low or zero transcript count observations in the exposed relative to the control group. All transcripts mapping to chromatin binding-, glutamate-, integrin-, oxidoreductase-, and aminoacylase-related GO terms exhibited this pattern of “all-or-nothing” transcript expression. As the data included considerable noise, the prevalence of this pattern among the differentially expressed GO annotated transcripts may simply be due to these patterns being the only ones strong enough to discern statistically, though their functional relevance in stress physiology requires further investigation. Our BlastKOALA KEGG pathway reconstruction of the 290 DE transcripts recovered 43 (14.8%) with functional annotations, including 37 pertaining to cellular metabolism, six related to genetic information processing, nine that function in cellular signal transduction to environmental stimuli, five related to cell growth and death, two related to glutamatergic and GABAergic synapses, respectively, and one related to neurotrophin signaling in neurons specifically (Figure 2B).
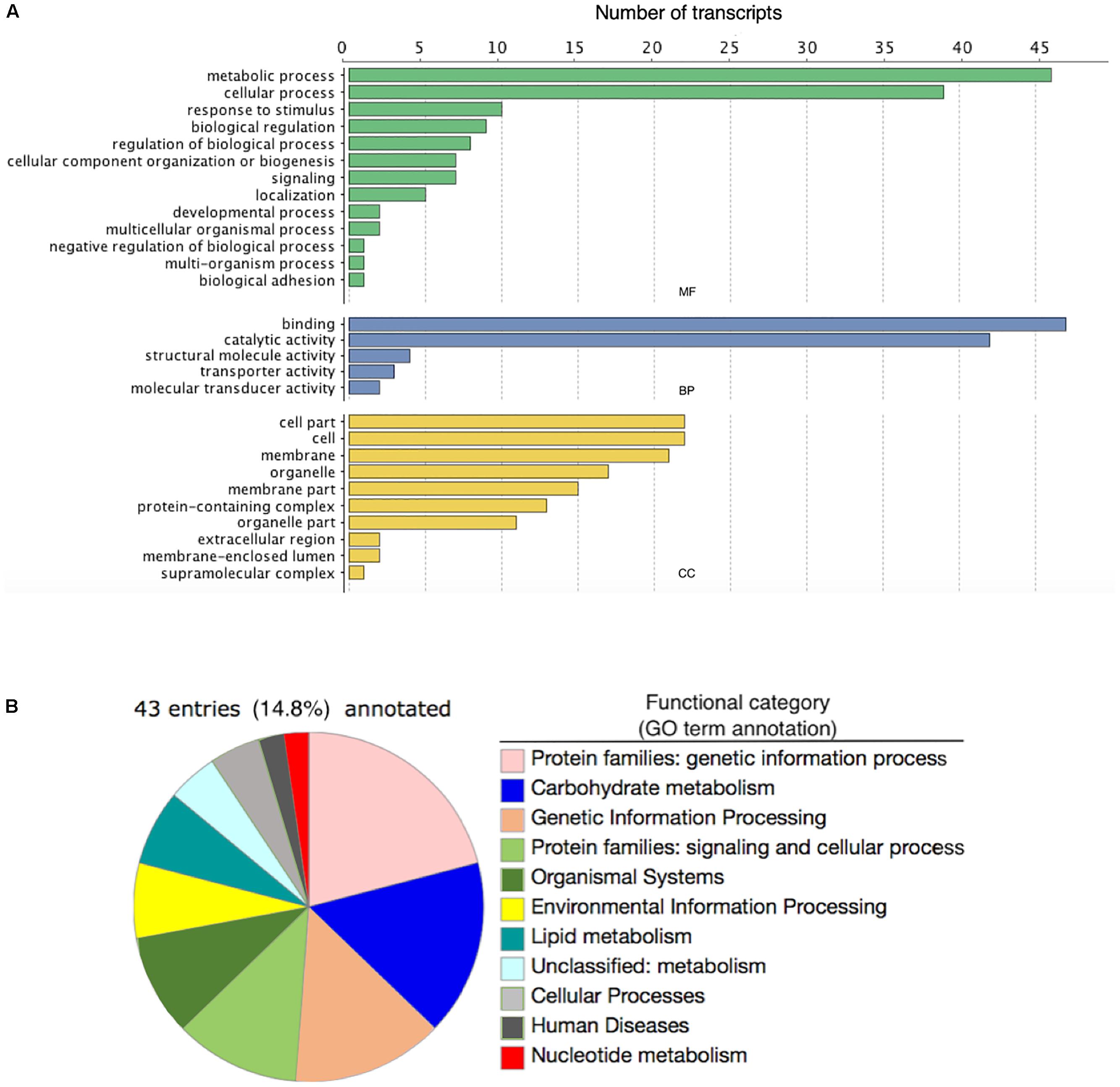
Figure 2. (A) The number of differentially expressed transcripts annotated with Gene Ontology terms corresponding to either a molecular function (MF; green bars), biological process (BP; blue bars), or cellular component (CC; yellow bars) in the brains of four adult male fall armyworm (Spodoptera frugiperda) moths exposed to recorded bat foraging and attack calls for 8 h. (B) Pie graph detailing the Kyoto Encyclopedia of Genes and Genomes (KEGG) Orthology annotations associated with differentially expressed transcripts in the male brains of adult fall armyworm moths exposed for 8 h to recorded bat foraging and attack calls.
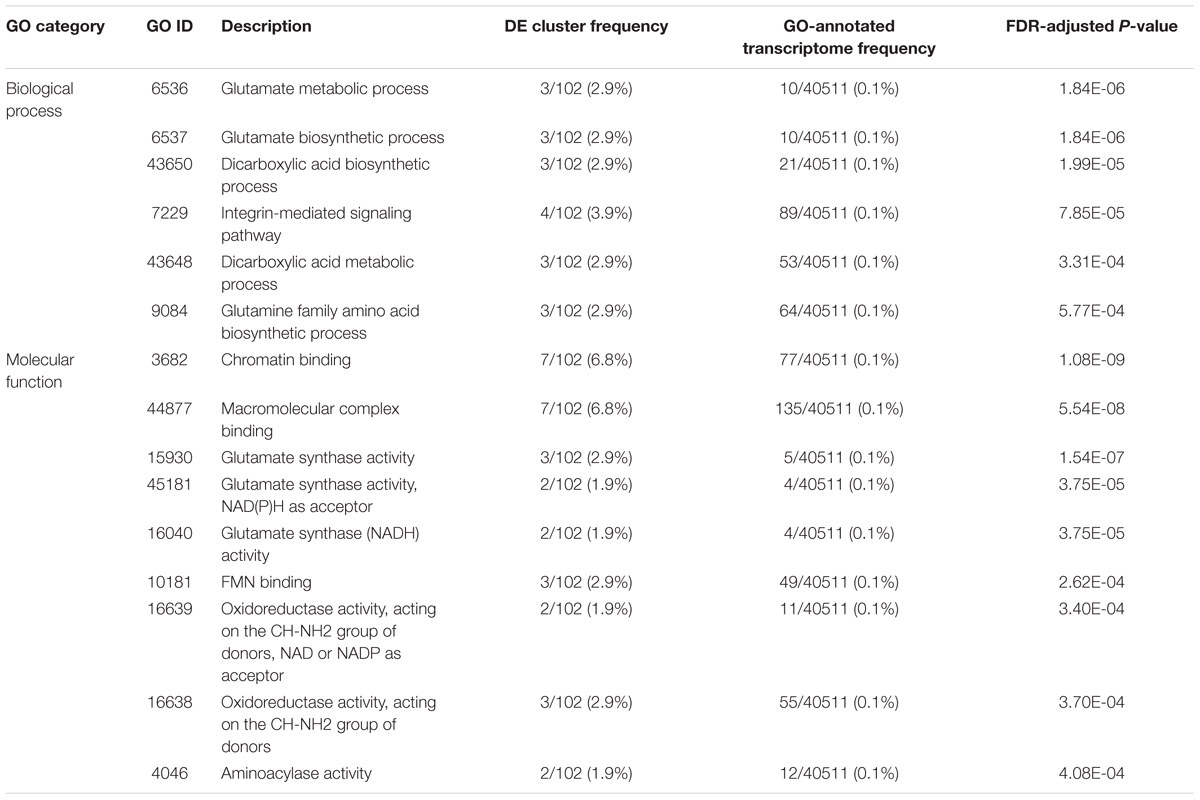
Table 4. List of statistically over-represented (hypergeometric test, FDR-adj. p < 0.05) Gene Ontology (GO) term annotations associated with the 290 differentially expressed (DE) transcripts identified after frequent, prolonged bat-ultrasound exposure in brain tissue of adult male Spodoptera frugiperda moths.
Discussion
A Comparison of Predator-Induced Gene Expression Responses in Other Animals
Our results build on a growing body of literature detailing auditory sensory mode and predator-induced shifts in gene expression in vertebrates and invertebrates (Nanda et al., 2008; Leder et al., 2009; Preisser, 2009; Sheriff and Thaler, 2014; Takahashi, 2014; Harris and Carr, 2016; Adamo, 2017a,b). Several studies have focused on describing the gene expression dynamics of large-scale predator-induced morphological changes that occur in organisms displaying predation-related polyphenisms, including multiple species of Daphnia (Schwarzenberger et al., 2009; Spanier et al., 2010; Rozenberg et al., 2015) and the Hokkaido salamander (Hynobius retardatus; Matsunami et al., 2015). Less striking predator-induced changes also have been studied in diverse taxa, including stickleback fish (Sanogo et al., 2011) and an intertidal snail (Chu et al., 2014). Exposure to auditory cues of aerial hawking bats for 8 h resulted in significant transcriptomic responses, as evidenced by the wide-ranging fold-changes (log2FC) in transcript expression reported here. In the brains of predator-stressed sticklebacks, low-to-moderate fold-changes ranged from 2 to 6 (log2FC; Sanogo et al., 2011), while predator-induced polyphenic Daphnia displayed changes ranging from 2 to 10 (log2FC; Rozenberg et al., 2015). Furthermore, the number of DE transcripts found here is comparable to that found in other RNA-seq studies on predator-induced gene expression among invertebrates. For instance, Daphnia pulex exposed to kairomones of predatory phantom midge (Chaoborus) larvae displayed 256 DE transcripts (Rozenberg et al., 2015), while only three transcripts were differentially regulated in the intertidal snail Nucella lapillus when exposed to seawater that flowed first through a chamber holding a predatory crab (Carcinus maenas) feeding on N. lapillus (Chu et al., 2014). Further, the number of DE transcripts from brain tissue after predator exposure can vary strongly based on predator identity, as shown by Matsunami et al. (2015) who found that Hokkaido salamander larvae exposed to predatory dragonfly naiads displayed 605 DE transcripts, while only 103 DE transcripts were found after exposure to predatory tadpoles. One primary difference between past studies of predator-induced transcriptional changes that must be considered when interpreting the results presented here is the time scale at which cues of predation are presented. In the case of predator-induced polyphenisms, exposure length depends highly on organism life history but ranges generally from a few to several days. Though our study assesses the effects of prolonged, frequent exposure to an auditory cue of predation over a single night, it should be noted that this time scale is much shorter than used in most other studies of predator-induced transcription. Clearly, the degree to which prey respond transcriptionally to cues of predation risk can vary broadly across taxa and no clear pattern has yet emerged. However, the ubiquity with which metazoan life responds transcriptionally to these cues of predation begs the detailed description of these gene pathways, their relevance to physiology and life history, and their evolution throughout the tree of life.
Functional Relevance of Differentially Regulated Genes
Furthermore, our results indicate a broad range of functional annotations related to our DE transcripts. For instance, upregulated transcripts coded for proteins related to cellular signaling, Hsp synthesis, antioxidant metabolism, mitochondrial metabolism, oxidoreductase activity, glutamate synthesis, ionotropic receptor activity, gene regulation, ion transport, and cilium assembly. Downregulated transcript annotations also displayed a large degree of functional variability relating to G-coupled protein signaling, cytochrome P450 activity, chromatin-mediated gene regulation, integrin signaling, glutamate biosynthesis, and voltage-dependent ion channels, among others. Several notable transcript upregulations corresponded to unexpected protein annotations, including a mitochondrial calcium uniporter protein (log2FC = 9.66), an RPGR homolog (log2FC = 9.86), mutant cadherin (log2FC = 5.60), mitochondrial choline dehydrogenase (log2FC = 6.78), and acyl-coenzyme A synthetase short-chain family member 3 (log2FC = 6.22). The mitochondrial calcium uniporter protein acts as a transmembrane transporter for uptake of calcium ions into mitochondria for use during respiration (Marchi and Pinton, 2014) after these ions are mobilized from intracellular stores by inositol triphosphate. Notably, another significantly upregulated gene among exposed individuals was type 2 inositol 1,4,5-triphosphate 5-phosphatase (log2FC = 6.06). In humans, this phosphatase hydrolyzes inositol triphosphate and functions as a signal-terminating enzyme, preventing further calcium release (Ross et al., 1991; Contreras et al., 2010).
The second most upregulated transcript codes for a RPGR homolog, a protein usually associated with cilia development in the photoreceptors of vertebrate eyes (Gakovic et al., 2011), although it localizes to other tissues and cell types as well (Khanna et al., 2005). We suggest that RPGR upregulation may be related to increased cilia development and neuronal connections but since its expression has not been studied in insect eyes or other tissues, further conclusions about the function of this protein under predator-stressed conditions in S. frugiperda cannot be made. Because S. frugiperda brains were excised without compromising pigment-storing ommatidial cells, the RPGR expression pattern observed here likely is intrinsic to brain tissue and may be related to neural tissues extending from innervations of the eye. Notably, the entire suite of phototransduction proteins found in the Drosophila visual system is also found to act in the fly’s auditory transduction system, with visual rhodopsins serving mechanical transduction and amplification roles in auditory neurons of the Johnston’s organ (Pumphrey, 1940).
Another upregulated transcript that may be related to neuronal development encoded a mutant cadherin protein found in humans. Cadherins are calcium-dependent cell-cell adhesion proteins that are integral in nearly every step of neural development in larval Drosophila (Fung et al., 2009), have been implicated in guiding new neuron development contributing to neural plasticity (Edsbagge et al., 2004), and are even involved in hair bundle development in vertebrate ears (Hirano and Takeichi, 2012). As expression of cadherins is usually repressed and localized only to synaptic areas in mature brain tissues (Hirano and Takeichi, 2012), the fact that it is highly upregulated in predator-cue exposed S. frugiperda coupled with RPGR upregulation suggests that neural plasticity and development of new neural connections upon exposure to novel environmental cues may play key roles in functionally responding to auditory predator cues.
Several strongly downregulated transcripts also mapped to unexpected protein annotations, including a 27 kDa hemolymph protein (log2FC = -10.40), DNA 6mA demethylase-like isoform X1 (log2FC = -7.18), decaprenyl-diphosphate synthase subunit 2 (DDSS2; log2FC = -6.80), FH1/FH2 domain-containing protein 3 (FHOD3; log2FC = -7.85), and Pb-1 (isoform log2FC = -9.10, -8.52, -7.60, -7.39, -6.94, 8.54). The 27 kDa hemolymph protein family consists of proteins found in diverse insect taxa but their function remains unknown. DNA 6mA demethylase is another enzyme correlated with a highly downregulated transcript. Methylation of 6mA has been studied primarily in prokaryotes, where it serves as the primary mechanism for epigenetic signaling via DNA methylation—as opposed to the primary mechanism found in eukaryotes, 5-methylcytosine methylation (Vanyushin et al., 1968). Demethylases associated with 6mA and 5-methylcytosine serve to remove methyl groups from DNA and RNA, affecting the transcription and translation of affected nucleic acid chains. In plants and vertebrates, 6mA methylation both increases and decreases transcription factor binding (Luo et al., 2015), while in Drosophila melanogaster loss of a putative 6mA demethylase resulted in increased transposon expression (Zhang et al., 2013). Notably, a transcript annotated with histone-lysine N-methyltransferase (log2FC = -2.54) and five transcript isoforms annotated with Pb-1 were downregulated after predator-cue exposure, although another Pb-1 isoform was also upregulated. These proteins are involved in histone H3 remodeling and binding, respectively (Chandrasekaran and Thompson, 2007; An et al., 2011). Although the functional significance of these downregulated genes in the brain of predator-exposed S. frugiperda is unclear, epigenetic mechanisms appear to be induced in some manner.
The enzyme DDSS2 catalyzes a reaction to supply decaprenyl diphosphate for use in ubiquinone-10 biosynthesis. Ubiquinone-10 is concentrated in mitochondria, where it acts as a component of the electron transport chain during aerobic cellular respiration (Ernster and Dallner, 1995), although it also is found in many diverse organelles at lower concentrations. In this context, ubiquinone-10 acts as an electron transport enzyme moving electrons from enzyme complexes I and II to III in the electron transport chain, a function only it and vitamin K2 are able to perform (Bhalerao and Clandinin, 2012). Ubiquinone-10 also serves as an antioxidant due to its weak electron affinity when reduced. In this state, electrons are held so loosely that the molecule readily gives up electrons to oxidized substrates. For instance, within mitochondria, ubiquinone-10 prevents the oxidation of DNA nucleotides during interactions between peroxidase and DNA-bound metal ions (López et al., 2010; Miyamae et al., 2013). Although the down-regulation of DDSS2 does not directly imply that lower levels of ubiquinone-10 were present in predator-cue exposed S. frugiperda, further studies should examine ubiquinone-10 responses to predator exposure. With knowledge of the increased mitochondrial metabolic activity suggested by several upregulated transcripts discussed previously, it is surprising that DDSS2 is downregulated, as a greater need for electron transport substrates and antioxidants with enhanced energy production might be expected. Clearly, there is still much to learn in elucidating the role of DDSS2, and mitochondrial metabolism in general, in the context of predator-induced stress responses.
Formin homology 1/formin homology 2 domain-containing protein 3 (FHOD3), another protein that mapped to a highly downregulated transcript in the predator-exposed S. frugiperda brain, acts as an actin regulator with a scaffolding function and has been found, in humans, to affect organogenesis, tissue homeostasis, and cancer-cell invasion (Katoh and Katoh, 2004). Actin, a protein that forms microfilaments and constitutes the actin cytoskeleton in all eukaryotic cells, plays a key role in cellular locomotion and shape (Lodish et al., 2000). FHOD family proteins are thought to bind to the growing barbed-end of actin polymers and serve both to deliver new actin monomers and promote actin polymerization, effectively mediating the growth of the actin cytoskeleton (Bechtold et al., 2014). FHOD family proteins are regulated by rho-GTPases, a member of which was downregulated after predator-exposure. Furthermore, actin-binding Lin11, Isl-1, Mec-3 protein 3 and alpha catenin were also down-regulated and act as a scaffold protein (Barrientos et al., 2007) and a cellular linking protein between cadherins and actin-containing filaments (Geoffrey and Robert, 2000; Drees et al., 2005; Yamada et al., 2005), respectively. Considering that a transcript encoding a mutant cadherin was upregulated in predator-exposed brains as well, these patterns suggest that the actin cytoskeleton is affected by predator-exposure and that changes in cellular morphology and motility may be involved.
Overrepresented Gene Ontology Terms and KEGG Pathway Reconstruction in Predator-Stressed Brain Tissue
Relative to the list of DE transcript annotations in this study, the overrepresented GO terms enriched in the brains of S. frugiperda after predator exposure were generally restricted to three biochemical pathways: (1) chromatin and macromolecule binding, (2) glutamate synthesis and metabolism, and (3) aminoacylase activity, although terms related to oxidoreductase activity, flavin mononucleotide binding, and integrin signaling also were overrepresented. To the best of our knowledge, these GO terms have not been implicated in any other study of predator-induced transcription. The small set of GO-annotated DE transcripts identified here limit the statistical detection of subtly over- and under-represented terms; regardless, we found 15 GO terms to be highly significantly overrepresented in our set of annotated DE transcripts relative to the frequency at which these terms were found in our GO-annotated transcriptome (p < 0.0004). Chromatin binding (p < 0.0000) and macromolecular complex binding (p < 0.0000) were the most highly overrepresented GO terms identified both with 7 out of 102 GO-annotated DE transcripts mapped to these terms. The binding of cellular proteins to chromatin can elicit varied cellular responses, such as transcriptional regulation, DNA replication, and chromatin remodeling (Ricke and Bielinsky, 2005). Considering that transcripts mapping to ash1 and Pb-1 protein annotations were also differentially regulated, the presence of these GO terms again implies that epigenetic modifications seem to be induced upon exposure to predator cues.
The set of GO terms pertaining to glutamate synthesis and metabolism included glutamate synthase activity, as well as glutamate biosynthesis and metabolism, glutamine family amino acid biosynthesis, and dicarboxylic acid biosynthesis and metabolism. Glutamate, an amino acid anion derived from its dicarboxylic state, glutamic acid, is used during protein synthesis, but is the most abundant excitatory neurotransmitter in the vertebrate brain (Locatelli, 2005). Although acetylcholine is the primary excitatory neurotransmitter in the insect nervous system (Wnuk et al., 2014), glutamate also plays an excitatory role (Leboulle, 2012) as glutamate immunoreactivity (Sinakevitch et al., 2001) and glutamate-induced ion currents (Cayre et al., 1999) have been observed in insect neurons. Intriguingly, application of glutamate to the mushroom body brain regions of the honeybee, Apis mellifera, facilitates glutamatergic neurotransmission and olfactory learning (Locatelli, 2005), and glutamate-mediated neurotransmission has also been implicated in the visual and tactile (Liang et al., 2012) sensory systems. Notably, one of the strongly upregulated (log2FC = 5.70) transcripts we found mapped to a fragment of the ionotropic receptor 75d (IR75d). Benton et al. (2009) found that IR75d and 69 other IR-family proteins carry ionotropic glutamate receptor-like amino acid positions and surmised that IRs may similarly act in chemosensory neuron signaling. Although 21 of these 69 novel IRs showed transcriptional responses to chemical signals in the Drosophila antenna, including IR75d, the remaining 46 displayed no chemosensory-related expression (Benton et al., 2009). Further, the presence of different IR subtypes on a given neuron also influences synaptogenesis, synaptic activity, and experience-dependent neural plasticity in Drosophila (Thomas and Sigrist, 2012). Knowing that biochemical pathways pertaining to glutamate production were altered in the brains of predator-cue exposed S. frugiperda coupled with evidence that IR75d was upregulated post-exposure, we suggest that IR75d and its relatives may be involved in the development and function of auditory mechanosensory neurons.
Manual analysis of the KEGG pathway reconstruction of DE transcripts revealed a variety of interconnected neuron-specific metabolic and signaling cascades that were affected by bat ultrasound exposure, including the mechanistic target of rapamycin (mTOR)/Akt, MAPK, Wnt, prolactin, Hippo, and calcium signaling systems, and associated regulatory responses, such as p53, renin-angiotensin, and NF-κB transcript expression. Notably, recent research on the conserved function of these biochemical pathways in the nervous systems of metazoan taxa across phyla describes the function and biological relevance of these pathways on an organismal scale (Mattson and Camandola, 2001; Lilienbaum and Israe, 2003; Pan, 2007; Lau and Bading, 2009; Tedeschi and Di Giovanni, 2009; Brown et al., 2012; Lin et al., 2012; Graber et al., 2013; Flentke et al., 2014; Mao et al., 2014; Patil et al., 2014; Layden et al., 2016; Guo et al., 2017; Haspula and Clark, 2018). For instance, synaptic glutamate (Sinakevitch et al., 2010; Thomas and Sigrist, 2012; Li et al., 2016), mTOR/Akt (Guo et al., 2017), intracellular calcium (Kaltschmidt et al., 2005; Lau and Bading, 2009), and prolactin signaling (Brown et al., 2012; Belugin et al., 2013), followed by differential p53 and NF-κB transcription (Kaltschmidt et al., 2005; Lau and Bading, 2009) are each implicated in the apoptotic and synaptic-activity mediated induction of neural plasticity, learning, and memory from diverse taxa spanning arthropods to chordates. Clearly, much work remains to divulge how the vast evolutionary divergences inherent between the conserved physiological cellular signaling and gene networks of most, if not all, metazoan taxa correlate with lineage and ecology-specific organismal responses to diverse stressors, including predation risk.
Conclusion and Future Directions
This study demonstrates that exposure to ecologically relevant auditory cues of predation risk in S. frugiperda results in varied but strong patterns of up- and down-regulation of a broad range of protein products within the moth brain. The most strongly up- and down-regulated transcripts found in this study correspond to many cellular functions which include mitochondrial metabolism, glutamate synthesis and metabolism, actin cytoskeleton morphology and motion, axon guidance, neural structure, and epigenetic modifications. This is a promising first step in developing a model for the transcriptional impacts of frequent and repeated exposure to bat predation cues in S. frugiperda, which may represent acute and chronic responses of cells to predator-induced stress. Several novel predator-cue induced transcriptional pathways are implicated in these results and present promising opportunities for future research. These broad predator-induced transcriptional responses are characteristic of those found in previous studies, such as in predator-stressed stickleback fish (Sanogo et al., 2011), Daphnia (Rozenberg et al., 2015), and the Hokkaido salamander (Matsunami et al., 2015). Contrary to our expectations, there is little overlap between previously reported responses to predator-induced stress, such as neuropeptide production and increased antioxidant activity, and the novel predator-induced functional annotations reported here. However, mitoferrin, a solute carrier responsible for iron uptake by red blood cells in vertebrates, was significantly upregulated in the brains of stickleback fish repeatedly exposed to a chemical cue of predation (Sanogo et al., 2011), although it was downregulated (log2FC = -6.06) in S. frugiperda post-exposure. In insects, the function of mitoferrin is less well understood, though D. melanogaster with mitoferrin mutations experienced problems with spermatogenesis and development to adulthood (Metzendorf and Lind, 2010). Apart from this similarity, the novel transcriptional responses to predation in S. frugiperda observed here may be specialized to auditory perception or found only in Lepidoptera. Furthermore, although efforts were made to avoid auditory habituation in this study, expression profiles described here bear similarities to past studies of bird-song habituation in the brains of zebra finches (Taeniopygia guttata), with both resulting in the downregulation of genes pertaining to cytoskeletal dynamics and mitochondrial metabolism (Dong et al., 2009).
One primary limitation of our study is a lack of time-series expression data that would have bolstered our ability to infer the functional relevance of specific transcripts for both short- and long-term physiological acclimations to auditory cues of predation. Further work, such as comparing expression profiles through time and between frequent and infrequent cue exposures, would aid in parsing the effects due to neural habituation/auditory stimulation, per se, and those related specifically to predator cue exposure. Specifically, producing a detailed time-course transcriptional profile of tissue-specific prey physiology beginning after the first moments of predator-cue exposure and proceeding over the course of hours to days in cue-exposed S. frugiperda or other predator–prey systems would provide comparative insights into the temporal dynamics of stress-induced transcription during acute relative to prolonged exposure to predation risk. Another limitation of this study is a lack of transcript validation via quantitative reverse-transcriptase polymerase chain reaction assays, yet we argue the novelty of the system and the foundational datasets we have produced that can inform future hypotheses warrant their use by the scientific community. Another confounding factor that may have contributed to the relatively noisy patterns of expression in exposed S. frugiperda brains shown here is the type of auditory stimulus we used. For instance, by using three bat calls from three different species, we have endeavored to replicate an ecologically relevant cue of predation risk, yet the nightly soundscape a moth is exposed to in situ varies hour-to-hour and night-to-night in sound intensity, conspecific and interspecific composition, and many other attributes that we did not incorporate into our experiments. We encourage future investigators to develop high quality, ultrasonic soundscape recordings in relevant field settings ahead of time, when possible, and replicate these via nightly broadcasts of each night’s recording. In conclusion, as more diverse, annotated insect genomes become available and the function of more genes are elucidated by experimental and comparative evidence, studies that assess the physiological effects of prolonged predation risk on prey across the tree of life will continue to divulge remarkably conserved patterns of stress-induced molecular mechanisms between lineages.
Author Contributions
SC conducted the experiments and developed this report. ST contributed to the conceptual development, logistical support, and proofreading of this work.
Funding
This work was funded through the University of Illinois at Urbana-Champaign NSF IGERT grant (NSF DGE IGERT-1069157) and fellowship support to SC from the Smithsonian Tropical Research Institute and the School of Integrative Biology at the University of Illinois at Urbana-Champaign. Additional support was provided by Illinois Natural History Survey research funds to ST. We thank the United States Department of Agriculture Animal and Plant Health Inspection Service for a permit (no. P526P-15-04080) allowing the purchase of S. frugiperda larvae. Much of the computing done for this work was conducted on the Biocluster High Performance Computing resource for the Carl R. Woese Institute for Genomic Biology at the University of Illinois at Urbana-Champaign. This research was based upon work supported by the National Science Foundation under grant no. ABI-1458641 to Indiana University.
Conflict of Interest Statement
The authors declare that the research was conducted in the absence of any commercial or financial relationships that could be construed as a potential conflict of interest.
Acknowledgments
An earlier version of this work was first made available online via the University of Illinois at Urbana-Champaign’s master’s thesis archive in 2016. We thank Dr. Inga Geipel, Dr. Kirsten Jung, Dr. Amy Cash Ahmed, Dr. Daniel Llano, Dr. May Berenbaum, Dr. Mark Davis, Dr. Jenny Drnevich, Dr. Alvaro Hernandez, Dr. Chris Fields, Dr. Beryl Jones, Dr. Rachel Page, Gosha Yudintsev, Daniel Bush, Luke Zehr, and Ian Traniello for their generous support at many steps throughout this research. We are grateful to the reviewers for their insightful and highly constructive comments during the peer review process. Publication of this article was funded in part by the University of Florida Open Access Publishing Fund.
Footnotes
- ^https://sourceforge.net/projects/cdbfasta/
- ^https://www.ncbi.nlm.nih.gov/bioproject/PRJNA318819
- ^https://github.com/cinel1/FallArmyworm.git
References
Abramsky, A. Z., Strauss, E., Subach, A., Kotler, B. P., Riechman, A., Url, S., et al. (2014). International association for ecology the effect of barn owls (Tyto alba) on the activity and microhabitat selection of Gerbillus. Oecologia 105, 313–319. doi: 10.1007/BF00328733
Acharya, L., and McNeil, J. N. (1998). Predation risk and mating behavior: the responses of moths to bat-like ultrasound. Behav. Ecol. 9, 552–558. doi: 10.1093/beheco/9.6.552
Adamo, S. A. (2010). Why should an immune response activate the stress response? Insights from the insects (the cricket Gryllus texensis). Brain Behav. Immun. 24, 194–200. doi: 10.1016/j.bbi.2009.08.003
Adamo, S. A. (2017a). Stress responses sculpt the insect immune system, optimizing defense in an ever-changing world. Dev. Comp. Immunol. 66, 24–32. doi: 10.1016/j.dci.2016.06.005
Adamo, S. A. (2017b). The stress response and immune system share, borrow, and reconfigure their physiological network elements: evidence from the insects. Horm. Behav. 88, 25–30. doi: 10.1016/j.yhbeh.2016.10.003
Adamo, S. A., Kovalko, I., and Mosher, B. (2013). The behavioural effects of predator-induced stress responses in the cricket (Gryllus texensis): the upside of the stress response. J. Exp. Biol. 216, 4608–4614. doi: 10.1242/jeb.094482
An, S., Yeo, K. J., Jeon, Y. H., and Song, J. J. (2011). Crystal structure of the human histone methyltransferase ASH1L catalytic domain and its implications for the regulatory mechanism. J. Biol. Chem. 286, 8369–8374. doi: 10.1074/jbc.M110.203380
Andrews, S. (2010). FastQC: A Quality Control Tool for High Throughput Sequence Data. [WWW Document]. Available at: http://www.Bioinformatics.Babraham.Ac.Uk/Projects/Fastqc/
Aruda, A. M., Baumgartner, M. F., Reitzel, A. M., and Tarrant, A. M. (2011). Heat shock protein expression during stress and diapause in the marine copepod Calanus finmarchicus. J. Insect. Physiol. 57, 665–675. doi: 10.1016/j.jinsphys.2011.03.007
Barrientos, T., Frank, D., Kuwahara, K., Bezprozvannaya, S., Pipes, G. C. T., Bassel-Duby, R., et al. (2007). Two novel members of the ABLIM protein family, ABLIM-2 and -3, associate with STARS and directly bind F-actin. J. Biol. Chem. 282, 8393–8403. doi: 10.1074/jbc.M607549200
Bechtold, M., Schultz, J., and Bogdan, S. (2014). Fhod proteins in actin dynamics-a formin’ class of its own. Small GTPases 5, 1–6. doi: 10.4161/21541248.2014.973765
Belugin, S., Diogenes, A. R., Patil, M. J., Ginsburg, E., Henry, M. A., and Akopian, A. N. (2013). Mechanisms of transient signaling via short and long prolactin receptor isoforms in female and male sensory neurons. J. Biol. Chem. 288, 34943–34955. doi: 10.1074/jbc.M113.486571
Benjamini, Y., and Heller, R. (2007). False discovery rates for spatial signals. J. Am. Stat. Assoc. 102, 1272–1281. doi: 10.1198/016214507000000941
Benjamini, Y., and Hochberg, Y. (1995). Controlling the false discovery rate: a practical and powerful approach to multiple testing. J. R. Stat. Soc. B 57, 289–300. doi: 10.2307/2346101
Benton, R., Vannice, K. S., Gomez-Diaz, C., and Vosshall, L. B. (2009). Variant ionotropic glutamate receptors as chemosensory receptors in Drosophila. Cell 136, 149–162. doi: 10.1016/j.cell.2008.12.001
Bhalerao, S., and Clandinin, T. R. (2012). Vitamin K2 takes charge. Science 336, 1241–1242. doi: 10.1126/science.1223812
Bolger, A. M., Lohse, M., and Usadel, B. (2014). Trimmomatic: a flexible trimmer for Illumina sequence data. Bioinformatics 30, 2114–2120. doi: 10.1093/bioinformatics/btu170
Boutet, E., Lieberherr, D., Tognolli, M., Schneider, M., Bansal, P., Bridge, A. J., et al. (2016). Uniprotkb/swiss-prot, the manually annotated section of the uniprot knowledgebase. Methods Mol. Biol. 1374, 23–54. doi: 10.1007/978-1-4939-3167-5_2
Bray, N. L., Pimentel, H., Melsted, P., and Pachter, L. (2016). Near-optimal probabilistic RNA-seq quantification. Nat. Biotechnol. 34, 525–527. doi: 10.1038/nbt.3519
Brown, R. S. E., Piet, R., Herbison, A. E., and Grattan, D. R. (2012). Differential actions of prolactin on electrical activity and intracellular signal transduction in hypothalamic neurons. Endocrinology 153, 2375–2384. doi: 10.1210/en.2011-2005
Camacho, C., Coulouris, G., Avagyan, V., Ma, N., Papadopoulos, J., Bealer, K., et al. (2009). BLAST+: architecture and applications. BMC Bioinformatics 10:421. doi: 10.1186/1471-2105-10-421
Cayre, M., Buckingham, S. D., Yagodin, S., and Sattelle, D. B. (1999). Cultured insect mushroom body neurons express functional receptors for acetylcholine, GABA, glutamate, octopamine, and dopamine. J. Neurophysiol. 81, 1–14. doi: 10.1152/jn.1999.81.1.1
Chandrasekaran, R., and Thompson, M. (2007). Polybromo-1-bromodomains bind histone H3 at specific acetyl-lysine positions. Biochem. Biophys. Res. Commun. 355, 661–666. doi: 10.1016/j.bbrc.2007.01.193
Chu, N. D., Miller, L. P., Kaluziak, S. T., Trussell, G. C., and Vollmer, S. V. (2014). Thermal stress and predation risk trigger distinct transcriptomic responses in the intertidal snail Nucella lapillus. Mol. Ecol. 23, 6104–6113. doi: 10.1111/mec.12994
Clinchy, M., Sheriff, M. J., and Zanette, L. Y. (2013). Predator-induced stress and the ecology of fear. Funct. Ecol. 27, 56–65. doi: 10.1111/1365-2435.12007
Cohen, A. C. (2001). Formalizing insect rearing and artificial diet technology. Am. Entomol. 47, 198–206. doi: 10.1093/ae/47.4.198
Contreras, L., Drago, I., Zampese, E., and Pozzan, T. (2010). Mitochondria: the calcium connection. Biochim. Biophys. Acta Bioenerg. 1797, 607–618. doi: 10.1016/j.bbabio.2010.05.005
Corcoran, A. J., Barber, J. R., and Conner, W. E. (2009). Tiger moth jams bat sonar. Science 325, 325–327. doi: 10.1126/science.1174096
Dong, S., Replogle, K. L., Hasadsri, L., Imai, B. S., Yau, P. M., Rodriguez-Zas, S., et al. (2009). Discrete molecular states in the brain accompany changing responses to a vocal signal. Proc. Natl. Acad. Sci. U.S.A. 106, 11364–11369. doi: 10.1073/pnas.0812998106
Drees, F., Pokutta, S., Yamada, S., Nelson, W. J., and Weis, W. I. (2005). α-catenin is a molecular switch that binds E-cadherin-β-catenin and regulates actin-filament assembly. Cell 123, 903–915. doi: 10.1016/j.cell.2005.09.021
Edsbagge, J., Zhu, S., Xiao, M. Y., Wigström, H., Mohammed, A. H., and Semb, H. (2004). Expression of dominant negative cadherin in the adult mouse brain modifies rearing behavior. Mol. Cell. Neurosci. 25, 524–535. doi: 10.1016/j.mcn.2003.12.005
Elvira, S., Gorría, N., Muñoz, D., Williams, T., and Caballero, P. (2010). A Simplified Low-Cost Diet for Rearing Spodoptera exigua (Lepidoptera: Noctuidae) and Its Effect on S. exigua Nucleopolyhedrovirus Production. J. Econ. Entomol. 103, 17–24. doi: 10.1603/EC09246
Endler, J. A. (1991). “Interactions between predators and prey,” in Behevioural Ecology: An Evolutionary Approach, eds J. A. Krebs and N. B. Davies (Oxford: Blackwell Scientific), 169–196. doi: 10.4319/lo.2013.58.2.0489
Ernster, L., and Dallner, G. (1995). Biochemical, physiological and medical aspects of ubiquinone function. BBA Mol. Basis Dis. 1271, 195–204. doi: 10.1016/0925-4439(95)00028-3
Even, N., Devaud, J.-M., and Barron, A. (2012). General Stress Responses in the Honey Bee. Insects 3, 1271–1298. doi: 10.3390/insects3041271
Flentke, G. R., Klingler, R. H., Tanguay, R. L., Carvan, M. J., and Smith, S. M. (2014). An evolutionarily conserved mechanism of calcium-dependent neurotoxicity in a zebrafish model of fetal alcohol spectrum disorders. Alcohol. Clin. Exp. Res. 38, 1255–1265. doi: 10.1111/acer.12360
Fleshner, M., Campisi, J., Amiri, L., and Diamond, D. M. (2004). Cat exposure induces both intra- and extracellular Hsp72: the role of adrenal hormones. Psychoneuroendocrinology 29, 1142–1152. doi: 10.1016/j.psyneuen.2004.01.007
Fung, S., Wang, F., Spindler, S. R., and Hartenstein, V. (2009). Drosophila E-cadherin and its binding partner Armadillo/ β-catenin are required for axonal pathway choices in the developing larval brain. Dev. Biol. 332, 371–382. doi: 10.1016/j.ydbio.2009.06.005
Gakovic, M., Shu, X., Kasioulis, I., Carpanini, S., Moraga, I., and Wright, A. F. (2011). The role of RPGR in cilia formation and actin stability. Hum. Mol. Genet. 20, 4840–4850. doi: 10.1093/hmg/ddr423
Gesi, M., Fornai, F., Lenzi, P., Ferrucci, M., Soldani, P., Ruffoli, R., et al. (2002). Morphological alterations induced by loud noise in the myocardium: the role of benzodiazepine receptors. Microsc. Res. Tech. 59, 136–146. doi: 10.1002/jemt.10186
Graber, T. E., McCamphill, P. K., and Sossin, W. S. (2013). A recollection of mTOR signaling in learning and memory. Learn. Mem. 20, 518–530. doi: 10.1101/lm.027664.112
Grabherr, M. G., Haas, B. J., Yassour, M., Levin, J. Z., Thompson, D. A., Amit, I., et al. (2011). Full-length transcriptome assembly from RNA-Seq data without a reference genome. Nat. Biotechnol. 29, 644–652. doi: 10.1038/nbt.1883
Guo, J.-N., Tian, L.-Y., Liu, W.-Y., Mu, J., and Zhou, D. (2017). Activation of the Akt/mTOR signaling pathway: a potential response to long-term neuronal loss in the hippocampus after sepsis. Neural. Regen. Res. 12, 1832–1842. doi: 10.4103/1673-5374.219044
Haas, B. J., Papanicolaou, A., Yassour, M., Grabherr, M., Blood, P. D., Bowden, J., et al. (2013). De novo transcript sequence reconstruction from RNA-seq using the Trinity platform for reference generation and analysis. Nat. Protoc. 8, 1494–1512. doi: 10.1038/nprot.2013.084
Harris, B. N., and Carr, J. A. (2016). The role of the hypothalamus-pituitary-adrenal/interrenal axis in mediating predator-avoidance trade-offs. Gen. Comp. Endocrinol. 23, 110–142. doi: 10.1016/j.ygcen.2016.04.006
Haspula, D., and Clark, M. A. (2018). Molecular basis of the brain renin angiotensin system in cardiovascular and neurologic disorders: uncovering a key role for the astroglial angiotensin type 1 receptor AT1R. J. Pharmacol. Exp. Ther. 366, 251–264. doi: 10.1124/jpet.118.248831
Hirano, S., and Takeichi, M. (2012). Cadherins in brain morphogenesis and wiring. Physiol. Rev. 92, 597–634. doi: 10.1152/physrev.00014.2011
Huang, F., and Subramanyam, B. (2004). Behavioral and reproductive effects of ultrasound on the Indian meal moth, Plodia Interpunctella. Entomol. Exp. Appl. 113, 157–164. doi: 10.1111/j.0013-8703.2004.00217.x
Huang, F., Subramanyam, B., and Taylor, R. (2003). Ultrasound affects spermatophore transfer, larval numbers, and larval weight of Plodia interpunctella (Hübner) (Lepidoptera: Pyralidae). J. Stored Prod. Res. 39, 413–422. doi: 10.1016/S0022-474X(02)00035-8
Jung, K., Kalko, E. K. V., and Von Helversen, O. (2007). Echolocation calls in Central American emballonurid bats: signal design and call frequency alternation. J. Zool. 272, 125–137. doi: 10.1111/j.1469-7998.2006.00250.x
Kaltschmidt, B., Widera, D., and Kaltschmidt, C. (2005). Signaling via NF-κB in the nervous system. Biochim. Biophys. Acta Mol. Cell Res. 1745, 287–299. doi: 10.1016/j.bbamcr.2005.05.009
Kanehisa, M., Sato, Y., and Morishima, K. (2016). BlastKOALA and GhostKOALA: KEGG tools for functional characterization of genome and metagenome sequences. J. Mol. Biol. 428, 726–731. doi: 10.1016/j.jmb.2015.11.006
Katoh, M., and Katoh, M. (2004). Identification and characterization of human FHOD3 gene in silico. Int. J. Mol. Med. 13, 615–620. doi: 10.3892/ijmm.13.4.615
Khanna, H., Hurd, T. W., Lillo, C., Shu, X., Parapuram, S. K., He, S., et al. (2005). RPGR-ORF15, which is mutated in retinitis pigmentosa, associates with SMC1. SMC3, and microtubule transport proteins. J. Biol. Chem. 280, 33580–33587. doi: 10.1074/jbc.M505827200
Kight, C. R., and Swaddle, J. P. (2011). How and why environmental noise impacts animals: an integrative, mechanistic review. Ecol. Lett. 14, 1052–1061. doi: 10.1111/j.1461-0248.2011.01664.x
Kirkpatrick, R. L., and Harein, P. K. (1965). Inhibition of reproduction of indian-meal moths, Plodia interpunctella, by exposure to amplified sound. J. Econ. Entomol. 58, 920–921. doi: 10.1093/jee/58.5.920
Langfelder, P., Zhang, B., and Horvath, S. (2008). Defining clusters from a hierarchical cluster tree: the Dynamic Tree Cut package for R. Bioinformatics 24, 719–720. doi: 10.1093/bioinformatics/btm563
Lau, D., and Bading, H. (2009). Synaptic activity-mediated suppression of p53 and induction of nuclear calcium-regulated neuroprotective genes promote survival through inhibition of mitochondrial permeability transition. J. Neurosci. 29, 4420–4429. doi: 10.1523/JNEUROSCI.0802-09.2009
Law, C. W., Chen, Y., Shi, W., and Smyth, G. K. (2014). Voom: precision weights unlock linear model analysis tools for RNA-seq read counts. Genome Biol. 15:R29. doi: 10.1186/gb-2014-15-2-r29
Layden, M. J., Johnston, H., Amiel, A. R., Havrilak, J., Steinworth, B., Chock, T., et al. (2016). MAPK signaling is necessary for neurogenesis in Nematostella vectensis. BMC Biol. 14:61. doi: 10.1186/s12915-016-0282-1
Leboulle, G. (2012). “Glutamate Neurotransmission in the Honey Bee Central Nervous System,” in Honeybee Neurobiology and Behavior, eds C. Galizia, D. Eisenhardt, and M. Giurfa (Dordrecht: Springer), 171–184. doi: 10.1007/978-94-007-2099-2_14
Leder, E. H., Merilä, J., and Primmer, C. R. (2009). A flexible whole-genome microarray for transcriptomics in three-spine stickleback (Gasterosteus aculeatus). BMC Genomics 10:426. doi: 10.1186/1471-2164-10-426
Leek, J. T., Johnson, W. E., Parker, H. S., Jaffe, A. E., and Storey, J. D. (2012). The SVA package for removing batch effects and other unwanted variation in high-throughput experiments. Bioinformatics 28, 882–883. doi: 10.1093/bioinformatics/bts034
Leek, J. T., Scharpf, R. B., Bravo, H. C., Simcha, D., Langmead, B., Johnson, W. E., et al. (2010). Tackling the widespread and critical impact of batch effects in high-throughput data. Nat Rev Genet 11, 733–739. doi: 10.1038/nrg2825
Li, Y., Dharkar, P., Han, T. H., Serpe, M., Lee, C. H., and Mayer, M. L. (2016). Novel functional properties of Drosophila CNS glutamate receptors. Neuron 92, 1036–1048. doi: 10.1016/j.neuron.2016.10.058
Liang, Z. S., Nguyen, T., Mattila, H. R., Rodriguez-Zas, S. L., Seeley, T. D., and Robinson, G. E. (2012). Molecular determinants of scouting behavior in honey bees. Science 335, 1225–1228. doi: 10.1126/science.1213962
Lilienbaum, A., and Israe, A. (2003). From Calcium to NF- B signaling pathways in neurons. Society 23, 2680–2698. doi: 10.1128/MCB.23.8.2680
Lima, S. L. (1990). Evolutionarily stable antipredator behavior among isolated foragers: on the consequences of successful escape. J. Theor. Biol. 143, 77–89. doi: 10.1016/S0022-5193(05)80289-9
Lin, W.-C., Chuang, Y.-C., Chang, Y.-S., Lai, M.-D., Teng, Y.-N., Su, I.-J., et al. (2012). Endoplasmic reticulum stress stimulates p53 expression through NF-κB activation. PLoS One 7:e39120. doi: 10.1371/journal.pone.0039120
Locatelli, F. (2005). Focal and temporal release of glutamate in the mushroom bodies improves olfactory memory in Apis mellifera. J. Neurosci. 25, 11614–11618. doi: 10.1523/JNEUROSCI.3180-05.2005
Lodish, H., Berk, A., Zipursky, S. L., Matsudaira, P., Baltimore, D., and Darnell, J. (2000). Receptor Tyrosine Kinases and Ras: Molecular Cell Biology, 4th Edn. New York, NY: W. H. Freeman.
López, L. C., Quinzii, C. M., Area, E., Naini, A., Rahman, S., Schuelke, M., et al. (2010). Treatment of CoQ10 deficient fibroblasts with ubiquinone, CoQ analogs, and vitamin C: time-and compound-dependent effects. PLoS One 5:e11897. doi: 10.1371/journal.pone.0011897
Luo, G. Z., Blanco, M. A., Greer, E. L., He, C., and Shi, Y. (2015). DNA N6-methyladenine: a new epigenetic mark in eukaryotes? Nat. Rev. Mol. Cell Biol. 16, 705–710. doi: 10.1038/nrm4076
MacDonald, J. (2008). Affycoretools: Functions Useful for Those Doing Repetitive Analyses with Affymetrix GeneChips. R Packag version. Available at: https://rdrr.io/github/jmacdon/affycoretools/
Maere, S., Heymans, K., and Kuiper, M. (2005). BiNGO: a Cytoscape plugin to assess overrepresentation of Gene Ontology categories in Biological Networks. Bioinformatics 21, 3448–3449. doi: 10.1093/bioinformatics/bti551
Mao, B., Gao, Y., Bai, Y., and Yuan, Z. (2014). Hippo signaling in stress response and homeostasis maintenance. Acta Biochim. Biophys. Sin. 47, 2–9. doi: 10.1093/abbs/gmu109
Marchi, S., and Pinton, P. (2014). The mitochondrial calcium uniporter complex: molecular components, structure and physiopathological implications. J. Physiol. 592, 829–839. doi: 10.1113/jphysiol.2013.268235
Matsunami, M., Kitano, J., Kishida, O., Michimae, H., Miura, T., and Nishimura, K. (2015). Transcriptome analysis of predator- and prey-induced phenotypic plasticity in the Hokkaido salamander (Hynobius retardatus). Mol. Ecol. 24, 3064–3076. doi: 10.1111/mec.13228
Mattson, M. P., and Camandola, S. (2001). NF-κB in neuronal plasticity and neurodegenerative disorders. J. Clin. Invest. 107, 247–254. doi: 10.1172/JCI11916
Metzendorf, C., and Lind, M. I. (2010). Drosophila mitoferrin is essential for male fertility: evidence for a role of mitochondrial iron metabolism during spermatogenesis. BMC Dev. Biol. 10:68. doi: 10.1186/1471-213X-10-68
Miyamae, T., Seki, M., Naga, T., Uchino, S., Asazuma, H., Yoshida, T., et al. (2013). Increased oxidative stress and coenzyme Q10 deficiency in juvenile fibromyalgia: amelioration of hypercholesterolemia and fatigue by ubiquinol-10 supplementation. Redox Rep. 18, 12–19. doi: 10.1179/1351000212Y.0000000036
Mora, E. C., Fernández, Y., Hechavarría, J., and Pérez, M. (2014). Tone-deaf ears in moths may limit the acoustic detection of two-tone bats. Brain Behav. Evol. 83, 275–285. doi: 10.1159/000361035
Mora, E. C., Macías, S., Vater, M., Coro, F., and Kössl, M. (2004). Specializations for aerial hawking in the echolocation system of Molossus molossus (Molossidae, Chiroptera). J. Comp. Physiol. A Neuroethol. Sens. Neural. Behav. Physiol. 190, 561–574. doi: 10.1007/s00359-004-0519-2
Müllner, D. (2013). fastcluster: fast hierarchical, agglomerative clustering routines for R and Python. J. Stat. Softw. 53, 1–18. doi: 10.18637/jss.v053.i09
Musacchia, F., Basu, S., Petrosino, G., Salvemini, M., and Sanges, R. (2015). Annocript: a flexible pipeline for the annotation of transcriptomes able to identify putative long noncoding RNAs. Bioinformatics 31, 2199–2201. doi: 10.1093/bioinformatics/btv106
Nanda, S. A., Qi, C., Roseboom, P. H., and Kalin, N. H. (2008). Predator stress induces behavioral inhibition and amygdala somatostatin receptor 2 gene expression. Genes Brain Behav. 7, 639–648. doi: 10.1111/j.1601-183X.2008.00401.x
Norman, A. P., and Jones, G. (2000). Size, peripheral auditory tuning and target strength in noctuid moths. Physiol. Entomol. 25, 346–353. doi: 10.1046/j.1365-3032.2000.00203.x
Pan, D. (2007). Hippo signaling in organ size control. Genes Dev. 21, 886–897. doi: 10.1101/gad.1536007
Patil, M. J., Henry, M. A., and Akopian, A. N. (2014). Prolactin receptor in regulation of neuronal excitability and channels. Channels 8, 193–202. doi: 10.4161/chan.28946
Pauwels, K., Stoks, R., and De Meester, L. (2005). Coping with predator stress: interclonal differences in induction of heat-shock proteins in the water flea Daphnia magna. J. Evol. Biol. 18, 867–872. doi: 10.1111/j.1420-9101.2005.00890.x
Pearce, S. L., Clarke, D. F., East, P. D., Elfekih, S., Gordon, K. H. J., Jermiin, L. S., et al. (2017). Genomic innovations, transcriptional plasticity and gene loss underlying the evolution and divergence of two highly polyphagous and invasive Helicoverpa pest species. BMC Biol. 15:63. doi: 10.1186/s12915-017-0402-6
Pfuhl, G., Kalinova, B., Valterova, I., and Berg, B. G. (2015). Simple ears - flexible behavior: Information processing in the moth auditory pathway. Curr. Zool. 61, 292–302. doi: 10.1093/czoolo/61.2.292
Preisser, E. L. (2009). The physiology of predator stress in free-ranging prey. J. Anim. Ecol. 78, 1103–1105. doi: 10.1111/j.1365-2656.2009.01602.x
Pumphrey, R. J. (1940). Hearing in insects. Biol. Rev. 15, 107–132. doi: 10.1111/j.1469-185X.1940.tb00944.x
R Core Team. (2014). R: A Language and Environment for Statistical Computing. Vienna: R Core Team. R Foundation Statistical Computation.
Ratcliffe, J. M., and Fullard, J. H. (2005). The adaptive function of tiger moth clicks against echolocating bats: an experimental and synthetic approach. J. Exp. Biol. 208, 4689–4698. doi: 10.1242/jeb.01927
Ratcliffe, J. M., Fullard, J. H., Arthur, B. J., and Hoy, R. R. (2011). Adaptive auditory risk assessment in the dogbane tiger moth when pursued by bats. Proc. R. Soc. B Biol. Sci. 278, 364–370. doi: 10.1098/rspb.2010.1488
Ratcliffe, J. M., Soutar, A. R., Muma, K. E., Guignion, C., and Fullard, J. H. (2008). Anti-bat flight activity in sound-producing versus silent moths. Can. J. Zool. 86, 582–587. doi: 10.1139/Z08-024
Rau, A., Gallopin, M., Celeux, G., and Jaffrézic, F. (2013). Data-based filtering for replicated high-throughput transcriptome sequencing experiments. Bioinformatics 29, 2146–2152. doi: 10.1093/bioinformatics/btt350
Ricke, R. M., and Bielinsky, A. K. (2005). Easy detection of chromatin binding proteins by the histone association assay. Biol. Proc. Online 7, 60–69. doi: 10.1251/bpo106
Ritchie, M. E., Phipson, B., Wu, D., Hu, Y., Law, C. W., Shi, W., et al. (2015). Limma powers differential expression analyses for RNA-sequencing and microarray studies. Nucleic Acids Res. 43:e47. doi: 10.1093/nar/gkv007
Robinson, M. D., McCarthy, D. J., and Smyth, G. K. (2009). edgeR: a Bioconductor package for differential expression analysis of digital gene expression data. Bioinformatics 26, 139–140. doi: 10.1093/bioinformatics/btp616
Robinson, M. D., and Oshlack, A. (2010). A scaling normalization method for differential expression analysis of RNA-seq data. Genome Biol. 11:R25. doi: 10.1186/gb-2010-11-3-r25
Roeder, K. D. (1966). Auditory system of noctuid moths. Science 154, 1515–1521. doi: 10.1126/science.154.3756.1515
Ross, T. S., Jefferson, A. B., Mitchell, C. A., and Majerus, P. W. (1991). Cloning and expression of human 75-kDa inositol polyphosphate-5-phosphatase. J. Biol. Chem. 266, 20283–20289.
Roszkowski, M., Manuella, F., von Ziegler, L., Durán-Pacheco, G., Moreau, J.-L., Mansuy, I. M., et al. (2016). Rapid stress-induced transcriptomic changes in the brain depend on beta-adrenergic signaling. Neuropharmacology 107, 329–338. doi: 10.1016/j.neuropharm.2016.03.046
Rozenberg, A., Parida, M., Leese, F., Weiss, L. C., Tollrian, R., and Manak, J. R. (2015). Transcriptional profiling of predator-induced phenotypic plasticity in Daphnia pulex. Front. Zool. 12:18. doi: 10.1186/s12983-015-0109-x
Rydell, J., Kaerma, S., Hedelin, H., and Skals, N. (2003). Evasive response to ultrasound by the crepuscular butterfly Manataria maculata. Naturwissenschaften 90, 80–83. doi: 10.1007/s00114-002-0391-2
Salvetti, F., Chelli, B., Gesi, M., Pellegrini, A., Giannaccini, G., Lucacchini, A., et al. (2000). Effect of noise exposure on rat cardiac peripheral benzodiazepine receptors. Life Sci. 66, 1165–1175. doi: 10.1016/S0024-3205(00)00422-7
Sanogo, Y. O., Hankison, S., Band, M., Obregon, A., and Bell, A. M. (2011). Brain transcriptomic response of threespine sticklebacks to cues of a predator. Brain Behav. Evol. 77, 270–285. doi: 10.1159/000328221
Schwarzenberger, A., Courts, C., and von Elert, E. (2009). Target gene approaches: gene expression in Daphnia magna exposed to predator-borne kairomones or to microcystin-producing and microcystin-free Microcystis aeruginosa. BMC Genomics 10:527. doi: 10.1186/1471-2164-10-527
Shannon, P., Markiel, A., Ozier, O., Baliga, N. S., Wang, J. T., Ramage, D., et al. (2003). Cytoscape: a software Environment for integrated models of biomolecular interaction networks. Genome Res. 13, 2498–2504. doi: 10.1101/gr.1239303
Sheriff, M. J., and Thaler, J. S. (2014). Ecophysiological effects of predation risk; an integration across disciplines. Oecologia 176, 607–611. doi: 10.1007/s00442-014-3105-5
Simão, F. A., Waterhouse, R. M., Ioannidis, P., Kriventseva, E. V., and Zdobnov, E. M. (2015). BUSCO: assessing genome assembly and annotation completeness with single-copy orthologs. Bioinformatics 31, 3210–3212. doi: 10.1093/bioinformatics/btv351
Sinakevitch, I., Farris, S. M., and Strausfeld, N. J. (2001). Taurine-, aspartate- and glutamate-like immunoreactivity identifies chemically distinct subdivisions of Kenyon cells in the cockroach mushroom body. J. Comp. Neurol. 439, 352–367. doi: 10.1002/cne.1355
Sinakevitch, I., Grau, Y., Strausfeld, N. J., and Birman, S. (2010). Dynamics of glutamatergic signaling in the mushroom body of young adult Drosophila. Neural Dev. 5:10. doi: 10.1186/1749-8104-5-10
Slos, S., and Stoks, R. (2008). Predation risk induces stress proteins and reduces antioxidant defense. Funct. Ecol. 22, 637–642. doi: 10.1111/j.1365-2435.2008.01424.x
Smith-Unna, R., Boursnell, C., Patro, R., Hibberd, J. M., and Kelly, S. (2016). TransRate: reference-free quality assessment of de novo transcriptome assemblies. Genome Res. 26, 1134–1144. doi: 10.1101/gr.196469.115
Smyth, G. K. (2004). Linear models and empirical bayes methods for assessing differential expression in microarray experiments. Stat. Appl. Genet. Mol. Biol. 3, 1–25. doi: 10.2202/1544-6115.1027
Spanier, K. I., Leese, F., Mayer, C., Colbourne, J. K., Gilbert, D., Pfrender, M. E., et al. (2010). Predator-induced defences in Daphnia pulex: selection and evaluation of internal reference genes for gene expression studies with real-time PCR. BMC Mol. Biol. 11:50. doi: 10.1186/1471-2199-11-50
Stelinski, L., Holdcraft, R., and Rodriguez-Saona, C. (2014). Female moth calling and flight behavior are altered hours following pheromone autodetection: possible implications for practical management with mating disruption. Insects 5, 459–473. doi: 10.3390/insects5020459
Surlykke, A., and Kalko, E. K. V. (2008). Echolocating bats cry out loud to detect their prey. PLoS One 3:e2036. doi: 10.1371/journal.pone.0002036
Surlykke, A., and Miller, L. A. (1982). Central branchings of three sensory axons from a moth ear (Agrotis segetum. Noctuidae). J. Insect Physiol. 28, 357–364. doi: 10.1016/0022-1910(82)90048-8
Takahashi, L. K. (2014). Olfactory systems and neural circuits that modulate predator odor fear. Front. Behav. Neurosci. 8:72. doi: 10.3389/fnbeh.2014.00072
Tedeschi, A., and Di Giovanni, S. (2009). The non-apoptotic role of p53 in neuronal biology: enlightening the dark side of the moon. EMBO Rep. 10, 576–583. doi: 10.1038/embor.2009.89
ter Hofstede, H. M., Goerlitz, H. R., Montealegre-Z, F., Robert, D., and Holderied, M. W. (2011). Tympanal mechanics and neural responses in the ears of a noctuid moth. Naturwissenschaften 98, 1057–1061. doi: 10.1007/s00114-011-0851-7
Thomas, U., and Sigrist, S. J. (2012). Glutamate receptors in synaptic assembly and plasticity: case studies on fly NMJs. Adv. Exp. Med. Biol. 970, 3–28. doi: 10.1007/978-3-7091-0932-8_1
Trematerra, P., and Pavan, G. (1995). Ultrasound production in the courtship behaviour of Ephestia cautella (Walk.), E. kuehniella Z. and Plodia interpunctella (Hb.) (Lepidoptera: Pyralidae). J. Stored Prod. Res. 31, 43–48. doi: 10.1016/0022-474X(94)00034-Q
Vanyushin, B. F., Belozersky, A. N., Kokurina, N. A., and Kadirova, D. X. (1968). 5-methylcytosine and 6-methylaminopurine in bacterial DNA [26]. Nature 218, 1066–1067. doi: 10.1038/2181066a0
Wnuk, A., Kostowski, W., Korczyńska, J., Szczuka, A., Symonowicz, B., Bieńkowski, P., et al. (2014). Brain GABA and glutamate levels in workers of two ant species (Hymenoptera: Formicidae): interspecific differences and effects of queen presence/absence. Insect Sci. 21, 647–658. doi: 10.1111/1744-7917.12076
Yack, J. E. (2004). The structure and function of auditory chordotonal organs in insects. Microsc. Res. Tech. 63, 315–337. doi: 10.1002/jemt.20051
Yack, J. E., Kalko, E. K. V., and Surlykke, A. (2007). Neuroethology of ultrasonic hearing in nocturnal butterflies (Hedyloidea). J. Comp. Physiol. A Neuroethol. Sens. Neural Behav. Physiol. 193, 577–590. doi: 10.1007/s00359-007-0213-2
Yamada, S., Pokutta, S., Drees, F., Weis, W. I., and Nelson, W. J. (2005). Deconstructing the cadherin-catenin-actin complex. Cell 123, 889–901. doi: 10.1016/j.cell.2005.09.020
Yamaguchi, K., Matsumoto, H., Ochiai, M., Tsuzuki, S., and Hayakawa, Y. (2012). Enhanced expression of stress-responsive cytokine-like gene retards insect larval growth. Insect Biochem. Mol. Biol. 42, 183–192. doi: 10.1016/j.ibmb.2011.11.009
Zha, Y.-P., Chen, J.-Y., Jin, Z.-B., Wang, C.-B., and Lei, C.-L. (2013). Effects of Ultrasound on the fecundity and development of the cotton bollworm, Helicoverpa armigera (Hübner) (Lepidoptera: Noctuidae). J. Agric. Urban Entomol. 29, 93–98. doi: 10.3954/13-05.1
Zha, Y.-P., Xu, F., Chen, Q.-C., and Lei, C.-L. (2008). Effect of ultrasound on acetylcholinesterase activity in Helicoverpa armigera (Lepidoptera: Noctuidae). Can. Entomol. 140, 563–568. doi: 10.4039/n08-025
Keywords: bat, moth, neurophysiology, stress, predation, Spodoptera frugiperda, transcriptomics, ultrasound
Citation: Cinel SD and Taylor SJ (2019) Prolonged Bat Call Exposure Induces a Broad Transcriptional Response in the Male Fall Armyworm (Spodoptera frugiperda; Lepidoptera: Noctuidae) Brain. Front. Behav. Neurosci. 13:36. doi: 10.3389/fnbeh.2019.00036
Received: 30 September 2018; Accepted: 11 February 2019;
Published: 26 February 2019.
Edited by:
Jacqueline Jeannette Blundell, Memorial University of Newfoundland, CanadaReviewed by:
Tara Susan Perrot, Dalhousie University, CanadaLiana Yvonne Zanette, University of Western Ontario, Canada
Copyright © 2019 Cinel and Taylor. This is an open-access article distributed under the terms of the Creative Commons Attribution License (CC BY). The use, distribution or reproduction in other forums is permitted, provided the original author(s) and the copyright owner(s) are credited and that the original publication in this journal is cited, in accordance with accepted academic practice. No use, distribution or reproduction is permitted which does not comply with these terms.
*Correspondence: Scott D. Cinel, cinel1@ufl.edu