- 1Department of Geriatrics, The Second Affiliated Hospital and Yuying Children’s Hospital of Wenzhou Medical University, Wenzhou, Zhejiang, China
- 2Department of Preventive Medicine, School of Public Health and Management, Wenzhou Medical University, Wenzhou, Zhejiang, China
- 3Department of Emergency, The First Affiliated Hospital of Wenzhou Medical University, Wenzhou, Zhejiang, China
Introduction: H-type hypertension (HHTN) is a subtype of hypertension that tends to worsen the prognosis of acute ischemic stroke (AIS). Recent studies have highlighted the vital role of gut microbiota in both hypertension and AIS, but there is little available data on the relationship between gut microbiota and the progression of AIS patients with HHTN. In this study, we investigated the microbial signature of AIS patients with HHTN and identified characteristic bacteria as biomarkers for predicting prognosis.
Methods: AIS patients with HHTN (n = 150) and without HHTN (n = 50) were enrolled. All patients received a modified Rankin Scale (mRS) assessment at 3 months after discharge. Fecal samples were collected from the participants upon admission, including 150 AIS patients with HHTN, 50 AIS patients with non-HHTN, and 90 healthy subjects with HHTN. These samples were analyzed using 16S rRNA sequencing to characterize the bacterial taxa, predict functions, and conduct correlation analysis between specific taxa and clinical features.
Results: Our results showed that the composition of the gut microbiota in HHTN patients differed significantly from that in non-HHTN patients. The abundance of the genera Bacteroides, Escherichia-Shigella, Lactobacillus, Bifidobacterium, and Prevotella in AIS patients with HHTN was significantly increased compared to AIS patients without HHTN, while the genus Streptococcus, Faecalibacterium, and Klebsiella were significantly decreased. Moreover, Bacteroides, Lactobacillus, Bifidobacterium, and Klebsiella in AIS patients with HHTN were more abundant than healthy subjects with HHTN, while Escherichia-Shigella, Blautia, and Faecalibacterium were less abundant. Moreover, the genera Butyricicoccus, Rothia, and Family_XIII_UCG-001 were negatively connected with the NIHSS score, and the genera Butyricicoccus and Rothia were observed to be negatively associated with the mRS score. The genera Butyricicoccus, Romboutsia, and Terrisporobacter were associated with a poor prognosis, whereas the increase in Butyricimonas and Odoribacter was correlated with good outcomes. Generated by eight genera and clinical indexes, the area under the curve (AUC) value of the receiver operating characteristic (ROC) curve achieved 0.739 to effectively predict the prognosis of AIS patients with HHTN.
Conclusion: These findings revealed the microbial signature of AIS patients with HHTN and further provided potential microbial biomarkers for the clinical diagnosis of AIS patients with HHTN.
1. Introduction
Acute ischemic stroke (AIS), one of the leading causes of disability and death worldwide (1, 2), is characterized by high incidence, high recurrence, and high mortality. Hypertension is regarded as a primary risk factor for AIS (1, 3), and most hypertensive patients are accompanied by an increase in serum homocysteine (Hcy) (4, 5), which is defined as H-type hypertension (HHTN), which means a combination of essential hypertension and a high level of Hcy (6). The prevalence of HHTN among hypertension patients varies widely, ranging from 75 to 80.3% (6, 7). Studies have reported that HHTN contributed to more cerebrovascular disease (CVD), such as stroke (8), and ultimately led to higher mortality (9, 10). Compared with simple stroke patients, patients with both HHTN and stroke were reported to have a higher death rate and a greater potential for cognitive disorders (11, 12).
To date, some clinicians primarily rely on risk factors such as hypertension, diabetes, dyslipidemia, and low folic acid (FA) to predict the outcomes of stroke patients (13, 14). The potential clinical risk factors increase the chance of adverse outcomes, and the biomarkers contribute to their prediction and clinical outcomes. Currently, the existing risk factors lack accuracy in evaluating the prognosis for AIS patients with HHTN. Thus, it is urgent to identify more effective biomarkers to predict the adverse outcome of the disease.
Recently, the close relationship between changes in gut microbiota (GM) composition and many diseases has been widely reported (15–17). It was reported that hypertension was closely related to the dramatically decreasing richness of the microbiota (18), and beneficial microbial supplementation was able to prevent the development of hypertension (19). GM also revealed its contribution to regulating hyperhomocysteinemia in Parkinson’s disease (PD) (20), and microbial treatment could mitigate the severity of hyperhomocysteinemia in mouse models (15). A growing body of evidence indicated that GM could be involved in the pathogenesis of neurological disorders (21, 22), such as stroke, and altered GM had been identified as one of the risk factors for stroke (23). Our previous studies revealed that the GM in patients with post-stroke depression (PSD) was characterized by the genera Streptococcus, Akkermansia, and Barnesiella, which were diagnostic microbial biomarkers of PSD (24).
Moreover, another study also demonstrated that post-stroke cognitive impairment (PSCI) patients observed a significant correlation with the abundance of Enterobacteriaceae, which was expected to be a biomarker of PSCI patients (25). Our previous study revealed that the abundance of Gammaproteobacteria and Enterobacteriaceae is negatively correlated with MoCA scores (26). Owing to the different expressions of GM in various apoplectic outcomes (27), some researchers propose using GM as an indicator to assess the prognosis of AIS (28). Moreover, bacteria producing short-chain fatty acids (SCFAs) were found to decrease in stroke patients with hyperlipidemia and the genera, including Faecalibacterium and Butyricicoccus, were able to facilitate the prognosis and diagnosis of stroke (29). Although diagnostic microbiota has been well studied in neurological diseases, the characteristic taxa in AIS patients and HHTN patients remain unclear.
To determine the microbial characteristics of AIS patients with HHTN, we conducted a prospective study to identify the characteristic taxa and their predictive role in adverse prognosis and further explored the relationship between GM, HHTN, and AIS. This study identified key bacteria that might be involved in the prognosis of AIS patients with HHTN.
2. Materials and methods
2.1. Participant recruitment
This study involved 200 AIS patients and 90 healthy participants with HHTN who were enrolled at the Second Hospital of Wenzhou Medical University in China from September 2020 to July 2021. The inclusion criteria for AIS patients were as follows: (1) admission within 72 h after the onset of AIS; (2) age ≥ 18 years; and (3) no special dietary habits, such as vegetarianism. The exclusion criteria were as follows: (1) patients who had taken medications such as antibiotics or prebiotics (within 3 months before admission) or medications affecting Hcy levels such as folic acid (FA), vitamins B6, and B12 (VB12) within 3 months; (2) secondary hypertensives caused by sleep apnea syndrome, pheochromocytoma, and so on; and (3) histories of severe gastrointestinal disease, gastrointestinal surgery, malignancy, or pregnancy. A total of 30 AIS patients and three healthy participants were excluded. Finally, we performed GM profiling on fecal samples from 290 subjects, including AIS patients with HHTN (ISHH), non-HHTN AIS patients (NISHH), and HHTN participants without AIS (HH). AIS patients were eligible for the diagnostic criteria of the American Stroke Association (30). ISHH patients were defined as having primary hypertension with systolic blood pressure (SBP) of ≥140 mmHg or diastolic blood pressure (DBP) of ≥90 mmHg, accompanied by Hcy of ≥10 μmol/L. NISHH patients were diagnosed as people without hypertension and Hcy of <10 μmol/L. This study was approved by the medical ethics committee of the Second Affiliated Hospital of Wenzhou Medical University, and informed consent was obtained from each participant.
2.2. Demographic and clinical characteristics
Demographic characteristics of each participant were collected by a trained researcher, which included age, gender, educational level, and marital status. Risky lifestyles (smoking and drinking) and previous histories such as diabetes, hyperlipidemia (HL), and CVD were also involved. Blood pressure was measured by a professionally trained nurse. At 0.5 h before blood pressure measurement, patients were advised to avoid strenuous exercise, smoking, coffee, and tea. The measurements were taken three times using an electronic sphygmomanometer, with a minimum rest period of 5 min between each measurement. The mean of these three values was calculated and recorded. Diabetes and hyperlipidemia were diagnosed by professionals in endocrinology. Laboratory indexes involved C-reactive protein (CRP), hypersensitive CRP (Hs-CRP), FA, Vitamin B12 (VB12), D-dimer, troponin, fasting glucose (FBG), glycosylated hemoglobin (HbAlc), triglycerides (TG), total cholesterol (TC), low-density lipoprotein cholesterol (LDL), high-density lipoprotein cholesterol (HDL), aspartate aminotransferase (AST), alanine aminotransferase (ALT), and thyroid stimulating hormone (TSH) were measured. The National Institutes of Health Stroke Scale (NIHSS) was used to assess the degree of neurological damage during hospitalization. The modified Rankin Scale (mRS) was used to assess the post-stroke functional prognosis of each patient 3 months later. In the current study, the mRS score of ≤1 was considered a good prognosis, while the mRS score of >1 was considered a poor prognosis.
2.3. Fecal collection and GM analysis
Fecal samples (200 mg) were obtained from patients during hospitalization or outpatient visits. Healthy control group members provided stool samples voluntarily at the health screening center. Each sample was collected and labeled using 2 mL sterile centrifuge tubes, immediately frozen in liquid nitrogen, and stored at −80°C. Total DNA was extracted from the stool samples by the E.Z.N.A.® Soil DNA Kit (Omega Bio-Tek, Norcross, GA, USA), and then, DNA concentration and purity were determined by using a NanoDrop2000 (Thermo Fisher Scientific, Wilmington, USA) for detection. Primers 338F (ACTCCTACGGGAGGCAGCAG) and 806R (GGACTACHVG GGTWTCTAAT) were involved in the PCR amplification of the high mutation region of 16 s rRNA. PCR products were recovered using a 2% agarose gel, sequenced on the Illumina MiSeq platform (Illumina, San Diego, USA), and spliced using FLASH software according to the manufacturer’s protocol.
The Ace and Shannon indices based on the Wilcoxon rank sum test were used to analyze the alpha diversity of the GM. Partial least squares-discriminant analysis (PLS-DA) was used to reflect differences in GM abundance in groups. Moreover, according to the relative abundance of GM, distributions of different groups at various taxonomic levels were determined, including phylum, class, order, family, genus, species, and ASV. In addition, based on the Kruskal–Wallis test, linear discriminant analysis (LDA) effect size (LEfSe) used an LDA score of >2 as the threshold. The Spearman correlation coefficient was used to evaluate the correlation between GM and clinical variables, visualized by heatmap. The receiver operating characteristic (ROC) curve was used to reflect the specificity and sensitivity of microbial characteristics in the prediction of stroke prognosis. All results of GM were performed by GraphPad Prism V.9.0.0 (La Jolla, CA, USA).
2.4. Statistical analysis
All data were analyzed by SPSS 26.0 software (SPSS, Chicago, USA). The continuous variables were expressed as the mean ± standard deviation (SD). The other variables were expressed by the median and quartiles. Categorical variables were expressed as numbers and percentages (%). The correlation between HHTN and prognosis was investigated by constructing the multivariate logistic regression models, and all variables were significantly different in the univariate analysis (p < 0.05). Relative risks were expressed as odds ratios (OR) with 95% confidence intervals (CI). A p-value of <0.05 was considered statistically significant.
3. Results
3.1. Baseline characteristics of ISHH, NISHH, and HH patients
This study enrolled 200 patients diagnosed with AIS, of whom 150 patients were assigned to the ISHH group and 50 to the NISHH group. The clinical demographics of ISHH and NISHH patients are presented in Table 1. In the ISHH group, the median age was 68 years old, and 70.7% were men, while in the NISHH group, the median age was 65 years old, and 48.0% were men. Diabetes, drinking, SBP, DBP, mean arterial pressure (MAP), mRS score, and laboratory indexes such as Hs-CRP, FA, VB12, uric acid (UA), Hcy, TG, HDL, and TSH showed significant differences between the ISHH and the NISHH groups. The marital status, educational level, CVD, hyperlipemia, smoking, and NIHSS score showed no significant differences between the two groups. Moreover, we recruited 90 healthy participants diagnosed with HHTN who were classified as HH. As shown in Table 1, the median age of HH participants was 71 years old, and 66.7% were men. Drinking, SBP, DBP, MAP, and laboratory indexes such as CRP, Hs-CRP, troponin, FBG, LDL, and FT3 showed differences between the ISHH and HH groups, while marital status, educational level, CVD, diabetes, hyperlipemia, and smoking showed no differences between the two groups. The multivariable logistic regression showed that the HHTN (OR = 2.542, p = 0.038) and NIHSS (OR = 1.236, p = 0.002) scores were independent risk factors for poor ISHH prognosis, while FA (OR = 0.908, p = 0.018) was a protective factor (Table 2).
3.2. Diversity and distribution of gut microbiota in three groups
As shown in Figure 1, the ACE (p > 0.05, Figure 1A) and Shannon (p > 0.05, Figure 1B) indices indicated that alpha diversity had no significant changes in the three groups. As for beta diversity, a comparative analysis of GM composition in various groups was visualized in the form of a PLS-DA diagram, which showed an obvious separation trend in three groups (Figure 1C). The Venn diagram exhibited 3,031 ASVs in the HH group, 4,271 ASVs in the ISHH group, and 2,261 ASVs in the NISHH group (Figure 1D). Meanwhile, 1,145 ASVs were shared in three groups, while the number of unique ASVs was 1,163 in the HH, 2184 in the ISHH, and 684 in the NISHH groups.
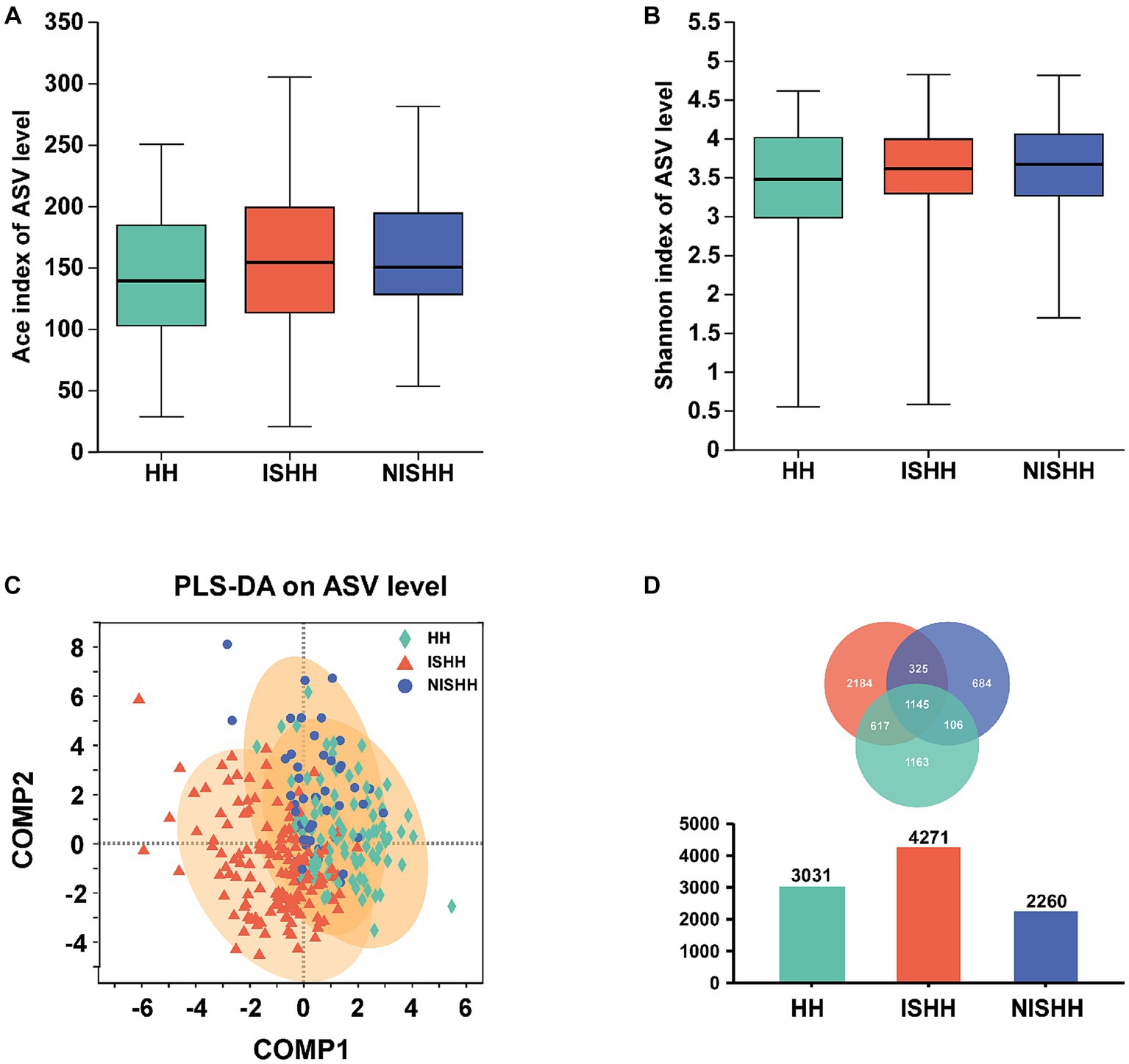
Figure 1. Analysis of gut microbiota among HH, ISHH, and NISHH. (A,B) Alpha-diversity analysis in three groups, comparing ACE and Shannon indices changes (Wilcoxon rank-sum test). (C) PLS-DA diagram on ASV level. (D) A Venn diagram reflected different species and overlaps on the ASV level.
The histogram of community composition in Figure 2 reflects the different microbial distributions. At the family level (Figure 2A), three groups were mainly composed of Lachnospiraceae, Enterobacteriaceae, Ruminococcaceae, Bacteroidaceae, Streptococcaceae, Lactobacillaceae, Bifidobacteriaceae, Prevotellaceae, Veillonellaceae, and Peptostreptococcaceae. However, compared with the NISHH group, the ISHH group was enriched in Bacteroidaceae, Lactobacillaceae, Bifidobacteriaceae, and Prevotellaceae, while the abundance of Enterobacteriaceae, Ruminococcaceae, and Streptococcaceae decreased. Meanwhile, compared with the HH group, the ISHH group showed that the abundance of Bacteroidaceae and Lactobacillaceae increased while Lachnospiraceae, Enterobacteriaceae, and Ruminococcaceae were observed to decrease. According to Figure 2B, at the genus level, Bacteroides (ISHH: 9.31%, NISHH:8.86%), Escherichia-Shigella (ISHH: 6.46%, NISHH: 5.60%), Lactobacillus (ISHH: 6.38%, NISHH: 4.78%), Bifidobacterium (ISHH: 3.94%, NISHH: 3.29%), and Prevotella (ISHH: 2.58%, NISHH: 1.87%) were observed to increase, while bacteria of genera Streptococcus (ISHH: 6.08%, NISHH: 7.77%), Faecalibacterium (ISHH: 4.28%, NISHH: 5.51%), and Klebsiella (ISHH: 2.92%, NISHH: 5.22%) were observed to decrease. At the same time, a comparison between the ISHH group and the HH group indicated that the ISHH group was enriched with Bacteroides (ISHH: 9.31%, HH: 8.40%), Lactobacillus (ISHH: 6.38%, HH: 4.87%), Bifidobacterium (ISHH: 3.94%, HH: 3.46%), and Klebsiella (ISHH: 2.92%, HH: 1.61%), while Escherichia-Shigella (ISHH: 6.46%, HH: 10.75%), Blautia (ISHH: 6.26%, HH: 7.31%), and Faecalibacterium (ISHH: 4.28%, HH: 6.22%) were less enriched than the HH group.
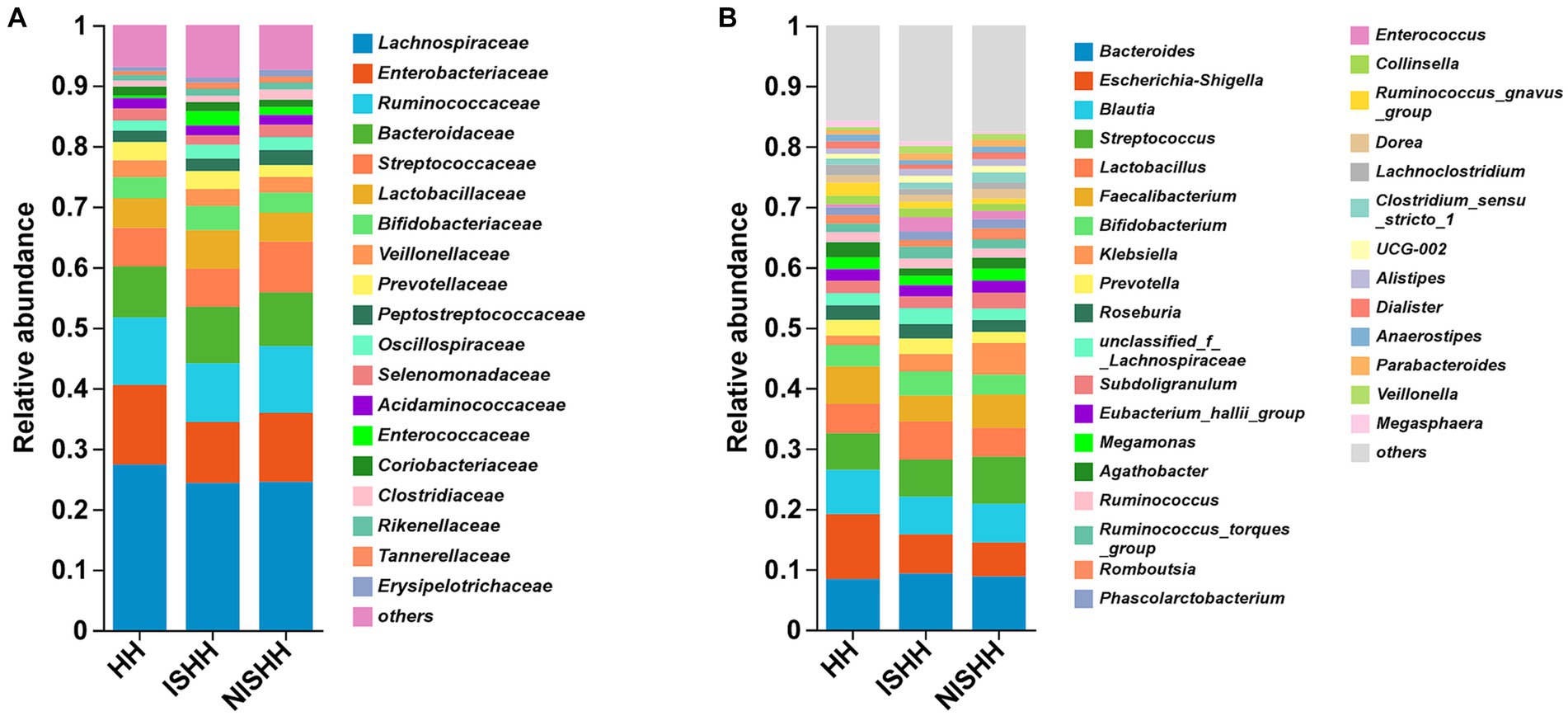
Figure 2. Distribution of gut microbiota among three groups. (A) Comparison of different taxa at the family level among three groups. (B) Comparison of different taxa at the genus level among three groups.
3.3. Characteristic microbiota between the ISHH group and the NISHH group
As shown in Figure 3A, the LEfSe cladogram revealed the most significant taxa, representing differences between the two groups from the phylum to genus level. As shown in Figure 3B, 18 taxa with LDA scores of >2 were selected as characteristic microbiota to distinguish between the two groups. Significant bacteria of the ISHH group mainly included Marinifilaceae, Butyricimonas, Odoribacter, and Coriobacteriaceae_UCG-002, while Romboutsia, Peptostreptococcales-Tissierellales, norank_f__Selenomonadaceae, Butyricicoccus, Butyricicoccaceae, Terrisporobacter, Micrococcaceae, Micrococcales, Rothia, Mailhella, F0332, Oscillospira, Scardovia, and Granulicatella were involved in the NISHH group. At the family level, the ISHH group exhibited lower relative abundances of Butyricicoccaceae (p < 0.05) and Micrococcaceae (p < 0.05) than the NISHH group, while the abundance of Marinifilaceae (p < 0.01) was increased (Figure 3C). At the genus level, compared with the NISHH group, in the ISHH group, the relative abundance of Romboutsia (p < 0.01), Butyricicoccus (p < 0.05), Terrisporobacter (p < 0.01), Lachnospiraceae_ND3007_group (p < 0.05), and Rothia (p < 0.05) was decreased (Figure 3D), while the relative abundance of Butyricimonas (p < 0.01) and Odoribacter (p < 0.05) was increased.
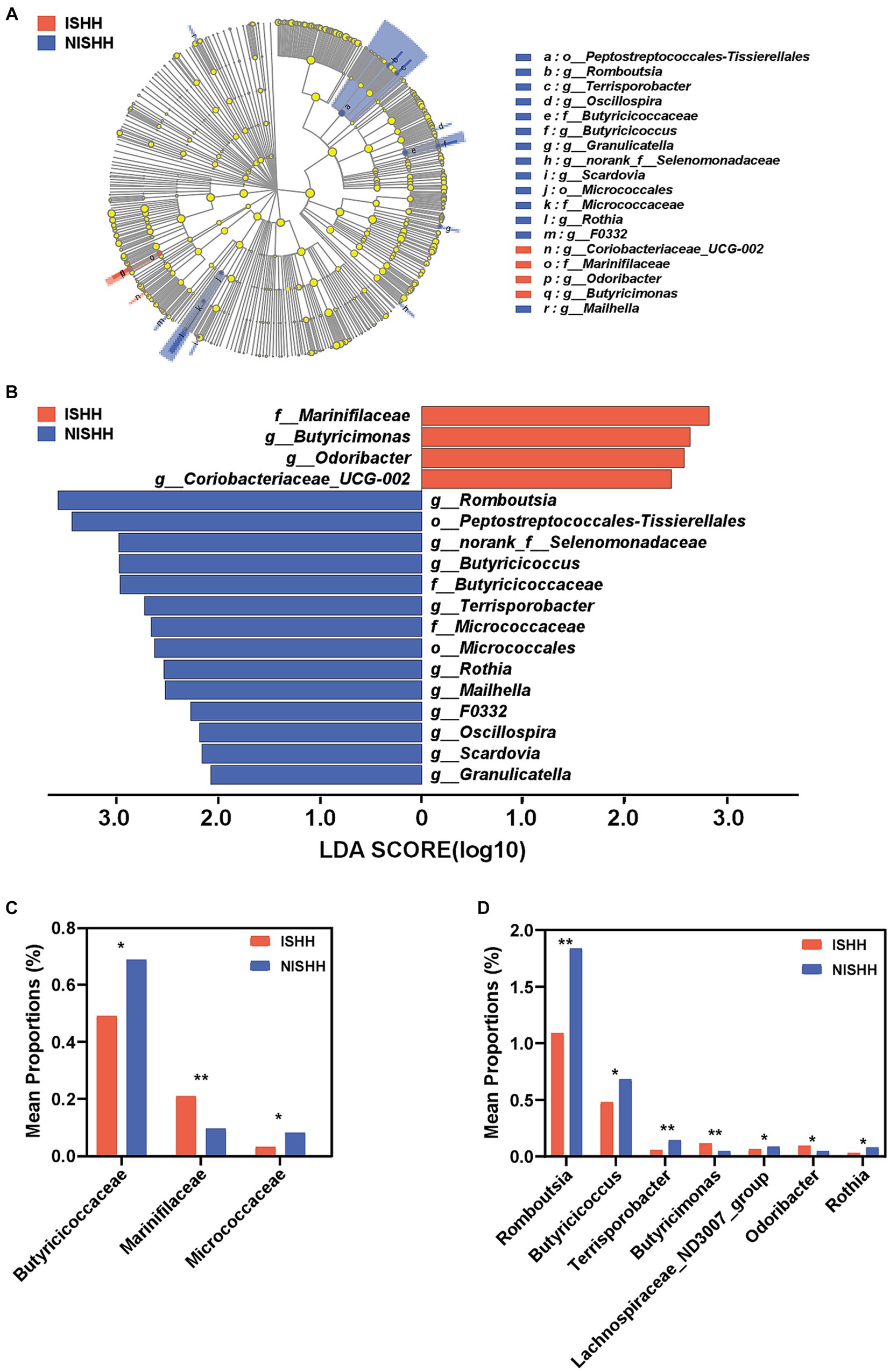
Figure 3. Taxonomic biomarkers of gut microbiota between ISHH and NISHH. (A) Cladogram showing the phylogenetic relationships of bacteria taxa. (B) LEfSe analysis revealed significant bacterial differences between the ISHH (red) and NISHH (blue) groups. The LDA scores (log10) > 2.0 and a p-value <0.05 were listed. (C) Histogram of bacterial differences at the family level. (D) Histogram of bacterial differences based at the genus level. *p < 0.05, compared with the NISHH group; Error bars indicate SD, **p < 0.01, compared with the NISHH group.
3.4. Characteristic microbiota between the ISHH and HH groups
As shown in Figure 4A, the LEfSe cladogram showed the significant taxa from the phylum to genus levels that could distinguish two groups. As shown in Figure 4B, the LDA bar chart exhibited 44 characteristic taxa (LDA > 2.0). As for differential bacteria at the family level, Figure 4C reflected that the relative abundance of Streptococcaceae (p < 0.05), Lactobacillaceae (p < 0.05), and Marinifilaceae (p < 0.01) were deceased in the HH group compared to the ISHH group. At the genus level (Figure 4D), the relative abundance of Lactobacillus (p < 0.05) and Veillonella (p < 0.05) was found to be decreased in the HH group. On the contrary, the relative abundance of Escherichia-Shigella (p < 0.05), Streptococcus (p < 0.05), Anaerostipes (p < 0.05), and Butyricicoccus (p < 0.05) were increased compared to the ISHH group.
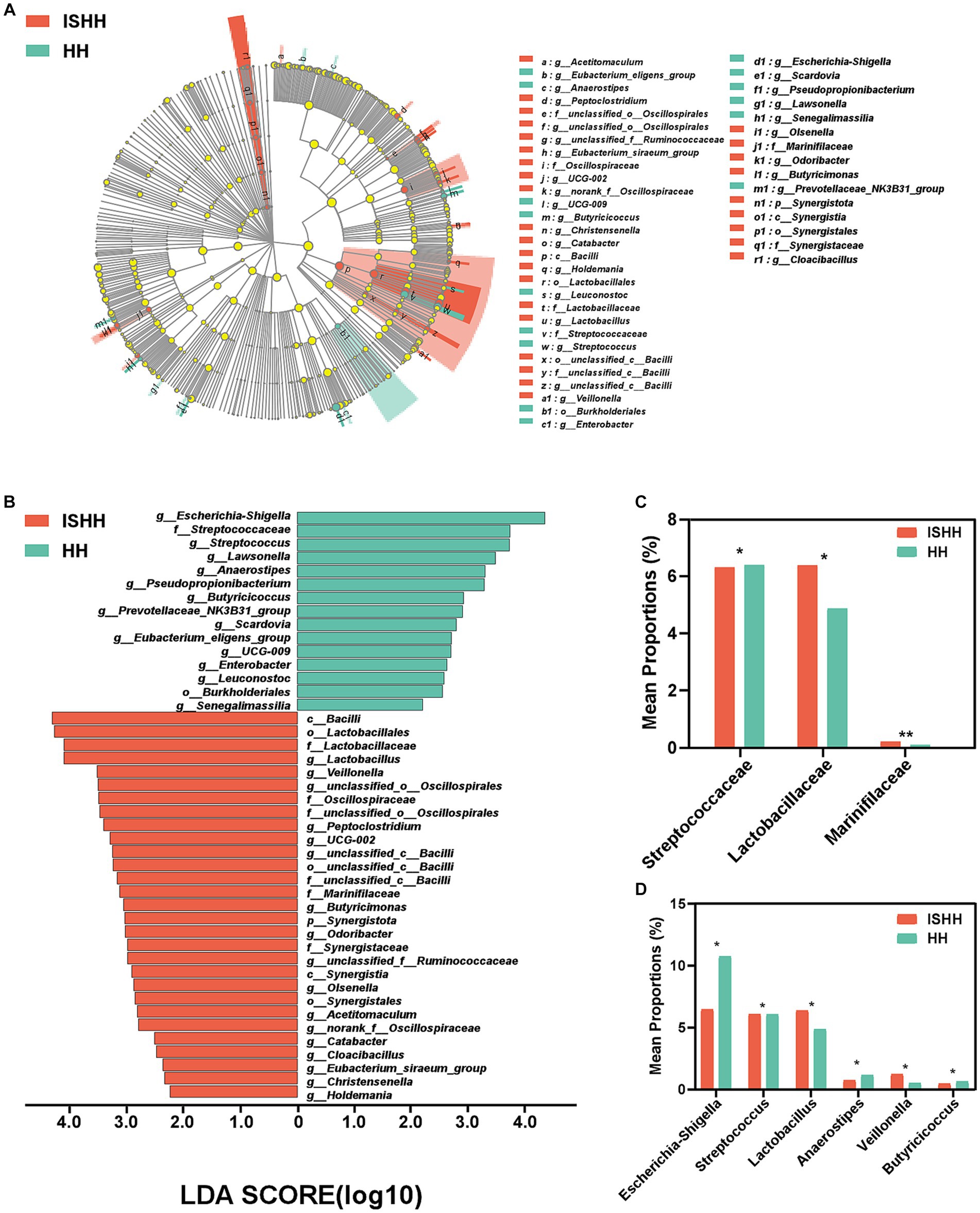
Figure 4. Taxonomic biomarkers of gut microbiota between ISHH and HH. (A,B) Cladogram representing the phylogenetic relationships of bacteria taxa and LDA scores between the ISHH and HH groups (LDA scores >2). (C,D) Histogram of bacterial differences at the family and the genus levels (Wilcoxon rank-sum test). Error bars indicate SD, *p < 0.05, **p < 0.01.
3.5. Correlation analysis between differential GM and clinical indicators
As shown in Figure 5, the heatmap indicated that the NIHSS score was negatively associated with Butyricicoccus (p < 0.01), Rothia (p < 0.01), and Family_XIII_UCG-001 (p < 0.05) and positively associated with Eubacterium_nodatum_group (p < 0.05). Simultaneously, the abundance of Butyricicoccus (p < 0.05) and Rothia (p < 0.01) was negatively associated with the mRS score. There was a negative effect of SBP on genera such as Coriobacteriaceae_UCG-002 (p < 0.05), Mailhella (p < 0.01), and F0332 (p < 0.01) and a positive effect of DBP on Butyricicoccusc (p < 0.05), as well as a negative effect of DBP on norank_f__Butyricicoccaceae (p < 0.05). MAP was negatively associated with the abundance of two genera (Mailhella and F0332, both p < 0.05), while Butyricimonas (p < 0.01) was positively associated with MAP.
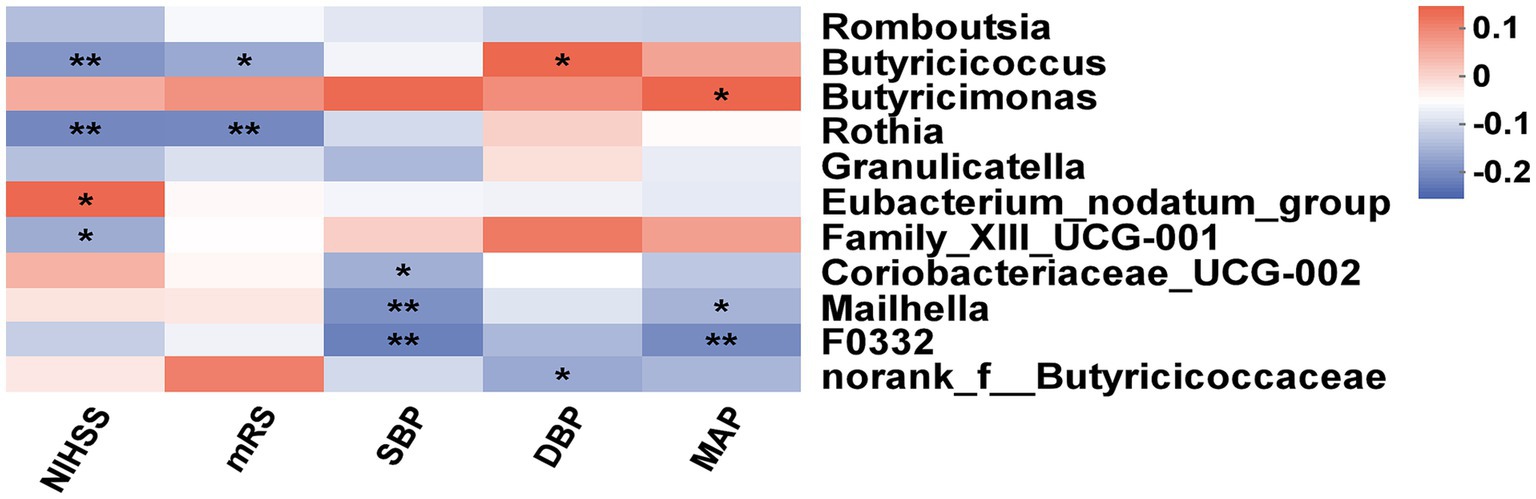
Figure 5. Correlation analysis of gut microbiota with clinical indexes. The heatmap between differential bacteria and clinical factors (NISHH score, mRS score, SBP, DBP, and MAP). The red color was positively correlated, and the blue color was negatively correlated. Deeper colors indicated higher correlation values. *p < 0.05, **p < 0.01.
3.6. Microbial biomarkers for the prognosis of ISHH patients
As shown in Figure 6, we screened out eight genera as microbial signatures. Butyricicoccus, Rothia, Family_XIII_UCG-001, and Eubacterium_nodatum_group were selected for their correlations with stroke prognostic indexes. In addition to the four genera closely related to the NIHSS and mRS scores, the remaining bacteria came from the top four abundances of different microbiota between the ISHH and NISHH groups, including Romboutsia, Terrisporobacter, Lachnospiraceae_ND3007_group, and Butyricimonas. The combination of eight bacteria achieved an AUC value of 0.631 (p < 0.01, 95% CI: 0.541–0.722) while adding clinically independent risk factors (NIHSS score and low FA) from multivariable logistic regression increased the AUC to 0.739 (P < 0.01, 95% CI: 0.659–0.820).
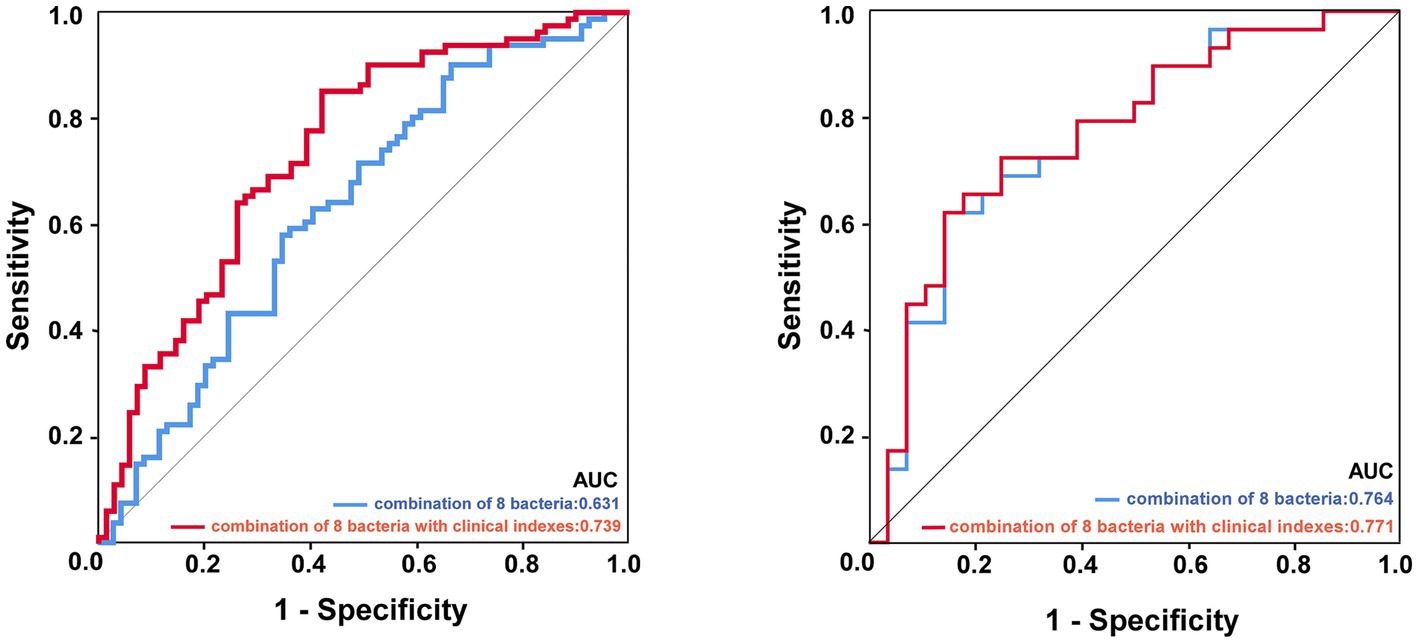
Figure 6. ROC curve analysis of potential microbial biomarkers distinguished the prognosis of ISHH. The image left: experimental cohort, the image right: validation cohort. The blue line represented the combination of eight characteristic bacteria, and the red line represented the combination of eight bacteria with two clinically independent risk factors.
4. Discussion
In this study, we revealed the characteristic alteration of GM in ISHH patients from occurrence to outcome. Then, we proposed that bacterial biomarkers, such as composition features and specific bacteria, might participate in the occurrence and development of the disease, further identifying the predictive value of characteristic microbiota.
In this study, an increase in NIHSS score, low FA, and the presence of HHTN were independent risk factors for poor outcomes after stroke onset. The NIHSS score was generally recognized as the tool to evaluate the severity of stroke (31), and a higher score normally indicated more serious neurological deficits (32), which are associated with worse outcomes of stroke (33). In this study, low FA was an independent risk factor for poor outcomes after stroke onset (Table 2), suggesting that supplementation with FA might be associated with a decreased risk of poor prognosis. A recent study showed that an insufficient intake of FA often leads to increased brain damage and a poor prognosis in stroke patients (34). Recent studies have shown that FA administration reduces the risk of stroke by 10–20% (35). FA has potential neuroprotective effects on cerebrovascular diseases (36). It was reported that HHTN participation in aggravating vascular diseases was regarded as a risk factor (11). Our results supported this finding, as the poorer outcome of stroke was performed in the ISHH group, which was specifically manifested by increased mRS scores, resulting in severe disability and a decline in life quality. Another recent report showed that the combination of hypertension and hyperhomocysteinemia played an important role in exacerbating Alzheimer’s disease (AD) and dementia, emphasizing the importance of understanding comorbid vascular risk factors (37). These findings suggested that HHTN, as the combination of hypertension and a high level of Hcy, could negatively affect the development of neurological diseases. However, an explicit mechanism to explain the association between HHTN and AIS remained unclear.
Recent studies have highlighted the influence of gut microbiota on the gut-brain axis and its potential role in stroke. A growing body of evidence suggests that altered microbiota are closely connected with AIS pathogenesis and clinical manifestations (38, 39). In this study, compared with the HH group, there was a significant difference in GM in the ISHH group, which was observed as the increase of proinflammatory bacteria such as Veillonella (40), as well as the decrease of genera that could produce SCFAs such as Butyricicoccus and Anaerostipe (41, 42). Furthermore, the comparison of the ISHH and NISHH groups revealed that decreases in taxa belonging to SCFA-producing bacteria (Butyricicoccus, Romboutsia, and Terrisporobacter) were associated with poor prognosis (43, 44), whereas increases in other bacteria (Butyricimonas and Odoribacter) were correlated with good outcomes. Interestingly, the decrease of Butyricicoccus was observed in the ISHH group compared with the HH and NISHH groups. Thus, we assumed that Butyricicoccus might take part in the occurrence and development of stroke in HHTN individuals. Butyricicoccus, one of the butyrate-producing genera belonging to Ruminococcaceae and Firmicutes (41), was generally considered a beneficial bacterium to human physiology (45, 46). The decrease of Butyricicoccus has been observed in various neurological disorders or mental diseases such as PD (47) and multiple sclerosis (48). Previously, we also identified the decrease of Butyricicoccus in stroke patients with hyperlipidemia and patients with PSD (24, 29), which reflected the correlations of Butyricicoccus with complications of stroke. Butyricicoccus was further identified as taking part in immune regulation, enabling it to upregulate expressions of anti-inflammatory factors and to promote neuronal recovery after stroke by activating microglia cells (49). Theo inflammation was responsible for the expansion of infarcts and post-stroke remodeling (50). It was reported that Butyricicoccus had the ability to regulate blood pressure in a hypertensive rat model characterized by microbiota dysbiosis and a dysfunctional autonomic nervous system (51). Our results supported this finding, as the relative abundance of Butyricicoccus was positively correlated with DBP (Figure 5). Butyrate, the metabolite of Butyricicoccus, might be a target for antihypertensive action by regulating the intrarenal renin-angiotensin system (52). In addition to Butyricicoccus, we also focused on other characteristic bacteria in the ISHH group. Rothia was negatively associated with both the mRS and NIHSS scores, indicating a correlation between decreased Rothia and a poor prognosis for the ISHH group. It was reported as an opportunistic pathogen associated with various infectious diseases in immunocompromised individuals (53). Recently, Chang et al. revealed that Rothia was enriched in the AIS group with good outcomes, indicating the beneficial function of Rothia in stroke (54), which was consistent with our result. Veillonella was a kind of proinflammatory taxa as well as Rothia and was more abundant in cachectic cancer patients (55). In this study, we assumed that increased Veillonella in ISHH patients might be a potential pathogen associated with stroke (Figure 4D). Furthermore, taxa decreased in ISHH patients, such as Romboutsia, Butyricicoccus, and Terrisporobacter, which belonged to the kind of bacteria that could produce SCFAs, which involved acetate, propionate, and butyrate (56). SCFAs were beneficial in modulating hypertension and AIS in animal models (57, 58). It was reported that bacteria producing SCFAs were significantly decreased in female rats with AIS, and these microbial dysbiosis could affect the signal profile to aggravate the stroke through the gut–brain axis (59). SCFAs, especially butyrate, play a vital role in inhibiting inflammation of brain cells or tissue (60). It was reported that butyrate could relieve symptoms of central nervous system diseases by promoting neuronal plasticity, enhancing memory, restoring cognitive damage, and reducing neurotoxicity and neuroinflammation (61–63). Chen et al. confirmed that supplementation with butyrate in model rats with AIS could effectively remodel the GM and intestinal permeability and improve the neurological deficits (64). Another study found that patients with hypertension had a lower abundance of genera that could produce SCFAs (57). SCFAs were also indicated to have the ability to alleviate hypertension, mitigate systemic inflammation, and decrease aortic atherosclerotic lesion area (65), which revealed the important association between SCFA-producing bacteria and vascular diseases (66). In this study, we revealed the alteration of SCFA-producing genera in ISHH, whose decreases were not only observed in the comparison with HH but also in the difference from NISHH. Therefore, decreases in SCFA-producing taxa were supposed to be involved in the pathogenesis of stroke. Gut dysfunction has emerged as a contributor to hypertension, the leading risk factor for stroke. The gut-brain axis has been shown to play a vital role in the prognosis of ISHH, which is associated with gut microbiota changes. In turn, the gut microbiota and its derived metabolites can influence stroke outcomes, and the relationship between gut microbiota, hypertension, and stroke outcomes may be a future research trend.
However, there were several limitations to this study. First, this study was conducted in a single center, so we could not observe the dynamic changes between participants and GM. Second, we still lacked information about microbial metabolites such as SCFAs, which restricted further research associated with GM and ISHH. Finally, the sample amounts for this study were limited. Thus, more patients should be involved in future research. Moreover, lifestyle information, such as lifestyle, exercise, and dietary habits, was not recorded. Despite the limitations of this study, we first described the characteristic GM in ISHH patients, which might be potential biomarkers for apoplectic prognosis.
In conclusion, this study revealed the characteristic bacteria of AIS patients with HHTN and established the model for prognosis based on microbial biomarkers such as Butyricicoccus, Romboutsia, and Terrisporobacter. These findings suggested that key bacteria might participate in the process of ISHH and could act as novel diagnostic biomarkers for prognosis, which might help predict the outcome of AIS patients with HHTN and facilitate the early warning of stroke in HHTN individuals.
Data availability statement
The datasets presented in this study can be found in online repositories. The names of the repository/repositories and accession number(s) can be found at: https://www.ncbi.nlm.nih.gov/, PRJNA1004022.
Ethics statement
The studies involving humans were approved by Ethics Committee of the Second Affiliated Hospital of Wenzhou Medical University. The studies were conducted in accordance with the local legislation and institutional requirements. The participants provided their written informed consent to participate in this study.
Author contributions
SY: Writing – original draft. JC: Data curation, Investigation, Writing – review & editing. YZ: Data curation, Investigation, Writing – review & editing. XL: Software, Writing – review & editing. QC: Investigation, Methodology, Project administration, Writing – review & editing. HX: Formal analysis, Methodology, Writing – review & editing. JL: Investigation, Project administration, Writing – review & editing. JS: Project administration, Writing – review & editing. SZ: Writing – review & editing.
Funding
The author(s) declare financial support was received for the research, authorship, and/or publication of this article. This work was supported by the Clinical Medical Research Project of the Zhejiang Medical Association (2022ZYC-D10).
Conflict of interest
The authors declare that the research was conducted in the absence of any commercial or financial relationships that could be construed as a potential conflict of interest.
Publisher’s note
All claims expressed in this article are solely those of the authors and do not necessarily represent those of their affiliated organizations, or those of the publisher, the editors and the reviewers. Any product that may be evaluated in this article, or claim that may be made by its manufacturer, is not guaranteed or endorsed by the publisher.
References
2. Renú, A , Millán, M , San Román, L , Blasco, J , Martí-Fàbregas, J , Terceño, M, et al. Effect of intra-arterial alteplase vs placebo following successful thrombectomy on functional outcomes in patients with large vessel occlusion acute ischemic stroke: the CHOICE randomized clinical trial. JAMA. (2022) 327:826–35. doi: 10.1001/jama.2022.1645
3. Wang, YJ , Li, ZX , Gu, HQ , Zhai, Y , Zhou, Q , Jiang, Y, et al. China stroke statistics: an update on the 2019 report from the national center for healthcare quality management in neurological diseases, China national clinical research center for neurological diseases, the Chinese stroke association, national center for chronic and non-communicable disease control and prevention, Chinese center for disease control and prevention and institute for global neuroscience and stroke collaborations. Stroke Vasc Neurol. (2022) 7:415–50. doi: 10.1136/svn-2021-001374
4. Ostrakhovitch, EA , and Tabibzadeh, S . Homocysteine and age-associated disorders. Ageing Res Rev. (2019) 49:144–64. doi: 10.1016/j.arr.2018.10.010
5. Zhao, M , Wang, X , He, M , Qin, X , Tang, G , Huo, Y, et al. Homocysteine and stroke risk: modifying effect of methylenetetrahydrofolate reductase C677T polymorphism and folic acid intervention. Stroke. (2017) 48:1183–90. doi: 10.1161/strokeaha.116.015324
6. Kong, Y , Li, X , Chang, L , Liu, Y , Jia, L , Gao, L, et al. Hypertension with high homocysteine is associated with default network gray matter loss. Front Neurol. (2021) 12:740819. doi: 10.3389/fneur.2021.740819
7. Du, S , Hong, X , Yang, Y , Ding, Z , and Yu, T . Association between body fat percentage and H-type hypertension in postmenopausal women. Front Public Health. (2022) 10:950805. doi: 10.3389/fpubh.2022.950805
8. Tan, Y , Nie, F , Wu, G , Guo, F , Wang, Y , and Wang, L . Impact of H-type hypertension on intraplaque neovascularization assessed by contrast-enhanced ultrasound. J Atheroscler Thromb. (2022) 29:492–501. doi: 10.5551/jat.61275
9. Zhang, ZY , Gu, X , Tang, Z , Guan, SC , Liu, HJ , Wu, XG, et al. Homocysteine, hypertension, and risks of cardiovascular events and all-cause death in the Chinese elderly population: a prospective study. J Geriatr Cardiol. (2021) 18:796–808. doi: 10.11909/j.issn.1671-5411.2021.10.005
10. Zhao, W , Gao, F , Lv, L , and Chen, X . The interaction of hypertension and homocysteine increases the risk of mortality among middle-aged and older population in the United States. J Hypertens. (2022) 40:254–63. doi: 10.1097/hjh.0000000000003002
11. Li, J , Jiang, S , Zhang, Y , Tang, G , Wang, Y , Mao, G, et al. H-type hypertension and risk of stroke in Chinese adults: a prospective, nested case-control study. J Transl Int Med. (2015) 3:171–8. doi: 10.1515/jtim-2015-0027
12. Wang, J , Xi, YX , Li, JQ , and Zhu, WW . Gender difference in association between H-type hypertension and subcortical ischemic vascular disease. Front Aging Neurosci. (2022) 14:998268. doi: 10.3389/fnagi.2022.998268
13. Bosetti, F , Koenig, JI , Ayata, C , Back, SA , Becker, K , Broderick, JP, et al. Translational stroke research: vision and opportunities. Stroke. (2017) 48:2632–7. doi: 10.1161/strokeaha.117.017112
15. Zinno, P , Motta, V , Guantario, B , Natella, F , Roselli, M , Bello, C, et al. Supplementation with dairy matrices impacts on homocysteine levels and gut microbiota composition of hyperhomocysteinemic mice. Eur J Nutr. (2020) 59:345–58. doi: 10.1007/s00394-019-01911-y
16. Fan, Y , and Pedersen, O . Gut microbiota in human metabolic health and disease. Nat Rev Microbiol. (2021) 19:55–71. doi: 10.1038/s41579-020-0433-9
17. Park, EM , Chelvanambi, M , Bhutiani, N , Kroemer, G , Zitvogel, L , and Wargo, JA . Targeting the gut and tumor microbiota in cancer. Nat Med. (2022) 28:690–703. doi: 10.1038/s41591-022-01779-2
18. Li, J , Zhao, F , Wang, Y , Chen, J , Tao, J , Tian, G, et al. Gut microbiota dysbiosis contributes to the development of hypertension. Microbiome. (2017) 5:14. doi: 10.1186/s40168-016-0222-x
19. Marques, FZ , Nelson, E , Chu, PY , Horlock, D , Fiedler, A , Ziemann, M, et al. High-fiber diet and acetate supplementation change the gut microbiota and prevent the development of hypertension and heart failure in hypertensive mice. Circulation. (2017) 135:964–77. doi: 10.1161/circulationaha.116.024545
20. Rosario, D , Bidkhori, G , Lee, S , Bedarf, J , Hildebrand, F , Le Chatelier, E, et al. Systematic analysis of gut microbiome reveals the role of bacterial folate and homocysteine metabolism in Parkinson's disease. Cell Rep. (2021) 34:108807. doi: 10.1016/j.celrep.2021.108807
21. Vascellari, S , Palmas, V , Melis, M , Pisanu, S , Cusano, R , Uva, P, et al. Gut microbiota and metabolome alterations associated with Parkinson’s disease. mSystems. (2020) 5:e00561-30. doi: 10.1128/mSystems.00561-20
22. Morais, LH , Schreiber, HL IV, and Mazmanian, SK . The gut microbiota-brain axis in behaviour and brain disorders. Nat Rev Microbiol. (2021) 19:241–55. doi: 10.1038/s41579-020-00460-0
23. Benakis, C , Brea, D , Caballero, S , Faraco, G , Moore, J , Murphy, M, et al. Commensal microbiota affects ischemic stroke outcome by regulating intestinal γδ t cells. Nat Med. (2016) 22:516–23. doi: 10.1038/nm.4068
24. Yao, S , Xie, H , Wang, Y , Shen, N , Chen, Q , Zhao, Y, et al. Predictive microbial feature analysis in patients with depression after acute ischemic stroke. Front Aging Neurosci. (2023) 15:1116065. doi: 10.3389/fnagi.2023.1116065
25. Ling, Y , Gong, T , Zhang, J , Gu, Q , Gao, X , Weng, X, et al. Gut microbiome signatures are biomarkers for cognitive impairment in patients with ischemic stroke. Front Aging Neurosci. (2020) 12:511562. doi: 10.3389/fnagi.2020.511562
26. Ling, Y , Gu, Q , Zhang, J , Gong, T , Weng, X , Liu, J, et al. Structural change of gut microbiota in patients with post-stroke comorbid cognitive impairment and depression and its correlation with clinical features. J Alzheimers Dis. (2020) 77:1595–608. doi: 10.3233/JAD-200315
27. Wang, J , Zhong, Y , Zhu, H , Mahgoub, OK , Jian, Z , Gu, L, et al. Different gender-derived gut microbiota influence stroke outcomes by mitigating inflammation. J Neuroinflammation. (2022) 19:245. doi: 10.1186/s12974-022-02606-8
28. Schiattarella, GG , Sannino, A , Toscano, E , Giugliano, G , Gargiulo, G , Franzone, A, et al. Gut microbe-generated metabolite trimethylamine-N-oxide as cardiovascular risk biomarker: a systematic review and dose-response meta-analysis. Eur Heart J. (2017) 38:2948–56. doi: 10.1093/eurheartj/ehx342
29. Chen, J , Chi, B , Ma, J , Zhang, J , Gu, Q , Xie, H, et al. Gut microbiota signature as predictors of adverse outcomes after acute ischemic stroke in patients with hyperlipidemia. Front Cell Infect Microbiol. (2022) 12:1073113. doi: 10.3389/fcimb.2022.1073113
30. Sacco, RL , Kasner, SE , Broderick, JP , Caplan, LR , Connors, JJ , Culebras, A, et al. An updated definition of stroke for the 21st century: a statement for healthcare professionals from the American Heart Association/American Stroke Association. Stroke. (2013) 44:2064–89. doi: 10.1161/STR.0b013e318296aeca
31. Koton, S , Pike, JR , Johansen, M , Knopman, DS , Lakshminarayan, K , Mosley, T, et al. Association of ischemic stroke incidence, severity, and recurrence with dementia in the atherosclerosis risk in communities cohort study. JAMA Neurol. (2022) 79:271–80. doi: 10.1001/jamaneurol.2021.5080
32. Rajashekar, D , Wilms, M , MacDonald, ME , Schimert, S , Hill, MD , Demchuk, A, et al. Lesion-symptom mapping with NIHSS sub-scores in ischemic stroke patients. Stroke Vasc Neurol. (2022) 7:124–31. doi: 10.1136/svn-2021-001091
33. Yoshimura, S , Sakai, N , Yamagami, H , Uchida, K , Beppu, M , Toyoda, K, et al. Endovascular therapy for acute stroke with a large ischemic region. N Engl J Med. (2022) 386:1303–13. doi: 10.1056/NEJMoa2118191
34. Dong, Z , Liang, X , Zhang, Q , Luo, S , Liu, H , Wang, X, et al. Folic acid deficiency enhances the Tyr705 and Ser727 phosphorylation of mitochondrial STAT3 in in vivo and in vitro models of ischemic stroke. Transl Stroke Res. (2021) 12:829–43. doi: 10.1007/s12975-020-00860-7
35. Głuszek, J , Wierzowiecka, M , Niklas, K , and Niklas, A . The importance of homocysteine in the development of cardiovascular complications in patients with rheumatoid arthritis. Reumatologia. (2020) 58:282–8. doi: 10.5114/reum.2020.99732
36. Marchesi, N , Linciano, P , Campagnoli, LIM , Fahmideh, F , Rossi, D , Costa, G, et al. Short- and long-term regulation of HuD: a molecular switch mediated by folic acid? Int J Mol Sci. (2023) 24:12201. doi: 10.3390/ijms241512201
37. Carey, A , and Fossati, S . Hypertension and hyperhomocysteinemia as modifiable risk factors for Alzheimer’s disease and dementia: new evidence, potential therapeutic strategies, and biomarkers. Alzheimers Dement. (2023) 19:671–95. doi: 10.1002/alz.12871
38. Peh, A , O'Donnell, JA , Broughton, BRS , and Marques, FZ . Gut microbiota and their metabolites in stroke: a double-edged sword. Stroke. (2022) 53:1788–801. doi: 10.1161/strokeaha.121.036800
39. Koszewicz, M , Jaroch, J , Brzecka, A , Ejma, M , Budrewicz, S , Mikhaleva, LM, et al. Dysbiosis is one of the risk factor for stroke and cognitive impairment and potential target for treatment. Pharmacol Res. (2021) 164:105277. doi: 10.1016/j.phrs.2020.105277
40. Rovery, C , Etienne, A , Foucault, C , Berger, P , and Brouqui, P . Veillonella montpellierensis endocarditis. Emerg Infect Dis. (2005) 11:1112–4. doi: 10.3201/eid1107.041361
41. Devriese, S , Eeckhaut, V , Geirnaert, A , Van den Bossche, L , Hindryckx, P , Van de Wiele, T, et al. Reduced mucosa-associated butyricicoccus activity in patients with ulcerative colitis correlates with aberrant claudin-1 expression. J Crohns Colitis. (2017) 11:229–36. doi: 10.1093/ecco-jcc/jjw142
42. Endo, A , Tanno, H , Kadowaki, R , Fujii, T , and Tochio, T . Extracellular fructooligosaccharide degradation in Anaerostipes hadrus for co-metabolism with non-fructooligosaccharide utilizers. Biochem Biophys Res Commun. (2022) 613:81–6. doi: 10.1016/j.bbrc.2022.04.134
43. Niu, J , Liu, X , Xu, J , Li, F , Wang, J , Zhang, X, et al. Effects of silage diet on meat quality through shaping gut microbiota in finishing pigs. Microbiol. Spectr. (2023) 11:e0241622. doi: 10.1128/spectrum.02416-22
44. Ye, X , Liu, Y , Hu, J , Gao, Y , Ma, Y , and Wen, D . Chlorogenic acid-induced gut microbiota improves metabolic endotoxemia. Front. Endocrinol. (2021) 12:762691. doi: 10.3389/fendo.2021.762691
45. Rodriguez, J , Hiel, S , Neyrinck, AM , Le Roy, T , Pötgens, SA , Leyrolle, Q, et al. Discovery of the gut microbial signature driving the efficacy of prebiotic intervention in obese patients. Gut. (2020) 69:1975–87. doi: 10.1136/gutjnl-2019-319726
46. Boesmans, L , Valles-Colomer, M , Wang, J , Eeckhaut, V , Falony, G , Ducatelle, R, et al. Butyrate producers as potential next-generation probiotics: safety assessment of the administration of butyricicoccus pullicaecorum to healthy volunteers. mSystems. (2018) 3:e00094-18. doi: 10.1128/mSystems.00094-18
47. Lubomski, M , Xu, X , Holmes, AJ , Yang, JYH , Sue, CM , and Davis, RL . The impact of device-assisted therapies on the gut microbiome in Parkinson's disease. J Neurol. (2022) 269:780–95. doi: 10.1007/s00415-021-10657-9
48. Bonnechère, B , Amin, N , and van Duijn, C . What are the key gut microbiota involved in neurological diseases? A systematic review. Int J Mol Sci. (2022) 23:13665. doi: 10.3390/ijms232213665
49. Eeckhaut, V , Machiels, K , Perrier, C , Romero, C , Maes, S , Flahou, B, et al. Butyricicoccus pullicaecorum in inflammatory bowel disease. Gut. (2013) 62:1745–52. doi: 10.1136/gutjnl-2012-303611
50. Lambertsen, KL , Finsen, B , and Clausen, BH . Post-stroke inflammation-target or tool for therapy? Acta Neuropathol. (2019) 137:693–714. doi: 10.1007/s00401-018-1930-z
51. Yang, T , Magee, KL , Colon-Perez, LM , Larkin, R , Liao, YS , Balazic, E, et al. Impaired butyrate absorption in the proximal colon, low serum butyrate and diminished central effects of butyrate on blood pressure in spontaneously hypertensive rats. Acta Physiol. (2019) 226:e13256. doi: 10.1111/apha.13256
52. Verhaar, BJH , Collard, D , Prodan, A , Levels, JHM , Zwinderman, AH , Bäckhed, F, et al. Associations between gut microbiota, faecal short-chain fatty acids, and blood pressure across ethnic groups: the HELIUS study. Eur Heart J. (2020) 41:4259–67. doi: 10.1093/eurheartj/ehaa704
53. Fatahi-Bafghi, M . Characterization of the Rothia spp. and their role in human clinical infections. Infect Genet Evol. (2021) 93:104877. doi: 10.1016/j.meegid.2021.104877
54. Chang, Y , Woo, HG , Jeong, JH , Kim, GH , Park, KD , and Song, TJ . Microbiota dysbiosis and functional outcome in acute ischemic stroke patients. Sci Rep. (2021) 11:10977. doi: 10.1038/s41598-021-90463-5
55. Ubachs, J , Ziemons, J , Soons, Z , Aarnoutse, R , van Dijk, DPJ , Penders, J, et al. Gut microbiota and short-chain fatty acid alterations in cachectic cancer patients. J Cachexia Sarcopenia Muscle. (2021) 12:2007–21. doi: 10.1002/jcsm.12804
56. Martin-Gallausiaux, C , Marinelli, L , Blottière, HM , Larraufie, P , and Lapaque, N . SCFA: mechanisms and functional importance in the gut. Proc Nutr Soc. (2021) 80:37–49. doi: 10.1017/s0029665120006916
57. Honarpisheh, P , Bryan, RM , and McCullough, LD . Aging microbiota-gut-brain axis in stroke risk and outcome. Circ Res. (2022) 130:1112–44. doi: 10.1161/circresaha.122.319983
58. Fang, Z , Chen, M , Qian, J , Wang, C , and Zhang, J . The bridge between ischemic stroke and gut microbes: short-chain fatty acids. Cell Mol Neurobiol. (2023) 43:543–59. doi: 10.1007/s10571-022-01209-4
59. Park, MJ , Pilla, R , Panta, A , Pandey, S , Sarawichitr, B , Suchodolski, J, et al. Reproductive senescence and ischemic stroke remodel the gut microbiome and modulate the effects of estrogen treatment in female rats. Transl Stroke Res. (2020) 11:812–30. doi: 10.1007/s12975-019-00760-5
60. Li, G , Lin, J , Zhang, C , Gao, H , Lu, H , Gao, X, et al. Microbiota metabolite butyrate constrains neutrophil functions and ameliorates mucosal inflammation in inflammatory bowel disease. Gut Microbes. (2021) 13:1968257. doi: 10.1080/19490976.2021.1968257
61. Zhang, L , Liu, C , Jiang, Q , and Yin, Y . Butyrate in energy metabolism: there is still more to learn. Trends Endocrinol Metab. (2021) 32:159–69. doi: 10.1016/j.tem.2020.12.003
62. He, J , Chu, Y , Li, J , Meng, Q , Liu, Y , Jin, J, et al. Intestinal butyrate-metabolizing species contribute to autoantibody production and bone erosion in rheumatoid arthritis. Sci Adv. (2022) 8:eabm1511. doi: 10.1126/sciadv.abm1511
63. Park, SY , Rao, C , Coyte, KZ , Kuziel, GA , Zhang, Y , Huang, W, et al. Strain-level fitness in the gut microbiome is an emergent property of glycans and a single metabolite. Cells. (2022) 185:513–29.e21. doi: 10.1016/j.cell.2022.01.002
64. Chen, R , Xu, Y , Wu, P , Zhou, H , Lasanajak, Y , Fang, Y, et al. Transplantation of fecal microbiota rich in short chain fatty acids and butyric acid treat cerebral ischemic stroke by regulating gut microbiota. Pharmacol Res. (2019) 148:104403. doi: 10.1016/j.phrs.2019.104403
65. Bartolomaeus, H , Balogh, A , Yakoub, M , Homann, S , Markó, L , Höges, S, et al. Short-chain fatty acid propionate protects from hypertensive cardiovascular damage. Circulation. (2019) 139:1407–21. doi: 10.1161/circulationaha.118.036652
Keywords: H-type hypertension, acute ischemic stroke, gut microbiota, biomarker, 16S rRNA
Citation: Yu S, Chen J, Zhao Y, Liao X, Chen Q, Xie H, Liu J, Sun J and Zhi S (2023) Association analysis of the gut microbiota in predicting outcomes for patients with acute ischemic stroke and H-type hypertension. Front. Neurol. 14:1275460. doi: 10.3389/fneur.2023.1275460
Edited by:
Pedro Ramos-Cabrer, CIC biomaGUNE, SpainReviewed by:
Nicoletta Marchesi, University of Pavia, ItalyJun-Jie Zhang, Indiana University Bloomington, United States
Copyright © 2023 Yu, Chen, Zhao, Liao, Chen, Xie, Liu, Sun and Zhi. This is an open-access article distributed under the terms of the Creative Commons Attribution License (CC BY). The use, distribution or reproduction in other forums is permitted, provided the original author(s) and the copyright owner(s) are credited and that the original publication in this journal is cited, in accordance with accepted academic practice. No use, distribution or reproduction is permitted which does not comply with these terms.
*Correspondence: Jing Sun, c3Vuand6QDEyNi5jb20=; Shaoce Zhi, emhpc2hhb2NlQHNpbmEuY29t
†These authors have contributed equally to this work