- 1Department of Emergency, The Second Affiliated Hospital of Wannan Medical College, Wuhu, China
- 2School of Clinical Medicine, Wannan Medical College, Wuhu, China
- 3Department of Neurology, The Second Affiliated Hospital of Wannan Medical College, Wuhu, China
Background: Post-stroke cognitive impairment (PSCI) after lacunar infarction was worth attention in recent years. An easy-to-use score model to predict the risk of PSCI was rare. This study aimed to explore the association between serum amyloid A (SAA) and cognitive impairment, and it also developed a nomogram for predicting the risk of PSCI in lacunar infarction patients.
Methods: A total of 313 patients with lacunar infarction were enrolled in this retrospective study between January 2021 and December 2021. They were divided into a training set and a validation set at 70%:30% randomly. The Chinese version of the Mini-Mental State Examination (MMSE) was performed to identify cognitive impairment 3 months after discharge. Univariate and multivariate logistic regression analyses were used to determine the independent risk factors for PSCI in the training set. A nomogram was developed based on the five variables, and the calibration curve and the receiver operating characteristic (ROC) curve were drawn to assess the predictive ability of the nomogram between the training set and the validation set. The decision curve analysis (DCA) was also conducted in both sets.
Results: In total, 52/313 (16.61%) participants were identified with PSCI. The SAA levels in patients with PSCI were significantly higher than non-PSCI patients in the training set (P < 0.001). After multivariate analysis, age, diabetes mellitus, white blood count, cystatin C, and SAA were independent risk predictors of PSCI. The nomogram demonstrated a good discrimination performance between the training set (AUC = 0.860) and the validation set (AUC = 0.811). The DCA showed that the nomogram had a well clinical utility in the two sets.
Conclusion: The increased SAA is associated with PSCI in lacunar infarction patients, and the nomogram developed with SAA can increase prognostic information for the early detection of PSCI.
Introduction
Stroke is the second leading cause of death around the world, which endangers the life quality and safety of patients due to high morbidity and high disability (1). Especially, post-stroke cognitive impairment (PSCI) is the most common critical issue concerning population health and the burden on caregivers in an aging society (2, 3). The survivors of stroke have an increased risk of progressive cognitive impairment, even minor stroke (4). The presence of PSCI also affects the treatment of stroke patients in turn and nearly increases two-fold the risk of adverse outcomes (5). Lacunar infarction accounts for about 25% of stroke patients, and approximately half of the patients develop cognitive impairment in subsequent years (6). With the increased duration of ischemia and decreased mortality with minor stroke, the number of patients with PSCI will be increased (7). Therefore, it is crucial to realize the associations between PSCI and predictive factors, especially in high-risk patients.
The available evidence confirms that hypertension, diabetes, smoking, and other vascular risk factors are highly correlated with the increased risks of PSCI (8). Besides, frontal lobe dysfunction and brain gray matter atrophy were also associated with cognitive impairment in lacunar patients (9). Recently, the developed SIGNAL2 scale and CHANGE scale based on the clinical characteristics and neuroimaging variables were useful to identify PSCI after stroke (10, 11). However, the scale depends on the neurologist's appraising of the MRI and was difficult to promote in the community. In addition, the expression of biomarkers, such as interleukin 6 (IL-6), C-reactive protein (CRP), serum uric acid (UA), and malondialdehyde (MDA), was also independently associated with PSCI in increasing studies (12, 13). Therefore, it is necessary to explore reliable biomarkers to identify patients at higher risk of PSCI easily and conveniently.
Serum amyloid A (SAA) protein is a protein of only 104 amino acids and is mainly synthesized in the liver (14). As an acute phase protein (APP), SAA was significantly upregulated in acute and chronic inflammatory conditions (such as trauma, infection, and ischemia), which was in response to the elevator of the inflammatory cytokines IL-6 and tumor necrosis factor (TNF)-α during the acute-phase response (15). Schweizer et al.'s study (16) found that SAA was a novel blood biomarker, which was independent to predict post-stroke infection among ischemic stroke patients. A recent study confirmed that the increased secretion of SAA could activate the inflammatory response of microglia and stimulate NLRP3 activation in microglia after stroke, which induced neurological inflammation (17). Although studies have indicated that elevated SAA was associated with short-term cognitive impairment after ischemic stroke (18), the role of SAA has not yet been evaluated in the cognitive impairment after lacunar infarction.
Therefore, we aimed to develop and verify a nomogram to predict the risk of PSCI in lacunar infarction patients, which will be convenient for clinicians to identify cognitive disorders early and conveniently.
Methods
Study design and patients
This study retrospectively enrolled patients with lacunar infarction who were hospitalized at the Second Affiliated Hospital of Wannan Medical College between January 2021 and December 2021. All patients were admitted to the hospital within 7 days of symptom onset with a National Institute of Health Stroke Scale (NIHSS) score ≤3. This study was approved by the Institutional Review Board of the Second Affiliated Hospital of Wannan Medical College (No. WYEFYLS202205) and conducted by the guiding principles of the Declaration of Helsinki.
The inclusion criteria were as follows: (1) age >18 years; (2) patients who met the diagnostic criteria for lacunar infarction confirmed on cranial computed tomography (CT) scan or magnetic resonance imaging (MRI) examination; and (3) patients who were able to complete scale measurements. The patients were excluded if they had any of the following: (1) previous diagnosis with dementia or Alzheimer's disease; (2) cardioembolic source or large-vessel diseases (large artery stenosis >50%); (3) patients with incomplete clinical data; (4) had been treated with intervention and thrombolytic therapy; and (5) loss to follow-up.
Finally, a total of 313 patients were enrolled in this study and were randomly divided into a training set and a validation set at 70%:30% (Figure 1).
Baseline clinical characteristics collection
The baseline clinical characteristics were collected within 24 h of admission from the health information system (HIS). The first part was demographic characteristics, such as age, gender, education time, initial National Institutes of Health Stroke Scale (NIHSS) score, and vital signs (blood pressure, heart rate, temperature, breath rate). The second part was comorbidities (diabetes mellitus, atrial fibrillation, coronary heart disease, hypertension, tumor, and chronic obstructive pulmonary disease). The third part was laboratory examinations, which included red blood count, white blood count, hemoglobin, platelet, cystatin C, and total cholesterol.
Serum amyloid A
All blood samples were collected in the morning from all patients within 24 h of admission, and all patients were fasting for more than 8 h. The blood samples were collected with heparin anticoagulant tubes and centrifuged at 1,000 g for 5 min to separate serum. The serum amyloid A level was measured by the latex-enhanced immunoturbidimetric method with the automatic biochemical analyzer (Hitachi-7600). The reference value of serum amyloid A ranged from 0 to 10.00 mg/L. All sample testing was performed by laboratory personnel blinded to the study.
Lacunar infarction definition
All patients routinely completed MRI examination or CT scanning. Lacunar infarction was defined as lacunar infarcts <20 mm in the subcortical or brain stem verified by CT or MRI (19, 20). Radiologic images were read by a radiologist and were reviewed by an experienced neurologist.
Assessment of cognitive function
Cognitive function was evaluated by experienced neurological physicians using the Chinese version of the Mini-Mental State Examination (MMSE) scale 3 months after lacunar infarction (21). The MMSE scale was widely used in cognitive function assessment in China (22, 23). The total score of MMSE was 30, and the lower scores indicated the worse cognitive function. According to the previous studies, a score of MMSE <24 was considered as a cognitive impairment in this study (24, 25).
Statistical analysis
The statistical analysis was performed by using SPSS 25.0 and R software (version 3.6.2). Continuous variables with normal distribution were expressed as mean ± standard deviation, and the interquartile range were not normally distributed. Categorical variables were presented as frequency (percentage).
Univariate analysis was applied to screen the potential risk factors for PSCI. To determine independent risk factors for PSCI in lacunar infarction patients, the variables with P < 0.05 in the univariate analysis were included in the multivariate logistic regression model. Then, the predictive nomogram was developed based on the independent risk factors by using the “rms” package in R software. The receiver operating characteristic (ROC) curve was generated, and the area under the curve (AUC) of ROC was used to evaluate the discrimination of the nomogram. Meanwhile, the AUC of the nomogram was compared with all the independent risk variables. Finally, the net benefit of the decision curve analysis (DCA) was drawn to estimate the clinical value of the nomogram in the training set and validation set, respectively. Linear regression analysis was used to analyze the association between the serum SAA and the MMSE score. The statistical significance for all variables was set at P < 0.05 (two-sided tests), and the regression coefficients reported 95% confidence intervals (CI).
Results
Clinical characteristics of patients in the training and validation set
A total of 313 patients with lacunar infarction were included in this study, of which 219 patients were in the training set and 94 patients were in the validation set; 38 (17.40%) patients in the training set and 14 (14.90%) patients in the validation set were diagnosed with PSCI, respectively. The characteristics of patients in the training set and the validation set were no significant differences in Table 1 (P > 0.05).
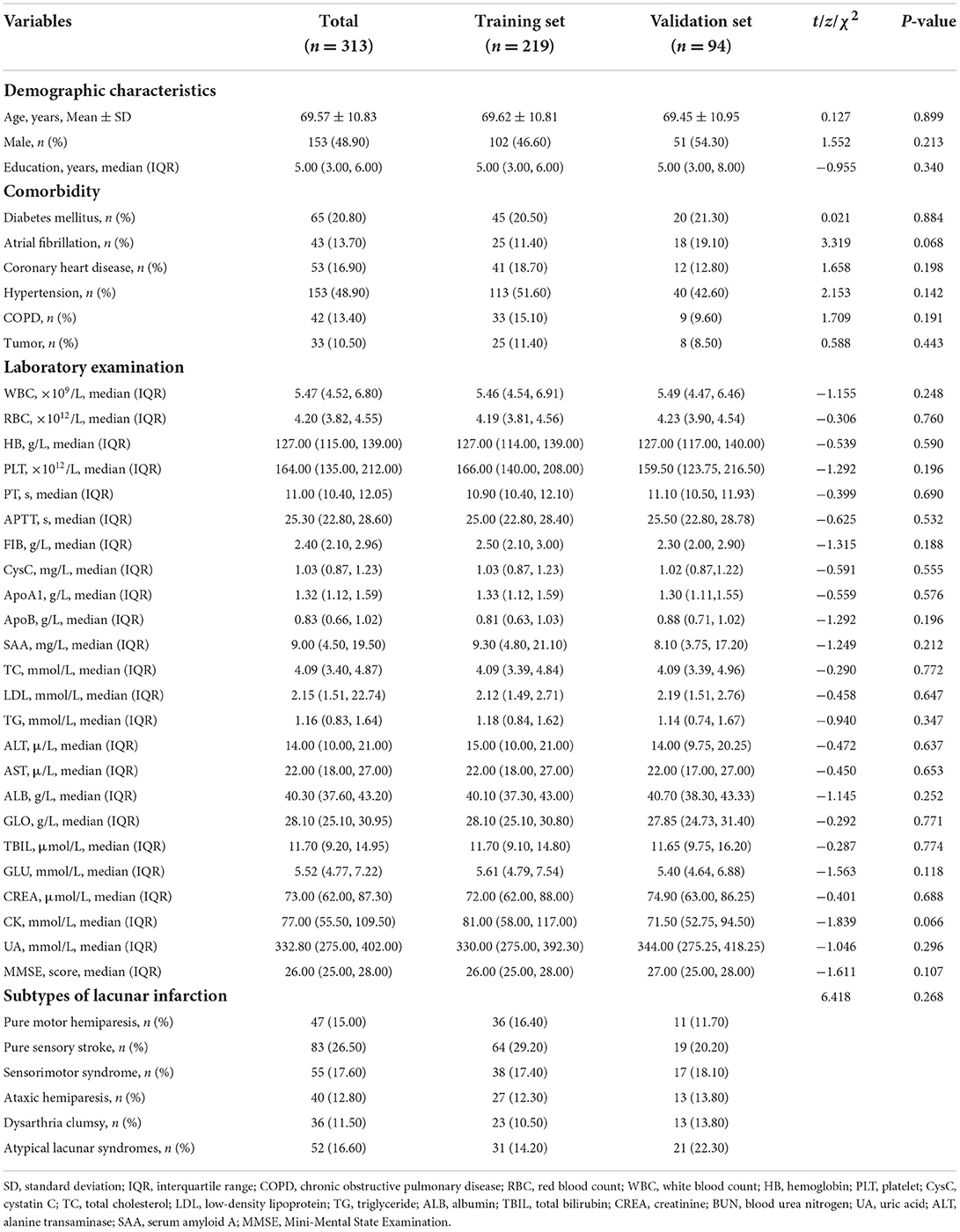
Table 1. Baseline and clinical characteristics of lacunar infarction patients in the training set and validation set.
Baseline characteristics of patients stratified by PSCI in the training set
Descriptive analysis revealed that significant differences between the two groups were confirmed for age (P < 0.001), years of education (P = 0.001), diabetes mellitus (P = 0.006), white blood count (P < 0.001), APTT (P = 0.036), cystatin C (P < 0.001), ApoA1 (P = 0.005), and serum amyloid A (P < 0.001) in Table 2.
Identifying the independent risk factors for PSCI
All the potential risk factors (P < 0.05) in the univariate regression analysis were included in the multivariate regression model. Multivariate logistic regression analysis revealed that age (OR = 1.099, 95%CI: 1.012–1.193, P = 0.025), diabetes mellitus (OR = 2.679, 95% CI: 1.029–6.976, P = 0.044), white blood count (OR = 1.271, 95% CI: 1.028–1.572, P = 0.027), cystatin C (OR = 3.118, 95% CI:1.053–9.228, P = 0.040), and serum amyloid A (OR = 1.031, 95% CI: 1.009–1.054, P = 0.007) were independent risk predictors of PSCI in patients with lacunar infarction (Table 3).
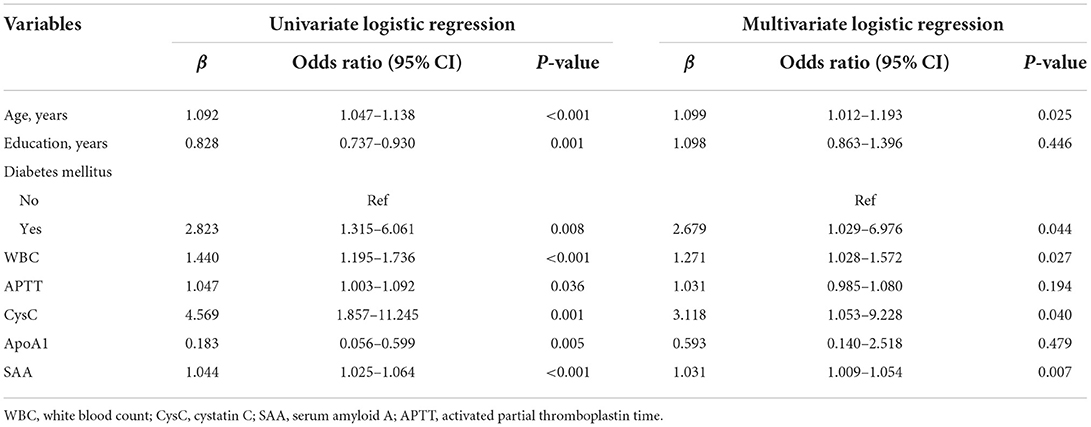
Table 3. Univariate and multivariate logistic regression analyses of risk factors for PSCI in the training set.
The predictive nomogram development
The nomogram was developed for predicting the risk of PSCI probability based on the results from the multivariate logistic model, which included five variables (Figure 2). A vertical line was drawn up to the “Point” axis to calculate the score of each variable, and the total score was summarized by the preliminary scores. The total score was located on the “Total Points” axis, and then, the predicted risk of PSCI probability could be located on the bottom axis.
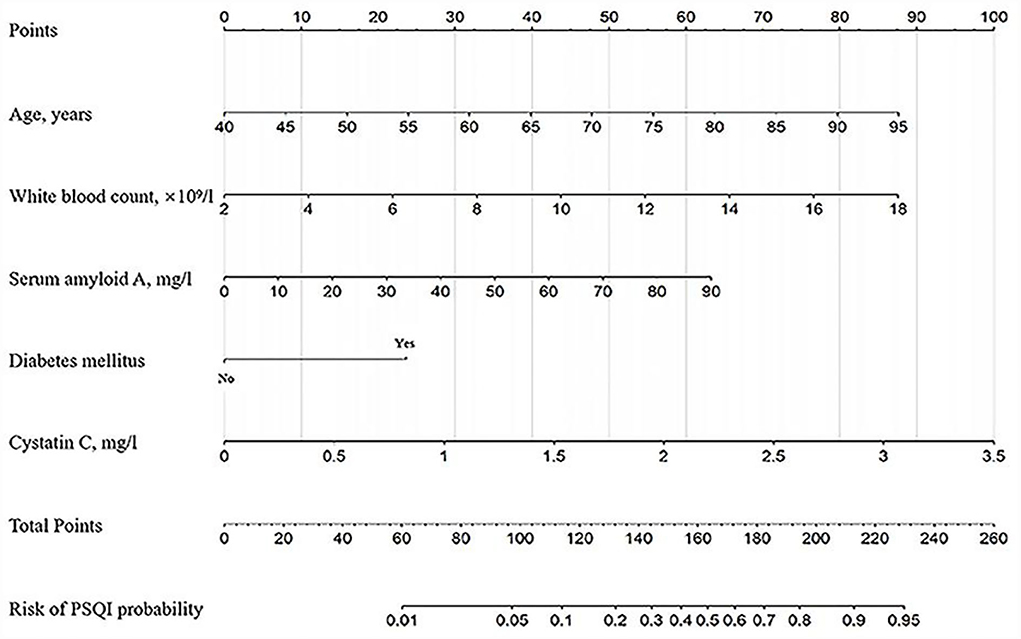
Figure 2. The nomogram for predicting the risk of PSCI probability based on the 5 independent prognostic factors. For example, a 65-year-old (40 points) patient with diabetes mellitus (25 points) with white blood counts of 12.0 × 109/L (55 points), serum amyloid A of 50 mg/L (35 points), and cystatin C of 1.5 mg/L (42 points) arrived at a total point of 197, with a probability of 80% to develop PSCI.
The performance of the nomogram
The calibration curve of the nomogram for the probability of PSCI demonstrated a good agreement between prediction and observation for both sets (Figure 3). The Hosmer-Lemeshow H test indicated that the model did not depart from perfect fit, which had non-statistical significance in the training set (P = 0.336) and validation set (P = 0.399).
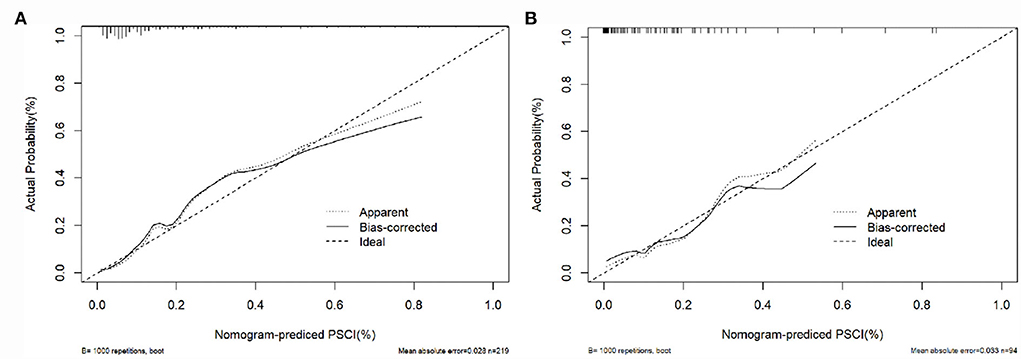
Figure 3. Calibration curves of the nomogram in the training set and the validation set. (A) The nomogram in the training set (n = 219); (B) the nomogram in the validation set (n = 94). The y-axis represents the observed rate of PSCI, and the x-axis represents the nomogram-predicted probability of PSCI. The dotted lines represented by the nomogram are closer to the diagonal gray lines representing a better prediction.
The AUC for the nomogram was 0.860 (95% CI: 0.794–0.925) in the training set (Figure 4A) and was confirmed to be 0.811 (95% CI: 0.686–0.936) through internal validation in the validation set (Figure 4B), which demonstrated that the nomogram had a greater discriminatory performance. In addition, the discrimination ability of the nomogram calculated by the AUC was superior to the other risk factors in the training set: age (0.734, 95%CI: 0.643–0.824, P < 0.001), diabetes mellitus (0.599, 95% CI: 0.493–0.704, P = 0.056), white blood count (0.691, 95% CI: 0.592–0.791, P < 0.001), cystatin C (0.711, 95% CI: 0.625–0.797, P < 0.001), and serum amyloid A (0.760, 95% CI: 0.674–0.846, P < 0.001; Figure 4A).
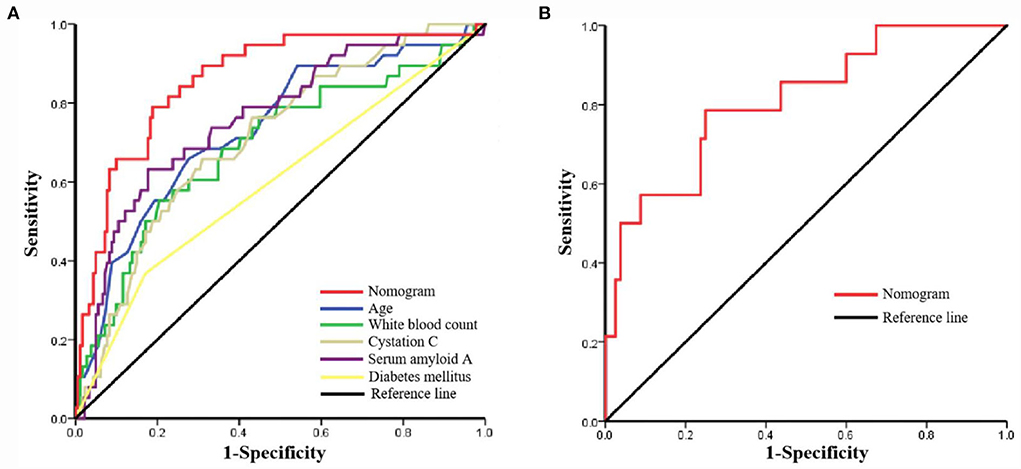
Figure 4. The receiver operating characteristic (ROC) curve of the nomogram in the training set and the validation set. (A) ROC in the training set; (B) ROC in the validation set.
Clinical use
Moreover, the DCA was used to assess the clinical validity of the nomogram, which indicated the predictive nomogram to be clinically useful (Figure 5).
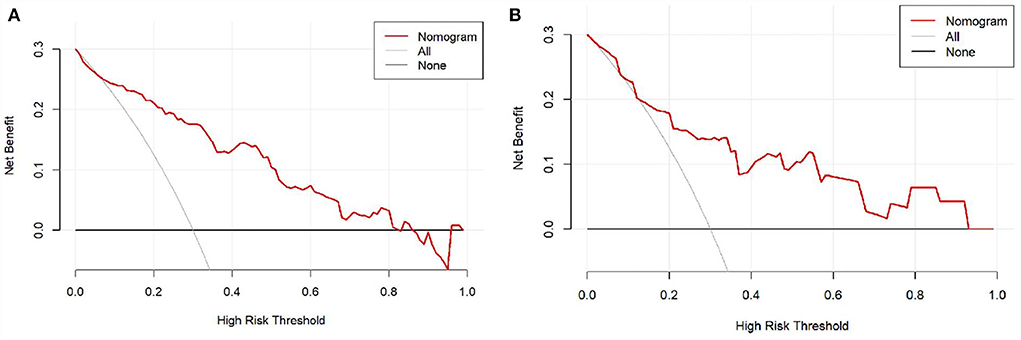
Figure 5. The decision curve analysis (DCA) of the nomogram in the training set and validation set. (A) DCA in the training set; (B) DCA in the validation set.
The association between the serum SAA and the probability of PSCI, the serum SAA, and the MMSE score
We found a positive correlation between the serum SAA and the probability of PSCI, in which the predicted probabilities for PSCI were more than 50% after 58 mg/L of serum amyloid A (Figure 6A). Besides, linear regression analysis showed that the level of serum SAA was negatively associated with the MMSE score (regression equation: y = 54.75–1.54x, P < 0.001; Figure 6B).
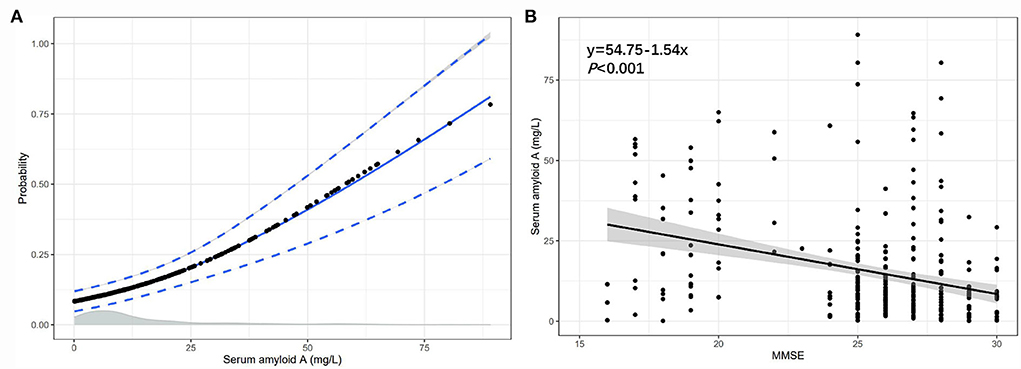
Figure 6. The association between the serum SAA and the probability of PSCI, the serum SAA, and the MMSE score. (A) The association of SAA levels with the probability of PSCI in patients with lacunar infarction; (B) The association between SAA levels and MMSE score.
Discussion
Post-stroke cognitive impairment is a clinical syndrome of cognitive impairment that occurs after an ischemic stroke (18). Approximately 37.3% of respondents developed PSCI in a retrospective study of 209 patients with mild ischemic stroke (11). Therefore, it is important to assess PSCI early and conveniently. In this study, we investigated the association of SAA concentrations with the risk of cognitive impairment after lacunar infarction. Several pivotal results were found in this analysis. First, the increased SAA levels were significantly associated with a higher risk of PSCI. Second, we proved that several traditional risk factors, such as age, diabetes mellitus, white blood count, and cystatin C, were independent risk predictors of PSCI in patients with lacunar infarction. Third, we constructed a nomogram model based on SSA that can predict PSCI effectively.
Each brain's morphology is unique, and aging changes brain morphology in both healthy and pathological conditions (26). Heart failure, atrial fibrillation, and renal insufficiency may contribute to acute stroke with increasing age, especially in patients over 85 years of age (27). Overton's research found that the older age groups had more possibility of having cognitive impairment (28). Morley's study also came to the data that nearly 40% of the persons older than 65 years had mild cognitive impairment in the United States (29). In our study, we found a positive association between age and the occurrence of cognitive impairment after lacunar infarction. The key point of brain aging is the cellular senescence of neurons and microglia (30). Evidence proved that since the age of 40 years, about 5% of neuron cells are destroyed every 10 years, which directly leads to a decrease in brain volume (29). In addition, as an important component of immunity for the central nervous system, microglia plays an indispensable role to maintain tissue homeostasis (31). Since microglia are found around lesions in neurodegenerative diseases such as Alzheimer's disease, Parkinson's disease, and multiple sclerosis, the release of inflammatory factors mediated by microglia is thought to be the key to the onset or progression of neurodegenerative diseases (32).
Diabetes mellitus is a kind of noninfectious and multiple organs affected chronic disease (33). Liccini's research concluded that 20% of patients aged between 50 and 65 years were diagnosed with diabetes mellitus who had cognitive impairment, and the situation may be more severe in diabetes mellitus who had metabolic syndrome (34–36). Van Sloten et al. revealed that diabetes-related microvascular dysfunction affected the exchange of gases, nutrients, proteins, and metabolites in the human body environment (37), which was a key factor in the occurrence of cognitive impairment (38). In addition, the health of people with diabetes could be negatively affected due to cognitive impairment. Therefore, we need to face up to the fact that stroke patients with diabetes have more prevalence of cognitive impairment (39), and clinicians should pay more attention to the cognitive abilities of diabetes patients.
Inflammatory responses are closely related to ischemic stroke; it could promote the development of ischemic injury and neuronal death after stroke (40, 41). The higher white cell counts within the normal range were associated with cognitive impairment in older adults (42, 43). Studies based on population have confirmed the relationship between inflammation and cognitive impairment, that is, the inflammatory states can negatively impact cognitive function (44, 45). Furthermore, the animal experiment revealed that white blood cells promoted the immune system to degenerate brain tissue in stroke animal models (46). In our study, we confirmed that white blood count played an important role in predicting cognitive impairment after lacunar infarction. This suggested that neuronal inflammation prevention may reduce cognitive impairment and improve neurological outcomes in stroke patients.
Cystatin C is an endogenous cysteine proteinase inhibitor that exists nearly in all human cells and body fluids; it belongs to the type 2 cystatin superfamily (47, 48). Sarnak's research showed that higher levels of cystatin C were associated with cognitive impairment (49). Meanwhile, the higher serum cystatin C was an independent risk factor for PSCI in patients with acute mild ischemic stroke (50), which can provide early prediction of cognitive decline in the elderly (51). This is consistent with the conclusion of our study. Cognitive impairment could have a negative impact on the daily life of patients; therefore, reducing the level of serum cystatin C may provide a new treatment for the prevention of PSCI, and it is of great significance to timely predict the occurrence of cognitive impairment (52).
Serum amyloid A is a protein secreted by hepatocytes (53). The synthesis of SAA is associated with inflammatory cytokines, which can rise rapidly when infection and inflammation occur (18, 54). It is widely used as a follow-up marker for diagnosis, prognosis, or treatment of disease (55, 56). SAA has been recognized as being associated with cognitive impairment (57). Xu's research found the relationship between cognitive function and SAA levels in patients with vascular dementia and investigated the higher levels of SAA in patients with vascular dementia (58, 59). The elevation of SAA exacerbates neuroinflammation and changes the morphology of microglia to increase their activity, eventually leading to brain damage and memory loss (54, 60, 61). Therefore, for patients with lacunar infarction with elevated SAA, it is necessary for clinicians and healthcare organizations to take preventive actions against cognitive impairment that may occur in the future.
The nomogram based on the five variables would improve the predictive ability for PSCI in lacunar infarction patients. Compared with five independent risk factors, the nomogram exhibited good discrimination ability by the ROC analysis. In addition, DCA was applied in the training set and validation set, which confirmed the net benefit based on the threshold probability.
There were some limitations in this study. First, the study detected only the serum SAA levels within 24 h of admission, but did not examine the serum SAA levels before the stroke and within 3 months of discharge dynamically. Second, the patients did not perform the cognitive function assessment during admission, although patients with neurological disease and dementia were excluded. Third, the independent variables included in the study lack the relevant indicators of magnetic resonance imaging (cerebral atrophy and gray matter lesions), genetic risk factors, and environmental risk factors. Finally, there might be some bias in the selection of patients, because this study enrolled mild stroke patients with NHISS <3 only. Therefore, these issues need further exploration in the future prospective external studies.
Conclusion
This study revealed the association of SAA level with PSCI, which was an independent risk factor to predict cognitive impairment in lacunar infarction patients. In addition, this study constructed the nomogram to predict PSCI based on the five independent risk factors, which has proven clinical utility and is useful for PSCI risk decision-making in patients with lacunar infarction undergoing clinical assessment.
Data availability statement
The original contributions presented in the study are included in the article/supplementary material, further inquiries can be directed to the corresponding author.
Ethics statement
The studies involving human participants were reviewed and approved by the Institutional Review Board of the Second Affiliated Hospital of Wannan Medical College. The patients/participants provided their written informed consent to participate in this study. Written informed consent was obtained from the individual(s) for the publication of any potentially identifiable images or data included in this article.
Author contributions
SY and LX designed this study and provided the funding support. SY and HP drafted the first manuscript and analyzed the data. JX, WL, and BW took part in the sample collection and acquired the data. LX and BW followed up with the patient. LX reviewed and edited the manuscript. All authors have read and approved the final manuscript.
Funding
This study was financially supported by the Key Research Fund Project of Wannan Medical College (WK2021ZF24 and WK2021ZF25) and the Summit plan of the Second Affiliated Hospital of Wannan Medical College (DFJH2022007).
Conflict of interest
The authors declare that the research was conducted in the absence of any commercial or financial relationships that could be construed as a potential conflict of interest.
Publisher's note
All claims expressed in this article are solely those of the authors and do not necessarily represent those of their affiliated organizations, or those of the publisher, the editors and the reviewers. Any product that may be evaluated in this article, or claim that may be made by its manufacturer, is not guaranteed or endorsed by the publisher.
References
1. Tao X, Qiu C, Feng X, Wang L. Predictive analysis of serum NO, PGI2, and Ox-LDL levels on disease progression in patients with lacunar cerebral infarction. Comput Math Methods Med. (2022) 2022:1221810. doi: 10.1155/2022/1221810
2. Nakamori M, Matsushima H, Tachiyama K, Hayashi Y, Imamura E, Mizoue T, et al. Association of ankle-brachial index with cognitive decline in patients with lacunar infarction. PLoS ONE. (2022) 17:e0263525. doi: 10.1371/journal.pone.0263525
3. Teng Z, Dong Y, Zhang D, An J, Lv P. Cerebral small vessel disease and post-stroke cognitive impairment. Int J Neurosci. (2017) 127:824–30. doi: 10.1080/00207454.2016.1261291
4. Mijajlovic MD, Pavlovic A, Brainin M, Heiss WD, Quinn TJ, Ihle-Hansen HB, et al. Post-stroke dementia - a comprehensive review. BMC Med. (2017) 15:11. doi: 10.1186/s12916-017-0779-7
5. D'Souza CE, Greenway MRF, Graff-Radford J, Meschia JF. Cognitive impairment in patients with stroke. Semin Neurol. (2021) 41:75–84. doi: 10.1055/s-0040-1722217
6. Cukierman-Yaffe T, McClure LA, Risoli T, Bosch J, Sharma M, Gerstein HC, et al. The relationship between glucose control and cognitive function in people with diabetes after a lacunar stroke. J Clin Endocrinol Metab. (2021) 106:e1521–e8. doi: 10.1210/clinem/dgab022
7. Kwon HS, Lee D, Lee MH Yu S, Lim JS Yu KH, et al. Post-stroke cognitive impairment as an independent predictor of ischemic stroke recurrence: PICASSO sub-study. J Neurol. (2020) 267:688–93. doi: 10.1007/s00415-019-09630-4
8. Lo Coco D, Lopez G, Corrao S. Cognitive impairment and stroke in elderly patients. Vasc Health Risk Manag. (2016) 12:105–16. doi: 10.2147/VHRM.S75306
9. Grau-Olivares M, Arboix A, Junque C, Arenaza-Urquijo EM, Rovira M, Bartres-Faz D. Progressive gray matter atrophy in lacunar patients with vascular mild cognitive impairment. Cerebrovasc Dis. (2010) 30:157–66. doi: 10.1159/000316059
10. Chander RJ, Lam BYK, Lin X, Ng AYT, Wong APL, Mok VCT, et al. Development and validation of a risk score (CHANGE) for cognitive impairment after ischemic stroke. Sci Rep. (2017) 7:12441. doi: 10.1038/s41598-017-12755-z
11. Kandiah N, Chander RJ, Lin X, Ng A, Poh YY, Cheong CY, et al. Cognitive impairment after mild stroke: development and validation of the SIGNAL2 risk score. J Alzheimers Dis. (2016) 49:1169–77. doi: 10.3233/JAD-150736
12. Zhang X, Bi X. Post-stroke cognitive impairment: a review focusing on molecular biomarkers. J Mol Neurosci. (2020) 70:1244–54. doi: 10.1007/s12031-020-01533-8
13. Sun J, Lv X, Gao X, Chen Z, Wei D, Ling Y, et al. The association between serum uric acid level and the risk of cognitive impairment after ischemic stroke. Neurosci Lett. (2020) 734:135098. doi: 10.1016/j.neulet.2020.135098
14. Sack GH Jr. Serum amyloid A (SAA) proteins. Subcell Biochem. (2020) 94:421–36. doi: 10.1007/978-3-030-41769-7_17
15. Abouelasrar Salama S, Gouwy M, Van Damme J, Struyf S. The turning away of serum amyloid A biological activities and receptor usage. Immunology. (2021) 163:115–27. doi: 10.1111/imm.13295
16. Schweizer J, Bustamante A, Lapierre-Fetaud V, Faura J, Scherrer N, Azurmendi Gil L, et al. SAA (Serum Amyloid A): a novel predictor of stroke-associated infections. Stroke. (2020) 51:3523–30. doi: 10.1161/STROKEAHA.120.030064
17. Yu J, Zhu H, Taheri S, Mondy W, Bonilha L, Magwood GS, et al. Serum amyloid A-mediated inflammasome activation of microglial cells in cerebral ischemia. J Neurosci. (2019) 39:9465–76. doi: 10.1523/JNEUROSCI.0801-19.2019
18. Zhang Y, Feng Y, Zuo J, Shi J, Zhang S, Yang Y, et al. Elevated serum amyloid A is associated with cognitive impairment in ischemic stroke patients. Front Neurol. (2021) 12:789204. doi: 10.3389/fneur.2021.789204
19. Su JH, Meng LW, Dong D, Zhuo WY, Wang JM, Liu LB, et al. Noninvasive model for predicting future ischemic strokes in patients with silent lacunar infarction using radiomics. BMC Med Imaging. (2020) 20:77. doi: 10.1186/s12880-020-00470-7
20. Sun T, Xie T, Zhang A, Fan L, Xu Z, Chen X, et al. Relation between left atrial structure and lacunar infarction in patients with hypertension. Aging. (2020) 12:17295–304. doi: 10.18632/aging.103697
21. Folstein MF, Folstein SE, McHugh PR. “Mini-mental state”. A practical method for grading the cognitive state of patients for the clinician. J Psychiatr Res. (1975) 12:189–98. doi: 10.1016/0022-3956(75)90026-6
22. Yao YY, Wei ZJ, Zhang YC Li X, Gong L, Zhou JW, et al. Functional disability after ischemic stroke: a community-based cross-sectional study in Shanghai, China. Front Neurol. (2021) 12:649088. doi: 10.3389/fneur.2021.649088
23. Zhu Y, Fang C, Zhang Q, Lu Y, Zhang R, Wang A, et al. Soluble ST2 and risk of cognitive impairment after acute ischemic stroke: a prospective observational study. BMC Geriatr. (2021) 21:330. doi: 10.1186/s12877-021-02288-6
24. Zhou S, Chen J, Cheng L, Fan K, Xu M, Ren W, et al. Age-dependent association between elevated homocysteine and cognitive impairment in a post-stroke population: a prospective study. Front Nutr. (2021) 8:691837. doi: 10.3389/fnut.2021.691837
25. Sitzia C, Sterlicchio M, Crapanzano C, Dozio E, Vianello E, Corsi Romanelli MM. Intra-erythrocytes magnesium deficiency could reflect cognitive impairment status due to vascular disease: a pilot study. J Transl Med. (2020) 18:458. doi: 10.1186/s12967-020-02645-w
26. Blinkouskaya Y, Cacoilo A, Gollamudi T, Jalalian S, Weickenmeier J. Brain aging mechanisms with mechanical manifestations. Mech Ageing Dev. (2021) 200:111575. doi: 10.1016/j.mad.2021.111575
27. Arboix A, Miguel M, Ciscar E, Garcia-Eroles L, Massons J, Balcells M. Cardiovascular risk factors in patients aged 85 or older with ischemic stroke. Clin Neurol Neurosurg. (2006) 108:638–43. doi: 10.1016/j.clineuro.2005.10.010
28. Overton M, Pihlsgard M, Elmstahl S. Prevalence and incidence of mild cognitive impairment across subtypes, age, and sex. Dement Geriatr Cogn Disord. (2019) 47:219–32. doi: 10.1159/000499763
29. Morley JE. An overview of cognitive impairment. Clin Geriatr Med. (2018) 34:505–13. doi: 10.1016/j.cger.2018.06.003
30. Raz N, Daugherty AM. Pathways to brain aging and their modifiers: free-radical-induced energetic and neural decline in senescence (FRIENDS) model - a mini-review. Gerontology. (2018) 64:49–57. doi: 10.1159/000479508
31. Subhramanyam CS, Wang C, Hu Q, Dheen ST. Microglia-mediated neuroinflammation in neurodegenerative diseases. Semin Cell Dev Biol. (2019) 94:112–20. doi: 10.1016/j.semcdb.2019.05.004
32. Xu L, He D, Bai Y. Microglia-mediated inflammation and neurodegenerative disease. Mol Neurobiol. (2016) 53:6709–15. doi: 10.1007/s12035-015-9593-4
33. Faselis C, Katsimardou A, Imprialos K, Deligkaris P, Kallistratos M, Dimitriadis K. Microvascular complications of type 2 diabetes mellitus. Curr Vasc Pharmacol. (2020) 18:117–24. doi: 10.2174/1570161117666190502103733
34. Liccini A, Malmstrom TK, Morley JE. Metformin use and cognitive dysfunction among patients with diabetes mellitus. J Am Med Dir Assoc. (2016) 17:1063–5. doi: 10.1016/j.jamda.2016.08.026
35. Zilliox LA, Chadrasekaran K, Kwan JY, Russell JW. Diabetes and cognitive impairment. Curr Diab Rep. (2016) 16:87. doi: 10.1007/s11892-016-0775-x
36. Lyu F, Wu D, Wei C, Wu A. Vascular cognitive impairment and dementia in type 2 diabetes mellitus: an overview. Life Sci. (2020) 254:117771. doi: 10.1016/j.lfs.2020.117771
37. Horton WB, Barrett EJ. Microvascular dysfunction in diabetes mellitus and cardiometabolic disease. Endocr Rev. (2021) 42:29–55. doi: 10.1210/endrev/bnaa025
38. van Sloten TT, Sedaghat S, Carnethon MR, Launer LJ, Stehouwer CDA. Cerebral microvascular complications of type 2 diabetes: stroke, cognitive dysfunction, and depression. Lancet Diabetes Endocrinol. (2020) 8:325–36. doi: 10.1016/S2213-8587(19)30405-X
39. Ward R, Li W, Abdul Y, Jackson L, Dong G, Jamil S, et al. NLRP3 inflammasome inhibition with MCC950 improves diabetes-mediated cognitive impairment and vasoneuronal remodeling after ischemia. Pharmacol Res. (2019) 142:237–50. doi: 10.1016/j.phrs.2019.01.035
40. Quan K, Wang A, Zhang X, Wang Y. Leukocyte count and adverse clinical outcomes in acute ischemic stroke patients. Front Neurol. (2019) 10:1240. doi: 10.3389/fneur.2019.01240
41. Kim H, Seo JS, Lee SY, Ha KT, Choi BT, Shin YI, et al. AIM2 inflammasome contributes to brain injury and chronic post-stroke cognitive impairment in mice. Brain Behav Immun. (2020) 87:765–76. doi: 10.1016/j.bbi.2020.03.011
42. Kao TW, Chang YW, Chou CC, Hu J, Yu YH, Kuo HK. White blood cell count and psychomotor cognitive performance in the elderly. Eur J Clin Invest. (2011) 41:513–20. doi: 10.1111/j.1365-2362.2010.02438.x
43. Koren-Morag N, Tanne D, Goldbourt U. White blood cell count and the incidence of ischemic stroke in coronary heart disease patients. Am J Med. (2005) 118:1004–9. doi: 10.1016/j.amjmed.2005.03.010
44. Narasimhalu K, Lee J, Leong YL, Ma L, De Silva DA, Wong MC, et al. Inflammatory markers and their association with post stroke cognitive decline. Int J Stroke. (2015) 10:513–8. doi: 10.1111/ijs.12001
45. Halazun HJ, Mergeche JL, Mallon KA, Connolly ES, Heyer EJ. Neutrophil-lymphocyte ratio as a predictor of cognitive dysfunction in carotid endarterectomy patients. J Vasc Surg. (2014) 59:768–73. doi: 10.1016/j.jvs.2013.08.095
46. Ren X, Hu H, Farooqi I, Simpkins JW. Blood substitution therapy rescues the brain of mice from ischemic damage. Nat Commun. (2020) 11:4078. doi: 10.1038/s41467-020-17930-x
47. Mussap M, Plebani M. Biochemistry and clinical role of human cystatin C. Crit Rev Clin Lab Sci. (2004) 41:467–550. doi: 10.1080/10408360490504934
48. Cui Z, Cao G, Wang Y, Ma Q, Wang C, Xu Y, et al. Effects of cystatin C on cognitive impairment in older chinese adults. Am J Alzheimers Dis Other Demen. (2020) 35:1533317520965101. doi: 10.1177/1533317520965101
49. Sarnak MJ, Katz R, Fried LF, Siscovick D, Kestenbaum B, Seliger S, et al. Cystatin C and aging success. Arch Intern Med. (2008) 168:147–53. doi: 10.1001/archinternmed.2007.40
50. Yan X, Chen H, Shang XL. Association between serum cystatin C level and post-stroke cognitive impairment in patients with acute mild ischemic stroke. Brain Behav. (2022) 12:e2519. doi: 10.1002/brb3.2519
51. Wei Y, Wei YK, Zhu J. Early markers of kidney dysfunction and cognitive impairment among older adults. J Neurol Sci. (2017) 375:209–14. doi: 10.1016/j.jns.2017.01.071
52. Nair P, Misra S, Nath M, Vibha D, Srivastava AK, Prasad K, et al. Cystatin C and risk of mild cognitive impairment: A systematic review and meta-analysis. Dement Geriatr Cogn Disord. (2020) 49:471–82. doi: 10.1159/000510219
53. Matsumoto J, Dohgu S, Takata F, Iwao T, Kimura I, Tomohiro M, et al. Serum amyloid A-induced blood-brain barrier dysfunction associated with decreased claudin-5 expression in rat brain endothelial cells and its inhibition by high-density lipoprotein in vitro. Neurosci. Lett. (2020) 738:135352. doi: 10.1016/j.neulet.2020.135352
54. Malle E, Sodin-Semrl S, Kovacevic A. Serum amyloid A: an acute-phase protein involved in tumour pathogenesis. Cell Mol Life Sci. (2009) 66:9–26. doi: 10.1007/s00018-008-8321-x
55. De Buck M, Gouwy M, Wang JM, Van Snick J, Opdenakker G, Struyf S, et al. Structure and expression of different serum amyloid A (SAA) vari ants and their concentration-dependent functions during host insults. Curr Med Chem. (2016) 2016, 23, 1725-1755. doi: 10.2174/0929867323666160418114600
56. Sun L, Ye RD. Serum amyloid A1: structure, function and gene polymorphism. Gene. (2016) 583:48–57. doi: 10.1016/j.gene.2016.02.044
57. Salahudeen MS, Chyou TY, Nishtala PS. Serum anticholinergic activity and cognitive and functional adverse outcomes in older people: a systematic review and meta-analysis of the literature. PLoS ONE. (2016) 11:e0151084. doi: 10.1371/journal.pone.0151084
58. Xu M, He XY, Huang P. The Relationship between serum amyloid A level and cognitive dysfunction in patients with vascular dementia: preliminary findings. Biomed Res Int. (2021) 2021:6676144. doi: 10.1155/2021/6676144
59. Kisilevsky R, Manley PN. Acute-phase serum amyloid A: perspectives on its physiological and pathological roles. Amyloid. (2012) 19:5–14. doi: 10.3109/13506129.2011.654294
60. Zhang C, Liu Y, Gilthorpe J, van der Maarel JR. MRP14 (S100A9) protein interacts with Alzheimer beta-amyloid peptide and induces its fibrillization. PLoS ONE. (2012) 7:e32953. doi: 10.1371/journal.pone.0032953
Keywords: serum amyloid A, cognitive impairment, lacunar infarction, nomogram, prediction model
Citation: Ye S, Pan H, Li W, Wang B, Xing J and Xu L (2022) High serum amyloid A predicts risk of cognitive impairment after lacunar infarction: Development and validation of a nomogram. Front. Neurol. 13:972771. doi: 10.3389/fneur.2022.972771
Received: 19 June 2022; Accepted: 26 July 2022;
Published: 24 August 2022.
Edited by:
Keith Pennypacker, University of Kentucky, United StatesReviewed by:
Adria Arboix, Sacred Heart University Hospital, SpainChaur-Jong Hu, Taipei Medical University, Taiwan
Copyright © 2022 Ye, Pan, Li, Wang, Xing and Xu. This is an open-access article distributed under the terms of the Creative Commons Attribution License (CC BY). The use, distribution or reproduction in other forums is permitted, provided the original author(s) and the copyright owner(s) are credited and that the original publication in this journal is cited, in accordance with accepted academic practice. No use, distribution or reproduction is permitted which does not comply with these terms.
*Correspondence: Li Xu, xuliwyefy2022@163.com
†These authors have contributed equally to this work and share first authorship