- 1Department of Radiology and Biomedical Imaging, University of California, San Francisco, San Francisco, CA, United States
- 2Departments of Neurology and Rehabilitation and Human Performance, Icahn School of Medicine at Mount Sinai, New York, NY, United States
- 3Brain Trauma Foundation, New York, NY, United States
- 4Department of Neurosurgery, Stanford University School of Medicine, Stanford, CA, United States
- 5Department of Bioengineering and Therapeutic Sciences, University of California, San Francisco, San Francisco, CA, United States
Concussion, or mild traumatic brain injury (mTBI), is a major public health concern, linked with persistent post-concussive syndrome, and chronic traumatic encephalopathy. At present, standard clinical imaging fails to reliably detect traumatic axonal injury associated with concussion and post-concussive symptoms. Diffusion tensor imaging (DTI) is an MR imaging technique that is sensitive to changes in white matter microstructure. Prior studies using DTI did not jointly investigate white matter microstructure in athletes, a population at high risk for concussive and subconcussive head traumas, with those in typical emergency room (ER) patients. In this study, we determine DTI scalar metrics in both ER patients and scholastic athletes who suffered concussions and compared them to those in age-matched healthy controls. In the early subacute post-concussion period, athletes demonstrated an elevated rate of regional decreases in axial diffusivity (AD) compared to controls. These regional decreases of AD were especially pronounced in the cerebellar peduncles, and were more frequent in athletes compared to the ER patient sample. The group differences may indicate differences in the mechanisms of the concussive impacts as well as possible compound effects of cumulative subconcussive impacts in athletes. The prevalence of white matter abnormality in cerebellar tracts lends credence to the hypothesis that post-concussive symptoms are caused by shearing of axons within an attention network mediated by the cerebellum, and warrant further study of the correlation between cerebellar DTI findings and clinical, neurocognitive, oculomotor, and vestibular outcomes in mTBI patients.
Introduction
Over 1.7 million Americans suffer a traumatic brain injury (TBI) each year (1). Moderate to severe TBI can often be diagnosed early through computed tomography (CT) and conventional magnetic resonance (MR) imaging. Concussion, or mild TBI (mTBI), however, makes up the great majority of TBI, but cannot be reliably detected by CT or conventional MR imaging techniques (2), which remain the standard of care.
The severity of a concussive injury is assessed by clinical evaluation of symptoms (3). Many concussed patients have symptoms including headaches, fatigue, insomnia, depression, attention problems, and memory problems (3), and while the majority recover within a few weeks, nearly a third continue to have persistent post-concussive symptoms (4–6). Current assessment does not reliably predict which mTBI patients will go on to suffer post-concussive symptoms (7); therefore, objective quantification of concussive injuries is needed.
Diffusion weighted imaging methods, including diffusion tensor imaging (DTI), provide useful tools for probing microstructural white matter changes in mTBI (2, 8). Traumatic axonal injury (TAI) may be inferred from CT or conventional MRI due to its association with small hemorrhages that those modalities can detect, but DTI can more directly detect changes in white matter microarchitecture (2). Past studies have found changes in DTI scalar metrics such as fractional anisotropy (FA), radial diffusivity (RD), and axial diffusivity (AD) in both acute and chronic mTBI patients, indicating microstructural alterations to white matter even in cases where CT and MRI scans were negative (7, 10). In the acute phase, FA has been seen to increase overall, while RD and AD have been seen to decrease. At chronic time points, the opposite effects are seen, with decreases in FA and increases in RD and AD (2, 9). Abnormal DTI scalar parameter values have been associated with cognitive functioning in mTBI patients (2, 8, 10, 11).
Prior DTI investigations of white matter microstructure have generally not studied different populations with concussions. However, athletes make up a population at high risk for concussive and subconcussive head traumas whose physical manifestations of injuries may differ from those due to mechanisms typically seen in the emergency room, such as motor vehicle collisions, assaults, and falls. The present study characterizes the early subacute abnormalities in DTI metrics in both athletes and ER patients after concussion, and compares the findings. In addition to the voxel-wise and tract-wise group comparisons of the conventional DTI literature, we also apply individual-patient tract-level analysis for greater clinical relevance and better robustness to the spatial heterogeneity of the white matter abnormalities associated with concussion (2, 7).
Methods
Subject Recruitment
This study was carried out in accordance with the recommendations of the institutional review board of the Weill Cornell Medical College (WCMC). All subjects gave written informed consent in accordance with the Declaration of Helsinki except, in the case of minors, legal guardians gave written informed consent with the assent of the subjects. The protocol was approved by the WCMC institutional review board, and data were collected from September of 2012 through September of 2016.
A concussion was defined as an event of blunt impact on the head with loss of consciousness (LOC), post-traumatic amnesia (PTA), or at least one of the following symptoms: dizziness, nausea, headaches, balance problems, blurred or double vision, or feeling dazed/confused. Although for the purpose of this research we did not rely on formal medical diagnosis of concussion necessary for clinical management of the injury (12), this definition is consistent with the guidance of the American Academy of Neurology (13). Eighteen scholastic athletes between ages 13 and 22 years were recruited for testing within 2 weeks of a concussion, as were 42 ER patients with concussion aged 7 years and older, of whom 18 were selected to match the age range of the athlete subjects. For the purpose of equity, athletes were enrolled independently of the level of contact involved in their participating sports. The recruited athlete and ER subjects were scanned for MR imaging as soon as possible. In addition, 38 control subjects aged 7 years and older with no prior history of head injury were recruited and received imaging, of whom 10 were selected to match the age range of the athlete subjects.
Subjects over 18 were required to have a high school diploma or GED; 18-year olds set to graduate high school on time were also included. Exclusion criteria for subjects were a prior history of eye disease, neurological/psychiatric conditions, or substance abuse (Table 1). Subjects with contraindications for an MRI were also excluded. For athletes and ER patients, additional exclusion criteria were acute intoxication at the time of the concussion and LOC or PTA for more than 24 h. Subjects' symptoms and cognitive performance were assessed with an extensive battery of tests as reported elsewhere (14, 15).
Magnetic Resonance Imaging
MR imaging was performed at WCMC on a 3T Siemens Trio scanner. In each imaging study, whole-brain diffusion imaging was performed using an echo-planar imaging sequence (TE = 85 ms, TR = 7,500 ms) with one b = 0 s/mm2 scan and b = 1,000 s/mm2 in 64 diffusion directions. Imaging was performed with 128 × 128 × 60 cubic voxels of 2 mm dimensions.
Image preprocessing was performed using tools within the Functional MRI of the Brain (FMRIB, Oxford University, Oxford, UK) Software Library (16–18). Correction for eddy currents and subject motion was performed and registered to the b = 0 s/mm2 volume using the FMRIB Linear Image Registration tool (19). Image volumes were checked for excessive subject movement between diffusion weighted images and were accepted if mean and median movement were <2 mm.
Non-brain voxels were then excluded using the FMRIB Brain Extraction tool (20). Using the diffusion-weighted data, a diffusion tensor model was generated using the FMRIB DTIFit algorithm, from which fractional anisotropy (FA), mean diffusivity (MD), radial diffusivity (RD), and axial diffusivity (AD) were determined at each voxel. Typical image quality is shown in Figure 1.
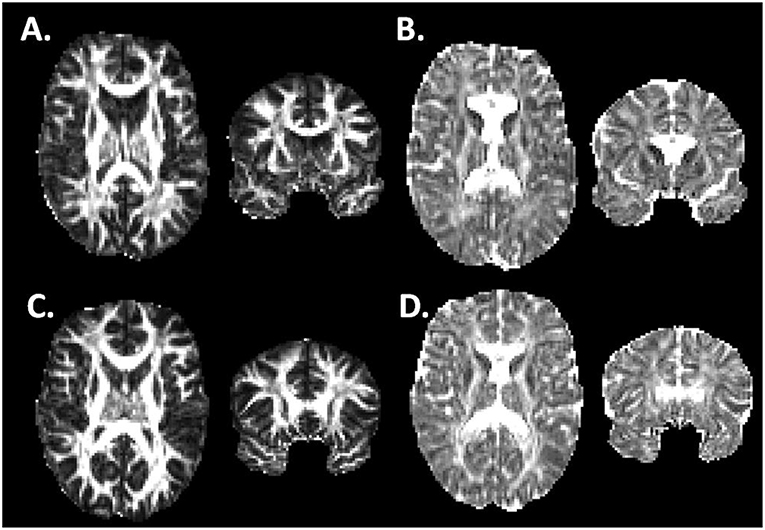
Figure 1. Fractional anisotropy and Axial Diffusivity in Athlete and Control Brains. (A,B) Fractional Anisotropy (A) and Axial Diffusivity (B) maps for one of the athlete subjects in horizontal and coronal views. (C,D) FA and AD maps for one of the control subjects in horizontal and coronal views. The imaging data has undergone image preprocessing, but has not yet undergone non-linear coregistration to a common template.
Tract-based Spatial Statistics (TBSS) were used to perform non-linear registration on the FA volumes to the FMRIB58_FA standard-space image, constructed from an average of 58 FA images taken of healthy subjects aged 20–50. After brain volumes were registered into a common space, a mean FA skeleton was generated using a threshold of FA ≥ 0.2 to limit the analysis to white matter voxels. TBSS alignment and white-matter skeleton generation was performed separately for each of the comparison groups (see Statistical Analyses) (21).
In addition to voxel-based analysis, masks were applied corresponding to the 27 white matter tracts previously labeled in the Johns Hopkins University (JHU) white-matter atlas (16), with bilateral tracts collapsed. Within each of these regions of interest (ROI), mean values for the four DTI scalar parameters were calculated for each subject.
Statistical Analyses
Using the FMRIB Software Library randomize tool, permutation tests (n = 5,000) were performed to evaluate significant differences between groups on a voxel-wise basis, using Threshold-Free Cluster Enhancement, with correction for family-wise error. For each comparison group, permutation tests were performed for FA, MD, RD, and AD to control for false-positive voxels (22).
Mean FA, MD, RD, and AD within each of the 27 white-matter ROIs were compared between groups with two-sided t-tests, with false-detection rate (FDR) correction for the multiple comparisons. Comparisons were also made between the groups in terms of the number of individuals with extremely high or low parameter values for each of the four DTI metrics in each white matter tract. For this purpose, an abnormal parameter value was defined as >2.2 control group standard deviations above or below the control mean, which is the threshold used by Yuh et al. (7) in their DTI study of acute mTBI in ER patients. Significance of these group differences were determined with Pearson's χ2 test.
Results
Subject Demographics
Table 2 summarizes characteristics of the athlete and ER subjects. In our dataset, patient, and control ages were imperfectly matched, while differences in gender were not significant. Despite the best effort to have the recruited athlete and ER subjects scanned for MR imaging quickly, the timing of imaging since concussion ranged from 3 to 23 days, with an overall mean (SD) of 9.9 (4.1) days. There was no statistical difference in the timing of imaging between the athlete and ER groups.
Voxel-Wise Group Comparisons
No significant differences between athlete and ER subjects were found in the voxel-wise comparison. For athletes, significant regional decreases were observed compared to controls in AD (Figure 2). These differences were seen in the uncinate fasciculus, external capsule, posterior limb of the internal capsule, and regions of the thalamus. All three of the cerebellar peduncles also saw significant decreases in AD.
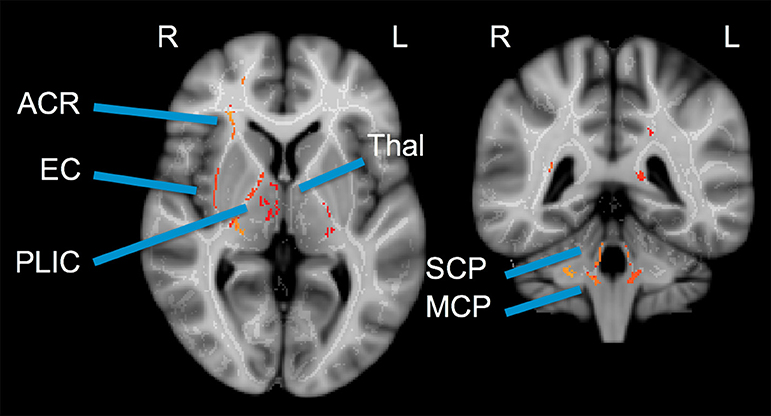
Figure 2. Cross-sectional voxel-wise comparison: Control > Patient Axial Diffusivity. Two cross-sections illustrating regions of significant decrease in athlete AD (acute post-injury time point) compared to controls. TBSS Analysis with Threshold-Free Cluster Enhancement FWE corrected at p < 0.025. ACR, Anterior Corona Radiata; EC, External Capsule; PLIC, Posterior Limb of Internal Capsule; Thal, Thalamus; SCP, Superior Cerebellar Peduncle; MCP, Medial Cerebellar Peduncle.
White Matter Tract Group Comparisons
Comparisons of white matter ROI means of DTI metrics demonstrated no significant group differences between athletes and controls or between ER patients and controls when adjusting for FDR (q = 0.2).
Outlier/Abnormal Tract Analysis Using Normative Control Subjects
For each athlete and control subject, the number of high outlier and low outlier regions were counted for each DTI parameter (Table 3). Athletes had more abnormally low FA and AD, and high RD ROIs. The finding of abnormal regional low AD was the only one which remained significant after applying a Bonferroni correction. In athletes, the most common tracts with abnormally low AD were the middle cerebellar peduncle (11 athletes), inferior cerebellar peduncle (8 athletes), superior cerebellar peduncle (8 athletes), uncinate fasciculus (7 athletes), and the superior fronto-occipital fasciculus (5 athletes).
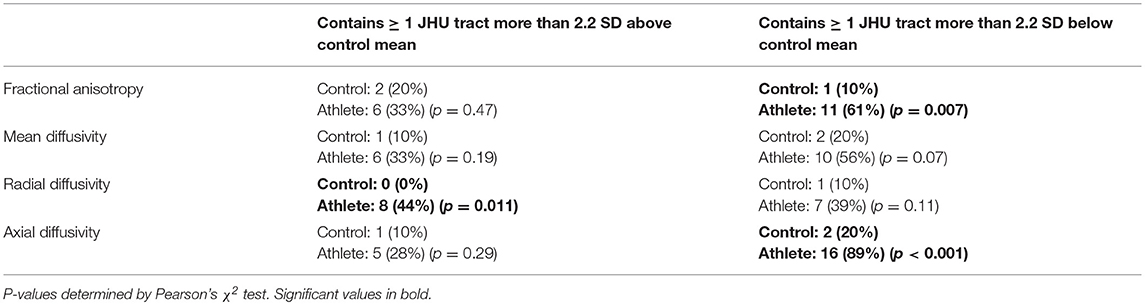
Table 3. Proportion of 18 athletes vs. 10 controls with one or more abnormally high/low DTI parameter value in a JHU atlas white matter tract.
The comparison of white matter ROIs in ER patients and controls showed similar trends, with significantly more ER patients demonstrating abnormally low FA and low AD in one or more ROIs compared to controls (Table 4). In ER patients, the most common tracts with abnormally low FA were the medial lemniscus (6 patients), posterior thalamic radiation (4 patients), and retrolenticular part of the internal capsule (4 patients). In ER patients, the most common tracts with abnormally low AD were the inferior cerebellar peduncle (6 patients), middle cerebellar peduncle (5 patients), and the uncinate fasciculus (4 patients).
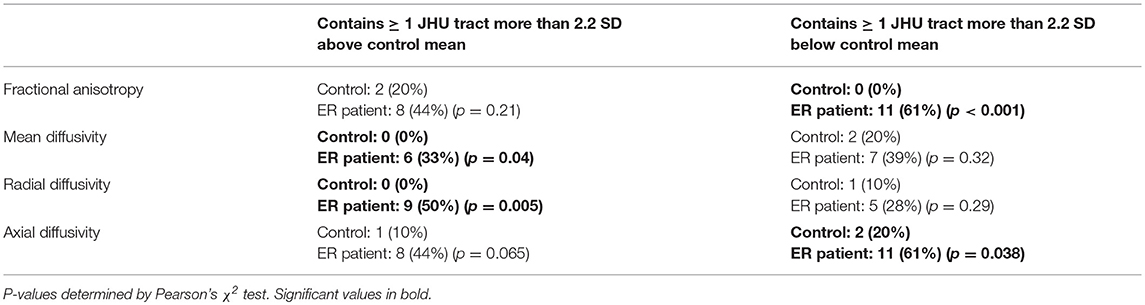
Table 4. Proportion of 18 ER patients vs. 10 controls with one or more abnormally high/low DTI parameter value in a JHU atlas white matter tract.
Athletes vs. ER Patients
Given the above results, we also compared the 18 athletes against the 18 ER patients. For the outlier analysis, we treated the ER patients as “controls” for determining means and standard deviations in ROI.
No significant differences were seen between the patient groups in voxel-wise or tract-wise group comparisons. However, the outlier analysis at the individual-subject level showed a significant number of athletes with one or more tracts exhibiting extremely low AD compared to the group of ER patients (Table 5). The athletes had the most low-AD counts in the fornix/stria terminalis (4 athletes), middle cerebellar peduncle (4 athletes), and the superior cerebellar peduncle (3 athletes).
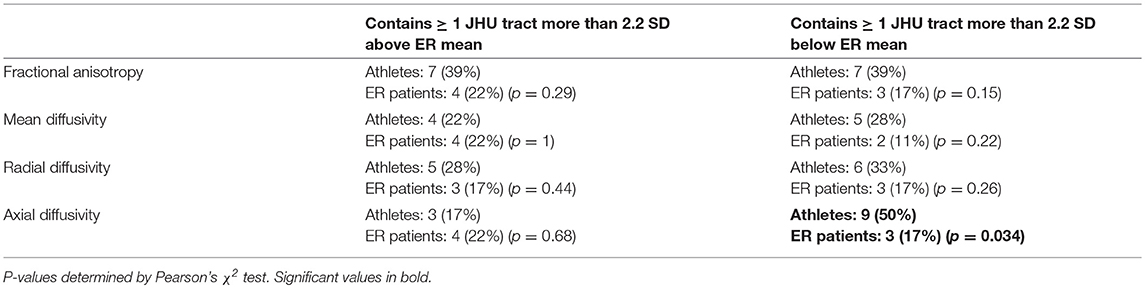
Table 5. Proportion of 18 athletes vs. 18 ER patients with one or more abnormally high/low DTI parameter value in a JHU atlas white matter tract.
Discussion
The present study shows early subacute post-concussion abnormalities in regional white matter microstructures in both scholastic athletes and ER patients. The abnormalities were observed as a greater frequency of extreme deviations in regionally defined DTI parameters, especially in the cerebellar peduncles of scholastic athletes, with our ROI-based outlier analysis. This method of analysis was modeled on that of (11) for chronic mTBI and Yuh et al. (7) for acute mTBI, in which high and low outliers in FA were compared between controls and patient subsets (7, 11). This method of classifying individual patients as having or lacking abnormal tracts can account for the spatial heterogeneity of mTBI, which often confounds group comparisons at both the voxel and tract spatial scales. This patient-specific analysis is also much more clinically relevant than findings at the group level.
Of particular note are our results demonstrating abnormally low AD both in the athlete and ER cohorts. The large majority of athletes had at least one white matter tract with a markedly low AD value, and while these low values were spatially heterogeneous, there was a striking consistency of having them in at least one of the three cerebellar peduncles. Some ER patients showed abnormally low AD values as well. Our direct comparison of ER patients and athletes suggests that those with sports concussions may be more prone to abnormalities in the cerebellar peduncles than typical ER concussion patients. The group differences may indicate differences in the mechanisms of the concussive impacts as well as possible compound effects of cumulative subconcussive impacts in athletes.
Our results are consistent with a prior DTI study showing decreases of white matter AD in male scholastic American football players at both 24 h and 8 days after concussion (23); however, that investigation focused on cerebral tracts and did not specifically investigate the cerebellum. A recent meta-analysis has shown variable findings from various DTI studies of sports concussions that may relate to differences in techniques and in the timing of imaging relative to the injury (24). Despite such differences, our study substantiates previous reports that suggest selective effects of TBI on the cerebellar peduncles—cerebellar white matter volume was found be reduced in children years after a TBI, implicating lasting cognitive and behavioral consequences (25), and FA alteration in cerebellum-related white matter tracts and associated cognitive deficits were found in acute-phase adult mTBI patients (26) and chronic phase combat veterans exposed to mTBI (27).
Neuropathological studies have shown cerebellar Purkinje cell loss in boxers (28) as well as in mice, non-human primates and combat veterans exposed to blast injuries (29, 30). The anatomic arrangement of the cerebellar peduncles as relatively unsupported bridges of white matter between the bulk of the cerebellum and the brainstem renders them uniquely vulnerable to shear stress from acceleration/deceleration effects, as shown in biomechanical studies (31–35).
These findings in the cerebellar peduncles provide some support to the hypothesis of predictive brain state disruption in TBI (36). By this hypothesis, the collection of clinically observed post-concussive symptoms could be explained by timing-related disruptions in an attention network mediated by the cerebellum (36), which was supported by a study that combined DTI, neurocognitive tests, and eye movement measurements (37). It should be noted that our analysis was data-driven across all of the major white matter tracts of the cerebrum and cerebellum; therefore, it was not influenced by any hypothesis regarding the location of injury such as the cerebellar peduncles. To further test the predictive brain state hypothesis, future studies can and should attempt to correlate cerebellar DTI findings to clinical, neurocognitive, oculomotor, and vestibular outcomes relevant to cerebellar function. This can pave the way toward targeted rehabilitation strategies aimed at improving the affected domains, especially attentional function which is one of the most disabling impairments in activities of daily living and can be ameliorated using goal-oriented mindfulness training regimens (38, 39).
A concern specific to our dataset is the wide window of “early subacute” post-concussion scan latencies. The differences between DTI parameters 3 days and 23 days after a concussion could be significant (9). The ROI-based outlier analysis proved to be a useful workaround for the range and variability in the timing of MR scan. Another limitation of the present study is that age matching between patients and controls was imperfect. On the whole, the control cohort was older than the concussion cohorts, as the total pool of control subjects was small. Since there are non-linear age-dependent changes in DTI measures that are also region-dependent, controlling for age by statistical regression is difficult (40). A linear regression, even done on a tract-by-tract basis, would not add any clarity to the results. Therefore, we did not attempt to use regression to adjust for age differences. However, the average age difference between the controls and mTBI patients is not expected to produce meaningful differences in the DTI measures, especially for most of the white matter tracts shown here to be significantly different between mTBI subjects and controls. Of special note, cerebellar white matter consists of early-maturing tracts (41) that reach their asymptotic DTI metric values during the first decade of life, with no significant changes in FA or MD during the rest of childhood or adolescence (42, 43). In particular, DTI metrics of all three cerebellar peduncles bilaterally plateau at 70 months of age (44). Therefore, no age-related changes in the cerebellar peduncles would be expected in the range of 13–25 years of the subject groups that we studied. Instead, any age-related differences between groups would have been observed in slower-maturing cerebral white matter, such as that of the frontal lobes. Finally, overall sample size was small. A larger statistical power may reveal more subtle group differences.
We did not exclude patients based on lesions that would be visible on routine clinical MRI. However, these otherwise healthy young athletes suffered concussions that rarely produce visible brain lesions, especially not in the cerebellum. Also, as our results are not explained by one or two outliers, we believe our results cannot be explained by lesions that could be detected by routine clinical MRI.
In designing future studies of athlete populations, it would perhaps be best to design image acquisition protocols that allow for more advanced diffusion models than DTI. For instance, neurite orientation dispersion and density imaging (NODDI) uses high angular resolution diffusion data with multiple b-values, and applies a three-compartment model that takes into account the neural microstructure in describing the diffusion pattern within a voxel (45). The NODDI model rests on certain assumptions regarding normal brain physiology, so in studying pathology such as mTBI, it is possible that the resulting NODDI parameters do not accurately describe the underlying brain architecture. However, Palacios et al have recently shown greater sensitivity in mTBI when studying changes in NODDI parameters compared to the DTI parameters analyzed in this study (46).
Ethics Statement
This study was carried out in accordance with the recommendations of the institutional review board of the Weill Cornell Medical College (WCMC). All subjects gave written informed consent in accordance with the Declaration of Helsinki except, in the case of minors, legal guardians gave written informed consent with the assent of the subjects. The protocol was approved by the WCMC institutional review board.
Author Contributions
JM, JG, and PM: conception and design. JM and JG: acquisition. JMM, EP, and PM: analysis. JMM, EP, JM, JG, and PM: interpretation.
Funding
This work was supported by U.S. Department of Defense contracts W911QY-12-C-0005 and W911QY-14-C-0086.
Conflict of Interest Statement
JG is director of Sync-Think, Inc., and the inventor of U.S. patent 7,384,399. JM holds stock option in Sync-Think. JG and JM are inventors of pending patent applications PCT/US2014/050774, PCT/US2016/027923, and US15585057 potentially related to the subject matter described in this article. PM declares research support from GE Healthcare as well as service on the Medical Advisory Board of the GE-NFL Head Health Initiative.
The remaining authors declare that the research was conducted in the absence of any commercial or financial relationships that could be construed as a potential conflict of interest.
Acknowledgments
The authors thank Brain Trauma Foundation's clinical research staff and the staff of the Citigroup Biomedical Imaging Center at Weill Cornell Medicine for assistance with data collection and maintenance. The content of this paper is adapted from author JMM's graduate thesis.
References
1. Faul M, Xu L, Wald M, Coronado V. Traumatic Brain Injury in the United States: Emergency Department Visits, Hospitalizations and Deaths 2002–2006. Atlanta, GA: Centers for Disease Control and Prevention, National Center for Injury Prevention and Control (2010).
2. Niogi SN, Mukherjee P. Diffusion tensor imaging of mild traumatic brain injury. J Head Trauma Rehabil. (2010) 25:241–55. doi: 10.1097/HTR.0b013e3181e52c2a
3. Gardner RC, Yaffe K, Yaffe K. Epidemiology of mild traumatic brain injury and neurodegenerative disease. Mol Cell Neurosci. (2015) 66:75–80. doi: 10.1016/j.mcn.2015.03.001
4. Bigler E. Neuropsychology and clinical neuroscience of persistent post-concussive syndrome J Int Neuropsychol Soc. (2008) 14:1–22. doi: 10.1017/S135561770808017X
5. McCrea M, Guskiewicz K, Marshall S, Barr W, Randolph C, Cantu R, et al. Acute effects and recovery time following concussion in collegiate football players: the NCAA Concussion Study. JAMA. (2003) 290:2556–63. doi: 10.1001/jama.290.19.2556
6. McMahon P, Hricik A, Yue J, Puccio A, Inoue T, Lingsma H, et al. Symptomatology and functional outcome in mild traumatic brain injury: results from the prospective TRACK-TBI study J Neurotrauma. (2014) 31:26–33. doi: 10.1089/neu.2013.2984
7. Yuh E, Cooper S, Mukherjee P, Yue J, Lingsma H, Gordon W, et al. Diffusion tensor imaging for outcome prediction in mild traumatic brain injury: a TRACK-TBI study. J Neurotrauma. (2014) 31:1457–77. doi: 10.1089/neu.2013.3171
8. Oehr L, Anderson J. Diffusion-tensor imaging findings and cognitive function following hospitalized mixed-mechanism mild traumatic brain injury: a systematic review and meta-analysis Arch Phys Med Rehabil. (2017) 98:2308–19. doi: 10.1016/j.apmr.2017.03.019
9. Eierud C, Craddock R, Fletcher S, Aulakh M, King-Casas B, Kuehl D, et al. Neuroimaging after mild traumatic brain injury: review and meta-analysis Neuro Clin. (2014) 4:283–94. doi: 10.1016/j.nicl.2013.12.009
10. Niogi S, Mukherjee P, Ghajar J, Johnson C, Kolster R, Lee H, et al. Structural dissociation of attentional control and memory in adults with and without mild traumatic brain injury. Brain. (2008) 131:3209–21. doi: 10.1093/brain/awn247
11. Niogi S, Mukherjee P, Ghajar J, Johnson C, Kolster R, Sarkar R, et al. Extent of microstructural white matter injury in post-concussive syndrome correlates with impaired cognitive reaction time: a 3T diffusion tensor imaging study of mild traumatic brain injury. Am J Neuroradiol. (2008) 29:967–73. doi: 10.3174/ajnr.A0970
12. McCrory P, Meeuwisse W, Dvorak J, Aubry M, Bailes J, Broglio S, et al. Consensus statement on concussion in sport-the 5th international conference on concussion in sport held in Berlin, October 2016. Br J Sports Med. (2017) 51:838–47. doi: 10.1136/bjsports-2017-097699
13. Giza C, Kutcher J, Ashwal S, Barth J, Getchius T, Gioia G, et al. Summary of evidence-based guideline update: evaluation and management of concussion in sports: report of the guideline development subcommittee of the american academy of neurology Neurology. (2013) 80:2250–7. doi: 10.1212/WNL.0b013e31828d57dd
14. Maruta J, Lumba-Brown A, Ghajar J. Concussion subtype identification with the rivermead post-concussion symptoms questionnaire. Front Neurol. (2018) 9:1034. doi: 10.3389/fneur.2018.01034
15. Maruta J, Spielman L, Rajashekar U, Ghajar J. Association of visual tracking metrics with post-concussion symptomatology. Front Neurol. (2018) 9:611. doi: 10.3389/fneur.2018.00611
16. Mori S, Wakana S, van Zijl P, Nagae-Poetscher L. MRI Atlas of Human White Matter. Amsterdam: Elsevier (2005).
17. Wakana S, Caprihan A, Panzenboeck M, Fallon J, Perry M, Gollub R, et al. Reproducibility of quantitative tractography methods applied to cerebral white matter. NeuroImage. (2007) 36:630–44. doi: 10.1016/j.neuroimage.2007.02.049
18. Hua K, Zhang J, Wakana S, Jiang H, Reich D, Calabresi P, et al. Tract probability maps in stereotaxic spaces: analysis of white matter anatomy and tract-specific quantification. NeuroImage. (2008) 39:336–47. doi: 10.1016/j.neuroimage.2007.07.053
19. Andersson J, Skare S, Ashburner J. How to correct susceptibility distortions in spin-echo echo-planar images: application to diffusion tensor imaging NeuroImage. (2003) 20:870–88. doi: 10.1016/S1053-8119(03)00336-7
20. Smith S. Fast robust automated brain extraction. Hum Brain Mapp. (2002) 17:143–55. doi: 10.1002/hbm.10062
21. Smith S, Jenkinson M, Johansen-Berg H, Rueckert D, Nichols T, Mackay C, et al. Tract-based spatial statistics: voxelwise analysis of multi-subject diffusion data. NeuroImage. (2006) 31:1487–505. doi: 10.1016/j.neuroimage.2006.02.024
22. Winkler A, Ridgway G, Webster M, Smith S, Nichols T. Permutation inference for the general linear model. NeuroImage. (2014) 92:381–97. doi: 10.1016/j.neuroimage.2014.01.060
23. Lancaster M, Olson D, McCrea M, Nelson L, AA L, Muftuler L. Acute white matter changes following sport-related concussion: a serial diffusion tensor and diffusion kurtosis tensor imaging study. Hum Brain Mapp. (2016) 37:3821–34. doi: 10.1002/hbm.23278
24. Kamins J, Bigler E, Covassin T, Henry L, Kemp S, Leddy J, et al. What is the physiological time to recovery after concussion? A systematic review. Br J Sports Med. (2017) 51:935–40. doi: 10.1136/bjsports-2016-097464
25. Spanos GE. Cerebellar atrophy after moderate-to-severe pediatric traumatic brain injury Am. J. Neuroradiol. (2007) 28:537–42. Available online at: http://www.ajnr.org/content/28/3/537.long
26. Wang ZE. Altered cerebellar white matter integrity in patients with mild traumatic brain injury in the acute stage. PLoS ONE. (2016) 11:e0151489. doi: 10.1371/journal.pone.0151489
27. MacDonald C, Johnson A, Cooper D, Malone T, Sorrell J, Shimony J, et al. Cerebellar white matter abnormalities following primary blast injury in US military personnel. PLOS ONE. (2013) 8:e55823. doi: 10.1371/journal.pone.0055823
28. Corsellis J, Bruton C, Freeman Browne D. The aftermath of boxing. Psychol Med. (1973) 3:270–303. doi: 10.1017/S0033291700049588
29. Lu J, Ng K, Ling G, Wu J, Poon D, Kan E, et al. Effect of blast exposure on the brain structure and cognition in Macaca fascicularis. J Neurotrauma. (2012) 29:1434–54. doi: 10.1089/neu.2010.1591
30. Meabon J, Huber B, Cross D, Richards T, Minoshima S, Pagulayan K, et al. Repetitive blast exposure in mice and combat veterans causes persistent cerebellar dysfunction Sci Transl Med. (2016) 8:321. doi: 10.1126/scitranslmed.aaa9585
31. Lampert P, Hardman J. Morphological changes in brains of boxers. JAMA. (1984) 251:2676–9. doi: 10.1001/jama.1984.03340440034023
32. Viano D, Casson I, Pellman E, Zhang L, King A, Yang K. Concussion in professional football: brain responses by finite element analysis: part 9. Neurosurgery. (2005) 57:891–915. doi: 10.1227/01.NEU.0000186950.54075.3B
33. Taylor P, Ford C. Simulation of blast-induced early-time intracranial wave physics leading to traumatic brain injury. J Biomech Eng. (2009) 131:061007. doi: 10.1115/1.3118765
34. Krave U, Al-Olama M, Hansson H. Rotational acceleration closed head flexion trauma generates more extensive diffuse brain injury than extension trauma. J Neurotrauma. (2011) 28:57–70. doi: 10.1089/neu.2010.1431
35. Goldstein L, Fisher A, Tagge C, Zhang X, Velisek L, Sullivan J, et al. Chronic traumatic encephalopathy in blast-exposed military veterans and a blast neurotrauma mouse model. Sci Transl Med. (2012) 4:134ra160. doi: 10.1126/scitranslmed.3003716
36. Ghajar J, Ivry R, Consortium CA. The predictive brain state: timing deficiency in traumatic brain injury? Neurorehabil Neural Repair. (2008) 22:217–27. doi: 10.1177/1545968308315600
37. Maruta J, Suh M, Niogi S, Mukherjee P, Ghajar J. Visual tracking synchronization as a metric for concussion screening J Head Trauma Rehabil. (2010) 25:293–305. doi: 10.1097/HTR.0b013e3181e67936
38. Chen A, Novakovic-Agopian T, Nycum T, Song S, Turner G, Hills N, et al. Training of goal-directed attention regulation enhances control over neural processing for individuals with brain injury. Brain. (2011) 134:1541–54. doi: 10.1093/brain/awr067
39. Novakovic-Agopian T, Kornblith E, Abrams G, Burciaga-Rosales J, Loya F, D'Esposito M, et al. Training in goal-oriented attention self-regulation improves executive functioning in veterans with chronic traumatic brain injury. J Neurotrauma. (2018) 35:2784–95. doi: 10.1089/neu.2017.5529
40. Lebel C, Beaulieu C. Longitudinal development of human brain wiring continues from childhood into adulthood. J Neurosci. (2011) 31:10937–47. doi: 10.1523/JNEUROSCI.5302-10.2011
41. Deoni S, Dean D, O'Muircheartaigh J, Dirks H, Jerskey B. Investigating white matter development in infancy and early childhood using myelin water faction and relaxation time mapping. NeuroImage. (2012) 63:1038–53. doi: 10.1016/j.neuroimage.2012.07.037
42. Travis K, Leitner Y, Feldman H, Ben-Shachar M. Cerebellar white matter pathways are associated with reading skills in children and adolescents. Hum Brain Mapp. (2015) 36:1536–53. doi: 10.1002/hbm.22721
43. Leitner Y, Travis K, Ben-Shachar M, Yeom K, Feldman H. Tract profiles of the cerebellar white matter pathways in children and adolescents. Cerebellum. (2015) 14:613–23. doi: 10.1007/s12311-015-0652-1
44. Bruckert L, Shpanskaya K, McKenna E, Borchers L, Yablonski M, Blecher T, et al. Age-dependent white matter characteristics of the cerebellar peduncles from infancy through adolescence. Cerebellum. (2019). doi: 10.1007/s12311-018-1003-9. [Epub ahead of print].
45. Zhang H, Schneider TCA, Alexander D. NODDI: practical in vivo neurite orientation dispersion and density imaging of the human brain. Neuroimage. (2012) 61:1000–16. doi: 10.1016/j.neuroimage.2012.03.072
Keywords: magnetic resonance, diffusion weighted imaging, acquired brain injury, fractional anisotropy, tract-based spatial statistics
Citation: Mallott JM, Palacios EM, Maruta J, Ghajar J and Mukherjee P (2019) Disrupted White Matter Microstructure of the Cerebellar Peduncles in Scholastic Athletes After Concussion. Front. Neurol. 10:518. doi: 10.3389/fneur.2019.00518
Received: 04 February 2019; Accepted: 01 May 2019;
Published: 15 May 2019.
Edited by:
Richard J. Servatius, Syracuse VA Medical Center, United StatesReviewed by:
Carlo Augusto Mallio, Campus Bio-Medico University, ItalyKimbra Kenney, Uniformed Services University of the Health Sciences, United States
Copyright © 2019 Mallott, Palacios, Maruta, Ghajar and Mukherjee. This is an open-access article distributed under the terms of the Creative Commons Attribution License (CC BY). The use, distribution or reproduction in other forums is permitted, provided the original author(s) and the copyright owner(s) are credited and that the original publication in this journal is cited, in accordance with accepted academic practice. No use, distribution or reproduction is permitted which does not comply with these terms.
*Correspondence: Pratik Mukherjee, cHJhdGlrLm11a2hlcmplZUB1Y3NmLmVkdQ==