- 1Department of Neuromedicine and Movement Science, Faculty of Medicine and Health Sciences, Norwegian University of Science and Technology, Trondheim, Norway
- 2Department of Neurology, St. Olav's Hospital, Trondheim University Hospital, Trondheim, Norway
- 3Department of Pharmacology and Clinical Neurosciences, Division of Neuro, Head, and Neck, Umeå University Hospital, Umeå, Sweden
Recent advances in cell reprogramming technologies enable the in vitro generation of theoretically unlimited numbers of cells, including cells of neural lineage and specific neuronal subtypes from human, including patient-specific, somatic cells. Similarly, as demonstrated in recent animal studies, by applying morphogenetic neuroengineering principles in situ, it is possible to reprogram resident brain cells to the desired phenotype. These developments open new exciting possibilities for cell replacement therapy in stroke, albeit not without caveats. Main challenges include the successful integration of engineered cells in the ischemic brain to promote functional restoration as well as the fact that the underlying mechanisms of action are not fully understood. In this review, we aim to provide new insights to the above in the context of connectomics of morphogenetically engineered neural networks. Specifically, we discuss the relevance of combining advanced interdisciplinary approaches to: validate the functionality of engineered neurons by studying their self-organizing behavior into neural networks as well as responses to stroke-related pathology in vitro; derive structural and functional connectomes from these networks in healthy and perturbed conditions; and identify and extract key elements regulating neural network dynamics, which might predict the behavior of grafted engineered neurons post-transplantation in the stroke-injured brain.
Introduction
Stem cell therapy for ischemic stroke may extend the therapeutic window from the acute into the sub-acute and chronic stage. As such, it is a particularly interesting approach, considering that more than 80% of stroke patients are de facto not eligible for the standard clinical treatment options, i.e., thrombolysis or thrombectomy (1). Numerous animal studies have demonstrated the potential of stem cell therapy alone, or in combination with in situ tissue engineering strategies and/or pharmacotherapeutics, for promoting functional restoration after stroke. The rationale behind stem cell therapy is that it can promote functional recovery through a range of possible mechanisms of action, including neuroprotection, modulation of the inflammatory response, cell replacement, remyelination, tissue/vascular remodeling and de novo neurogenesis (2–8). Although promising and valuable in the quest of elucidating relevant recovery mechanisms at the experimental level, clinical translation of stem cell-based approaches tends to be largely confounded by significant challenges. These include poor graft survival and integration with the host tissue, which indeed is hardly surprising, given the intricacies inherent in aspiring to create safe, functional in situ biointerfaces that could effectively re-establish functional connectivity in multiple foci (9–12). As a result, numerous past and ongoing clinical trials of various stem cell-based therapies have largely failed to fully confirm preclinical findings by promoting significant and/or long-lasting gain of motor, sensory, or cognitive function in stroke patients (10, 11, 13–15).
Apart from the conceivable limitations of extrapolating findings from animal studies to the clinic, a substantial barrier to clinical translation is the lack of a thorough understanding of the underlying mechanisms determining cell graft behavior post-transplantation in the ischemic brain. This is further confounded by the intrinsic complexity of stroke lesions and, with regard to cell replacement and functional integration of the engrafted cells, also the lack of direct empirical evidence supporting neuronal replacement in human patients (1).
It is also worth considering the potential role of endogenous plasticity in response to ischemic injury, not least, the manner in which associated mechanisms may influence transplant behavior (16). Plastic responses after stroke can be demonstrated as neurogenic niche activation and cytogenesis in the ipsilesional brain hemisphere, as well as rewiring of surviving neural networks and recruitment of intact synapses contralateral, but also ipsilateral to the lesion (17–22). Such plastic responses and, not least, concomitant inflammatory processes triggered by brain ischemia, may conceivably play a significant role in determining functional outcomes after stroke (12, 23, 24). The underlying mechanisms regulating such responses are however unclear and, consequently difficult to harness in an appropriate or timely manner. Furthermore, the fact that intrinsic neuroplasticity can be adaptive, but also maladaptive, suggests that any attempt to harness it that fails to fully comprehend or suitably engage fundamental underlying mechanisms may inadvertently exacerbate lesion-induced deficits rather than contribute toward functional restoration.
Several questions therefore arise that warrantee rigorous discussion in the scientific community. In this review, we aim to contribute to this discussion by providing a concise overview of novel and emerging theoretical and methodological perspectives which may be significant for improving the robustness of pre-clinical studies with a view to clinical translation. Specifically, we propose the investigation of in vitro engineered neural networks in the context of connectomics and discuss how morphogenetic neuroengineering can be supported by advanced interdisciplinary approaches, including in vitro electrophysiology, microfluidics, and computational modeling, to obtain robust preclinical models that can promote our understanding of cell replacement therapy for stroke beyond the state-of-the-art.
Morphogenetic Neuroengineering
The differentiation and development of projection neurons in the mammalian neocortex is regulated by tight molecular control, which determines and orchestrates sequential cell fate decisions over different temporospatial scales [reviewed in Greig et al. (25)]. An understanding of fundamental mechanisms regulating these processes led to the development of morphogenetic neuroengineering approaches based on controlled expression of transcription factors (26, 27). In 2002, a proof-of-principle study by the Göetz lab demonstrated that the Pax6 gene controls the neurogenic potential of radial glia, and also that its forced expression can induce astrocytes to a neuronal fate in vitro (28). A few years later, the same group showed that upregulation of Olig2 expression after brain injury, including focal ischemia, suppresses Pax6 expression, while in vivo transduction with a dominant-negative form of Olig2 in the acute and chronic phase after injury can induce neurogenesis in the neocortex (29).
A major barrier in morphogenetic neuroengineering with a view to clinical translation for cell replacement therapy was broken by Sinya Yamanaka's research. In a seminal paper in 2006, Yamanaka and Takahashi demonstrated conversion of adult somatic cells into induced pluripotent stem cells (iPSCs) after transduction with only four genes (Oct4, Sox2, Klf4 and c-Myc) (30). This method paved the way toward autologous sourcing and generation of theoretically unlimited numbers of stem cells applicable to a range of possible therapeutic approaches in regenerative medicine, including treatment of central nervous system (CNS) lesions, such as ischemic stroke.
Recent advances in cell reprogramming technologies have led to the development of efficient, reproducible, high-yield protocols for the generation of iPSCs and induced neural stem cells (iNSCs), as well as protocols for the generation of induced neurons (iNs) and specific neuronal subtypes, including spinal motoneurons, dopaminergic, cholinergic, and medium spiny neurons, and also cortical neurons, by direct conversion of somatic cells (31–37); reviewed in Gascon et al. (38). Such direct conversion protocols bypass the pluripotency state through forced expression of lineage-specific transcription factors regulating brain development and may also effectively preserve the age-related and epigenetic imprint of the cell (31, 39). Similar principles can be applied for direct reprogramming of glia, including astrocytes, NG2 glia, microglia, and pericytes, into neurons (40–44). Interestingly, a number of studies have also demonstrated in vivo reprogramming of resident brain and spinal cord astrocytes and endogenous neural progenitors into neurons (44–51).
Reprogramming through the iPSC/iNSC state or by direct conversion is highly relevant for cell-based therapies for brain ischemia. A number of studies have demonstrated the potential of iPSC and iNSC-derived neurons for cell replacement therapy in stroke (4, 52). Furthermore, neurons derived from immature progenitors and pluripotent cells have the potential to establish long-range target-specific functional connections in the adult mammalian CNS (49, 53–57) reviewed in Wuttke et al. (58).
However, unequivocal evidence of functional integration of iPSC/iNSC derived neurons in the ischemic brain post transplantation is scant, not least regarding the potential of these cells to establish long-term efferent connections in a target-specific manner (1). Tornero and co-authors were the first to show that cortical neuronal progenitors, differentiated from human fibroblast-derived iPSCs, survive after intracortical transplantation in the rat brain after stroke and also receive afferent inputs, as suggested by observed monosynaptic responses from the grafted neurons after stimulation of the intact cortical region adjacent to the graft (59). Furthermore, in a recent study, the same group demonstrated that transplanted iNSC-derived neurons can functionally integrate with the local thalamocortical circuitry and receive direct synaptic inputs from the relevant brain regions (60).
Taken together, such evidence suggests that the state-of-the-art morhogenetic neuroengineering approaches for the generation of neuronal types relevant for cell replacement therapy in stroke hold promise. Their potential for clinical translation, however, is not without caveats. A fundamental, currently unresolved, question as whether transplanted engineered neurons can promote improved functional outcomes after stroke is the extent to which they can morphologically and functionally integrate with the host tissue.
Several related questions arise: Do engineered neurons have the capacity to establish efferent connections, in addition to afferent ones, post-transplantation? If so, where in the brain are transplanted neurons likely to project and form synapses? How does the transplant respond to evolving stroke related pathology, including the spontaneous reorganization of the relevant neural circuitry after the injury, as well associated dynamic changes at the micro-, meso-, and macroscale? Is it possible to anticipate such responses and, importantly, predict whether functional integration of the transplanted neurons in the host brain will elicit adaptive or maladaptive processes?
Connectomics of the Healthy and Lesioned Brain
Adaptive and Maladaptive Neuroplasticity
To achieve therapeutic interventions for stroke beyond the state-of-the-art we need to consider stem cell therapy in the context of the extremely complex, highly interconnected structural and functional circuitry of the human brain, especially the altered, highly plastic, structure-function relationships triggered by an ischemic lesion. Endogenous neuroplasticity after stroke and its relevance for the functional integration of morphogenetically engineered transplants to elicit recovery of motor, sensory, or cognitive deficits as a result of brain ischemia, can be briefly discussed in the context of Hebbian and homeostatic mechanisms.
During development, the CNS is characterized by a high level of plasticity, where functional brain networks and circuits are shaped by Hebbian mechanisms, such as long-term potentiation (LTP) and long-term depression (LTD) (61, 62) constituting the synaptic basis of associative learning (63). These processes are regulated by evolutionarily conserved mechanisms and involve functional and structural changes which occur in a well-orchestrated spatiotemporal manner. However, once development has been completed, Hebbian mechanisms alone cannot explain how the mature brain maintains normal function. In the same manner, Hebbian laws per se cannot sufficiently explain plastic network responses, such as synaptic scaling (64, 65), which are observed following externally induced perturbations. This suggested that there may be another form of plasticity underlying such responses. Compelling experimental evidence over the last two decades supports that another form of plasticity, i.e., homeostatic plasticity, does exist (64, 66–69). Indeed, homeostatic plasticity operates in tandem with Hebbian plasticity along complex temporal and spatial scales and serves to maintain normal neural network function as well as regulate network responses to perturbation (67, 70).
It is well-documented that any major disturbance to normal neural network function tends to result in severe, permanent functional deficits, but also trigger adaptive and maladaptive network responses, which are demonstrated as changes in network structure and function (12, 18, 19, 66, 69–74). Such alterations may represent various underlying forms of neuroplasticity, which involve both homeostatic and Hebbian mechanisms (67, 75, 76), and which can be described as morphology-activity, (i.e., structure-function) relationships. Such relationships are highly complex and, as a result, poorly understood. However, elucidation of how different forms of plasticity may result in adaptive or maladaptive neural network responses and, thereby, how such processes may promote or hinder functional restoration after a perturbation such as stroke is of fundamental importance for our ability to achieve functional repair.
As mentioned earlier, plastic responses to an ischemic event include spontaneous functional recovery (77), rewiring of surviving neuronal networks and axonal ramification, and the recruitment of intact synapses post-lesioning (78), demonstrated as intra- and interhemispheric neural network remodeling (74). Furthermore, there is increasing consensus as to the likelihood that such responses are also influenced by evolving inflammatory processes (12). The underlying mechanisms regulating such responses largely involve structural as well as functional changes in brain circuits closely associated with the ones directly affected by stroke. These circuits can further be engaged and modified through experience-dependent plasticity (17, 79).
It is a reasonable assumption to make that there is a critical period of increased neuroplasticity after stroke, which can be harnessed to promote functional recovery, as exemplified in a number of preclinical as well as clinical studies (12, 17, 77, 80–84). Moreover, recent evidence suggests that recovery after stroke can be mediated through the engagement of neural networks in a functional hierarchy both in the contralesional as well as ipsilesional brain hemisphere and also upstream as well as downstream of the lesion (85). Furthermore, it is becoming increasingly evident that any cortical remapping that may occur after stroke is both activity dependent, but also based on competition (86).
Neural Transplants as Part of the Brain Connectome
Where does cell replacement therapy fit in this context? To seek answers to this question, it is important to consider the behavior of engineered neural transplants in the ischemic brain in terms of connectomes (87, 88) i.e., the changing structure-function relationships and complex interplay between affected and intact neural ensembles in the brain in the context of evolving stroke pathology.
Highly relevant insights as to how the brain connectomes underpin the behavior of a neural system and determine functional outcome after a perturbation can be found in an excellent review by Fornito and co-authors (89). In this paper, the authors discuss how the highly interconnected, complex topology of the neural architecture of the brain, which is characterized by a fine, coordinated balance between regional segregation and specialization of function, but also highly precise functional segregation and integration of distal neuronal ensembles across multiple temporal scales, can shape the brain's response to perturbations (89–93). Specifically, brain network topology can determine not only disease progression, but also adaptive or maladaptive responses to pathological perturbations. By applying fundamental principles from graph theory, it is possible to extract key functional and structural elements of the brain's connectome that may explain or predict such responses (94).
According to graph theory, a neural assembly consists of key elements, namely nodes and edges, and as such, metrics that define properties of the graph can be applied to make inferences about the neural connectome, for example, as it is revealed by electrophysiological activity (92, 94–96). Nodes incorporate neurons, neuronal populations, and macroscopic brain regions, while edges determine structural, functional, or effective connectivity and, as a result of the latter, the directionality of internodal neural communication. In this manner, structural connectivity can be indicative of morphology-activity relationships between neurons, whereas functional connectivity, which is independent of the physical proximity of the neurons, provides a temporal correlation of their activity. On the other hand, effective connectivity can determine whether there is a causal relationship between the activity of different neurons (94). Topological properties of particular interest include economical wiring, hierarchical modularity, small-world organization enabling functional segregation, integration and specialization through high network clustering and characteristic short path lengths (93, 95–100), as well as highly-connected network hubs. Connections between hubs can be describes as rich club, i.e., central core connections, if they extend over large anatomical distances and link distinct functional systems (101). As such, rich club connections mediate high-volume network traffic and integrated brain function.
Connectomics of Adaptive and Maladaptive Responses to Brain Ischemia
As a result of high interconnectivity of neural networks and circuits, the damage induced by lesions such as focal cerebral ischemia dissipates in the brain and progressively spreads to affect distal loci (81). This diaschisis phenomenon constitutes a maladaptive response and may explain the deafferentiation and aberrant synchronization observed in brain regions remote from the one directly affected by the stroke (74, 89, 92). Excitotoxicity thus plays a central part in the damage sustained to these remote nodes after a stroke. Associated maladaptive responses include anterograde and retrograde neuronal degeneration, for example, as a result of neuronal loss, excitotoxicity and impaired axonal transport, but also dedifferentiation. Apart from loss of tissue at the lesion site, neuronal degeneration involves structural deterioration over time of nodes connected to the primary lesion site, while dedifferentiation refers to the gradual loss of specialized function in the affected brain region, followed by increased, non-specialized activity in associated nodes (89, 92) (Figure 1A).
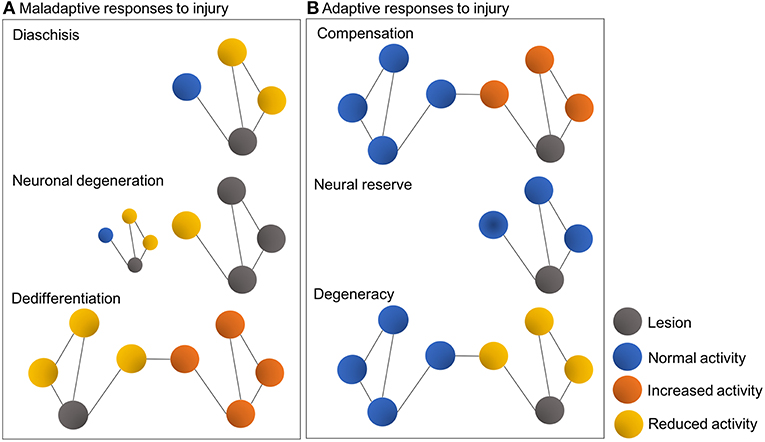
Figure 1. Schematic representations illustrating typical maladaptive (A) and adaptive responses (B) to brain injury in the context of connectomes and graph theory. Colored circles represent nodes, while lines represent interconnecting edges [Figure modified from Fornito et al. (89)].
On the other hand, adaptive network responses include lateralization, i.e., compensation for lost function by increased structure-function interactions and the engagement of intact neuronal ensembles within the affected system, or within other systems. Other adaptive responses may include neural reserve, a process by which remaining intact tissue in the immediately affected region can sustain the previous level of behavior, and also degeneracy, where a second system, without demonstrating apparent changes, can adequately support the activity of the affected one (89, 102). Thus, the effects of a perturbation critically depend on the topological centrality and degeneracy of the affected region, as pathology of central regions can exacerbate maladaptive responses, whereas degeneracy can facilitate adaptive ones (89, 95, 96). By the same token, spontaneous or induced gain of function after stroke may imply partial restoration of neural networks previously involved in impaired function or, alternatively, a compensatory or substitution mechanism, which is contingent on the recruitment of other networks (74) (Figure 1B).
However, in any perturbed system, not least, the ischemic brain, which is characterized by highly complex pathophysiological mechanisms and associated cellular and molecular cascades, such responses are neither unequivocal, nor mutually exclusive. Furthermore, as mentioned earlier, the resilience of the perturbed system and the manner in which it may reconfigure its structure-function relationships in response to the lesion largely depends on the topological substrates being affected. It follows that a neural transplant, effectively, a new topological element (likely, a node) within the local circuitry, becomes another integral component in the system's connectome. As such, the input and output functions of the transplant node are shaped by other key elements of the connectome, as for example, its interactions with other nodes, in an interdependent, reciprocal manner.
Assessing the Potential of Morphogenetically Engineered Neurons to Become Integrated Within the Connectome
What are the exact processes that determine whether and/or to what extent neurons engineered in vitro (and by the same token, in vivo), have the capacity to become part of the local connectome in a manner that may promote gain of function after stroke?
Detailed study and elucidation of relevant mechanisms in stroke patients is not feasible and is thus de facto limited to methods and analyses based on indirect and often incomplete observations or measures. Relevant research must therefore rely on robust preclinical models. Notwithstanding various excellent studies employing cell replacement strategies, coupled with cutting-edge methods for monitoring and assessment of functional integration of the engineered neurons in experimental stroke animals (16, 60), there is currently no unequivocal evidence supporting that these neurons become fully integrated into the ischemic brain. As a result, pertinent questions remain unanswered, especially with regard to the intrinsic capacity of engineered neurons to engage with local circuitry, whether we can predict their responses to evolving pathology, as well as determine whether their morphological and functional integration into the host brain will elicit adaptive or maladaptive responses and, ultimately, promote repair or inadvertently exacerbate the lesion-induced deficits. We may start addressing such questions by combining in vitro morphogenetic neuroengineering with electrophysiology studies and computational modeling.
Reductionist in vitro Paradigms That Capture Complex Network Dynamics in Healthy and Perturbed Conditions
State-of-the-art platforms for the study of in vitro neural networks include microelectrode arrays (MEAs). MEAs represent a breakthrough in in vitro electrophysiology platforms, as they enable direct observation of evolving network dynamics through extracellular recordings, as well as disease modeling and modulation of network activity by electrical, chemical, opto- or chemogenetic manipulation to selectively perturb network function or engage its plasticity (94, 103–111).
This approach largely exploits intrinsic attributes of in vitro neural networks, including emergence/morphogenesis, as a result of cell behaviors driven by local cell-cell interactions, and self-organized criticality (SoC) (112). A universal characteristic of in vitro cultured neurons, irrespective of source (i.e., rodent or human) or specific subtype, is that they self-organize into functional complex networks. This spontaneous process, which occurs in the absence of any external instructive influence (113), involves the network's gradual transition through distinct morphology-activity states underscored by increasing structural and functional complexity as a result of emerging neuronal activity, axonal elongation and pathfinding, synapse formation and pruning (113, 114). Over time, the network gains morphological and functional maturity, ultimately reaching SoC, a critical state characterized by a fine balance between excitation and inhibition (113, 115–118). Once at the SoC state, the neural network exhibits neuronal avalanches, i.e., characteristic cascades of activity defined by rich, stable spatiotemporal patterns and power-law distributions of variables (114, 119). Criticality has been closely, yet not exclusively, associated with self-organization (112, 120–124).
How does SoC displayed by in vitro neural networks relate to normal or impaired brain function? The idea that the brain operates in a near-critical state is not new (119, 125, 126), yet the presence of SoC states in the brain has, to a certain extent, been the subject of debate until relatively recently (119, 127–129). However, emerging evidence, including findings from magnetoencephalography (MEG) studies in human subjects, strongly supports the presence of SoC in the brain, not only as a mechanism underlying spatially local or internodal measures of brain activity, for example, as relevant for cortical function, but also as a global phenomenon (123, 130).
This suggests that within the brain connectome, SoC states play a putative role in information transmission and processing, including segregation and integration of function along different spatiotemporal scales (114, 123, 124, 131). As such, critical states in the brain emerge from the complex underlying morphology-activity relationships shaping the system's behavior and are stipulated to underscore adaptation processes in the context of neuroplasticity. It is currently unknown how changes in network organization over different spatiotemporal scales, for example, as a result of ischemic insult, may affect such states (114).
In vitro biological substrates that combine morphogenetically engineered neurons with MEAs can be used to obtain insights into such mechanisms. For example, neural avalanches can be observed and recorded as synchronous local field potential (LFP) spikes across multiple electrode locations. Dependent on the number of embedded recording electrodes available on the MEA, which may range from a few dozen (typically 60–120 electrodes) to several thousand, the observed LFPs may arise from neuronal clusters, effectively individual nodes within the network, but also represent single-cell activity, as in the case of very high-density (>11,000 electrodes) complementary metal-oxide semiconductor (CMOS) integrated circuit MEAs (118). This effectively means that the derived neural substrates can faithfully recapitulate fundamental aspects of neural network behavior, thus enabling the study of complex network dynamics in reductionist in vitro paradigms. Maintenance of the relevant MEA-based neural networks in optimal culture conditions enables long-term monitoring of network behavior up to several weeks or months (132) (Figure 2).
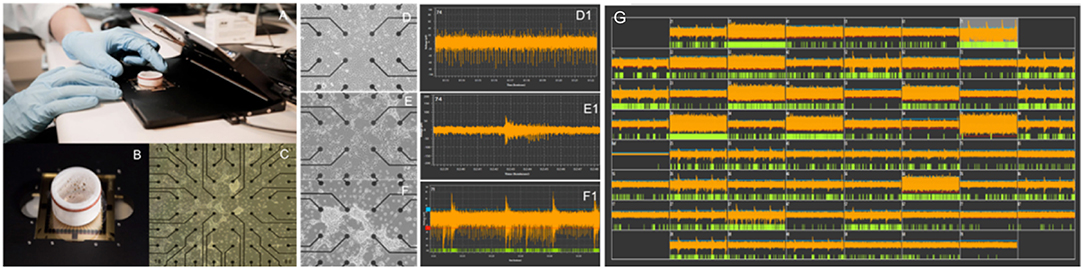
Figure 2. Evolution of spontaneous electrical activity in a morphogenetically engineered neural network on a 60-electrode MEA. (A) Standard in vitro electrophysiology platform (Multi Channel Systems, Germany). (B) Standard planar 60-electrode MEA with protective ring for long-term neuronal culture. (C) Overview image showing a neural network on a 60-electrode MEA. Neuronal clusters appear as dense white regions on the MEA. (D–F) Self-organization of engineered neural networks on an MEA over time and corresponding development of spontaneous activity shown as tonic firing (D1), unsynchronized bursts (E1), and pacemaker bursts (F1) at 20, 28, and 40 days in vitro, respectively. (G) Shows the mature neural network at >45 days in vitro.
Verification of Functionality
In light of the above, an immediate application of in vitro morphogenetically engineered neurons on MEAs can be the validation of the neurons' functionality prior to transplantation in the ischemic brain. This is a crucial but often overlooked parameter, given that fundamental intrinsic properties of engineered neurons, such as self-organization, spontaneous firing activity, or capacity to reach SoC states, can be expected to provide a gauge as to their potential to survive and integrate with the host tissue post-transplantation. As such, electrophysiological recordings from engineered neurons on MEAs is a highly complementary method to transcriptional analyses of such neurons after reprogramming and should constitute an integral part of their characterization before proceeding to in vivo studies.
Importantly, apart from verification of the inherent capacity of in vitro engineered neurons to self-organize into functional networks, reductionist in vitro paradigms such as MEA-based biological substrates also constitute a powerful tool for assessing the behavior of these networks in healthy and perturbed conditions by enabling the study of their connectomes.
Several recent studies have demonstrated that in vitro neural networks, including human engineered neuronal cultures, demonstrate physiological maturation over time suggestive of complex underlying structure-function relationships (133–138). Functional maturation can be further enhanced typically by culturing neurons on a feeder layer of astrocytes (139, 140), or by adapting the differentiation protocol to generate both neurons and astrocytes (141), thus optimizing neuronal polarization, axonal and dendritic arborization, and synapse formation in the derived networks (142–144). In this manner, in vitro neural networks recapitulate fundamental topological properties of the brain, including key features mentioned earlier, such as hierarchical modularity and small-world organization (98, 100).
Various methods for MEA data analysis can be applied to make inferences about the functional, structural or effective connectivity of the network, as well as its dynamical or critical states (94, 145). Such inferences are particularly relevant for obtaining insights as to the state of a neural network (including sub-critical and super-critical states) (113, 115, 146–148) but also for identifying evolving pathology, and predicting the manner in which the network will respond, i.e., adaptive or maladaptive.
Mimicking Stroke-Related Pathology
As mentioned earlier, one of the fundamental yet unanswered questions pertaining to the functional integration of in vitro engineered neurons in the ischemic brain post-transplantation is lack of unequivocal evidence from in vivo stroke models as to the ability of these grafts to extend axons and form efferent connections with appropriate targets. A corollary to the above is the capacity of such grafts to become incorporated in the local circuitry while pathophysiological processes of the injury and/or spontaneous plasticity of the host brain are in progress. MEA-based neural substrates provide an excellent platform for mimicking key aspects of such processes in a controlled manner.
There are currently no reported studies in the literature investigating stroke-related pathology in human engineered neural networks using MEA-based platforms. However, a number of reports have demonstrated the utility of these platforms for CNS disease modeling, often in the context of neurodegenerative disorders, epilepsy, toxicology, and drug screening, as well studies of neural network plasticity and response to selective modulation (108, 149–151).
Beyond the state-of-the-art modeling of engineered neural network responses to stroke may be achieved by the incorporation of defined topography and/or dimensionality in the MEA-based substrate. For example, a host of different biological and synthetic scaffolds can be applied for bioengineering of in vitro neural networks in three-dimensional (3D) cultures as opposed to the conventional two-dimensional (2D) ones (152–155). Such systems, which may also combine the use of a scaffolding substrates with 3D- rather than planar 2D-MEAs, can help recapitulate structure-function relationships shaped by cell morphology, cell-to-cell interactions, and axonal outgrowth in all directions within the extracellular microenvironment. In this manner, the derived in vitro connectomes can better approximate the micro-, meso-, and macroscale dynamics of the brain, thus enabling self-organized neural network behavior of increased complexity, compared to 2D systems (150, 156, 157). Importantly, 3D substrates are compatible with co-culture of neurons with other relevant cell types, including astrocytes and microglia, thus adding to the physiological relevance of the assay (142, 144, 158–162).
Hence, the 3D configuration can be used to assess emerging morphology-activity relationships in unperturbed engineered neural networks in vitro, but also to study their responses to stroke related pathology, as for example, after oxygen-glucose deprivation or induced excitotoxicity. The versatility and relevance of these platforms for in vitro connectomics of engineered neurons, can further be enhanced with the incorporation of microfluidics devices, which enable in vitro culture of modular neuronal ensembles with definable connectivity at the micro- and nanoscale level.
Custom-designed microfluid devices can be fabricated using a variety of methods including etching techniques, photo- and e-beam lithography, embossing, replica molding, and laser photoablation (163). Such systems enable the culture of in vitro neural networks by confining neuronal somata within designated compartments, i.e., nodes, and controlling afferent and efferent inter-nodal connectivity by predefined, directional axonal outgrowth through micropatterned channels only permissible to neuronal axons and neurites (164). As such, microfluidic devices can be superior to conventional culture systems as they enable spatial and temporal control of the neuronal microenvironment (163). Importantly, such microfluidic devices can be interfaced with MEAs, thus enabling longitudinal monitoring of intra- and internodal network dynamics in normal and perturbed conditions.
These integrated in vitro platforms may thus constitute a powerful tool for the investigation of the connectomics of morphogenetically engineered neural networks alone, or in co-culture with other relevant cells, including different relevant neuronal subtypes, glia, and microglia (142, 158, 165, 166). A potential application of multi-nodal microfluidic devices integrated with MEAs is to test whether compartmentalized engineered neurons can form integrated multi-nodal functional networks which demonstrate efferent, as well as afferent connectivity. Such studies can thus help address a pertinent, yet unelucidated question regarding engineered neural networks, namely, whether they can form efferent synapses. Furthermore, the topology and molecular/flow gradients of the microfluidic platform can be exploited to induce disease-related pathologies in a selective or controlled manner and monitor dynamic intra- and inter-nodal responses over time (167, 168). Added microtopography features, such as the incorporation of synaptic chambers (169), enable monitoring of axons and synapses at cross-sections between nodes, and also facilitate added manipulations, as for example axotomy (170, 171). Depending on electrode alignment in the MEA interface, such microfluidic designs can also enable monitoring of spike propagation along axons between nodes (172), as well as selective electrical stimulation.
Distinctive Advantages of in vitro Models
Clearly, advanced in vitro modeling platforms are highly versatile and can recapitulate complex morphology-activity relationships underpinning brain function (173–175). Such in vitro systems afford unique scope for mimicking stroke-related pathology and determining dynamic behavior of engineered neurons in the context of functional integration. Thus, although in vitro models are reductionist in nature, they are by no means simplistic and, compared to in vivo models, they enable specific manipulations and observations in a highly controlled manner. Especially with regard to connectomics studies, in vitro models have the distinctive advantage of allowing the study of emerging, dynamic network behavior at the micro- and mesoscale across large populations of neurons in different assemblies, including their internodal interactions (166, 176). Advanced in vitro models are thus highly complementary to in vivo ones, constituting valid alternatives and providing valuable insights that can instruct the design of in vivo stroke transplantation studies with improved predictive validity for clinical translation. As such in vitro modeling platforms are also aligned with the 3R principles (i.e., Replace, Reduce, Refine) pertaining to the use of experimental animals.
Deep Learning; Making Inferences and Predictions About Behavior
Notwithstanding the tremendous potential afforded by these advanced in vitro platforms for studying engineered neural networks in the context of stroke modeling, it must be emphasized that MEA-based electrophysiology tends to generate very high volumes of data (typically, a standard 10 min recording from a 60-electrode MEA can generate 0.5 GB of data; indicatively, the corresponding data volume increases to 1,5 GB when using a 4,096-electrode MEA). As a result, the study of connectomes, which requires extraction of functional, structural and effective connectivity and, by the same token, inference about the underlying neural network state, or prediction about its dynamic responses to stroke-related pathology, including anticipated behavior post-transplantation in vivo, cannot be achieved with standard software analysis methods. Advanced computational modeling applying deep learning principles is thus necessary.
As mentioned earlier, connectomics analyses may apply graph theory and relevant theoretical models to determine underlying structure-function relationships within a neural network and infer its critical states (94, 109, 116, 117, 172). For example, advanced computational modeling may apply principles from cellular automata (CAs) or random Boolean networks (RBNs), which examine the relationships between constituent key elements of a system based, respectively, on proximal or random connections (177). Furthermore, machine learning or deep learning approaches, such as artificial neural networks (ANNs) and artificial recurrent neural networks (RNNs), are highly relevant computational models for MEA data analysis. Such models take inspiration from biological neural networks (BNNs). Briefly, in these models the neurons, i.e., the computing elements of the system, are arranged in layers through which information flows in a feed-forward manner. Interestingly, temporal dynamics of the systems can be captured by RNNs by assigning recurrencies (cycles) within the connection topology of the neurons in the system. As a result, the system possesses a memory of previous inputs; in other words, the activation state of the network at a given time represents a function of its previous activation states. As such, an RNN can be seen as an untrained reservoir of dynamics that operates in a near-chaotic state. This enables control of the system by selecting only a single linear redout layer for training (178).
RNNs are thus well-suited to capturing dynamic network behavior of in vitro neural networks, including their critical states. These attributes of RNNs make them an excellent computational paradigm for the investigation of mechanisms by which neuronal populations, including engineered neural networks, solve various computational problems in healthy and perturbed conditions (178–182). In this way, we can start addressing fundamental questions that can help determine the functional capacity of engineered neurons. Moreover, such models can be applied to decipher as well as predict the behavior of engineered neurons in response to stroke-related pathology in vitro and also post-transplantation in vivo. Importantly, the relevant computational models can be applied to make inferences about underlying critical states, including spontaneous and induced plasticity of these networks.
Finally, closed-loop hybrid systems are highly relevant in this context as they can be used for advanced simulations of spontaneous and induced plasticity, including bi-directional learning. For example, by interfacing an engineered neural network on an MEA with a simulated agent (i.e., computer hardware) we could elicit real-time agent behavior powered by the neural network using reservoir computing (183). Furthermore, by selecting a pacemaker node within the engineered neural network as the target for electrical stimulation, we were able to alter the pacemaker activity in a manner suggestive of LTP, and at the same time demonstrate learning behavior in the simulated agent (183). Other reported studies have also demonstrated the capacity of embodied neural network cultures for goal-directed behavior and learning (184–186). Such approaches are highly promising for the future development and application of relevant experimental paradigms for in vitro and in silico assessment of the endogenous as well as induced plasticity of engineered neural network, as for example, in terms of guided closed-loop neuromodulation approaches post-transplantation.
Conclusions
In this review, we have discussed how the combination of interdisciplinary methods and theoretical principles can help develop robust pre-clinical experimental paradigms that can help validate the functional capacity of morphogenetically engineered neurons as well as provide significant insights as to their potential for promoting functional outcomes after transplantation in the stroke lesioned brain. This integration of approaches is both timely and necessary and can be expected to make significant contributions toward the safe translation of stem cell therapy in the clinic.
Author Contributions
AS and IS contributed equally to all aspects pertaining to the design and preparation of this manuscript, including idea for the review, literature search and selection, writing, editing, and finalizing the manuscript.
Conflict of Interest Statement
The authors declare that the research was conducted in the absence of any commercial or financial relationships that could be construed as a potential conflict of interest.
Acknowledgments
The authors would like to thank Ola Huse Ramstad and Vibeke Devold Valderhaug for the images in Figure 2. This work has been supported with funding from the Liaison Committee for Education, Research, and Innovation in Central Norway (Helse Midt-Norge RHF); and the Joint Research Committee between St. Olav's Hospital and the Faculty of Medicine and Health Sciences, NTNU (Felles Forskingsutvalg).
References
1. Kokaia Z, Darsalia V. Human neural stem cells for ischemic stroke treatment. Results Probl Cell Differ. (2018) 66:249–63. doi: 10.1007/978-3-319-93485-3_11
2. Bible E, Qutachi O, Chau DY, Alexander MR, Shakesheff KM, Modo M. Neo-vascularization of the stroke cavity by implantation of human neural stem cells on vegf-releasing plga microparticles. Biomaterials. (2012) 33:7435–46. doi: 10.1016/j.biomaterials.2012.06.085
3. Dunnett SB, Bjorklund A. Mechanisms and use of neural transplants for brain repair. Prog Brain Res. (2017) 230:1–51. doi: 10.1016/bs.pbr.2016.11.002
4. Kokaia Z, Lindvall O. Stem cell repair of striatal ischemia. Prog Brain Res. (2012) 201:35–53. doi: 10.1016/B978-0-444-59544-7.00003-2
5. Massensini AR, Ghuman H, Saldin LT, Medberry CJ, Keane TJ, Nicholls FJ, et al. Concentration-dependent rheological properties of ecm hydrogel for intracerebral delivery to a stroke cavity. Acta Biomater. (2015) 27:116–30. doi: 10.1016/j.actbio.2015.08.040
6. Medberry CJ, Crapo PM, Siu BF, Carruthers CA, Wolf MT, Nagarkar SP, et al. Hydrogels derived from central nervous system extracellular matrix. Biomaterials. (2013) 34:1033–40. doi: 10.1016/j.biomaterials.2012.10.062
7. Mine Y, Tatarishvili J, Oki K, Monni E, Kokaia Z, Lindvall O. Grafted human neural stem cells enhance several steps of endogenous neurogenesis and improve behavioral recovery after middle cerebral artery occlusion in rats. Neurobiol Dis. (2013) 52:191–203. doi: 10.1016/j.nbd.2012.12.006
8. Terrigno M, Busti I, Alia C, Pietrasanta M, Arisi I, D'onofrio M, et al. Neurons generated by mouse escs with hippocampal or cortical identity display distinct projection patterns when co-transplanted in the adult brain. Stem Cell Reports. (2018) 10:1016–29. doi: 10.1016/j.stemcr.2018.01.010
9. Bernstock JD, Peruzzotti-Jametti L, Ye D, Gessler FA, Maric D, Vicario N, et al. Neural stem cell transplantation in ischemic stroke: a role for preconditioning and cellular engineering. J Cereb Blood Flow Metab. (2017) 37:2314–9. doi: 10.1177/0271678X17700432
10. Boltze J, Nitzsche F, Jolkkonen J, Weise G, Posel C, Nitzsche B, et al. Concise review: increasing the validity of cerebrovascular disease models and experimental methods for translational stem cell research. Stem Cells. (2017) 35:1141–53. doi: 10.1002/stem.2595
11. Modo MM, Jolkkonen J, Zille M, Boltze J. Future of animal modeling for poststroke tissue repair. Stroke. (2018) 49:1099–106. doi: 10.1161/STROKEAHA.117.018293
12. Sandvig I, Augestad IL, Haberg AK, Sandvig A. Neuroplasticity in stroke recovery. The role of microglia in engaging and modifying synapses and networks. Eur J Neurosci. (2018) 47:1414–28. doi: 10.1111/ejn.13959
13. Bosetti F, Koenig JI, Ayata C, Back SA, Becker K, Broderick JP, et al. Translational stroke research: vision and opportunities. Stroke. (2017) 48:2632–7. doi: 10.1161/STROKEAHA.117.017112
14. Mangin G, Kubis N. Cell therapy for ischemic stroke: how to turn a promising preclinical research into a successful clinical story. Stem Cell Rev. (2018) 15:176–93. doi: 10.1007/s12015-018-9864-3
15. Savitz SI. Are stem cells the next generation of stroke therapeutics? Stroke. (2018) 49:1056–7. doi: 10.1161/STROKEAHA.118.019561
16. De La Rosa-Prieto C, Laterza C, Gonzalez-Ramos A, Wattananit S, Ge R, Lindvall O, et al. Stroke alters behavior of human skin-derived neural progenitors after transplantation adjacent to neurogenic area in rat brain. Stem Cell Res Ther. (2017) 8:59. doi: 10.1186/s13287-017-0513-6
17. Alia C, Spalletti C, Lai S, Panarese A, Lamola G, Bertolucci F, et al. Neuroplastic changes following brain ischemia and their contribution to stroke recovery: novel approaches in neurorehabilitation. Front Cell Neurosci. (2017) 11:76. doi: 10.3389/fncel.2017.00076
18. Caleo M. Rehabilitation and plasticity following stroke: insights from rodent models. Neuroscience. (2015) 311:180–94. doi: 10.1016/j.neuroscience.2015.10.029
19. Caleo M. Plasticity of transcallosal pathways after stroke and their role in recovery. J Physiol. (2018) 596:1789–90. doi: 10.1113/JP276081
20. Danilov AI, Kokaia Z, Lindvall O. Ectopic ependymal cells in striatum accompany neurogenesis in a rat model of stroke. Neuroscience. (2012) 214:159–70. doi: 10.1016/j.neuroscience.2012.03.062
21. Kazanis I, Feichtner M, Lange S, Rotheneichner P, Hainzl S, Oller M, et al. Lesion-induced accumulation of platelets promotes survival of adult neural stem / progenitor cells. Exp Neurol. (2015) 269:75–89. doi: 10.1016/j.expneurol.2015.03.018
22. Kazanis I, Gorenkova N, Zhao JW, Franklin RJ, Modo M, Ffrench-Constant C. The late response of rat subependymal zone stem and progenitor cells to stroke is restricted to directly affected areas of their niche. Exp Neurol. (2013) 248:387–97. doi: 10.1016/j.expneurol.2013.06.025
23. Kanazawa M, Ninomiya I, Hatakeyama M, Takahashi T, Shimohata T. Microglia and monocytes/macrophages polarization reveal novel therapeutic mechanism against stroke. Int J Mol Sci. (2017) 18:E2135. doi: 10.3390/ijms18102135
24. Lambertsen KL, Finsen B, Clausen BH. Post-stroke inflammation-target or tool for therapy? Acta Neuropathol. (2018) 137:693–714. doi: 10.1007/s00401-018-1930-z
25. Greig LC, Woodworth MB, Galazo MJ, Padmanabhan H, Macklis JD. Molecular logic of neocortical projection neuron specification, development and diversity. Nat Rev Neurosci. (2013) 14:755–69. doi: 10.1038/nrn3586
26. Gates MA, Tai CC, Macklis JD. Neocortical neurons lacking the protein-tyrosine kinase B receptor display abnormal differentiation and process elongation in vitro and in vivo. Neuroscience. (2000) 98:437–47. doi: 10.1016/S0306-4522(00)00106-8
27. Klein R. Role of neurotrophins in mouse neuronal development. Faseb J. (1994) 8:738–44. doi: 10.1096/fasebj.8.10.8050673
28. Heins N, Malatesta P, Cecconi F, Nakafuku M, Tucker KL, Hack MA, et al. Glial cells generate neurons: the role of the transcription factor Pax6. Nat Neurosci. (2002) 5:308–15. doi: 10.1038/nn828
29. Buffo A, Vosko MR, Erturk D, Hamann GF, Jucker M, Rowitch D, et al. Expression pattern of the transcription factor Olig2 in response to brain injuries: implications for neuronal repair. Proc Natl Acad Sci USA. (2005) 102:18183–8. doi: 10.1073/pnas.0506535102
30. Yamanaka S, Takahashi K. Induction of pluripotent stem cells from mouse fibroblast cultures. Tanpakushitsu Kakusan Koso. (2006) 51:2346–51. doi: 10.1111/j.1365-2184.2008.00493.x
31. Abernathy DG, Kim WK, Mccoy MJ, Lake AM, Ouwenga R, Lee SW, et al. Micrornas induce a permissive chromatin environment that enables neuronal subtype-specific reprogramming of adult human fibroblasts. Cell Stem Cell. (2017) 21:332–348 E9. doi: 10.1016/j.stem.2017.08.002
32. Abernathy DG, Yoo AS. Microrna-dependent genetic networks during neural development. Cell Tissue Res. (2015) 359:179–85. doi: 10.1007/s00441-014-1899-4
33. Miskinyte G, Gronning Hansen M, Monni E, Lam M, Bengzon J, Lindvall O, et al. Transcription factor programming of human es cells generates functional neurons expressing both upper and deep layer cortical markers. PLoS ONE. (2018) 13:E0204688. doi: 10.1371/journal.pone.0204688
34. Pfisterer U, Wood J, Nihlberg K, Hallgren O, Bjermer L, Westergren-Thorsson G, et al. Efficient induction of functional neurons from adult human fibroblasts. Cell Cycle. (2011) 10:3311–6. doi: 10.4161/cc.10.19.17584
35. Torper O, Pfisterer U, Wolf DA, Pereira M, Lau S, Jakobsson J, et al. Generation of induced neurons via direct conversion in vivo. Proc Natl Acad Sci USA. (2013) 110:7038–43. doi: 10.1073/pnas.1303829110
36. Drouin-Ouellet J, Pircs K, Barker RA, Jakobsson J, Parmar M. Direct neuronal reprogramming for disease modeling studies using patient-derived neurons: what have we learned? Front Neurosci. (2017) 11:530. doi: 10.3389/fnins.2017.00530
37. Vierbuchen T, Ostermeier A, Pang ZP, Kokubu Y, Sudhof TC, Wernig M. Direct conversion of fibroblasts to functional neurons by defined factors. Nature. (2010) 463:1035–41. doi: 10.1038/nature08797
38. Gascon S, Masserdotti G, Russo GL, Gotz M. Direct neuronal reprogramming: achievements, hurdles, and new roads to success. Cell Stem Cell. (2017) 21:18–34. doi: 10.1016/j.stem.2017.06.011
39. Lu YL, Yoo AS. Mechanistic insights into microrna-induced neuronal reprogramming of human adult fibroblasts. Front Neurosci. (2018) 12:522. doi: 10.3389/fnins.2018.00522
40. Karow M, Sanchez R, Schichor C, Masserdotti G, Ortega F, Heinrich C, et al. Reprogramming of pericyte-derived cells of the adult human brain into induced neuronal cells. Cell Stem Cell. (2012) 11:471–6. doi: 10.1016/j.stem.2012.07.007
41. Magnusson JP, Goritz C, Tatarishvili J, Dias DO, Smith EM, Lindvall O, et al. A latent neurogenic program in astrocytes regulated by notch signaling in the mouse. Science. (2014) 346:237–41. doi: 10.1126/science.346.6206.237
42. Pereira M, Birtele M, Shrigley S, Benitez JA, Hedlund E, Parmar M, et al. Direct reprogramming of resident ng2 glia into neurons with properties of fast-spiking parvalbumin-containing interneurons. Stem Cell Reports. (2017) 9:742–51. doi: 10.1016/j.stemcr.2017.07.023
43. Torper O, Gotz M. Brain repair from intrinsic cell sources: turning reactive glia into neurons. Prog Brain Res. (2017) 230:69–97. doi: 10.1016/bs.pbr.2016.12.010
44. Trudler D, Lipton SA. Novel direct conversion of microglia to neurons. Trends Mol Med. (2019) 25: 72–4. doi: 10.1016/j.molmed.2018.12.005
45. Hanisch UK, Kettenmann H. Microglia: active sensor and versatile effector cells in the normal and pathologic brain. Nat Neurosci. (2007) 10:1387–94. doi: 10.1038/nn1997
46. Niu W, Zang T, Zou Y, Fang S, Smith DK, Bachoo R, et al. In vivo reprogramming of astrocytes to neuroblasts in the adult brain. Nat Cell Biol. (2013) 15:1164–75. doi: 10.1038/ncb2843
47. Su Z, Niu W, Liu ML, Zou Y, Zhang CL. In vivo conversion of astrocytes to neurons in the injured adult spinal cord. Nat Commun. (2014) 5:3338. doi: 10.1038/ncomms4338
48. Torper O, Ottosson DR, Pereira M, Lau S, Cardoso T, Grealish S, et al. In vivo reprogramming of striatal Ng2 glia into functional neurons that integrate into local host circuitry. Cell Rep. (2015) 12:474–81. doi: 10.1016/j.celrep.2015.06.040
49. Kronenberg G, Gertz K, Cheung G, Buffo A, Kettenmann H, Gotz M, et al. Modulation of fate determinants olig2 and pax6 in resident glia evokes spiking neuroblasts in a model of mild brain ischemia. Stroke. (2010) 41:2944–9. doi: 10.1161/STROKEAHA.110.583039
50. Wang LL, Zhang CL. Engineering new neurons: in vivo reprogramming in mammalian brain and spinal cord. Cell Tissue Res. (2018) 371:201–12. doi: 10.1007/s00441-017-2729-2
51. Barker RA, Gotz M, Parmar M. New approaches for brain repair-from rescue to reprogramming. Nature. (2018) 557:329–34. doi: 10.1038/s41586-018-0087-1
52. Delavaran H, Sjunnesson H, Arvidsson A, Lindvall O, Norrving B, Van Westen D, et al. Proximity of brain infarcts to regions of endogenous neurogenesis and involvement of striatum in ischaemic stroke. Eur J Neurol. (2013) 20:473–9. doi: 10.1111/j.1468-1331.2012.03877.x
53. Avaliani N, Sorensen AT, Ledri M, Bengzon J, Koch P, Brustle O, et al. Optogenetics reveal delayed afferent synaptogenesis on grafted human-induced pluripotent stem cell-derived neural progenitors. Stem Cells. (2014) 32:3088–98. doi: 10.1002/stem.1823
54. Bjorklund A, Stenevi U. Reconstruction of the nigrostriatal dopamine pathway by intracerebral nigral transplants. Brain Res. (1979) 177:555–60. doi: 10.1016/0006-8993(79)90472-4
55. Falkner S, Grade S, Dimou L, Conzelmann KK, Bonhoeffer T, Gotz M, et al. Transplanted embryonic neurons integrate into adult neocortical circuits. Nature. (2016) 539:248–53. doi: 10.1038/nature20113
56. Grealish S, Parmar M. Plug and play brain: understanding integration of transplanted neurons for brain repair. Cell Stem Cell. (2016) 19:679–80. doi: 10.1016/j.stem.2016.11.008
57. Thompson LH, Bjorklund A. Reconstruction of brain circuitry by neural transplants generated from pluripotent stem cells. Neurobiol Dis. (2015) 79:28–40. doi: 10.1016/j.nbd.2015.04.003
58. Wuttke TV, Markopoulos F, Padmanabhan H, Wheeler AP, Murthy VN, Macklis JD. Developmentally primed cortical neurons maintain fidelity of differentiation and establish appropriate functional connectivity after transplantation. Nat Neurosci. (2018) 21:517–29. doi: 10.1038/s41593-018-0098-0
59. Tornero D, Wattananit S, Gronning Madsen M, Koch P, Wood J, Tatarishvili J, et al. Human Induced pluripotent stem cell-derived cortical neurons integrate in stroke-injured cortex and improve functional recovery. Brain. (2013) 136:3561–77. doi: 10.1093/brain/awt278
60. Tornero D, Tsupykov O, Granmo M, Rodriguez C, Gronning-Hansen M, Thelin J, et al. Synaptic inputs from stroke-injured brain to grafted human stem cell-derived neurons activated by sensory Stimuli. Brain. (2017) 140:692–706. doi: 10.1093/brain/aww347
61. Bliss TV, Lomo T. Long-lasting potentiation of synaptic transmission in the dentate area of the anaesthetized rabbit following stimulation of the perforant path. J Physiol. (1973) 232:331–56. doi: 10.1113/jphysiol.1973.sp010273
62. Ito M, Kano M. Long-lasting depression of parallel fiber-purkinje cell transmission induced by conjunctive stimulation of parallel fibers and climbing fibers in the cerebellar cortex. Neurosci Lett. (1982) 33:253–8. doi: 10.1016/0304-3940(82)90380-9
63. Yee AX, Hsu YT, Chen L. A metaplasticity view of the interaction between homeostatic and hebbian plasticity. Philos Trans R Soc Lond B Biol Sci. (2017) 372:20160155. doi: 10.1098/rstb.2016.0155
64. Turrigiano GG. The self-tuning neuron: synaptic scaling of excitatory synapses. Cell. (2008) 135:422–35. doi: 10.1016/j.cell.2008.10.008
65. Turrigiano GG. The dialectic of hebb and homeostasis. Philos Trans R Soc Lond B Biol Sci. (2017) 372:20160258. doi: 10.1098/rstb.2016.0258
66. Costa RP, Mizusaki BE, Sjostrom PJ, Van Rossum MC. Functional consequences of pre- and postsynaptic expression of synaptic plasticity. Philos Trans R Soc Lond B Biol Sci. (2017) 372:20160153. doi: 10.1098/rstb.2016.0153
67. Keck T, Toyoizumi T, Chen L, Doiron B, Feldman DE, Fox K, et al. Integrating hebbian and homeostatic plasticity: the current state of the field and future research directions. Philos Trans R Soc Lond B Biol Sci. (2017) 372:20160158. doi: 10.1098/rstb.2016.0158
68. Turrigiano G. Homeostatic synaptic plasticity: local and global mechanisms for stabilizing neuronal function. Cold Spring Harb Perspect Biol. (2012) 4:A005736. doi: 10.1101/cshperspect.a005736
69. Zenke F, Gerstner W, Ganguli S. The temporal paradox of hebbian learning and homeostatic plasticity. Curr Opin Neurobiol. (2017) 43:166–76. doi: 10.1016/j.conb.2017.03.015
70. Turrigiano GG, Leslie KR, Desai NS, Rutherford LC, Nelson SB. Activity-dependent scaling of quantal amplitude in neocortical neurons. Nature. (1998) 391:892–6. doi: 10.1038/36103
71. Dalise S, Ambrosio F, Modo M. Brain plasticity and recovery in preclinical models of stroke. Arch Ital Biol. (2014) 152:190–215. doi: 10.12871/00039829201442
72. Takeuchi N, Izumi SI. Rehabilitation using repetitive transcranial magnetic stimulation. Brain Nerve. (2017) 69:227–38. doi: 10.11477/mf.1416200732
73. Gennaro M, Mattiello A, Mazziotti R, Antonelli C, Gherardini L, Guzzetta A, et al. Focal stroke in the developing rat motor cortex induces age- and experience-dependent maladaptive plasticity of corticospinal system. Front Neural Circ. (2017) 11:47. doi: 10.3389/fncir.2017.00047
74. Xerri C, Zennou-Azogui Y, Sadlaoud K, Sauvajon D. Interplay between intra- and interhemispheric remodeling of neural networks as a substrate of functional recovery after stroke: adaptive versus maladaptive reorganization. Neuroscience. (2014) 283:178–201. doi: 10.1016/j.neuroscience.2014.06.066
75. Hayashi Y, Okamoto K, Bosch M, Futai K. Roles of neuronal activity-induced gene products in hebbian and homeostatic synaptic plasticity, tagging, and capture. Adv Exp Med Biol. (2012) 970:335–54. doi: 10.1007/978-3-7091-0932-8_15
76. Vitureira N, Goda Y. Cell biology in neuroscience: the interplay between hebbian and homeostatic synaptic plasticity. J Cell Biol. (2013) 203:175–86. doi: 10.1083/jcb.201306030
77. Hokstad A, Indredavik B, Bernhardt J, Langhammer B, Gunnes M, Lundemo C, et al. Upright activity within the first week after stroke is associated with better functional outcome and health-related quality of life: a norwegian multi-site study. J Rehabil Med. (2016) 48:280–6. doi: 10.2340/16501977-2051
78. Cossetti C, Alfaro-Cervello C, Donega M, Tyzack G, Pluchino S. New perspectives of tissue remodelling with neural stem and progenitor cell-based therapies. Cell Tissue Res. (2012) 349:321–9. doi: 10.1007/s00441-012-1341-8
79. Murphy TH, Corbett D. Plasticity during stroke recovery: from synapse to behaviour. Nat Rev Neurosci. (2009) 10:861–72. doi: 10.1038/nrn2735
80. Askim T, Bernhardt J, Salvesen O, Indredavik B. Physical activity early after stroke and its association to functional outcome 3 months later. J Stroke Cerebrovasc Dis. (2014) 23:E305–E312. doi: 10.1016/j.jstrokecerebrovasdis.2013.12.011
81. Pizzorusso T, Medini P, Landi S, Baldini S, Berardi N, Maffei L. Structural and functional recovery from early monocular deprivation in adult rats. Proc Natl Acad Sci USA. (2006) 103:8517–22. doi: 10.1073/pnas.0602657103
82. Teasell RW, Foley NC, Salter KL, Jutai JW. A blueprint for transforming stroke rehabilitation care in canada: the case for change. Arch Phys Med Rehabil. (2008) 89:575–8. doi: 10.1016/j.apmr.2007.08.164
83. Bernhardt J, Churilov L, Ellery F, Collier J, Chamberlain J, Langhorne P, et al. Prespecified dose-response analysis for a very early rehabilitation trial (Avert). Neurology. (2016) 86:2138–45. doi: 10.1212/WNL.0000000000002459
84. Saso A, Moe-Nilssen R, Gunnes M, Askim T. Responsiveness of the berg balance scale in patients early after stroke. Physiother Theory Pract. (2016) 32:251–61. doi: 10.3109/09593985.2016.1138347
85. Lo EH. A new penumbra: transitioning from injury into repair after stroke. Nat Med. (2008) 14:497–500. doi: 10.1038/nm1735
86. Ward NS, Brown MM, Thompson AJ, Frackowiak RS. Neural correlates of motor recovery after stroke: a longitudinal fmri study. Brain. (2003) 126:2476–96. doi: 10.1093/brain/awg245
87. Sporns O, Chialvo DR, Kaiser M, Hilgetag CC. Organization, development and function of complex brain networks. Trends Cogn Sci. (2004) 8:418–25. doi: 10.1016/j.tics.2004.07.008
88. Sporns O, Tononi G, Kotter R. The human connectome: a structural description of the human brain. Plos Comput Biol. (2005) 1:E42. doi: 10.1371/journal.pcbi.0010042
89. Fornito A, Zalesky A, Breakspear M. The connectomics of brain disorders. Nat Rev Neurosci. (2015) 16:159–72. doi: 10.1038/nrn3901
90. Breakspear M, Stam CJ. Dynamics of a neural system with a multiscale architecture. Philos Trans R Soc Lond B Biol Sci. (2005) 360:1051–74. doi: 10.1098/rstb.2005.1643
91. Bullmore E, Sporns O. Complex brain networks: graph theoretical analysis of structural and functional systems. Nat Rev Neurosci. (2009) 10:186–98. doi: 10.1038/nrn2575
92. Fornito A, Bullmore ET. Connectomics: a new paradigm for understanding brain disease. Eur Neuropsychopharmacol. (2015) 25:733–48. doi: 10.1016/j.euroneuro.2014.02.011
93. Tononi G, Sporns O, Edelman GM. A measure for brain complexity: relating functional segregation and integration in the nervous system. Proc Natl Acad Sci USA. (1994) 91:5033–7. doi: 10.1073/pnas.91.11.5033
94. Poli D, Pastore VP, Massobrio P. Functional connectivity in in vitro neuronal assemblies. Front Neural Circ. (2015) 9:57. doi: 10.3389/fncir.2015.00057
95. Avena-Koenigsberger A, Misic B, Sporns O. Communication dynamics in complex brain networks. Nat Rev Neurosci. (2017) 19:17–33. doi: 10.1038/nrn.2017.149
96. Bassett DS, Zurn P, Gold JI. On The nature and use of models in network neuroscience. Nat Rev Neurosci. (2018) 19:566–78. doi: 10.1038/s41583-018-0038-8
97. Humphries MD, Gurney K. Network 'small-world-ness': a quantitative method for determining canonical network equivalence. PLoS ONE. (2008) 3:E0002051. doi: 10.1371/journal.pone.0002051
98. Sporns O, Kotter R. Motifs in brain networks. Plos Biol. (2004) 2:E369. doi: 10.1371/journal.pbio.0020369
99. Sporns O, Zwi JD. The small world of the cerebral cortex. Neuroinformatics. (2004) 2:145–62. doi: 10.1385/NI:2:2:145
100. Tognoli E, Kelso JA. Enlarging the scope: grasping brain complexity. Front Syst Neurosci. (2014) 8:122. doi: 10.3389/fnsys.2014.00122
101. Van Den Heuvel MP, Sporns O. Rich-club organization of the human connectome. J Neurosci. (2011) 31:15775–86. doi: 10.1523/JNEUROSCI.3539-11.2011
102. Noppeney U, Friston KJ, Price CJ. Degenerate neuronal systems sustaining cognitive functions. J Anat. (2004) 205:433–42. doi: 10.1111/j.0021-8782.2004.00343.x
103. Berdondini L, Massobrio P, Chiappalone M, Tedesco M, Imfeld K, Maccione A, et al. Extracellular recordings from locally dense microelectrode arrays coupled to dissociated cortical cultures. J Neurosci Methods. (2009) 177:386–96. doi: 10.1016/j.jneumeth.2008.10.032
104. Bonifazi P, Difato F, Massobrio P, Breschi GL, Pasquale V, Levi T, et al. In vitro large-scale experimental and theoretical studies for the realization of bi-directional brain-prostheses. Front Neural Circuits. (2013) 7:40. doi: 10.3389/fncir.2013.00040
105. Kanagasabapathi TT, Massobrio P, Tedesco M, Martinoia S, Wadman WJ, Decre MM. An experimental approach towards the development of an in vitro cortical-thalamic co-culture model. Conf Proc Ieee Eng Med Biol Soc. (2011) 2011:648–51. doi: 10.1109/IEMBS.2011.6090144
106. Letourneau PC. Cell-to-substratum adhesion and guidance of axonal elongation. Dev Biol. (1975) 44:92–101. doi: 10.1016/0012-1606(75)90379-6
107. Lu C, Chen Q, Zhou T, Bozic D, Fu Z, Pan JQ, et al. Micro-electrode array recordings reveal reductions in both excitation and inhibition in cultured cortical neuron networks lacking Shank3. Mol Psychiatry. (2016) 21:159–68. doi: 10.1038/mp.2015.173
108. Massobrio P, Giachello CN, Ghirardi M, Martinoia S. Selective modulation of chemical and electrical synapses of helix neuronal networks during in vitro development. BMC Neurosci. (2013) 14:22. doi: 10.1186/1471-2202-14-22
109. Massobrio P, Massobrio G, Martinoia S. Interfacing cultured neurons to microtransducers arrays: a review of the neuro-electronic junction models. Front Neurosci. (2016) 10:282. doi: 10.3389/fnins.2016.00282
110. Massobrio P, Tessadori J, Chiappalone M, Ghirardi M. In vitro studies of neuronal networks and synaptic plasticity in invertebrates and in mammals using multielectrode arrays. Neural Plast. (2015) 2015:196195. doi: 10.1155/2015/196195
111. Muthmann JO, Amin H, Sernagor E, Maccione A, Panas D, Berdondini L, et al. Spike detection for large neural populations using high density multielectrode arrays. Front Neuroinform. (2015) 9:28. doi: 10.3389/fninf.2015.00028
112. Bak P, Tang C, Wiesenfeld K. Self-organized criticality. Phys Rev A Gen Phys. (1988) 38:364–74. doi: 10.1103/PhysRevA.38.364
113. Tetzlaff C, Okujeni S, Egert U, Worgotter F, Butz M. Self-organized criticality in developing neuronal networks. Plos Comput Biol. (2010) 6:E1001013. doi: 10.1371/journal.pcbi.1001013
114. Yada Y, Mita T, Sanada A, Yano R, Kanzaki R, Bakkum DJ, et al. development of neural population activity toward self-organized criticality. Neuroscience. (2017) 343:55–65. doi: 10.1016/j.neuroscience.2016.11.031
115. Pasquale V, Massobrio P, Bologna LL, Chiappalone M, Martinoia S. Self-organization and neuronal avalanches in networks of dissociated cortical neurons. Neuroscience. (2008) 153:1354–69. doi: 10.1016/j.neuroscience.2008.03.050
116. Massobrio P, Pasquale V, Martinoia S. Emergence of critical dynamics in large-scale in vitro cortical networks. Conf Proc Ieee Eng Med Biol Soc. (2015) 2015:4737–40. doi: 10.1109/EMBC.2015.7319452
117. Massobrio P, Pasquale V, Martinoia S. Self-organized criticality in cortical assemblies occurs in concurrent scale-free and small-world networks. Sci Rep. (2015) 5:10578. doi: 10.1038/srep10578
118. Hoffmann H, Payton DW. Optimization by self-organized criticality. Sci Rep. (2018) 8:2358. doi: 10.1038/s41598-018-20275-7
119. Beggs JM, Plenz D. Neuronal avalanches in neocortical circuits. J Neurosci. (2003) 23:11167–77. doi: 10.1523/JNEUROSCI.23-35-11167.2003
120. Bornholdt S, Rohl T. Self-organized critical neural networks. Phys Rev E Stat Nonlin Soft Matter Phys. (2003) 67:066118. doi: 10.1103/PhysRevE.67.066118
121. Rubinov M, Sporns O, Thivierge JP, Breakspear M. Neurobiologically realistic determinants of self-organized criticality in networks of spiking neurons. Plos Comput Biol. (2011) 7:E1002038. doi: 10.1371/journal.pcbi.1002038
122. Droste F, Do AL, Gross T. Analytical investigation of self-organized criticality in neural networks. J R Soc Interface. (2013) 10:20120558. doi: 10.1098/rsif.2012.0558
123. Daffertshofer A, Ton R, Kringelbach ML, Woolrich M, Deco G. Distinct criticality of phase and amplitude dynamics in the resting brain. Neuroimage. (2018) 180:442–7. doi: 10.1016/j.neuroimage.2018.03.002
124. Shew WL, Plenz D. The functional benefits of criticality in the cortex. Neuroscientist. (2013) 19:88–100. doi: 10.1177/1073858412445487
125. Linkenkaer-Hansen K, Smit DJ, Barkil A, Van Beijsterveldt TE, Brussaard AB, Boomsma DI, et al. Genetic contributions to long-range temporal correlations in ongoing oscillations. J Neurosci. (2007) 27:13882–9. doi: 10.1523/JNEUROSCI.3083-07.2007
126. Beggs JM, Timme N. Being critical of criticality in the brain. Front Physiol. (2012) 3:163. doi: 10.3389/fphys.2012.00163
127. He BJ, Zempel JM, Snyder AZ, Raichle ME. The temporal structures and functional significance of scale-free brain activity. Neuron. (2010) 66:353–69. doi: 10.1016/j.neuron.2010.04.020
128. Beggs JM, Plenz D. Neuronal avalanches are diverse and precise activity patterns that are stable for many hours in cortical slice cultures. J Neurosci. (2004) 24:5216–29. doi: 10.1523/JNEUROSCI.0540-04.2004
129. He BJ. Scale-free brain activity: past, present, and future. Trends Cogn Sci. (2014) 18:480–7. doi: 10.1016/j.tics.2014.04.003
130. Sethna JP, Dahmen KA, Myers CR. Crackling noise. Nature. (2001) 410:242–50. doi: 10.1038/35065675
131. Millman D, Mihalas S, Kirkwood A, Niebur E. Self-organized criticality occurs in non-conservative neuronal networks during up states. Nat Phys. (2010) 6:801–5. doi: 10.1038/nphys1757
132. Potter SM, Demarse TB. A new approach to neural cell culture for long-term studies. J Neurosci Methods. (2001) 110:17–24. doi: 10.1016/S0165-0270(01)00412-5
133. Chen X, Dzakpasu R. Observed network dynamics from altering the balance between excitatory and inhibitory neurons in cultured networks. Phys Rev E Stat Nonlin Soft Matter Phys. (2010) 82:031907. doi: 10.1103/PhysRevE.82.031907
134. Chiappalone M, Bove M, Vato A, Tedesco M, Martinoia S. Dissociated cortical networks show spontaneously correlated activity patterns during in vitro development. Brain Res. (2006) 1093:41–53. doi: 10.1016/j.brainres.2006.03.049
135. Chiappalone M, Vato A, Berdondini L, Koudelka-Hep M, Martinoia S. Network dynamics and synchronous activity in cultured cortical neurons. Int J Neural Syst. (2007) 17:87–103. doi: 10.1142/S0129065707000968
136. Edwards D, Sommerhage F, Berry B, Nummer H, Raquet M, Clymer B, et al. Comparison of nmda and ampa channel expression and function between embryonic and adult neurons utilizing microelectrode array systems. Acs Biomater Sci Eng. (2017) 3:3525–33. doi: 10.1021/acsbiomaterials.7b00596
137. Odawara A, Katoh H, Matsuda N, Suzuki I. Physiological maturation and drug responses of human induced pluripotent stem cell-derived cortical neuronal networks in long-term culture. Sci Rep. (2016) 6:26181. doi: 10.1038/srep26181
138. Kayama T, Suzuki I, Odawara A, Sasaki T, Ikegaya Y. Temporally coordinated spiking activity of human induced pluripotent stem cell-derived neurons co-cultured with astrocytes. Biochem Biophys Res Commun. (2018) 495:1028–33. doi: 10.1016/j.bbrc.2017.11.115
139. Kaech S, Banker G. Culturing hippocampal neurons. Nat Protoc. (2006) 1:2406–15. doi: 10.1038/nprot.2006.356
140. Lischka FW, Efthymiou A, Zhou Q, Nieves MD, Mccormack NM, Wilkerson MD, et al. Neonatal mouse cortical but not isogenic human astrocyte feeder layers enhance the functional maturation of induced pluripotent stem cell-derived neurons in culture. Glia. (2018) 66:725–48. doi: 10.1002/glia.23278
141. Gunhanlar N, Shpak G, Van Der Kroeg M, Gouty-Colomer LA, Munshi ST, Lendemeijer B, et al. A simplified protocol for differentiation of electrophysiologically mature neuronal networks from human induced pluripotent stem cells. Mol Psychiatry. (2018) 23:1336–44. doi: 10.1038/mp.2017.56
142. Aebersold MJ, Thompson-Steckel G, Joutang A, Schneider M, Burchert C, Forro C, et al. Simple and inexpensive paper-based astrocyte co-culture to improve survival of low-density neuronal networks. Front Neurosci. (2018) 12:94. doi: 10.3389/fnins.2018.00094
143. Allen NJ, Eroglu C. Cell biology of astrocyte-synapse interactions. Neuron. (2017) 96:697–708. doi: 10.1016/j.neuron.2017.09.056
144. Terrasso AP, Silva AC, Filipe A, Pedroso P, Ferreira AL, Alves PM, et al. Human neuron-astrocyte 3d co-culture-based assay for evaluation of neuroprotective compounds. J Pharmacol Toxicol Methods. (2017) 83:72–9. doi: 10.1016/j.vascn.2016.10.001
145. Hesse J, Gross T. Self-organized criticality as a fundamental property of neural systems. Front Syst Neurosci. (2014) 8:166. doi: 10.3389/fnsys.2014.00166
146. Pajevic S, Plenz D. Efficient network reconstruction from dynamical cascades identifies small-world topology of neuronal avalanches. Plos Comput Biol. (2009) 5:E1000271. doi: 10.1371/journal.pcbi.1000271
147. Pajevic S, Plenz D. The organization of strong links in complex networks. Nat Phys. (2012) 8:429–36. doi: 10.1038/nphys2257
148. Massobrio P, De Arcangelis L, Pasquale V, Jensen HJ, Plenz D. Criticality as a signature of healthy neural systems. Front Syst Neurosci. (2015) 9:22. doi: 10.3389/fnsys.2015.00022
149. Colombi I, Mahajani S, Frega M, Gasparini L, Chiappalone M. Effects of antiepileptic drugs on hippocampal neurons coupled to micro-electrode arrays. Front Neuroeng. (2013) 6:10. doi: 10.3389/fneng.2013.00010
150. Frega M, Pasquale V, Tedesco M, Marcoli M, Contestabile A, Nanni M, et al. Cortical cultures coupled to micro-electrode arrays: a novel approach to perform in vitro excitotoxicity testing. Neurotoxicol Teratol. (2012) 34:116–27. doi: 10.1016/j.ntt.2011.08.001
151. Vassallo A, Chiappalone M, De Camargos Lopes R, Scelfo B, Novellino A, Defranchi E, et al. A multi-laboratory evaluation of microelectrode array-based measurements of neural network activity for acute neurotoxicity testing. Neurotoxicology. (2017) 60:280–92. doi: 10.1016/j.neuro.2016.03.019
152. Aregueta-Robles UA, Martens PJ, Poole-Warren LA, Green RA. Tissue engineered hydrogels supporting 3d neural networks. Acta Biomater. (2018) e58034. doi: 10.1016/j.actbio.2018.11.044
153. Cvetkovic C, Basu N, Krencik R. Synaptic microcircuit modeling with 3d cocultures of astrocytes and neurons from human pluripotent stem cells. J Vis Exp. (2018). doi: 10.3791/58034
154. Bento AR, Quelhas P, Oliveira MJ, Pego AP, Amaral IF. Three-dimensional culture of single embryonic stem-derived neural/stem progenitor cells in fibrin hydrogels: neuronal network formation and matrix remodelling. J Tissue Eng Regen Med. (2017) 11:3494–507. doi: 10.1002/term.2262
155. Chwalek K, Tang-Schomer MD, Omenetto FG, Kaplan DL. In vitro bioengineered model of cortical brain tissue. Nat Protoc. (2015) 10:1362–73. doi: 10.1038/nprot.2015.091
156. Tedesco M, Frega M, Martinoia S, Pesce M, Massobrio P. Interfacing 3d engineered neuronal cultures to micro-electrode arrays: an innovative in vitro experimental model. J Vis Exp. (2015) 105:E53080. doi: 10.3791/53080
157. Zuo L, Yu S, Briggs CA, Kantor S, Pan JY. design and fabrication of a three-dimensional multi-electrode array for neuron electrophysiology. J Biomech Eng. (2017) 139. doi: 10.1115/1.4037948
158. Celikkaya H, Cosacak MI, Papadimitriou C, Popova S, Bhattarai P, Biswas SN, et al. Gata3 promotes the neural progenitor state but not neurogenesis in 3d traumatic injury model of primary human cortical astrocytes. Front Cell Neurosci. (2019) 13:23. doi: 10.3389/fncel.2019.00023
159. East E, Golding JP, Phillips JB. A versatile 3d culture model facilitates monitoring of astrocytes undergoing reactive gliosis. J Tissue Eng Regen Med. (2009) 3:634–46. doi: 10.1002/term.209
160. Freudenberg U, Hermann A, Welzel PB, Stirl K, Schwarz SC, Grimmer M, et al. A star-peg-heparin hydrogel platform to aid cell replacement therapies for neurodegenerative diseases. Biomaterials. (2009) 30:5049–60. doi: 10.1016/j.biomaterials.2009.06.002
161. Phillips JB. Monitoring neuron and astrocyte interactions with a 3d cell culture system. Methods Mol Biol. (2014) 1162:113–24. doi: 10.1007/978-1-4939-0777-9_9
162. Watson PMD, Kavanagh E, Allenby G, Vassey M. Bioengineered 3d glial cell culture systems and applications for neurodegeneration and neuroinflammation. Slas Discov. (2017) 22:583–601. doi: 10.1177/2472555217691450
163. Rothbauer M, Zirath H, Ertl P. Recent advances in microfluidic technologies for cell-to-cell interaction studies. Lab Chip. (2018) 18:249–70. doi: 10.1039/C7LC00815E
164. Van De Wijdeven R, Ramstad OH, Bauer US, Halaas O, Sandvig A, Sandvig I. Structuring a multi-nodal neural network in vitro within a novel design microfluidic chip. Biomed Microdevices. (2018) 20:9. doi: 10.1007/s10544-017-0254-4
165. Lam D, Enright HA, Cadena J, Peters SKG, Sales AP, Osburn JJ, et al. Tissue-specific extracellular matrix accelerates the formation of neural networks and communities in a neuron-glia co-culture on a multi-electrode array. Sci Rep. (2019) 9:4159. doi: 10.1038/s41598-019-40128-1
166. Lee SR, Hyung S, Bang S, Lee Y, Ko J, Lee S, et al. Modeling neural circuit, blood-brain barrier, and myelination on a microfluidic 96 well plate. Biofabrication. (2019) 11:035013. doi: 10.1088/1758-5090/ab1402
167. Fernandes JT, Chutna O, Chu V, Conde JP, Outeiro TF. A novel microfluidic cell co-culture platform for the study of the molecular mechanisms of Parkinson's disease and other synucleinopathies. Front Neurosci. (2016) 10:511. doi: 10.3389/fnins.2016.00511
168. Vu TQ, De Castro RM, Qin L. bridging the gap: microfluidic devices for short and long distance cell-cell communication. Lab Chip. (2017) 17:1009–23. doi: 10.1039/C6LC01367H
169. Kilinc D, Peyrin JM, Soubeyre V, Magnifico S, Saias L, Viovy JL, et al. Wallerian-like degeneration of central neurons after synchronized and geometrically registered mass axotomy in a three-compartmental microfluidic chip. Neurotox Res. (2011) 19:149–61. doi: 10.1007/s12640-010-9152-8
170. Taylor AM, Dieterich DC, Ito HT, Kim SA, Schuman EM. Microfluidic local perfusion chambers for the visualization and manipulation of synapses. Neuron. (2010) 66:57–68. doi: 10.1016/j.neuron.2010.03.022
171. Virlogeux A, Moutaux E, Christaller W, Genoux A, Bruyere J, Fino E, et al. Reconstituting corticostriatal network on-a-chip reveals the contribution of the presynaptic compartment to Huntington's disease. Cell Rep. (2018) 22:110–22. doi: 10.1016/j.celrep.2017.12.013
172. Bakkum DJ, Frey U, Radivojevic M, Russell TL, Muller J, Fiscella M, et al. Tracking axonal action potential propagation on a high-density microelectrode array across hundreds of sites. Nat Commun. (2013) 4:2181. doi: 10.1038/ncomms3181
173. Bassett DS, Bullmore E. Small-world brain networks. Neuroscientist. (2006) 12:512–23. doi: 10.1177/1073858406293182
174. Goel A, Buonomano DV. Timing as an intrinsic property of neural networks: evidence from in vivo and in vitro experiments. Philos Trans R Soc Lond B Biol Sci. (2014) 369:20120460. doi: 10.1098/rstb.2012.0460
175. Watts DJ, Strogatz SH. Collective dynamics of 'small-world' networks. Nature. (1998) 393:440–2. doi: 10.1038/30918
176. Tang-Schomer MD, Jackvony T, Santaniello S. Cortical network synchrony under applied electrical field in vitro. Front Neurosci. (2018) 12:630. doi: 10.3389/fnins.2018.00630
177. Mcculloch WS, Pitts W. A logical calculus of the ideas immanent in nervous activity. Bull Math Biol. (1990) 52:99–115; Discussion 73–97. doi: 10.1007/BF02459570
178. Ben Amor H, Corblin F, Fanchon E, Elena A, Trilling L, Demongeot J, et al. Formal methods for hopfield-like networks. Acta Biotheor. (2013) 61:21–39. doi: 10.1007/s10441-013-9169-5
179. Barak O. Recurrent neural networks as versatile tools of neuroscience research. Curr Opin Neurobiol. (2017) 46:1–6. doi: 10.1016/j.conb.2017.06.003
180. Kuroki S, Isomura T. Task-related synaptic changes localized to small neuronal population in recurrent neural network cortical models. Front Comput Neurosci. (2018) 12:83. doi: 10.3389/fncom.2018.00083
181. Miconi T. Biologically plausible learning in recurrent neural networks reproduces neural dynamics observed during cognitive tasks. Elife. (2017) 6:20899. doi: 10.7554/eLife.20899
182. Nichele S, Giskeodegard A, Tufte G. Evolutionary growth of genome representations on artificial cellular organisms with indirect encodings. Artif Life. (2016) 22:76–111. doi: 10.1162/ARTL_a_00191
183. Aaser P, Knudsen M, Huse Ramstad O, Van De Wijdewen R, Nichele S, Sandvig I, et al. Towards making a cyborg: A closed-loop reservoirneuro system. In: European Conference on Artificial Life. MIT Press (2017). p. 430–7.
184. Demarse TB, Wagenaar DA, Blau AW, Potter SM. The neurally controlled animat: biological brains acting with simulated bodies. Auton Robots. (2001) 11:305–10. doi: 10.1023/A:1012407611130
185. Chao ZC, Bakkum DJ, Potter SM. Shaping embodied neural networks for adaptive goal-directed behavior. PLoS Comput Biol. (2008) 4:E1000042. doi: 10.1371/journal.pcbi.1000042
Keywords: neural networks, neuroengineering, cell reprogramming, disease modeling, multielectrode arrays, computational modeling, electrophysiology, cell therapy
Citation: Sandvig A and Sandvig I (2019) Connectomics of Morphogenetically Engineered Neurons as a Predictor of Functional Integration in the Ischemic Brain. Front. Neurol. 10:630. doi: 10.3389/fneur.2019.00630
Received: 18 January 2019; Accepted: 28 May 2019;
Published: 12 June 2019.
Edited by:
Swathi Kiran, Boston University, United StatesReviewed by:
Magdalena Götz, Helmholtz Center Munich, GermanyZaal Kokaia, Lund Stem Cell Center, Sweden
Copyright © 2019 Sandvig and Sandvig. This is an open-access article distributed under the terms of the Creative Commons Attribution License (CC BY). The use, distribution or reproduction in other forums is permitted, provided the original author(s) and the copyright owner(s) are credited and that the original publication in this journal is cited, in accordance with accepted academic practice. No use, distribution or reproduction is permitted which does not comply with these terms.
*Correspondence: Ioanna Sandvig, aW9hbm5hLnNhbmR2aWdAbnRudS5ubw==