- 1Rehab Technologies, Istituto Italiano di Tecnologia, Genova, Italy
- 2Department of Informatics, Bioengineering, Robotics and Systems Engineering (DIBRIS), University of Genova, Genova, Italy
- 3Section of Human Physiology, Department of Experimental Medicine (DIMES), University of Genova, Genova, Italy
- 4Department of Neurology and Neurorehabilitation, Istituto Neurologico Nazionale C. Mondino, Pavia, Italy
- 5Department of Brain and Behavioral Sciences, University of Pavia, Pavia, Italy
Neurological diseases causing motor/cognitive impairments are among the most common causes of adult-onset disability. More than one billion of people are affected worldwide, and this number is expected to increase in upcoming years, because of the rapidly aging population. The frequent lack of complete recovery makes it desirable to develop novel neurorehabilitative treatments, suited to the patients, and better targeting the specific disability. To date, rehabilitation therapy can be aided by the technological support of robotic-based therapy, non-invasive brain stimulation, and neural interfaces. In this perspective, we will review the above methods by referring to the most recent advances in each field. Then, we propose and discuss current and future approaches based on the combination of the above. As pointed out in the recent literature, by combining traditional rehabilitation techniques with neuromodulation, biofeedback recordings and/or novel robotic and wearable assistive devices, several studies have proven it is possible to sensibly improve the amount of recovery with respect to traditional treatments. We will then discuss the possible applied research directions to maximize the outcome of a neurorehabilitation therapy, which should include the personalization of the therapy based on patient and clinician needs and preferences.
Introduction
According to the World Health Organization (WHO), neurological disorders and injuries account for the 6.3% of the global burden of disease (GBD) (1, 2). With more than 6% of DALY (disability-adjusted life years) in the world, neurological disorders represent one of the most widespread clinical condition. Among neurological disorders, more than half of the burden in DALYs is constituted by cerebral-vascular disease (55%), such as stroke. Stroke, together with spinal cord injury (SCI), accounts for 52% of the adult-onset disability and, over a billion people (i.e., about a 15% of the population worldwide) suffer from some form of disability (3). These numbers are likely to increase in the coming years due to the aging of the population (4), since disorders affecting people aged 60 years and older contribute to 23% of the total GBD (5).
Standard physical rehabilitation favors the functional recovery after stroke, as compared to no treatment (6). However, the functional recovery is not always satisfactory as only 20% of patients fully resume their social life and job activities (7). Hence, the need of more effective and patient-tailored rehabilitative approaches to maximize the functional outcome of neurological injuries as well as patients’ quality of life (8). Modern technological methodologies represent one of the most recent advances in neurorehabilitation, and an increasing body of evidence supports their role in the recovery from brain and/or medullary insults. This manuscript provides a perspective on how technologies and methodologies could be combined in order to maximize the outcome of neurorehabilitation.
Current Systems and Therapeutic Approaches for Neurorehabilitation
The great progress made in interdisciplinary fields, such as neural engineering (9, 10), has allowed to investigate many neural mechanisms, by detecting and processing the neural signals at high spatio-temporal resolution, and by interfacing the nervous system with external devices, thus restoring neurological functions lost due to disease/injury. The progress continues in parallel to technological advancements. The last two decades there has seen a large proliferation of technological approaches for human rehabilitation, such as robots, wearable systems, brain stimulation, and virtual environments. In the next sections, we will focus on: robotic therapy, non-invasive brain stimulation (NIBS), and neural interfaces.
Robotic Devices
Robots for neurorehabilitation are designed to support the administration of physical exercises to the upper or lower extremities, with the purpose of promoting neuro-motor recovery. This technology has a relatively long history, dating back to the early 1990s (11). Robot devices for rehabilitation differ widely in terms of mechanical design, number of degrees of freedom, and control architectures. As regards the mechanical design, robots may have either a single point of interaction (i.e., end effector) with the user body (endpoint robots or manipulanda) or multiple points of interaction (exoskeletons and wearable robots) (12).
Endpoint robots for the upper extremity, include Inmotion2 (IMT, USA) (13), KINARM End-Point (BKIN, Canada), and Braccio di Ferro (14) (Figure 1A1, left). Only some of these devices have been tested in randomized clinical trials (15), confirming an improvement of upper limb motor function after stroke (16). However, convincing evidence in favor of significant changes in activities of daily living (ADL) indicators is lacking (17), possibly because performance in ADL is highly affected by hand functionality. A good example of lower limb endpoint robot is represented by gait trainer GT1 (Reha-Stim, Germany). Its efficacy was tested by Picelli et al. (18), who demonstrated an improvement in multiple clinical measures in subjects with Parkinson’s disease following robotic-assisted rehabilitation when compared to physical rehabilitation alone (18). Endpoint robots are also available for postural rehabilitation. For instance, Hunova (Movendo Technology, Italy, launched in 2017) is equipped with a seat and a platform that induce multidirectional movements to improve postural stability (Figure 1A1, right).
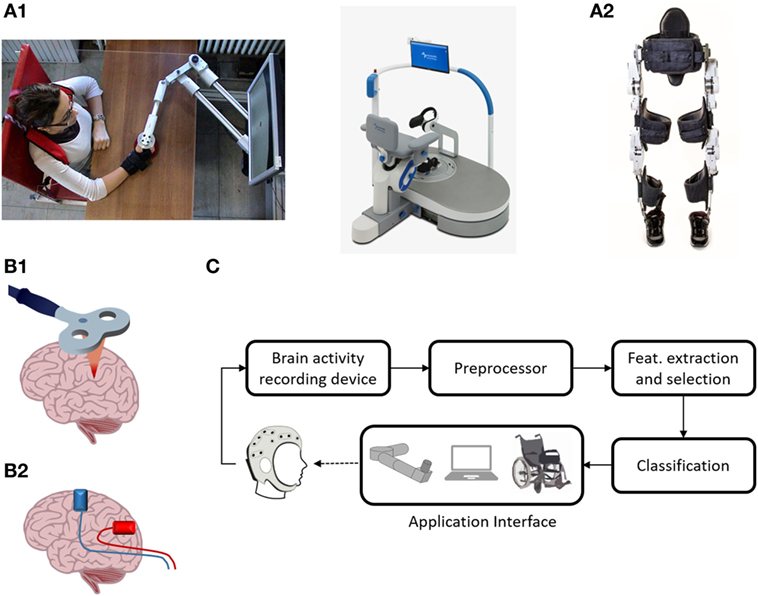
Figure 1. Neurorehabilitation therapies. (A1) Endpoint robots: on the left the “Braccio di Ferro” manipulandum, on the right the postural robot Hunova. Braccio di ferro (14) is a planar manipulandum with 2-DOF, developed at the University of Genoa (Italy). It is equipped with direct-drive brushless motors and is specially designed to minimize endpoint inertia. It uses the H3DAPI programming environment, which allows to share exercise protocol with other devices. Written informed consent was obtained from the subject depicted in the panel. Movendo Technology’s Hunova is a robotic device that permits full-body rehabilitation. It has two 2-DOF actuated and sensorized platforms located under the seat and on the floor level that allow it to rehabilitate several body districts, including lower limb (thanks to the floor-level platform), the core, and the back, using the platform located underneath the seat. Different patient categories (orthopedic, neurological, and geriatric) can be treated, and interact with the machine through a GUI based on serious games. (A2) Wearable device: the recent exoskeleton Twin. Twin is a fully modular device developed at IIT and co-funded by INAIL (the Italian National Institute for Insurance against Accidents at Work). The device can be easily assembled/disassembled by the patient/therapist. It provides total assistance to patients in the 5–95th percentile range with a weight up to 110 kg. Its modularity is implemented by eight quick release connectors, each located at both mechanical ends of each motor, that allow mechanical and electrical connection with the rest of the structure. It can implement three different walking patterns that can be fully customized according to the patient’s needs via a GUI on mobile device, thus enabling personalization of the therapy. Steps can be triggered via an IMU-based machine state controller. (B1) Repetitive transcranial magnetic stimulation (rTMS) representation. rTMS refers to the application of magnetic pulses in a repetitive mode. Conventional rTMS applied at low frequency (0.2–1 Hz) results in plastic inhibition of cortical excitability, whereas when it is applied at high frequency (≥5Hz), it leads to excitation (19). rTMS can also be applied in a “patterned mode.” Theta burst stimulation involves applying bursts of high frequency magnetic stimulation (three pulses at 50 Hz) repeated at intervals of 200 ms (20). Intermittent TBS increases cortical excitability for a period of 20–30 min, whereas continuous TBS leads to a suppression of cortical activity for approximately the same amount of time (20). (B2) Transcranial current stimulation (tCS) representation. tCS uses ultra-low intensity current, to manipulate the membrane potential of neurons and modulate spontaneous firing rates, but is insufficient on its own to discharge resting neurons or axons (21). tCS is an umbrella term for a number of brain modulating paradigms, such as transcranial direct current stimulation (22), transcranial alternating current stimulation (23), and transcranial random noise stimulation (24). (C) A typical BCI system. Five stages are represented: brain-signal acquisition, preprocessing, feature extraction/selection, classification, and application interface. In the first stage, brain-signal acquisition, suitable signals are acquired using an appropriate modality. Since the acquired signals are normally weak and contain noise (physiological and instrumental) and artifacts, preprocessing is needed, which is the second stage. In the third stage, some useful data or so-called “features” are extracted. These features, in the fourth stage, are classified using a suitable classifier. Finally, in the fifth stage, the classified signals are transmitted to a computer or other external devices for generating the desired control commands to the devices. In neurofeedback applications, the application interface is a real-time display of brain activity, which enables self-regulation of brain functions (25).
Typical lower limb exoskeletons range from large systems, equipped with treadmill and weight support, and intended for hospital use, like the Lokomat (Hocoma, Switzerland) and the LOPES system (26), to more lightweight devices intended for overground walking, like Ekso (Ekso Bionics, USA), Indego (Parker Hannafin, USA), Rewalk (Rewalk Robotics, USA), and the most recent one, Twin (IIT-INAIL, Italy). Notably, Twin has been developed according to long interactions with focus groups of disabled patients (Figure 1A2). A few exoskeletons for the upper limb have also been developed. They also range from lab systems—e.g., the KINARM Exoskeleton (BKIN, Canada) or the Armeo Spring and Power (Hocoma, Switzerland)—to wearable, modular devices (27–29).
One common feature of rehabilitation robots, is that they are equipped with movement and/or force sensors, so that they integrate functionalities both for the assessment [i.e., quantify users’ movements and exchanged forces (30)] and the treatment (i.e., administer highly reproducible, repetitive exercise protocols, and interaction modalities).
In spite of the increasing volume of published studies, the number of high-quality clinical trials on robot-assisted therapy is still relatively low. A large multi-center RCT comparing robot therapy, intensive physical therapy, and usual care (31) confirmed that robots are indeed effective, but found no significant advantage over conventional physical therapy. A systematic comparison of different approaches (32) suggested that robot therapy is among the most effective techniques for the rehabilitation of both upper and lower limbs. Moreover, recent studies concluded that robot-assisted gait training in combination with physiotherapy is more likely to achieve independent walking than gait training alone (33, 34).
A major limitation of endpoint robotic approach is that the improvement is limited to the body regions involved in training. In a clinical setting, robotic rehabilitation may be cost and time-consuming, and for this reason, it is difficult to imagine the combination of different endpoint robotic devices in the patient who have an impairment that affects multiple body areas, i.e., post-stroke hemiplegia. Moreover, early robots for neuroehabilitation were specifically aimed at substituting labor-intensive physical rehabilitation with minimal human intervention, producing an automatic and repetitive treatment. This initial trend, however, minimizes the importance of both therapist knowledge and patient–physician relationship. However, the ability to precisely quantify sensorimotor performance during exercise in terms of movement kinematics and exchanged forces is leading to a new revolution in rehabilitation, toward evidence-based and knowledge-driven approaches. Modern rehabilitation devices automatically adapt task difficulty and assistance modalities to individual performance (35). In the future, they may incorporate models of the recovery process (36) to predict the rehabilitation outcome (37) that will be fitted on patient’s features.
Another stimulating challenge is the development of lightweight robots suitable for the use outside of the hospitals, in domestic or community environments and in conjunction with ADL, e.g., over ground walking in unstructured environments. This implies a modular structure, which facilitates donning and transportability.
Non-Invasive Brain Stimulation
Non-invasive brain stimulation techniques are a promising adjuvant strategy for enhancing post-injury recovery. In recent years, more than 1,400 studies were performed in humans, with at least one-fifth of these focusing on stroke rehabilitation. NIBS techniques involve modulation of the central nervous system by electrically activating neurons in the brain (38) and can be used to influence cortical excitability, neuroplasticity, and behavior (39, 40). Repetitive transcranial magnetic stimulation (rTMS, Figure 1B1) and transcranial current stimulation (tCS, Figure 1B2) are the most common and widely used techniques (39). Because of its relative ease of use, portability and decreased safety risk compared to rTMS, tCS is emerging as an effective and versatile clinical tool to prime the brain activity prior to or during neurorehabilitation. Starting from the hypothesis on training-induced plasticity, NIBS could be applied to foster plasticity induction, also in the spinal cord as shown in animals (41, 42) and in humans (43).
Related to rehabilitation, one of the major challenges is to design interventions that are efficient, promote motor learning, consolidate skills, and augment retention. For example, NIBS approach to stroke rehabilitation has focused on excitation of the unaffected hemisphere, of the affected hemisphere, or inhibition of unaffected hemisphere, also combining neuromodulation of both hemispheres (44). To date, a number of sham-controlled studies based on NIBS have been performed, but the evidence remains inconsistent. A Cochrane review failed to support the efficacy of rTMS for stroke rehabilitation (45), although other studies (46, 47) concluded that low frequency rTMS was effective in improving ADL and aphasia. A recent review (48) concluded that rTMS may produce both short- and long-term improvement on motor recovery in stroke patients, in particular when neuromodulation is initiated early after stroke, and with better results in case of sub-cortical lesions with respect to cortical ones. As regards tCS, it appeared to be useful for motor recovery in a sub population of patients with chronic stroke and low functional impairment (49) and very well tolerated (50), but a Cochrane review (51) failed to support its effectiveness. A possible explanation for these inconsistent conclusions is the lack of a correct patient stratification, and thus a tailored stimulation protocol (51, 52).
Some ethical and technical considerations deserve discussion. First, use of NIBS, calls for greater caution on pediatric population, given the higher stakes and uncertain future effects for brains still undergoing rapid and formative development (53). Second, a careful evaluation of the use of NIBS must also be warranted in adults, regarding informed consent and patient selection (54). Any direct interference with neural activity, even beneficial, might be described more accurately as “minimally invasive” (55). Moreover, when considering that most of the studies mainly focused on the short-term, short-lasting effects of NIBS, it is important to evaluate the long-term effects of modulating cortical electric fields in patients with cortical impairment.
Careful monitoring is particularly important when considering that, despite researchers’ discussion of and explicit warnings against unsupervised use of NIBS (56), brain stimulation products are already commercially available and without proper guidance or information. Thus NIBS could be conducted carelessly with unknown and potentially harmful effects.
Neural Interfaces
In recent years, it is possible to include also neural interfaces among the strategies for neurorehabilitation and indeed the use of these systems in clinical applications is increasing (57, 58).
A neural interface is essentially a system mediating the communication between the brain and an external device (59, 60). Several modalities have been used for brain signal acquisition (61), which include electroencephalography (EEG) (62), magnetoencephalography (MEG) (63), functional magnetic resonance imaging (64), and functional near-infrared spectroscopy (65). Among neural interfaces, the so-called “BCIs” (Figure 1C) were essentially conceived as communication tools for paralyzed or locked-in patients (62) and were mainly based on the use of the processed EEG signal. Typical BCI techniques include the use of evoked potentials (such as P300) (66) or motor imagery (67), and enable the user to communicate with a speller device (68) or to control the movement of an end effector, either virtual (69) or real (70). From a clinical point of view, the BCI approach proves to be beneficial in potentiating the impaired motor function, as demonstrated for stroke (71, 72). MEG BCI training allowed patients with chronic stroke to voluntary modulate the μ-rhythm amplitude over the affected hemisphere with the possibility to voluntary control grasping using a robotic hand orthosis (73). More recently, a BCI-orthosis training was tested as add-on to physical therapy in a sham-controlled study (74); after an EEG BCI training protocol the strength in hand muscles significantly improved when compared to sham group. Noteworthy, the results of the above cited studies were achieved in patients in a chronic stage, for whom very limited possibilities are available if treated with standard rehabilitative care. The motor improvement is a consequence of the cortical changes occurring during the interaction with the controlled object, as demonstrated both with invasive and non-invasive studies (75, 76). This promising evidence made BCIs appealing for different types of neurorehabilitation practices, not only in presence of motor disability, but also for the recovery of impaired cognitive functions (76–80). In this framework, a particular form of BCI is that of neurofeedback, in which neural data are visually displayed to the user (81). This technique has proven to be mainly effective in the treatment of attention deficits/hyperactivity, but also for other cognitive dysfunctions (82, 83) and in stroke (84, 85).
Over the past decade also invasive brain-machine interfaces and neural prostheses in general have been the subject of extensive research with promising findings for the treatment of neuro-related impairments (86). The development of these devices will hopefully have a profound social impact on the quality of life, although translation to clinical application is far to be implemented due to the technological barriers (e.g., wired systems or limited bandwidth for wireless systems) and to the limits imposed by the invasiveness of the procedure (e.g., tissue reaction to the brain implant) (87).
Neural prosthesis can be combined with functional electrical stimulation (88, 89). In this scenario, the use of a controlled end effector is substituted by direct stimulation of the involved muscles, therefore, natural movements are recreated by bridging two areas disconnected because of the impairment/disease (88, 89). A system was recently developed allowing a quadriplegic patient chronically implanted with microwire arrays to move the arm by means of muscle stimulation triggered by the recorded and decoded brain signals (90).
Examples of latest-generation neural prostheses involve direct stimulation of central or peripheral neural tissue. Recent animal studies demonstrated locomotion restoration after SCI by spatiotemporal modulation of the spinal cord (91) and restoration of motor function after stroke by activity-dependent stimulation of the motor cortex (92). Whereas, recent human studies demonstrated the restoration of hand reaching and grasping by non-invasive neuromuscular stimulation of hand muscles (93) and prosthetic hand control by invasive stimulation of peripheral nerve (94). In addition, faster and more effective closed loop stimulation protocols are being investigated also in in vitro preparations (95).
Challenges and Open Issues
We have so far presented the main methodologies for neurorehabilitation and, for each field, the most innovative trends currently under investigation. However, novel rehabilitation approaches are characterized by a synergistic tactic, in which these techniques are used in combination and also mixed with kinematic information (from the robot) and patients’ biosignals, such as EEG or EMG (electromyography) (Figure 2A).
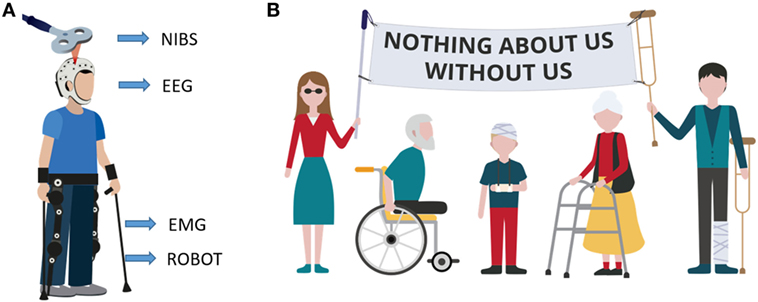
Figure 2. Innovative patient-tailored approach. (A) Example of multimodal rehabilitative approach. Subject is using an exoskeleton while receiving brain stimulation. Both exoskeleton motors and stimulation parameters are updated based on subject’s biofeedback signals (electroencephalography and/or EMG) and on subject performance (ROBOT) while, at the same time brain stimulation non-invasive brain stimulation and exoskeleton assistance (ROBOT) influence the biosignals. (B) Motto of the disabled population. The motto means that any choice (in any field) regarding them must be taken with their direct participation. Rehabilitation research must follow the same policy.
An example of such a multimodal approach was shown to the general public during the world cup in 2014, when the kick off was given by a paraplegic man using a lower limbs exoskeleton controlled by brain activity. Two years later, it was demonstrated that the combined use of gait rehabilitation with a BMI was able to induce partial neurological recovery in paraplegic patients (96). This represents a valid proof-of-concept for the combination of robotic devices driven by neural activity. Moreover, the number of clinical-oriented versions of this approach is increasing: exoskeletons powered by BCI have been used during post stroke rehabilitation (97, 98). Similar results were obtained with a BCI system for locomotion rehabilitation, based on the use of an avatar in a virtual reality environment (99).
Experiences where assisted locomotion has been used in conjunction with neuromodulation are already present in the literature. Spinal tDCS was applied in patients with SCI undergoing assisted locomotion using driven gait orthosis (Lokomat, Hocoma AG, Volketswil, Switzerland) (100). Results showed that anodal spinal tDCS and assisted locomotion increased spinal reflexes amplitude, suggesting functional effects when the spinal cord is detached from the rest of the central nervous system. These findings open an important avenue of research designed to rescue residual spinal functions by spinal tDCS in SCI patients (100).
Although the combined effect of neuromodulation with robotic therapy still needs to be clinically validated (101), it clearly shows the combined direction of neuromodulation-based rehabilitation. Explorative directions in neuromodulation also include a combination with traditional BMI approaches (102) as well as investigation of non-classical stimulation sites (103). Another potentially useful synergy in the rehabilitative field is represented by the association of biofeedback (104, 105) with robotic rehabilitation (106, 107).
Besides the technological and scientific improvement of neurorehabilitative treatment, a very important trend followed by current research is that of a personalized treatment. This is not just intended to focus on a particular disease and address the symptoms shared by different populations of patients, but is truly envisioned as a personalized method for a single individual. The idea of a patient-tailored approach is not new: standardized algorithms have been proposed for stroke, based on the clinical history of the patient, time elapsed after insult, topography of the lesion, type, and severity of functional impairment (108). In this view, it will be desirable to identify solid biomarkers not only in the acute settings, but also in the middle and chronic stages of neurological diseases. This modern approach, recently named as “Rehabilomics,” will be useful not only for outcome prediction, but also to foresee the best personalized rehabilitative treatment. Well known biomarkers in stroke are represented by measures of function and structure through neuroimaging after stroke (109) and by biochemical dosages (for example, uric acid, Cu/Zn superoxide dismutase, and urinary 8-OHdG) (110, 111). The technological improvement will help to identify novel biomarkers in neurorehabilitation. For example, a non-linear, composite, model made of robotic measurement in the upper limb was able to predict motor recovery at 90 days from stroke (112). “Technological” measures seem to be complementary rather than substitutive to standard biomarkers (113).
Overall, there is a great need for the development and testing of novel innovative interventional strategies individually tailored to patients’ prerequisites. The neurorehabilitation scientific community is finally showing an effort in this direction, by taking into account patients’ specific requests (Figure 2B). For example, during the sixth International Brain–Computer Interface Meeting in 2016 (114), a virtual forum of BCI users was presented, allowing patients to remotely participate at the conference sessions and also to send video-message with their views and requirements in order to help the scientists shaping the future research directions. This is exactly the approach that should be taken when designing a therapy or experimental protocol targeting a specific set of population. The patients’ motto “Nothing about us, without us” clearly indicates that patients must be involved in experimental studies, since the very beginning.
Conclusion
In the coming years, science and medicine have to create a integrated dialog with patients, since they will be the first end-users of any technological development. To date, important advances have been made in robotic-based therapy, NIBS and neural interfaces, as integrations and/or alternatives to standard therapy. However, in order to be really effective, neurorehabilitation research must be primarily person-centered (i.e., “personalized”). Personalization calls for flexible solutions, such as combining the main technologies, in order to adapt to the different patient’s features and preferences. And this is exactly the direction which should be undertaken in neurorehabilitation.
Author Contributions
MS, LDM, and MC conceived the manuscript. MC and LDM coordinated the activities. MS and MC prepared the figures. MS, MC, RDI, and LA revised the manuscript. All the authors wrote and approved the final version of the manuscript.
Conflict of Interest Statement
The authors declare that the research was conducted in the absence of any commercial or financial relationships that could be construed as a potential conflict of interest.
The handling Editor declared a shared affiliation, though no other collaboration, with one of the authors RI.
Acknowledgments
The original graphics used in the figures of the manuscript were developed by Silvia Chiappalone. The authors wish to tank Dr. Giovanni Milandri for carefully proofreading the manuscript.
Funding
This work was supported by the Istituto Nazionale per l’Assicurazione contro gli Infortuni sul Lavoro (INAIL) under grant agreement POR1.
References
2. Chin JH, Vora N. The global burden of neurologic diseases. Neurology (2014) 83(4):349–51. doi:10.1212/WNL.0000000000000610
3. WHO. Disability and Health Fact sheet No 352. WHO (2013). Available from: https://www.leprosy-information.org/resource/disability-and-health-fact-sheet-n-352 (Accessed: December 22, 2017).
4. EC. The 2015 ageing report: economic and budgetary projections for the 28 EU member states (2013-2060). European Economy. (2013). doi:10.2765/877631
5. Prince MJ, Wu F, Guo Y, Gutierrez Robledo LM, O’Donnell M, Sullivan R, et al. The burden of disease in older people and implications for health policy and practice. Lancet (2015) 385(9967):549–62. doi:10.1016/S0140-6736(14)61347-7
6. Pollock A, Baer G, Campbell P, Choo PL, Forster A, Morris J, et al. Physical rehabilitation approaches for the recovery of function and mobility following stroke. Cochrane Database Syst Rev (2014) 97(4):3109–17. doi:10.1002/14651858.CD001920.pub3
7. Di Carlo A. Human and economic burden of stroke. Age Ageing (2009) 38(1):4–5. doi:10.1093/ageing/afn282
8. Caramenti M, Bartenbach V, Gasperotti L, Oliveira da Fonseca L, Berger TW, Pons JL. Challenges in nerurorehabilitation and neural engineering. In: Pons JL, Raya R, González J, editors. Emerging Therapies in Neurorehabilitation II. Switzerland: Springer International Publishing (2016). doi:10.1007/978-3-319-24901-8
10. Duran DM. What is neural engineering? J Neural Eng (2006) 4(4):1–2. Available from: http://stacks.iop.org/1741-2552/4/i=4/a=E01 (Accessed: December 22, 2017).
11. Sanguineti V, Casadio M, Masia L, Squeri V, Morasso PG. Robot-assisted neuro-rehabilitation. In: Farina D, Jensen W, Akay M, editors. Introduction to Neural Engineering for Motor Rehabilitation. Hoboken, NJ, USA: John Wiley & Sons, Inc (2013). p. 515–28.
12. Maciejasz P, Eschweiler J, Gerlach-Hahn K, Jansen-Troy A, Leonhardt S. A survey on robotic devices for upper limb rehabilitation. J Neuroeng Rehabil (2014) 11(1):3. doi:10.1186/1743-0003-11-3
13. Aisen ML, Krebs HI, Hogan N, McDowell F, Volpe BT. The effect of robot-assisted therapy and rehabilitative training on motor recovery following stroke. Arch Neurol (1997) 54(4):443–6. doi:10.1001/archneur.1997.00550160075019
14. Casadio M, Morasso PG, Sanguineti V, Arrichiello V. Braccio di Ferro: a new haptic workstation for neuromotor rehabilitation. Technol Health Care (2006) 13:1–20. Available from: https://content.iospress.com/journals/technology-and-health-care/Preprint/Preprint (Accessed: December 22, 2017).
15. Kwakkel G, Kollen BJ, Krebs HI. Effects of robot-assisted therapy on upper limb recovery after stroke: a systematic review. Neurorehabil Neural Repair (2008) 22(2):111–21. doi:10.1177/1545968307305457
16. Prange GB, Jannink MJ, Groothuis-Oudshoorn CG, Hermens HJ, Ijzerman MJ. Systematic review of the effect of robot-aided therapy on recovery of the hemiparetic arm after stroke. J Rehabil Res Dev (2006) 43(2):171–84. doi:10.1682/JRRD.2005.04.0076
17. Mehrholz J, Platz T, Kugler J, Pohl M. Electromechanical and robot-assisted arm training for improving arm function and activities of daily living after stroke. Cochrane Database Syst Rev (2008) (4):CD006876. doi:10.1002/14651858.CD006876.pub2
18. Picelli A, Melotti C, Origano F, Waldner A, Gimigliano R, Smania N. Does robotic gait training improve balance in Parkinson’s disease? A randomized controlled trial. Parkinsonism Relat Disord (2012) 18(8):990–3. doi:10.1016/j.parkreldis.2012.05.010
19. Hallett M. Transcranial magnetic stimulation: a primer. Neuron (2007) 55(2):187–99. doi:10.1016/j.neuron.2007.06.026
20. Huang YZ, Edwards MJ, Rounis E, Bhatia KP, Rothwell JC. Theta burst stimulation of the human motor cortex. Neuron (2005) 45(2):201–6. doi:10.1016/j.neuron.2004.12.033
21. Fricke K, Seeber AA, Thirugnanasambandam N, Paulus W, Nitsche MA, Rothwell JC. Time course of the induction of homeostatic plasticity generated by repeated transcranial direct current stimulation of the human motor cortex. J Neurophysiol (2011) 105(3):1141–9. doi:10.1152/jn.00608.2009
22. Nitsche MA, Doemkes S, Karakose T, Antal A, Liebetanz D, Lang N, et al. Shaping the effects of transcranial direct current stimulation of the human motor cortex. J Neurophysiol (2007) 97(4):3109–17. doi:10.1152/jn.01312.2006
23. Antal A, Boros K, Poreisz C, Chaieb L, Terney D, Paulus W. Comparatively weak after-effects of transcranial alternating current stimulation (tACS) on cortical excitability in humans. Brain Stimul (2008) 1(2):97–105. doi:10.1016/j.brs.2007.10.001
24. Terney D, Chaieb L, Moliadze V, Antal A, Paulus W. Increasing human brain excitability by transcranial high-frequency random noise stimulation. J Neurosci (2008) 28(52):14147–55. doi:10.1523/JNEUROSCI.4248-08.2008
25. Naseer N, Hong KS. fNIRS-based brain-computer interfaces: a review. Front Hum Neurosci (2015) 9:3. doi:10.3389/fnhum.2015.00003
26. Veneman JF, Kruidhof R, Hekman EE, Ekkelenkamp R, Van Asseldonk EH, van der Kooij H. Design and evaluation of the LOPES exoskeleton robot for interactive gait rehabilitation. IEEE Trans Neural Syst Rehabil Eng (2007) 15(3):379–86. doi:10.1109/TNSRE.2003.818185
27. Lo HS, Xie SQ. Exoskeleton robots for upper-limb rehabilitation: state of the art and future prospects. Med Eng Phys (2012) 34(3):261–8. doi:10.1016/j.medengphy.2011.10.004
28. Jarrasse N, Proietti T, Crocher V, Robertson J, Sahbani A, Morel G, et al. Robotic exoskeletons: a perspective for the rehabilitation of arm coordination in stroke patients. Front Hum Neurosci (2014) 8:947. doi:10.3389/fnhum.2014.00947
29. Contreras-Vidal JL, Bhagat NA, Brantley J, Cruz-Garza JG, He Y, Manley Q, et al. Powered exoskeletons for bipedal locomotion after spinal cord injury. J Neural Eng (2016) 13(3):031001. doi:10.1088/1741-2560/13/3/031001
30. Balasubramanian S, Colombo R, Sterpi I, Sanguineti V, Burdet E. Robotic assessment of upper limb motor function after stroke. Am J Phys Med Rehabil (2012) 91(11 Suppl 3):S255–69. doi:10.1097/PHM.0b013e31826bcdc1
31. Lo AC, Guarino PD, Richards LG, Haselkorn JK, Wittenberg GF, Federman DG, et al. Robot-assisted therapy for long-term upper-limb impairment after stroke. N Engl J Med (2010) 362(19):1772–83. doi:10.1056/NEJMoa0911341
32. Langhorne P, Coupar F, Pollock A. Motor recovery after stroke: a systematic review. Lancet Neurol (2009) 8(8):741–54. doi:10.1016/S1474-4422(09)70150-4
33. Mehrholz J, Elsner B, Werner C, Kugler J, Pohl M. Electromechanical-assisted training for walking after stroke. Cochrane Database Syst Rev (2013) (7):CD006185. doi:10.1002/14651858.CD006185.pub3
34. Morone G, Paolucci S, Cherubini A, De Angelis D, Venturiero V, Coiro P, et al. Robot-assisted gait training for stroke patients: current state of the art and perspectives of robotics. Neuropsychiatr Dis Treat (2017) 13:1303–11. doi:10.2147/NDT.S114102
35. Marchal-Crespo L, Reinkensmeyer DJ. Review of control strategies for robotic movement training after neurologic injury. J Neuroeng Rehabil (2009) 6:20. doi:10.1186/1743-0003-6-20
36. Casadio M, Sanguineti V. Learning, retention and slacking: a model of the dynamics of recovery in robot therapy. IEEE Trans Neural Syst Rehabil Eng (2012) 20(3):286–96. doi:10.1109/TNSRE.2012.2190827
37. Reinkensmeyer DJ, Burdet E, Casadio M, Krakauer JW, Kwakkel G, Lang CE, et al. Computational neurorehabilitation: modeling plasticity and learning to predict recovery. J Neuroeng Rehabil (2016) 13(1):42. doi:10.1186/s12984-016-0148-3
38. Dymond AM, Coger RW, Serafetinides EA. Intracerebral current levels in man during electrosleep therapy. Biol Psychiatry (1975) 10(1):101–4.
39. Hummel FC, Cohen LG. Drivers of brain plasticity. Curr Opin Neurol (2005) 18(6):667–74. doi:10.1097/01.wco.0000189876.37475.42
40. Nitsche MA, Cohen LG, Wassermann EM, Priori A, Lang N, Antal A, et al. Transcranial direct current stimulation: state of the art 2008. Brain Stimul (2008) 1(3):206–23. doi:10.1016/j.brs.2008.06.004
41. Ahmed Z. Trans-spinal direct current stimulation modulates motor cortex-induced muscle contraction in mice. J Appl Physiol (1985) (2011) 110(5):1414–24. doi:10.1152/japplphysiol.01390.2010
42. Ahmed Z. Electrophysiological characterization of spino-sciatic and cortico-sciatic associative plasticity: modulation by trans-spinal direct current and effects on recovery after spinal cord injury in mice. J Neurosci (2013) 33(11):4935–46. doi:10.1523/JNEUROSCI.4930-12.2013
43. Einhorn J, Li A, Hazan R, Knikou M. Cervicothoracic multisegmental transpinal evoked potentials in humans. PLoS One (2013) 8(10):e76940. doi:10.1371/journal.pone.0076940
44. Wessel MJ, Zimerman M, Hummel FC. Non-invasive brain stimulation: an interventional tool for enhancing behavioral training after stroke. Front Hum Neurosci (2015) 9:265. doi:10.3389/fnhum.2015.00265
45. Hao Z, Wang D, Zeng Y, Liu M. Repetitive transcranial magnetic stimulation for improving function after stroke. Cochrane Database Syst Rev (2013) (5):CD008862. doi:10.1002/14651858.CD008862.pub2
46. Hsu WY, Cheng CH, Liao KK, Lee IH, Lin YY. Effects of repetitive transcranial magnetic stimulation on motor functions in patients with stroke: a meta-analysis. Stroke (2012) 43(7):1849–57. doi:10.1161/STROKEAHA.111.649756
47. Ren CL, Zhang GF, Xia N, Jin CH, Zhang XH, Hao JF, et al. Effect of low-frequency rTMS on aphasia in stroke patients: a meta-analysis of randomized controlled trials. PLoS One (2014) 9(7):e102557. doi:10.1371/journal.pone.0102557
48. Zhang L, Xing G, Fan Y, Guo Z, Chen H, Mu Q. Short- and long-term effects of repetitive transcranial magnetic stimulation on upper limb motor function after stroke: a systematic review and meta-analysis. Clin Rehabil (2017) 31(9):1137–53. doi:10.1177/0269215517692386
49. Marquez J, van Vliet P, McElduff P, Lagopoulos J, Parsons M. Transcranial direct current stimulation (tDCS): does it have merit in stroke rehabilitation? A systematic review. Int J Stroke (2015) 10(3):306–16. doi:10.1111/ijs.12169
50. Russo C, Souza Carneiro MI, Bolognini N, Fregni F. Safety review of transcranial direct current stimulation in stroke. Neuromodulation (2017) 20(3):215–22. doi:10.1111/ner.12574
51. Elsner B, Kugler J, Pohl M, Mehrholz J. Transcranial direct current stimulation (tDCS) for improving activities of daily living, and physical and cognitive functioning, in people after stroke. Cochrane Database Syst Rev (2016) 3:CD009645. doi:10.1002/14651858.CD009645.pub3
52. Di Pino G, Pellegrino G, Assenza G, Capone F, Ferreri F, Formica D, et al. Modulation of brain plasticity in stroke: a novel model for neurorehabilitation. Nat Rev Neurol (2014) 10(10):597–608. doi:10.1038/nrneurol.2014.162
53. Cohen Kadosh R, Levy N, O’Shea J, Shea N, Savulescu J. The neuroethics of non-invasive brain stimulation. Curr Biol (2012) 22(4):R108–11. doi:10.1016/j.cub.2012.01.013
54. Iwry J, Yaden DB, Newberg AB. Noninvasive brain stimulation and personal identity: ethical considerations. Front Hum Neurosci (2017) 11:281. doi:10.3389/fnhum.2017.00281
55. Fitz NS, Reiner PB. The challenge of crafting policy for do-it-yourself brain stimulation. J Med Ethics (2015) 41(5):410–2. doi:10.1136/medethics-2013-101458
56. Wurzman R, Hamilton RH, Pascual-Leone A, Fox MD. An open letter concerning do-it-yourself users of transcranial direct current stimulation. Ann Neurol (2016) 80(1):1–4. doi:10.1002/ana.24689
57. Birbaumer N, Chaudhary U. Learning from brain control: clinical application of brain–computer interfaces. e-Neuroforum. (2015) 6(4):87–95. doi:10.1007/s13295-015-0015-x
58. Chaudhary U, Birbaumer N, Ramos-Murguialday A. Brain-computer interfaces for communication and rehabilitation. Nat Rev Neurol (2016) 12(9):513–25. doi:10.1038/nrneurol.2016.113
59. Fetz EE. Volitional control of neural activity: implications for brain-computer interfaces. J Physiol (2007) 579(Pt 3):571–9. doi:10.1113/jphysiol.2006.127142
60. Hatsopoulos NG, Donoghue JP. The science of neural interface systems. Annu Rev Neurosci (2009) 32:249–66. doi:10.1146/annurev.neuro.051508.135241
61. Andersen RA, Musallam S, Pesaran B. Selecting the signals for a brain-machine interface. Curr Opin Neurobiol (2004) 14(6):720–6. doi:10.1016/j.conb.2004.10.005
62. Wolpaw JR, Birbaumer N, McFarland DJ, Pfurtscheller G, Vaughan TM. Brain-computer interfaces for communication and control. Clin Neurophysiol (2002) 113(6):767–91. doi:10.1016/S1388-2457(02)00057-3
63. Mellinger J, Schalk G, Braun C, Preissl H, Rosenstiel W, Birbaumer N, et al. An MEG-based brain-computer interface (BCI). Neuroimage (2007) 36(3):581–93. doi:10.1016/j.neuroimage.2007.03.019
64. Weiskopf N, Mathiak K, Bock SW, Scharnowski F, Veit R, Grodd W, et al. Principles of a brain-computer interface (BCI) based on real-time functional magnetic resonance imaging (fMRI). IEEE Trans Biomed Eng (2004) 51(6):966–70. doi:10.1109/TBME.2004.827063
65. Ferrari M, Quaresima V. A brief review on the history of human functional near-infrared spectroscopy (fNIRS) development and fields of application. Neuroimage (2012) 63(2):921–35. doi:10.1016/j.neuroimage.2012.03.049
66. Birbaumer N, Weber C, Neuper C, Buch E, Haapen K, Cohen L. Physiological regulation of thinking: brain-computer interface (BCI) research. Prog Brain Res (2006) 159:369–91. doi:10.1016/S0079-6123(06)59024-7
67. Pfurtscheller G, Neuper C, Schlogl A, Lugger K. Separability of EEG signals recorded during right and left motor imagery using adaptive autoregressive parameters. IEEE Trans Rehabil Eng (1998) 6(3):316–25. doi:10.1109/86.712230
68. Wang C, Guan C, Zhang H. P300 brain-computer interface design for communication and control applications. Conf Proc IEEE Eng Med Biol Soc (2005) 5:5400–3. doi:10.1109/IEMBS.2005.1615703
69. Flotzinger D, Kalcher J, Pfurtscheller G. EEG classification by learning vector quantization. Biomed Tech (Berl) (1992) 37(12):303–9. doi:10.1515/bmte.1992.37.12.303
70. Galan F, Nuttin M, Lew E, Ferrez PW, Vanacker G, Philips J, et al. A brain-actuated wheelchair: asynchronous and non-invasive brain-computer interfaces for continuous control of robots. Clin Neurophysiol (2008) 119(9):2159–69. doi:10.1016/j.clinph.2008.06.001
71. Irimia DC, Cho W, Ortner R, Allison BZ, Ignat BE, Edlinger G, et al. Brain-computer interfaces with multi-sensory feedback for stroke rehabilitation: a case study. Artif Organs (2017) 41(11):E178–84. doi:10.1111/aor.13054
72. Monge-Pereira E, Ibanez-Pereda J, Alguacil-Diego IM, Serrano JI, Spottorno-Rubio MP, Molina-Rueda F. Use of electroencephalography brain-computer interface systems as a rehabilitative approach for upper limb function after a stroke: a systematic review. PM R (2017) 9(9):918–32. doi:10.1016/j.pmrj.2017.04.016
73. Buch E, Weber C, Cohen LG, Braun C, Dimyan MA, Ard T, et al. Think to move: a neuromagnetic brain-computer interface (BCI) system for chronic stroke. Stroke (2008) 39(3):910–7. doi:10.1161/STROKEAHA.107.505313
74. Ramos-Murguialday A, Broetz D, Rea M, Laer L, Yilmaz O, Brasil FL, et al. Brain-machine interface in chronic stroke rehabilitation: a controlled study. Ann Neurol (2013) 74(1):100–8. doi:10.1002/ana.23879
75. Grosse-Wentrup M, Mattia D, Oweiss K. Using brain-computer interfaces to induce neural plasticity and restore function. J Neural Eng (2011) 8(2):025004. doi:10.1088/1741-2560/8/2/025004
76. Downey JE, Brane L, Gaunt RA, Tyler-Kabara EC, Boninger ML, Collinger JL. Motor cortical activity changes during neuroprosthetic-controlled object interaction. Sci Rep (2017) 7(1):16947. doi:10.1038/s41598-017-17222-3
77. van Gerven M, Farquhar J, Schaefer R, Vlek R, Geuze J, Nijholt A, et al. The brain-computer interface cycle. J Neural Eng (2009) 6(4):041001. doi:10.1088/1741-2560/6/4/041001
78. Holtzheimer PE, Husain MM, Lisanby SH, Taylor SF, Whitworth LA, McClintock S, et al. Subcallosal cingulate deep brain stimulation for treatment-resistant depression: a multisite, randomised, sham-controlled trial. Lancet Psychiatry (2017) 4(11):839–49. doi:10.1016/S2215-0366(17)30371-1
79. Martin S, Armstrong E, Thomson E, Vargiu E, Sola M, Dauwalder S, et al. A qualitative study adopting a user-centered approach to design and validate a brain computer interface for cognitive rehabilitation for people with brain injury. Assist Technol (2017):1–9. doi:10.1080/10400435.2017.1317675
80. Tonin L, Pitteri M, Leeb R, Zhang H, Menegatti E, Piccione F, et al. Behavioral and cortical effects during attention driven brain-computer interface operations in spatial neglect: a feasibility case study. Front Hum Neurosci (2017) 11:336. doi:10.3389/fnhum.2017.00336
81. Sitaram R, Ros T, Stoeckel L, Haller S, Scharnowski F, Lewis-Peacock J, et al. Closed-loop brain training: the science of neurofeedback. Nat Rev Neurosci (2017) 18(2):86–100. doi:10.1038/nrn.2016.164
82. Lubar JF. Neocortical dynamics: implications for understanding the role of neurofeedback and related techniques for the enhancement of attention. Appl Psychophysiol Biofeedback (1997) 22(2):111–26. doi:10.1023/A:1026276228832
83. Sueyoshi K, Sumiyoshi T. Electrophysiological markers of motivation in psychosis. Clin EEG Neurosci (2018) 49(1):8–11. doi:10.1177/1550059417745933
84. Dettmers C, Braun N, Busching I, Hassa T, Debener S, Liepert J. [Neurofeedback-based motor imagery training for rehabilitation after stroke]. Nervenarzt (2016) 87(10):1074–81. doi:10.1007/s00115-016-0185-y
85. Wang T, Mantini D, Gillebert CR. The potential of real-time fMRI neurofeedback for stroke rehabilitation: a systematic review. Cortex (2017). doi:10.1016/j.cortex.2017.09.006
86. Thakor NV. Translating the brain-machine interface. Sci Transl Med (2013) 5(210):210s217. doi:10.1126/scitranslmed.3007303
87. Kipke DR, Shain W, Buzsaki G, Fetz E, Henderson JM, Hetke JF, et al. Advanced neurotechnologies for chronic neural interfaces: new horizons and clinical opportunities. J Neurosci (2008) 28(46):11830–8. doi:10.1523/JNEUROSCI.3879-08.2008
88. Do AH, Wang PT, King CE, Abiri A, Nenadic Z. Brain-computer interface controlled functional electrical stimulation system for ankle movement. J Neuroeng Rehabil (2011) 8:49. doi:10.1186/1743-0003-8-49
89. Friedenberg DA, Schwemmer MA, Landgraf AJ, Annetta NV, Bockbrader MA, Bouton CE, et al. Neuroprosthetic-enabled control of graded arm muscle contraction in a paralyzed human. Sci Rep (2017) 7(1):8386. doi:10.1038/s41598-017-08120-9
90. Bouton CE, Shaikhouni A, Annetta NV, Bockbrader MA, Friedenberg DA, Nielson DM, et al. Restoring cortical control of functional movement in a human with quadriplegia. Nature (2016) 533(7602):247–50. doi:10.1038/nature17435
91. Wenger N, Moraud EM, Gandar J, Musienko P, Capogrosso M, Baud L, et al. Spatiotemporal neuromodulation therapies engaging muscle synergies improve motor control after spinal cord injury. Nat Med (2016) 22(2):138–45. doi:10.1038/nm.4025
92. Guggenmos DJ, Azin M, Barbay S, Mahnken JD, Dunham C, Mohseni P, et al. Restoration of function after brain damage using a neural prosthesis. Proc Natl Acad Sci U S A (2013) 110(52):21177–82. doi:10.1073/pnas.1316885110
93. Crema A, Malesevic N, Furfaro I, Raschella F, Pedrocchi A, Micera S. A wearable multi-site system for NMES-based hand function restoration. IEEE Trans Neural Syst Rehabil Eng (2017) 26(2):428–40. doi:10.1109/TNSRE.2017.2703151
94. Micera S, Navarro X, Carpaneto J, Citi L, Tonet O, Rossini PM, et al. On the use of longitudinal intrafascicular peripheral interfaces for the control of cybernetic hand prostheses in amputees. IEEE Trans Neural Syst Rehabil Eng (2008) 16(5):453–72. doi:10.1109/TNSRE.2008.2006207
95. Zrenner C, Eytan D, Wallach A, Thier P, Marom S. A generic framework for real-time multi-channel neuronal signal analysis, telemetry control, and sub-millisecond latency feedback generation. Front Neurosci (2010) 4:173. doi:10.3389/fnins.2010.00173
96. Donati AR, Shokur S, Morya E, Campos DS, Moioli RC, Gitti CM, et al. Long-term training with a brain-machine interface-based gait protocol induces partial neurological recovery in paraplegic patients. Sci Rep (2016) 6:30383. doi:10.1038/srep30383
97. Bundy DT, Souders L, Baranyai K, Leonard L, Schalk G, Coker R, et al. Contralesional brain-computer interface control of a powered exoskeleton for motor recovery in chronic stroke survivors. Stroke (2017) 48(7):1908. doi:10.1161/Strokeaha.116.016304
98. Frolov AA, Mokienko O, Lyukmanov R, Biryukova E, Kotov S, Turbina L, et al. Post-stroke rehabilitation training with a motor-imagery-based brain-computer interface (BCI)-controlled hand exoskeleton: a randomized controlled multicenter trial. Front Neurosci (2017) 11:400. doi:10.3389/fnins.2017.00400
99. Luu TP, He Y, Brown S, Nakagame S, Contreras-Vidal JL. Gait adaptation to visual kinematic perturbations using a real-time closed-loop brain-computer interface to a virtual reality avatar. J Neural Eng (2016) 13(3):036006. doi:10.1088/1741-2560/13/3/036006
100. Hubli M, Dietz V, Schrafl-Altermatt M, Bolliger M. Modulation of spinal neuronal excitability by spinal direct currents and locomotion after spinal cord injury. Clin Neurophysiol (2013) 124(6):1187–95. doi:10.1016/j.clinph.2012.11.021
101. Simonetti D, Zollo L, Milighetti S, Miccinilli S, Bravi M, Ranieri F, et al. Literature review on the effects of tDCS coupled with robotic therapy in post stroke upper limb rehabilitation. Front Hum Neurosci (2017) 11:268. doi:10.3389/fnhum.2017.00268
102. Wang W, Collinger JL, Perez MA, Tyler-Kabara EC, Cohen LG, Birbaumer N, et al. Neural interface technology for rehabilitation: exploiting and promoting neuroplasticity. Phys Med Rehabil Clin N Am (2010) 21(1):157–78. doi:10.1016/j.pmr.2009.07.003
103. Wessel MJ, Hummel FC. Non-invasive cerebellar stimulation: a promising approach for stroke recovery? Cerebellum (2017). doi:10.1007/s12311-017-0906-1
104. Huang H, Wolf SL, He J. Recent developments in biofeedback for neuromotor rehabilitation. J Neuroeng Rehabil (2006) 3:11. doi:10.1186/1743-0003-3-11
105. Giggins OM, Persson UM, Caulfield B. Biofeedback in rehabilitation. J Neuroeng Rehabil (2013) 10:60. doi:10.1186/1743-0003-10-60
106. Hu XL, Tong KY, Li R, Xue JJ, Ho SK, Chen P. The effects of electromechanical wrist robot assistive system with neuromuscular electrical stimulation for stroke rehabilitation. J Electromyogr Kinesiol (2012) 22(3):431–9. doi:10.1016/j.jelekin.2011.12.010
107. Semprini M, Cuppone AV, Delis I, Squeri V, Panzeri S, Konczak J. Biofeedback signals for robotic rehabilitation: assessment of wrist muscle activation patterns in healthy humans. IEEE Trans Neural Syst Rehabil Eng (2017) 25(7):883–92. doi:10.1109/TNSRE.2016.2636122
108. Stinear CM. Prediction of motor recovery after stroke: advances in biomarkers. Lancet Neurol (2017) 16(10):826–36. doi:10.1016/S1474-4422(17)30283-1
109. Burke E, Cramer SC. Biomarkers and predictors of restorative therapy effects after stroke. Curr Neurol Neurosci Rep (2013) 13(2):329. doi:10.1007/s11910-012-0329-9
110. Hsieh YW, Lin KC, Korivi M, Lee TH, Wu CY, Wu KY. The reliability and predictive ability of a biomarker of oxidative DNA damage on functional outcomes after stroke rehabilitation. Int J Mol Sci (2014) 15(4):6504–16. doi:10.3390/ijms15046504
111. Ciancarelli I, Di Massimo C, De Amicis D, Pistarini C, Tozzi Ciancarelli MG. Uric acid and Cu/Zn superoxide dismutase: potential strategies and biomarkers in functional recovery of post-acute ischemic stroke patients after intensive neurorehabilitation. Curr Neurovasc Res (2015) 12(2):120–7. doi:10.2174/1567202612666150311104900
112. Krebs HI, Krams M, Agrafiotis DK, DiBernardo A, Chavez JC, Littman GS, et al. Robotic measurement of arm movements after stroke establishes biomarkers of motor recovery. Stroke (2014) 45(1):200–4. doi:10.1161/STROKEAHA.113.002296
113. Mostafavi SM, Mousavi P, Dukelow SP, Scott SH. Robot-based assessment of motor and proprioceptive function identifies biomarkers for prediction of functional independence measures. J Neuroeng Rehabil (2015) 12:105. doi:10.1186/s12984-015-0104-7
Keywords: brain–computer interface, motor impairment, neurologic disorder, neuromodulation, personalization
Citation: Semprini M, Laffranchi M, Sanguineti V, Avanzino L, De Icco R, De Michieli L and Chiappalone M (2018) Technological Approaches for Neurorehabilitation: From Robotic Devices to Brain Stimulation and Beyond. Front. Neurol. 9:212. doi: 10.3389/fneur.2018.00212
Received: 22 December 2017; Accepted: 16 March 2018;
Published: 09 April 2018
Edited by:
Fabio Blandini, Fondazione Istituto Neurologico Nazionale Casimiro Mondino (IRCCS), ItalyReviewed by:
Stefano Tamburin, University of Verona, ItalyAlessio Baricich, Azienda Ospedaliero Universitaria Maggiore della Carita, Italy
Copyright: © 2018 Semprini, Laffranchi, Sanguineti, Avanzino, De Icco, De Michieli and Chiappalone. This is an open-access article distributed under the terms of the Creative Commons Attribution License (CC BY). The use, distribution or reproduction in other forums is permitted, provided the original author(s) and the copyright owner are credited and that the original publication in this journal is cited, in accordance with accepted academic practice. No use, distribution or reproduction is permitted which does not comply with these terms.
*Correspondence: Michela Chiappalone, bWljaGVsYS5jaGlhcHBhbG9uZUBpaXQuaXQ=
†Senior equal author contribution.