- 1Department of Respiration, Liyuan Hospital, Tongji Medical College, Huazhong University of Science and Technology, Wuhan, China
- 2Department of Orthopedics, Liyuan Hospital, Tongji Medical College, Huazhong University of Science and Technology, Wuhan, China
As the second most common cancer in the world, the development of lung cancer is closely related to factors such as heredity, environmental exposure, and lung microenvironment, etc. Early screening and diagnosis of lung cancer can be helpful for the treatment of patients. Currently, CT screening and histopathologic biopsy are widely used in the clinical detection of lung cancer, but they have many disadvantages such as false positives and invasive operations. Microbes are another genome of the human body, which has recently been shown to be closely related to chronic inflammatory, metabolic processes in the host. At the same time, they are important players in cancer development, progression, treatment, and prognosis. The use of microbes for cancer therapy has been extensively studied, however, the diagnostic role of microbes is still unclear. This review aims to summarize recent research on using microbes for lung cancer detection and present the current shortcomings of microbes in collection and detection. Finally, it also looks ahead to the clinical benefits that may accrue to patients in the future about screening and early detection.
1 Introduction
Influenced by smoking and environmental factors, lung cancer is a disease with a high incidence worldwide. Small-cell lung cancer (SCLC) and non-small cell lung cancer (NSCLC) are the two histological subtypes of lung cancer. SCLC accounts for 15% of lung cancers and is usually caused by smoking. At the same time, NSCLC is divided into squamous (LUSC), adenocarcinomas (LUAD), large cell carcinomas, and bronchial carcinoid cancers, of which LUAD is the most common cancer and is usually seen in non-smoking women. Due to the characteristics of insidious disease onset and easy invasion in the early stage, the five-year survival rate of lung cancer patients is only 19.8% (1). Although the emergence of immunotherapy has provided a chance of survival for patients with advanced disease, 60% of patients do not have immunotherapy driver genes, and even the immune response rate of patients with NSCLC is only 15-25% (2).In addition, some patients undergo immunotherapy for a certain period and develop immune escape, while others discontinue treatment due to severe immune-related adverse effects (3). If the disease is diagnosed early, the five-year survival rate of lung cancer patients can be increased to 59% (4).
Currently, lung cancer detection includes asymptomatic screening and diagnostic evaluation. The National Comprehensive Cancer Network (NCCN) has shown that lung cancer mortality can be reduced by a relative 20% with low-dose CT (LDCT) chest screening in high-risk populations (5). However, LDCT radiation exposure induces cancer, and false positives on LDCT images are high, with 24.2% of screened individuals still requiring further testing (6). For another, the definitive diagnosis of lung cancer is still based on invasive biopsy histopathologic testing. Still, biopsy has disadvantages such as high price, susceptibility to complications, and the need for sufficient diseased lung tissue (7). Therefore, we are eager to find potential markers that can help in the specific detection of lung cancer patients. Since tumor cells are transformed from normal cells, biomarkers are an objective assessment for the early detection of tumors. Some circulating antigens such as CYFRA21-1, CEA, NSE, and SCC-Ag have been used as markers for the clinical detection of lung cancer (7, 8) (Table 1).
The microbiota is a community of all microbial species, including bacteria, fungi, viruses, archaea, and protozoa. Depending on their preferences for nourishment and oxygen, different microbes are often found on human body surfaces that come into touch with the external environment, including the skin, mouth, respiratory system, gastrointestinal tract, and urine tract (4). The human microbiota begins to colonize at birth. It is closely related to the maternal microbiota, how the infant is born, how he or she is fed, and the environment in which he or she survives. These microbes remain relatively stable in the body as adults through a complex symbiotic relationship with the host (12). The host can provide the environment for the microbes to metabolize nutrients. Conversely, the microbes can regulate the host’s metabolism, nutritional response, and immune function and provide the host with vitamins and trace elements (13). With the emergence of next-generation sequencing (NGS) in recent years, microbes have gained widespread attention in the development of cancer and its treatment. However, there are still many difficulties in their use for early prevention and detection. This review focuses on the possible mechanisms and research progress in using microbes for lung cancer detection and discusses the deficiencies in sample collection and testing, which provide new ideas for the precise detection of lung cancer.
2 Current status of microbes used for cancer detection
The use of microbes to aid in cancer detection is well-founded, with studies showing that 15.4-17.8 percent of cancers are associated with microbial infections (14). The International Agency for Research on Cancer (IARC) has identified 11 microbes as class I carcinogens, including bacteria, viruses, and parasites, such as Helicobacter pylori (Hp), Hepatitis B virus, HPV, EBV, and Schistosoma chinensis, among others (15). Since the largest tribe of microbiota in the body is the gut microbiota, their association with gastrointestinal tumors has been the most studied. In the stomach, the oncoprotein cytotoxin-associated gene A (CagA) and vacuolar toxin A (VacA) produced by Hp increase the production of inflammatory cytokines and immune cells, as well as the methylation of tumor suppressor genes CpG islands, which ultimately lead to gastric adenocarcinoma (16). The liver has a rich circulatory system. Hepatitis B and C viruses, aflatoxin B1, and gastrointestinal flora can enter the circulation and have a major impact on the liver through the hepatic portal system (17). In addition, several studies have demonstrated that host microbes, such as Fusobacterium nucleatum, Escherichia coli, and Bacteroides fragilis, influence colorectal carcinogenesis by inducing intestinal inflammation (18, 19).
3 Possibility of microbes for lung cancer detection
Meta-analyses have shown that some bacteria can directly promote lung cancer. For example, HP promotes the development of lung cancer 3.24 times faster than non-HP lung cancer patients (OR = 3.24, 95%CI = 1.11–9.47) (20), and chlamydial infection can increase the risk of lung cancer in men under 55 years of age (21). Also, Mycobacterium tuberculosis (TB) has a strong association with lung cancer, especially LUAD, while TB is also seen as a risk factor for non-smoking patients(RR = 1.8, 95%CI = 1.4-2.2 in non-smoking patients. RR = 2.9, 95%CI = 1.6-5.3 in smoking patients. RR = 1.6, 95%CI = 1.2-2.1 in LUAD patients) (22). In addition to direct carcinogenesis, some microbes can indirectly affect cancer, and the pathological mechanisms of microbial effects on lung cancer have been discussed in several studies (23, 24). Microbes have been found to exacerbate lung cancer progression through various processes, including altering host genes, activating oncogenic pathways, developing chronic inflammation, affecting the immune reaction, and generating metabolites (25). (i) Microbes can produce enzymatically active protein toxins that directly damage DNA or promote the production of reactive oxygen species (ROS) leading to DNA damage. For example, colibactin and cytolethal distending toxin (CDT) can lead to genomic destabilization and induce mutations (24, 26), while Bacteroides fragilis toxin (BFT) indirectly damages DNA by producing superoxide dismutase (SOD) (27). (ii) Microbial dysregulation creates an inflammatory cellular microenvironment that activates oncogenic pathways and exacerbates tumor progression (28). For example, Veillonella and Prevotella upregulate the expression of inflammatory mediators IL-1, IL-23, TNF, and IL-17, leading to the activation of ERK, PI-3K, and P53 signaling pathways, and promoting tumor cell proliferation (29, 30). (iii) Microbes bind to pattern recognition receptors (PRR), activating signaling pathways and inducing an inflammatory response. For example, toll-like receptors (TLRs) activate the cytokine Th17 by upregulating the NF-κB/STAT3 signaling pathway, which induces epithelial transformation and promotes tumorigenesis as well as host immune escape. Lipopolysaccharide from Gram-negative bacilli activates the TLR4/MYD88 innate immune signaling pathway leading to an increase in IL23 and IL17, which promotes an inflammatory response and exacerbates tumor progression (27, 30). (iv) Symbiotic microbes normally play an important role in both innate and adaptive immunity. In contrast, microbial dysregulation inhibits T and NK cell activity and promotes dendritic cell (DC), Treg, and M2 macrophage recruitment, resulting in host immune tolerance and tumor evasion of immune surveillance (23, 31). (v) Microbial metabolites are also involved in cancer metabolism. For example, bacterial metabolism can produce the carcinogen acetaldehyde. Furthermore, cyanotoxin production by cyanobacteria increases procyclic acidic repetitive protein 1 (PARP1) which enhances inflammation and promotes lung cancer (23, 30).In contrast, short-chain fatty acids (SCFA) and secondary bile acids (SBC) affect host immunity and promote the production of the anti-inflammatory factor IL-10, thereby reducing the incidence of cancer (32, 33). Thus, it appears that dysregulation of microbial homeostasis and reduction of commensal microbes affect multiple mechanisms of lung carcinogenesis. Still up for debate, however, is the exact causative link between microbes and lung cancer due to the intricacy of their interaction. More research into the processes is expected to pave the way for the prediction and dynamic monitoring of lung cancer (Figure 1).
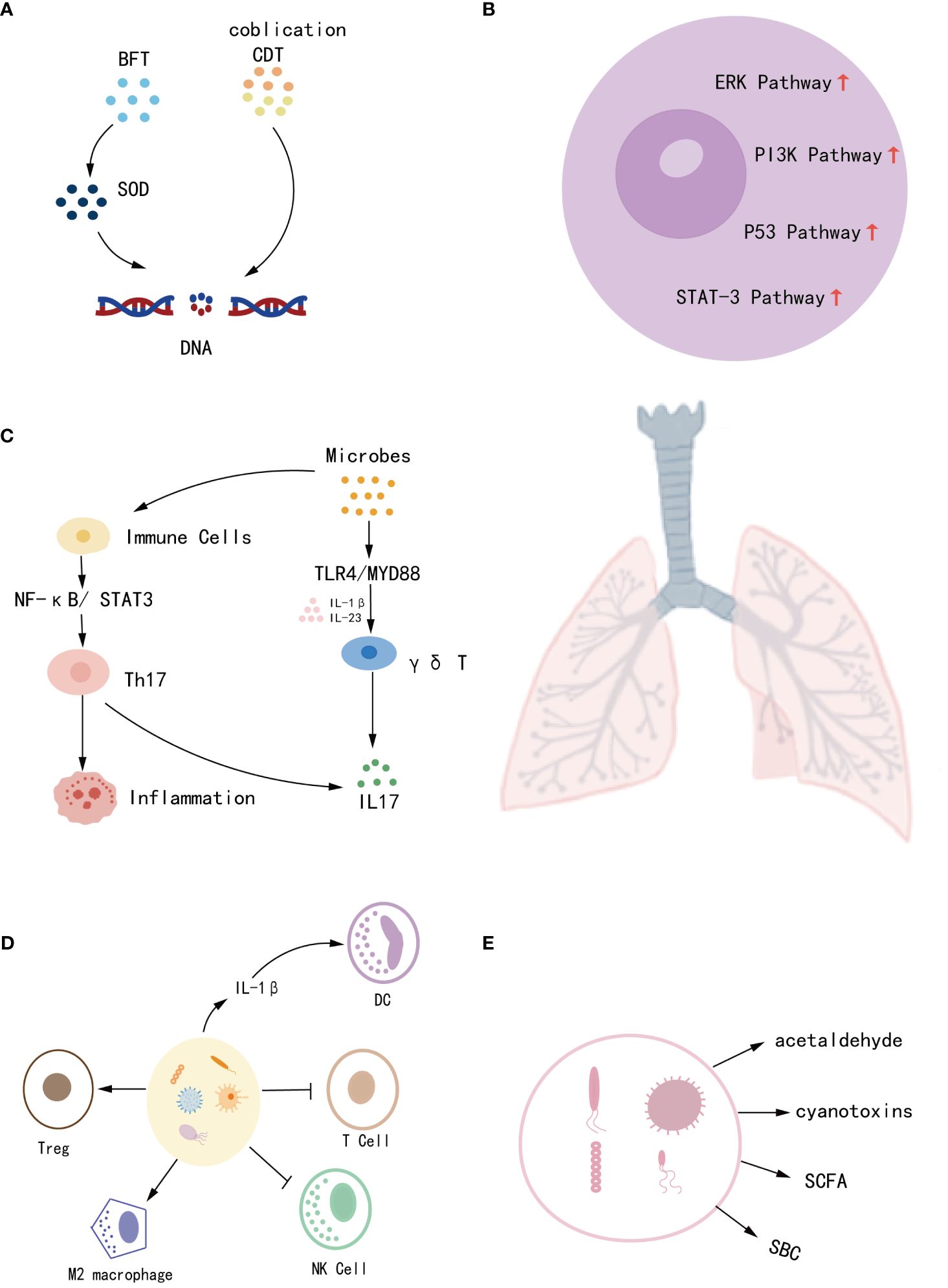
Figure 1 The mechanisms by which microbes contribute to the development of lung cancer include (A) disruption of host genes, (B) induction of chronic inflammation, (C) activation of oncogenic pathways, (D) influence on immune response, and (E) production of metabolites.
4 Methods of detecting microbes
Earlier, the detection of microbes was limited to the culture of patient specimens. Bacteria can be identified at the level of cultured genera, which has the advantage of economy and isolation of viable bacteria, however, there are also disadvantages such as long detection times, fewer detectable species, and susceptibility to contamination (34). NGS technology further detects microbes in normal human lungs by gene amplification. Depending on the species detected, it is classified into 16S rRNA and18S rRNA. 16S rRNA, currently used for diagnosing bacteria and archaea, is the most commonly used and advanced technique (35). It sequences highly conserved sequences of microbes and subsequently performs operational taxonomic units (OTUs) classification (OTUs refer to microbes undergoing 16rRNA sequencing, and microbes that have 97% similarity in the rRNA sequences were classified and distinguished by a classifier algorithm (36)). Nevertheless, the results are limited to the phylum and genus level and do not provide a more specific detection (37). Whole-genome sequencing (WGS) allows for comprehensive classification and higher specificity of microbes by sequencing the genome with random primers. However, it has the disadvantage of being expensive and requiring the processing of large amounts of downstream data (38). Despite advances in microbial detection, sequencing tools are less sensitive to TB than traditional microbial cultures. Hence, combining sequencing with culture is a better assay that increases both the sensitivity of the assay and the abundance of functional microbes (39).
5 Sources of specimens of microbes
Microbiological detection of lung cancer has its unique advantages. This is because microbiological testing can be accomplished by collecting saliva, sputum, bronchoalveolar lavage fluid (BALF), bronchial epithelial brushings, and stool, which are noninvasive and convenient (Figure 2). However, different studies have shown different dominant genera in lung cancer patients, possibly due to interference from environmental factors, different collection methods and sample sources, and different selections of healthy controls (HC), etc. (10). We hypothesize whether a method could be devised to accurately control the type of sampling and reduce errors (25). We then explored the relationship between different sources of microbes and the diagnosis of lung cancer (Table 2). In addition, the differences between the microorganisms of different samples are demonstrated in Table 3.
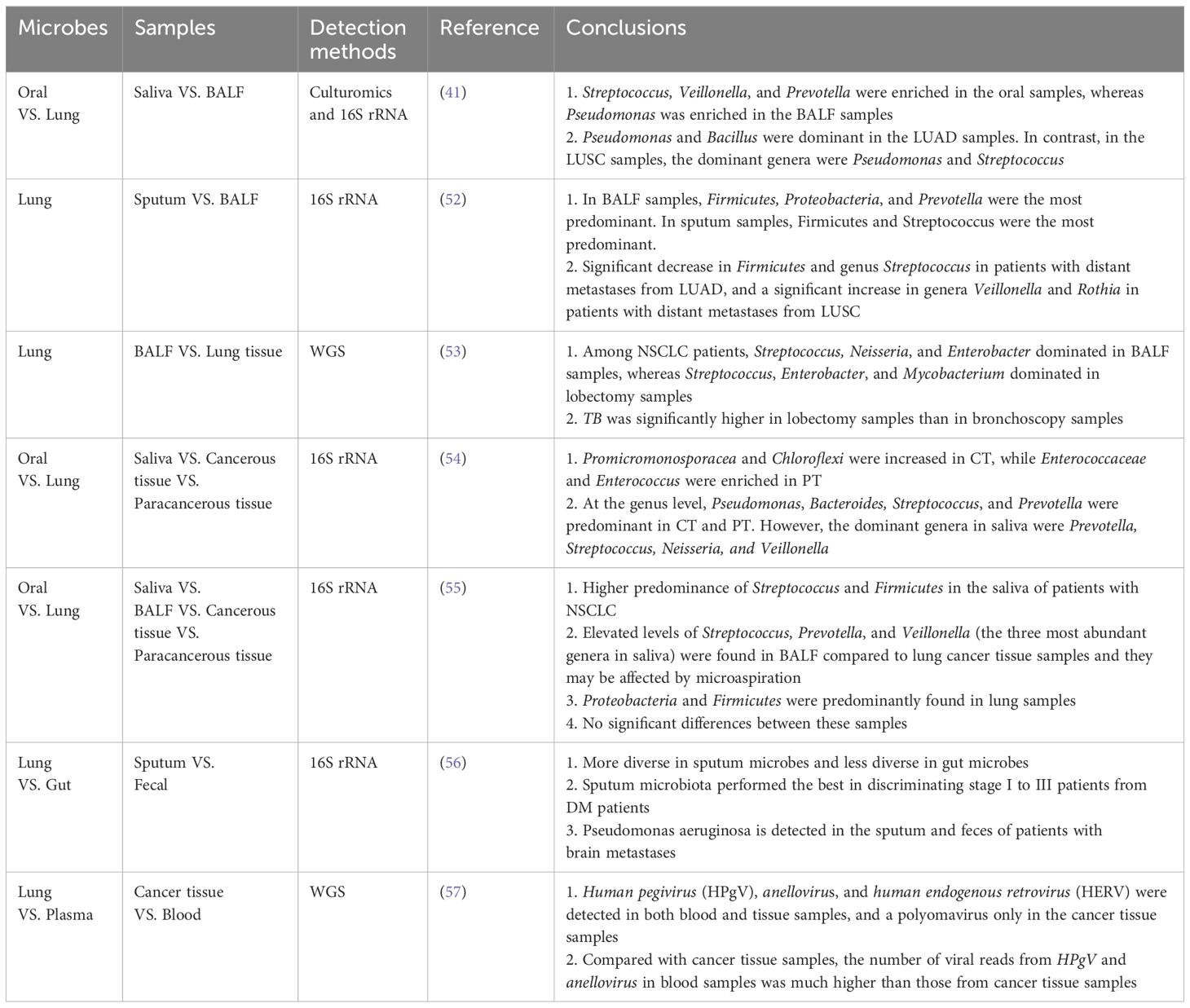
Table 3 Current literature on comparisons of differences between oral microbes, lung microbes, gut microbes, and plasma microbes.
5.1 Oral microbes
The oral microbiome is second only to the gut microbiome in complexity, and it is also a source of microbiome for the lungs. When oral and exogenous microbiomes enter the lungs with respiration and become colonized, they may cause chronic infections and modulate host immunity (58, 59). Yan et al. were the first to identify that the saliva of lung cancer patients was significantly different from HC in the genera Capnocytophaga and Veillonella and suggested that these two microbes might be potential markers for the detection of lung cancer (40). Sun et al. also stated that Streptococcus and Veillonella were predominant in both their oral and lung samples (41). A meta-analysis in 2016 concluded that patients with periodontal conditions had a 1.26-fold increased risk of developing lung cancer in comparison to HC (HR = 1.24, 95%CI = 1.13-1.36) (60). These findings can demonstrate the potential viability of oral microbes as diagnostic markers for lung cancer.
However, the use of oral microbes alone as detection markers is susceptible to interference by smoking, environmental factors, and other chronic diseases of the host. Consequently, the researchers considered the combination of other components of saliva, such as DNA, mRNA, and proteins, with microbes for multi-omics analysis. Wei et al. developed an electric field-induced release and measurement (EFIRM) technique, which was used to identify EGFR mutations in body fluids. Their clinical study showed that EFIRM detected exon 19 deletion and exon 21L858R mutation with AUCs of 0.94 and 0.96, respectively (61). According to Zhang et al., a logistic regression model with an AUC value of 0.94 and a sensitivity of 82.81% that included five mRNA biomarkers (CCNI, EGFR, FGF19, FRS2, and GREB1) could distinguish between lung cancer patients and controls (62). Xiao et al. verified that the sensitivity of using protein levels such as haptoglobin, zinc-a-2-glycoprotein, and calreticulin for lung cancer differentiation was 88.5%, and the specificity was 92.3% (63). Liu et al. indicated that multi-omics analysis of saliva explains molecular interactions and facilitates causal inference. However, saliva’s standardized collection and analysis techniques need to be further improved (64).
5.2 Lower respiratory tract and lung microbes
In the past, healthy lungs were regarded as sterile. However, it has been shown that the lungs contain their microbiota since the development of high-throughput sequencing technologies. These microbes originate from the air, oral cavity, and upper respiratory tract and are influenced by bacterial migration, clearance, and replication. They can be regulated to replicate by local microenvironmental states or cleared by host coughing, ciliary motility, and innate and adaptive immunity, culminating in a dynamic equilibrium state in the body (65). Despite low lung microbial populations, they are critical for host inflammation and immunity (66). Lung microbes in healthy individuals are predominantly in the Firmicutes and Bacteroides (67). Lung cancer, conversely, is associated with local microbial dysbiosis in the lungs, specifically an increase in the total microbiota and a decrease in diversity. Dong et al. demonstrated that Veillonella is significantly enriched in lung cancer and is the most strongly correlated potential marker for diagnosing lung cancer. Prevotella is also associated with lung cancer and acts synergistically with Veillonella can directly drive the PI3K/AKT (protein kinase B) and ERK/MAPK (extracellular signal-regulated kinase) signaling pathways, promoting lung cancer development (68, 69). Veillonella also promotes lung cancer development by inducing the recruitment of immune cells Th17 and neutrophils and by regulating tribes of microbiota, such as the aggregation of the pro-inflammatory bacterium Pseudomonas aeruginosa (10). In contrast, the lower respiratory tract is more relevant to the staging and subtyping of lung microbes than the oral and upper respiratory tracts, making it an ideal substitute for lung cancer microbes during collection (52). Among the collection methods are sputum, bronchoalveolar lavage fluid, and lung tissue biopsy.
Sputum is a more representative sample than oral fluid as it is produced from the lower respiratory tract’s bronchi and tiny bronchioles. It may serve as a substitute for gathering lung bacteria collection when there is no oral microbial contamination. Druzhinin et al. used 16SrRNA for sputum microbiological detection. A significant increase in the abundance of Haemophilus and Bergeyella and a decrease in the abundance of Atopobium were found in lung cancer patients (42). Leng et al. investigated sputum microbes using droplet digital PCR and showed that Acidovorax and Veillonella have complementary roles. Their combination showed an AUC of 0.91, a sensitivity of 80%, and a specificity of 89.26% for the detection of squamous lung carcinoma. Additionally, Acidovorax also plays a function in distinguishing LUAD from LUSC with a sensitivity of 63.64% and a specificity of 96.30% (43). The investigators found that sputum microbes had a higher diagnostic sensitivity for LUSC. This may be because sputum is mainly secreted by the large airways and main bronchial tubes and LUSC also originates in the central lung tissue, whereas LUAD originates in the peripheral lung tissue (43).
Bronchoalveolar lavage examination is also a means of early detection due to the difference in microbiological composition between HC and lung cancer individuals. LEE et al. found that Megasphaera and Veillonella were more prevalent in lung cancer. When predicting lung cancer, Veillonella had an AUC of 0.863, sensitivity of 65.0%, and specificity of 100.0%, while Megasphaera had an AUC of 0.781, sensitivity of 75.0%, and specificity of 25.0%. Whereas the joint prediction of these two genera could show a higher AUC (0.888), their correlation needs further investigation (39). Subsequently, Marshall et al. constructed linear discriminant analysis (LDA) scores using the lung microbiome predictor of cancer (LMPC) to distinguish cancer status and predict cancer. They found that people with higher LDA scores had earlier onset of disease. However, this study focused only on the smoking cohort, and the absolute risk model was consistent with the incidence of cancer in ‘high-risk’ individuals. This suggests that LDA scores may be more applicable to high-risk individuals (45).
In addition, the finding that bacteria exist in tumors dates back more than a century. More in-depth studies were not carried out as contamination of tumor samples could not be ruled out (70). In 2011, Apostolou et al. found the presence of Mycoplasma in 32 resected lung cancer samples, and they proposed that chronic infection of the lungs is an important cause of tumorigenesis (46). Until 2020 Nejman et al. found that most tumors and surrounding normal tissues contained different types of bacteria and that these were predominantly identified in cancer and immune cells (71). Liu et al. subsequently found that the abundance of Proteobacteria (mainly Acinetobacter and Acidobacterium) was significantly lower in lung cancer patients by sequencing lung tissues and that the prevalence of Phylum Firmicutes (Streptococcus) and Bacteroidetes (Prevotella) was higher (47). Overall, sources of intratumoral microbes include microbial infiltration due to disruption of the mucosal barrier, microbial infiltration of adjacent tissues, and microbial transfer from the oral cavity and intestines (27). Intratumoral microbes can change the tumor microenvironment to affect the growth and metastasis of tumor cells. Still, the microbial abundance within the tumor is low and contamination during collection should be strictly avoided. In addition, the relationship between tumor and microbial action is unclear (active or passive? symbiotic or parasitic)?, and lung tissue biopsy specimens are not readily available. Researchers tend to use bronchoscopy or sputum specimens (70).
5.3 Gut microbes
Intestinal flora is a general term for the tribe of microbes in the gastrointestinal tract, accounting for 99% of the commensal bacteria in the human body. It aids in the breakdown and digestion of food, maintains normal physiological and immune functions of the intestinal tract, as well as the production of nutrients (25). Anaerobic bacteria are the main components of gut microbes and are also the main producers the leading producers of SCFA, butyric acid, in the human body. Possible reasons for some similarities between lung and gut microbes are: (i) The lungs and intestines are commensurate in embryonic origin, and the same mucous membrane covers them. (ii) Microbes inhaled by the mouth can enter the lungs and intestines. Bacteria from the digestive tract may crosstalk with respiratory microbes as a result of reflux (24). (iii) The channel through which the lung interacts with the intestine through blood vessels and lymphatics is known as the lung-gut axis (25). Through this axis, microbes that generate a local immunomodulatory response to the body also elicit a systemic response. In addition, ectopic intestinal flora and metabolites (such as SCFA) can elicit extraintestinal organ-specific immune responses (72). Due to the crosstalk between lung and intestinal microbes, it has been shown that gut microbial dysbiosis is highly related to lung disorders, including chronic obstructive pulmonary disease, asthma, pulmonary hypertension, and lung cancer (71).
The initial recommendation by Shen et al. was that lung cancer patients had higher absolute and relative abundances of Haemophilus and Streptococcus, which could serve as potential indicators for lung cancer detection. However, additional validation is required before these findings can be applied in clinical settings (48). Considering the complexity of microbial-host interactions and the fact that differences in microbes alone may not explain the role of microbes in cancer (73), Vernocchi, as well as Ni et al., proposed the use of combined microbe-metabolites for detection using multi-omics. Ni pointed out that Agathobacter and Blaudia are the main differential genera of early-stage NCLC. In contrast, metabolites such as serum phospholipids and fatty acids can regulate tumor cells’ growth, proliferation, and metastasis, and their combination with microbes could provide new ideas for lung cancer detection (49). Additionally, Zheng et al. used machine learning SVM to select 13 microbial markers based on OTUs for predicting the status and occurrence of diseases (36). However, Lim et al. indicated that fewer gut microbes were associated with lung cancer compared to oral microbes and that the gut microbes had limited predictive performance when machine learning was used to construct predictive models of non-smoking patient status (oral microbiome AUC 0.95 vs. gut microbiome AUC 0.76) (74). This may be related to oral microbes migrating to the lungs. Multiple researchers have also suggested that lower respiratory tract and lung microbes may be more associated with the development of lung cancer than gut microbes, which is more conducive to lung cancer diagnosis (37, 56).
5.4 Plasma microbes
Many microbes are present in healthy blood, and plasma microbial cell-free DNA (mcfDNA) may be diagnostic markers for liquid biopsies in lung cancer patients, according to recent studies (38, 75). In gastrointestinal tumors such as gastric, hepatic, and colorectal cancers, mcfDNA was found to have the ability to distinguish normal subjects from cancer patients (38). Subsequently, Poore et al. found that mcfDNA can also have good diagnostic ability in lung cancer through WGS combined with machine learning models (50). Zhou et al. showed a higher DNA abundance of Selenomonas, Streptococcus, and Veillonella in the circulating blood of lung cancer patients compared to HC, and the combined lung cancer detection of the three genera had a sensitivity of 75% and a specificity of 78% (76). Chen et al. proposed that the combination of human circulating free RNA (cfRNA) with the microbial cfRNA detection of lung cancer had an 8% increase in mean recall (the number of positive samples detected as a proportion of the actual number of positive samples) over human cfRNA detection alone (51).
In conclusion, the exploration of microbes has progressed from a high number of oral and gut microbes to a smaller and more refined number of intra-tumor microbes and plasma microbes. The goal of our study is to aspire to a balance between noninvasive and accurate detection.
6 Collection of microbes from different sources
6.1 Saliva
Subjects avoid brushing their teeth, eating, drinking, or chewing gum for at least 1 hour before saliva collection (77). Saliva collection is generally categorized as mouthwash, irritant saliva, and non-irritant saliva (78). Studies have shown that the microbial diversity of microbes collected by mouthwash does not differ from those collected by drooling (79). Still, mouthwash is more favorable for patients with dry mouths and takes less time (80). Since ethanol-free mouthwash is more favorable for sample transport, subjects were asked to rinse their mouths with 0.9% saline for approximately 30 seconds (81). For preservation, saliva samples should be centrifuged and the supernatant should be removed after collection at 4°C. The cellular precipitate is resuspended in sterile phosphate-buffered saline (PBS) and stored at -80°C. If cryogenic storage is not possible, the samples can also be made more stable by the addition of a preservative to make the cellular DNA more stable (82).
6.2 Sputum
When collecting sputum, subjects are required to blow their noses and rinse their mouths in advance as a means of minimizing the effects of postnasal drip and oral microbes (43). Typically, they are asked to cough up sputum spontaneously. When this is unsuccessful, participants are induced to undergo a 20-minute sputum nebulization with 3% saline solution (83), or sputum sampling using a lung flute (84). Sputum nebulization is time-consuming, and carries the risk of bronchospasm and respiratory infections, thus limiting its clinical use. The lung flute is a sound wave with a frequency of 18-22 Hz generated through exhalation, which enhances the mucus clearance system in the airways, making mucus secretions thinner and easier to expel through coughing (84). Sputum samples were collected in sterile containers and processed within 2 hours. Then, the specimens were selected microscopically by the investigator as a way to minimize contamination with oral squamous cells (43). Samples were treated on ice with 4 volumes of 0.1% dithiothreitol and 4 volumes of PBS, after which the samples were centrifuged and the supernatant removed. Finally, the remaining cell sediment was stored at -80°C until use (43, 85).
6.3 Bronchoalveolar lavage fluid
Subjects first received local anesthesia with lidocaine, followed by sedation with midazolam and fentanyl. Bronchial tubes were washed with 30-50 ml of sterile 0.9% physiological saline (39, 52), after which 3 ml of BALF was collected from each patient. The supernatant was removed by centrifugation at 4°C, 1 ml of DNA/RNA shielding was added, and the samples were finally stored in centrifuge tubes at -80°C (86).
6.4 Lung tissue
The most important aspect is to ensure proper sampling. Lung tissue was taken from the tumor, the area adjacent to the tumor, or an additional area away from the tumor (1-5 cm), as required. Surgically resected or punctured lung tissue samples were rapidly frozen in liquid nitrogen within 20 minutes and stored at -80°C until use (87).
6.5 Stool
The prerequisite for fecal collection is that subjects are asked to be free of antibiotic and glucocorticoid therapy within 3 months of specimen collection (88). Since the gut is mostly populated by anaerobic microbes, anaerobic fecal collection devices preserve as much microbial diversity as possible compared to conventional fecal collection devices (89). In addition, a fresh rectal swab can be used instead when the critical patient is unable to provide a fecal sample. However, the time to process the swab must be less than or equal to 2 days, after which time the quality of the sample will deteriorate rapidly (90). Stools and swabs should be frozen at -80°C immediately after collection and transported to the laboratory using dry ice (88). The fecal microbiome will remain stable at -80°C for up to two years with the addition of RNAlater, 95% ethanol, or preservation using FIT tubes and FOBT cards (91). If samples cannot be stored at -80°C, sample storage solutions such as Bgene-Gut, OMNIgene-Gut or RNAlater can be added to help preserve the microbiome as well (92).
6.6 Circulate blood
3-5 mL of the subject’s blood was obtained by venipuncture through aseptic manipulation and stored in K2EDTA tubes at 4°C (93). Cells were removed by centrifugation within 24 hours of blood collection. Finally, the plasma obtained after isolation was stored at -80°C for a long period (93) (Figure 3).
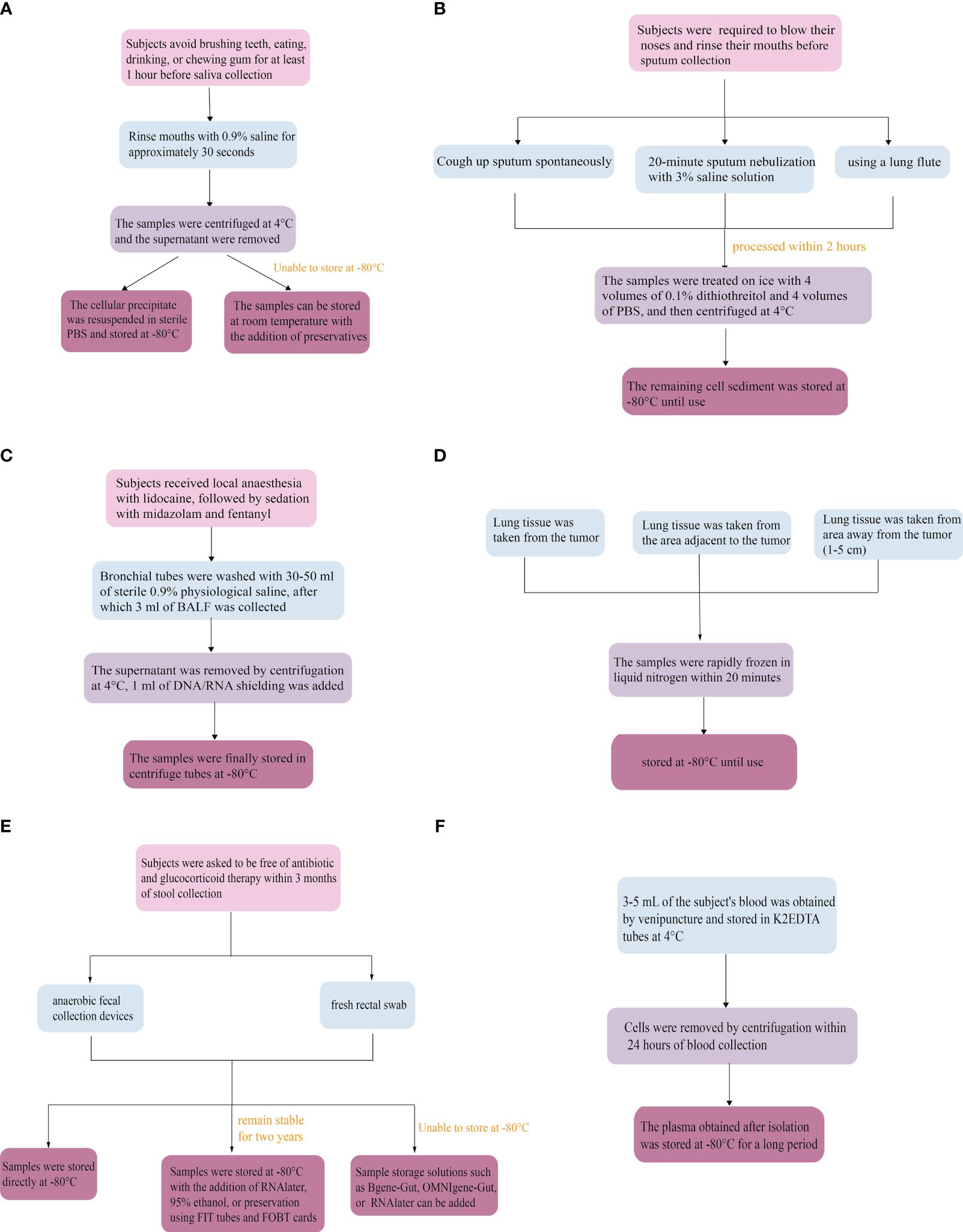
Figure 3 Microbiological collection methods. (A) Saliva, (B) Sputum, (C) Bronchoalveolar lavage fluid, (D) Lung tissue, (E) Stool, (F) Circulate blood.
7 Relationship between microbes and lung cancer typing and staging
Since microbes play an important role in the development of lung cancer by interacting with the host, we speculated whether microbes are heterogeneous and dynamically change on different pathological types and stages of lung cancer (94). Tasy et al. showed that there was a significant difference in β-diversity (diversity between different groups) between lower airway microbes of NSCLC and SCLC patients, which may be related to the different localization of the tumors. Furthermore, compared with stage I-IIIA NSCLC patients, the lower airways of stage IIIB-IV NSCLC patients were enriched with many oral commensals such as Haemophilus, Fusobacterium, Gemella, Prevotella, and Granulicatella. This may be due to the role of airway suction in causing microbial dysbiosis resulting in local inflammation and immunity, promoting NSCLC progression (95). In addition, Lu et al. demonstrated a significant increase in Pseudomonas aeruginosa abundance in sputum from NCLC patients with brain metastases (stage IV) by WGS (56). Yu et al. showed that Thermus was more abundant in patients with advanced lung cancer (stage IIIB-IV) and Legionella in patients with metastatic lung cancer (87). Further study by Huang et al. indicated that Veillonella and Rothia were increased in patients with LUSC metastasis (M1), whereas Firmicutes and Streptococcus were increased in LUAD distant metastasis. It may be because of microbial changes due to altered molecular biology during tumor metastasis (52). Li et al. combined microbes and mRNAs to construct a model for distinguishing lung cancer patients undergoing early (I) and intermediate to late (II-IV) stages using the Random Forest algorithm. The model had a prediction rate of 0.809 (96). From the above studies, we found that the dominant microbial species were different in patients with different histologic types and different stages. However, the veracity of the studies is limited by many factors such as the low incidence of SCLC, small sample size, and limited current studies. As well as most of the studies were only cross-sectional difference studies without dynamic monitoring (97). Therefore, microbes for typing and staging detection of lung cancer need to be further investigated.
8 Current problems with the use of microbes for the detection
8.1 Differences in individual samples
There is a complex symbiotic relationship between microbes and their hosts, leading to their influence by endogenous and exogenous factors in the human body. Endogenous factors include recombination, mutation, and amplification of genes, while exogenous factors include diet, sleep, exercise, chronic diseases, and drug use. Of these, antibiotics, chemotherapeutic drugs, and gastrointestinal infections have the greatest impact on microbes (98). How much these factors affect the composition of microbes and how we can analyze the data uniformly is open to further discussion (98).
Then, it is also well recognized that exposure to tobacco smoke increases the risk of lung cancer; nevertheless, a certain percentage of lung cancer cases also occur among non-smokers. Hence, some scholars also believe that non-smoking lung cancer belongs to “another type” of lung cancer (99). Smokers have reduced microbial diversity in the lungs, and the biology is enriched in the metabolic pathway of cigarettes (24). In contrast, non-smoking lung cancer patients have higher microbiological diversity, which may be related to chronic bacterial infections of the lungs (16). Therefore, their differences need to be considered when utilizing microbes for detection.
8.2 Differences in sample collection
Anaerobic bacteria dominate oral microbiology and intestinal microbiology, while aerobic bacteria dominate lower respiratory tract and lung microbiology. Therefore, different collection methods are required for different sources of microbes. In addition, when performing joint multi-omics analyses, the content and type of DNA, RNA, and proteins are also closely related to the collection method (64). For example, when collecting oral microbes, individual salivary glands have fewer non-salivary components, such as shed epithelium and food debris, making them more suitable for oral multi-omics analysis. In contrast, whole salivary glands are more suitable for functional analysis of saliva (64). We need to choose the most appropriate collection method according to different samples.
8.3 Limitations of microbes
A large number of studies and experiments have shown differences in the composition and number of microbes between tumor patients and normal subjects, and have explained that microbes are associated with lung cancer in a variety of ways. However, most of the clinical trials suffer from small sample sizes and researchers emphasize that there is no direct evidence of the exact role of microbes in carcinogenesis. Microbes are thought to promote cancer but not directly cause it (41). Sears et al. also stated that microbial detection is only valid when there is clinical suspicion of a tumor that current tests cannot diagnose. In the absence of confirmation of the location of the tumor, the test needs to be obtained from other specimens such as blood and urine (11). In addition, the role of less abundant microbes such as viruses and fungi in cancer has been neglected due to the limitations of the current level of microbial detection. The complex mechanisms of microbial action and causality in cancer have also not been investigated for the time being (37). Considering that there is no evidence of a direct role of microbes in cancer, it is not feasible to use differences between microbes from different patients to detect cancer alone. Therefore, it is necessary to combine other samples for testing (11).
9 Conclusion and discussion
Current diagnostic and screening tools for lung cancer are still limited. LDCT is radioactive and false-positive, while the biopsy is invasive and costly, so we need more convenient and accurate indicators. Many studies have shown that microbial responses to the host are bidirectional and that there are interactions between commensal microbes, host immune responses as well as lung cancer microecology (13). On the one hand, microbial dysbiosis promotes the development of cancer, and in animal experiments, antibiotic-administered mice are ineffective for radiotherapy and immunotherapy (100). On the other hand, we can artificially boost the beneficial bacteria to promote the efficacy of anti-tumor immunity and chemotherapeutic drugs and minimize immune-related adverse reactions (25). Additionally, we can restore the microbial ecology to support tumor treatment through dietary modification, oral probiotics, or fecal transplantation (101).In this paper, we summarize the mechanisms and methods of microbial lung cancer detection in recent years and further explore their feasibility and limitations. Firstly, we found that microbes can promote cancer progression by causing genetic damage to the host, affecting immunity, leading to chronic inflammation, and influencing metabolite. Second, lower respiratory tract microbes, lung microbes, and blood microbes can respond to the progression of cancer and are more favorable for early detection than oral microbes and intestinal microbes. Third, microbes can be detected in culture and non-culture. In the future, the combination of culture and sequencing (especially WGS) will be more accurate for detecting microbes in lung cancer. Moreover, some researchers have suggested that WGS needs to be based on a high microbial nucleic acid load basis. In contrast, macro-transcriptomics, macro-proteomics, and metabolomics can describe the downstream functions and metabolism of the active microbiota. Multi-omics analyses can be used to further explain microbe-host interactions (34, 102). Fourth, because of the complexity of the connection between microbes and their hosts, characterizing intergroup variations alone is insufficient to explain the function of microbes in different stages and types of lung cancer. Consequently, combining various biomarkers is required to perform precise diagnostic assessments, as well as multi-sample dynamic detection.
In addition, different experimental studies have yielded different species of dominant microbes in lung cancer patients, but there are some microbes and the effects they produce that have been repeatedly mentioned. These are precisely the focus of our subsequent studies (10). For example, Veillonella is potentially similar to Prevotella in up-regulating the ERK and PI3K signaling pathways to exert oncogenic effects (69). Streptococcus can induce chronic lung inflammation by upregulating pathways as well, but the exact role needs to be further determined. Acidovorax has a remodeling effect on the immune microenvironment of tumor cells, whereas Haemophilus and Capnocytophaga may be opportunistic pathogens, resulting from an impaired immune system.
Other biomarkers can be used in combination with microbes for detection. Liu et al. found that lung cancer patients grouped according to different serological markers also behaved differently regarding gut microbiology (103). Chen et al. used WGS to combine Klebsiella, Mycobacterium, Pedobacter, Prevotella, Xanthomonas, and NSE in alveolar lavage fluid for the detection of lung cancer, with an AUC value of 0.959, a specificity of 85.7% and a sensitivity of 100% (104). Various metabolites such as sphingomyelin and cysteinyl-valine were statistically different between lung cancer and normal samples (105). Chen et al. showed that L-valine and Lachnospiraceae_UCG-006 mutually regulate with each other through biological pathways (106). Ni et al. also indicated that Agathobacter and Blaudia are closely related to serum glycerophospholipid and sphingolipid lipid metabolism. They are all potential markers for the combined multi-omics detection of lung cancer (49).
Although microbes can be used as potential biomarkers for detection, there are many challenges in their practical use, with standardization being key to clinical use. It is important to note that the microbe is not used alone for lung cancer detection, but rather it is integrated into other markers of lung cancer for standardized procedural diagnosis. Future research will require longitudinal refinement of specimen collection methods, testing tools, and the incorporation of machine learning to build more accurate diagnostic models. For example, with the help of computers, efforts should be made to decontaminate, normalize data, and classify and analyze experimental specimens. Comparative analyses of oral, pulmonary, intestinal, and blood microbes and multi-omics analysis of microbes and gene-protein metabolites are needed for cross-sectional analysis. Finally, large-scale, multi-sample clinical validation is needed to perform. In addition, we also expect that the new methods can more accurately diagnose “harmful microbes” to provide clues for tumor staging and dynamic detection of microbial changes, which will allow for a dynamic view of the disease.
Author contributions
SZ: Data curation, Writing – original draft. WZ: Software, Visualization, Writing – review & editing. HG: Data curation, Investigation, Validation, Writing – review & editing. YN: Formal analysis, Methodology, Project administration, Writing – review & editing. JS: Data curation, Project administration, Supervision, Validation, Writing – review & editing. PL: Funding acquisition, Resources, Writing – review & editing. YZ: Funding acquisition, Resources, Supervision, Writing – review & editing.
Funding
The author(s) declare that no financial support was received for the research, authorship, and/or publication of this article.
Acknowledgments
The authors are grateful to all peers who provided their valuable support.
Conflict of interest
The authors declare that the research was conducted in the absence of any commercial or financial relationships that could be construed as a potential conflict of interest.
Publisher’s note
All claims expressed in this article are solely those of the authors and do not necessarily represent those of their affiliated organizations, or those of the publisher, the editors and the reviewers. Any product that may be evaluated in this article, or claim that may be made by its manufacturer, is not guaranteed or endorsed by the publisher.
References
1. Allemani C, Matsuda T, Di Carlo V, Harewood R, Matz M, Nikšić M, et al. Global surveillance of trends in cancer survival 2000-14 (CONCORD-3): analysis of individual records for 37 513 025 patients diagnosed with one of 18 cancers from 322 population-based registries in 71 countries. Lancet. (2018) 391:1023–75. doi: 10.1016/S0140-6736(17)33326-3
2. Zhang M, Liu D, Zhou H, Liu X, Li X, Cheng Y, et al. Intestinal flora characteristics of advanced non-small cell lung cancer in China and their role in chemotherapy based on metagenomics: A prospective exploratory cohort study. Thorac Cancer. (2021) 12:3293–303. doi: 10.1111/1759-7714.14199
3. Thompson NA, Stewart GD, Welsh SJ, Doherty GJ, Robinson MJ, Neville BA, et al. The MITRE trial protocol: a study to evaluate the microbiome as a biomarker of efficacy and toxicity in cancer patients receiving immune checkpoint inhibitor therapy. BMC Cancer. (2022) 22:99. doi: 10.1186/s12885-021-09156-x
4. Huynh M, Crane MJ, Jamieson AM. The lung, the niche, and the microbe: Exploring the lung microbiome in cancer and immunity. Front Immunol. (2023) 13:1094110. doi: 10.3389/fimmu.2022.1094110
5. Lee EK Ella A. Lung cancer screening. Semin Respir Crit Care Med. (2022) 43:839–50. doi: 10.1055/s-0042-1757885
6. Guan Y, Ren M, Guo D, He Y. Research progress on lung cancer screening. Chin J Lung Cancer. (2020) 23:954–60. doi: 10.3779/j.issn.1009-3419.2020.101.37
7. Nooreldeen R, Bach H. Current and future development in lung cancer diagnosis. Int J Mol Sci. (2021) 22:8661. doi: 10.3390/ijms22168661
8. Mohamed E, García Martínez DJ, Hosseini M-S, Yoong SQ, Fletcher D, Hart S, et al. Identification of biomarkers for the early detection of non-small cell lung cancer: a systematic review and meta-analysis. Carcinogenesis. (2024) 45:1–22. doi: 10.1093/carcin/bgad091
9. Ning J, Ge T, Jiang M, Jia K, Wang L, Li W, et al. Early diagnosis of lung cancer: which is the optimal choice? Aging (Albany NY). (2021) 13:6214–27. doi: 10.18632/aging.202504
10. Huang J, Huang J. Microbial biomarkers for lung cancer: current understandings and limitations. J Clin Med. (2022) 11:7298. doi: 10.3390/jcm11247298
11. Sears CL, Salzberg SL. Microbial diagnostics for cancer: A step forward but not prime time yet. Cancer Cell. (2020) 37:625–7. doi: 10.1016/j.ccell.2020.04.010
12. Rastogi S, Mohanty S, Sharma S, Tripathi P. Possible role of gut microbes and host’s immune response in gut–lung homeostasis. Front Immunol. (2022) 13:954339. doi: 10.3389/fimmu.2022.954339
13. Bredin P, Naidoo J. The gut microbiome, immune check point inhibition and immune-related adverse events in non-small cell lung cancer. Cancer Metastasis Rev. (2022) 41:347–66. doi: 10.1007/s10555-022-10039-1
14. de Martel C, Ferlay J, Franceschi S, Vignat J, Bray F, Forman D, et al. Global burden of cancers attributable to infections in 2008: a review and synthetic analysis. Lancet Oncol. (2012) 13:607–15. doi: 10.1016/S1470-2045(12)70137-7
15. de Martel C, Georges D, Bray F, Ferlay J, Clifford GM. Global burden of cancer attributable to infections in 2018: a worldwide incidence analysis. Lancet Glob Health. (2020) 8:e180–90. doi: 10.1016/S2214-109X(19)30488-7
16. Sepich-Poore GD, Zitvogel L, Straussman R, Hasty J, Wargo JA, Knight R. The microbiome and human cancer. Science. (2021) 371:eabc4552. doi: 10.1126/science.abc4552
17. Wong CR, Nguyen MH, Lim JK. Hepatocellular carcinoma in patients with non-alcoholic fatty liver disease. World J Gastroenterol. (2016) 22:8294–303. doi: 10.3748/wjg.v22.i37.8294
18. Wu S, Rhee K-J, Albesiano E, Rabizadeh S, Wu X, Yen H-R, et al. A human colonic commensal promotes colon tumorigenesis via activation of T helper type 17 T cell responses. Nat Med. (2009) 15:1016–22. doi: 10.1038/nm.2015
19. Arthur JC, Perez-Chanona E, Mühlbauer M, Tomkovich S, Uronis JM, Fan T-J, et al. Intestinal inflammation targets cancer-inducing activity of the microbiota. Science. (2012) 338:120–3. doi: 10.1126/science.1224820
20. Zhuo W-L, Zhu B, Xiang Z-L, Zhuo X-L, Cai L, Chen Z-T. Assessment of the relationship between Helicobacter pylori and lung cancer: a meta-analysis. Arch Med Res. (2009) 40:406–10. doi: 10.1016/j.arcmed.2009.05.002
21. Kocazeybek B. Chronic Chlamydophila pneumoniae infection in lung cancer, a risk factor: a case-control study. J Med Microbiol. (2003) 52:721–6. doi: 10.1099/jmm.0.04845-0
22. Liang H-Y, Li X-L, Yu X-S, Guan P, Yin Z-H, He Q-C, et al. Facts and fiction of the relationship between preexisting tuberculosis and lung cancer risk: A systematic review. Int J Cancer. (2009) 125:2936–44. doi: 10.1002/ijc.24636
23. Mao Q, Jiang F, Yin R, Wang J, Xia W, Dong G, et al. Interplay between the lung microbiome and lung cancer. Cancer Lett. (2018) 415:40–8. doi: 10.1016/j.canlet.2017.11.036
24. Pizzo F, Maroccia Z, Hammarberg Ferri I, Fiorentini C. Role of the microbiota in lung cancer: insights on prevention and treatment. Int J Mol Sci. (2022) 23:6138. doi: 10.3390/ijms23116138
25. Zhao Y, Liu Y, Li S, Peng Z, Liu X, Chen J, et al. Role of lung and gut microbiota on lung cancer pathogenesis. J Cancer Res Clin Oncol. (2021) 147:2177–86. doi: 10.1007/s00432-021-03644-0
26. Druzhinin VG, Matskova LV, Fucic A. Induction and modulation of genotoxicity by the bacteriome in mammals. Mutat Res Rev Mutat Res. (2018) 776:70–7. doi: 10.1016/j.mrrev.2018.04.002
27. Liu W, Xu J, Pi Z, Chen Y, Jiang G, Wan Y, et al. Untangling the web of intratumor microbiota in lung cancer. Biochim Biophys Acta (BBA) - Rev Cancer. (2023) 1878:189025. doi: 10.1016/j.bbcan.2023.189025
28. Boesch M, Horvath L, Baty F, Pircher A, Wolf D, Spahn S, et al. Compartmentalization of the host microbiome: how tumor microbiota shapes checkpoint immunotherapy outcome and offers therapeutic prospects. J Immunother Cancer. (2022) 10:e005401. doi: 10.1136/jitc-2022-005401
29. Meng Y-F, Fan Z-Y, Zhou B, Zhan H-X. Role of the intratumoral microbiome in tumor progression and therapeutics implications. Biochim Biophys Acta (BBA) - Rev Cancer. (2023) 1878:189014. doi: 10.1016/j.bbcan.2023.189014
30. Xu N, Wang L, Li C, Ding C, Li C, Fan W, et al. Microbiota dysbiosis in lung cancer: evidence of association and potential mechanisms. Transl Lung Cancer Res. (2020) 9:1554–68. doi: 10.21037/tlcr-20-156
31. McLean AEB, Kao SC, Barnes DJ, Wong KKH, Scolyer RA, Cooper WA, et al. The emerging role of the lung microbiome and its importance in non-small cell lung cancer diagnosis and treatment. Lung Cancer. (2022) 165:124–32. doi: 10.1016/j.lungcan.2022.01.011
32. Nicholson JK, Holmes E, Kinross J, Burcelin R, Gibson G, Jia W, et al. Host-gut microbiota metabolic interactions. Science. (2012) 336:1262–7. doi: 10.1126/science.1223813
33. Campbell C, Kandalgaonkar MR, Golonka RM, Yeoh BS, Vijay-Kumar M, Saha P. Crosstalk between gut microbiota and host immunity: impact on inflammation and immunotherapy. Biomedicines. (2023) 11(2):294. doi: 10.3390/biomedicines11020294
34. Ojala T, Kankuri E, Kankainen M. Understanding human health through metatranscriptomics. Trends Mol Med. (2023) 29:376–89. doi: 10.1016/j.molmed.2023.02.002
35. Ranjan R, Rani A, Metwally A, McGee HS, Perkins DL. Analysis of the microbiome: Advantages of whole genome shotgun versus 16S amplicon sequencing. Biochem Biophys Res Commun. (2016) 469:967–77. doi: 10.1016/j.bbrc.2015.12.083
36. Zheng Y, Fang Z, Xue Y, Zhang J, Zhu J, Gao R, et al. Specific gut microbiome signature predicts the early-stage lung cancer. Gut Microbes. (2020) 11:1030–42. doi: 10.1080/19490976.2020.1737487
37. Ramírez-Labrada AG, Isla D, Artal A, Arias M, Rezusta A, Pardo J, et al. The influence of lung microbiota on lung carcinogenesis, immunity, and immunotherapy. Trends Cancer. (2020) 6:86–97. doi: 10.1016/j.trecan.2019.12.007
38. Chen H, Ma Y, Liu Z, Li J, Li X, Yang F, et al. Circulating microbiome DNA: An emerging paradigm for cancer liquid biopsy. Cancer Lett. (2021) 521:82–7. doi: 10.1016/j.canlet.2021.08.036
39. Lee SH, Sung JY, Yong D, Chun J, Kim SY, Song JH, et al. Characterization of microbiome in bronchoalveolar lavage fluid of patients with lung cancer comparing with benign mass like lesions. Lung Cancer. (2016) 102:89–95. doi: 10.1016/j.lungcan.2016.10.016
40. Yan X, Yang M, Liu J, Gao R, Hu J, Li J, et al. Discovery and validation of potential bacterial biomarkers for lung cancer. Am J Cancer Res. (2015) 5:3111–22.
41. Sun Y, Liu Y, Li J, Tan Y, An T, Zhuo M, et al. Characterization of lung and oral microbiomes in lung cancer patients using culturomics and 16S rRNA gene sequencing. Microbiol Spectr. (2023) 11:e0031423. doi: 10.1128/spectrum.00314-23
42. Druzhinin VG, Matskova LV, Demenkov PS, Baranova ED, Volobaev VP, Minina VI, et al. Taxonomic diversity of sputum microbiome in lung cancer patients and its relationship with chromosomal aberrations in blood lymphocytes. Sci Rep. (2020) 10:9681. doi: 10.1038/s41598-020-66654-x
43. Leng Q, Holden VK, Deepak J, Todd NW, Jiang F. Microbiota biomarkers for lung cancer. Diagnostics (Basel). (2021) 11:407. doi: 10.3390/diagnostics11030407
44. Gomes S, Cavadas B, Ferreira JC, Marques PI, Monteiro C, Sucena M, et al. Profiling of lung microbiota discloses differences in adenocarcinoma and squamous cell carcinoma. Sci Rep. (2019) 9:12838. doi: 10.1038/s41598-019-49195-w
45. Marshall EA, Filho FSL, Sin DD, Lam S, Leung JM, Lam WL. Distinct bronchial microbiome precedes clinical diagnosis of lung cancer. Mol Cancer. (2022) 21:68. doi: 10.1186/s12943-022-01544-6
46. Apostolou P, Tsantsaridou A, Papasotiriou I, Toloudi M, Chatziioannou M, Giamouzis G. Bacterial and fungal microflora in surgically removed lung cancer samples. J Cardiothorac Surg. (2011) 6:137. doi: 10.1186/1749-8090-6-137
47. Liu Y, O’Brien JL, Ajami NJ, Scheurer ME, Amirian ES, Armstrong G, et al. Lung tissue microbial profile in lung cancer is distinct from emphysema. Am J Cancer Res. (2018) 8:1775–87.
48. Shen W, Tang D, Deng Y, Li H, Wang T, Wan P, et al. Association of gut microbiomes with lung and esophageal cancer: a pilot study. World J Microbiol Biotechnol. (2021) 37:128. doi: 10.1007/s11274-021-03086-3
49. Ni B, Kong X, Yan Y, Fu B, Zhou F, Xu S. Combined analysis of gut microbiome and serum metabolomics reveals novel biomarkers in patients with early-stage non-small cell lung cancer. Front Cell Infect Microbiol. (2023) 13:1091825. doi: 10.3389/fcimb.2023.1091825
50. Poore GD, Kopylova E, Zhu Q, Carpenter C, Fraraccio S, Wandro S, et al. Microbiome analyses of blood and tissues suggest cancer diagnostic approach. Nature. (2020) 579:567–74. doi: 10.1038/s41586-020-2095-1
51. Chen S, Jin Y, Wang S, Xing S, Wu Y, Tao Y, et al. Cancer type classification using plasma cell-free RNAs derived from human and microbes. eLife. (2022) 11:e75181. doi: 10.7554/eLife.75181
52. Huang D, Su X, Yuan M, Zhang S, He J, Deng Q, et al. The characterization of lung microbiome in lung cancer patients with different clinicopathology. Am J Cancer Res. (2019) 9:2047–63.
53. Zheng L, Sun R, Zhu Y, Li Z, She X, Jian X, et al. Lung microbiome alterations in NSCLC patients. Sci Rep. (2021) 11:11736. doi: 10.1038/s41598-021-91195-2
54. Zhou Y, Zeng H, Liu K, Pan H, Wang B, Zhu M, et al. Microbiota profiles in the saliva, cancerous tissues and its companion paracancerous tissues among Chinese patients with lung cancer. BMC Microbiol. (2023) 23:237. doi: 10.1186/s12866-023-02882-1
55. Bingula R, Filaire E, Molnar I, Delmas E, Berthon J-Y, Vasson M-P, et al. Characterisation of microbiota in saliva, bronchoalveolar lavage fluid, non-malignant, peritumoural and tumour tissue in non-small cell lung cancer patients: a cross-sectional clinical trial. Respir Res. (2020) 21:129. doi: 10.1186/s12931-020-01392-2
56. Lu H, Gao NL, Tong F, Wang J, Li H, Zhang R, et al. Alterations of the human lung and gut microbiomes in non-small cell lung carcinomas and distant metastasis. Microbiol Spectr. (2021) 9:e00802–21. doi: 10.1128/Spectrum.00802-21
57. Cai H-Z, Zhang H, Yang J, Zeng J, Wang H. Preliminary assessment of viral metagenome from cancer tissue and blood from patients with lung adenocarcinoma. J Med Virol. (2021) 93:5126–33. doi: 10.1002/jmv.26887
58. Zhang K, He C, Qiu Y, Li X, Hu J, Fu B. ASSOCIATION OF ORAL MICROBIOTA AND PERIODONTAL DISEASE WITH LUNG CANCER: A SYSTEMATIC REVIEW AND META-ANALYSIS. J Evidence-Based Dental Pract. (2023) 23:101897. doi: 10.1016/j.jebdp.2023.101897
59. Dong J, Li W, Wang Q, Chen J, Zu Y, Zhou X, et al. Relationships between oral microecosystem and respiratory diseases. Front Mol Biosci. (2022) 8:718222. doi: 10.3389/fmolb.2021.718222
60. Zeng X-T, Xia L-Y, Zhang Y-G, Li S, Leng W-D, Kwong JSW. Periodontal disease and incident lung cancer risk: A meta-analysis of cohort studies. J Periodontol. (2016) 87:1158–64. doi: 10.1902/jop.2016.150597
61. Wei F, Lin C-C, Joon A, Feng Z, Troche G, Lira ME, et al. Noninvasive saliva-based EGFR gene mutation detection in patients with lung cancer. Am J Respir Crit Care Med. (2014) 190:1117–26. doi: 10.1164/rccm.201406-1003OC
62. Zhang L, Xiao H, Zhou H, Santiago S, Lee JM, Garon EB, et al. Development of transcriptomic biomarker signature in human saliva to detect lung cancer. Cell Mol Life Sci. (2012) 69:3341–50. doi: 10.1007/s00018-012-1027-0
63. Xiao H, Zhang L, Zhou H, Lee JM, Garon EB, Wong DTW. Proteomic analysis of human saliva from lung cancer patients using two-dimensional difference gel electrophoresis and mass spectrometry. Mol Cell Proteomics. (2012) 11(2):M111.012112. doi: 10.1074/mcp.M111.012112
64. Liu J, Huang D, Cai Y, Cao Z, Liu Z, Zhang S, et al. Saliva diagnostics: emerging techniques and biomarkers for salivaomics in cancer detection. Expert Rev Mol Diagnostics. (2022) 22:1077–97. doi: 10.1080/14737159.2022.2167556
65. Dickson RP, Martinez PFJ, Huffnagle PGB. The role of the microbiome in exacerbations of chronic lung diseases. Lancet. (2014) 384:691. doi: 10.1016/S0140-6736(14)61136-3
66. Cong J, Zhang X. How human microbiome talks to health and disease. Eur J Clin Microbiol Infect Dis. (2018) 37:1595–601. doi: 10.1007/s10096-018-3263-1
67. Blainey PC, Milla CE, Cornfield DN, Quake SR. Quantitative analysis of the human airway microbial ecology reveals a pervasive signature for cystic fibrosis. Sci Transl Med. (2012) 4:153ra130. doi: 10.1126/scitranslmed.3004458
68. Dong Q, Chen ES, Zhao C, Jin C. Host-microbiome interaction in lung cancer. Front Immunol. (2021) 12:679829. doi: 10.3389/fimmu.2021.679829
69. Tsay J-CJ, Wu BG, Badri MH, Clemente JC, Shen N, Meyn P, et al. Airway microbiota is associated with upregulation of the PI3K pathway in lung cancer. Am J Respir Crit Care Med. (2018) 198:1188–98. doi: 10.1164/rccm.201710-2118OC
70. Xue C, Chu Q, Zheng Q, Yuan X, Su Y, Bao Z, et al. Current understanding of the intratumoral microbiome in various tumors. Cell Rep Med. (2023) 4:100884. doi: 10.1016/j.xcrm.2022.100884
71. Nejman D, Livyatan I, Fuks G, Gavert N, Zwang Y, Geller LT, et al. The human tumor microbiome is composed of tumor type-specific intracellular bacteria. Science. (2020) 368:973–80. doi: 10.1126/science.aay9189
72. Nath S, Kitsios GD, Bos LDJ. Gut-lung crosstalk during critical illness. Curr Opin Crit Care. (2023) 29:130–7. doi: 10.1097/MCC.0000000000001015
73. Integrative HMP (iHMP) Research Network Consortium. The integrative human microbiome project: dynamic analysis of microbiome-host omics profiles during periods of human health and disease. Cell Host Microbe. (2014) 16:276–89. doi: 10.1016/j.chom.2014.08.014
74. Lim MY, Hong S, Hwang KH, Lim EJ, Han J-Y, Nam Y-D. Diagnostic and prognostic potential of the oral and gut microbiome for lung adenocarcinoma. Clin Transl Med. (2021) 11:e508. doi: 10.1002/ctm2.508
75. Damgaard C, Magnussen K, Enevold C, Nilsson M, Tolker-Nielsen T, Holmstrup P, et al. Viable bacteria associated with red blood cells and plasma in freshly drawn blood donations. PloS One. (2015) 10:e0120826. doi: 10.1371/journal.pone.0120826
76. Zhou H, Liao J, Leng Q, Chinthalapally M, Dhilipkannah P, Jiang F. Circulating bacterial DNA as plasma biomarkers for lung cancer early detection. Microorganisms. (2023) 11:582. doi: 10.3390/microorganisms11030582
77. Brzychczy-Sroka B, Talaga-Ćwiertnia K, Sroka-Oleksiak A, Gurgul A, Zarzecka-Francica E, Ostrowski W, et al. Standardization of the protocol for oral cavity examination and collecting of the biological samples for microbiome research using the next-generation sequencing (NGS): own experience with the COVID-19 patients. Sci Rep. (2024) 14:3717. doi: 10.1038/s41598-024-53992-3
78. Lim Y, Totsika M, Morrison M, Punyadeera C. The saliva microbiome profiles are minimally affected by collection method or DNA extraction protocols. Sci Rep. (2017) 7:8523. doi: 10.1038/s41598-017-07885-3
79. Jo R, Nishimoto Y, Umezawa K, Yama K, Aita Y, Ichiba Y, et al. Comparison of oral microbiome profiles in stimulated and unstimulated saliva, tongue, and mouth-rinsed water. Sci Rep. (2019) 9:16124. doi: 10.1038/s41598-019-52445-6
80. Bang E, Oh S, Ju U, Chang HE, Hong J-S, Baek H-J, et al. Factors influencing oral microbiome analysis: from saliva sampling methods to next-generation sequencing platforms. Sci Rep. (2023) 13:10086. doi: 10.1038/s41598-023-37246-2
81. Yano Y, Vogtmann E, Shreves AH, Weinstein SJ, Black A, Diaz-Mayoral N, et al. Evaluation of alcohol-free mouthwash for studies of the oral microbiome. PloS One. (2023) 18:e0284956. doi: 10.1371/journal.pone.0284956
82. Armstrong AJS, Parmar V, Blaser MJ. Assessing saliva microbiome collection and processing methods. NPJ Biofilms Microbiomes. (2021) 7:81. doi: 10.1038/s41522-021-00254-z
83. Shen J, Liao J, Guarnera MA, Fang H, Cai L, Stass SA, et al. Analysis of microRNAs in sputum to improve computed tomography for lung cancer diagnosis. J Thorac Oncol. (2014) 9:33–40. doi: 10.1097/JTO.0000000000000025
84. Anjuman N, Li N, Guarnera M, Stass SA, Jiang F. Evaluation of lung flute in sputum samples for molecular analysis of lung cancer. Clin Transl Med. (2013) 2:15. doi: 10.1186/2001-1326-2-15
85. Cameron SJS, Lewis KE, Huws SA, Hegarty MJ, Lewis PD, Pachebat JA, et al. A pilot study using metagenomic sequencing of the sputum microbiome suggests potential bacterial biomarkers for lung cancer. PloS One. (2017) 12:e0177062. doi: 10.1371/journal.pone.0177062
86. Kim G, Park C, Yoon YK, Park D, Lee JE, Lee D, et al. Prediction of lung cancer using novel biomarkers based on microbiome profiling of bronchoalveolar lavage fluid. Sci Rep. (2024) 14:1691. doi: 10.1038/s41598-024-52296-w
87. Yu G, Gail MH, Consonni D, Carugno M, Humphrys M, Pesatori AC, et al. Characterizing human lung tissue microbiota and its relationship to epidemiological and clinical features. Genome Biol. (2016) 17:163. doi: 10.1186/s13059-016-1021-1
88. Zhuang H, Cheng L, Wang Y, Zhang Y-K, Zhao M-F, Liang G-D, et al. Dysbiosis of the gut microbiome in lung cancer. Front Cell Infection Microbiol. (2019) 9:112. doi: 10.3389/fcimb.2019.00112
89. Martínez N, Hidalgo-Cantabrana C, Delgado S, Margolles A, Sánchez B. Filling the gap between collection, transport and storage of the human gut microbiota. Sci Rep. (2019) 9:8327. doi: 10.1038/s41598-019-44888-8
90. Bokulich NA, Maldonado J, Kang D-W, Krajmalnik-Brown R, Caporaso JG. Rapidly processed stool swabs approximate stool microbiota profiles. mSphere. (2019) 4:e00208–19. doi: 10.1128/mSphere.00208-19
91. Zouiouich S, Byrd DA, Hua X, Karwa S, Wan Y, Shi J, et al. Stability of the fecal and oral microbiome over two years at –80°C for multiple collection methods. Cancer Epidemiol Biomarkers Prev. (2023) 32:444–51. doi: 10.1158/1055-9965.EPI-22-0883
92. Park C, Yun KE, Chu JM, Lee JY, Hong CP, Nam YD, et al. Performance comparison of fecal preservative and stock solutions for gut microbiome storage at room temperature. J Microbiol. (2020) 58:703–10. doi: 10.1007/s12275-020-0092-6
93. Jing Q, Leung CHC, Wu AR. Cell-free DNA as biomarker for sepsis by integration of microbial and host information. Clin Chem. (2022) 68:1184–95. doi: 10.1093/clinchem/hvac097
94. Paudel KR, Dharwal V, Patel VK, Galvao I, Wadhwa R, Malyla V, et al. Role of lung microbiome in innate immune response associated with chronic lung diseases. Front Med (Lausanne). (2020) 7:554. doi: 10.3389/fmed.2020.00554
95. Tsay J-CJ, Wu BG, Sulaiman I, Gershner K, Schluger R, Li Y, et al. Lower airway dysbiosis affects lung cancer progression. Cancer Discovery. (2021) 11:293–307. doi: 10.1158/2159-8290.CD-20-0263
96. Li W, Liu B, Wang W, Sun C, Che J, Yuan X, et al. Lung cancer stage prediction using multi-omics data. Comput Math Methods Med. (2022) 2022:2279044. doi: 10.1155/2022/2279044
97. Ran Z, Liu J, Wang F, Xin C, Shen X, Zeng S, et al. Analysis of pulmonary microbial diversity in patients with advanced lung cancer based on high-throughput sequencing technology. Zhongguo Fei Ai Za Zhi. (2020) 23:1031–8. doi: 10.3779/j.issn.1009-3419.2020.103.16
98. Peterson SN, Bradley LM, Ronai ZA. The gut microbiome: an unexpected player in cancer immunity. Curr Opin Neurobiol. (2020) 62:48–52. doi: 10.1016/j.conb.2019.09.016
99. Lan Q, Hsiung CA, Matsuo K, Hong Y-C, Seow A, Wang Z, et al. Genome-wide association analysis identifies new lung cancer susceptibility loci in never-smoking women in Asia. Nat Genet. (2012) 44:1330–5. doi: 10.1038/ng.2456
100. Goubet A-G, Daillère R, Routy B, Derosa L, M. Roberti P, Zitvogel L. The impact of the intestinal microbiota in therapeutic responses against cancer. Comptes Rendus Biologies. (2018) 341:284–9. doi: 10.1016/j.crvi.2018.03.004
101. Ma P-J, Wang M-M, Wang Y. Gut microbiota: A new insight into lung diseases. Biomedicine Pharmacotherapy. (2022) 155:113810. doi: 10.1016/j.biopha.2022.113810
102. Gao J, Yi X, Wang Z. The application of multi-omics in the respiratory microbiome: Progresses, challenges and promises. Comput Struct Biotechnol J. (2023) 21:4933–43. doi: 10.1016/j.csbj.2023.10.016
103. Liu F, Li J, Guan Y, Lou Y, Chen H, Xu M, et al. Dysbiosis of the gut microbiome is associated with tumor biomarkers in lung cancer. Int J Biol Sci. (2019) 15:2381–92. doi: 10.7150/ijbs.35980
104. Chen Q, Hou K, Tang M, Ying S, Zhao X, Li G, et al. Screening of potential microbial markers for lung cancer using metagenomic sequencing. Cancer Med. (2023) 12:7127–39. doi: 10.1002/cam4.5513
105. Liu B, Li Y, Suo L, Zhang W, Cao H, Wang R, et al. Characterizing microbiota and metabolomics analysis to identify candidate biomarkers in lung cancer. Front Oncol. (2022) 12:1058436. doi: 10.3389/fonc.2022.1058436
Keywords: lung cancer, microbes, detection, diagnosis, biomarker
Citation: Zhou S, Zhu W, Guo H, Nie Y, Sun J, Liu P and Zeng Y (2024) Microbes for lung cancer detection: feasibility and limitations. Front. Oncol. 14:1361879. doi: 10.3389/fonc.2024.1361879
Received: 05 January 2024; Accepted: 29 April 2024;
Published: 08 May 2024.
Edited by:
So Hee Kwon, Yonsei University, Republic of KoreaReviewed by:
Junfeng Zhang, Nanjing University of Chinese Medicine, ChinaWang Ke, Guangxi Medical University, China
Copyright © 2024 Zhou, Zhu, Guo, Nie, Sun, Liu and Zeng. This is an open-access article distributed under the terms of the Creative Commons Attribution License (CC BY). The use, distribution or reproduction in other forums is permitted, provided the original author(s) and the copyright owner(s) are credited and that the original publication in this journal is cited, in accordance with accepted academic practice. No use, distribution or reproduction is permitted which does not comply with these terms.
*Correspondence: Ping Liu, liuping791005@139.com; Yulan Zeng, 1989ly0551@hust.edu.cn