Conceptualizing psychological resilience through resting-state functional MRI in a mentally healthy population: a systematic review
- 1Department of Special Education and Counselling, The Education University of Hong Kong, Hong Kong, Hong Kong SAR, China
- 2Integrated Centre for Wellbeing, The Education University of Hong Kong, Hong Kong, Hong Kong SAR, China
- 3Bioanalytical Laboratory for Educational Sciences, The Education University of Hong Kong, Hong Kong, Hong Kong SAR, China
- 4Department of Counselling and Psychology, Hong Kong Shue Yan University, Hong Kong, Hong Kong SAR, China
- 5Brain and Mind Institute, The Chinese University of Hong Kong, Hong Kong, Hong Kong SAR, China
- 6Department of Health Sciences, The Hong Kong Metropolitan University, Hong Kong, Hong Kong SAR, China
Conceptualizations and operational definitions of psychological resilience vary across resilience neuroimaging studies. Data on the neural features of resilience among healthy individuals has been scarce. Furthermore, findings from resting-state functional magnetic resonance imaging (fMRI) studies were inconsistent across studies. This systematic review summarized resting-state fMRI findings in different modalities from various operationally defined resilience in a mentally healthy population. The PubMed and MEDLINE databases were searched. Articles that focused on resting-state fMRI in relation to resilience, and published before 2022, were targeted. Orbitofrontal cortex, anterior cingulate cortex, insula and amygdala, were reported the most from the 19 included studies. Regions in emotional network was reported the most from the included studies. The involvement of regions like amygdala and orbitofrontal cortex indicated the relationships between emotional processing and resilience. No common brain regions or neural pathways were identified across studies. The emotional network appears to be studied the most in association with resilience. Matching fMRI modalities and operational definitions of resilience across studies are essential for meta-analysis.
1. Introduction
Psychological resilience (from hereafter will be referred to resilience), a critical counteracting factor of psychopathology, is defined as the ability to rebound from a difficult situation. There are multiple conceptualizations of resilience in the field of resilience research. For instance, researchers classified resilience into two separate concepts in earlier studies: state resilience and trait resilience (van der Werff et al., 2013a). State resilience was the temporary and immediate response usually measured immediately after a stress-induced task. On the other hand, trait resilience provided more substantial information, like resilience-related personality constructs, and was more stable like a personality trait van der Werff et al., 2013a). In more recent resilience research, resilience has been conceptualized as an outcome, as a positive adaptation to adversity (Peltonen et al., 2014; Kalisch et al., 2015, 2017). Put it in terms of psychopathology prevention, stress resilience is being able to recover quickly based on experience and adaptation from a stressor exposure (a trauma) (Fleshner et al., 2011). In line with this concept, resilience is further characterized as a process, a dynamic adaptation to adversity over time (Stainton et al., 2019). Change over time is the critical difference between the outcome and process models. This model implied that resilience is not a stable trait, and it can change constantly depending on the situation of the stressor. More recently, another new approach in conceptualizing resilience has been proposed by Kalisch et al. (2019), which adds different layers of protective factors to the process model. This dynamic network approach considers resilience not as an entity but as multiple resilience factors that are responsible to different aspects of lives (Kalisch et al., 2019).
A body of literature was conducted in the past decade to understand the conceptual definition of resilience and the factors that associate with it. In the early years, resilience studies focused on at-risk teens on childhood maltreatment or trauma, which were popular in child psychiatry and developmental psychology (Vernon, 2004). Experience of childhood adversity increases the risk of developing a broad range of types of psychological disorders (Green et al., 2010), while resilience plays a critical role in positively adapting under aversive environment. Later, the focus of resilience research shifted to psychopathology, such as post-traumatic stress disorder (PTSD) and other stress-induced disorders (Horn et al., 2016). The differences between patients and normal controls were the interests of resilience research during that era. Since the comparison were made between patients and normal control, disorder-specific results of resilience were typically found in this type of research. In recent years, more focuses were put on understanding the socioeconomic and neurobiology aspects of individuals, i.e., poverty and the interplay with psychological factors on resilience (Hallegatte et al., 2020; Eaton et al., 2022). The more recent resilience research has steered away from the clinical population and focused on mentally healthy population. When focusing on mentally normal people, the protective factors of resilience are the vital interest.
1.1. Mentally healthy participants on resilience research
Recent resilience research emphasizes the importance of focusing on investigating protective factors rather than psychopathology. In contrast, earlier literature defined resilience based on the developments of clinical cases (Vernon, 2004). Individuals exposed to a stressor (i.e., a trauma or childhood adversity) who did not develop stress-induced disorders (i.e., PTSD) were classified as a high resilience group and comparisons were made with the clinical groups. However, a group of those who did not experience any trauma and have high resilience would be missed in this line of research. Moreover, research on a particular disorder implied disorder-specific mechanisms for resilience (Kalisch et al., 2015), which cannot be generalized to the public. This effect is more evident in neurological research on resilience, as some disorders had genetically prompted neurological differences. Recent neurological and clinical research on resilience started to recruit three specific subject groups to tackle the issue of only identifying disorder-specific mechanisms (van der Werff et al., 2013b; Kennis et al., 2015; Singh et al., 2018; Whittaker et al., 2018; Jeon et al., 2020). Besides the clinical group, the other two groups were random non-clinical individuals. Those related to the clinical cases or who experienced the same trauma as the clinical group but did not develop a psychological disorder were defined as the high resilience group. In addition, a random control group was also included. When comparing the clinical group with the other two groups, the differences in the neurological results may indicate the disorder-specific mechanisms involved (van der Werff et al., 2013b; Kennis et al., 2015; Singh et al., 2018; Whittaker et al., 2018; Jeon et al., 2020). These disorder-specific mechanisms may not be related to resilience. On the other hand, resilience research can focus on the general resilience mechanisms that promote generalization to protect individuals from different stress-induced disorders by steering away from clinical patients. For example, excluding the clinical group, results only from comparing the high resilience group with the random control group would be able to provide general resilience mechanisms. In fact, a few local scholars had targeted the non-clinical population only when conducting resilience research (Kong et al., 2015; Shao et al., 2018). And there is an increasing trend to target non-clinical individuals in more recent resilience research. To access the resilience levels among non-clinical individuals, there were methodological challenges in examining resilience among non-clinical individuals.
1.2. Operational definitions of resilience
In resilience research, the literature adopted a number of different methodologies to operationally define resilience (van Harmelen et al., 2017; Veer et al., 2021). To access the group of non-clinical individuals, a battery of psychometric scales has been developed to assess resilience. Different self-reported questionnaires were adapted to measure the level of resilience across studies.
Windle et al. (2011) reviewed various resilience measurements. For instance, one of the most commonly used was the scale developed by Connor and Davidson: Connor Davison resilience scale (CD-RISC) (Connor and Davidson, 2003; Windle et al., 2011). The CD-RISC can assess the overall perceived resilience of oneself (Connor and Davidson, 2003). This measure scored the highest rating overall, including reliability and validity, among nineteen other resilience measures [for more details, please refer to Windle et al. (2011)]. It was well-adopted and used in the field of resilience research. One limitation of this measure is the ambiguity of the conceptual difference between CD-RISC and coping.
In addition to the CD-RISC, the State and Trait Resilience Inventory (Hiew et al., 2000), the Resilience Scale for Adults (Friborg et al., 2003), and the Brief Resilience Scale (Smith et al., 2008) are also commonly used for assessing resilience across studies. The State and Trait Resilience Inventory captured both state resilience and trait resilience separately (Hiew et al., 2000). The population targeted during the development of this measure was university students (Hiew et al., 2000). However, the conceptualization of resilience had been shifted away from classifying as state or trait binarily (Kalisch et al., 2015, 2017), which limited the generalizability of the result from this scale. The Resilience Scale for Adults and the Brief Resilience Scale were the other two out of three recommended measures after reviewing nineteen other measures on resilience besides the CD-RISC (Windle et al., 2011). Both measures scored high in reliability and validity ratings (Windle et al., 2011). The Resilience Scale for Adults assessed resilience on multiple levels (Friborg et al., 2003). This measure was ideal with the current trend of conceptualized resilience as an active dynamic adaptation to adversity (Kalisch et al., 2015, 2017). However, this measure was limited to adults only, which lacked the potential for early identification, as it cannot be generalized and measured in a young age group (Windle et al., 2011). The Brief Resilience Scale examined resilience as an outcome, meaning the ability to recover from stress (Windle et al., 2011). Most of the items from this scale focused on individual levels, while neglecting the level of family and community (Windle et al., 2011). Nevertheless, this measure was limited to access the process of achieving the outcome, including individual resources and assets.
Although these subjective measures were well-developed and well-validated, they could not avoid the subjective bias and individual differences in terms of stressors (traumas). Thus, the outcome-based measures were adopted in more recent literature. The score in this measure was calculated by the ratio of self-reported general health conditions to the perception of stress, which can minimize the individual differences in the types of stress exposures (Veer et al., 2021). With different operationalizations of resilience, each had its advantages and limitations. However, different operationally defined resilience can lead to varying interpretations of outcomes in resilience research. Given that no studies have compared these various operational definitions of resilience in the same subject, it remains unclear whether these operational definitions point to the same resilience outcome. Furthermore, subjective bias was inevitable using self-reported methods. One way to address this research question in a more objective way is to examine the common and distinct neural correlates across these various operational definitions of resilience.
1.3. Neural mechanism of resilience
Resilience can be viewed from a neurological perspective. Neuroimaging data can reduce subject frauds of psychological measurement and serve as compensatory tools to validate subjective measures. There were different neuroimaging tools accessible to researchers, including electroencephalography (EEG), Magnetic Resonance Imaging (MRI), functional MRI (fMRI), and functional near-infrared spectroscopy (fNIRS). EEG utilizes electrodes to measure the electrical activity of the brain (Noachtar and Rémi, 2009). Early literature found that resilient functioning was related to greater left frontal EEG activity, and greater left hemisphere EEG activity in non-maltreated children compared to maltreated children (Curtis and Cicchetti, 2007). Recent literature also revealed the relationship between negative emotions and resilience utilizing EEG data (Chen D. et al., 2018). The main drawback of EEG in neuroimaging research was the low spatial resolution, meaning the signal received from the electrodes failed to pinpoint the exact location of the activity that occurred (Noachtar and Rémi, 2009). Another one of the most used and reliable tools in this field of research is MRI, a non-radioactive and non-invasive technology that can provide clear three-dimensional anatomical images (Katti et al., 2011). MRI is mainly used for structural data, measuring the volume or size of specific brain regions (Mills et al., 2017). However, when examining the neural mechanisms of resilience, functions of different brain regions can provide more information than the structural matter. Functional connectivity and brain activation data were often the interest of resilience research by adopting the fMRI method to analyze the time series of voxel changes in the Blood-Oxygen-Level Dependent (BOLD) signals. BOLD signals allowed researchers to understand the brain functioning at a given situation or time-point when incorporated with other behavioral testing. For example, Dennison et al. (2016) found that a greater BOLD signal in the left pallidum was associated with lower depressive symptoms in maltreated youth. This study accessed behavioral data on depression symptoms and incorporated it with BOLD signals to provide said results (Dennison et al., 2016). Like fMRI, fNIRS also indirectly measures brain function by the concentration change of oxygenated and deoxygenated hemoglobin (Mehta and Parasuraman, 2013). Compared with fMRI, low spatial resolution and penetration depths are the most significant drawbacks for fNIRS, similar to EEG. Due to the purpose of this review, specific locations of functions are essential to understanding the underlying logic of resilience from a neurological perspective. Thus, this review will focus on studies of resilience using fMRI.
With the natural built-in mechanism of human beings, resilience is proposed to be quantified via neuroimaging, resulting in the development of neural features for resilience. In neuroimaging studies on resilience, different perspectives and factors were examined, like psychological and socio-environmental factors. For psychological factors of resilience, a study indicated a circuity of subgenual anterior cingulate (sgACC) to insula as a neural correlate to resilience (Shao et al., 2018). This study provided evidence that group differences were presented in the change in resting-state functional connectivity between sgACC and insula between high resilience and low resilience group (Shao et al., 2018). These regions were associated with experience and emotional regulation (van der Werff et al., 2013b), which are important resilience factors. For socio-environmental factors, recent literature has targeted social background (e.g., poverty) to be associated with resilience (Holz et al., 2020). An fMRI study found that childhood poverty was associated with less dorsolateral prefrontal cortex (dlPFC) activities during emotion regulation in adulthood (Kim et al., 2013). This area requires a prolonged maturation period and is mainly responsible for executive functioning, planning, and regulation. Also, it is one of the key areas that can predict resilience (Moreno-López et al., 2020). The studies mentioned above yielded different neurological outcomes based on the resilience factors that were being focused on and highlighted the importance of identifying the underlying neurological mechanisms for resilience. There is an existing neural model for vulnerability and resilience (Homberg and Jagiellowicz, 2022). The author of this model suggested that differential susceptibility: genes, protective factors or traits that can affect individuals to pay more attention toward positive environmental stimuli or a negative one, is linked to brain functioning; thus, become more resilient or vulnerable (Slagt et al., 2019; Homberg and Jagiellowicz, 2022). This model suggested that increased salience network (SN) activity, increased SN and default mode network (DMN) connectivity, and increased SN and central executive network (CEN) connectivity are related to attention shifting and cognitive flexibility (Homberg and Jagiellowicz, 2022). The current review aims to examine the underlying neural mechanisms of resilience in high resilient individuals. Additional information can be provided on this existing neural model of vulnerability and resilience.
1.4. Advantage of resting-state fMRI for resilience research
When utilizing fMRI, data can be collected through task-based or resting-state designs. In task-based designs, individuals are typically asked to complete a task, and the changes in neural activation are examined. A recent review was performed by Eaton et al. (2022) on different neuroimaging research on resilience among young people. They included eight studies that used a task-based approach (Heitzeg et al., 2008; Hanson et al., 2015; Dennison et al., 2016; Luking et al., 2018; Callaghan et al., 2019; Rodman et al., 2019; Maciejewski et al., 2020; Wymbs et al., 2020). Three of them used implicit emotion processing tasks (Heitzeg et al., 2008; Dennison et al., 2016; Wymbs et al., 2020), while others used tasks including reward processing (Hanson et al., 2015; Luking et al., 2018) and interference tasks (inhibition) (Callaghan et al., 2019; Maciejewski et al., 2020). In Eaton et al. (2022) review, they summarized the eight included task-based studies that high resilient young people are suggested to have lower amygdala responses to negative stimuli, tighter coupling of a prefrontal cortex (PFC)-amygdala circuit (Heitzeg et al., 2008; Rodman et al., 2019), and greater or normal ventral striatal activation toward positive or rewarding stimuli (Hanson et al., 2015; Luking et al., 2018). The results from amygdala and PFC-amygdala circuit were yield from studies that applied emotion tasks (Heitzeg et al., 2008; Rodman et al., 2019); whereas results from ventral striatum were from studies that applied reward processing tasks (Heitzeg et al., 2008; Rodman et al., 2019). Inconsistent tasks limited the interpretation of the neurological results relating to resilience. However, it is unclear whether these neurological results were directly related to the process of resilience or only the emotional regulation component of resilience. Moreover, there are concerns of inconsistent task difficulties among different research and variabilities in individual ability and performance (Constable, 2006). An alternative is using resting-state design that is task-free and only requires participants not to think of anything particular during the scan.
Data from resting-state fMRI is more suitable for capturing neural resilience mechanisms from the perspective of neural functions at rest, meaning trying to relax and not think of anything during the scan. Although intrinsic activity (e.g., mind wandering) is a disadvantage of utilizing data from resting-state fMRI studies (Raichle and Snyder, 2007; Finn, 2021), there is not enough existing literatures that have adopted naturistic paradigm nor a combination of both task and rest. When compared task-based fMRI to resting-state fMRI approaches, there are more concerning limitations regarding task-based fMRI approach in the field of resilience as pointed out. Past literature has shown a good utilization of resting-state fMRI in measuring brain activities and connections associated with resilience. A few studies have shown associations of resting-state connectivity of different areas and networks to resilience in PTSD patients (Rabinak et al., 2011; Yin et al., 2011). Those areas included the posterior cingulate cortex (PCC)/precuneus region and thalamus (Rabinak et al., 2011; Yin et al., 2011). Also, positive functional connections were found between the thalamus to the right medial frontal gyrus and the thalamus to the left rostral anterior cingulate cortex (ACC) (Rabinak et al., 2011; Yin et al., 2011). These areas are generally involved in emotional regulation, inhibition, and higher executive functioning. Moreover, resting-state fMRI can capture the baseline differences in neural activities and connectivity at rest without the effect of other stimuli and conditions. This is essential for identifying the potential neural markers for resilience.
It’s noteworthy to mention that there are other neurological factors that would affect resilience-dependent change in neural activities captured in resting-state fMRI, i.e., neuroendocrine and monoamines (Russo et al., 2012; Watanabe and Takeda, 2022). Activation of the hypothalamic-pituitary-adrenal (HPA) axis, one of the well-studied neuroendocrine systems, causes a widespread of hormonal and neurochemical changes, which was found to be affecting resilience (Russo et al., 2012; Lau et al., 2021). For instance, hormones like cortisol and dehydroepiandrosterone (DHEA) are released from the adrenal cortex in response to stress (Russo et al., 2012; Lau et al., 2021). The ability to restore the ratio of these two hormones back to normal after a stressful event was found to be affecting resilience (Lau et al., 2021). Moreover, a recent review had indicated that monoamines such as dopamine, serotonin, and noradrenaline were involved in resilience (Watanabe and Takeda, 2022). These monoamines were found to be affecting neural activities in brain regions that are highly associated with resilience, such as ventromedial prefrontal cortex (vmPFC), ACC, PCC, and medial prefrontal cortex (mPFC) (Kim et al., 2013; Shao et al., 2018; Eaton et al., 2022; Watanabe and Takeda, 2022). These findings provide a possible linkage in the psycho-neuro-endocrinological explanation of resilience.
In a short summary of the above neuroimaging studies, there is a research gap in exploring the underlying neural mechanisms of different operationally defined resilience. With only limited studies assessing the neural correlates of resilience among mentally healthy individuals, the protective mechanisms of resilience are still largely unknown (Waugh et al., 2008; New et al., 2009; Daniels et al., 2012; Reynaud et al., 2013; van Rooij et al., 2016; Iadipaolo et al., 2018). In addition, more objective neural markers are still needed for identifying resilience to help with prevention and intervention and developing a good model to predict individuals who may be at-risk.
1.5. Aims of the current study
To the best of my knowledge, there is no systematic review summarized the findings from resting-state fMRI studies from different operationally defined resilience in a mentally healthy population in the field. The most recent review by Eaton et al. (2022) examined a similar topic but only focused on youth only. Moreover, they included studies targeting protective factors and wellbeings (Eaton et al., 2022). Therefore, some of the studies they included did not have a targeting group considered as resilient. The objective of the current review is to examine the different operationally defined resilience across studies from a neurological perspective. Findings from the neural mechanisms provide insights into resilience’s underlying/core concept. The review results can contribute to the current field of studies exploring resilience by conceptualizing resilience from the neurological perspective while identifying the similarities and the differences between different operational definitions of resilience.
2. Methods
2.1. Databases and search terms
Studies were identified using the PubMed and MEDLINE databases. The following search terms were used in both databases: (Neuroimaging OR fMRI) AND (resting OR resting-state OR default mode network OR DMN OR intrinsic brain activity OR spontaneous brain activity) AND resilience. Only human research was included in this review. The search was completed on 31st December 2021. A total of 19 articles were included in this review based on a list of inclusion and exclusion criteria.
2.2. Study selection
A list of inclusion and exclusion criteria was predetermined prior to searching for eligible articles. The inclusion criteria included: (1) original peer-reviewed research; (2) having a group of participants was considered high resilience (not the healthy control group) or operationally defined resilience, i.e., quantified resilience by a resilience questionnaire score; and (3) using resting-state fMRI. In addition, any ineligible article type (i.e., review articles, conference proceedings, editorial, commentary, perspective, book chapter, book review, and dissertation), and any non-English articles were excluded. Moreover, articles that only targeted clinical participants were also excluded. See Figure 1 for the Preferred Reporting Items for Systematic Reviews and Meta-Analyses (PRISMA) flow of study selection (Page et al., 2021).
2.3. Search strategy
The initial search hits were recorded (N = 706) and sent to the reference manager (EndNote), where 530 duplicates were removed (N = 706–530 = 176). Then, two authors completed the abstract and full-text screening individually based on the predetermined inclusion and exclusion criteria. They cross-checked the results after both had identified the final numbers of included articles, resulting in a total of 19 articles included for this review.
2.4. Data extraction and replicability assessments
The 19 papers included in this review were organized and scrutinized, with a replicability assessment performed on the quality of the imaging data. Extracted data included (1) basic demographic of the participants (i.e., age and gender, etc.); (2) operational definitions of resilience (how did the article measure resilience); (3) fMRI scanning details (i.e., scan duration, eye-open/eye-close condition, etc.); (4) pre-processing steps of imaging data (i.e., motion correction and control, etc.); (5) modality used for imaging analyses (local activation or long-range functional connectivity); (6) regions-of-interests (ROIs; if any); (7) main results and resultant brain regions; and (8) control variables. Table 1 lists some of the extracted data for each of the articles. Replicability assessment was conducted based on the pre-processing steps taken for the imaging data and the scanning conditions. It is crucial to consider these aspects in neuroimaging analyses as different processing steps and different scanning conditions may yield different results. Each processing step and better scanning conditions will grant one point to the study, and the sum of points is the research’s replicability. Table 2 lists all the criteria included in the replicability assessment. Higher scores from this assessment suggested higher replicability of the research.
Included studies were divided into two groups based on the study designs: correlational studies and comparison studies. In the field of neuroimaging research on resilience, there are various types of operational definitions of resilience used based on the purposes of the study. A universally accepted operational definition of resilience is still lacking; therefore, a grouping strategy based on the operational definition of resilience for this review is not viable. To better organize and summarize all the findings from the included studies, grouping based on study design was adopted for this review. Only studies with correlational design (correlate resilience with neural mechanisms) would be included in the group of correlational studies. On the other hand, studies in comparison groups would compare results between an operationally defined high resilience group and random controls. Due to the various types of operational definitions of resilience, neural results from the included studies were scattered. A grouping based on the network systems of the reported neural regions was adopted in this review. The primary focus of the network systems would be on cognition and emotion, as these two domains were proven to be significantly related to resilience (Kalisch et al., 2015, 2019). After data extraction and replicability assessments, results from each included study were further summarized into different networks, including cognitive and emotional networks (Catalino et al., 2020).
2.5. Preliminary meta-analysis
For the included studies, activation likelihood estimation (ALE) meta-analysis was performed to examine the common neural features of resilience among healthy individuals. Limited number of studies were included, and the fMRI modalities in these included studies were diverse. Studies of each group would be further considered to the following inclusion criterion to be included for the preliminary meta-analysis. Studies were included only if four or more studies were adopting the same fMRI modalities: local or ROIs to ROIs, whole-brain or same a priori ROIs. Based on these criteria, only four studies (Fujisawa et al., 2015; Kong et al., 2015, 2018; Wang et al., 2020) that examined the local activation with a whole-brain approach were included. The coordinate-based ALE meta-analysis was conducted by GingerALE version 3.0.2 (The BrainMap Database1; San Antonio, TX, USA). The reported coordinates in MNI space were imported into the software. The ALE image was thresholded using uncorrected p < 0.001 and a cluster-level inference threshold of p < 0.05 with 5,000 permutations to better control for the false-positive rates.
3. Results
3.1. Overview
In this review, a total of 19 studies were included based on the inclusion and exclusion criteria. The included studies were published between 2013 and 2021. The age of the subjects included in the 19 studies ranged from 10.9 to 44 years. Two studies only recruited male participants (Kennis et al., 2015; Hemington et al., 2018). Two studies had exact gender matched across groups (van der Werff et al., 2013b; Shao et al., 2018). All studies with pre-selected region-of-interest (ROI) are summarized in Table 3. The average score of the replicability assessment is 4.42 (SD = 1.84, total score = 10). Only one paper scored higher than 6 (score = 9) (Miyagi et al., 2020). Other papers scored lower than 6. Due to the lack of a universally accepted operational definition of resilience, included studies were divided into two groups based on the study designs: correlational studies and comparison studies. Studies with the same study design are reported together.
3.2. Correlational studies
Ten studies adopted a single group correlational design, in which scores of resilience measured by different scales were correlated with resting-state fMRI data. Within these ten studies, three studies explored the correlation between resilience and local activation of the brain (Kong et al., 2015; Wang et al., 2020); while five studies looked at the correlation between resilience and long-range functional connectivity (Kilpatrick et al., 2015; Uchida et al., 2015; Santarnecchi et al., 2018; Shi et al., 2019, 2021; Miyagi et al., 2020). One study explored both local activation and long-range functional connectivity (Fujisawa et al., 2015). The average score of the replicability assessment among the studies in the group was similar to the overall average (M = 4.3. SD = 2.11). Miyagi et al. (2020) conducted the highest scored study, which focused on the correlation between long-range functional connectivity and resilience. The associations of resilience with local brain activation and long-range functional connectivity among the ten included studies in this group were summarized below.
3.2.1. Local activation
All of the four studies which explored the correlation between resilience and local activation of the brain adopted a whole-brain approach (Table 4; Figure 2). Each of these four studies utilized a different MRI modality, including amplitude of low-frequency fluctuation (ALFF) (Wang et al., 2020), fractional ALFF (fALFF) (Kong et al., 2018), group spatial independent component analysis (gICA) (Fujisawa et al., 2015), and regional homogeneity (Reho) (Kong et al., 2015). Three out of four studies used the CD-RISC in terms of the operational definition of resilience (Kong et al., 2015, 2018; Wang et al., 2020). The remaining study adopted the Posttraumatic Growth Inventory (PTGI) score for measuring resilience (Fujisawa et al., 2015). Two studies found the activation of the orbitofrontal cortex (OFC) was correlated with resilience measured by the CD-RISC (Kong et al., 2018; Wang et al., 2020). This cortical area is included in the emotional network (EN) (Catalino et al., 2020) that is responsible for emotional processing. Fujisawa et al. (2015) found that resilience was positively correlated with local activation in the left rostral prefrontal cortex and left superior parietal lobule (SPL). These two areas were included in the DMN and CEN, respectively (Catalino et al., 2020). Last but not least, the brain areas found in Kong et al. (2015) were all included in SN but in an opposite direction of the correlation when compared with the findings from Fujisawa et al. (2015). Kong et al. (2015) found that the local activation of right dorsal ACC (dACC), right rostral ACC (rACC), and bilateral insula were negatively correlated with resilience. The preliminary coordinate-based ALE meta-analysis was conducted with these four studies. No common clusters were found.
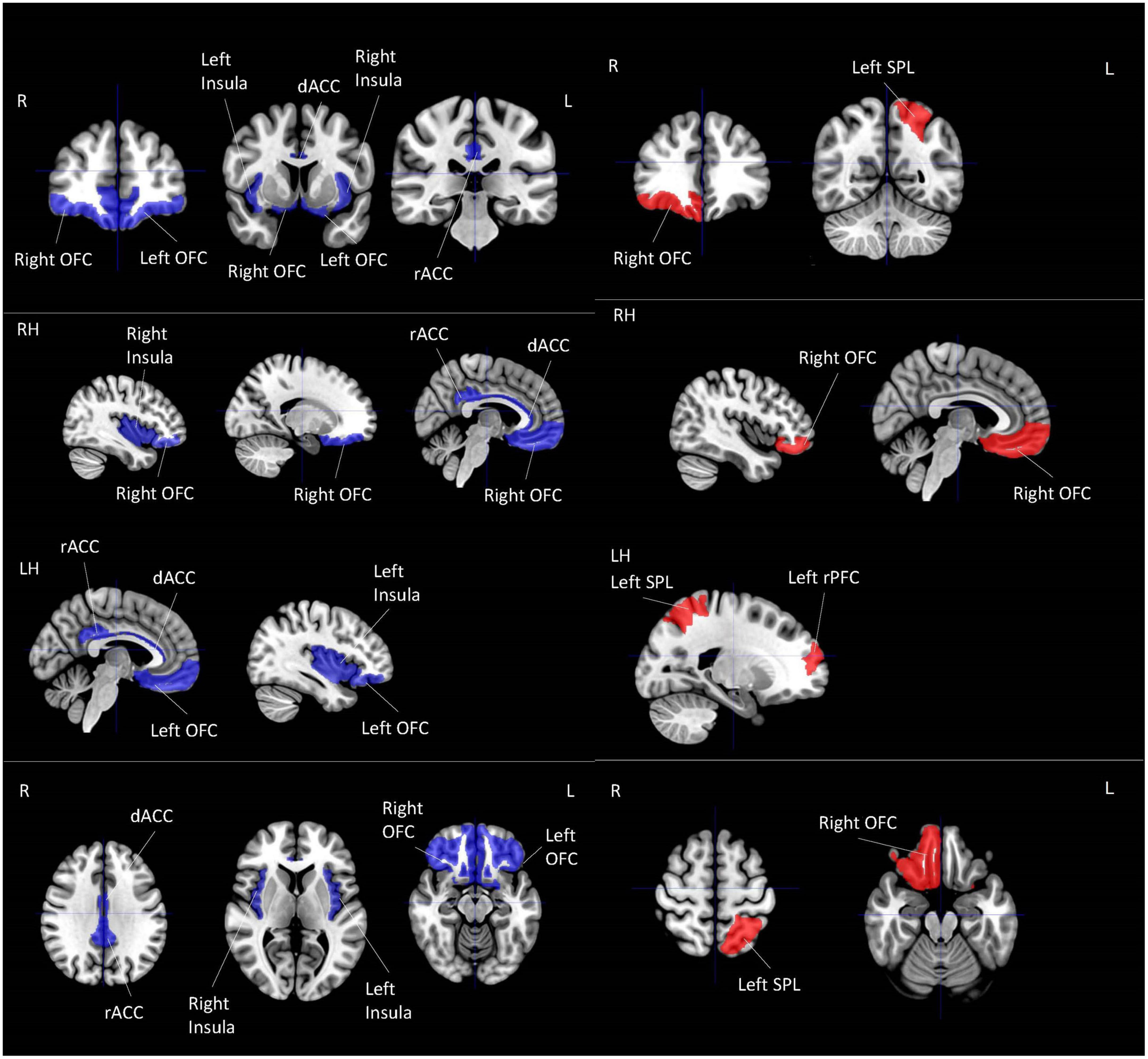
Figure 2. Local activation for correlational studies. Red represents positive correlation with resilience. Blue represents negative correlation with resilience. All highlighted regions showed in the figure are only used for approximate representation of the reported regions. It does not represent exact coordinates nor clusters of the reported results. R, Right; L, Left; RH, right hemisphere; LH, left hemisphere; OFC, orbitofrontal cortex; SPL, superior parietal lobule; PFC, prefrontal cortex; dACC, dorsal anterior cingulate cortex; rACC, rostral anterior cingulate cortex.
3.2.2. Long-range functional connectivity
Including Fujisawa et al. (2015) study, seven studies examined the long-range functional connectivity and its correlation with resilience (Table 5; Figure 3). A study examined functional connectivity while weights in independent component analysis (ICA) value and conducted a partial least squares (PLS) multivariate analysis (Kilpatrick et al., 2015). Other studies adopted different modalities, including ROI-to-ROI functional connectivity (Miyagi et al., 2020; Shi et al., 2021), ROIs-based functional connectivity (Shi et al., 2019), voxel-wise connectivity analysis (Fujisawa et al., 2015; Santarnecchi et al., 2018), and seed-to-voxel correlations (Uchida et al., 2015). All seven studies adopted different measures of operationally defined resilience, including PTGI scores (Fujisawa et al., 2015), CD-RISC (Miyagi et al., 2020), 25-items resilience questionnaire (Shi et al., 2019, 2021), coping orientation to the problems experienced (Santarnecchi et al., 2018), resilience personality scores (Kilpatrick et al., 2015), and scores from the reappraisal task (Uchida et al., 2015).
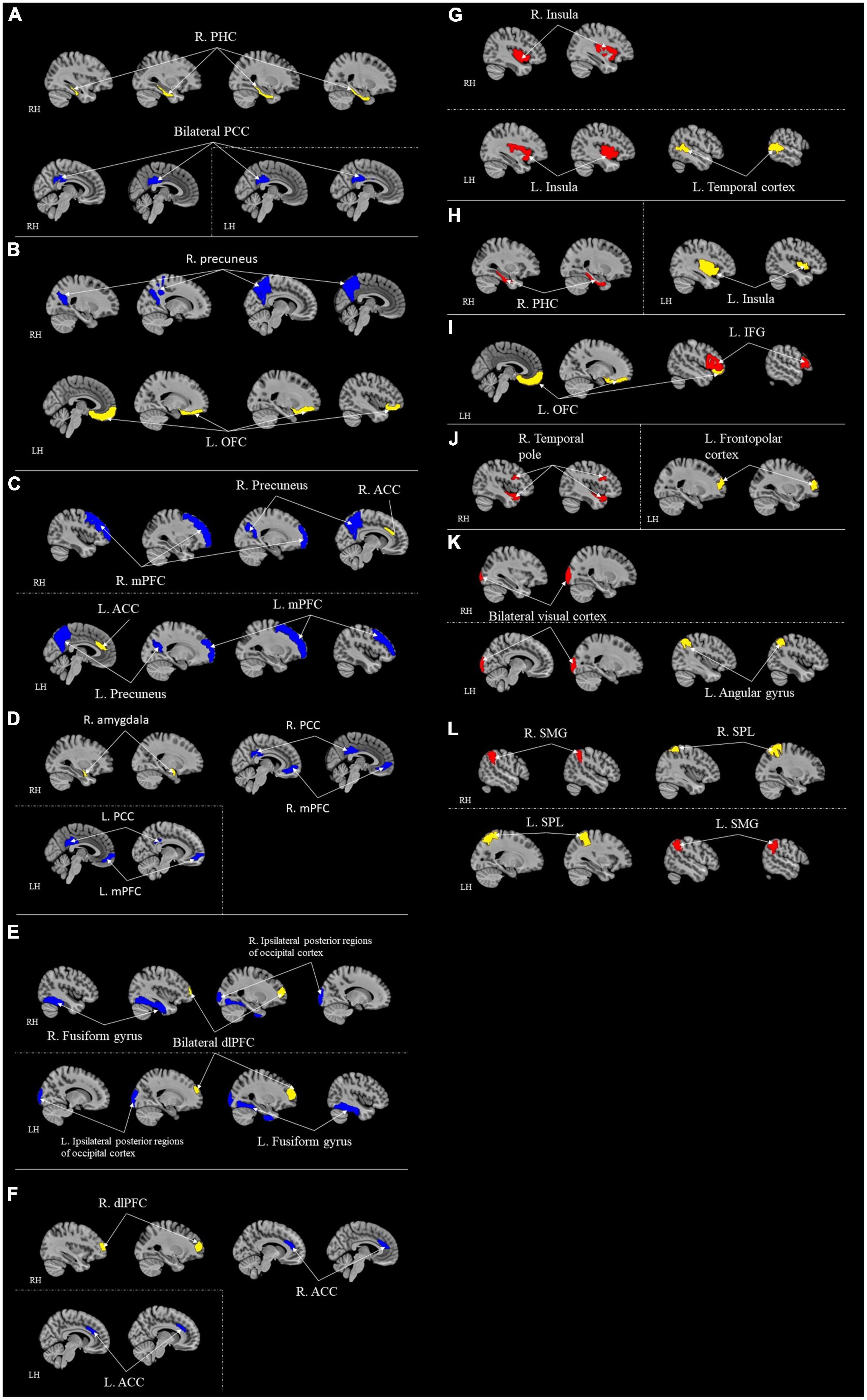
Figure 3. ROI-to-ROI functional connectivity for correlational studies. Red represents positive correlation with resilience. Blue represents negative correlation with resilience. Yellow represents the seed regions. Each sub-section represents connectivity from a same seed: (A) R. PHC (Miyagi et al., 2020); (B) L. OFC (Shi et al., 2019); (C) Bilateral ACC (Santarnecchi et al., 2018); (D) R. Amygdala (Uchida et al., 2015); (E) Bilateral dlPFC (Uchida et al., 2015); (F) R. dlPFC (Uchida et al., 2015); (G) Temporal cortex (Shi et al., 2021); (H) L. Insula (Shi et al., 2019); (I) L. OFC (Shi et al., 2019); (J) L. Frontopolar cortex (Santarnecchi et al., 2018); (K) Angular gyrus (Santarnecchi et al., 2018); (L) Bilateral SPL (Santarnecchi et al., 2018). Kilpatrick et al. (2015) contained too many regions that it is difficult and complicated to represent in figures; thus, it is excluded from this figure. All highlighted regions showed in the figure are only used for approximate representation of the reported regions. It does not represent exact coordinates nor clusters of the reported results. R, Right; L, Left; RH, right hemisphere; LH, left hemisphere; PHC, parahippocampal cortex; RSC, retrosplenial cortex; PCC, posterior cingulate cortex; IFG, inferior frontal gyrus; ACC, anterior cingulate cortex; OFC, orbitofrontal cortex; mPFC, medial prefrontal cortex; dlPFC, dorsolateral prefrontal cortex; PHG, parahippocampus gyrus; SMG, supramarginal gyrus; SPL, superior parietal lobule.
Among these seven studies, only three adopted a whole-brain approach (Fujisawa et al., 2015; Miyagi et al., 2020; Shi et al., 2021). Other four studies chose different a priori ROIs for their analyses (Kilpatrick et al., 2015; Uchida et al., 2015; Santarnecchi et al., 2018; Miyagi et al., 2020) (refer to Table 5 for ROIs details). Miyagi et al. (2020) took seven different a priori ROIs. Santarnecchi et al. (2018) chose three regions for a priori ROIs. Eight a priori ROIs were adopted in Uchida et al. (2015) study. Kilpatrick et al. (2015) predetermined 20 different regions as their a priori ROIs. The ACC and mPFC are the two of the most common regions that more than one study chose to be a priori ROIs.
Following were the specific seed to target functional connectivity findings from each included study in this group. Shi et al. (2021) found that the functional connectivity between temporal cortex and insula was positively correlated to resilience. Fujisawa et al. (2015) found that functional connectivity from superior parietal lobule to supramarginal gyrus was positively correlated with resilience. Uchida et al. (2015) found the functional connectivity from the right amygdala seed to the mPFC and the PCC, from bilateral dlPFC to ipsilateral posterior regions of occipital cortex and fusiform gyrus, and from right dlPFC to ACC were negatively correlated with resilience. Shi et al. (2019) found the function connectivity from the left insula to the right parahippocampus gyrus (PHG), and from the left OFC and the left inferior frontal gyrus (IFG) were positively correlated with resilience. This study also found that functional connectivity from the left OFC to the right precuneus was negatively correlated with resilience (Shi et al., 2019). Santarnecchi et al. (2018) found the connectivity between the left frontopolar cortex and right temporal pole and left angular gyrus and visual cortex bilaterally (occipital pole) were positively correlated with resilience. A negative correlation was also found in this study between resilience and the connectivity between ACC and medial prefrontal and precuneus cortices bilaterally (Santarnecchi et al., 2018). Kilpatrick et al. (2015) found that the functional connectivity between regions in the DMN and the SN were positively correlated with resilience.
After summarizing the results into network levels, two studies found resultant areas included in the CEN (Fujisawa et al., 2015; Uchida et al., 2015). Five studies found resulting regions included in DMN (Kilpatrick et al., 2015; Uchida et al., 2015; Santarnecchi et al., 2018; Miyagi et al., 2020; Shi et al., 2021). Four other studies found resulting regions included in SN (Uchida et al., 2015; Santarnecchi et al., 2018; Shi et al., 2019, 2021). Attention network (AN) was also involved in two studies (Uchida et al., 2015; Santarnecchi et al., 2018). Three studies found resultant regions included in the memory network (MN) (Santarnecchi et al., 2018; Shi et al., 2019; Miyagi et al., 2020). Two studies found resultant regions included in the EN (Uchida et al., 2015; Shi et al., 2019). MN included regions like hippocampal formations, the cingulate cortex, and the angular gyrus responsible for recognition memory functions, encoding, and vision-related memory processing (Catalino et al., 2020). Notably, CEN is involved in the most connectivity compared to other networks (five pathways).
3.3. Comparison studies
The rest of the nine studies were comparison studies (Table 6; Figures 4, 5). None of them investigated the local activation of different brain regions. Thus, the long-range functional connectivity was the focus of these nine studies. The average score of the replicability assessment among these nine studies was also close to the overall average (M = 4.45, SD = 1.59). The lowest scored research is in this group (score = 1) (Hirshfeld-Becker et al., 2019), indicating an incomplete report of the pre-processing steps for the neuroimaging data. All nine studies adopted the ROI-to-ROI approach for data analysis.
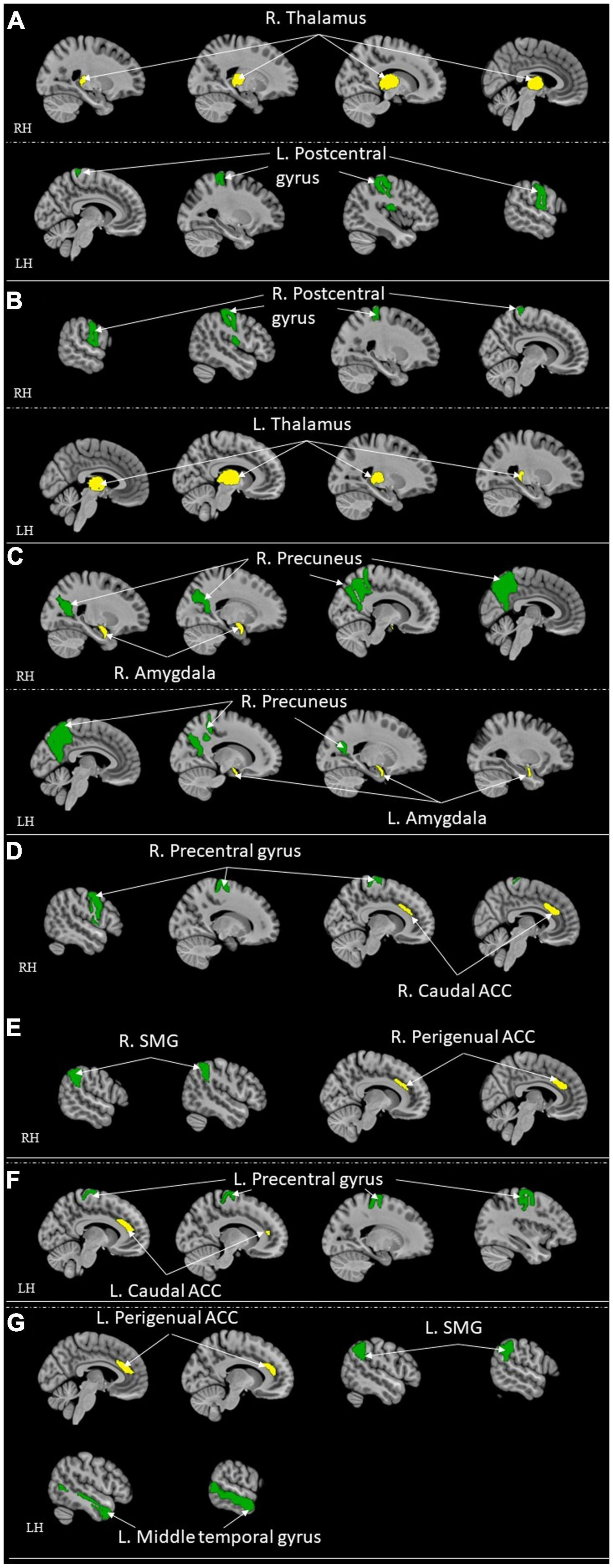
Figure 4. ROI-to-ROI functional connectivity for comparison studies: healthy control > high resilient individuals. Green represents the target regions. Yellow represents the seed regions. Each sub-section represents connectivity from a same seed: (A) R. Thalamus (Jeon et al., 2020); (B) L. Thalamus (Jeon et al., 2020); (C) Bilateral Amygdala (Singh et al., 2018); (D) R. Caudal ACC (Kennis et al., 2015); (E) R. Perigenual ACC (Kennis et al., 2015); (F) L. Caudal ACC (Kennis et al., 2015); (G) L. Perigenual ACC (Kennis et al., 2015). All highlighted regions showed in the figure are only used for approximate representation of the reported regions. It does not represent exact coordinates nor clusters of the reported results. R, Right; L, Left; RH, right hemisphere; LH, left hemisphere; ACC, anterior cingulate cortex; SMG, supramarginal gyrus.
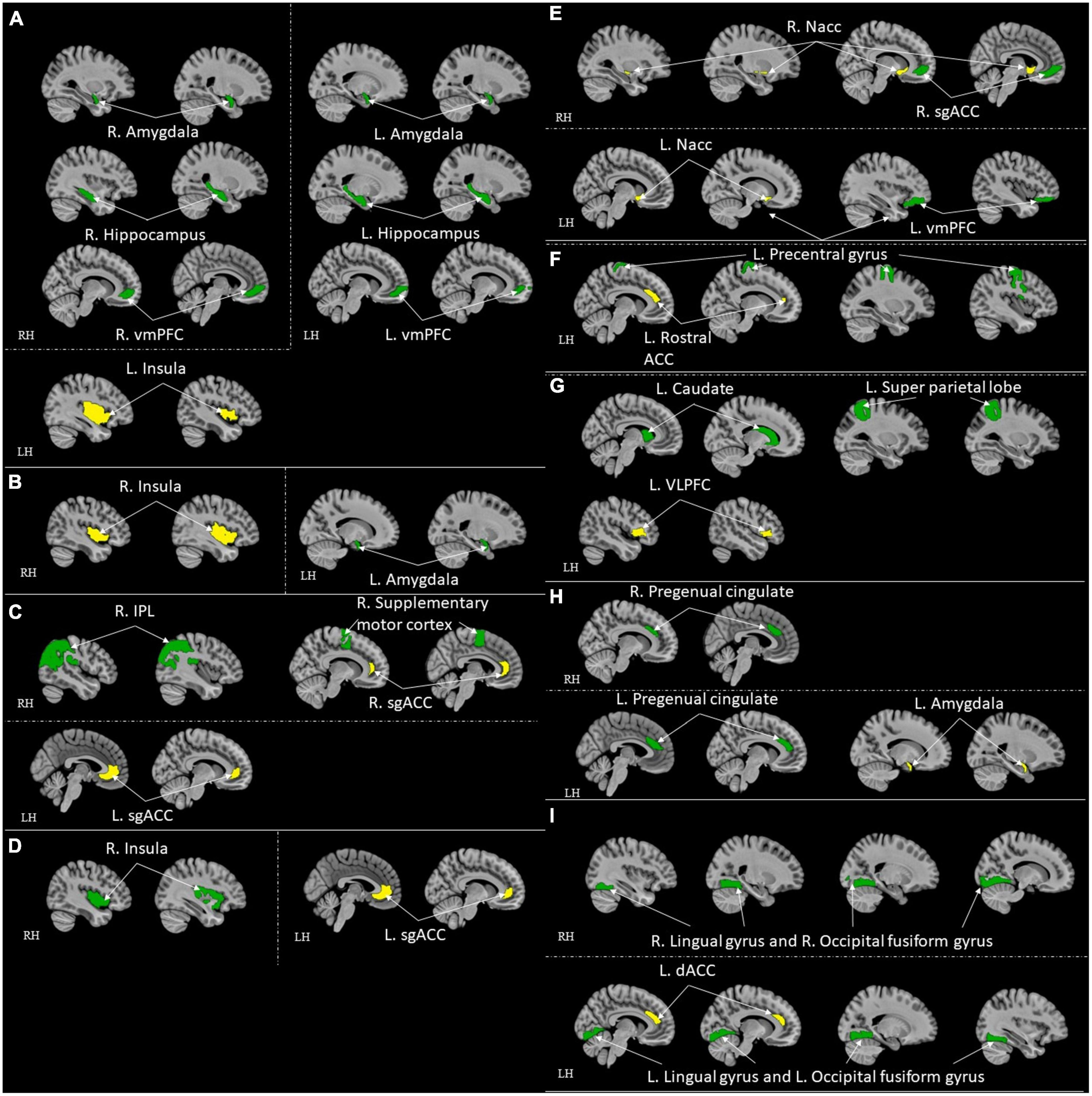
Figure 5. ROI-to-ROI functional connectivity for comparison studies: high resilient individuals > healthy control. Green represents the target regions. Yellow represents the seed regions. Each sub-section represents connectivity from a same seed: (A) L. Insula (Jeong et al., 2019); (B) R. Insula (Jeong et al., 2019); (C) Bilateral sgACC (Singh et al., 2014; Hirshfeld-Becker et al., 2019); (D) L. sgACC (Shao et al., 2018); (E) R. Nacc (Whittaker et al., 2018); (F) L. Rostral ACC (Kennis et al., 2015); (G) L. VLPFC (Singh et al., 2014); (H) L. Amygdala (Singh et al., 2014); (I) L. dACC (van der Werff et al., 2013b). All highlighted regions showed in the figure are only used for approximate representation of the reported regions. It does not represent exact coordinates nor clusters of the reported results. R, Right; L, Left; RH, right hemisphere; LH, left hemisphere; vmPFC, ventromedial prefrontal cortex; sgACC, subgenual anterior cingulate; IPL, inferior parietal lobule; ACC, anterior cingulate cortex; VLPFC, ventrolateral prefrontal cortex; dACC, dorsal ACC.
Different a priori ROIs were investigated among these nine studies (refer to Table 6 for ROIs details). Jeon et al. (2020) had bilateral thalamus as the two a priori ROIs. Jeong et al. (2019) chose seven a priori ROIs for their study. Hirshfeld-Becker et al. (2019) adopted six a priori ROIs. Shao et al. (2018) chose bilateral sgACC as two a priori ROIs. Four a priori ROIs were selected in Singh et al. (2018) study. Whittaker et al. (2018) took bilateral Nacc as two a priori ROIs. Kennis et al. (2015) had five bilateral a priori ROIs. Singh et al. (2018) adopted nine a priori ROIs. van der Werff et al. (2013b) took six a priori ROIs. The ACC, PCC, mPFC, and amygdala were the most used a priori ROIs across these nine studies.
The operational definitions of the high resilience group were different among all nine studies. One study used the score from a subjective scale, CD-RISC (Shao et al., 2018). Another study used high-stress occupation as an indicator (firefighter) (Jeong et al., 2019). The other seven studies in this group chose at-risk individuals, who are closely related to the clinical patient, without ever diagnosed any axis-1 disorders as an indicator of high resilience, and compared with normal random controls (van der Werff et al., 2013b; Singh et al., 2014, 2018; Kennis et al., 2015; Whittaker et al., 2018; Hirshfeld-Becker et al., 2019; Jeon et al., 2020).
Modalities were also different among these nine studies. Four studies utilized correlation maps and Fishers z-transform with second-level group jeon analysis (Kennis et al., 2015; Shao et al., 2018; Jeong et al., 2019; Jeon et al., 2020). One study adopted gICA and dual regression procedures for analyses (Singh et al., 2014). Another study utilized ROIs-based functional connectivity (Singh et al., 2018). Hirshfeld-Becker et al. (2019) investigate the data with general linear modal analyses. Whittaker et al. (2018) explored the voxel-wise differences in a two-sample t-test. Inverse transformation matrices were adopted in the study by van der Werff et al. (2013b).
Following were the specific seed to sink functional connectivity findings from each included study in this group. Jeon et al. (2020) found that the control had higher functional connectivity between the right thalamus and left post-central gyrus and the left thalamus and right post-central gyrus when compared with the high resilience group. Jeong et al. (2019) found that the high resilience group had higher functional connectivity from the left insula to the bilateral amygdalae, to the bilateral hippocampi, and to the vmPFC, and from the right insula to the left amygdala when compared to the control. Hirshfeld-Becker et al. (2019) found that the high resilience group had higher functional connectivity between sgACC and right inferior parietal lobule (IPL)/precentral gyrus when compared to the control. Shao et al. (2018) found that the high resilience group had higher functional connectivity from the sgACC to the right insula when compared to the control. Singh et al. (2018) found that the high resilience group had functional connectivity from the amygdala and precuneus when compared to the control. Whittaker et al. (2018) found that high resilience group had higher functional connectivity between the Nacc and ventromedial prefrontal cortex (sgACC) when compared to the control. Kennis et al. (2015) found that control had higher functional connectivity from bilateral caudal ACC to bilateral precentral gyrus, from bilateral perigenual ACC to bilateral superior medial gyrus (SMG, and left perigenual ACC to left middle temporal gyrus when compared to high resilience group. They also found that high resilience had higher functional connectivity from the left rACC to the left precentral/middle frontal gyrus when compared to control (Kennis et al., 2015). Singh et al. (2014) found that the high resilience group had higher functional connectivity between the left ventrolateral prefrontal cortex (VLPFC) and the left superior parietal lobule, the left amygdala, the pregenual cingulate, sgACC and the right supplementary motor cortex, the left VLPFC and the left caudate when compared to control. van der Werff et al. (2013b) found that the high resilience group had more negative connectivity between the lingual gyrus and the occipital fusiform gyrus when compared with the control group.
Four studies found resultant regions involved in DMN (Kennis et al., 2015; Singh et al., 2018; Whittaker et al., 2018; Jeong et al., 2019). CEN is involved in the resultant regions found in four different studies (Singh et al., 2014; Kennis et al., 2015; Hirshfeld-Becker et al., 2019). Four studies found resultant regions included in SN (van der Werff et al., 2013b; Singh et al., 2014; Shao et al., 2018; Jeong et al., 2019). Only Jeong et al. (2019) had resultant regions involved in MN. Eight out of nine studies had resulting areas involved in EN (Singh et al., 2014, 2018; Kennis et al., 2015; Shao et al., 2018; Whittaker et al., 2018; Hirshfeld-Becker et al., 2019; Jeong et al., 2019; Jeon et al., 2020). Notably, SN is the network involved in most connectivity compared to other networks.
4. Discussion
To our knowledge, this is the first systematic review to summarize the results from resting-state fMRI research focusing on the non-psychiatric high resilience group. The summarized neural findings across studies that adopted various operational definitions of resilience can inform the underlying neurological mechanisms of the positive aspects of resilience.
Under the group of correlational studies, OFC was negatively correlated with resilience, and it was the only shared local activation findings from more than one study (Kong et al., 2018; Wang et al., 2020). For long-range functional connectivity, most of the regions in the reported connectivity pathways for both the correlational group and comparison group are within EN (Singh et al., 2014, 2018; Kennis et al., 2015; Uchida et al., 2015; Santarnecchi et al., 2018; Shao et al., 2018; Whittaker et al., 2018; Hirshfeld-Becker et al., 2019; Jeong et al., 2019; Shi et al., 2019; Jeon et al., 2020). EN included regions like OFC, amygdala, hypothalamus, and hippocampus, etc. (Catalino et al., 2020). Align with the literature, these regions are correlated with resilience (Simeon et al., 2007; Yu and Zhang, 2007; Russo et al., 2011; Leaver et al., 2018).
Among correlational studies, only four studies explore the local activation of the brain and its relation to resilience. Notably, the activation in OFC was negatively correlated with resilience in two studies (Kong et al., 2018; Wang et al., 2020). Increased activation of this region has been found to be related to a number of stress-induced disorders (Bing et al., 2013; Liu et al., 2014; Xu et al., 2014; Qiu et al., 2015). However, the opposite effect was found in males specifically (Wang et al., 2020). The differences in hormonal systems and brain development between sex might cause sex-specific results (Wang et al., 2020). Gender should be considered as a vital factor when studying neuroimaging research in the future. Moreover, the OFC is involved in encoding reward value and emotional regulation (Berridge and Kringelbach, 2013; Shiba et al., 2016; Figure 6); it is a part of EN (Catalino et al., 2020). This finding might reflect the poor ability of reward processing and emotional regulation among low resilience individuals. The OFC is found to be closely related to hope (Wang et al., 2017), life satisfaction (Kong et al., 2015), emotion regulation (Wager et al., 2008; Berridge and Kringelbach, 2013; Shiba et al., 2016) and resilience (Simeon et al., 2007; Yu and Zhang, 2007), in which hope, life satisfaction, and emotion regulation are highly associated with resilience. For instance, hope and resilience have been found to be strongly and significantly related to each other (Hidayat and Nurhayati, 2019; Myers et al., 2019). Hope was found to be positively correlated with resilience and a significant predictor for resilience (Hidayat and Nurhayati, 2019; Myers et al., 2019). When paired together, hope and resilience were significant predictors of quality of life and wellbeing (Kirmani et al., 2015; Li et al., 2016; Long et al., 2020). Besides, emotional regulation strategies were essential for promoting resilience and preventing stress (Tugade and Fredrickson, 2007; Thomas and Zolkoski, 2020). It has been reported that better resilience often leads to better life satisfaction (Samani et al., 2007; Abolghasemi and Taklavi Varaniyab, 2010; Moradi and Mirkohi, 2020). These findings indicate the functional role of OFC in resilience, and appropriate regulation of the functioning of OFC from regions like dlPFC (Golkar et al., 2012) could be a potential feature of high resilience. However, with limited studies in this part, this finding may need further validation in future research with large sample size and a more standard measure of resilience. From the limited results for local activation included in this review, there is no common region across different resilience measures. Both studies that found the correlation between OFC and resilience had CD-RISC as their operational definition of resilience (Kong et al., 2018; Wang et al., 2020). Other regions such as rPFC, SPL, ACC, and insula were sparsely reported across the included studies (Fujisawa et al., 2015; Kong et al., 2015). For instance, the left rostral prefrontal cortex and superior parietal lobule were found to associate positively with resilience (Fujisawa et al., 2015) these regions are mainly responsible for complex cognitive functioning, including memory, problem-solving, judgment, and perception (Burgess et al., 2007; Volle et al., 2011). In another study, the ACC and insula were also reported to negatively correlate with resilience (Kong et al., 2015). The ACC is mainly responsible for higher-level functions such as reward anticipation, attention allocation, and emotion (Pardo et al., 1990; Bush et al., 2002; Decety and Jackson, 2004; Figure 6). The insula is primarily responsible for emotions (Phan et al., 2002; Vilares et al., 2012; Figure 6). From previous literature, insula was found to be involved in risk perception and evaluation (Zhou et al., 2014; Lau et al., 2015). These perception and evaluation are one form of cognitive appraisal, which is one of the key factors that influencing resilience (Hooberman et al., 2010; Kalisch et al., 2015).
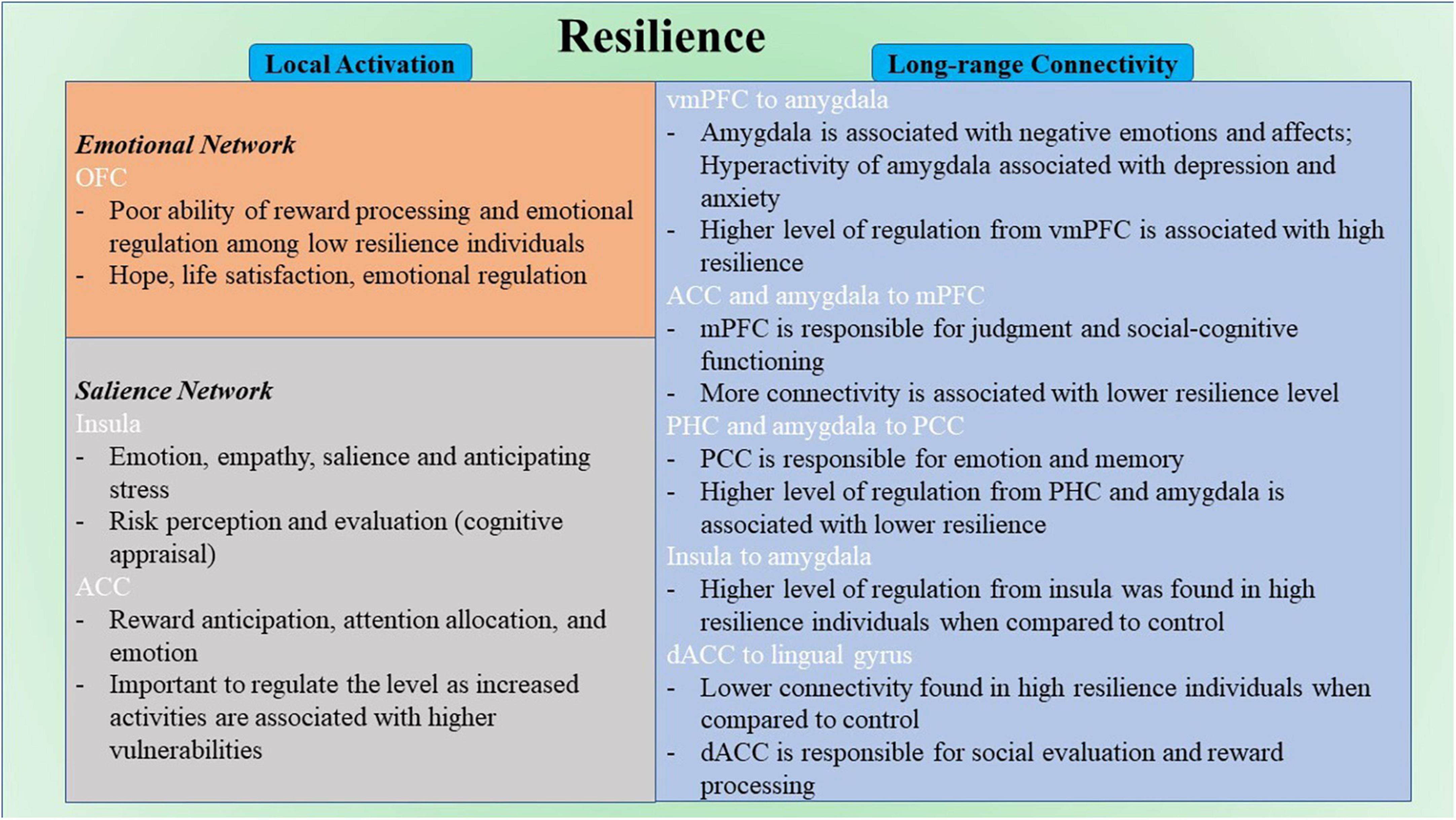
Figure 6. Schematic diagram for neural correlates of resilience. ACC, anterior cingulate cortex; dACC, dorsal anterior cingulate cortex; mPFC, medial prefrontal cortex; OFC, orbitofrontal cortex; PCC, posterior cingulate cortex; PHC, parahippocampal cortex; vmPFC, ventromedial prefrontal cortex.
The other seven studies in the correlational group explored the long-range functional connectivity and its relation to resilience. Four out of seven studies took a ROI-to-ROI approach. With differences among the a priori ROIs and MRI modality, there was no common pathway that was correlated with resilience between multiple studies. However, the regions in EN are the most involved in the reported connectivity pathways (Uchida et al., 2015; Santarnecchi et al., 2018; Shi et al., 2019). The brain regions include the amygdala and OFC. This result highlighted the importance of emotional regulation in resilience. The amygdala was found to be linked to negative emotions and affects (Murray, 2007; Figure 6). Hyperactivity of the amygdala was shown to be associated with depression and anxiety (Linden, 2006). The connectivity strength from left amygdala to left precuneus was found to be positively associated with depressive symptoms in individuals with subthreshold depression individuals (Peng et al., 2020). On the other hand, high resilience is associated with lower amygdala function (Leaver et al., 2018). A higher level of regulation of the functional activity of the amygdala from other brain regions like the vmPFC (Motzkin et al., 2015; Figure 6) would be one of the keys to staying resilient. In addition, regions in DMN were reported across most studies in this group (Kilpatrick et al., 2015; Uchida et al., 2015; Santarnecchi et al., 2018; Miyagi et al., 2020; Shi et al., 2021). The DMN is found to be active during rest and mind-wandering (Buckner et al., 2008; Andrews-Hanna, 2012). Although more studies reported regions that included the DMN, more pathways were reported that involved regions in EN. Regions like mPFC and PCC were included in this network. The pathways from ACC and right amygdala to mPFC were found to be negatively correlated to resilience (Uchida et al., 2015; Santarnecchi et al., 2018). The mPFC is mainly responsible for judgment and social-cognitive functioning (Mitchell et al., 2002; Figure 6). The pathways from the right parahippocampal cortex (PHC) and the right amygdala to PCC were found to be negatively correlated to resilience (Uchida et al., 2015; Miyagi et al., 2020). The PCC is mainly responsible for emotion and memory (Maddock et al., 2001, 2003; Figure 6). All seven included studies in this group used different resilience measures. However, no same pathways were reported from different studies in this group. There is a high possibility that different modality and different operational definitions of resilience target different neural resilience mechanisms, leading to various results across studies. Other regions such as PHC and angular gyrus were also reported in different pathways correlated with resilience. The pathway from the right PHC to the left retrosplenial cortex and PCC was found to be negatively correlated with resilience (Miyagi et al., 2020). However, the pathway from the left insula to the right PHG was found to be positively correlated with resilience (Shi et al., 2019). The PHG is responsible for scene recognition (Aguirre et al., 1998; Epstein and Kanwisher, 1998). The pathway between the left angular gyrus and the bilateral occipital pole was found to be positively correlated with resilience (Santarnecchi et al., 2018). The angular gyrus is mainly responsible for attention and memory retrieval (Park et al., 2008; Seghier, 2013).
Among comparison studies, a similar pattern was found in these nine studies compared with the seven studies in the correlational studies group that explored the long-range functional connectivity. Similarly, because all nine studies took a ROI-to-ROI approach, the connectivity results were mixed. However, seven out of nine studies found resultant pathways that included the amygdala and ACC (Singh et al., 2014; Kennis et al., 2015; Shao et al., 2018; Whittaker et al., 2018; Hirshfeld-Becker et al., 2019; Jeong et al., 2019). Eight out of nine studies had found brain regions involved in EN (Singh et al., 2014, 2018; Kennis et al., 2015; Shao et al., 2018; Whittaker et al., 2018; Hirshfeld-Becker et al., 2019; Jeong et al., 2019; Jeon et al., 2020). Among all the resultant pathways, 18 had regions involved in EN. Specifically, the ability to regulate emotions through SN–EN connectivity within the high resilience group was better compared with control. The pathways that involved regions in SN were also reported in four studies (van der Werff et al., 2013b; Singh et al., 2014; Shao et al., 2018; Jeong et al., 2019). The SN is found to be mainly involved in detecting changes in sensory stimuli (Downar et al., 2000), and modulating the switch between DMN and the central executive network (Menon, 2015). Regions reported in SN include insula and dACC. The connectivity from left insula to vmPFC, bilateral amygdalae and hippocampi, and right insula to left amygdala were found to be more in high resilience individuals compared to control (Jeong et al., 2019). The connectivity from the left sgACC to right insula was also found to be higher in high resilience individuals when compared with control (Shao et al., 2018). The insula is mainly responsible for empathy (Singer, 2006), emotions (Phan et al., 2002), and salience (Taylor et al., 2009; Menon and Uddin, 2010; Figure 6). The connectivity from left dACC to the bilateral lingual gyrus and the occipital fusiform gyrus was found to be lower among high resilience individuals compared with control (van der Werff et al., 2013b). The dACC is mainly responsible for social evaluation (Dedovic et al., 2016) and reward processing (Bush et al., 2002; Figure 6). Mostly reported regions within these pathways included the amygdala, the insula, and the ACC. Insula is involved in a number of complex functioning, including anticipation of stress (Simmons et al., 2012). Activation of this region were found to be among high resilient individuals when presented with aversive stimuli (Waugh et al., 2008). Align with the summarized result from the correlational group and previous literature, emotion regulation is highly associated with resilience (Karreman and Vingerhoets, 2012; Mestre et al., 2017; Polizzi and Lynn, 2021). The ACC is the most reported region that included studies in this group. Similar to the amygdala, increased activities of ACC are associated with higher vulnerabilities (Bolsinger et al., 2018). Therefore, it is also important to regulate the level of the functional activity of ACC to maintain a high resilience level. Similar to the studies in the correlational group, different operational definitions were used, and no common pathway was found among all included studies in this group. Other regions such as amygdala and ACC were also in individual studies (Singh et al., 2014, 2018; Kennis et al., 2015; Shao et al., 2018; Hirshfeld-Becker et al., 2019; Jeong et al., 2019).
Among all 19 studies, the CD-RISC was the most commonly used scale for defining resilience. A total of five studies used this scale to operationally define resilience (Kong et al., 2015; Shao et al., 2018; Miyagi et al., 2020; Wang et al., 2020). Two out of these five studies found a similar relationship between OFC and resilience (Kong et al., 2018; Wang et al., 2020). In two of these five studies, ACC was found to be included in the resultant regions that related to resilience (Kong et al., 2015; Shao et al., 2018). Both OFC and ACC are included in the EN, indicating the critical role of emotional regulation on resilience (Catalino et al., 2020). Although seven studies used the absence of psychopathologies as an indicator of resilience, the types of disorders vary (van der Werff et al., 2013b; Singh et al., 2014, 2018; Kennis et al., 2015; Whittaker et al., 2018; Hirshfeld-Becker et al., 2019; Jeon et al., 2020). This explained the result difference among these seven studies. The rest of the studies adopted different operational definitions of resilience and explained the various results summarized in this review. Even studies adopted same operational definitions of resilience, different neural feature were found in relation to resilience. The demographic of the participants and the methodological difference may cause the differences. When more studies examined the same operational definitions of resilience, a meta-analysis can be conducted for a better understanding of this difference.
The existing neural model for vulnerability and resilience (Homberg and Jagiellowicz, 2022) pointed the importance of attention shifting and cognitive flexibility. The connectivity between the PCC and the vmPFC was found to be associated with cognitive flexibility (Lau et al., 2020). Yet, this connection was not found in the included studies in this review, as for attention shifting.
The summarized results from this review align with the resting-state fMRI findings from other similar reviews in resilience and neurological research. Bolsinger et al. (2018) conducted similar review targeting adults who experienced traumatic events. Similar to the finding from this review, they also found the relationship between the amygdala, ACC, and resilience. Similar results of ACC were also indicated in the review by van der Werff et al. (2013b). They reviewed structural, resting-state, and task-based neuroimaging studies of resilience in adults. Holz et al. (2020) reviewed the relationship between environmental factors, neurological mechanisms, and resilience. They also found the involvement of the brain area in reward processing associated with resilience. Lastly, Eaton et al. (2022) conducted a review targeting the brain’s structure, function and connectivity, and resilience in youth. Notably, Eaton et al. (2022) targeted studies with a younger population only, which excluded most of the studies included in the current review. In the findings of fMRI studies in their review, areas involved in reward processing and emotional regulation were suggested to be associated with resilience. In the review from Eaton et al. (2022), the included task-based studies also found similar resultant regions, like PFC and amygdala, and functions regarding emotional regulation and reward processing (Heitzeg et al., 2008; Hanson et al., 2015; Luking et al., 2018; Rodman et al., 2019). Lower amygdala responses to negative stimuli, and tighter coupling of a PFC-amygdala circuit were found among high resilience individuals in task-based studies (Heitzeg et al., 2008; Rodman et al., 2019; Eaton et al., 2022). One of the studies in the current review also found that the right amygdala to mPFC connectivity was negatively associated with resilience (Uchida et al., 2015). This linked the findings between task-based studies and rest-state studies on resilience; however, there were no other studies had similar results. Align with similar reviews in resilience, similar findings on the association between emotion regulation, reward processing, and resilience were found. A suitable strategy for regulating emotions is essential for promoting resilience (Tugade and Fredrickson, 2007). Emotional regulation is also one of the main focuses of preventive interventions promoting resilience (Greenberg, 2006). Properly regulating emotion is one of the key protective factors of resilience (Troy and Mauss, 2011). On the other hand, hypoactivity of the OFC was associated with a decreased level of dopamine receptors (Volkow et al., 2002). The dopamine system plays a vital role in mediating reward processing (Stein, 2009). The association between OFC and the dopamine system suggests that hypoactivity of the OFC may influence the reward system via the dopamine system. In addition, Reward stimuli were found to have a buffering effect on stress (Dutcher and Creswell, 2018). Summarized from previous literature and the results from the included studies in this review, reward processing is also one of the key protective factors of resilience.
5. Limitations
Some limitations should be noted in this review. First, no quantitative analysis can be performed due to the limited studies included in each category. Although there were 19 included papers, the study designs were different, resulted in only nine to ten papers per category. In addition, this review adopted strict inclusion criteria. Only papers that clearly defined resilience were included. Resilience is a board topic when considering from different aspects. By limiting the focus, it helps this review to focus on the main purpose of assessing the neural correlates of resilience among mentally healthy individuals, and the protective mechanisms of resilience. Second, among the included studies, the average score for replicability was below 50% of the total score (M = 4.42). This score indicated a limit to the robustness of the results reported from each of the included study. With the lack of the control of the fMRI data or failed to report on the pre-processing steps of the data, it led to a concern of the trustworthiness of the results published. Replicability has been a concern among the field of resting-state fMRI studies (Chen X. et al., 2018). Future studies are suggested to account for and to report proper processing steps for imaging data for the robustness of the results. Third, reverse inference could be an issue for resting-state fMRI studies. Reasons backward from the neural activation to a cognitive function is a common concern over the interpretation of resting-state fMRI findings in the field of cognitive neuroscience (Poldrack, 2006; Wager et al., 2016). However, there is no common task that is universally accepted for assessing resilience in task-based fMRI. As mentioned in this review, inconsistent task, inconsistent difficulties, and variation on individual abilities limited the interpretation of neural result in relation to resilience. Since this review focused on resting-state fMRI studies, there are no specific cognitive process engaged. The inferences made by the findings in other relating neuroimaging studies with specific cognitive process in question might not be a major concern for resilience research. Anyhow, combination of resting-state fMRI and task-based fMRI, e.g., by adopting a naturalistic paradigm is recommended for future resilience neuroimaging studies. Future studies should also carefully consider the selection of brain regions of interests and the probability of the cognitive process in question in order to improve the confidence in reverse inferences (Poldrack, 2006). Lastly, as mentioned in the introduction, other neurological factors like neuroendocrine and monoamines can affect resilience-dependent change in neural activities captured in resting-state fMRI (Russo et al., 2012; Watanabe and Takeda, 2022). While this review is primarily focused on the resting-state studies in relation to resilience, future studies are suggested to include other neurological factors that may cause resilience-dependent change in neural activities.
6. Conclusion
This systematic review explored the resting-state neural correlates of resilience among high resilience individuals. Based on the findings, low resting-state activity of ACC, amygdala, and OFC and high resting-state insula activity could be the potential neural feature of high resilient. Brain regions involved in reward processing and emotional regulation were found in multiple studies associated with resilience. This result highlighted the importance of strategies for regulating emotions and perceiving rewards to enhance resilience. Future neuroimaging studies on resilience should consider adopting multiple resting-state fMRI modalities as well as operational definitions of resilience for plausible meta-analysis.
Author contributions
AT: study concept and design, data collection, data analysis, interpretation, and writing the manuscript. M-KL and XG: data interpretation and reviewing the manuscript. WL: study concept and design, data analysis, interpretation, and reviewing the manuscript. All authors contributed to the article and approved the submitted version.
Funding
This study was supported by the Early Career Scheme from the Research Grants Council (WL, Ref no.:28602319). The funder was not involved in the study design, collection, analysis, interpretation of data, the writing of this manuscript, or the decision to submit it for publication.
Conflict of interest
The authors declare that the research was conducted in the absence of any commercial or financial relationships that could be construed as a potential conflict of interest.
Publisher’s note
All claims expressed in this article are solely those of the authors and do not necessarily represent those of their affiliated organizations, or those of the publisher, the editors and the reviewers. Any product that may be evaluated in this article, or claim that may be made by its manufacturer, is not guaranteed or endorsed by the publisher.
Abbreviations
ACC, anterior cingulate cortex; ALE, activation likelihood estimation; ALFF, amplitude of low-frequency fluctuation; AN, attention network; BOLD, blood-oxygen-level dependent; CD-RISC, Connor Davison resilience Scale; CEN, central executive network; dACC, dorsal anterior cingulate cortex; DHEA, dehydroepiandrosterone; dlPFC, dorsolateral prefrontal cortex; DMN, dorsolateral prefrontal cortex; DMN, default mode network; EEG, electroencephalography; EN, emotional network; fALFF, fractional amplitude of low-frequency fluctuation; fMRI, functional magnetic resonance imaging; FNIRS, functional near-infrared spectroscopy; gICA, group spatial independent component analysis; HPA, hypothalamic-pituitary-adrenal; ICA, independent component analysis; IFG, inferior frontal gyrus; IPL, inferior parietal lobule; MN, memory network; mPFC, medial prefrontal cortex; MRI, magnetic resonance imaging; Nacc, nucleus accumbens; OFC, orbitofrontal cortex; PCC, posterior cingulate cortex; PFC, prefrontal cortex; PHC, parahippocampal cortex; PHG, parahippocampus gyrus; PLS, partial least squares; PRISMA, preferred reporting items for systematic reviews and meta-analyses; PTGI, posttraumatic growth inventory; PTSD, post-traumatic stress disorder; rACC, rostral anterior cingulate cortex; Reho, regional homogeneity; ROI, regions-of-interest; sgACC, subgenual anterior cingulate; SMG, superior medial gyrus; SN, salience network; SPL, superior parietal lobule; VLPFC, ventrolateral prefrontal cortex; vmPFC, ventromedial prefrontal cortex; VTA, ventral tegmental area.
Footnotes
References
Abolghasemi, A., and Taklavi Varaniyab, S. (2010). Resilience and perceived stress: Predictors of life satisfaction in the students of success and failure. Procedia Soc. Behav. Sci. 5, 748–752. doi: 10.1016/j.sbspro.2010.07.178
Aguirre, G., Zarahn, E., and D’Esposito, M. (1998). An area within human ventral cortex sensitive to stimuli: evidence and implications. Neuron 21, 373–383. doi: 10.1016/s0896-6273(00)80546-2
Andrews-Hanna, J. (2012). The brain’s default network and its adaptive role in internal mentation. Neuroscientist 18, 251–270. doi: 10.1177/1073858411403316
Berridge, K., and Kringelbach, M. (2013). Neuroscience of affect: brain mechanisms of pleasure and displeasure. Curr. Opin. Neurobiol. 23, 294–303. doi: 10.1016/j.conb.2013.01.017
Bing, X., Ming-Guo, Q., Ye, Z., Jing-Na, Z., Min, L., Han, C., et al. (2013). Alterations in the cortical thickness and the amplitude of low-frequency fluctuation in patients with post-traumatic stress disorder. Brain Res. 1490, 225–232. doi: 10.1016/j.brainres.2012.10.048
Bolsinger, J., Seifritz, E., Kleim, B., and Manoliu, A. (2018). Neuroimaging Correlates of Resilience to Traumatic Events-A Comprehensive Review. Front. Psychiatry 9:693. doi: 10.3389/fpsyt.2018.00693
Buckner, R., Andrews-Hanna, J., and Schacter, D. (2008). The brain’s default network: anatomy, function, and relevance to disease. Ann. N. Y. Acad. Sci. 1124, 1–38. doi: 10.1196/annals.1440.011
Burgess, P., Dumontheil, I., and Gilbert, S. (2007). The gateway hypothesis of rostral prefrontal cortex (area 10) function. Trends Cogn. Sci. 11, 290–298. doi: 10.1016/j.tics.2007.05.004
Bush, G., Vogt, B., Holmes, J., Dale, A., Greve, D., Jenike, M., et al. (2002). Dorsal anterior cingulate cortex: a role in reward-based decision making. Proc. Natl. Acad. Sci. U. S. A. 99, 523–528. doi: 10.1073/pnas.012470999
Callaghan, B., Gee, D., Gabard-Durnam, L., Telzer, E., Humphreys, K., Goff, B., et al. (2019). Decreased Amygdala Reactivity to Parent Cues Protects Against Anxiety Following Early Adversity: An Examination Across 3 Years. Biol. Psychiatry Cogn. Neurosci. Neuroimaging 4, 664–671. doi: 10.1016/j.bpsc.2019.02.001
Catalino, M., Yao, S., Green, D., Laws, E., Golby, A., and Tie, Y. (2020). Mapping cognitive and emotional networks in neurosurgical patients using resting-state functional magnetic resonance imaging. Neurosurg. Focus 48, E9. doi: 10.3171/2019.11.FOCUS19773
Chen, D., Wu, J., Yao, Z., Lei, K., Luo, Y., and Li, Z. (2018). Negative association between resilience and event-related potentials evoked by negative emotion. Sci. Rep. 8:7149. doi: 10.1038/s41598-018-25555-w
Chen, X., Lu, B., and Yan, C. (2018). Reproducibility of R-fMRI metrics on the impact of different strategies for multiple comparison correction and sample sizes. Hum. Brain Mapp. 39, 300–318. doi: 10.1002/hbm.23843
Connor, K., and Davidson, J. (2003). Development of a new resilience scale: the Connor-Davidson Resilience Scale (CD-RISC). Depress Anxiety 18, 76–82. doi: 10.1002/da.10113
Constable, R. T. (2006). “Challenges in fMRI and its limitations,” in Functional MRI: Basic Principles and Clinical Applications, eds S. H. Faro and F. B. Mohamed (New York, NY: Springer), doi: 10.1007/0-387-34665-1_4
Curtis, W., and Cicchetti, D. (2007). Emotion and resilience: a multilevel investigation of hemispheric electroencephalogram asymmetry and emotion regulation in maltreated and nonmaltreated children. Dev. Psychopathol. 19, 811–840. doi: 10.1017/S0954579407000405
Daniels, J., Hegadoren, K., Coupland, N., Rowe, B., Densmore, M., Neufeld, R., et al. (2012). Neural correlates and predictive power of trait resilience in an acutely traumatized sample: a pilot investigation. J. Clin. Psychiatry 73, 327–332. doi: 10.4088/JCP.10m06293
Decety, J., and Jackson, P. (2004). The functional architecture of human empathy. Behav. Cogn. Neurosci. Rev. 3, 71–100. doi: 10.1177/1534582304267187
Dedovic, K., Slavich, G., Muscatell, K., Irwin, M., and Eisenberger, N. (2016). Dorsal Anterior Cingulate Cortex Responses to Repeated Social Evaluative Feedback in Young Women with and without a History of Depression. Front. Behav. Neurosci. 10:64. doi: 10.3389/fnbeh.2016.00064
Dennison, M., Sheridan, M., Busso, D., Jenness, J., Peverill, M., Rosen, M., et al. (2016). Neurobehavioral markers of resilience to depression amongst adolescents exposed to child abuse. J. Abnorm. Psychol. 125, 1201–1212. doi: 10.1037/abn0000215
Dosenbach, N. U., Nardos, B., Cohen, A. L., Fair, D. A., Power, J. D., et al. (2010). Prediction of individual brain maturity using fMRI. Science, 329, 1358–1361.
Downar, J., Crawley, A., Mikulis, D., and Davis, K. D. (2000). A multimodal cortical network for the detection of changes in the sensory environment. Nat. Neurosci. 3, 277–283. doi: 10.1038/72991
Dutcher, J., and Creswell, J. (2018). The role of brain reward pathways in stress resilience and health. Neurosci. Biobehav. Rev. 95, 559–567. doi: 10.1016/j.neubiorev.2018.10.014
Eaton, S., Cornwell, H., Hamilton-Giachritsis, C., and Fairchild, G. (2022). Resilience and young people’s brain structure, function and connectivity: A systematic review. Neurosci. Biobehav. Rev. 132, 936–956. doi: 10.1016/j.neubiorev.2021.11.001
Epstein, R., and Kanwisher, N. (1998). A cortical representation of the local visual environment. Nature 392, 598–601. doi: 10.1038/33402
Finn, E. (2021). Is it time to put rest to rest? Trends Cogn. Sci. 25, 1021–1032. doi: 10.1016/j.tics.2021.09.005
Fleshner, M., Maier, S., Lyons, D., and Raskind, M. (2011). The neurobiology of the stress-resistant brain. Stress 14, 498–502.
Friborg, O., Hjemdal, O., Rosenvinge, J., and Martinussen, M. (2003). A new rating scale for adult resilience: what are the central protective resources behind healthy adjustment?. Int. J. Methods Psychiatr. Res. 12, 65–76. doi: 10.1002/mpr.143
Fujisawa, T., Jung, M., Kojima, M., Saito, D., Kosaka, H., and Tomoda, A. (2015). Neural Basis of Psychological Growth following Adverse Experiences: A Resting-State Functional MRI Study. PLoS One 10:e0136427. doi: 10.1371/journal.pone.0136427
Golkar, A., Lonsdorf, T., Olsson, A., Lindstrom, K., Berrebi, J., Fransson, P., et al. (2012). Distinct contributions of the dorsolateral prefrontal and orbitofrontal cortex during emotion regulation. PLoS One 7:e48107. doi: 10.1371/journal.pone.0048107
Green, J., McLaughlin, K., Berglund, P., Gruber, M., Sampson, N., Zaslavsky, A., et al. (2010). Childhood adversities and adult psychiatric disorders in the national comorbidity survey replication I: associations with first onset of DSM-IV disorders. Arch. Gen. Psychiatry 67, 113–123. doi: 10.1001/archgenpsychiatry.2009.186
Greenberg, M. (2006). Promoting resilience in children and youth: preventive interventions and their interface with neuroscience. Ann. N. Y. Acad. Sci. 1094, 139–150. doi: 10.1196/annals.1376.013
Hallegatte, S., Vogt-Schilb, A., Rozenberg, J., Bangalore, M., and Beaudet, C. (2020). From Poverty to Disaster and Back: a Review of the Literature. Econ. Disast. Clim. Change 4, 223–247. doi: 10.1007/S41885-020-00060-5
Hanson, J., Hariri, A., and Williamson, D. (2015). Blunted Ventral Striatum Development in Adolescence Reflects Emotional Neglect and Predicts Depressive Symptoms. Biol. Psychiatry 78, 598–605. doi: 10.1016/j.biopsych.2015.05.010
Heitzeg, M., Nigg, J., Yau, W., Zubieta, J., and Zucker, R. (2008). Affective circuitry and risk for alcoholism in late adolescence: differences in frontostriatal responses between vulnerable and resilient children of alcoholic parents. Alcohol. Clin. Exp. Res. 32, 414–426. doi: 10.1111/j.1530-0277.2007.00605.x
Hemington, K., Rogachov, A., Cheng, J., Bosma, R., Kim, J., Osborne, N., et al. (2018). Patients with chronic pain exhibit a complex relationship triad between pain, resilience, and within- and cross-network functional connectivity of the default mode network. Pain 159, 1621–1630. doi: 10.1097/j.pain.0000000000001252
Hidayat, N., and Nurhayati, S. R. (2019). The Effect of Social Support and Hope on Resilience in Adolescents. Humaniora 10:219. doi: 10.21512/humaniora.v10i3.5852
Hiew, C. C., Mori, T., Shimizu, M., and Tominaga, M. (2000). Measurement of resilience development: Preliminary results with a state-trait resilience inventory. J. Learn. Curricul. Dev. 1, 111–117.
Hirshfeld-Becker, D., Gabrieli, J., Shapero, B., Biederman, J., Whitfield-Gabrieli, S., and Chai, X. (2019). Intrinsic Functional Brain Connectivity Predicts Onset of Major Depression Disorder in Adolescence: A Pilot Study. Brain Connect. 9, 388–398. doi: 10.1089/brain.2018.0646
Holz, N., Tost, H., and Meyer-Lindenberg, A. (2020). Resilience and the brain: a key role for regulatory circuits linked to social stress and support. Mol. Psychiatry 25, 379–396. doi: 10.1038/s41380-019-0551-9
Homberg, J., and Jagiellowicz, J. (2022). A neural model of vulnerability and resilience to stress-related disorders linked to differential susceptibility. Mol. Psychiatry 27, 514–524. doi: 10.1038/s41380-021-01047-8
Hooberman, J., Rosenfeld, B., Rasmussen, A., and Keller, A. (2010). Resilience in trauma-exposed refugees: the moderating effect of coping style on resilience variables. Am. J. Orthopsychiatry 80, 557–563. doi: 10.1111/j.1939-0025.2010.01060.x
Horn, S., Charney, D., and Feder, A. (2016). Understanding resilience: New approaches for preventing and treating PTSD. Exp. Neurol. 284, 119–132.
Iadipaolo, A., Marusak, H., Paulisin, S., Sala-Hamrick, K., Crespo, L., Elrahal, F., et al. (2018). Distinct neural correlates of trait resilience within core neurocognitive networks in at-risk children and adolescents. Neuroimage Clin. 20, 24–34. doi: 10.1016/j.nicl.2018.06.026
Jeon, S., Lee, Y., Park, I., Kim, N., Kim, S., Jun, J., et al. (2020). Resting State Functional Connectivity of the Thalamus in North Korean Refugees with and without Posttraumatic Stress Disorder. Sci. Rep. 10:3194. doi: 10.1038/s41598-020-59815-5
Jeong, H., Park, S., Dager, S., Lim, S., Lee, S., Hong, H., et al. (2019). Altered functional connectivity in the fear network of firefighters with repeated traumatic stress. Br. J. Psychiatry 214, 347–353. doi: 10.1192/bjp.2018.260
Kalisch, R., Baker, D., Basten, U., Boks, M., Bonanno, G., Brummelman, E., et al. (2017). The resilience framework as a strategy to combat stress-related disorders. Nat. Hum. Behav. 1, 784–790. doi: 10.1038/s41562-017-0200-8
Kalisch, R., Cramer, A., Binder, H., Fritz, J., Leertouwer, I., Lunansky, G., et al. (2019). Deconstructing and Reconstructing Resilience: A Dynamic Network Approach. Perspect. Psychol. Sci. 14, 765–777. doi: 10.1177/1745691619855637
Kalisch, R., Müller, M., and Tüscher, O. (2015). A conceptual framework for the neurobiological study of resilience. Behav. Brain Sci. 38, e92. doi: 10.1017/S0140525X1400082X
Karreman, A., and Vingerhoets, A. J. J. M. (2012). Attachment and well-being: The mediating role of emotion regulation and resilience. Pers. Individ. Differ. 53, 821–826.
Katti, G., Ara, S., and Shireen, A. (2011). Magnetic resonance imaging (MRI)–A review. Int. J. Dent. Clin. 3, 65–70.
Kennis, M., Rademaker, A., van Rooij, S., Kahn, R., and Geuze, E. (2015). Resting state functional connectivity of the anterior cingulate cortex in veterans with and without post-traumatic stress disorder. Hum. Brain Mapp. 36, 99–109. doi: 10.1002/hbm.22615
Kilpatrick, L., Istrin, J., Gupta, A., Naliboff, B., Tillisch, K., Labus, J., et al. (2015). Sex commonalities and differences in the relationship between resilient personality and the intrinsic connectivity of the salience and default mode networks. Biol. Psychol. 112, 107–115. doi: 10.1016/j.biopsycho.2015.09.010
Kim, P., Evans, G., Angstadt, M., Ho, S., Sripada, C., Swain, J., et al. (2013). Effects of childhood poverty and chronic stress on emotion regulatory brain function in adulthood. Proc. Natl. Acad. Sci. U. S. A. 110, 18442–18447. doi: 10.1073/pnas.1308240110
Kirmani, M. N., Sharma, P., Anas, M., and Sanam, R. (2015). Hope, Resilience and Subjective Well-being among college going Adolescent Girls. Int. J. Human. Soc. Sci. Stud. 2, 262–270.
Kong, F., Ma, X., You, X., and Xiang, Y. (2018). The resilient brain: psychological resilience mediates the effect of amplitude of low-frequency fluctuations in orbitofrontal cortex on subjective well-being in young healthy adults. Soc. Cogn. Affect. Neurosci. 13, 755–763. doi: 10.1093/scan/nsy045
Kong, F., Wang, X., Hu, S., and Liu, J. (2015). Neural correlates of psychological resilience and their relation to life satisfaction in a sample of healthy young adults. Neuroimage 123, 165–172. doi: 10.1016/j.neuroimage.2015.08.020
Lau, W., Leung, M., Chan, C., Wong, S., and Lee, T. (2015). Can the neural-cortisol association be moderated by experience-induced changes in awareness? Sci. Rep. 5:16620. doi: 10.1038/srep16620
Lau, W., Leung, M., and Zhang, R. (2020). Hypofunctional connectivity between the posterior cingulate cortex and ventromedial prefrontal cortex in autism: Evidence from coordinate-based imaging meta-analysis. Prog. Neuropsychopharmacol. Biol. Psychiatry 103:109986. doi: 10.1016/j.pnpbp.2020.109986
Lau, W., Tai, A., Chan, J., Lau, B., and Geng, X. (2021). Integrative psycho-biophysiological markers in predicting psychological resilience. Psychoneuroendocrinology 129:105267. doi: 10.1016/j.psyneuen.2021.105267
Leaver, A., Yang, H., Siddarth, P., Vlasova, R., Krause, B., St Cyr, N., et al. (2018). Resilience and amygdala function in older healthy and depressed adults. J. Affect. Disord. 237, 27–34. doi: 10.1016/j.jad.2018.04.109
Li, M., Yang, Y., Liu, L., and Wang, L. (2016). Effects of social support, hope and resilience on quality of life among Chinese bladder cancer patients: a cross-sectional study. Health Qual. Life Outcomes 14:73. doi: 10.1186/s12955-016-0481-z
Linden, D. (2006). How psychotherapy changes the brain–the contribution of functional neuroimaging. Mol. Psychiatry 11, 528–538. doi: 10.1038/sj.mp.4001816
Liu, J., Ren, L., Womer, F., Wang, J., Fan, G., Jiang, W., et al. (2014). Alterations in amplitude of low frequency fluctuation in treatment-naïve major depressive disorder measured with resting-state fMRI. Hum. Brain Mapp. 35, 4979–4988. doi: 10.1002/hbm.22526
Long, L., Bistricky, S., Phillips, C., D’Souza, J., Richardson, A., Lai, B., et al. (2020). The Potential Unique Impacts of Hope and Resilience on Mental Health and Well-Being in the Wake of Hurricane Harvey. J. Trauma Stress 33, 962–972. doi: 10.1002/jts.22555
Luking, K., Nelson, B., Infantolino, Z., Sauder, C., and Hajcak, G. (2018). Ventral striatal function interacts with positive and negative life events to predict concurrent youth depressive symptoms. Biol. Psychiatry Cogn. Neurosci. Neuroimaging 3, 937–946. doi: 10.1016/j.bpsc.2018.07.007
Maciejewski, D., Brieant, A., Lee, J., King-Casas, B., and Kim-Spoon, J. (2020). Neural Cognitive Control Moderates the Relation between Negative Life Events and Depressive Symptoms in Adolescents. J. Clin. Child Adolesc. Psychol. 49, 118–133. doi: 10.1080/15374416.2018.1491005
Maddock, R., Garrett, A., and Buonocore, M. (2001). Remembering familiar people: the posterior cingulate cortex and autobiographical memory retrieval. Neuroscience 104, 667–676. doi: 10.1016/s0306-4522(01)00108-7
Maddock, R., Garrett, A., and Buonocore, M. (2003). Posterior cingulate cortex activation by emotional words: fMRI evidence from a valence decision task. Hum. Brain Mapp. 18, 30–41. doi: 10.1002/hbm.10075
Mehta, R., and Parasuraman, R. (2013). Neuroergonomics: a review of applications to physical and cognitive work. Front. Hum. Neurosci. 7:889. doi: 10.3389/fnhum.2013.00889
Menon, V. (2015). “Salience Network,” in Brain mapping: An encyclopedic reference, ed. A. Toga (Cambridge, MA: Academic Press), 597–611.
Menon, V., and Uddin, L. (2010). Saliency, switching, attention and control: a network model of insula function. Brain Struct. Funct. 214, 655–667. doi: 10.1007/s00429-010-0262-0
Mestre, J., Núñez-Lozano, J., Gómez-Molinero, R., Zayas, A., and Guil, R. (2017). Emotion Regulation Ability and Resilience in a Sample of Adolescents from a Suburban Area. Front. Psychol. 8:1980. doi: 10.3389/fpsyg.2017.01980
Mills, A., Sakai, O., Anderson, S., and Jara, H. (2017). Principles of Quantitative MR Imaging with Illustrated Review of Applicable Modular Pulse Diagrams. Radiographics 37, 2083–2105. doi: 10.1148/rg.2017160099
Mitchell, J., Heatherton, T., and Macrae, C. (2002). Distinct neural systems subserve person and object knowledge. Proc. Natl. Acad. Sci. U. S. A. 99, 15238–15243. doi: 10.1073/pnas.232395699
Miyagi, T., Oishi, N., Kobayashi, K., Ueno, T., Yoshimura, S., Murai, T., et al. (2020). Psychological resilience is correlated with dynamic changes in functional connectivity within the default mode network during a cognitive task. Sci. Rep. 10:17760. doi: 10.1038/s41598-020-74283-7
Moradi, S., and Mirkohi, M. G. (2020). Comparing the Role of Hope and Resilience in Predicting life Satisfaction in Older Adults. J. Gerontol. 5, 71–81.
Moreno-López, L., Ioannidis, K., Askelund, A., Smith, A., Schueler, K., and van Harmelen, A. (2020). The Resilient Emotional Brain: A Scoping Review of the Medial Prefrontal Cortex and Limbic Structure and Function in Resilient Adults With a History of Childhood Maltreatment. Biol. Psychiatry Cogn. Neurosci. Neuroimaging 5, 392–402. doi: 10.1016/j.bpsc.2019.12.008
Motzkin, J., Philippi, C., Wolf, R., Baskaya, M., and Koenigs, M. (2015). Ventromedial prefrontal cortex is critical for the regulation of amygdala activity in humans. Biol. Psychiatry 77, 276–284. doi: 10.1016/j.biopsych.2014.02.014
Murray, E. (2007). The amygdala, reward and emotion. Trends Cogn. Sci. 11, 489–497. doi: 10.1016/j.tics.2007.08.013
Myers, D. R., Polson, C., McGee, J., and Gillespie, R. (2019). Hope and Resilience Among Vulnerable, Community-Dwelling Older Persons. Innov. Aging 3, S531–S531. doi: 10.1093/geroni/igz038.1953
New, A., Fan, J., Murrough, J., Liu, X., Liebman, R., Guise, K., et al. (2009). A functional magnetic resonance imaging study of deliberate emotion regulation in resilience and posttraumatic stress disorder. Biol. Psychiatry 66, 656–664. doi: 10.1016/j.biopsych.2009.05.020
Noachtar, S., and Rémi, J. (2009). The role of EEG in epilepsy: a critical review. Epilepsy Behav. 15, 22–33. doi: 10.1016/j.yebeh.2009.02.035
Page, M., McKenzie, J., Bossuyt, P., Boutron, I., Hoffmann, T., Mulrow, C., et al. (2021). The P 2020 statement: an updated guideline for reporting systematic reviews. Syst. Rev. 10:89. doi: 10.1186/s13643-021-01626-4
Pardo, J., Pardo, P., Janer, K., and Raichle, M. (1990). The anterior cingulate cortex mediates processing selection in the Stroop attentional conflict paradigm. Proc. Natl. Acad. Sci. U. S. A. 87, 256–259. doi: 10.1073/pnas.87.1.256
Park, H., Kim, J., Lee, S., Seok, J., Chun, J., Kim, D., et al. (2008). Corpus callosal connection mapping using cortical gray matter parcellation and DT-MRI. Hum. Brain Mapp. 29, 503–516. doi: 10.1002/hbm.20314
Peltonen, K., Qouta, S., Diab, M., and Punamäki, R. (2014). Resilience among children in war: The role of multilevel social factors. Traumatology 20, 232–240. doi: 10.1037/h0099830
Peng, X., Lau, W., Wang, C., Ning, L., and Zhang, R. (2020). Impaired left amygdala resting state functional connectivity in subthreshold depression individuals. Sci. Rep. 10:17207. doi: 10.1038/s41598-020-74166-x
Phan, K., Wager, T., Taylor, S., and Liberzon, I. (2002). Functional neuroanatomy of emotion: a meta-analysis of emotion activation studies in PET and fMRI. Neuroimage 16, 331–348. doi: 10.1006/nimg.2002.1087
Poldrack, R. (2006). Can cognitive processes be inferred from neuroimaging data? Trends Cogn. Sci. 10, 59–63. doi: 10.1016/j.tics.2005.12.004
Polizzi, C. P., and Lynn, S. J. (2021). Regulating Emotionality to Manage Adversity: A Systematic Review of the Relation Between Emotion Regulation and Psychological Resilience. Cogn. Ther. Res. 45, 577–597. doi: 10.1007/s10608-020-10186-1
Qiu, C., Feng, Y., Meng, Y., Liao, W., Huang, X., Lui, S., et al. (2015). Analysis of Altered Baseline Brain Activity in Drug-Naive Adult Patients with Social Anxiety Disorder Using Resting-State Functional MRI. Psychiatry Investig. 12, 372–380. doi: 10.4306/pi.2015.12.3.372
Rabinak, C., Angstadt, M., Welsh, R., Kenndy, A., Lyubkin, M., Martis, B., et al. (2011). Altered amygdala resting-state functional connectivity in post-traumatic stress disorder. Front. Psychiatry 2:62. doi: 10.3389/fpsyt.2011.00062
Raichle, M., and Snyder, A. (2007). A default mode of brain function: a brief history of an evolving idea. Neuroimage 37, 1083–1090.
Reynaud, E., Guedj, E., Souville, M., Trousselard, M., Zendjidjian, X., and El Khoury-Malhame, M. (2013). Relationship between emotional experience and resilience: an fMRI study in fire-fighters. Neuropsychologia 51, 845–849. doi: 10.1016/j.neuropsychologia.2013.01.007
Rodman, A., Jenness, J., Weissman, D., Pine, D., and McLaughlin, K. (2019). Neurobiological Markers of Resilience to Depression Following Childhood Maltreatment: The Role of Neural Circuits Supporting the Cognitive Control of Emotion. Biol. Psychiatry 86, 464–473. doi: 10.1016/j.biopsych.2019.04.033
Russo, S., Murrough, J., Han, M., Charney, D., and Nestler, E. (2012). Neurobiology of resilience. Nat. Neurosci. 15, 1475–1484. doi: 10.1038/nn.3234
Russo, S. J., Murrough, J., Han, M., Charney, D., and Nestler, E. (2011). Neurobiology of resilience. Resil. Ment. Health 15, 1475–1484. doi: 10.1017/CBO9780511994791.003
Samani, S., Jokar, B., and Sahragard, N. (2007). Effects of Resilience on Mental Health and Life Satisfaction. Iran. J. Psychiatry Clin. Psychol. 13, 290–295.
Santarnecchi, E., Sprugnoli, G., Tatti, E., Mencarelli, L., Neri, F., Momi, D., et al. (2018). Brain functional connectivity correlates of coping styles. Cogn. Affect. Behav. Neurosci. 18, 495–508. doi: 10.3758/s13415-018-0583-7
Seghier, M. (2013). The angular gyrus: multiple functions and multiple subdivisions. Neuroscientist 19, 43–61. doi: 10.1177/1073858412440596
Shao, R., Lau, W., Leung, M., and Lee, T. (2018). Subgenual anterior cingulate-insula resting-state connectivity as a neural correlate to trait and state stress resilience. Brain Cogn. 124, 73–81. doi: 10.1016/j.bandc.2018.05.001
Shen, X., Tokoglu, F., Papademetris, X., and Constable, R. T. (2013). Groupwise whole-brain parcellation from resting-state fMRI data for network node identification. Neuroimage, 82, 403–415.
Shi, L., Ren, Z., and Qiu, J. (2021). High Thought Control Ability, High Resilience: The Effect of Temporal Cortex and Insula Connectivity. Neuroscience 472, 60–67. doi: 10.1016/j.neuroscience.2021.07.034
Shi, L., Sun, J., Wei, D., and Qiu, J. (2019). Recover from the adversity: functional connectivity basis of psychological resilience. Neuropsychologia 122, 20–27. doi: 10.1016/j.neuropsychologia.2018.12.002
Shiba, Y., Santangelo, A., and Roberts, A. (2016). Beyond the Medial Regions of Prefrontal Cortex in the Regulation of Fear and Anxiety. Front. Syst. Neurosci. 10:12. doi: 10.3389/fnsys.2016.00012
Simeon, D., Yehuda, R., Cunill, R., Knutelska, M., Putnam, F., and Smith, L. (2007). Factors associated with resilience in healthy adults. Psychoneuroendocrinology 32, 1149–1152. doi: 10.1016/j.psyneuen.2007.08.005
Simmons, A., Fitzpatrick, S., Strigo, I., Potterat, E., Johnson, D., Matthews, S., et al. (2012). Altered insula activation in anticipation of changing emotional states: neural mechanisms underlying cognitive flexibility in Special Operations Forces personnel. Neuroreport 23, 234–239. doi: 10.1097/WNR.0b013e3283503275
Singer, T. (2006). The neuronal basis and ontogeny of empathy and mind reading: review of literature and implications for future research. Neurosci. Biobehav. Rev. 30, 855–863. doi: 10.1016/j.neubiorev.2006.06.011
Singh, M., Chang, K., Kelley, R., Saggar, M., Reiss, A., and Gotlib, I. (2014). Early signs of anomalous neural functional connectivity in healthy offspring of parents with bipolar disorder. Bipolar Disord. 16, 678–689. doi: 10.1111/bdi.12221
Singh, M., Leslie, S., Packer, M., Weisman, E., and Gotlib, I. (2018). Limbic Intrinsic Connectivity in Depressed and High-Risk Youth. J. Am. Acad. Child Adolesc. Psychiatry 57, 775–785.e3.
Slagt, M., Dubas, J., Ellis, B., van Aken, M., and Deković, M. (2019). Linking emotional reactivity for better and for worse" to differential susceptibility to parenting among kindergartners. Dev. Psychopathol. 31, 741–758. doi: 10.1017/S0954579418000445
Smith, B., Dalen, J., Wiggins, K., Tooley, E., Christopher, P., and Bernard, J. (2008). The brief resilience scale: assessing the ability to bounce back. Int. J. Behav. Med. 15, 194–200. doi: 10.1080/10705500802222972
Stainton, A., Chisholm, K., Kaiser, N., Rosen, M., Upthegrove, R., Ruhrmann, S., et al. (2019). Resilience as a multimodal dynamic process. Early Interv. Psychiatry 13, 725–732. doi: 10.1111/eip.12726
Stein, D. J. (2009). The Psychobiology of Resilience. CNS Spectrums 14, 41–47. doi: 10.1017/S1092852900027280
Taylor, K., Seminowicz, D., and Davis, K. (2009). Two systems of resting state connectivity between the insula and cingulate cortex. Hum. Brain Mapp. 30, 2731–2745. doi: 10.1002/hbm.20705
Thomas, C., and Zolkoski, S. (2020). Preventing Stress Among Undergraduate Learners: The Importance of Emotional Intelligence, Resilience, and Emotion Regulation. Front. Educ. 5:94. doi: 10.3389/FEDUC.2020.00094
Troy, A. S., and Mauss, I. B. (2011). “Resilience in the face of stress: Emotion regulation as a protective factor,” in Resilience and Mental Health: Challenges Across the Lifespan, eds S. M. Southwick, B. T. Litz, D. Charney, and M. J. Friedman (Cambridge, MA: Cambridge University Press), doi: 10.1017/CBO9780511994791.004
Tugade, M. M., and Fredrickson, B. L. (2007). Regulation of positive emotions: Emotion regulation strategies that promote resilience. J. Happiness Stud. 8, 311–333. doi: 10.1007/s10902-006-9015-4
Uchida, M., Biederman, J., Gabrieli, J., Micco, J., de Los Angeles, C., Brown, A., et al. (2015). Emotion regulation ability varies in relation to intrinsic functional brain architecture. Soc. Cogn. Affect. Neurosci. 10, 1738–1748. doi: 10.1093/scan/nsv059
van der Werff, S., Pannekoek, J., Veer, I., van Tol, M., Aleman, A., Veltman, D., et al. (2013a). Resilience to childhood maltreatment is associated with increased resting-state functional connectivity of the salience network with the lingual gyrus. Child Abuse Negl. 37, 1021–1029. doi: 10.1016/j.chiabu.2013.07.008
van der Werff, S., van den Berg, S., Pannekoek, J., Elzinga, B., and van der Wee, N. (2013b). Neuroimaging resilience to stress: a review. Front. Behav. Neurosci. 7:39. doi: 10.3389/fnbeh.2013.00039
van Harmelen, A., Kievit, R., Ioannidis, K., Neufeld, S., Jones, P., Bullmore, E., et al. (2017). Adolescent friendships predict later resilient functioning across psychosocial domains in a healthy community cohort. Psychol. Med. 47, 2312–2322. doi: 10.1017/S0033291717000836
van Rooij, S., Stevens, J., Ely, T., Fani, N., Smith, A., Kerley, K., et al. (2016). Childhood Trauma and COMT Genotype Interact to Increase Hippocampal Activation in Resilient Individuals. Front. Psychiatry 7:156. doi: 10.3389/fpsyt.2016.00156
Veer, I., Riepenhausen, A., Zerban, M., Wackerhagen, C., Puhlmann, L., Engen, H., et al. (2021). Psycho-social factors associated with mental resilience in the Corona lockdown. Transl Psychiatry. 11:67. doi: 10.1038/s41398-020-01150-4
Vernon, R. F. (2004). “A Brief History of Resilience,” in Community Planning to Foster Resilience in Children, eds C. S. Clauss-Ehlers and M. D. Weist (Boston, MA: Springer).
Vilares, I., Howard, J., Fernandes, H., Gottfried, J., and Kording, K. (2012). Differential representations of prior and likelihood uncertainty in the human brain. Curr. Biol. 22, 1641–1648. doi: 10.1016/j.cub.2012.07.010
Volkow, N., Fowler, J., Wang, G., Ding, Y., and Gatley, S. (2002). Role of dopamine in the therapeutic and reinforcing effects of methylphenidate in humans: results from imaging studies. Eur. Neuropsychopharmacol. 12, 557–566. doi: 10.1016/s0924-977x(02)00104-9
Volle, E., Gonen-Yaacovi, G., Costello Ade, L., Gilbert, S., and Burgess, P. (2011). The role of rostral prefrontal cortex in prospective memory: a voxel-based lesion study. Neuropsychologia 49, 2185–2198. doi: 10.1016/j.neuropsychologia.2011.02.045
Wager, T., Davidson, M., Hughes, B., Lindquist, M., and Ochsner, K. (2008). Prefrontal-subcortical pathways mediating successful emotion regulation. Neuron 59, 1037–1050. doi: 10.1016/j.neuron.2008.09.006
Wager, T. D., Atlas, L., Botvinick, M., Chang, L., Coghill, R., Davis, K., et al. (2016). Pain in the ACC? Proc. Natl. Acad. Sci. U. S. A. 113, E2474–E2475.
Wang, S., Xu, X., Zhou, M., Chen, T., Yang, X., Chen, G., et al. (2017). Hope and the brain: Trait hope mediates the protective role of medial orbitofrontal cortex spontaneous activity against anxiety. Neuroimage 157, 439–447. doi: 10.1016/j.neuroimage.2017.05.056
Wang, S., Yang, C., Zhao, Y., Lai, H., Zhang, L., and Gong, Q. (2020). Sex-linked neurofunctional basis of psychological resilience in late adolescence: a resting-state functional magnetic resonance imaging study. Eur. Child Adolesc. Psychiatry 29, 1075–1087. doi: 10.1007/s00787-019-01421-6
Watanabe, N., and Takeda, M. (2022). Neurophysiological dynamics for psychological resilience: A view from the temporal axis. Neurosci. Res. 175, 53–61.
Waugh, C., Wager, T., Fredrickson, B., Noll, D., and Taylor, S. (2008). The neural correlates of trait resilience when anticipating and recovering from threat. Soc. Cogn. Affect. Neurosci. 3, 322–332. doi: 10.1093/scan/nsn024
Whittaker, J., Foley, S., Ackling, E., Murphy, K., and Caseras, X. (2018). The Functional Connectivity Between the Nucleus Accumbens and the Ventromedial Prefrontal Cortex as an Endophenotype for Bipolar Disorder. Biol. Psychiatry 84, 803–809. doi: 10.1016/j.biopsych.2018.07.023
Windle, G., Bennett, K., and Noyes, J. (2011). A methodological review of resilience measurement scales. Health Qual. Life Outcomes 9:8. doi: 10.1186/1477-7525-9-8
Wymbs, N., Orr, C., Albaugh, M., Althoff, R., O’Loughlin, K., Holbrook, H., et al. (2020). Social supports moderate the effects of child adversity on neural correlates of threat processing. Child. Abuse Negl. 102:104413. doi: 10.1016/j.chiabu.2020.104413
Xu, K., Liu, H., Li, H., Tang, Y., Womer, F., Jiang, X., et al. (2014). Amplitude of low-frequency fluctuations in bipolar disorder: a resting state fMRI study. J. Affect. Dis. 152, 237–242.
Yin, Y., Jin, C., Hu, X., Duan, L., Li, Z., Song, M., et al. (2011). Altered resting-state functional connectivity of thalamus in earthquake-induced posttraumatic stress disorder: a functional magnetic resonance imaging study. Brain Res. 1411, 98–107. doi: 10.1016/j.brainres.2011.07.016
Yu, X., and Zhang, J. (2007). Factor analysis and psychometric evaluation of the Connor-Davidson Resilience Scale (CD-RISC) with chinese people. Soc. Behav. Pers. 35, 19–30. doi: 10.2224/sbp.2007.35.1.19
Keywords: psychological resilience, resting-state, functional magnetic resonance imaging (fMRI), healthy population, operational definitions of resilience, neural mechanisms
Citation: Tai APL, Leung M-K, Geng X and Lau WKW (2023) Conceptualizing psychological resilience through resting-state functional MRI in a mentally healthy population: a systematic review. Front. Behav. Neurosci. 17:1175064. doi: 10.3389/fnbeh.2023.1175064
Received: 27 February 2023; Accepted: 03 July 2023;
Published: 19 July 2023.
Edited by:
Mario Liotti, Simon Fraser University, CanadaReviewed by:
Ivan V. Brak, State Scientific Research Institute of Physiology and Basic Medicine, RussiaMasaki Takeda, Kochi University of Technology, Japan
Copyright © 2023 Tai, Leung, Geng and Lau. This is an open-access article distributed under the terms of the Creative Commons Attribution License (CC BY). The use, distribution or reproduction in other forums is permitted, provided the original author(s) and the copyright owner(s) are credited and that the original publication in this journal is cited, in accordance with accepted academic practice. No use, distribution or reproduction is permitted which does not comply with these terms.
*Correspondence: Way K. W. Lau, wkwlau@hkmu.edu.hk; Xiujuan Geng, gengx@cuhk.edu.hk