- 1Department of Hematology, Shenzhen People’s Hospital (The Second Clinical Medical College, Jinan University, The First Affiliated Hospital, Southern University of Science and Technology), Shenzhen, China
- 2Department of Pathology, Shenzhen People’s Hospital (The Second Clinical Medical College, Jinan University, The First Affiliated Hospital, Southern University of Science and Technology), Shenzhen, China
- 3Medical Center, Geneplus-Beijing, Beijing, China
- 4Geneplus-Beijing Institute, Beijing, China
- 5Department of Oncology, Xiangya Hospital, Central South University, Changsha, China
- 6Sun Yat-sen University Cancer Center, State Key Laboratory of Oncology in South China, Collaborative Innovation Center for Cancer Medicine, Guangzhou, China
- 7Department of Pathology, Sun Yat-sen University Cancer Center, Guangzhou, China
Primary diffuse large B cell lymphoma of the central nervous system (CNS DLBCL) is a rare malignancy with a distinct genetic profile. The clinicopathological significance of the mutation patterns remains unknown. Forty cases of primary CNS DLBCL were subjected to targeted exome sequencing covering 413 genes, including MYD88, CD79B and PIM1. Mutational analysis recognized two groups. The CDP (including CD79B and/or PIM1mutations) group was identified in 27 cases (67.5%), and the non-CDP (without CD79B and PIM1 mutations) group was identified in 13 cases 32.5%). The CDP group tended to occur in older patients (median age 57.0 vs. 48.4 years, p=0.015). Patients in the CDP group had a significantly longer 2-year overall survival (OS) (76% and 40%, p=0.0372) than those in the non-CDP group. Multivariate analysis revealed that age less than 60 years, no MYC and BCL2 double expression, and CDP group were three independent risk factors indicating favorable OS. PyClone analysis revealed the subcloning heterogeneity between the groups. In addition, transcriptional sequencing was successfully performed in 8 cases. A total of 131 genes were significantly differentially expressed between these two groups. The major categories of biological processes that were significantly altered between these two groups related to intracellular metabolism mechanisms. We developed a new molecular classification to divide CNS DLBCL into CDP and non-CDP groups based on CD79B and PIM1 mutational status. Patients with PIM1 and/or CD79B mutations had favorable long-term survival after high-dose methotrexate-based polychemotherapy.
Introduction
Primary central nervous system (CNS) diffuse large B-cell lymphoma (DLBCL) is a rare malignancy that only accounts for <1% of all non-Hodgkin lymphomas (NHL) and 2.4–3% of all brain tumors. In recent decades, an increased incidence of CNS DLBCL has been reported among patients aged >60 years (1). Currently, the treatment of CNS DLBCL is primarily based on high-dose methotrexate (HD-MTX), followed by whole-brain radiation (WBR) and/or autologous hematopoietic stem cell transplantation (ASCT) (2). Patients with CNS DLBCL have a remarkably worse outcome than patients with systemic DLBCL.
The extreme genetic and phenotypic heterogeneity of DLBCL presents a great challenge to the development of precision therapies. Since 2000, gene expression profiling has first subclassified DLBCL into the geminal-center B cell type (GCB), activated B cell type (ABC) and unclassified type (UC). This “cell-of-origin” (COO) methodology showed great prognostic significance and dramatically changed our clinical practice (3). However, the majority of primary CNS DLBCL belongs to the non-GCB subtype, which limits the use of COO methodology by immunohistochemistry of systemic DLBCL to CNS DLBCL to predict patient outcome.
With the development of next-generation sequencing (NGS), the molecular classification of DLBCL has developed from expression profiling to genetic profiling. Genetic subtypes of DLBCL show enormous potential in predicting prognosis and guiding precision therapy (4–6). Systemic DLBCL can be subdivided into seven genetic subtypes, including MCD (including MYD88L265P and CD79B mutations), N1 (including NOTCH1 mutations), A53 (characterized by TP53 mutations and deletions), BN2 (including BCL6 translocations and NOTCH2 mutations), ST2 (with recurrent SGK1 and TET2 mutations), EZB Myc+ and EZB Myc- (4). CNS DLBCLs frequently harbored MYD88, CD79B and/or PIM1 mutations. According to the classification, approximately 37% of CNS DLBCL cases fall into the so-called MCD subtype (7, 8). Unfortunately, the molecular classification of systemic DLBCL is not applicable to CNS DLBCL to predict patient outcome. Therefore, a new molecular classification is needed to elucidate the relationship between the mutation patterns and clinicopathological features, as well as the patients’ outcomes.
In present study, we attempted to classify primary CNS DLBCLs into two biologically relevant subgroups based on the mutational status of CD79B and PIM1 by targeted exome sequencing covering 413 genes. Patients harboring CD79B and/or PIM1 mutations belonged to the so-called CDP group, while those without CD79B or PIMI mutations belonged to the so-called non-CDP group. We analyzed the clinical characteristics and prognosis between the two molecular subgroups. In addition, we performed transcriptional sequencing to find out significantly differentially expressed genes between these two molecular subgroups.
Materials and Methods
Patients and Clinicopathological Data
A retrospective cohort of forty patients with primary CNS DLBCL at Shenzhen People’s Hospital and Sun Yat-sen University Cancer Center from January 2016 to October 2019 was enrolled. The exclusion criteria were lymphomas of the dura, intravascular large B-cell lymphoma, lymphomas with evidence of systemic disease or secondary lymphoma, and all immunodeficiency-associated lymphomas. All patients were of Chinese origin and received HD-MTX-based polychemotherapy. Most patients received rituximab treatment at the same time. This study was approved by the ethical committee at Shenzhen People’s Hospital (Reference number: LL-KY-2020199). All patients provided written informed consent for the collection and publication of their medical information. The authenticity of this article has been validated by uploading the key raw data onto the Research Data Deposit public platform (www.researchdata.org.cn), with the approval RDD number as RDDA2021002020. The clinical characteristics of all patients in the present cohort are summarized in Table 1.
Immunohistochemical (IHC) Staining and EBV-Encoded RNA (EBER) In Situ Hybridization (ISH)
The specimens of these forty cases were formalin fixed and paraffin embedded (FFPE) and then sectioned at 4.0 mm thickness. The sections were stained using hematoxylin and eosin staining and were used for IHC and ISH examination. IHC was performed to analyze the expression of CD20, CD3, CD10, BCL6, MUM1, MYC, BCL2 and Ki67 in all cases using a BenchMark ULTRA automatic immunostaining device according to the manufacturer’s instructions. The EBV Probe In Situ Hybridization Kit (ISH-6021, Zhongshan Golden Bridge Biotechnology Co. Ltd., Beijing, China) was used to detect EBERs according to the manufacturer’s protocol. The Hans algorithm was used to classify the COO subtypes of CNS DLBCL
Targeted Exome Next-Generation Sequencing
The FFPE tissue samples isolated from forty CNS DLBCL patients were sequenced using commercial DNA sequencing services (GenePlus Co. Beijing, China). Genomic DNA was isolated from FFPE tumor samples using the QIAamp DNA FFPE Tissue kit (Qiagen GmbH, Hilden, Germany). For library preparation, tumor DNA was sheared into 200–250-bp fragments using a Covaris S2 instrument (Woburn, MA, USA), and indexed NGS libraries were prepared using the DNA Library Preparation Kit for MGISeq-2000 (BGI, Shenzhen, China). All libraries were hybridized to custom-designed biotinylated oligonucleotide probes (IDT, Coralville, IA, USA) covering 413 genes (Table S1). DNA sequencing was performed using the MGISeq-2000 Sequencing System (BGI, Shenzhen, China) per the manufacturer’s guidelines, which generated 3 Gb of data from tumor DNA. Additional detailed information regarding target region captures, NGS and somatic mutation calling of tumor DNA were as previously described (9, 10). The raw sequence data reported in this paper have been deposited in the Genome Sequence Archive of the BIG Data Center at the Beijing Institute of Genomics, Chinese Academy of Science, under the accession number HRA001659 (http://bigd.big.ac.cn/gsa-human). Code is available from corresponding author on reasonable request.
PyClone Analysis
PyClone was used to analyze the clonal population structure of tumor samples from each patient (11). The copy number information of each single nucleotide variation (SNV) was used as input. Variants located in the cluster with the greatest mean cancer cell fraction (CCF) were defined as clonal, and the rest were subclonal.
RNA Sequencing and Differential Gene Expression Analysis
The techniques of performing transcriptional sequencing on FFPE tumor tissue samples were used following the “K. TotalRNA” protocol (12). In brief, we used an RNase H-based method to deplete rRNA from purified total FFPE RNA, followed by library preparation. The libraries were sequenced on BGISeq500 to generate at least 65 million (mean=81 million, sd=6 million) paired-end raw reads with a length of 100 bp for each sample. The computational analysis of RNA-seq data was performed as previously described (12, 13). Briefly, the sequencing reads that passed quality checking were mapped to the human genome reference (hg19) using HISAT2 (14) (version 2.1.0, default setting). FeatureCounts (15) was used to compute the read counts. For differential gene expression analysis between different groups, the original gene expression data were normalized by TMM (the trimmed mean of M values) (16). Only genes with a mean of greater than 15 reads and nonzero values across all samples were retained for normalization, which resulted in a total of 17,375 genes for downstream analysis. The normalized counts were fit into a negative binomial GLM for differential testing using edgeR (17), with tagwise dispersion and risk stratification as the single factor. Multiple testing was corrected by the Benjamini-Hochberg procedure (18) to control the false discovery rate (FDR) and to obtain the adjusted p values.
Gene Ontology (GO) enrichment analysis was performed by GOseq (19), where the differentially expressed genes identified as described above were supplied as the input for genes of interest. ClusterProfiler (20) was also used as a comparison. Gene set enrichment analysis was performed according to Subramanian et al. (21).
Statistical Analysis
The χ2 test or Fisher’s exact test was used to compare categorical variables, and the nonparametric test was used for continuous variables. The Kaplan–Meier method with the log-rank test was used to calculate the probability of PFS and OS. The effect of risk factors on PFS and OS was evaluated by the Cox proportional hazards regression model. Statistical analysis was performed using the IBM Statistical Package for Social Sciences (SPSS) version 21.0. A P value less than 0.05 was considered significant.
Results
Clinicopathological Characteristic
The age of patients with primary CNS DLBCL in the present cohort ranged from 32 to 80 years, with a median age of 56 years and a male-to-female ratio of 1.1:1. All patients were Chinese. Twenty-four patients (24/40, 60.0%) harbored a solitary tumor, while sixteen patients harbored multiple tumors (16/40, 40.0%). All forty cases showed diffuse and/or perivascular infiltration patterns. Cytomorphologically, the tumors consisted of atypical cells with medium-sized to large round, oval, irregular, or polymorphic nuclei and distinct nucleoli, corresponding to centroblasts or immunoblasts (Figure 1A). Twenty-nine cases (29/40, 72.5%) belonged to the non-GCB subtype according to Han’s classification, while eleven cases (11/40, 27.5%) belonged to the GCB subtype. All cases expressed CD20 (Figure 1B), and most cases (85.0%, 34/40) had a Ki67 proliferation fraction >70% (Figure 1C). MYC and BCL2 double expression was found in 50% (15/30) of available cases (Figures 1D, E). All cases were negative for EBER ISH (Figure 1F). No MYC/BCL2/BCL6 double/triple hit was found in any cases. Among these 40 patients, 39 patients were successfully followed up, with a median follow-up period of 445 days (ranged 37 to 1354 days).
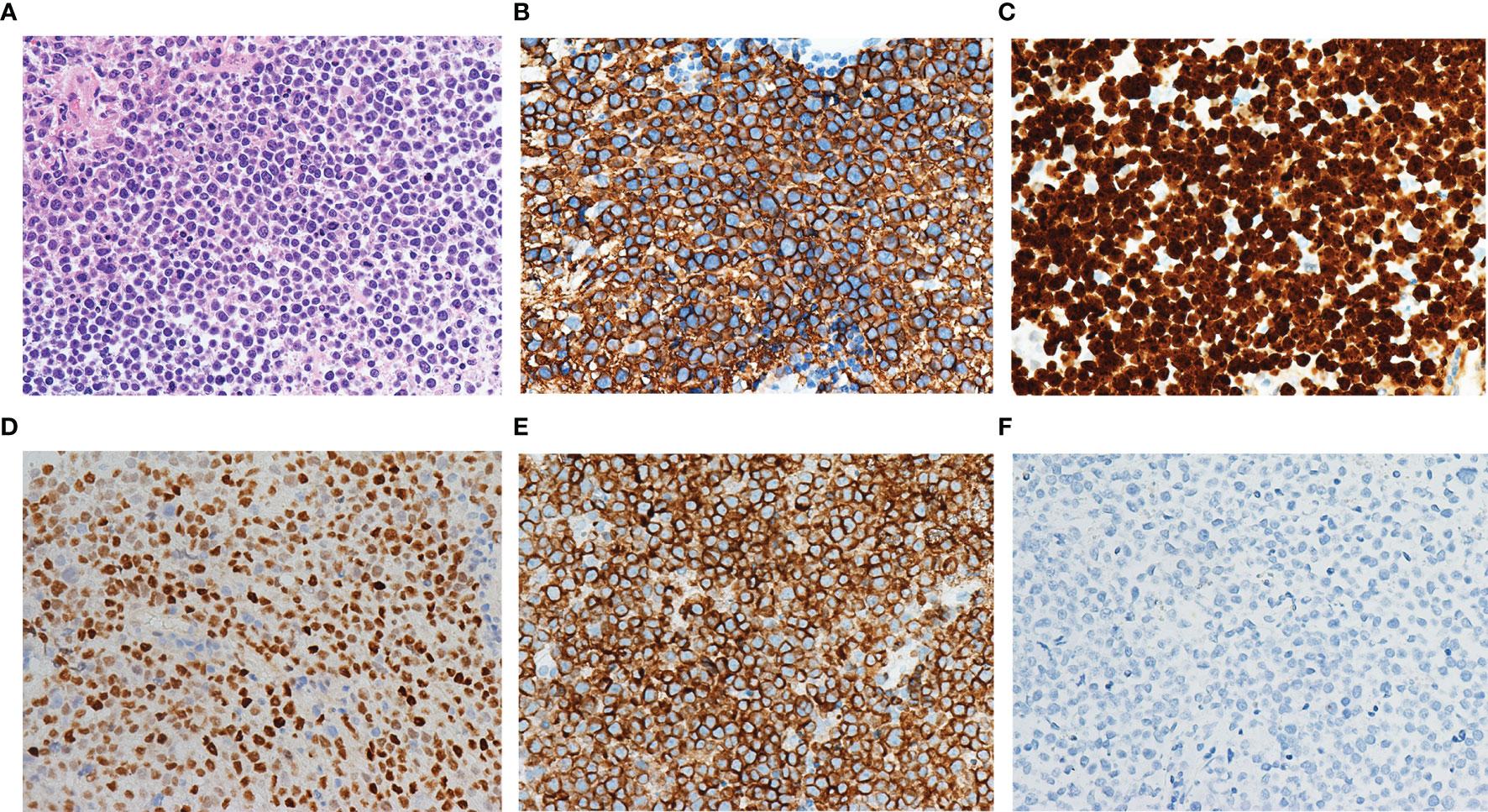
Figure 1 Morphology and immunophenotype of primary CNS DLBCL. The tumor consisted of atypical cells with medium-sized to large round, oval, irregular, or polymorphic nuclei and distinct nucleoli (A). The tumor cells expressed CD20 (B) and had a Ki67 proliferation fraction above 90% (C). MYC (D) and BCL2 (E) double expression were found. All cases were negative for EBER ISH (F).
Mutational Pattern of Primary CNS DLBCL
All cases of primary CNS DLBCL harbored at least one somatic mutated gene. As shown in Figure S1A, MYD88 (58.5%), CD79B (51.2%) and PIM1 (41.4%) were the three most frequently mutated genes (Figure S1B). Compared to the TCGA database, primary CNS DLBCL showed a mutation pattern distinct from that of DLBCL-NOS. In particular, primary CNS DLBCL had significantly more MYD88 mutations and fewer CREBBP mutations (Figures S1C, D), which was consistent with previously published data (4, 22–26). By univariate survival analysis, CD79B mutation correlated with better PFS but showed no benefit to OS, while PIM1 mutation and MYD88 mutation alone showed no influence on either PFS or OS (Figure S2).
Molecular Classification of Primary CNS DLBCL Based on PIM1 and CD79B Mutation Status
To further explore the clinicopathological significance of the mutated genes in primary CNS DLBCL, we performed hierarchical cluster analysis. Mutational analysis recognized two groups. Group 1, the so-called CDP (with CD79B and/or PIM1 mutation) group, was identified in 27 cases (67.5%). Group 2, the so-called non-CDP (without CD79B or PIM1 mutation) group, was found in 13 cases (32.5%). The CDP group tended to be observed in older patients (median age 57.0 vs. 48.4 y, p=0.015) and had significantly higher tumor mutation burden (TMB) (11.6 ± 3.3 vs 8.7 ± 5.0, p = 0.019) (Table 1). The mutated profiles and main clinicopathological characteristics of these two groups are shown in Figure 2. Interestingly, in the survival analysis, patients in the CDP group had significantly longer 2-year OS (76% and 40%, p=0.037) but not significantly longer 2-year PFS (50.0% and 29%, p=0.078) than those in the non-CDP group (Figure 3). Multivariate analysis revealed that age less than 60, no MYC and BCL2 double expression by IHC, and CDP group by NGS were three independent risk factors indicating favorable OS (Table 2).
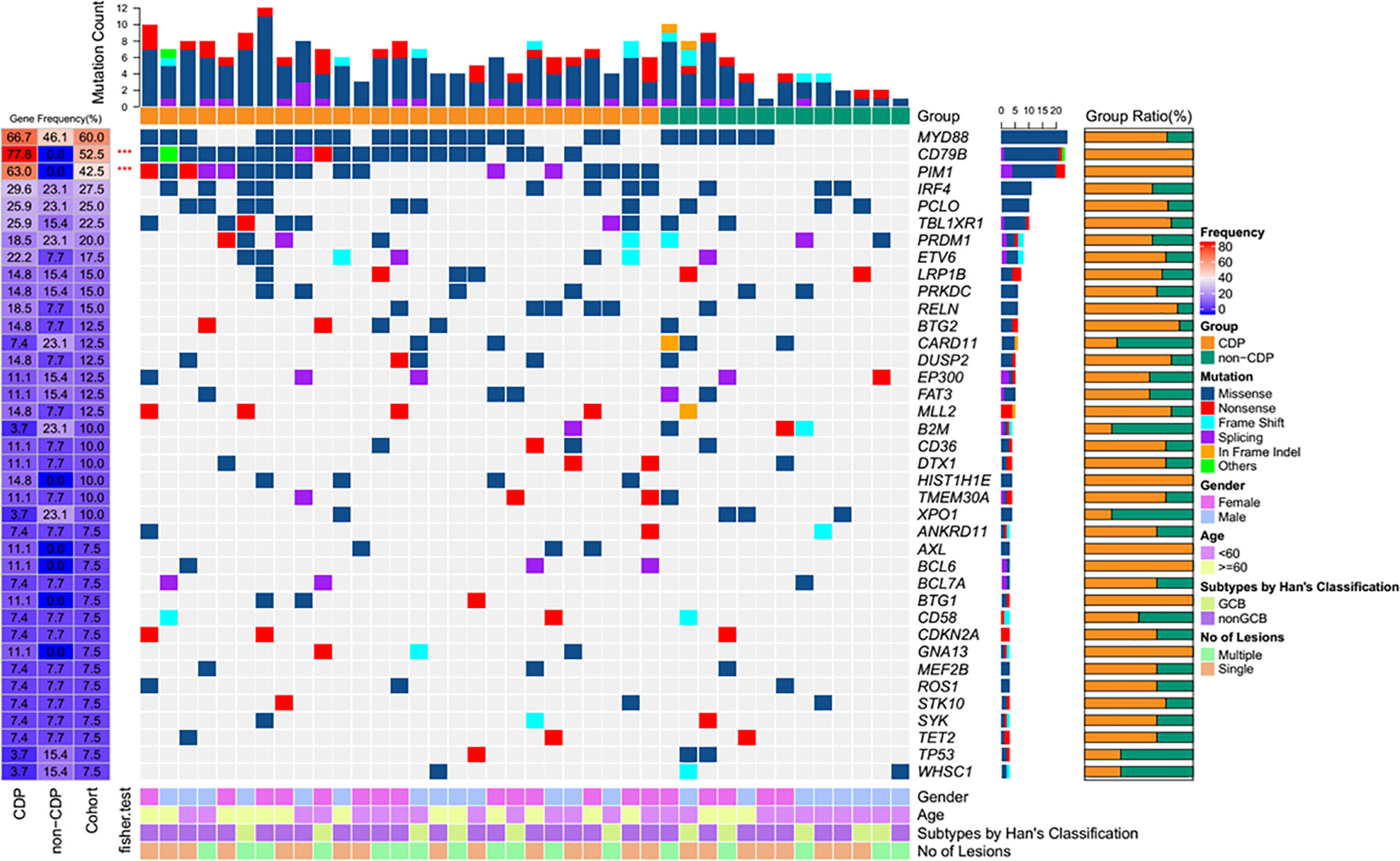
Figure 2 Heatmap of frequently mutated genes and clinical characteristics of the CDP and non-CDP groups.
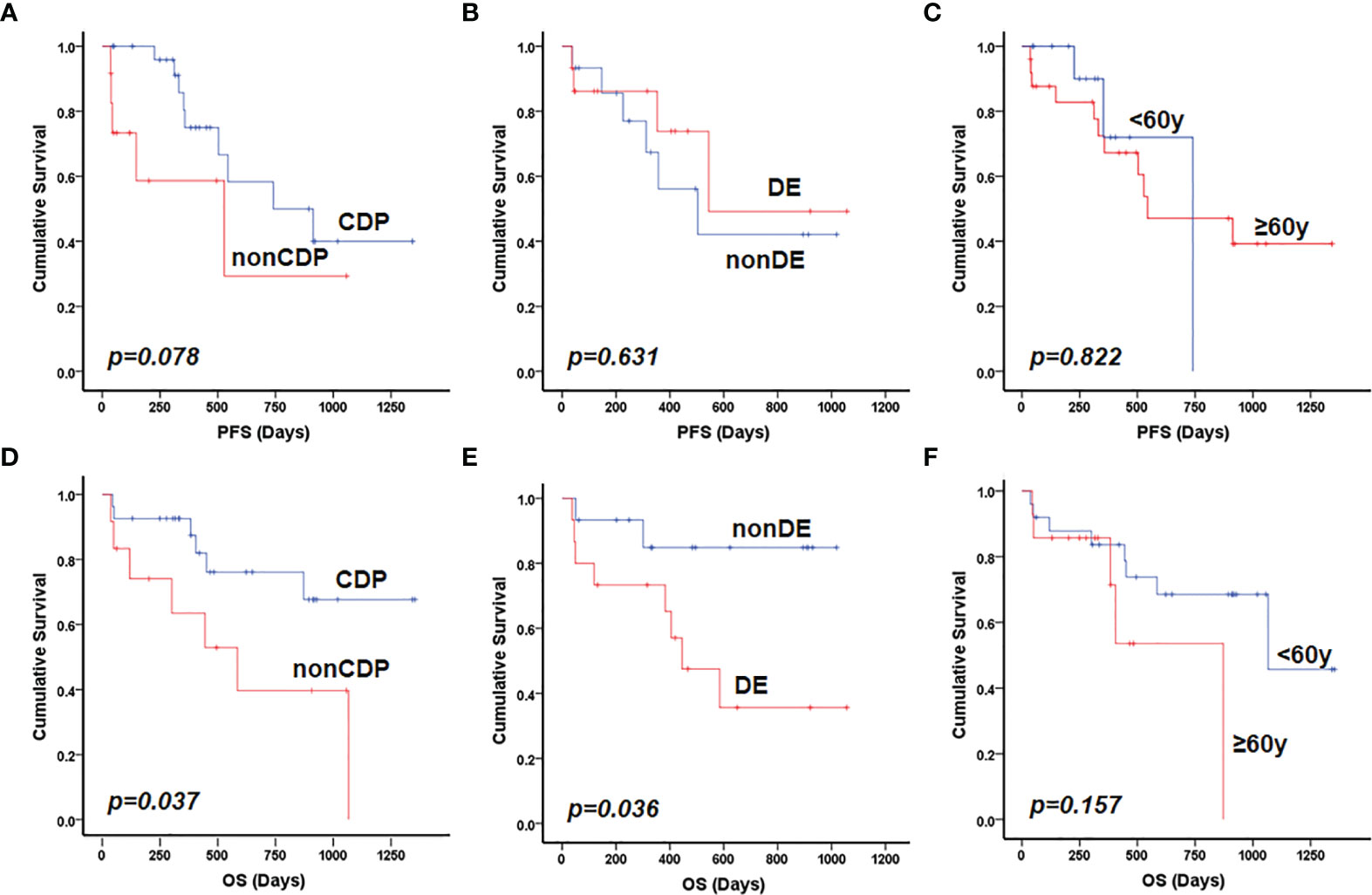
Figure 3 Prognostic factors for patients with primary CNS DLBCL. Patients in the CDP group had longer PFS compared with those in non-CDP group with borderline statistical significance (p=0.078) (A); MYC and BCL2 double expression (B) and older patient age (>60 years) (C) were not significantly related to inferior PFS. Both non-CDP group (D) and BCL2 and MYC double expression (E) but not older patient age (>60 years) (F) were significantly associated with inferior OS in the present cohort by univariate analysis. DE referred to double expression of MYC and BCL2 proteins.
PyClone analysis revealed that there were fewer main clones of the most frequently mutated genes in the non-CDP group than in the CDP group. Among them, 83 main clonal and 230 subclonal gene sites were found in the CDP group (Figure 4A), while 34 main clonal and 79 subclonal gene sites were found in the non-CDP group (Figure 4B). We found that clonal and subclonal genes were less shared between the CDP group and non-CDP group (Figure 4C). Distribution of high-frequency genes in CDP group and non-CDP group was shown in Figure S3. Spectrum display of high frequency mutation genes in CDP group and non-CDP group were shown in Figures S3A, B. The allele frequency distribution of respectively clonal and subclonal genes in the CDP group and non-CDP were shown in Figure S3C and Figure S3D. We define the frequency of high-frequency mutation genes as not less than 15% for guaranteed that there are at least two mutations in the non-CDP group and observed that the CDP group and non-CDP group have significant differences in these high-frequency subclonal genes (Figures 4D, E), indicating the subcloning heterogeneity between the groups.
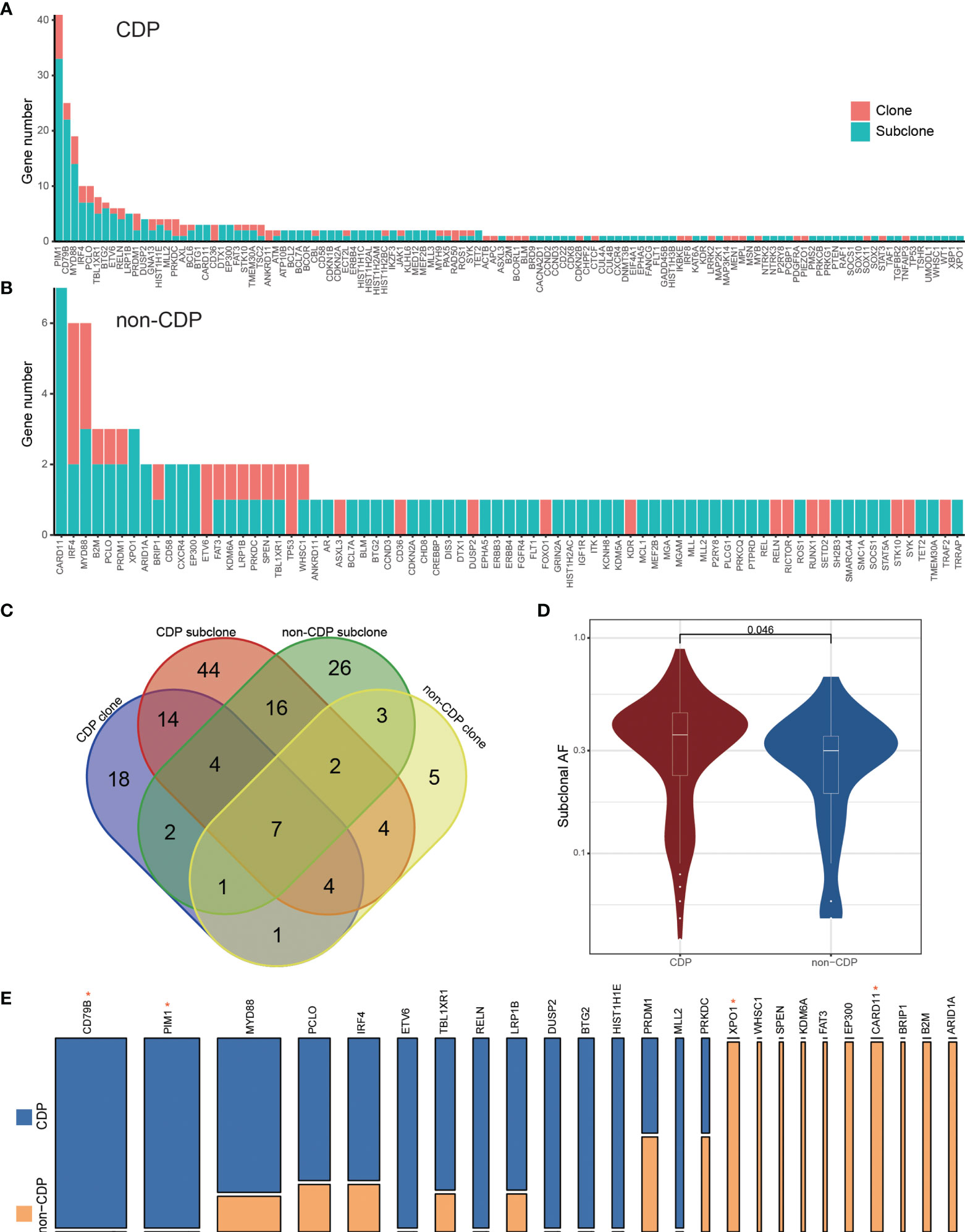
Figure 4 PyClone analysis to CDP and non-CDP groups. The clone and subclonal distribution of mutant genes in the CDP group (A) and non-CDP group (B). (C) The Venn diagram shows the shared genes status between groups. (D) shows the significant difference of allele frequencies in high-frequency subcloned mutant genes between the high CDP and non-CDP groups. (E) specifically displays the proportion of high-frequency subcloned mutant genes between the CDP group and non-CDP group. The width of the column represents the number of patients carrying the mutant gene, and the height represents the mutant gene proportion of the two groups. * represents Fisher's exact test, P value < 0.05.
Differential Risk-Related Enrichment of Gene Sets in the CDP and Non-CDP Groups
To investigate transcriptional aberrations between the CDP and non-CDP groups, RNA sequencing analysis was successfully conducted in 8 samples (including 2 samples in the CDP group and 6 samples in the non-CDP group) (Table S1). A total of 131 genes were identified as significantly differentially expressed with a p value ≤0.05 and |log2FC| ≥1 (Figure 5A). GO enrichment analysis of these genes suggested that some major categories of biological processes, especially intracellular metabolic mechanisms, were significantly altered in the CDP group compared to the non-CDP group (Figures 5B, C).
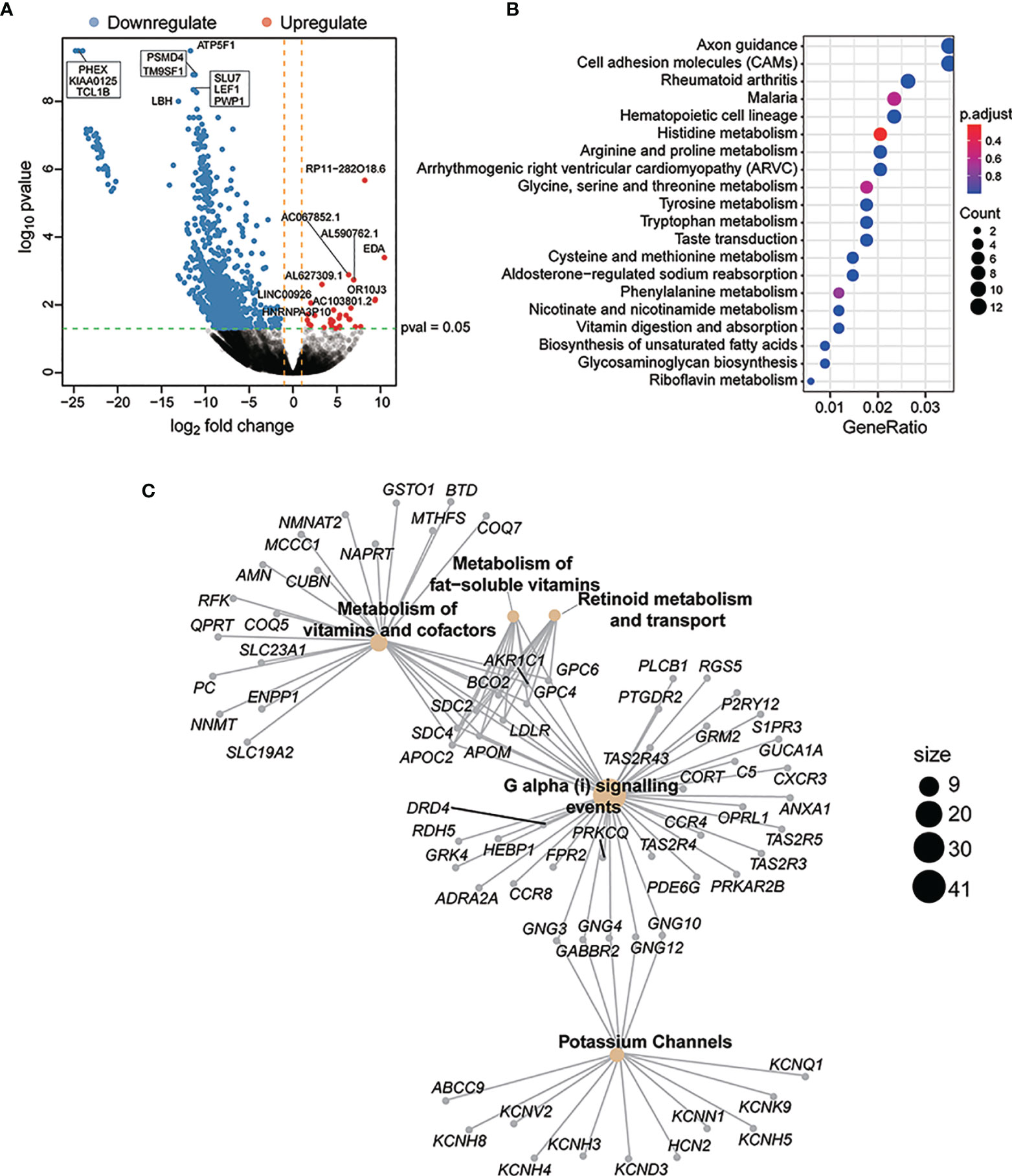
Figure 5 Differential risk-related enrichment of gene sets in CDP and non-CDP groups. A total of 131 genes were identified as significantly differentially expressed with a p value ≤0.05 and |log2F C|≥1 (A). GO enrichment analysis of these genes suggested that some major categories of biological processes, especially for intracellular metabolism mechanism, had been significantly altered for the CDP group compared to the non-CDP group. The y-axis represented different gene function entries, and the x-axis represented the proportion of differentially expressed genes in the corresponding entries to all genes in the entry. The “count”of the circle represented the number of differentially expressed genes enriched in the corresponding entry (B). Reactome network revealed differential gene expression between CDP and non-CDP groups. The yellow dots indicated significantly enriched Reactome entries, the “size” of the dots indicated the number of genes, and the gray dots indicated genes (C).
Discussion
Recently, the classification of DLBCL has promoted the clinical application of precision therapy in systemic DLBCL. However, as an independent rare subtype of DLBCL, due to the following potential reasons, current classification of systemic DLBCL is not applicable to CNS DLBCL: first, most CNS DLBCLs belong to non-GCB subtype based on IHC classification; second, most CNS DLBCL cases harbor MYD88 and CD79B mutations, resulting in a higher frequency of the MCD subtype of CNS DLBCL than systemic DLBCL; and third, the molecular classification of systemic DLBCL originates from patients who receive R-CHOP-like chemoimmunotherapy (R-CHOP is the abbreviated name for the combination of drugs that are commonly used as chemotherapy for DLBCL, including rituximab, cyclophosphamide, doxorubicin, vincristine and prednisone), while the standard treatment of CNS DLBCL is HD-MTX-based chemoimmunotherapy, which has different antitumor mechanisms. To date, molecular classification specific to CNS DLBCL is lacking. Thus, a new molecular classification is needed to elucidate the relationship between the mutation patterns and clinicopathological features and to be used for risk stratification and prognostic prediction.
In our study, we used NGS technology to perform targeted deep sequencing on the coding regions of 413 lymphoma-related genes. The mutation frequency of MYD88 was the highest, followed by CD79B, PIM1 and IRF4, which was consistent with previous studies (4, 22–26). According to previous studies, more than 60% of systemic DLBCL patients can be cured with R-CHOP chemotherapy (27–29). However, patients with CNS DLBCL have a remarkably worse outcome (30–33). The International Extranodal Lymphoma Study Group identified five clinical variables that are correlated with prognoses of CNS DLBCL: age >60 years, elevated lactate dehydrogenase level, Eastern Cooperative Oncology Group (ECOG) performance status >1, high cerebrospinal fluid protein concentration, and location of the tumor in deep brain regions (34). Researchers from Memorial Sloan Kettering Cancer Center (MSKCC) also reported another prognostic score for CNS DLBCL in 2006, which included age more than 50 years and KPS score more than 70 as two independent risk factors indicating unfavorable survival (35). Our present study once again supports the result that age >60 years is an unfavorable risk factor for CNS DLBCL by multivariate analysis. It is worth noting that both the IELSG score and the MSKCC score were developed in pre-rituximab era, patients enrolled received HD-MTX based chemotherapy and/or radiotherapy. Few patients received rituximab in those two studies. However, in our study, most patients enrolled received rituximab in combination with HD-MTX. As was shown in IESLG32 trial, application of rituximab significantly improved survival of CNS DLBCL, and IELSG score did not show any prognostic value when rituximab was used (36). So we believed that new prognostic model is needed to more accurately predict CNS PCNSL’s prognosis in rituximab era. Moreover, we found that MYC and BCL2 double expression by IHC significantly related to unfavorable OS. Besides, the prognostic significance of CD79B mutation in primary DLBCL of the CNS remain controversial. Some researchers concluded that CD79B mutation predicts better PFS, while others reported that CD79B mutation is an unfavorable risk factor for predicting PFS (37, 38). However, cohorts of these studies were relatively small due to the rarity of this disease; and the discrepancy may due to random sampling error. In present study, we first reported that PIM1 and CD79B mutation status impacts the outcome of primary CNS DLBCL after high-dose methotrexate-based polychemotherapy. CNS DLBCL patients without PIM1 and CD79B mutations had inferior long-term survival after HD-MTX-based chemoimmunotherapy in the present study, even though they were younger and had a lower MCD subtype. Therefore, in terms of application, our data suggest that a new molecular classification based on the mutational status of CD79B and PIM1 could be used to predict patient outcomes in CNS DLBCL. What’s more, we found that CDP group had higher TMB than non-CDP group. Since PD1/PDL1-based immunotherapy showed promising efficacy in part of CNS DLBCL (39), it is possible that CDP group may be more sensitive to immune checkpoint inhibitor treatment than non-CDP group, which deserve further exploration.
Although we established such a molecular classification for CNS DLBCL in present study, the underlying mechanism by which CD79B and PIM1 mutational status impact the outcome of primary CNS DLBCL after HD-MTX-based polychemotherapy remains unknown. We tried to make some explanations. On one hand, the PyClone analysis showed that the CDP and non-CDP groups had different subcloning heterogeneity. As was proven in other cancers, such as lung cancer, MDS, or rectal cancer, intratumor heterogeneity is a common phenomenon, representing the evolutionary process of tumor cells (40–42). The clonal hierarchy has a distinct ranking, and the resultant invariant combinations of main clone and subclone mutations yield the specific clinical phenotype and treatment response. On the other hand, RNA sequencing revealed that the altered genes between the two groups primarily enriched in pathways related to intracellular metabolism mechanisms. Metabolic mechanisms are one main factor influencing treatment sensitivity. For example, P-glycoprotein, which is a transmembrane efflux pump, can promote drug efflux in cancer cells, thus reducing the drug concentration in cancer cells and inducing multidrug resistance (43). Another example is that the intergenic single-nucleotide polymorphism of DHFR and FPGS could affect the levels of MTX in the serum, which results in inadequate treatment intensity and disease relapse after HD-MTX treatment in acute lymphoblastic leukemia patients (44). All patients included in the present cohort received HD-MTX-based chemoimmunotherapy, which is coincidentally an anti-metabolic treatment. Different intracellular metabolic mechanisms may induce different sensitivities to HD-MTX-based treatment.
This study is limited in some ways. Although a relatively large cohort of CNS DLBCL was included, the number of cases was still small due to the rarity of this disease, and some of the results may require verification. Some highly mutated genes in primary CNS DLBCL, such as MUC16, ODZ4 and SLIT2 reported in the previous studies were not covered in our present NGS panel (26, 45). In addition, all patients in the present cohort solely received HD-MTX-based polychemotherapy, while no patient received BTK inhibitors, programmed death 1 (PD-1) and/or thiotepa. Therefore, our molecular classification may only be applicable to patients who receive similar treatments. Furthermore, although we have tried to explain the underlying reasons why this molecular classification impacts patient outcomes based on PyClone analysis and limited cases of RNA sequencing, the mechanism is still unknown.
In conclusion, we developed a new molecular classification to divide CNS DLBCL into CDP and non-CDP groups based on the mutational status of CD79B and PIM1. CNS DLBCL patients with PIM1 and/or CD79B mutation had favorable long-term survival after HD-MTX-based chemoimmunotherapy. The potential molecular mechanism awaits further investigation.
Data Availability Statement
The data presented in the study are deposited in the Genome Sequence Archive of the BIG Data Center at the Beijing Institue of Genomics, Chinese Academy of Science, under the accession number HRA001659 (HTTP://bigd.big.ac.cn/gsa-human). Code is available from corresponding author on resonable request.
Ethics Statement
The studies involving human participants were reviewed and approved by the ethical committee at Shenzhen People’s Hospital (Reference number: LL-KY-2020199). The patients/participants provided their written informed consent to participate in this study.
Author Contributions
Conceptualization: XZ and YH. Data acquisition: PK. Methodology: JZ, MZ, and LL. Data analysis: LL, FL, YX, XG, YG, XX, and XY. Writing original draft and editing: JZ, MZ, and YH. Data curation: JZ and YH. Project administration: XZ and YH. All authors read and approved the final manuscript.
Conflict of Interest
Authors LL, FL, YX, XG, YG, XX, XY were employed by company Geneplus-Beijing.
The remaining authors declare that the research was conducted in the absence of any commercial or financial relationships that could be construed as a potential conflict of interest.
Publisher’s Note
All claims expressed in this article are solely those of the authors and do not necessarily represent those of their affiliated organizations, or those of the publisher, the editors and the reviewers. Any product that may be evaluated in this article, or claim that may be made by its manufacturer, is not guaranteed or endorsed by the publisher.
Acknowledgments
The National Natural Science Foundation of China (No. 81600168 and 81702082), the Sanming Project of Medicine in Shenzhen (No. SZSM201512006), the Medical Science and Technology Foundation of Guangdong Province (A2019401).
Supplementary Material
The Supplementary Material for this article can be found online at: https://www.frontiersin.org/articles/10.3389/fonc.2022.824632/full#supplementary-material
References
1. Grimm KE, O'Malley DP. Aggressive B Cell Lymphomas in the 2017 Revised WHO Classification of Tumors of Hematopoietic and Lymphoid Tissues. Ann Diagn Pathol (2019) 38:6–10. doi: 10.1016/j.anndiagpath.2018.09.014
2. Seidel S, Schlegel U. Have Treatment Protocols for Primary CNS Lymphoma Advanced in the Past 10 Years. Expert Rev Anticancer Ther (2019) 19(10):909–15. doi: 10.1080/14737140.2019.1677157
3. Alizadeh AA, Eisen MB, Davis RE, Ma C, Lossos IS, Rosenwald A, et al. Distinct Types of Diffuse Large B-Cell Lymphoma Identified by Gene Expression Profiling. Nature (2000) 403(6769):503–11. doi: 10.1038/35000501
4. Wright GW, Huang DW, Phelan JD, Coulibaly ZA, Roulland S, Young RM, et al. A Probabilistic Classification Tool for Genetic Subtypes of Diffuse Large B Cell Lymphoma With Therapeutic Implications. Cancer Cell (2020) 37(4):551–68.e14. doi: 10.1016/j.ccell.2020.03.015
5. Schmitz R, Wright GW, Huang DW, Johnson CA, Phelan JD, Wang JQ, et al. Genetics and Pathogenesis of Diffuse Large B-Cell Lymphoma. N Engl J Med (2018) 378(15):1396–407. doi: 10.1056/NEJMoa1801445
6. Chapuy B, Stewart C, Dunford AJ, Kim J, Kamburov A, Redd RA, et al. Molecular Subtypes of Diffuse Large B Cell Lymphoma Are Associated With Distinct Pathogenic Mechanisms and Outcomes. Nat Med (2018) 24(5):679–90. doi: 10.1038/s41591-018-0016-8
7. Ho KG, Grommes C. Molecular Profiling of Primary Central Nervous System Lymphomas - Predictive and Prognostic Value? Curr Opin Neurol (2019) 32(6):886–94. doi: 10.1097/WCO.0000000000000759
8. Lionakis MS, Dunleavy K, Roschewski M, Widemann BC, Butman JA, Schmitz R, et al. Inhibition of B Cell Receptor Signaling by Ibrutinib in Primary CNS Lymphoma. Cancer Cell (2017) 31(6):833–43.e5. doi: 10.1016/j.ccell.2017.04.012
9. Lv X, Zhao M, Yi Y, Zhang L, Guan Y, Liu T, et al. Detection of Rare Mutations in CtDNA Using Next Generation Sequencing. J Visualized Experiments JoVE (2017) (126):56342. doi: 10.3791/56342
10. Robinson JT, Thorvaldsdóttir H, Winckler W, Guttman M, Lander ES, Getz G, et al. Integrative Genomics Viewer. Nat Biotechnol (2011) 29(1):24–6. doi: 10.1038/nbt.1754
11. Roth A, Khattra J, Yap D, Wan A, Laks E, Biele J, et al. PyClone: Statistical Inference of Clonal Population Structure in Cancer. Nat Methods (2014) 11(4):396–8. doi: 10.1038/nmeth.2883
12. Li J, Fu C, Speed TP, Wang W, Symmans WF. Accurate RNA Sequencing From Formalin-Fixed Cancer Tissue To Represent High-Quality Transcriptome From Frozen Tissue. JCO Precis Oncol (2018) 2018:2:PO.17.00091. doi: 10.1200/PO.17.00091
13. Liu H, Kuang X, Zhang Y, Ye Y, Li J, Liang L, et al. ADORA1 Inhibition Promotes Tumor Immune Evasion by Regulating the ATF3-PD-L1 Axis. Cancer Cell (2020) 37(3):324–39.e8. doi: 10.1016/j.ccell.2020.02.006
14. Kim D, Paggi JM, Park C, Bennett C, Salzberg SL. Graph-Based Genome Alignment and Genotyping With HISAT2 and HISAT-Genotype. Nat Biotechnol (2019) 37(8):907–15. doi: 10.1038/s41587-019-0201-4
15. Liao Y, Smyth GK, Shi W. Featurecounts: An Efficient General Purpose Program for Assigning Sequence Reads to Genomic Features. Bioinformatics (2014) 30(7):923–30. doi: 10.1093/bioinformatics/btt656
16. Robinson MD, Oshlack A. A Scaling Normalization Method for Differential Expression Analysis of RNA-Seq Data. Genome Biol (2010) 11(3):R25. doi: 10.1186/gb-2010-11-3-r25
17. Robinson MD, McCarthy DJ, Smyth GK. Edger: A Bioconductor Package for Differential Expression Analysis of Digital Gene Expression Data. Bioinformatics (2010) 26(1):139–40. doi: 10.1093/bioinformatics/btp616
18. Benjamini Y, Hochberg Y. Controlling the False Discovery Rate: A Practical and Powerful Approach to Multiple Testing. J R Stat Society: Ser B (Methodological) (1995) 57(1):289–300. doi: 10.1111/j.2517-6161.1995.tb02031.x
19. Young MD, Wakefield MJ, Smyth GK, Oshlack A. Gene Ontology Analysis for RNA-Seq: Accounting for Selection Bias. Genome Biol (2010) 11(2):R14. doi: 10.1186/gb-2010-11-2-r14
20. Yu G, Wang LG, Han Y, He QY. Clusterprofiler: An R Package for Comparing Biological Themes Among Gene Clusters. OMICS (2012) 16(5):284–7. doi: 10.1089/omi.2011.0118
21. Subramanian A, Tamayo P, Mootha VK, Mukherjee S, Ebert BL, Gillette MA, et al. Gene Set Enrichment Analysis: A Knowledge-Based Approach for Interpreting Genome-Wide Expression Profiles. Proc Natl Acad Sci USA (2005) 102(43):15545–50. doi: 10.1073/pnas.0506580102
22. Bodor C, Alpar D, Marosvari D, Galik B, Rajnai H, Batai B, et al. Molecular Subtypes and Genomic Profile of Primary Central Nervous System Lymphoma. J Neuropathol Exp Neurol (2020) 79(2):176–83. doi: 10.1093/jnen/nlz125
23. Zheng M, Perry AM, Bierman P, Loberiza F Jr., Nasr MR, Szwajcer D, et al. Frequency of MYD88 and CD79B Mutations, and MGMT Methylation in Primary Central Nervous System Diffuse Large B-Cell Lymphoma. Neuropathol Off J Japanese Soc Neuropathol (2017) 37(6):509–16. doi: 10.1111/neup.12405
24. Nakamura T, Tateishi K, Niwa T, Matsushita Y, Tamura K, Kinoshita M, et al. Recurrent Mutations of CD79B and MYD88 Are the Hallmark of Primary Central Nervous System Lymphomas. Neuropathol Appl Neurobiol (2016) 42(3):279–90. doi: 10.1111/nan.12259
25. Fukumura K, Kawazu M, Kojima S, Ueno T, Sai E, Soda M, et al. Genomic Characterization of Primary Central Nervous System Lymphoma. Acta Neuropathologica (2016) 131(6):865–75. doi: 10.1007/s00401-016-1536-2
26. Vater I, Montesinos-Rongen M, Schlesner M, Haake A, Purschke F, Sprute R, et al. The Mutational Pattern of Primary Lymphoma of the Central Nervous System Determined by Whole-Exome Sequencing. Leukemia (2015) 29(3):677–85. doi: 10.1038/leu.2014.264
27. Coiffier B, Lepage E, Briere J, Herbrecht R, Tilly H, Bouabdallah R, et al. CHOP Chemotherapy Plus Rituximab Compared With CHOP Alone in Elderly Patients With Diffuse Large-B-Cell Lymphoma. N Engl J Med (2002) 346(4):235–42. doi: 10.1056/NEJMoa011795
28. Habermann TM, Weller EA, Morrison VA, Gascoyne RD, Cassileth PA, Cohn JB, et al. Rituximab-CHOP Versus CHOP Alone or With Maintenance Rituximab in Older Patients With Diffuse Large B-Cell Lymphoma. J Clin Oncol (2006) 24(19):3121–7. doi: 10.1200/JCO.2005.05.1003
29. Pfreundschuh M, Trümper L, Osterborg A, Pettengell R, Trneny M, Imrie K, et al. CHOP-Like Chemotherapy Plus Rituximab Versus CHOP-Like Chemotherapy Alone in Young Patients With Good-Prognosis Diffuse Large-B-Cell Lymphoma: A Randomised Controlled Trial by the MabThera International Trial (MInT) Group. Lancet Oncol (2006) 7(5):379–91. doi: 10.1016/S1470-2045(06)70664-7
30. Norden AD, Drappatz J, Wen PY, Claus EB. Survival Among Patients With Primary Central Nervous System Lymphoma, 1973-2004. J Neuro-Oncol (2011) 101(3):487–93. doi: 10.1007/s11060-010-0269-7
31. Perkins A, Liu G. Primary Brain Tumors in Adults: Diagnosis and Treatment. Am Fam Physician (2016) 93(3):211–7.
32. Yin W, Xia X, Wu M, Yang H, Zhu X, Sun W, et al. The Impact of BCL-2/MYC Protein Expression and Gene Abnormality on Primary Central Nervous System Diffuse Large B-Cell Lymphoma. Int J Clin Exp Pathol (2019) 12(6):2215–23.
33. Schorb E, Fox CP, Kasenda B, Linton K, Martinez-Calle N, Calimeri T, et al. Induction Therapy With the MATRix Regimen in Patients With Newly Diagnosed Primary Diffuse Large B-Cell Lymphoma of the Central Nervous System - an International Study of Feasibility and Efficacy in Routine Clinical Practice. Br J Haematol (2020) 189(5):879–87. doi: 10.1111/bjh.16451
34. Ferreri AJ, Blay JY, Reni M, Pasini F, Spina M, Ambrosetti A, et al. Prognostic Scoring System for Primary CNS Lymphomas: The International Extranodal Lymphoma Study Group Experience. J Clin Oncol (2003) 21(2):266–72. doi: 10.1200/JCO.2003.09.139
35. Abrey LE, Ben-Porat L, Panageas KS, Yahalom J, Berkey B, Curran W, et al. Primary Central Nervous System Lymphoma: The Memorial Sloan-Kettering Cancer Center Prognostic Model. J Clin Oncol Off J Am Soc Clin Oncol (2006) 24(36):5711–5. doi: 10.1200/JCO.2006.08.2941
36. Ferreri AJ, Cwynarski K, Pulczynski E, Ponzoni M, Deckert M, Politi LS, et al. Chemoimmunotherapy With Methotrexate, Cytarabine, Thiotepa, and Rituximab (MATRix Regimen) in Patients With Primary CNS Lymphoma: Results of the First Randomisation of the International Extranodal Lymphoma Study Group-32 (IELSG32) Phase 2 Trial. Lancet Haematol (2016) 3(5):e217–27. doi: 10.1016/S2352-3026(16)00036-3
37. Braggio E, Van Wier S, Ojha J, McPhail E, Asmann YW, Egan J, et al. Genome-Wide Analysis Uncovers Novel Recurrent Alterations in Primary Central Nervous System Lymphomas. Clin Cancer Res an Off J Am Assoc Cancer Res (2015) 21(17):3986–94. doi: 10.1158/1078-0432.CCR-14-2116
38. Zhou Y, Liu W, Xu Z, Zhu H, Xiao D, Su W, et al. Analysis of Genomic Alteration in Primary Central Nervous System Lymphoma and the Expression of Some Related Genes. Neoplasia (New York NY) (2018) 20(10):1059–69. doi: 10.1016/j.neo.2018.08.012
39. Monabati A, Nematollahi P, Dehghanian A, Safaei A, Sadeghipour A, Movahedinia S, et al. Immune Checkpoint Molecules in Primary Diffuse Large B-Cell Lymphoma of the Central Nervous System. Basic Clin Neurosci (2020) 11(4):491–8. doi: 10.32598/bcn.11.4.2542.1
40. Kunimasa K, Hirotsu Y, Nakamura H, Tamiya M, Iijima Y, Ishida H, et al. Rapid Progressive Lung Cancers Harbouring Multiple Clonal Driver Mutations With Big Bang Evolution Model. Cancer Genet (2020) 241:51–6. doi: 10.1016/j.cancergen.2019.12.006
41. Nagata Y, Makishima H, Kerr CM, Przychodzen BP, Aly M, Goyal A, et al. Invariant Patterns of Clonal Succession Determine Specific Clinical Features of Myelodysplastic Syndromes. Nat Commun (2019) 10(1):5386. doi: 10.1038/s41467-019-13001-y
42. Frydrych LM, Ulintz P, Bankhead A, Sifuentes C, Greenson J, Maguire L, et al. Rectal Cancer Sub-Clones Respond Differentially to Neoadjuvant Therapy. Neoplasia (New York NY) (2019) 21(10):1051–62. doi: 10.1016/j.neo.2019.08.004
43. Liu J, Zhao L, Shi L, Yuan Y, Fu D, Ye Z, et al. A Sequentially Responsive Nanosystem Breaches Cascaded Bio-Barriers and Suppresses P-Glycoprotein Function for Reversing Cancer Drug Resistance. ACS Appl Mater Interfaces (2020) 12(49):54343–55. doi: 10.1021/acsami.0c13852
44. Tulstrup M, Moriyama T, Jiang C, Grosjean M, Nersting J, Abrahamsson J, et al. Effects of Germline DHFR and FPGS Variants on Methotrexate Metabolism and Relapse of Leukemia. Blood (2020) 136(10):1161–8. doi: 10.1182/blood.2020005064
Keywords: central nervous system, diffuse large B-cell lymphoma, molecular classification, PIM1, CD79B
Citation: Zhou J, Zuo M, Li L, Li F, Ke P, Zhou Y, Xu Y, Gao X, Guan Y, Xia X, Yi X, Zhang X and Huang Y (2022) PIM1 and CD79B Mutation Status Impacts the Outcome of Primary Diffuse Large B-Cell Lymphoma of the CNS. Front. Oncol. 12:824632. doi: 10.3389/fonc.2022.824632
Received: 29 November 2021; Accepted: 17 January 2022;
Published: 09 February 2022.
Edited by:
Shimin Hu, University of Texas MD Anderson Cancer Center, United StatesReviewed by:
Habibe Kurt, Warren Alpert Medical School of Brown University, United StatesMadhu M. Ouseph, Cornell University, United States
Andres Quesada, University of Texas MD Anderson Cancer Center, United States
Copyright © 2022 Zhou, Zuo, Li, Li, Ke, Zhou, Xu, Gao, Guan, Xia, Yi, Zhang and Huang. This is an open-access article distributed under the terms of the Creative Commons Attribution License (CC BY). The use, distribution or reproduction in other forums is permitted, provided the original author(s) and the copyright owner(s) are credited and that the original publication in this journal is cited, in accordance with accepted academic practice. No use, distribution or reproduction is permitted which does not comply with these terms.
*Correspondence: Yuhua Huang, huangyh@sysucc.org.cn; Xinyou Zhang, zhangxinyou0518@sina.com
†These authors have contributed equally to this work
‡These authors share last authorship