- 1Institute of Neuroscience and Medicine – Neuromodulation, Jülich Research Center, Jülich, Germany
- 2Department of Neurosurgery, Stanford University, Stanford, CA, USA
- 3Department of Neuromodulation, University of Cologne, Cologne, Germany
In the nervous system, synchronization processes play an important role, e.g., in the context of information processing and motor control. However, pathological, excessive synchronization may strongly impair brain function and is a hallmark of several neurological disorders. This focused review addresses the question of how an abnormal neuronal synchronization can specifically be counteracted by invasive and non-invasive brain stimulation as, for instance, by deep brain stimulation for the treatment of Parkinson’s disease, or by acoustic stimulation for the treatment of tinnitus. On the example of coordinated reset (CR) neuromodulation, we illustrate how insights into the dynamics of complex systems contribute to successful model-based approaches, which use methods from synergetics, non-linear dynamics, and statistical physics, for the development of novel therapies for normalization of brain function and synaptic connectivity. Based on the intrinsic multistability of the neuronal populations induced by spike timing-dependent plasticity (STDP), CR neuromodulation utilizes the mutual interdependence between synaptic connectivity and dynamics of the neuronal networks in order to restore more physiological patterns of connectivity via desynchronization of neuronal activity. The very goal is to shift the neuronal population by stimulation from an abnormally coupled and synchronized state to a desynchronized regime with normalized synaptic connectivity, which significantly outlasts the stimulation cessation, so that long-lasting therapeutic effects can be achieved.
1. Introduction
Several brain disorders, such as Parkinson’s disease (PD), essential tremor, epilepsy, and tinnitus are characterized by abnormally strong, pathological neuronal synchronization (1–10). In PD, for example, excessive synchronization is associated with motor impairment (2, 7, 11–15), whereas neurons exhibit an asynchronous firing under physiological conditions (2, 16). Reduction of synchronized oscillations in β-band frequencies (8−35 Hz) by medication with dopaminergic drugs is positively correlated with amelioration of motor symptoms in PD patients (7, 13, 14). On the other hand, low-frequency (5−20 Hz) stimulation of subthalamic nucleus (STN) or its afferent fibers, which is intended to boost synchronous oscillations at these frequencies, results in a significant clinical deterioration of PD symptoms and motor functions in human patients and parkinsonian rodents (15, 17–22).
In tinnitus, abnormal patterns of enhanced synchronized neuronal activity were observed in human patients and animal models in brain areas differentiated from peripheral input (5, 6, 10, 23–27). In particular, pathologically elevated and persistent neuronal rhythms at δ-band (1.5−4 Hz) as well as at γ-band (>30 Hz) frequencies were found in patients with chronic tinnitus as compared to healthy controls (5, 6, 26–28), which correlate with tinnitus-related distress and tinnitus loudness. Furthermore, a positive association was found between normalization of this neuronal activity and reduction of tinnitus severity (25, 27, 29). Taken together, abnormal neuronal synchronization can significantly impair neural processes and brain functions and serves as target for novel therapies.
Nowadays, the standard therapy for medically refractory PD patients is electrical high-frequency (HF) deep brain stimulation (DBS) (30). For this, an electrical HF (>100 Hz) pulse train is delivered to a target brain area through a depth electrode. The treatment of severe neurological and psychiatric diseases such as PD, tremor, dystonia, chronic and phantom pain, major depression, obsessive–compulsive disorder, Tourette syndrome, and epilepsy with DBS is a rapidly growing and promising field (7, 30–32). The mechanism of HF DBS is, as yet, not fully understood. One may distinguish between local and non-local effects of HF stimulation. Locally, in the vicinity of the stimulation electrode, axons rather than cell bodies (somas) get activated (21, 33), while the latter can even be effectively inhibited by HF stimulation (34–36) via, e.g., synaptic inhibition or depolarization blockade (37–39). The stimulation-induced axonal activity propagates antidromically and orthodromically (21, 40) and can change the firing in the output structures downstream to the neuronal target population. In particular, the globus pallidum interior (GPi) might strongly be involved in the mechanisms of DBS (41–43). HF stimulation of the STN regularizes GPi firing (41), and this restores the responsiveness of the thalamus (42). The pathological discharge patterns can be replaced by HF spiking or suppressed depending on whether the efferent fibers of the stimulated nucleus are excitatory or inhibitory, respectively (41, 44, 45). Several contributing mechanisms, resulting in the observed effects of HF DBS might be membrane inhibition, jamming, excitation of excitatory and inhibitory afferents, excitation of efferents and plasticity (46). In particular, HF stimulation of afferent axons projecting to STN can account for therapeutic effects of HF DBS (21). The stimulation-induced effects are considered as a combination of local and non-local impacts of the stimulation, where HF DBS seems to strongly alter the neuronal firing and modulate the pathological neuronal activity.
KEY CONCEPT 1. Deep brain stimulation (DBS)
Standard therapy for medically refractory movements disorders, e.g., Parkinson’s disease and essential tremor. It requires a surgical treatment, where depth electrodes are chronically implanted in target areas like the thalamic ventralis intermedius nucleus or the subthalamic nucleus. For standard DBS electrical high-frequency (>100 Hz) charge-balanced pulses are permanently delivered via depth electrodes.
Human data demonstrate that HF DBS of the STN can suppress the pathological neuronal activity in the β band, which correlates with induced improvement of PD symptoms (47–50), whereas low-frequency stimulation at around 20 Hz enhances synchronization at similar frequencies. A modeling study (51) suggested that the HF periodic DBS may induce a chaotic desynchronization, while a desynchronizing impact of a periodic forcing on synchronized populations seems to be a rather general phenomenon (52). The effect of HF DBS may, however, depend on whether excitatory or inhibitory structures are stimulated (53). PD-related oscillatory activity suppressed during HF stimulation reemerges after cessation of the stimulation within a few tens of seconds (36, 48, 49). Other studies did not, however, observe any marked attenuation of the β-band power after STN DBS has been discontinued (54, 55). Analogously, the therapeutic effects of HF DBS on PD symptoms also quickly disappear after stimulation cessation (56). These observations reflect the fact that standard HF DBS has neither long-lasting electrophysiological nor clinical aftereffects, and the HF DBS therapy can be effective only when the neuronal target structures are permanently stimulated. In 309 PD patients (57), it was shown that best motor outcome of STN DBS was achieved with stimulation contacts localized within the STN as compared with the zona incerta. Accordingly, not just fibers, but neuronal populations appear to be optimal target for DBS. In this context, it might be interesting that in a computational study in isolated oscillatory STN neurons HF DBS suppresses the neuronal spiking, where the results obtained in this rather simple model resemble the clinically observed relation between stimulation amplitude and stimulation frequency required for therapeutic efficacy (58). However, in spite of many beneficial effects, in some patients DBS may not help, or may cause side effects, or the therapeutic effects may wear off over time (59–62).
KEY CONCEPT 2. Desynchronization
A process inverse to synchronization, where initially synchronized oscillating systems desynchronize as parameters change or they do so under the influence of appropriate external stimulation. Desynchronization is important, for example, in neuroscience and medicine, were pathologically strong synchronization of neurons may severely impair brain function as, e.g., in Parkinson’s disease or epilepsy.
KEY CONCEPT 3. Long-lasting stimulation aftereffects
The impact of the stimulation on the neuronal population, which essentially outlasts the stimulation cessation. Apart from acute therapeutic effects, i.e., effects occurring during stimulation, a significant suppression of symptoms and abnormal neuronal dynamics is preserved for a long post-stimulation time after the stimulation is completely switched off.
With the objective of finding more effective stimulation techniques, which specifically counteract an abnormal neuronal synchronization, a model-based development of novel stimulation methods has been initiated (63). Accordingly, synchronization control became a focus of the research nowadays, and several desynchronizing methods have been developed with the methods of non-linear dynamics and statistical physics (64–77). Some of these methods are based on closed-loop (delayed) feedback techniques, where the mean field of the neuronal ensemble is measured, preprocessed, and fed back as a stimulation signal, which desynchronizes the stimulated neurons in an intrinsic demand-controlled way (68–70, 72–74, 76). Other methods utilize phase-resetting techniques (64–67).
In computational models used for developing and optimizing novel stimulation techniques, one should take into account spike timing-dependent plasticity (STDP) (78–85), a fundamental property of neuronal tissue, according to which synaptic weights depend on the neuronal firing pattern. It was shown experimentally that synaptic plasticity enhances neuronal synchronization (86). From the kindling phenomenon, in the context of epilepsy (87), where preparatory stimulation induces the spontaneous production of epileptic seizures, it is well known that neural networks may learn pathologically strong synaptic interactions (88, 89). The novel desynchronizing stimulation protocols are designed to invert this pathological process via anti-kindling (90). The very goal is to stimulate in a way that the formerly affected neuronal populations unlearn their pathological connectivity and, hence, their tendency to produce pathological synchronization, where the physiological neuronal activity is re-established on a long-term basis (53, 90–95). Put otherwise, the stimulation aims at inducing long-lasting therapeutic effects, which outlast the cessation of stimulation.
KEY CONCEPT 4. Spike timing-dependent plasticity (STDP)
A fundamental property of the nervous system, where neurons continuously regulate the strength of their synaptic connections in relation to the mutual timing properties of their firing or bursting.
KEY CONCEPT 5. Anti-kindling
A process inverse to the kindling phenomenon know in the context of epilepsy, where neural networks may learn pathologically strong interactions and spontaneously produce epileptic seizures. Anti-kindling manifests itself when due to desynchronizing stimulation neuronal populations unlearn their pathological connectivity, and physiological neuronal activity is re-established on a long-term basis.
So far, several theoretical predictions concerning the anti-kindling properties of one of the novel desynchronizing techniques, coordinated reset (CR) neuromodulation (53, 66, 67, 90–93), have been verified experimentally. The mechanism of CR neuromodulation is based on the phase reset of oscillatory neuronal activity (66, 67). This technique appears to be effective under a variety of conditions and stimulation modalities since the phase reset is a universal phenomenon. A phase reset of rhythmically active neurons can be achieved, e.g., by hyperpolarizing or depolarizing electrical pulses (96–99), excitatory, or inhibitory post-synaptic potentials (100–104), sensory stimulation (105–108), and transcranial magnetic stimulation (109). The resetting impact and the induced transient desynchronization of an electrical short-pulse stimulation, on which the CR technique is based, have been reported in vivo for coupled neuronal bursters in the paddle fish (99). Long-lasting desynchronizing effects of CR stimulation have been investigated in detail in theoretical studies (53, 90, 91), and the results have experimentally been confirmed in vitro in epileptic rat hippocampal slice (110). The beneficial therapeutic long-lasting aftereffects of electrical CR stimulation at weak intensity have been observed in the 1-methyl-4-phenyl-1,2,3,6-tetrahydropyridine (MPTP)-treated macaque monkeys, in contrast to CR stimulation at high intensity and to standard HF DBS (111). It was shown that unilateral weak-intensity CR stimulation delivered to the STN of parkinsonian MPTP monkeys for only 2 h per day during 5 consecutive days leads to significant and sustained therapeutic aftereffects for at least 30 days, while standard 130 Hz DBS and strong-intensity CR stimulation have no or only little aftereffects, respectively (111). Lasting aftereffects of electrical CR stimulation of the STN were also observed in Parkinson patients stimulated during three consecutive days in two daily sessions of up to 2 h (112).
KEY CONCEPT 6. Coordinated reset (CR) neuromodulation
An effectively desynchronizing control technique, where a population of synchronized neurons is stimulated via several stimulation sites in such a way that spatially and timely coordinated phase resets are achieved in subpopulations assigned to each of the stimulation sites. This method was suggested for counteraction of abnormal neuronal synchronization characteristic for several neurological diseases. CR-induced long-lasting desynchronization has been verified in pre-clinical and clinical studies.
As discussed above, the effects of HF DBS may be quite different, depending on the target, e.g., neuronal populations as opposed to fibers, being stimulated. In contrast, computational studies showed that CR stimulation effectively induces anti-kindling by direct somatic stimulation as well as by excitatory or inhibitory synaptically mediated stimulation (94, 95). The latter stimulation setup might correspond to stimulation of afferent or efferent fibers, or sensory stimulation, where the stimulation signals arrive at the neural target population as post-synaptic potentials. Acoustic CR stimulation has been suggested for desynchronizing the neural synchrony underlying tinnitus (94) and successively verified in a clinical proof-of-concept study in tinnitus patients treated with non-invasive acoustic CR stimulation (26, 27, 29). It turned out that acoustic CR stimulation can significantly counteract both tinnitus symptoms and the underlying pathological neuronal synchronization in a tinnitus-related network of brain areas together with normalization of effective connectivity between these brain areas (26, 27, 29). Below we illustrate the mechanism and properties of CR neuromodulation in more detail and compare it to noise stimulation in neuronal networks with STDP.
2. STDP-Induced Multistability
It is well known that synapses in neuronal networks are involved in adaptation processes, where their efficacy is governed by spike timing-dependent plasticity (STDP), and the synaptic weights are either potentiated or depressed depending on the order of the spiking times of pre- and post-synaptic neurons (78–85). Adaptive synapses greatly extend the complexity and richness of the neuronal dynamics and functions of neuronal networks. STDP can, for instance, induce an overwhelming multistability of neuronal dynamical regimes (90, 95, 115, 116). Depending on the initial conditions, the system may converge to one or another of many coexisting stable states, which are characterized by different synaptic connectivities and extents of synchronization among neurons. This phenomenon is illustrated in Figure 1. The mean coupling C(t) of the neuronal population saturates at different limit states depending on the initial distribution of the time-dependent synaptic weights cij(t), which define the coupling strength from pre-synaptic neuron j to post-synaptic neuron i (Figure 1A). The neuronal population with STDP may thus exhibit diverse stable regimes of different connectivity strength. In these regimes, the collective dynamics of the neurons is also different. In particular, the oscillations of the local field potential (LFP) differ by their amplitude, such that stronger coupling leads to more pronounced LFP oscillations (Figure 1B). The LFP amplitude reflects the amount of synchronization among neurons, and it increases if the neurons self-organize in a synchronized rhythmic firing (Figure 1C). The coupling strength and neuronal synchronization are in correspondence with each other: stronger coupling results in stronger synchronization.
KEY CONCEPT 7. Multistability
Co-existence of two (in the case of bistability) or more different stable regimes in the state space of a system for the same values of system parameters. These states can be realized for different initial conditions or under perturbations shifting the system state to the corresponding regime. In biological systems, multistability is a common phenomenon.
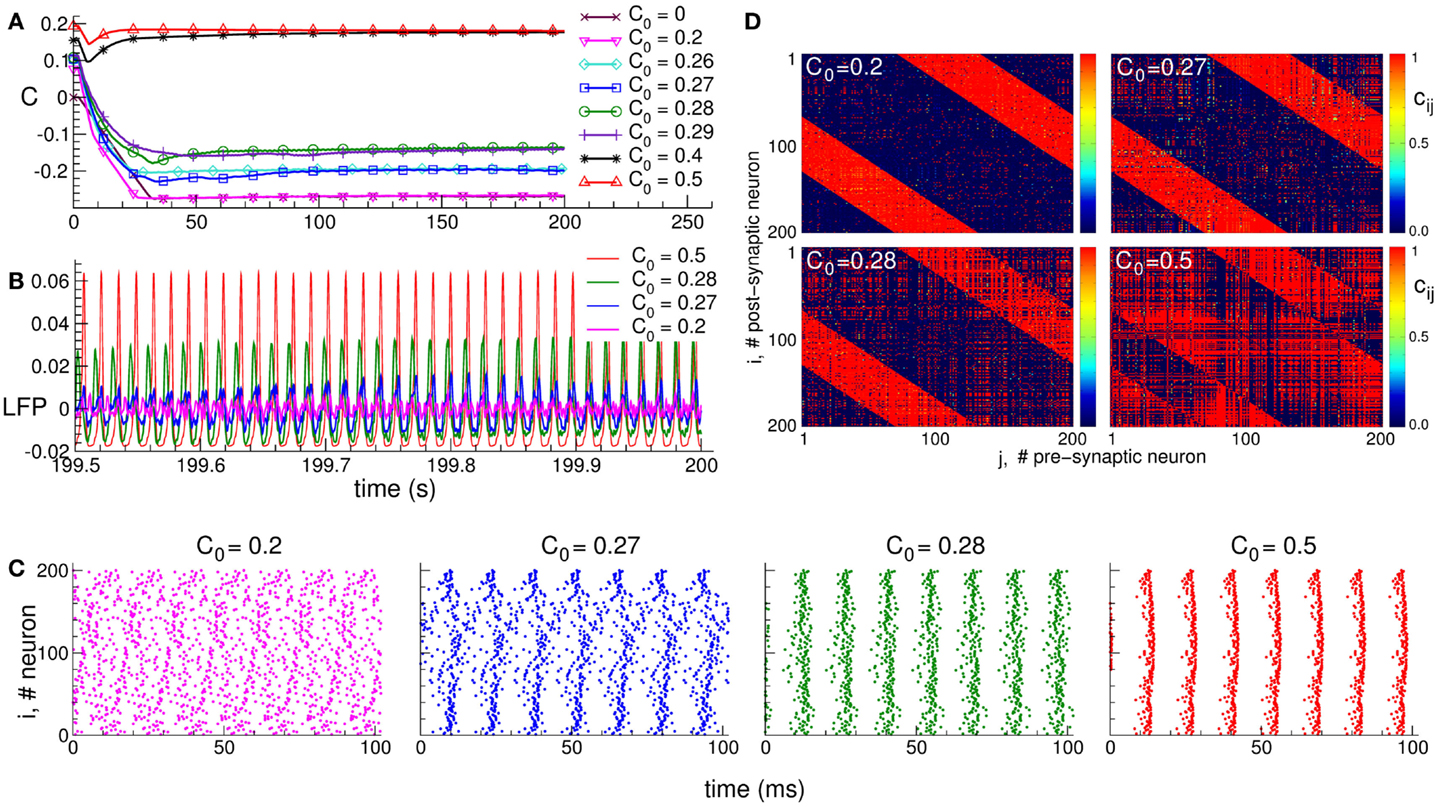
Figure 1. Plasticity-induced multistability of synchronized and desynchronized states in an ensemble of Hodgkin–Huxley neurons (113, 114) with STDP. (A) Time courses of the mean synaptic weights , where N is the number of neurons, cij(t) is the individual synaptic weight from pre-synaptic neuron j to post-synaptic neuron i, and is the spatial coupling profile in the form of a Mexican hat with distance dij between neurons, and parameters σ1 and σ2. The positive (negative) value of Mij indicates an excitatory (inhibitory) interaction, which corresponds to closely (distantly) located neurons. Boundary conditions are periodic. Initial synaptic weights {cij(0)} are randomly Gaussian distributed around the mean value C0 (indicated in the legend) with SD 0.01. (B) Time courses of the DC-balanced local field potential , where sj(t) is the post-synaptic potential of neuron j, of four stable synchronized and desynchronized regimes observed for the initial coupling matrices from (A) with mean values C0 indicated in the legend. (C) The corresponding raster plots of the neuronal spikes for the four stable states mentioned above. The initial mean values C0 are indicated in the plots. (D) The corresponding coupling matrices developed in the neuronal ensemble due to STDP for these four stable states. The initial mean values C0 are indicated in the plots. The oblique red stripes visible in the plots are the inhibitory connections, whereas the other connections are excitatory according to the considered Mexican hat coupling profile. The plots are adapted from Ref. (95), where further details can be found.
The dependence of the final coupling regimes on the initial connectivity is based on the above interrelation between the coupling strength and neuronal synchronization. Strong initial coupling synchronizes neurons from the very beginning. The synchronized neuronal dynamics then influences the synaptic weights via STDP, and the latter get even more potentiated, if the neurons are synchronized strongly enough with narrowly distributed relative spike timing (90, 95). This, in turn, enhances the neuronal synchronization. Such a self-organization process eventually results in a stable strongly coupled and synchronized regime. On the other hand, if the initial coupling and, thus, neuronal synchronization are weak, the synaptic weights will be depressed, and a stable weakly coupled and desynchronized regime will be established in the neuronal population due to STDP. Along with the above two limiting regimes, many other regimes of intermediate coupling strength and synchronization may coexist (Figure 1).
The structure of the synaptic connectivity also varies when the STDP-induced regimes of strong or weak coupling are realized in the neuronal population. In the weakly coupled and weakly synchronized regime, for instance, the excitatory connections are suppressed, whereas the inhibitory connections are potentiated (Figure 1D for C0 = 0.2). For other coupling regimes established in the neuronal ensemble due to STDP, an increase of the excitation is accompanied by a simultaneous decrease of the inhibition among neurons (Figure 1D). This relation is reflected by the extent of synchronization in the neuronal population, such that the synaptic connectivity dominated by excitatory connections leads to strongly synchronized neurons. On the other hand, enhanced inhibitory interactions with suppressed excitatory connections lead to a weakly synchronized neuronal activity.
The discussed relation between the connectivity and collective dynamics of the neuronal ensembles with adaptive synapses opens an approach for the control of neuronal synchronization. The STDP-induced multistability plays an important role to this. Indeed, by an appropriate stimulation the neuronal ensemble can be shifted from abnormally synchronized regime to a weakly coupled and desynchronized regime, which stably coexist with each other. For this, the neurons have to be stimulated in such a way that they approach a weakly synchronized regime, i.e., the stimulated neurons get desynchronized. Since the synaptic connectivity is influenced by the neuronal activity via STDP, the desynchronizing neuronal firing will depress the excitatory synapses. Accordingly, a desynchronizing stimulation will also normalize the connectivity such that an anti-kindling process will be initiated: the stimulated neuronal population will be shifted from a strongly coupled and synchronized regime to a stable weakly coupled and desynchronized regime. The latter regime persists after stimulation cessation, which manifests the long-lasting effect of a desynchronizing stimulation in neuronal networks with STDP. Below we illustrate this approach for two stimulation techniques: CR neuromodulation and stimulation by independent noise.
3. Anti-Kindling by Coordinated Reset Neuromodulation
According to the CR neuromodulation algorithm (66, 67), the neuronal target population is stimulated via several stimulation sites, such that the entire population is divided into the same number of sub-populations, each of them receiving the stimulation mostly from one of the stimulation sites (Figure 2A). The stimulation signals for electrical CR stimulation are brief trains of high-frequency charge-balanced electrical pulses administered to the neuronal tissue via different stimulation sites (Figure 2B, upper plot). Electrical CR neuromodulation requires an electrode implantation into the neuronal target population or into fibers projecting onto a target population, as, for example, in the case of DBS (30). In fact, CR neuromodulation has initially been developed for the application to electrical DBS (66, 67). The stimulation signals can also have the form of post-synaptic potentials (Figure 2B, lower plot). The latter stimulation modality is realized when excitatory or inhibitory axons terminals or fibers are electrically stimulated, and the stimulation signals arrive at the neuronal target population as post-synaptic potentials evoked in the dendrites. Furthermore, such an indirect, synaptically mediated stimulation modality can model the impact of sensory, e.g., acoustic CR neuromodulation (29, 94).
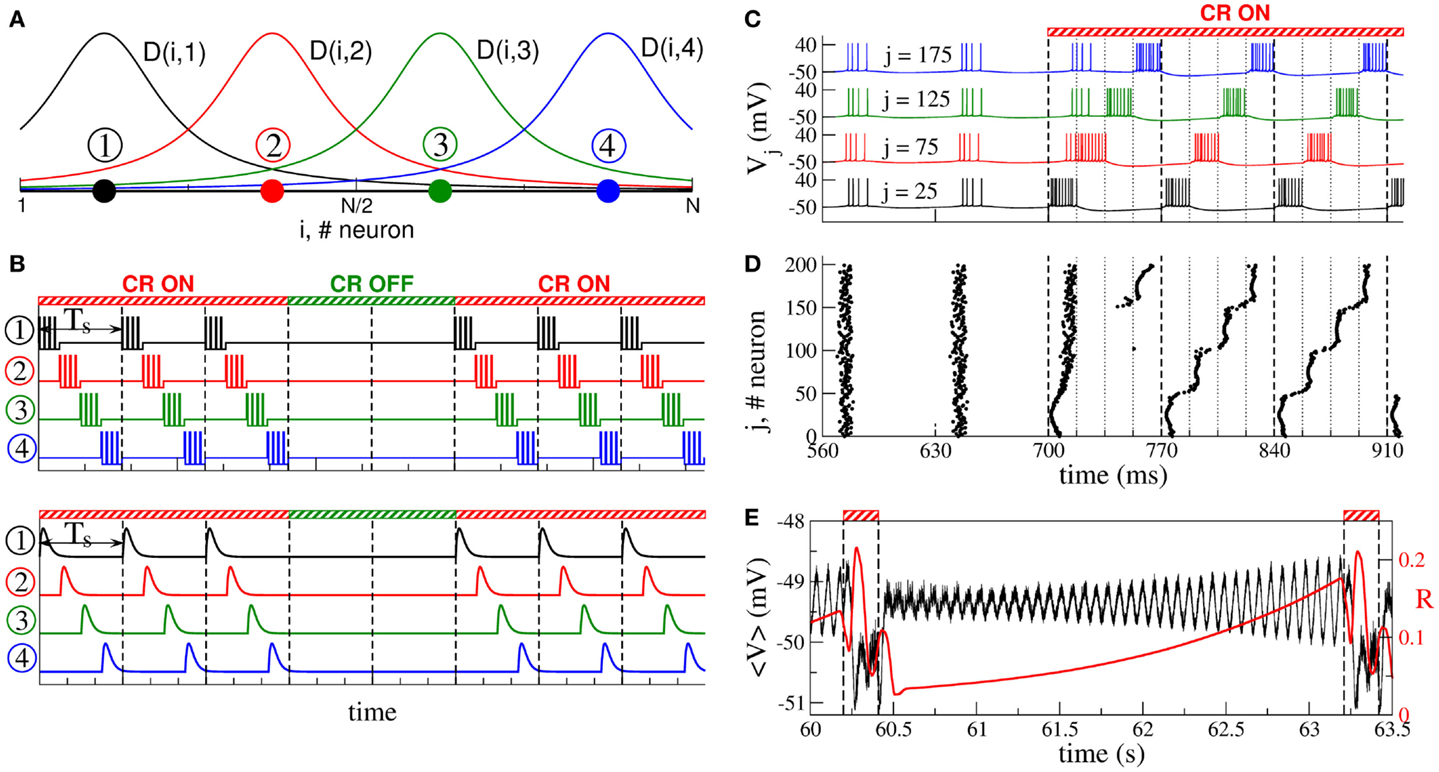
Figure 2. Stimulation setup of CR neuromodulation and its impact on synchronized neurons. (A) Schematic localization of four stimulation sites (filled circles) within the neuronal population and the corresponding spatial profiles (solid curves) of current decay in the neuronal tissue with the distance from the stimulation site. (B) Stimulation signals of electrical CR neuromodulation composed of short-pulse trains of charge-balanced pulses (upper plot), and stimulation signals of synaptically mediated, e.g., sensory CR neuromodulation in the form of post-synaptic potentials (lower plot). Vertical dashed lines separate the stimulation cycles of length Ts, where each stimulation site is activated once. (C) Time courses of the membrane potentials Vj of four neurons selected from the ensemble of coupled adaptive exponential integrate-and-fire (aEIF or AdEx) bursting neurons (117–119) without STDP stimulated by CR neuromodulation. The neurons are assigned to each of the four stimulation sites from plot (A) located at the same lattice coordinates (indices j are indicated in the plot). Stimulation begins at t = 700 ms and shifts the bursting of the neurons with respect to each other. The vertical dashed lines comprise the CR stimulation cycles of length Ts = 70 ms, and the dotted lines indicate the time intervals, where the corresponding stimulation site is active. (D) The corresponding raster plot of the burst onsets of all N = 200 neurons. (E) Time courses of the ensemble mean field of AdEx neurons (black curve) and the order parameter (120) (red curve, scale on the right vertical axis), where ψj is the phase of neuron j (121), between two successive ON epochs (indicated by dashed vertical lines and bars on the top of the plot) of 3 stimulation cycles. The plots are adapted from Ref. (122), where further details can be found.
During one stimulation cycle of length Ts, the stimulation sites are sequentially activated and deliver stimulation signals to different sub-populations of the neuronal target ensemble (Figures 2A,B). The stimulation signals are administered via stimulation sites in a time-coordinated manner, such that the next stimulation site is activated with a delay of Ts/M (M is the number of stimulation sites) after the activation of the preceding stimulation site. The stimulation period Ts is optimally chosen close to the mean period of the synchronized neurons. Within one cycle of length Ts each stimulation site is activated once. The stimulated neurons are sequentially reset by the corresponding stimulation signals such that, after a few stimulation cycles, the sub-populations assigned to different stimulation sites get phase shifted with respect to each other (Figures 2C,D). The total synchronization is thus replaced by a cluster state (66, 67, 122, 123). If the stimulation is then switched off, the neurons typically relax from the cluster state to a desynchronized state, which is characterized by low-amplitude oscillations of the mean field (Figure 2E), as discussed in Section 2. The stimulation-free neurons without STDP will eventually resynchronize if left unperturbed (Figure 2E). Hence, to maintain a desynchronized neuronal firing, CR stimuli have to be administered repetitively, where a few stimulation ON cycles are recurrently followed by a few stimulation OFF cycles (Figures 2B,E). In experimental and clinical studies, 3 ON cycles and 2 OFF cycles were used (Figure 2B) (29, 111, 112).
The direct electrical as well as the indirect, synaptically mediated CR neuromodulation can have a well-pronounced desynchronizing effect on the stimulated neuronal population. When administered to neuronal populations with STDP, CR neuromodulation does not only influence the collective dynamics but also the pattern of the synaptic couplings among the neurons, i.e., it may induce a rewiring of the stimulated neuronal ensemble: the control of the collective dynamics enables a control of the connectivity. Time courses of the mean synaptic weight C(t) before, during, and after direct electrical CR stimulation and excitatory synaptically mediated CR stimulation are shown in Figures 3A,B, respectively. Both stimulation modalities have very similar impact on strongly coupled and synchronized neurons. Weak stimulation only slightly perturbs the connectivity, and the neurons remain strongly coupled after stimulation offset (Figures 3A,B, blue curves). Optimal CR neuromodulation of intermediate intensity induces a long-lasting anti-kindling: synaptic connectivity is reshaped during the stimulation, and the stimulated neuronal population is shifted to a weakly coupled and, thus, desynchronized regime, which persists after stimulation is switched off (Figures 3A,B, green curves). Too strong CR neuromodulation, however, fails to induce such aftereffects, and only an acute (during stimulation) normalization of synaptic connectivity can be observed (Figures 3A,B, red curves).
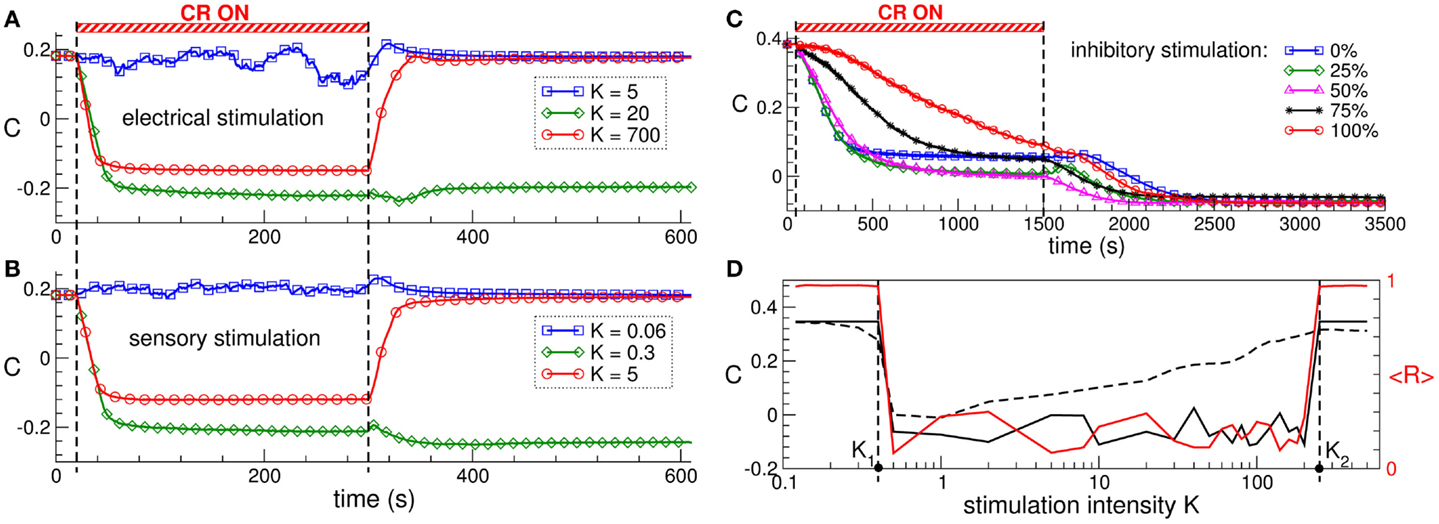
Figure 3. Stimulation-induced anti-kindling of neuronal ensembles with STDP by electrical and sensory CR neuromodulation. (A,B) Time courses of the mean synaptic weights C(t) (see Figure 1 for definition) of the ensemble of Hodgkin-Huxley neurons (113, 114) with STDP for stimulation intensities K indicated in the legends. The stimulation time interval is indicated by the red bar and by the vertical dashed lines for (A) direct electrical stimulation and (B) indirect, synaptically mediated, e.g., sensory excitatory stimulation administered to a strongly coupled and synchronized regime as in Figure 1 for C0 = 0.5. (C) Time courses of the mean synaptic weights C(t) of the ensemble of FitzHugh–Rinzel bursting neurons (124, 125) with STDP for different fractions of the neuronal population of randomly selected neurons receiving an inhibitory synaptically mediated CR stimulation as indicated in the legend. The rest of the neuronal ensemble receives an excitatory stimulation. The stimulation time interval is indicated by the red bar and by the vertical dashed lines. (D) Optimal range of the stimulation intensity for long-lasting effects of synaptically mediated inhibitory CR neuromodulation for FitzHugh–Rinzel bursting neurons with STDP. Dashed and solid black curves depict the mean synaptic weights Con and Coff registered at the ends of CR stimulation epoch and post-stimulation transient, respectively. The red solid curve shows the order parameter <R(t)> time averaged over the last 3 s of the post-stimulation transient (the scale on the right vertical axis). Vertical dashed lines indicate the parameter interval K∈(K1, K2), where the inhibitory CR stimulation is effective in inducing an anti-kindling. The plots are adapted from Ref. (95), where further details can be found.
Synaptically mediated CR neuromodulation is also effective in inducing anti-kindling if a fraction of neurons receives an inhibitory stimulation up to a fully inhibitory input (Figure 3C). Any of these stimulation modalities exhibits an optimal range of the stimulation intensity, where a long-lasting suppression of abnormal synaptic connectivity and neuronal synchronization takes place (Figure 3D). The emergence of the upper bound of the optimal parameter range is based on the non-linear mechanism of CR neuromodulation and indicates that CR neuromodulation is most effective for low stimulation intensities. This constitutes an essential difference to the standard HF DBS, where the stimulation strength has to be of sufficient strength in order to effectively suppress the PD symptoms, on the one hand, but the rise of adverse effects bounds its admissible range, on the other hand (17, 126–128). The efficacy of weak CR neuromodulation has been confirmed in a pre-clinical study of CR neuromodulation in the MPTP-treated parkinsonian monkeys (111). Sustained long-lasting aftereffects on motor function in MPTP monkeys can be induced by CR stimulation of the subthalamic nucleus (STN) at the stimulation intensity equal to a third of the stimulation strength necessary for an acute suppression of symptoms by the standard HF DBS. In contrast, delivering CR stimulation at a larger stimulation strength equal to that of HF DBS led to only weak and considerably shorter CR aftereffects (111).
The observed similarity of the stimulation efficacy in inducing anti-kindling by direct electrical CR stimulation and indirect, excitatory, or inhibitory synaptically mediated CR stimulation (Figure 3) provides a basis for a diversity of possible modalities for the control of pathological neuronal synchronization by CR neuromodulation. On the one hand, the local effects (realized by direct stimulation of the neurons’ somata or stimulation of afferent axon terminals) and the non-local effects (realized by stimulation of efferent fibers) of deep brain CR stimulation may be similar or even identical. This is in contrast to the stimulation effects of the standard HF DBS, where local and non-local effects may differ considerably (33). CR neuromodulation can thus normalize the neuronal activity not only in the directly stimulated neuronal population but also in the structures downstream, which might significantly extend the spectrum of possible targets structures for brain stimulation. On the other hand, a basic prerequisite for sensory CR stimulation is fulfilled, where CR stimulation is shown to be effective for stimulation with excitatory or inhibitory post-synaptic potentials, which models an indirect stimulation of the neuronal target population by sensory input. Hence, the DBS-oriented concept of CR-induced desynchronization (66, 67) and anti-kindling (90) might be extended to a more general CR neuromodulation concept, which can be realized by invasive as well as non-invasive, sensory (e.g., acoustic) stimulation. Sensory CR stimulation has been suggested and successfully applied for counteraction of tinnitus symptoms and normalization of the effective connectivity and functional patterns of activity in tinnitus patients (26, 27, 29, 94).
4. Self-Organized Noise Resistance
Synchronization in networks of coupled neurons can be destroyed by an independent random input, which is known to be a powerful method for counteracting synchronization in coupled oscillators without STDP (130). In neuronal networks with adaptive synapses, the situation becomes more complicated. In fact, a random noise stimulation administered to a population of strongly coupled and synchronized neurons with STDP may enhance the amount of synaptic coupling among the neurons (Figure 4A) (129). Stimulation-free neurons get strongly coupled, and a unidirectional (coupling only in one direction between two neurons) hierarchical coupling topology is established due to STDP for strong enough initial coupling (Figures 4A–C for K = 0) (129, 131, 132). If a random independent input is applied to the neuronal ensemble with STDP, the amount of coupling in the stimulated ensemble is significantly enhanced (Figure 4A). The random perturbations have a constructive effect on the dynamics of the synaptic weights, and the noise promotes the development of bidirectional (coupling in both directions between two neurons) synaptic connections among neurons (Figure 4C).
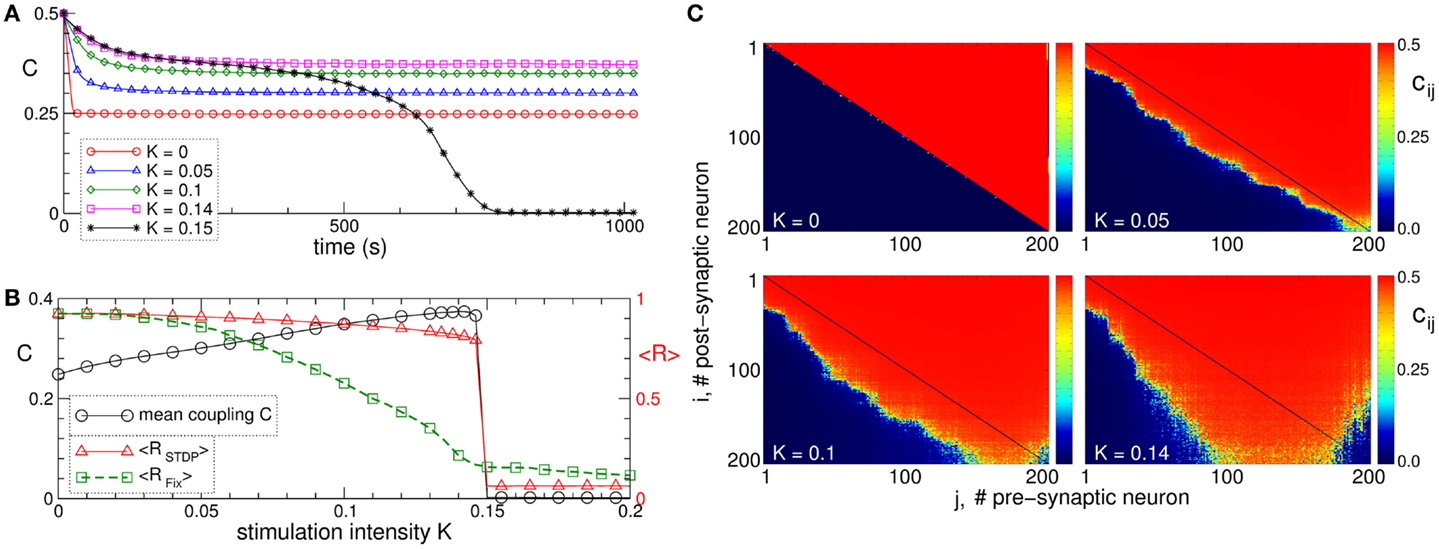
Figure 4. Constructive effect of independent random input on the synaptic weights and self-organized noise resistance of the ensemble of Hodgkin–Huxley neurons (113, 114) with STDP. (A) Time courses of the mean synaptic weight C(t) for different intensities of the random stimulation as indicated in the legend, where each neuron receives an independent random excitatory synaptic input. The initial synaptic weights cij(0) are randomly Gaussian distributed around the mean value C0 = 0.5 with SD 0.02. (B) Mean synaptic weight C(t) (scale on the left vertical axis) and the time-averaged order parameters <RSTDP> and <RFix> (scale on the right vertical axis) for the ensemble with and without STDP, respectively, versus input intensity K. For the network without STDP, the coupling matrix is fixed as for the stimulation-free case (see plot C for K = 0). (C) Coupling matrices established in the neuronal ensemble due to STDP for the input intensities indicated in the plots. For illustration, the neurons are sorted with respect to increasing natural spiking frequency. The plots are adapted from Ref. (129), where further details can be found.
The mean coupling increases as the input strength grows and demonstrates a resonance-like behavior, where there exists an optimal noise intensity, resulting in a maximal coupling among neurons (Figure 4B, black curve). The neurons counteract the desynchronizing effect of the noise by reorganizing their synaptic connectivity and increasing its strength, such that the amount of synchronization in the neural ensemble with STDP is relatively well preserved for moderately strong noise (Figure 4B, red curve). On the other hand, synchronization is strongly suppressed by the noise stimulation in the same neuronal network for fixed connectivity (Figure 4B, green curve). This mechanism constitutes a self-organized resistance to independent noise in the neuronal networks with STDP, where the latter preserves the existing level of neural synchrony by a homeostatic regulation of the synaptic connectivity in response to external noise perturbations. Therefore, an independent noise can by no means be considered as an effective method for desynchronization of oscillatory neural networks with STDP. Also, from the clinical standpoint these results may be important. In fact, they may contribute to a deeper understanding of why maskers and noisers show limited efficacy in counteracting tinnitus (133), the latter being associated with abnormal neural synchrony (10, 29).
5. Discussion
Computational, pre-clinical, and clinical proof-of-concept studies revealed that CR neuromodulation is effective in inducing a long-lasting desynchronization for a number of stimulation setups and demonstrates a great applicability (26, 27, 29, 53, 90, 91, 94, 95, 110–112). In particular, the computationally revealed fact that CR stimulation is effective, no matter whether it is directly delivered to the neurons’ somata or indirectly, via excitatory and/or inhibitory synapses (94, 95) may turn out to be crucial for several reasons:
• The effects of CR DBS might be considerably more robust with respect to variations of target structures as opposed to HF DBS. As discussed above, the effects of HF DBS strongly depend on the target.
• CR-induced desynchronization and anti-kindling might be achieved by means of a variety of invasive as well as non-invasive stimulation modalities, e.g., by DBS (111, 112), by acoustic (26, 27, 29), and by other types of sensory stimulation as well as by spinal cord stimulation (134, 135), e.g., to counteract abnormal neuronal synchrony underlying neurogenic pain (136–138).
CR neuromodulation has also been suggested to counteract cerebral hypo-activity found in a number of diseases including Alzheimer’s disease, schizophrenia, major depression, and bipolar affective disorders (139–143). A simple periodic stimulation may activate the stimulated neurons, but can also induce an undesirable enhancement of neuronal synchronization, significantly deteriorate symptoms, and even lead to kindling processes and evoke epileptiform and other types of abnormal activity (18, 20, 47, 89, 110). As computationally shown, CR neuromodulation can specifically counteract neuronal hypo-activity in a safe manner without promoting pathological synchronization by a multi-frequency and phase-shifted activation of the stimulated neuronal networks (144).
In the open-loop stimulation protocol of CR neuromodulation discussed above, the repetitive stimulus administration, after initial parameter calibration, is organized regardless of the state of the stimulated ensemble. CR neuromodulation can also be utilized with closed-loop stimulation protocol in a demand-controlled way, where parameters and timing of the stimulation can be adapted to the ongoing neuronal activity (66, 67). Here, two strategies can, for instance, be suggested (i) demand-controlled timing of the administration of identical stimuli, where the same stimulus is administered whenever the population tends to resynchronize, or (ii) demand-controlled length of administered pulse trains, where the length of the pulse trains is longer for stronger synchronization measured at the onset of the stimulation, see in Ref. (66, 67) for details. Note, closed-loop stimulation is naturally realized by feedback methods (68–70, 72–74, 76), which can be considered as further candidates for control of abnormal neuronal synchronization.
The closed-loop approach has already been tested for standard HF DBS under acute conditions in MPTP-monkeys (145) and PD patients (146). To this end, a short train (comprising 7 pulses at 130 Hz) was delivered through a pair of electrodes located in the globus pallidum interior (GPi) at a predetermined, fixed latency (80 ms) following each action potential recorded through an electrode placed in the primary motor cortex (M1) (145). This type of stimulation caused a stronger decrease of the firing rate of pallidal neurons together with a more pronounced decrease of the oscillatory neuronal activity along with a better amelioration of the MPTP-induced akinesia as compared to the standard continuous 130 Hz DBS. After cessation of this type of closed-loop DBS the initial firing pattern reverted back to the pre-stimulus levels (145). Another study (146) confirmed the efficacy of the closed-loop adaptive DBS (aDBS) in PD patients, where the onsets and offsets of HF stimulation were triggered by a threshold crossing by LFP in the β-band measured via the same stimulation electrode implanted in STN. For the same stimulation intensity and stimulation frequency (130 Hz), the aDBS can be about 30% more effective than standard continuous HF DBS, while <50% of the total electrical energy is delivered in the aDBS mode as compared to continuous HF DBS. Moreover, despite of the used fixed beta threshold, the triggered stimulation duration (per 10-s block) progressively drops over time during stimulation in the aDBS mode, which suggests that aDBS might lead to positive adaptive effects in pathological parkinsonian networks (146).
We have included computational data obtained in neuronal networks with STDP stimulated by independent noise for two reasons. First, these findings demonstrate how important it is to include STDP in neuronal networks in order to design and optimize desynchronizing stimulation techniques. Second, these findings illustrate how important the choice of the right stimulation technique is. Stimulation techniques that might intuitively be expected to have beneficial effects may actually have surprisingly counter-productive effects.
In several computational studies, parameters of CR neuromodulation have been optimized in model neuronal networks of different complexity with and without STDP (66, 67, 90, 95, 122, 123, 147). In particular, the impact of stimulation strength, number of stimulation sites, and stimulation timing was investigated in detail and related to the properties of the stimulated neuronal tissue. The obtained theoretical and experimental results indicate the robustness and broad applicability of CR neuromodulation, which may finally become a novel concept for the treatment of neurological disorders characterized by abnormal neuronal synchronization.
Conflict of Interest Statement
Dr. Peter A. Tass had a contractual relationship with ANM Adaptive Neuromodulation GmbH. No financial interests exist related to the presented results. Dr. Oleksandr V. Popovych declares that the research was conducted in the absence of any commercial or financial relationships that could be construed as a potential conflict of interest.
Authors Biography
Peter A. Tass develops stimulation techniques with computational methods. He studied medicine (MD, University of Ulm and Heidelberg, Germany), physics (Ph.D., University of Stuttgart, Germany), mathematics (diploma, University of Stuttgart, Germany), and made a Habilitation in physiology (RWTH Aachen Univ., Germany). He directs the Institute of Neuroscience and Medicine – Neuromodulation at Juelich Research Center, Juelich, Germany and is a full professor in neuromodulation at Cologne University, Germany. Furthermore, Peter A. Tass is consulting professor at the Department of Neurosurgery, Stanford University, Stanford, CA, USA.
References
1. Lenz FA, Kwan HC, Martin RL, Tasker RR, Dostrovsky JO, Lenz YE. Single-unit analysis of the human ventral thalamic nuclear group – tremor-related activity in functionally identified cells. Brain (1994) 117:531–43. doi: 10.1093/brain/117.3.531
Pubmed Abstract | Pubmed Full Text | CrossRef Full Text | Google Scholar
2. Nini A, Feingold A, Slovin H, Bergmann H. Neurons in the globus pallidus do not show correlated activity in the normal monkey, but phase-locked oscillations appear in the MPTP model of parkinsonism. J Neurophysiol (1995) 74:1800–5.
3. Mormann F, Lehnertz K, David P, Elger CE. Mean phase coherence as a measure for phase synchronization and its application to the EEG of epilepsy patients. Physica D (2000) 144:358–69. doi:10.1016/j.clinph.2013.09.047
4. da Silva FHL, Blanes W, Kalitzin SN, Parra J, Suffczynski P, Velis DN. Dynamical diseases of brain systems: different routes to epileptic seizures. IEEE Trans Biomed Eng (2003) 50:540–8. doi:10.1109/TBME.2003.810703
Pubmed Abstract | Pubmed Full Text | CrossRef Full Text | Google Scholar
5. Weisz N, Moratti S, Meinzer M, Dohrmann K, Elbert T. Tinnitus perception and distress is related to abnormal spontaneous brain activity as measured by magnetoencephalography. PLoS Med (2005) 2(6):e153. doi:10.1371/journal.pmed.0020153
Pubmed Abstract | Pubmed Full Text | CrossRef Full Text | Google Scholar
6. Weisz N, Muller S, Schlee W, Dohrmann K, Hartmann T, Elbert T. The neural code of auditory phantom perception. J Neurosci (2007) 27:1479–84. doi:10.1523/JNEUROSCI.3711-06.2007
Pubmed Abstract | Pubmed Full Text | CrossRef Full Text | Google Scholar
7. Hammond C, Bergman H, Brown P. Pathological synchronization in Parkinson’s disease: networks, models and treatments. Trends Neurosci (2007) 30:357–64. doi:10.1016/j.tins.2007.05.004
Pubmed Abstract | Pubmed Full Text | CrossRef Full Text | Google Scholar
8. Kane A, Hutchison WD, Hodaie M, Lozano AM, Dostrovsky JO. Enhanced synchronization of thalamic theta band local field potentials in patients with essential tremor. Exp Neurol (2009) 217:171–6. doi:10.1016/j.expneurol.2009.02.005
Pubmed Abstract | Pubmed Full Text | CrossRef Full Text | Google Scholar
9. Schnitzler A, Munks C, Butz M, Timmermann L, Gross J. Synchronized brain network associated with essential tremor as revealed by magnetoencephalography. Mov Disord (2009) 24:1629–35. doi:10.1002/mds.22633
Pubmed Abstract | Pubmed Full Text | CrossRef Full Text | Google Scholar
10. Roberts LE, Eggermont JJ, Caspary DM, Shore SE, Melcher JR, Kaltenbach JA. Ringing ears: the neuroscience of tinnitus. J Neurosci (2010) 30:14972–9. doi:10.1523/JNEUROSCI.4028-10.2010
Pubmed Abstract | Pubmed Full Text | CrossRef Full Text | Google Scholar
11. Levy R, Hutchison WD, Lozano AM, Dostrovsky JO. High-frequency synchronization of neuronal activity in the subthalamic nucleus of parkinsonian patients with limb tremor. J Neurosci (2000) 20:7766–75.
12. Levy R, Ashby P, Hutchison WD, Lang AE, Lozano AM, Dostrovsky JO. Dependence of subthalamic nucleus oscillations on movement and dopamine in Parkinson’s disease. Brain (2002) 125:1196–209. doi:10.1093/brain/awf128
Pubmed Abstract | Pubmed Full Text | CrossRef Full Text | Google Scholar
13. Kühn AA, Kupsch A, Schneider GH, Brown P. Reduction in subthalamic 8-35 hz oscillatory activity correlates with clinical improvement in Parkinson’s disease. Eur J Neurosci (2006) 23:1956–60. doi:10.1111/j.1460-9568.2006.04717.x
Pubmed Abstract | Pubmed Full Text | CrossRef Full Text | Google Scholar
14. Kühn AA, Tsui A, Aziz T, Ray N, Brücke C, Kupsch A, et al. Pathological synchronisation in the subthalamic nucleus of patients with Parkinson’s disease relates to both bradykinesia and rigidity. Exp Neurol (2009) 215:380–7. doi:10.1016/j.expneurol.2008.11.008
Pubmed Abstract | Pubmed Full Text | CrossRef Full Text | Google Scholar
15. Jenkinson N, Brown P. New insights into the relationship between dopamine, beta oscillations and motor function. Trends Neurosci (2011) 34:611–8. doi:10.1016/j.tins.2011.09.003
Pubmed Abstract | Pubmed Full Text | CrossRef Full Text | Google Scholar
16. Wilson CL, Puntis M, Lacey MG. Overwhelmingly asynchronous firing of rat subthalamic nucleus neurones in brain slices provides little evidence for intrinsic interconnectivity. Neuroscience (2004) 123:187–200. doi:10.1016/j.neuroscience.2003.09.008
Pubmed Abstract | Pubmed Full Text | CrossRef Full Text | Google Scholar
17. Moro E, Esselink RJA, Xie J, Hommel M, Benabid AL, Pollak P. The impact on Parkinson’s disease of electrical parameter settings in STN stimulation. Neurology (2002) 59:706–13. doi:10.1212/WNL.59.5.706
Pubmed Abstract | Pubmed Full Text | CrossRef Full Text | Google Scholar
18. Timmermann L, Wojtecki L, Gross J, Lehrke R, Voges J, Maarouf M, et al. Ten-Hertz stimulation of subthalamic nucleus deteriorates motor symptoms in Parkinson’s disease. Mov Disord (2004) 19:1328–33. doi:10.1002/mds.20198
Pubmed Abstract | Pubmed Full Text | CrossRef Full Text | Google Scholar
19. Eusebio A, Chen CC, Lu CS, Lee ST, Tsai CH, Limousin P, et al. Effects of low-frequency stimulation of the subthalamic nucleus on movement in Parkinson’s disease. Exp Neurol (2008) 209:125–30. doi:10.1016/j.expneurol.2007.09.007
Pubmed Abstract | Pubmed Full Text | CrossRef Full Text | Google Scholar
20. Barnikol UB, Popovych OV, Hauptmann C, Sturm V, Freund HJ, Tass PA. Tremor entrainment by patterned low-frequency stimulation. Phil Trans R Soc A (2008) 366:3545–73. doi:10.1098/rsta.2008.0104
Pubmed Abstract | Pubmed Full Text | CrossRef Full Text | Google Scholar
21. Gradinaru V, Mogri M, Thompson KR, Henderson JM, Deisseroth K. Optical deconstruction of parkinsonian neural circuitry. Science (2009) 324:354–9. doi:10.1126/science.1167093
Pubmed Abstract | Pubmed Full Text | CrossRef Full Text | Google Scholar
22. Chen CC, Lin WY, Chan HL, Hsu YT, Tu PH, Lee ST, et al. Stimulation of the subthalamic region at 20 hz slows the development of grip force in Parkinson’s disease. Exp Neurol (2011) 231:91–6. doi:10.1016/j.expneurol.2011.05.018
Pubmed Abstract | Pubmed Full Text | CrossRef Full Text | Google Scholar
23. Seki S, Eggermont JJ. Changes in spontaneous firing rate and neural synchrony in cat primary auditory cortex after localized tone-induced hearing loss. Hear Res (2003) 180:28–38. doi:10.1016/S0378-5955(03)00074-1
Pubmed Abstract | Pubmed Full Text | CrossRef Full Text | Google Scholar
24. Eggermont JJ. Correlated neural activity as the driving force for functional changes in auditory cortex. Hear Res (2007) 229:69–80. doi:10.1016/j.heares.2007.01.008
Pubmed Abstract | Pubmed Full Text | CrossRef Full Text | Google Scholar
25. Dohrmann K, Elbert T, Schlee W, Weisz N. Tuning the tinnitus percept by modification of synchronous brain activity. Restor Neurol Neurosci (2007) 25:371–8.
26. Silchenko AN, Adamchic I, Hauptmann C, Tass PA. Impact of acoustic coordinated reset neuromodulation on effective connectivity in a neural network of phantom sound. Neuroimage (2013) 77:133–47. doi:10.1016/j.neuroimage.2013.03.013
Pubmed Abstract | Pubmed Full Text | CrossRef Full Text | Google Scholar
27. Adamchic I, Toth T, Hauptmann C, Tass PA. Reversing pathologically increased EEG power by acoustic coordinated reset neuromodulation. Hum Brain Mapp (2014) 35:2099–118. doi:10.1002/hbm.22314
Pubmed Abstract | Pubmed Full Text | CrossRef Full Text | Google Scholar
28. Lorenz I, Muller N, Schlee W, Hartmann T, Weisz N. Loss of alpha power is related to increased gamma synchronization – a marker of reduced inhibition in tinnitus? Neurosci Lett (2009) 453:225–8. doi:10.1016/j.neulet.2009.02.028
Pubmed Abstract | Pubmed Full Text | CrossRef Full Text | Google Scholar
29. Tass PA, Adamchic I, Freund HJ, von Stackelberg T, Hauptmann C. Counteracting tinnitus by acoustic coordinated reset neuromodulation. Restor Neurol Neurosci (2012) 30:137–59. doi:10.3233/RNN-2012-110218
Pubmed Abstract | Pubmed Full Text | CrossRef Full Text | Google Scholar
30. Benabid AL, Pollak P, Gervason C, Hoffmann D, Gao DM, Hommel M, et al. Longterm suppression of tremor by chronic stimulation of ventral intermediate thalamic nucleus. Lancet (1991) 337:403–6. doi:10.1016/0140-6736(91)91175-T
Pubmed Abstract | Pubmed Full Text | CrossRef Full Text | Google Scholar
31. Kringelbach ML, Jenkinson N, Owen SLF, Aziz TZ. Translational principles of deep brain stimulation. Nat Rev Neurosci (2007) 8:623–35. doi:10.1038/nrn2196
Pubmed Abstract | Pubmed Full Text | CrossRef Full Text | Google Scholar
32. Wu C, Sharan AD. Neurostimulation for the treatment of epilepsy: a review of current surgical interventions. Neuromodulation (2013) 16:10–24. doi:10.1111/j.1525-1403.2012.00501.x
Pubmed Abstract | Pubmed Full Text | CrossRef Full Text | Google Scholar
33. McIntyre CC, Grill WM, Sherman DL, Thakor NV. Cellular effects of deep brain stimulation: model-based analysis of activation and inhibition. J Neurophysiol (2004) 91:1457–69. doi:10.1152/jn.00989.2003
Pubmed Abstract | Pubmed Full Text | CrossRef Full Text | Google Scholar
34. Benabid AL, Benazzous A, Pollak P. Mechanisms of deep brain stimulation. Mov Disord (2002) 17:S73–4. doi:10.1002/mds.10145
35. Welter ML, Houeto JL, Bonnet AM, Bejjani PB, Mesnage V, Dormont D, et al. Effects of high-frequency stimulation on subthalamic neuronal activity in parkinsonian patients. Arch Neurol (2004) 61:89–96. doi:10.1001/archneur.61.1.89
Pubmed Abstract | Pubmed Full Text | CrossRef Full Text | Google Scholar
36. Meissner W, Leblois A, Hansel D, Bioulac B, Gross CE, Benazzouz A, et al. Subthalamic high frequency stimulation resets subthalamic firing and reduces abnormal oscillations. Brain (2005) 128:2372–82. doi:10.1093/brain/awh616
Pubmed Abstract | Pubmed Full Text | CrossRef Full Text | Google Scholar
37. Dostrovsky JO, Levy R, Wu JP, Hutchison WD, Tasker RR, Lozano AM. Microstimulation-induced inhibition of neuronal firing in human globus pallidus. J Neurophysiol (2000) 84:570–4.
38. Benazzouz A, Gao DM, Ni ZG, Piallat B, Bouali-Benazzouz R, Benabid AL. Effect of high-frequency stimulation of the subthalamic nucleus on the neuronal activities of the substantia nigra pars reticulata and ventrolateral nucleus of the thalamus in the rat. Neuroscience (2000) 99:289–95. doi:10.1016/S0306-4522(00)00199-8
Pubmed Abstract | Pubmed Full Text | CrossRef Full Text | Google Scholar
39. Beurrier C, Bioulac B, Audin J, Hammond C. High-frequency stimulation produces a transient blockade of voltage-gated currents in subthalamic neurons. J Neurophysiol (2001) 85:1351–6.
40. Hammond C, Ammari R, Bioulac B, Garcia L. Latest view on the mechanism of action of deep brain stimulation. Mov Disord (2008) 23:2111–21. doi:10.1002/mds.22120
Pubmed Abstract | Pubmed Full Text | CrossRef Full Text | Google Scholar
41. Hashimoto T, Elder CM, Okun MS, Patrick SK, Vitek JL. Stimulation of the subthalamic nucleus changes the firing pattern of pallidal neurons. J Neurosci (2003) 23:1916–23.
42. Rubin JE, Terman D. High frequency stimulation of the subthalamic nucleus eliminates pathological thalamic rhythmicity in a computational model. J Comput Neurosci (2004) 16:211–35. doi:10.1023/B:JCNS.0000025686.47117.67
Pubmed Abstract | Pubmed Full Text | CrossRef Full Text | Google Scholar
43. Miocinovic S, Parent M, Butson CR, Hahn PJ, Russo GS, Vitek JL, et al. Computational analysis of subthalamic nucleus and lenticular fasciculus activation during therapeutic deep brain stimulation. J Neurophysiol (2006) 96:1569–80. doi:10.1152/jn.00305.2006
Pubmed Abstract | Pubmed Full Text | CrossRef Full Text | Google Scholar
44. Anderson ME, Postupna N, Ruffo M. Effects of high-frequency stimulation in the internal globus pallidus on the activity of thalamic neurons in the awake monkey. J Neurophysiol (2003) 89:1150–60. doi:10.1152/jn.00475.2002
Pubmed Abstract | Pubmed Full Text | CrossRef Full Text | Google Scholar
45. McIntyre CC, Savasta M, Goff LKL, Vitek JL. Uncovering the mechanism(s) of action of deep brain stimulation: activation, inhibition, or both. Clin Neurophysiol (2004) 115:1239–48. doi:10.1016/j.clinph.2003.12.024
Pubmed Abstract | Pubmed Full Text | CrossRef Full Text | Google Scholar
46. Benabid AL, Wallace B, Mitrofanis J, Xia R, Piallat B, Chabardes S, et al. A putative generalized model of the effects and mechanism of action of high frequency electrical stimulation of the central nervous system. Acta Neurol Belg (2005) 105:149–57.
47. Brown P, Mazzone P, Oliviero A, Altibrandi MG, Pilato F, Tonali PA, et al. Effects of stimulation of the subthalamic area on oscillatory pallidal activity in Parkinson’s disease. Exp Neurol (2004) 188:480–90. doi:10.1016/j.expneurol.2004.05.009
Pubmed Abstract | Pubmed Full Text | CrossRef Full Text | Google Scholar
48. Kühn AA, Kempf F, Brücke C, Doyle LG, Martinez-Torres I, Pogosyan A, et al. High-frequency stimulation of the subthalamic nucleus suppresses β oscillatory activity in patients with Parkinson’s disease in parallel with improvement in motor performance. J Neurosci (2008) 28(24):6165–73. doi:10.1523/JNEUROSCI.0282-08.2008
Pubmed Abstract | Pubmed Full Text | CrossRef Full Text | Google Scholar
49. Bronte-Stewart H, Barberini C, Koop MM, Hill BC, Henderson JM, Wingeier B. The STN beta-band profile in Parkinson’s disease is stationary and shows prolonged attenuation after deep brain stimulation. Exp Neurol (2009) 215:20–8. doi:10.1016/j.expneurol.2008.09.008
Pubmed Abstract | Pubmed Full Text | CrossRef Full Text | Google Scholar
50. Eusebio A, Thevathasan W, Gaynor LD, Pogosyan A, Bye E, Foltynie T, et al. Deep brain stimulation can suppress pathological synchronisation in parkinsonian patients. J Neurol Neurosurg Psychiatry (2011) 82:569–73. doi:10.1136/jnnp.2010.217489
Pubmed Abstract | Pubmed Full Text | CrossRef Full Text | Google Scholar
51. Wilson CJ, Beverlin B II, Netoff T. Chaotic desynchronization as the therapeutic mechanism of deep brain stimulation. Front Syst Neurosci (2011) 5:50. doi:10.3389/fnsys.2011.00050
52. Popovych OV, Tass PA. Macroscopic entrainment of periodically forced oscillatory ensembles. Prog Biophys Mol Biol (2011) 105:98–108. doi:10.1016/j.pbiomolbio.2010.09.018
Pubmed Abstract | Pubmed Full Text | CrossRef Full Text | Google Scholar
53. Hauptmann C, Tass PA. Therapeutic rewiring by means of desynchronizing brain stimulation. Biosystems (2007) 89:173–81. doi:10.1016/j.biosystems.2006.04.015
Pubmed Abstract | Pubmed Full Text | CrossRef Full Text | Google Scholar
54. Foffani G, Ardolino G, Egidi M, Caputo E, Bossi B, Priori A. Subthalamic oscillatory activities at beta or higher frequency do not change after high-frequency DBS in Parkinson’s disease. Brain Res Bull (2006) 69:123–30. doi:10.1016/j.brainresbull.2005.11.012
Pubmed Abstract | Pubmed Full Text | CrossRef Full Text | Google Scholar
55. Priori A, Ardolino G, Marceglia S, Mrakic-Sposta S, Locatelli M, Tamma F, et al. Low-frequency subthalamic oscillations increase after deep brain stimulation in Parkinson’s disease. Brain Res Bull (2006) 71:149–54. doi:10.1016/j.brainresbull.2006.08.015
Pubmed Abstract | Pubmed Full Text | CrossRef Full Text | Google Scholar
56. Temperli P, Ghika J, Villemure JG, Burkhard P, Bogousslavsky J, Vingerhoets F. How do parkinsonian signs return after discontinuation of subthalamic DBS? Neurology (2003) 60:78–81. doi:10.1212/WNL.60.1.78
Pubmed Abstract | Pubmed Full Text | CrossRef Full Text | Google Scholar
57. Welter ML, Schüpbach M, Czernecki V, Karachi C, Fernandez-Vidal S, Golmard JL, et al. Optimal target localization for subthalamic stimulation in patients with Parkinson disease. Neurology (2014) 82:1352–61. doi:10.1212/WNL.0000000000000315
Pubmed Abstract | Pubmed Full Text | CrossRef Full Text | Google Scholar
58. Pyragas K, Novicenko V, Tass PA. Mechanism of suppression of sustained neuronal spiking under high-frequency stimulation. Biol Cybern (2013) 107:669–84. doi:10.1007/s00422-013-0567-1
Pubmed Abstract | Pubmed Full Text | CrossRef Full Text | Google Scholar
59. Tasker RR. Depp brain stimulation is preferable to thalamotomy for tremor suppression. Surg Neurol (1998) 49:145–54. doi:10.1016/S0090-3019(97)00459-X
Pubmed Abstract | Pubmed Full Text | CrossRef Full Text | Google Scholar
60. Volkmann J. Deep brain stimulation for the treatment of Parkinson’s disease. J Clin Neurophysiol (2004) 21:6–17. doi:10.1097/00004691-200401000-00003
Pubmed Abstract | Pubmed Full Text | CrossRef Full Text | Google Scholar
61. Rodriguez-Oroz MC, Obeso JA, Lang AE, Houeto JL, Pollak P, Rehncrona S, et al. Bilateral deep brain stimulation in Parkinson’s disease: a multicentre study with 4 years follow-up. Brain (2005) 128:2240–9. doi:10.1093/brain/awh571
Pubmed Abstract | Pubmed Full Text | CrossRef Full Text | Google Scholar
62. Deuschl G, Schade-Brittinger C, Krack P, Volkmann J, Schäfer H, Bötzel K, et al. A randomized trial of deep-brain stimulation for Parkinson’s disease. N Engl J Med (2006) 355:896–908. doi:10.1056/NEJMoa060281
Pubmed Abstract | Pubmed Full Text | CrossRef Full Text | Google Scholar
63. Tass PA. Phase Resetting in Medicine and Biology: Stochastic Modelling and Data Analysis. Berlin: Springer (1999).
64. Tass PA. Desynchronizing double-pulse phase resetting and application to deep brain stimulation. Biol Cybern (2001) 85:343–54. doi:10.1007/s004220100268
Pubmed Abstract | Pubmed Full Text | CrossRef Full Text | Google Scholar
65. Tass PA. Desynchronization of brain rhythms with soft phase-resetting techniques. Biol Cybern (2002) 87:102–15. doi:10.1007/s00422-002-0322-5
Pubmed Abstract | Pubmed Full Text | CrossRef Full Text | Google Scholar
66. Tass PA. A model of desynchronizing deep brain stimulation with a demand-controlled coordinated reset of neural subpopulations. Biol Cybern (2003) 89:81–8. doi:10.1007/s00422-003-0425-7
Pubmed Abstract | Pubmed Full Text | CrossRef Full Text | Google Scholar
67. Tass PA. Desynchronization by means of a coordinated reset of neural sub-populations – a novel technique for demand-controlled deep brain stimulation. Prog Theor Phys Suppl (2003) 150:281–96. doi:10.1143/PTPS.150.281
68. Rosenblum MG, Pikovsky AS. Controlling synchronization in an ensemble of globally coupled oscillators. Phys Rev Lett (2004) 92:114102. doi:10.1103/PhysRevLett.92.114102
69. Hauptmann C, Popovych O, Tass PA. Effectively desynchronizing deep brain stimulation based on a coordinated delayed feedback stimulation via several sites: a computational study. Biol Cybern (2005) 93:463–70. doi:10.1007/s00422-005-0020-1
Pubmed Abstract | Pubmed Full Text | CrossRef Full Text | Google Scholar
70. Popovych OV, Hauptmann C, Tass PA. Effective desynchronization by nonlinear delayed feedback. Phys Rev Lett (2005) 94:164102. doi:10.1103/PhysRevLett.94.164102
71. Kiss IZ, Rusin CG, Kori H, Hudson JL. Engineering complex dynamical structures: sequential patterns and desynchronization. Science (2007) 316:1886–9. doi:10.1126/science.1140858
Pubmed Abstract | Pubmed Full Text | CrossRef Full Text | Google Scholar
72. Pyragas K, Popovych OV, Tass PA. Controlling synchrony in oscillatory networks with a separate stimulation-registration setup. Europhys Lett (2007) 80:40002. doi:10.1209/0295-5075/80/40002
73. Tukhlina N, Rosenblum M, Pikovsky A, Kurths J. Feedback suppression of neural synchrony by vanishing stimulation. Phys Rev E Stat Nonlin Soft Matter Phys (2007) 75:011918. doi:10.1103/PhysRevE.75.011918
74. Luo M, Wu YJ, Peng JH. Washout filter aided mean field feedback desynchronization in an ensemble of globally coupled neural oscillators. Biol Cybern (2009) 101:241–6. doi:10.1007/s00422-009-0334-5
Pubmed Abstract | Pubmed Full Text | CrossRef Full Text | Google Scholar
75. Danzl P, Hespanha J, Moehlis J. Event-based minimum-time control of oscillatory neuron models. Biol Cybern (2009) 101:387–99. doi:10.1007/s00422-009-0344-3
Pubmed Abstract | Pubmed Full Text | CrossRef Full Text | Google Scholar
76. Popovych OV, Tass PA. Synchronization control of interacting oscillatory ensembles by mixed nonlinear delayed feedback. Phys Rev E Stat Nonlin Soft Matter Phys (2010) 82:026204. doi:10.1103/PhysRevE.82.026204
77. Nabi A, Moehlis J. Single input optimal control for globally coupled neuron networks. J Neural Eng (2011) 8:065008. doi:10.1088/1741-2560/8/6/065008
78. Hebb D. The Organization of Behavior: A Neuropsychological Theory. Wiley Book in Clinical Psychology (New York: Wiley) (1949).
79. Gerstner W, Kempter R, van Hemmen JL, Wagner H. A neuronal learning rule for sub-millisecond temporal coding. Nature (1996) 383:76–8. doi:10.1038/383076a0
Pubmed Abstract | Pubmed Full Text | CrossRef Full Text | Google Scholar
80. Markram H, Lübke J, Frotscher M, Sakmann B. Regulation of synaptic efficacy by coincidence of postsynaptic APs and EPSPs. Science (1997) 275:213–5. doi:10.1126/science.275.5297.213
Pubmed Abstract | Pubmed Full Text | CrossRef Full Text | Google Scholar
81. Bi GQ, Poo MM. Synaptic modications in cultured hippocampal neurons: dependence on spike timing, synaptic strength, and postsynaptic cell type. J Neurosci (1998) 18:10464–72.
82. Feldman DE. Timing-based LTP and LTD at vertical inputs to layer II/III pyramidal cells in rat barrel cortex. Neuron (2000) 27:45–56. doi:10.1016/S0896-6273(00)00008-8
Pubmed Abstract | Pubmed Full Text | CrossRef Full Text | Google Scholar
83. Bi GQ. Spatiotemporal specificity of synaptic plasticity: cellular rules and mechanisms. Biol Cybern (2002) 87:319–32. doi:10.1007/s00422-002-0349-7
Pubmed Abstract | Pubmed Full Text | CrossRef Full Text | Google Scholar
84. Wittenberg GM, Wang SSH. Malleability of spike-timing-dependent plasticity at the CA3-CA1 synapse. J Neurosci (2006) 26:6610–7. doi:10.1523/JNEUROSCI.5388-05.2006
Pubmed Abstract | Pubmed Full Text | CrossRef Full Text | Google Scholar
85. Caporale N, Dan Y. Spike timing-dependent plasticity: a Hebbian learning rule. Annu Rev Neurosci (2008) 31:25–46. doi:10.1146/annurev.neuro.31.060407.125639
Pubmed Abstract | Pubmed Full Text | CrossRef Full Text | Google Scholar
86. Nowotny T, Zhigulin VP, Selverston AI, Abarbanel HDI, Rabinovich MI. Enhancement of synchronization in a hybrid neural circuit by spike-timing dependent plasticity. J Neurosci (2003) 23:9776–85.
87. Goddar G. Development of epileptic seizures through brain stimulation at low intensity. Nature (1967) 214:1020–1. doi:10.1038/2141020a0
Pubmed Abstract | Pubmed Full Text | CrossRef Full Text | Google Scholar
88. Speckmann E, Elger C. The neurophysiological basis of epileptic activity: a condensed overview. Epilepsy Res Suppl (1991) 2:1–7.
89. Morimoto K, Fahnestock M, Racine RJ. Kindling and status epilepticus models of epilepsy: rewiring the brain. Prog Neurobiol (2004) 73:1–60. doi:10.1016/j.pneurobio.2004.03.009
Pubmed Abstract | Pubmed Full Text | CrossRef Full Text | Google Scholar
90. Tass PA, Majtanik M. Long-term anti-kindling effects of desynchronizing brain stimulation: a theoretical study. Biol Cybern (2006) 94:58–66. doi:10.1007/s00422-005-0028-6
Pubmed Abstract | Pubmed Full Text | CrossRef Full Text | Google Scholar
91. Tass PA, Hauptmann C. Therapeutic modulation of synaptic connectivity with desynchronizing brain stimulation. Int J Psychophysiol (2007) 64:53–61. doi:10.1016/j.ijpsycho.2006.07.013
Pubmed Abstract | Pubmed Full Text | CrossRef Full Text | Google Scholar
92. Hauptmann C, Tass PA. Cumulative and after-effects of short and weak coordinated reset stimulation: a modeling study. J Neural Eng (2009) 6:016004. doi:10.1088/1741-2560/6/1/016004
93. Hauptmann C, Tass PA. Restoration of segregated, physiological neuronal connectivity by desynchronizing stimulation. J Neural Eng (2010) 7:056008. doi:10.1088/1741-2560/7/5/056008
94. Tass PA, Popovych OV. Unlearning tinnitus-related cerebral synchrony with acoustic coordinated reset stimulation: theoretical concept and modelling. Biol Cybern (2012) 106:27–36. doi:10.1007/s00422-012-0479-5
Pubmed Abstract | Pubmed Full Text | CrossRef Full Text | Google Scholar
95. Popovych OV, Tass PA. Desynchronizing electrical and sensory coordinated reset neuromodulation. Front Hum Neurosci (2012) 6:58. doi:10.3389/fnhum.2012.00058
96. Best EN. Null space in the Hodgkin–Huxley equations: a critical test. Biophys J (1979) 27:87–104. doi:10.1016/S0006-3495(79)85204-2
Pubmed Abstract | Pubmed Full Text | CrossRef Full Text | Google Scholar
97. Demir SS, Butera RJ, DeFranceschi AA, Clark JW, Byrne JH. Phase sensitivity end entrainment in a modeled bursting neuron. Biophys J (1997) 72:579–94. doi:10.1016/S0006-3495(97)78697-1
Pubmed Abstract | Pubmed Full Text | CrossRef Full Text | Google Scholar
98. Tateno T, Robinson HPC. Phase resetting curves and oscillatory stability in interneurons of rat somatosensory cortex. Biophys J (2007) 92:683–95. doi:10.1529/biophysj.106.088021
Pubmed Abstract | Pubmed Full Text | CrossRef Full Text | Google Scholar
99. Neiman A, Russell D, Yakusheva T, DiLullo A, Tass PA. Response clustering in transient stochastic synchronization and desynchronization of coupled neuronal bursters. Phys Rev E Stat Nonlin Soft Matter Phys (2007) 76:021908. doi:10.1103/PhysRevE.76.021908
100. Perkel DH, Schulman JH, Segundo JP, Bullock TH, Moore GP. Pacemaker neurons – effects of regularly spaced synaptic input. Science (1964) 145:61–3. doi:10.1126/science.145.3627.61
Pubmed Abstract | Pubmed Full Text | CrossRef Full Text | Google Scholar
101. Pinsker HM. Aplysia bursting neurons as endogenous oscillators.1. phase-response curves for pulsed inhibitory synaptic input. J Neurophysiol (1977) 40:527–43.
102. Lerma J, Garcia-Austt E. Hippocampal theta rhythm during paradoxical sleep – effects of afferent stimuli and phase-relationships with phasic events. Electroencephalogr Clin Neurophysiol (1985) 60:46–54. doi:10.1016/0013-4694(85)90950-2
Pubmed Abstract | Pubmed Full Text | CrossRef Full Text | Google Scholar
103. Jackson A, Spinks RL, Freeman TCB, Wolpert DM, Lemon RN. Rhythm generation in monkey motor cortex explored using pyramidal tract stimulation. J Physiol (2002) 541:685–99. doi:10.1113/jphysiol.2001.015099
Pubmed Abstract | Pubmed Full Text | CrossRef Full Text | Google Scholar
104. Prinz AA, Thirumalai V, Marder E. The functional consequences of changes in the strength and duration of synaptic inputs to oscillatory neurons. J Neurosci (2003) 23:943–54.
105. Givens B. Stimulus-evoked resetting of the dentate theta rhythm: relation to working memory. Neuroreport (1996) 8:159–63. doi:10.1097/00001756-199612200-00032
Pubmed Abstract | Pubmed Full Text | CrossRef Full Text | Google Scholar
106. Makeig S, Westerfield M, Jung TP, Enghoff S, Townsend J, Courchesne E, et al. Dynamic brain sources of visual evoked responses. Science (2002) 295:690–4. doi:10.1126/science.1066168
Pubmed Abstract | Pubmed Full Text | CrossRef Full Text | Google Scholar
107. Jansen BH, Agarwal G, Hegde A, Boutros NN. Phase synchronization of the ongoing EEG and auditory EP generation. Clin Neurophysiol (2003) 114:79–85. doi:10.1016/S1388-2457(02)00327-9
Pubmed Abstract | Pubmed Full Text | CrossRef Full Text | Google Scholar
108. Ross B, Herdman AT, Pantev C. Stimulus induced desynchronization of human auditory 40-hz steady-state responses. J Neurophysiol (2005) 94:4082–93. doi:10.1152/jn.00469.2005
Pubmed Abstract | Pubmed Full Text | CrossRef Full Text | Google Scholar
109. Van der Werf YD, Paus T. The neural response to transcranial magnetic stimulation of the human motor cortex. I. Intracortical and cortico-cortical contributions. Exp Brain Res (2006) 175:231–45. doi:10.1007/s00221-006-0551-2
Pubmed Abstract | Pubmed Full Text | CrossRef Full Text | Google Scholar
110. Tass PA, Silchenko AN, Hauptmann C, Barnikol UB, Speckmann EJ. Long-lasting desynchronization in rat hippocampal slice induced by coordinated reset stimulation. Phys Rev E Stat Nonlin Soft Matter Phys (2009) 80:011902. doi:10.1103/PhysRevE.80.011902
111. Tass PA, Qin L, Hauptmann C, Doveros S, Bezard E, Boraud T, et al. Coordinated reset has sustained aftereffects in parkinsonian monkeys. Ann Neurol (2012) 72:816–20. doi:10.1002/ana.23663
Pubmed Abstract | Pubmed Full Text | CrossRef Full Text | Google Scholar
112. Adamchic I, Hauptmann C, Barnikol UB, Pawelczyk N, Popovych O, Barnikol TT, et al. Coordinated reset neuromodulation for Parkinson’s disease: proof-of-concept study. Mov Disord (2014) 29:1679–84. doi:10.1002/mds.25923
Pubmed Abstract | Pubmed Full Text | CrossRef Full Text | Google Scholar
113. Hodgkin A, Huxley AF. A quantitative description of membrane current and application to conduction and excitation. J Physiol (1952) 117:500–44.
114. Hansel D, Mato G, Meunier C. Phase dynamics of weakly coupled Hodgkin-Huxley neurons. Europhys Lett (1993) 23:367–72. doi:10.1209/0295-5075/23/5/011
115. Kalitzin S, van Dijk BW, Spekreijse H. Self-organized dynamics in plastic neural networks: bistability and coherence. Biol Cybern (2000) 83:139–50. doi:10.1007/s004220000157
Pubmed Abstract | Pubmed Full Text | CrossRef Full Text | Google Scholar
116. Maistrenko YL, Lysyansky B, Hauptmann C, Burylko O, Tass PA. Multistability in the Kuramoto model with synaptic plasticity. Phys Rev E Stat Nonlin Soft Matter Phys (2007) 75:066207. doi:10.1103/PhysRevE.75.066207
117. Brette R, Gerstner W. Adaptive exponential integrate-and-fire model as an effective description of neuronal activity. J Neurophysiol (2005) 94:3637–42. doi:10.1152/jn.00686.2005
Pubmed Abstract | Pubmed Full Text | CrossRef Full Text | Google Scholar
118. Touboul J, Brette R. Dynamics and bifurcations of the adaptive exponential integrate-and-fire model. Biol Cybern (2008) 99:319–34. doi:10.1007/s00422-008-0267-4
Pubmed Abstract | Pubmed Full Text | CrossRef Full Text | Google Scholar
119. Naud R, Marcille N, Clopath C, Gerstner W. Firing patterns in the adaptive exponential integrate-and-fire model. Biol Cybern (2008) 99:335–47. doi:10.1007/s00422-008-0264-7
Pubmed Abstract | Pubmed Full Text | CrossRef Full Text | Google Scholar
121. Pikovsky A, Rosenblum M, Kurths J. Synchronization, A Universal Concept in Nonlinear Sciences. Cambridge: Cambridge University Press (2001).
122. Lysyansky B, Popovych OV, Tass PA. Optimal number of stimulation contacts for coordinated reset neuromodulation. Front Neuroeng (2013) 6:5. doi:10.3389/fneng.2013.00005
123. Lysyansky B, Popovych OV, Tass PA. Desynchronizing anti-resonance effect of m: n on-off coordinated reset stimulation. J Neural Eng (2011) 8:036019. doi:10.1088/1741-2560/8/3/036019
124. Rinzel JA. A formal classification of bursting mechanisms in excitable systems. In: Teramoto E, Yamaguti M, editors. Mathematical Topics in Population Biology, Morphogenesis and Neurosciences, Lecture Notes in Biomathematics 71. New York, NY: Springer-Verlag (1987). p. 267–81.
125. Izhikevich EM. Synchronization of elliptic bursters. SIAM Rev (2001) 43:315–44. doi:10.1137/S0036144500382064
126. Rizzone M, Lanotte M, Bergamasco B, Tavella A, Torre E, Faccani G, et al. Deep brain stimulation of the subthalamic nucleus in Parkinson’s disease: effects of variation in stimulation parameters. J Neurol Neurosurg Psychiatry (2001) 71:215–9. doi:10.1136/jnnp.71.2.215
Pubmed Abstract | Pubmed Full Text | CrossRef Full Text | Google Scholar
127. Volkmann J, Herzog J, Kopper F, Deuschl G. Introduction to the programming of deep brain stimulators. Mov Disord (2002) 17:S181–7. doi:10.1002/mds.10162
Pubmed Abstract | Pubmed Full Text | CrossRef Full Text | Google Scholar
128. Kuncel AM, Grill WM. Selection of stimulus parameters for deep brain stimulation. Clin Neurophysiol (2004) 115:2431–41. doi:10.1016/j.clinph.2004.05.031
Pubmed Abstract | Pubmed Full Text | CrossRef Full Text | Google Scholar
129. Popovych OV, Yanchuk S, Tass PA. Self-organized noise resistance of oscillatory neural networks with spike timing-dependent plasticity. Sci Rep (2013) 3:2926. doi:10.1038/srep02926
130. Sakaguchi H. Cooperative phenomena in coupled oscillator systems under external fields. Prog Theor Phys (1988) 79:39–46. doi:10.1143/PTP.79.39
131. Masuda N, Kori H. Formation of feedforward networks and frequency synchrony by spike-timing-dependent plasticity. J Comput Neurosci (2007) 22:327–45. doi:10.1007/s10827-007-0022-1
Pubmed Abstract | Pubmed Full Text | CrossRef Full Text | Google Scholar
132. Bayati M, Valizadeh A. Effect of synaptic plasticity on the structure and dynamics of disordered networks of coupled neurons. Phys Rev E Stat Nonlin Soft Matter Phys (2012) 86:011925. doi:10.1103/PhysRevE.86.011925
133. Hobson J, Chisholm E, El Refaie A. Sound therapy (masking) in the management of tinnitus in adults. Cochrane Database Syst Rev (2012) 11:CD006371. doi:10.1002/14651858.CD006371.pub3
134. Linderoth B, Foreman RD. Physiology of spinal cord stimulation: review and update. Neuromodulation (1999) 2:150–64. doi:10.1046/j.1525-1403.1999.00150.x
Pubmed Abstract | Pubmed Full Text | CrossRef Full Text | Google Scholar
135. Oakley JC, Prager JP. Spinal cord stimulation – mechanisms of action. Spine (2002) 27:2574–83. doi:10.1097/00007632-200211150-00034
Pubmed Abstract | Pubmed Full Text | CrossRef Full Text | Google Scholar
136. Sarnthein J, Stern J, Aufenberg C, Rousson V, Jeanmonod D. Increased EEG power and slowed dominant frequency in patients with neurogenic pain. Brain (2006) 129:55–64. doi:10.1093/brain/awh631
Pubmed Abstract | Pubmed Full Text | CrossRef Full Text | Google Scholar
137. Sarnthein J, Jeanmonod D. High thalamocortical theta coherence in patients with neurogenic pain. Neuroimage (2008) 39:1910–7. doi:10.1016/j.neuroimage.2007.10.019
Pubmed Abstract | Pubmed Full Text | CrossRef Full Text | Google Scholar
138. Walton KD, Dubois M, Llinas RR. Abnormal thalamocortical activity in patients with complex regional pain syndrome (CRPS) type I. Pain (2010) 150:41–51. doi:10.1016/j.pain.2010.02.023
Pubmed Abstract | Pubmed Full Text | CrossRef Full Text | Google Scholar
139. Candy JM, Perry RH, Perry EK, Irving D, Blessed G, Fairbairn AF, et al. Pathological-changes in the nucleus of Meynert in Alzheimer’s and Parkinson’s diseases. J Neurol Sci (1983) 59:277–89. doi:10.1016/0022-510X(83)90045-X
Pubmed Abstract | Pubmed Full Text | CrossRef Full Text | Google Scholar
140. Wolkin A, Sanfilipo M, Wolf AP, Angrist B, Brodie JD, Rotrosen J. Negative symptoms and hypofrontality in chronic-schizophrenia. Arch Gen Psychiatry (1992) 49:959–65. doi:10.1001/archpsyc.1992.01820120047007
Pubmed Abstract | Pubmed Full Text | CrossRef Full Text | Google Scholar
141. Salehi A, Lucassen PJ, Pool CW, Gonatas NK, Ravid R, Swaab DF. Decreased neuronal-activity in the nucleus basalis of Meynert in Alzheimer’s-disease as suggested by the size of the Golgi-apparatus. Neuroscience (1994) 59:871–80. doi:10.1016/0306-4522(94)90291-7
Pubmed Abstract | Pubmed Full Text | CrossRef Full Text | Google Scholar
142. Kishimoto H, Yamada K, Iseki E, Kosaka K, Okoshi T. Brain imaging of affective disorders and schizophrenia. Psychiatry Clin Neurosci (1998) 52:S212–4. doi:10.1111/j.1440-1819.1998.tb03224.x
Pubmed Abstract | Pubmed Full Text | CrossRef Full Text | Google Scholar
143. Bearden CE, Hoffman KM, Cannon TD. The neuropsychology and neuroanatomy of bipolar affective disorder: a critical review. Bipolar Disord (2001) 3:106–50. doi:10.1034/j.1399-5618.2001.030302.x
Pubmed Abstract | Pubmed Full Text | CrossRef Full Text | Google Scholar
144. Lysyansky B, Popovych OV, Tass PA. Multi-frequency activation of neuronal networks by coordinated reset stimulation. Interface Focus (2011) 1:75–85. doi:10.1098/rsfs.2010.0010
Pubmed Abstract | Pubmed Full Text | CrossRef Full Text | Google Scholar
145. Rosin B, Slovik M, Mitelman R, Rivlin-Etzion M, Haber SN, Israel Z, et al. Closed-loop deep brain stimulation is superior in ameliorating parkinsonism. Neuron (2011) 72:370–84. doi:10.1016/j.neuron.2011.08.023
Pubmed Abstract | Pubmed Full Text | CrossRef Full Text | Google Scholar
146. Little S, Pogosyan A, Neal S, Zavala B, Zrinzo L, Hariz M, et al. Adaptive deep brain stimulation in advanced Parkinson disease. Ann Neurol (2013) 74:449–57. doi:10.1002/ana.23951
Pubmed Abstract | Pubmed Full Text | CrossRef Full Text | Google Scholar
Keywords: neuronal synchronization, electrical stimulation, sensory stimulation, spike timing-dependent plasticity, anti-kindling, coordinated reset neuromodulation, noise stimulation
Citation: Popovych OV and Tass PA (2014) Control of abnormal synchronization in neurological disorders. Front. Neurol. 5:268. doi: 10.3389/fneur.2014.00268
Received: 12 May 2014; Accepted: 28 November 2014;
Published online: 16 December 2014.
Edited by:
Hubert R. Dinse, Ruhr-University Bochum, GermanyReviewed by:
Maxim Bazhenov, University of California at Riverside, USARolf P. Würtz, Ruhr-University Bochum, Germany
Copyright: © 2014 Popovych and Tass. This is an open-access article distributed under the terms of the Creative Commons Attribution License (CC BY). The use, distribution or reproduction in other forums is permitted, provided the original author(s) or licensor are credited and that the original publication in this journal is cited, in accordance with accepted academic practice. No use, distribution or reproduction is permitted which does not comply with these terms.
*Correspondence:by5wb3BvdnljaEBmei1qdWVsaWNoLmRl;cC50YXNzQGZ6LWp1ZWxpY2guZGU=