- Industrial and Manufacturing Systems Engineering, Iowa State University, Ames, IA, United States
This paper developed human-autonomy teaming (HAT) characteristics and requirements by comparing and synthesizing two aerospace case studies (Single Pilot Operations/Reduced Crew Operations and Long-Distance Human Space Operations) and the related recent HAT empirical studies. Advances in sensors, machine learning, and machine reasoning have enabled increasingly autonomous system technology to work more closely with human(s), often with decreasing human direction. As increasingly autonomous systems become more capable, their interactions with humans may evolve into a teaming relationship. However, humans and autonomous systems have asymmetric teaming capabilities, which introduces challenges when designing a teaming interaction paradigm in HAT. Additionally, developing requirements for HAT can be challenging for future operations concepts, which are not yet well-defined. Two case studies conducted previously document analysis of past literature and interviews with subject matter experts to develop domain knowledge models and requirements for future operations. Prototype delegation interfaces were developed to perform summative evaluation studies for the case studies. In this paper, a review of recent literature on HAT empirical studies was conducted to augment the document analysis for the case studies. The results of the two case studies and the literature review were compared and synthesized to suggest the common characteristics and requirements for HAT in future aerospace operations. The requirements and characteristics were grouped into categories of team roles, autonomous teammate types, interaction paradigms, and training. For example, human teammates preferred the autonomous teammate to have human-like characteristics (e.g., dialog-based conversation, social skills, and body gestures to provide cue-based information). Even though more work is necessary to verify and validate the requirements for HAT development, the case studies and recent empirical literature enumerate the types of functions and capabilities needed for increasingly autonomous systems to act as a teammate to support future operations.
1 Introduction
Human-Autonomy Teaming (HAT) requirements and characteristics for future aerospace operations have been developed through the synthesis of a new literature review of empirical HAT studies and two previously conducted case students in Single Pilot Operations (SPO) (Tokadlı et al., 2021a) and beyond low-Earth orbit (LEO) space operations (Tokadlı and Dorneich, 2018). Current automated systems, while highly capable, do not yet approach the level of sophistication needed to be considered true teammates. The requirements and characteristics developed in this work enumerate the capabilities needed to support HAT concepts in future aerospace operations, and serve as a roadmap of the types of capabilities that need to be developed to get closer to team-like systems.
While developing advanced intelligent systems, it is often challenging to predict and define the capabilities needed for future concepts of operation. This is particularly challenging when designing for future concepts of operation, which are often ill-defined and still under development. Thus, it is often the case that work does not touch on the specifics of a concept of operations but instead focuses more on the human interaction and perception of autonomous systems in teaming settings. Therefore, this work focuses on synthesizing the HAT requirements in the aerospace domain for two future concepts of operations: SPO in commercial air transportation and Space Operations beyond LEO. The common requirements and characteristics for HAT of this work provide some initial guidance on how to develop autonomous systems with the necessary capabilities to function as an autonomous teammate in future aerospace operations and address many human factors issues of increasingly autonomous systems working in a tightly coupled manner with humans.
2 Related work
2.1 Automation, autonomy, and human-autonomy teaming
Current concepts of operations in many domains integrate advanced technology to increase efficiency and throughput, maximize the ability to operate at full capacity in more varied situations, and decrease the level of human supervision required (Cummings, 2014; Lyons et al., 2021). As these increasingly autonomous systems expand their capabilities to sense the situation in real-time, make judgments about the best course of action, and take independent action, they start playing the role of a teammate to humans rather than simply a tool. In this context, HAT is the collaboration between increasingly autonomous systems and humans to jointly accomplish mission goals, execute tasks, and make decisions as a team.
Increasingly autonomous system capabilities necessitate a distinction between automation and autonomy based on their capabilities and limitations as well as their interaction with humans. Automation refers to a system that will do what it is programmed to do without independent action (Vagia et al., 2016; Demir et al., 2017a). For example, a human driver can activate longitudinal control functionality and transition from full manual mode to supervisory control in the vehicle. This necessitates human driver to actively monitor the driver assistance automation and take control when it is needed. Autonomy is a system that can behave with intention, set goals, learn, and respond to situations with greater independence and even without direct human direction (Shively, et al., 2016; Woods, 2016). For example, human-in-the-loop autonomous convoys with leader-follower configuration consist of human-operated leader and autonomy-operated follower vehicles which share the same travel route while maintaining safe following distance. Vehicle-to-vehicle communication systems support the exchange of information between the vehicle operators (human and autonomy) to operate the vehicles and coordinate the maneuvers to keep convoy together and ensure traffic safety. While autonomous follower vehicle augments the driving decision of the human-controlled leader vehicle, the autonomy still must make additional driving decisions because of the vehicle-to-vehicle data transmission delay and the changing traffic dynamics around the follower vehicle (Locomation, Inc., 2022; Nahavandi et al., 2022).
A human-autonomy team consists of one or more human teammates interacting with one or more autonomous systems where each can function in a partially self-directed manner and where they collaborate to achieve shared mission goals (Demir et al., 2017b; McDermott et al., 2018; McNeese et al., 2018). In this context, an autonomous system will have more authority, responsibility, and capability than an automation tool (Shively, et al., 2017). However, it is less clear what functions and capabilities make an autonomous system truly a teammate (Kaliardos, 2022). Shifting from automation being a tool to an autonomous system being a teammate introduces several challenges: 1) an under-defined concept of operations of future operations in HAT, 2) developing the teaming skills of an autonomous system, and 3) determining the most effective HAT interaction paradigms.
2.2 All-human teaming to human-autonomy teaming
In all-human teaming, each team member is a functional unit, acting as a complex and essential component of the team (Salas et al., 2017). Team members must coordinate and communicate to exchange, integrate, and synthesize information to pursue common goals (Salas et al., 2008). Salas et al. (2015) identified considerations based on the dimensions of teamwork, which consist of six core processes and emergent states (coordination, cooperation, conflict, communication, coaching, and cognition). Cooperation is an essential component of teamwork that drives behaviors. Conflict occurs because of an incompatibility of beliefs, interests, or views held by one or more teammates, which leads to errors and breakdowns in team performance (Salas et al., 2017). Coordination is necessary to enable multiple team members to perform interdependent tasks and transform team resources into outcomes. Communication enables teammates to exchange information to affect the team’s attitudes, behaviors, and cognition. Coaching is a leadership behavior to help teammates achieve team goals (Fleishman, et al., 1991; Hackman and Wageman, 2005). Cognition allows teams to have a shared understanding of how to engage in the tasks (Salas et al., 2015).
A long history of research in human-automation interaction has identified many human factors issues when automation is introduced into the work process. Early work identified the ironies of automation (Bainbridge, 1983), where for instance automation can increase in already-high workload tasks, or the automation chooses inappropriate actions due to a failure to understand the context of the situation. Automation can also cause other human factors issues such as decreases in situational awareness (Kaber et al., 1999), poor function allocation design (Dorneich et al., 2003), lack of automation system transparency (Woods, 2016; Dorneich, et al., 2017), and miscalibrated trust (Lee and See, 2004). These traditional automation-related human factors issues are exacerbated when the automation because increasingly capable, intelligent, and autonomous. The tightly coupled nature of HAT requires that the design of the intelligent system mitigate these human factor’s issues.
2.3 Applying human-autonomy teaming
The level of autonomous system development varies across applications and domains. For instance, in healthcare, assistive robots are being developed to address the shortage of available qualified healthcare workers (Iroju et al., 2017). Surgical assistance robots have been developed to support surgical operations with increased precision and safety (Keefe, 2015), and rehabilitation or therapy robots have been assisting patients with physical and mental disabilities (Diehl et al., 2014; Iroju et al., 2017). Social assistive robot interaction with patients reduced stress level (Wada and Shibata, 2007), and encouraged patients to continue programs (Kidd and Breazeal, 2008). While these systems are teaming with humans in healthcare, their level of autonomy is designed as non-autonomous. In such a teaming setting, humans are always supervisors and/or operators to provide treatment to the patients by working with the robots.
In order to be effective, autonomous teammates should be able to adapt to changing conditions in the work environment. Autonomous teammates will be able to initiate changes in their behavior without initiation by the human operator based on their assessment of the needs of the human operator (Feigh et al., 2012). HAT design allows both the human and autonomous teammates to make decisions and dynamically adjust task allocation (Dorneich et al., 2003; Hardin and Goodrich, 2009; Goodrich, 2010; Goodrich, 2013; Chen and Barnes, 2014; Barnes et al., 2015). Teammates should be able to delegate functions to both human and autonomous teammates by communicating mission objectives and plans using human-like methods (Miller et al., 2004; Patel et al., 2013). For example, smart manufacturing systems have adaptive behavior that enables them to join their human operators in decision making, to adapt to disturbances without human supervision, and to assist in planning modifications (Park, 2013). This contrasts with healthcare systems, which still require human supervision.
Embodiment of the autonomous system can be another important factor for the human perception of autonomous system’s teammate likeness. Human-like appearance has impact on human interaction in addition to human-like attitudes and behaviors. A survey study on robot’s appearance and capabilities showed that the participants were influenced by the appearance of the robots rather than their capabilities to find them suitable for certain jobs (Lohse et al., 2008). Several studies found that more human-like appearance and behaviors increase the interaction and the expectation of humans on autonomous system’s capabilities (e.g., Gockley et al., 2005; Hayashi et al., 2010; de Graaf et al., 2015). Similar results were observed during the SPO case study experiments where the pilots stated the need of human-like appearance to increase their acceptance and expectations on its capabilities as well as social interactions (Tokadlı et al., 2021b). If expectations are not met, trust and the frequency of interactions would decrease in time (Gockley et al., 2005; Tokadlı et al., 2021b).
In social assistive robotics in healthcare research, it has been found that a humanoid robot with a personality can have positive affect on patients’ motivational states by augmenting human care (Leite et al., 2013). For example, an ethnographic study results on humanoid social robot interactions in and elderly care facility resulted with the acceptance of the robot due to greetings, communicating with participants by using their names (Sabelli et al., 2011). These robots were employed in the elderly care facility to operate as conversational partner.
3 Approach
This work aims to develop a set of high-level system feature and behavior requirements for HAT design in future aerospace operations to identify the capabilities and functions needed for an autonomous system to fully participate in HAT as a teammate. Two case studies drew upon a review of the empirical literature in HAT using a systematic approach to develop a future concept of operation and related requirements. In addition, a review of recent literature on HAT evaluation studies was performed, focused on work published after the case studies were completed. The results of the two case studies and the empirical literature review were synthesized to define common HAT characteristics and requirements for future aerospace operations (see Figure 1).
3.1 Analyze case studies
Previous work explored two different aerospace operation concepts with HAT: Single Pilot Operations in commercial air transportation (SPO) and Space Operations beyond LEO. These case studies were investigated to compare differences and similarities in human-autonomy teaming in work environments based on the concepts of operations. The systematic five-step approach described below was applied to both case studies.
Step 1:. Eliciting Knowledge was performed to synthesize documented and operational knowledge of work domains to develop an understanding of existing concepts of operation, stakeholders and their needs, and use cases. Documented knowledge was analyzed using document analysis of literature to investigate what people should do. Subject Matter Expert (SME) interviews were conducted to investigate what people would do.
Step 2:. Generating Domain Knowledge Models of current operations comprehensively represent the work domain of decision-making processes and tasks. The literature related to ‘human-autonomy teaming’ and information on future operations for the target work domain were reviewed and synthesized develop domain knowledge models of current operations. Qualitative data analysis (QDA) was used to organize the information units from document analysis. (QDA) is based on a thematic analysis approach where each document was reviewed thoroughly and investigated patterns across a set of data and identified themes based on the patterns. The document review process was performed twice to ensure details were captured and the information units on each document were coded appropriately. QDA software was used to generate network and relationship maps between themes and the documents. These artifacts helped organize and categorize the qualitative data to build domain knowledge models: an Abstraction Hierarchy (AH) and a Decision-Action Diagram (DAD). An AH identifies the constraints, boundaries, and limitations of the work system, as well as the goals and functions of the system (Rasmussen, 1986; Vicente, 1999). A DAD illustrates the decision-making process by identifying the flow of decision-making functions and the branching points for all potential alternative decision paths regarding the functions to follow (Stanton et al., 2005). These domain knowledge models were first developed to represent current operations and then were extrapolated to represent future concepts of operation that included HAT. Functions and goals that would change in future operations were identified and extrapolated to account for future conditions. For example, future space operations would have long communication delays, which cause a breakdown in today’s tight coordination loop with Earth mission control. Thus in future operations, crews will rely on increasing autonomous systems to perform functions, and new tasks or changes to existing tasks are needed because of HAT integration. By grounding the process of developing the extrapolated domain knowledge models in a systematic way, the resulting representation should be complete and capture the changes from the current operations, be able to address the potential breakdowns, changes, new functions, and include the revised roles and responsibilities.
Step 3:. Deriving preliminary HAT requirements were based on the extrapolated domain knowledge models. Separate sets of HAT requirements and characteristics were identified for each case study (see Tokadlı et al. (2021a); Tokadlı and Dorneich (2018) for details).
Step 4:. –Conducting Summative evaluation studies helped to test the hypotheses and assumptions on developing HAT. Requirements and interaction paradigms guide the development of HAT prototypes based on the known teaming needs (e.g., coordination, communication) and autonomous system capabilities. A Playbook delegation interface system was developed to test HAT concepts in SPO. A Cognitive Assistant was used to test Hat concepts in long-distance space operations.
Step 5:. Revising HAT requirements finalize the set of requirements based on the findings in Step 4. The results of the evaluation study were used to confirm and revise the requirements and characteristics of HAT in each case study separately.
3.2 Review recent empirical literature in HAT
Empirical literature was reviewed based on the following criteria: 1) the publication includes HAT concepts, and 2) presents an empirical study to evaluate HAT in aerospace operations. The following search keywords are used in both Google Scholar and Aerospace Research Central (ARC): space missions, human-autonomy teaming, human-machine teaming, and reduced crew operation. Because the document analyses (Step 1) of the case studies consisted of relevant literature up to 2019, the current literature search was limited to between 2019 and 2023. A total of 12 relevant publications were reviewed to compare with the findings of the two case studies. The new literature focused on papers that included empirical studies to support their recommendations and findings. Thus, the literature review and the two case studies provided an empirical basis for the synthesis to develop the requirements and characteristics.
3.3 Synthesize and compare
The results of the case studies were compared using teaming themes, which were defined through literature review and document analysis. These themes were identified as team formation, autonomous system design, interaction modes, teaming roles, and expected teammate characteristics and processes. The similarities between case studies and the findings from the recent empirical literature on HAT were synthesized to establish common requirements and characteristics of HAT in aerospace operations.
4 Summary of case studies
The previous studies investigated Single Pilot Operations/Reduced Pilot Operations (SPO/RCO) in commercial aviation (see details of case study from Tokadlı et al. (2021a); Tokadlı et al. (2021b)) and space operations beyond LEO (see details of case study from Tokadlı and Dorneich. (2018); Tokadlı and Dorneich, (2022)) as future aerospace operations with HAT concepts. These case studies were selected because they represented differences in the integration of an autonomous system in the team composition. While SPO/RCO reduces the number of pilots in the cockpit from two to one and add an autonomous system to replace the eliminated pilot, space operations beyond LEO either adds an autonomous teammate without removing any human astronaut or replace one astronaut with an autonomous system. This section summarizes each case study by providing concept of operations, case study analysis and findings through the experiments.
4.1 Case study 1: single pilot operations/reduced crew operations
4.1.1 Concept of operations
SPO/RCO reduces the number of pilots in the cockpit for commercial air transportation by removing the second pilot from the cockpit. The human and autonomous system interactions will become more tightly coupled than with today’s automation. The autonomous system will be required to interact with the human pilot more as a teammate than a tool. Increasingly autonomous system and the onboard pilot in the cockpit will need to share and perform the second pilot’s functions. Additionally, the increasingly autonomous system must coordinate with the onboard pilot and ground personnel (Matessa, 2018), support managing flight tasks and making flight and task allocation decisions. Even with increased autonomous system support, SPO may cause an increase in the pilot workload, specifically when the autonomous system fails to understand the pilot’s intention and other context information (Zhang et al., 2021), as well as introduce significant safety issues (Dorneich et al., 2016). Hence, HAT for SPO/RCO concepts must be studied to identify the transition plan from dual-pilot operations to SPO and develop risk mitigation techniques (Ho et al., 2017; Shively, 2017).
4.1.2 Case study analysis
The five-step guidance was applied to investigate what kind of HAT support SPO concepts need and how HAT can be implemented to support the single pilot. Semi-structured interviews with four SMEs (licensed pilots) were conducted to elicit operational knowledge and gather feedback on the document analysis output (Step 1). The interviews discussed how commercial airline pilots fly and make decisions in today’s operations and their perspectives on future SPO concepts and potential challenges with HAT in the cockpit (Tokadlı et al., 2021a). An AH and a DAD were developed as domain knowledge models for today’s dual-pilot operations and extrapolated for future HAT concepts (Step 2). Next, the extrapolated domain knowledge models were developed based on the potential breakdowns in the operational tasks and coordination in the cockpit and with the ground station, and potential new tasks needed to be performed HAT in the cockpit. Later, the extrapolated models were used to identify preliminary HAT requirements (Step 3). A HAT Playbook interaction concept was implemented as an SPO autonomous system prototype integrated with a flight simulator platform. An evaluation was conducted to evaluate pilot teaming interactions with the system and the assumptions based on the extrapolated domain knowledge models (Step 4) (Tokadlı et al., 2021b). The objectives of this study were to elicit feedback from pilots based on hands-on experience with a prototype autonomous teammate, compare their experience between HAT and all-human teaming in the cockpit, and assess the HAT requirements. Twenty pilots completed the evaluation study by using a modular flight simulator with a prototype SPO HAT cockpit and autonomous teammate support (please see Tokadlı et al. (2021b) for more details).
4.1.3 Findings
Figure 2 represents side-by-side extrapolated domain knowledge models. The AH of the SPO HAT concept includes five generic levels (vertical levels) and three decomposition levels (horizontal levels): team management, system management, and flight management (Tokadlı et al., 2021a). Team management was defined as the functions and responsibilities to coordinate between the pilot, autonomous teammate, and ground-based team. System management was defined the functions and responsibilities of managing systems during the flight. Flight management was defined as the functions and responsibilities to complete flight tasks safely and efficiently. This decomposition level included information on how to make decisions about flight re-routing, weather and navigation, air traffic separation, and flight plan monitoring. It also helped to identify where the autonomous system versus human pilot would need to take more role and responsibility. Hence, the autonomous system functionalities and capabilities were derived based on this model. The DAD represented the collaborative decision-making process between human and autonomous teammates to safely complete a flight from an autonomous teammate’s perspective (Tokadlı et al., 2021a). Similar to AH, DAD helped to identify where the autonomous system can support the decision-making and create opportunities for efficient decision-making process. For example, in dual-pilot operations, two pilots can check in and see if the any of them needs medical attention. In SPO, the autonomous system should be able to act as a “check-in” system to understand whether pilot is incapacitated, or his/her performance is degraded. Under the performance degradation, the task allocation should be reconsidered to ensure the flight safety and support the pilot performance.
Thirty-three requirements were developed as a result of Steps 1 and 2. The requirements were categorized into team coordination and collaboration, task-authority-role allocation, HAT information visualization, training, and regulations (Tokadlı et al., 2021a). Key functions include collaborative decision-making, flight tasks, responding to emergencies, assessing team progression, error detection, and assessment.
During the interviews and post-experiment discussions, SMEs highlighted that core teaming and judgment skills are key for teaming and decision-making during a flight, specifically in the context of Crew Resource Management (CRM). In today’s two-pilot operations, pilots can assume a certain level of skill in judgment based on the training they receive. Compared with today’s two-pilot operations, assessing an autonomous teammate’s judgment performance might initially be challenging for pilots. SMEs identified that current training would need to be adapted for pilots to understand how the autonomous teammate can help decision-making.
In the experiments, pilots provided feedback on the prototype system for teaming skills and expectations from an autonomous teammate (Tokadlı et al., 2021b). With the autonomous teammate’s participation in the flight, pilots experienced that their role transitioned into that of a supervisor. They were willing to allocate as many tasks as possible to the autonomous co-pilot. Their role would be to monitor the autonomous co-pilot and flight to ensure a safe flight. By being able to allocate most of the tasks to their autonomous co-pilot, pilots did not feel that their workload would increase much compared to the dual-pilot operation experience. During tasks, pilots relied on the Playbook interface to gain insight into the autonomous co-pilot’s actions and reasoning. However, pilots stated that the autonomous system needed to participate more fully in the decision-making process in ways more similar to a human teammate. As the autonomous co-pilot gains more human-like judgment capabilities, it would change how the pilots perceive and collaborate with the autonomous systems (Tokadlı et al., 2021b). Even though some pilots’ perspectives on working with an autonomous co-pilot changed after the experiment, they still stated that they preferred to work with human co-pilots rather than an autonomous system. Post-experiment discussions revealed that pilots struggled with distinguishing “automation” and “autonomy” because of the lack of human-like characteristics and interaction.
4.2 Case study 2: long-distance space operations
4.2.1 Concept of operations
The current roadmap of space operations beyond LEO includes missions to the lunar vicinity, the Moon, near-Earth asteroids, and Mars (International Space Exploration Coordination Group, 2017). Operations to the lunar vicinity and the Moon enable researchers to investigate the risks of human space exploration while staying close to Earth (International Space Exploration Coordination Group, 2017). Operations beyond LEO, particularly to Mars and beyond, introduce operational challenges due to the communication delay between Earth and space vehicles. Information exchange and real-time guidance from Mission Control will be delayed or unavailable to the space crew. Communication delays to Mars can be as long as 20 min one way. This implies that where current space crews relay on Mission Control to lead most responses to emergencies, in Mars missions the crew will be responsible, with little real-time support from Mission Control. Cognitive Assistants can assist the human crew in making decisions, taking actions, and offering capabilities Mission Control can no longer provide.
4.2.2 Case study analysis
The five-step process was applied to investigate what kind of HAT support space missions beyond LEO need, and how HAT can be implemented to support the space crew. Semi-structured interviews were conducted with two former NASA astronauts to elicit operational knowledge and gather feedback on the document analysis output (Step 1). The interviews discussed the current space decision-making practices to deal with off-nominal situations, the current guidelines developed for space operations, the collaborative work between Mission Control and space crew, and the type of support systems or tools which have been used for the space missions to deal with off-nominal situations (Tokadlı and Dorneich, 2018). AH and DAD models were developed for current concept of operations and extrapolated future concepts of operations (Step 2). Next, the extrapolated domain knowledge models were developed based on the potential breakdowns in the mission tasks and coordination between the space crew and the Mission control, and potential new tasks needed to be performed HAT in the space vehicle. Later, the extrapolated models were. Then, they were used to identify preliminary HAT requirements (Step 3). A computer-based simulator test platform of a Mars space station and a prototype autonomous system, Cognitive Assistant (CA), was developed to evaluate teaming interactions in HAT with different feedback modalities (Step 4). In this step, forty-four participants completed the evaluation study (see Tokadlı and Dorneich, 2022 for more details).
4.2.3 Findings
Figure 3 represents side-by-side extrapolated domain knowledge models. The domain knowledge models (AH and DAD) of the long-distance human-autonomy space operation concept began with the current decision-making processes and the collaboration between Mission Control and the space crew while dealing with an off-nominal situation which the crew does not have any previous experience with such event and any procedural support. The domain knowledge models on current concept of operations identified several communication breakdown points that may negatively impact mission processes, success, and safety. The extrapolated domain knowledge models introduced a potential way to mitigate those communication breakdowns so that the space crew can have continuous support without any disturbance due to communication delay with Mission Control (Tokadlı and Dorneich, 2018). For example, in case of encountering an off-nominal event, the procedure development or modification support from Mission Control Center will be delayed (see Figure 3). These tasks and responsibilities were identified to allocate space crew with HAT capabilities to mitigate any issues caused by the delay.
Twenty-two requirements were described for future space operations with HAT (Tokadlı and Dorneich, 2018). The summative evaluation (Step 4) investigated the factors contributing to the human teammate’s perception of an autonomous system (Tokadlı and Dorneich, 2022). In this study, participants evaluated two different CA feedback modalities. The centralized feedback modality supported the interaction between the participants and the CA through a single, text- and auditory-based interface. The distributed feedback modality provided the same information but added a graphical depiction of CA actions throughout the interface. Participants reported a higher perception of teammate-likeness with the distributed feedback modality due to higher transparency on autonomous system’s actions and information exchange. They had a greater awareness of the autonomous system and increased confidence that the CA supported decision-making and task sharing. Additionally, the teaming performance revealed that the participants spent less time resolving low task load events than the high task load events. Interestingly, in low task load conditions, the participants took less time with the centralized modality than distributed. However, in high workload conditions, the opposite occurred. Based on participants’ feedback, transparency level with distributed modality, the participants had better idea regarding what the CA was performing and why, and this information help them to move faster instead of trying to figure out what CA was doing in centralized modality (Tokadlı and Dorneich, 2022). While centralized feedback had some benefits during low task load, it was at a significant disadvantage at high task load, where participants increasingly leveraged the distributed feedback mode to track CA actions.
5 Recent HAT empirical study literature
Several research areas are relevant to developing a human-autonomy team to support new concepts of operations that include increasingly autonomous systems. The emerging literature in human-autonomy teaming establishes the current state of the science on the characteristics and needs of human and autonomous teammates.
The case studies were each initiated with a thorough literature review to develop the concept of operations, extrapolate domain knowledge models to future operations, and inform the evaluations. For this paper, more recent work has been reviewed here to incorporate new findings in the evaluation of HAT systems in order to inform a synthesis of the two case studies to develop HAT characteristics and requirements for HAT in aerospace systems. This section summarizes insights gained from recent literature on HAT evaluation studies and whether they can be used as evidence for supporting human-autonomy teaming requirements. Table 1 summarizes 12 papers relevant to the scope of the review.
When reviewing the work, several themes emerged: teaming skills and characteristics, autonomous teammate capabilities, training, and human-like aspects (see Table 2).
With the introduction of autonomous systems into human teaming, the roles, tasks, and interactions should be designed to support the engagement, mode awareness, vigilance, and awareness of teammate(s) (Neis, 2020). Zhang et al. (2021) found that human pilots preferred to have the final authority on the autonomous system and be able to intervene whenever it is necessary to keep the flight safe. Similar perspectives were gathered from participants in the SPO Case Study from both interviews and the summative evaluation (Tokadlı et al., 2021b). Even though humans want to remain in control and charge, monitoring each other’s HAT performance can also help support coordination and identify possible errors. For example, a human pilot monitors the autonomous system to detect errors due to potential sensor failures, while the autonomous system can monitor pilot progress through procedures (Zhang et al., 2021).
Essential all-human teaming skills such as coordination, communication, and cooperation should be maintained for HAT, according to recent empirical HAT studies. By allocating some tasks to the existing automation systems and autonomous teammates, human teammate(s) have more time and fewer distractions to focus on essential information (Faulhaber et al., 2022).
Well-defined procedures and role allocations support cooperation between pilots. By adapting best practices from dual-pilot operations to SPO, a single pilot and autonomous teammate should fluidly hand over tasks to each other depending on the situation (Zhang et al., 2021). Some example functions that can be allocated between an autonomous system and humans include checking displayed information, updating logs, comparing against predictions, checking engine health, planning escape routes, checking weather/atmosphere conditions, maneuvering, docking/undocking, system maintenance, assigned activity support, payload assistance, crew assistance, data analysis, and interpretation (Karasinski et al., 2020; Neis, 2020). While allocating tasks between humans and autonomous systems dynamically during operations, autonomous systems should provide human teammates with information on their capabilities (Ulusoy and Reisman, 2022). For example, this can be supported with a procedure categorized by autonomous system capabilities. The autonomous system should support collision avoidance, assist in situation awareness, and notify when capabilities are lost or diminished (Weiss et al., 2022).
Communication is another key teaming skill. If the autonomous system can explain its actions and reasoning, this can build trust between teammates (Le Vie et al., 2021; Zhang et al., 2021; Faulhaber et al., 2022; Faulhaber et al., 2022). In some cases, the participants preferred explanations that help prevent and mitigate anomalies and provide actionable information to make informed decisions (Barkouki et al., 2023).
Pilots mentioned several ways that they missed the ability of autonomous systems to communicate in ways similar to human-human dialogs (Zhang et al., 2021). Communication between humans and autonomous systems must allow the system to understand human intentions, think ahead, and react appropriately to complex and changing situations (Zhang et al., 2021). This communication skill should be bi-directional (Shively et al., 2017). In addition to verbal communication, the autonomous system should be able to engage in non-verbal communication to provide contextual information using social cues (Chan, et al., 2021). Cue-based communication can support the pilot’s perception of the autonomous system’s presence. Some studies recommended that the autonomous system should have human-like behaviors and anthropomorphic features (Zhang et al., 2021; Ulusoy and Reisman, 2022). Similarly, the evaluations of case studies showed that the human teammates’ perception of teammate-likeness increased with an embodied autonomous teammate that provided cues of actions taken (Tokadlı et al., 2021a; Tokadlı and Dorneich, 2022).
Training varies for emerging autonomous systems and can focus on areas such as system capabilities or interactions. Current training processes should be adjusted for HAT concepts to enable human crew members to perform newly allocated tasks and new procedures (Faulhaber et al., 2022). There might be a need for novel training techniques to support crews. For example, just-in-time training might be provided for critical tasks when the crew is not able to receive mission control support (Karasinski et al., 2020). Training duration may increase (Alvarez, 2020). Insufficient training in HAT may result in over- and under-trust issues (Karasinski et al., 2020).
6 Common HAT characteristics for aerospace operations
This work defines characteristics as the distinctive features, skills, or qualities that identify the system in its work context. Characteristics are used in this work to describe what makes an autonomous system a teammate in the work context of HAT. Several characteristics emerged from the results of the two case studies and the review of recent literature on HAT empirical studies. Areas of commonality in the development of HAT systems included team roles, expected teammate capabilities, autonomous teammate embodiment, interaction paradigms, and communication modalities. This section proposes the generalizable characteristics recommended for future aerospace operations in HAT concepts.
6.1 Teaming roles
A human teammate should have the authority and final say, while an autonomous teammate should participate in decision-making and task execution. In human-automation interaction (HAI) research, the human operators want to remain in charge of the operations but also want to have the benefits of automated functions and capabilities (Degani et al., 2000; Miller and Funk, 2001). However, the system triggers automatically change the authority level between human and the system to adapt the changing conditions (Degani et al., 2000). Similar to what conventional HAI research indicated, the case studies and literature demonstrate a prevailing desire of humans to remain in charge and supervise the autonomous teammate. Pilots want to have the final authority on the autonomous system and be able to intervene whenever it is necessary to keep the flight safe (Zhang et al., 2021). However, this comes with an expectation that the autonomous system should be able to act independently when working on tasks, make decisions related to its tasks, and learn the human teammates’ preferences over time so it will not repeatedly ask permission for similar tasks.
6.2 Expected teammate capabilities
An autonomous teammate should be able to monitor and manage teammate incapacitation; provide crew, task, and maintenance assistance; engage in human-like social conversation; convey teammate appreciation; conduct wellbeing checks; and support multiple levels of interdependency. Several teammate capabilities have been identified through case studies and the recent empirical study literature. HAT capability requirements are detailed in Section 7.
6.3 Autonomous teammate embodiment
An autonomous teammate should be designed as an embodied system that supports human teammates with physical task execution, verbal and non-verbal communication, and social interaction. Both case studies showed that human teammates prefer a physically present autonomous teammate rather than an embedded system with the rest of the vehicle or station (Tokadlı et al., 2021b; Tokadlı and Dorneich, 2022). Based on the previous research on embodiment (e.g. (Hayashi et al., 2010; de Graaf et al., 2015; Martini et al., 2016; Abubshait and Wiese, 2017; Wiese et al., 2018)), human-like characteristics and appearance have impact on human interaction. This work also supports that the embodiment of autonomous teammates contributes to an increased perception of teammate-likeness. Additionally, embodied autonomous systems can more readily execute physical tasks (e.g., execute extravehicular activities), have a social human-like verbal conversation (see Section 6.5), and have body gestures that can be perceived as cue-based information (e.g., the pilots tend to confirm checklist tasks by checking the body motion of the other pilot) (Tokadlı et al., 2021b; Zhang et al., 2021).
6.4 Interaction paradigm
The HAT Interface should support the dynamic collaboration between human and autonomous teammates and enable information exchange that approaches human-human communication. Human and autonomous teammates should naturally allocate tasks to each other when necessary, during missions (Zhang et al., 2021). In the study conducted by Zhang et al. (2021), participants preferred that autonomous systems communicate in ways similar to human-human dialog to understand human intentions and think ahead or react appropriately to complex and changing situations. Delegation-based interaction was evaluated in both case studies, enabling human and autonomous teammates to coordinate, execute, and adapt tasks as needed. Participants felt that they could allocate functions to the autonomous teammate when needed or when they preferred to be in the supervisor role during the mission. The delegation interface paradigm supported exchanging information between the human and autonomous teammates and enabled them to coordinate and plan tasks transparently (Miller et al., 2004; Tokadlı et al., 2021b). This interface paradigm depends on a shared task language between the humans and autonomous system teammates to promote shared understating and flexible operations (Miller et al., 1999; Miller and Parasuraman, 2007).
6.5 Communication modalities
Human and autonomous teammates should have conversational, bi-directional communication, and non-verbal communication. In addition, when necessary, a graphical user interface should support the exchange of written and visual information (Tokadlı et al., 2021b; O’Neil et al., 2022; Tokadlı and Dorneich, 2022). Multimodal interaction can enable HATs to adapt the interaction based on changing operating conditions. Bi-directional communication is a necessity for effective information exchange. Effective human-like communication can support human teammates’ trust when they receive explanations from the autonomous system (Le Vie et al., 2021; Zhang et al., 2021; Faulhaber et al., 2022; Faulhaber et al., 2022; Barkouki et al., 2023).
In addition to the recent empirical literature findings, both case studies showed that the participants preferred non-verbal information in addition to verbal communication. This would be possible for autonomous systems with human-like behaviors and anthropomorphic features (Zhang et al., 2021; Ulusoy and Reisman, 2022) (see Section 6.3). For example, in commercial dual-pilot operations, pilots monitor each other by checking body gestures and the visual feedback from interfaces. Suppose an autonomous system now acts as the second crew member. In that case, there is utility in having its action be visible through the interfaces to support the human pilot’s awareness (Tokadlı et al., 2021b). A graphical user interface with a graphical depiction of the actions of an autonomous teammate can also help maintain the human teammate’s awareness, specifically when the autonomous teammate is not physically present (Tokadlı and Dorneich, 2022). Maintaining this communication modality also contributes to an increased perception of teammate-likeness.
7 Common HAT requirements for aerospace operations
In this work, a requirement defines a system or service’s necessary attribute, capability, functionality, or quality (The MITRE Corporation, 2014). Based on the guidance on Turk (2006), the requirements were developed to follow the suggested format (e.g., use of “shall” statements) to provide what is needed rather than how to accomplish them. Since the future concept of operations are still ill-defined, these requirements serve as a reference starting point for systems and human factors engineers or researchers to define what is needed to truly achieve teaming between human and autonomous system. Thus, they can further define the detailed low-level system/sub-system requirements based on the concept of operations in the future. Comparing and synthesizing HAT requirements from the case studies and the recent empirical literature review resulted in 36 requirements (see Table 3). The requirements were categorized into themes: teaming, capability, allocation, interface, and training.
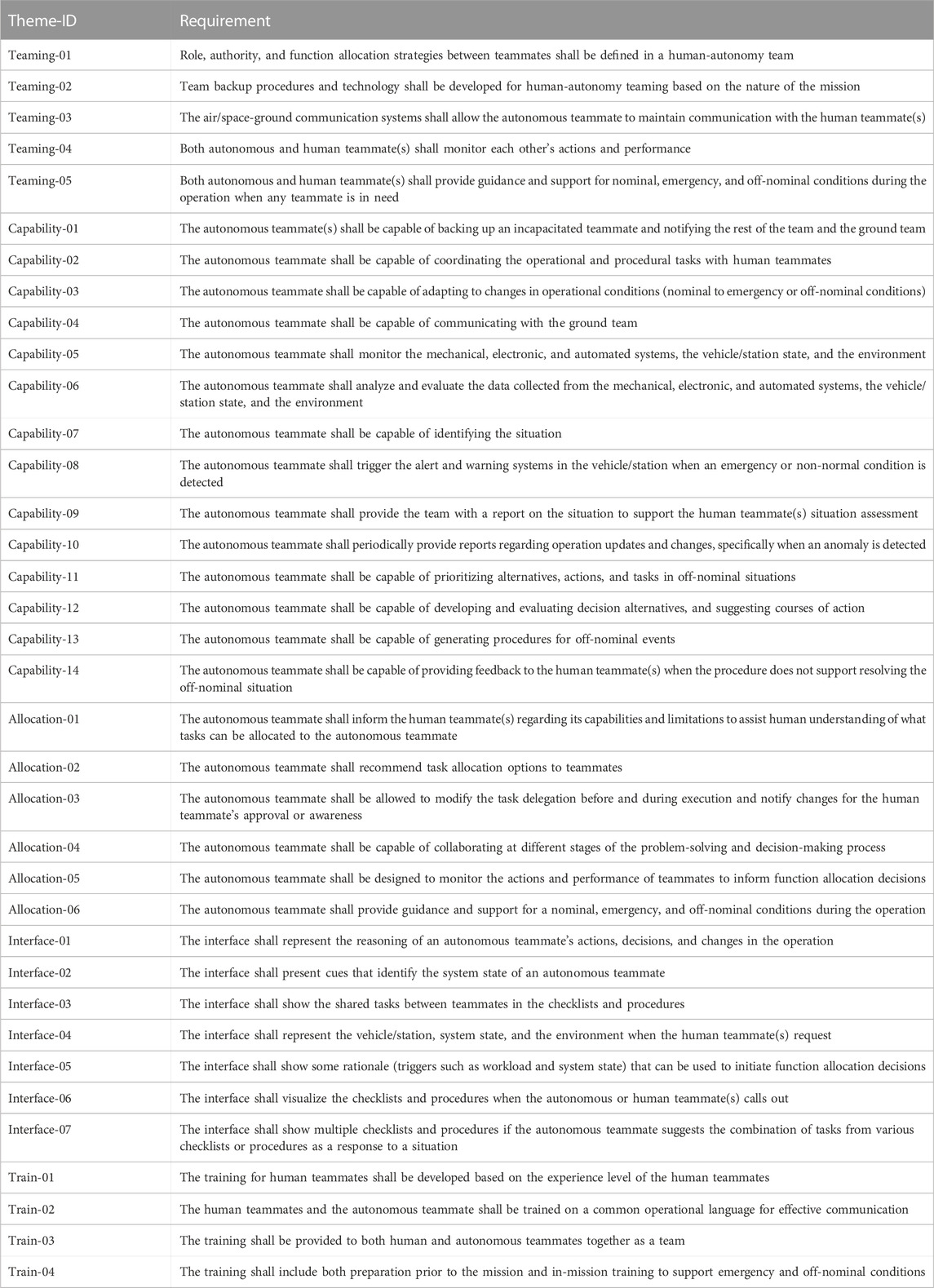
TABLE 3. Teaming, functional capabilities, allocation, training, and graphical user interface information exchange requirements.
Teaming-01. Teammates’ roles, tasks, and interactions should be strategized to maintain an optimal level of teammate engagement and situation awareness (Neis, 2020). As an example from aviation, crew resource management (CRM) methods developed for dual-pilot operations and would need to be re-designed for the mixed, flexible, and distributed HAT within SPO (Johnson, et al., 2012; Comerford et al., 2013; Faulhaber et al., 2022). CRM focuses on improving teamwork through communication and interactions between the flight crew members and ground personnel and how teams can reduce errors and make safer decisions (Salas et al., 2006; National Business Aviation Association, 2023). The autonomous teammate should follow current CRM best practices (Matessa et al., 2017).
Teaming-02; Capability-01. In HAT, a backup strategy should be identified to manage failures, errors, and incapacitation cases. In the space mission beyond LEO case study, there is a strong need for a backup system when the collaborative work between the crews on Earth and in space is interrupted (Tokadlı and Dorneich, 2018). For example, in one case study, human teammates expected an autonomous teammate to monitor crew health conditions and performance and detect and notify them if a teammate becomes incapacitated. In the case of a human teammate’s incapacitation, the autonomous teammate should initiate a backup strategy and take over tasks. If the autonomous teammate cannot perform any allocated task(s), it should assist in allocating the task to another human.
Teaming-03; Capability-04, 05, 07, and 09. To provide sufficient support while performing safety-critical tasks, ground and flight/space crews should be allowed to communicate as freely as needed (Johnson, et al., 2012; Neogi et al., 2016). Like all-human teaming, communication in HAT is a necessary and effective tool to improve team performance while exchanging information and monitoring each other’s actions (Shively et al., 2017; Faulhaber et al., 2022). Effective communication can support human teammates’ trust, including autonomous agents explaining their actions or decision-making process (Le Vie et al., 2021; Zhang et al., 2021; Faulhaber et al., 2022). Autonomous systems should be able to engage their teammates with non-verbal communication to provide contextual information using social cues (Chan, et al., 2021). However, HAT may necessitate additional communications requirements for safety-critical tasks, including potential monitoring and risk management requirements to make real-time decisions (Comerford, et al., 2013; Neogi et al., 2016). Technology must support the coordination between the air and the ground. For example, the autonomous teammate in the SPO cockpit should be capable of communicating vehicle-to-vehicle and ground-to-vehicle to send and receive brief safety messages and execute lost link procedures if voice communication cannot be established (Goodrich et al., 2016).
Teaming-04; Capability-07. Humans and autonomous systems should monitor each other to identify possible failures (Zhang et al., 2021). An autonomous teammate could monitor the pilot’s capabilities and health to detect pilot incapacitation and intervene to safely land the aircraft (Comerford et al., 2013; Matessa et al., 2017). The human pilot should still be required to monitor the autonomous system to deal with errors due to potential sensor failures (Zhang et al., 2021). Alternatively, in some off-nominal or contingency situations, the autonomous teammate may not always be capable of performing all of its tasks. If necessary, a pilot should be alerted to take over the tasks (Matessa et al., 2017).
Teaming-05. The guidance of autonomous teammates could be categorized as crew, task, and maintenance assistance. To assist the crew, the autonomous teammate could monitor the crew, vehicle, environment, and mission states (ref. Capability-05 and Teaming-04) and provide updates and notify them if any action is necessary (ref. Capability-10). In both case studies, one teammate needs to read the step-by-step checklist tasks aloud to guide the other teammate during execution. Today’s space operations heavily rely on real-time guidance and support from the Mission Control Center on Earth. For example, this might include task-specific guidance during extravehicular activities (Clancey, 2003; McCann et al., 2005) or resolution support during off-nominal and emergency conditions (Love and Reagan, 2013; Fischer and Mosier, 2014). In space missions beyond LEO, the autonomous teammate could execute extravehicular activities or provide step-by-step action guidance to the human astronaut to accomplish the activity since Mission Control cannot perform this duty. For example, Mission Control supports maintenance issues by guiding the space crew to properly and quickly locate the issues in the space station structures, based on Mission Control’s ability to monitor the entire system’s health. With the communication delay, this can be delegated to an autonomous teammate as well as providing information regarding situation severity and mitigations.
Capability-02; Allocation-01 to 06. The interaction paradigm should shift towards more dialog-based coordination and back-and-forth delegation decisions between human and autonomous teammates to maintain coordination in HAT. Allowing flexible coordination can be possible with a dynamic function/role allocation strategy. Allocating some tasks to the autonomous teammate (e.g., flap settings, landing gear settings) can allow human teammate(s) more time to focus on essential information and create fewer distractions (Faulhaber et al., 2022). For example, a single pilot and autonomous system should fluidly hand over tasks to each other depending on the situation (Zhang et al., 2021). Like a human teammate, an autonomous teammate could dynamically modify task assignments between the human and autonomous teammates as conditions change (Johnson, 2010; Comerford et al., 2013; Neogi et al., 2016; Ho et al., 2017). However, allocating the tasks should be done with an awareness of each teammate’s capabilities and limitations (Ulusoy and Reisman, 2022). For example, a procedure should be categorized by autonomous system capabilities. Knowing what tasks the autonomous teammate can perform helps humans make better decisions, specifically when delegating tasks in emergencies.
Capability-11; Interface-03, 04, 06, and 07. A HAT should be able to manage resources and the impacts of unexpected events based on understanding the big picture (Chan et al., 2021). This necessitates that the autonomous teammate be capable of prioritizing tasks and plans during the mission. For example, the single pilot and autonomous teammate need to monitor and coordinate priorities (Comerford, et al., 2013). Some required SPO tasks of the autonomous teammate may include checklists, task reminders, challenge-and-response protocols, and recall of information or instructions provided by the single pilot, ATC personnel, or ground support (Matessa et al., 2017).
Capability-14. Checklists and procedures enable human teammates to form expectations of how the systems should respond at each step and to understand when the system does not respond as expected (Zhang et al., 2021). When the checklist or procedure task(s) does not help resolve the situation, the autonomous system should detect it and propose alternatives based on its system assessment.
Train-01 to 04. Current operational and crew training strategies should be adjusted to suit the HAT concept to help human teammates efficiently prepare for missions with the autonomous teammate(s). This adjustment includes considering training duration, experience level (novice vs. expert), and training methods. Insufficient training in HAT may result in trust issues (Karasinski et al., 2020). Pilots indicated they wanted to be aware of an autonomous teammate’s experience and knowledge level to calibrate better their expectations of its performance (Tokadlı et al., 2021a). Training programs of HAT could be supported with various steps. For example, a certification can indicate the human teammate’s expertise level (and maybe the autonomous system’s experience level). In SPO, novice pilots will lose the opportunity they had in dual-pilot operations to train with expert pilots. This type of apprenticeship training would need to be provided differently in SPO. In space operations, in-mission training might be provided for critical tasks when the crew cannot receive mission control support or has not encountered such a situation (Karasinski et al., 2020).
8 Conclusion
HAT is a promising concept to support complex operations; its integration requires human teammates to develop and maintain a shared mental model, situation awareness, mode awareness, and collaboration with the autonomous teammate. Conversely, it is less clear what functions and capabilities make an autonomous system truly a teammate. This paper detailed the capabilities and characteristics of HAT system in future aerospace concepts of operations to identify the gaps between the capabilities of today’s automation and what is needed in HAT. A systematic analysis of the empirical HAT studies in the literature and two previous case studies of future operations were synthesized to enumerate the common capabilities that are needed for autonomous system in teaming settings. Much of the current HAT literature has focused on the “perception of teammate-likeness” (Hugues et al., 2016; Haring et al., 2021; Tokadlı and Dorneich, 2022). In contrast, the goal of this work was to be concrete about the functions and capabilities that are needed for autonomous system to truly be considered and accepted as a teammate. Although rapidly developing with advances in sensors, artificial intelligence, and interaction technology, today’s automated systems do not yet approach the capabilities needed to realize HAT. The functions and capabilities identify the areas that need more development.
The HAT requirements and characteristics can inform future autonomous system development in aerospace operations to get closer to teammate-like systems. However, more work is necessary to verify and validate these requirements for HAT development and test them in realistic mission environments. Future work should focus on areas such as an autonomous teammate’s potential learning ability, core teaming processes (e.g., conflict, coaching, cognition), physical embodiment and social interactions. In more complex concept of operations, the social interaction skills with and without embodiment of the autonomous system in HAT should be examined to better understand the human perception of teammate likeness.
HAT is an important concept in many domains. This work focused only on the aerospace domain and was restricted to two use cases. Future work will be done to expand analysis to incorporate more use cases within aerospace (e.g., urban air mobility), and extend to other domains (e.g., healthcare, manufacturing).
Data availability statement
The original contributions presented in the study are included in the article further inquiries can be directed to the corresponding author.
Ethics statement
The studies involving human participants were reviewed and approved by Iowa State University Institutional Review Board. Patients/participants provided their written informed consent to participate in this study.
Author contributions
GT, MD contributed to conception and design of the study. GT executed the case studies and collected the data. GT conducted the literature review. GT wrote the first draft of the manuscript, under the supervision of MD. MD, GT both contributed to the revision process. MD provided editorial oversight throughout both the writing and revision processes. All authors contributed to the article and approved the submitted version.
Acknowledgments
We want to thank our SMEs and participants for their open discussion and the information they provided. Any opinions, recommendations, findings, or conclusion expressed herein are those of the authors.
Conflict of interest
The authors declare that the research was conducted in the absence of any commercial or financial relationships that could be construed as a potential conflict of interest.
Publisher’s note
All claims expressed in this article are solely those of the authors and do not necessarily represent those of their affiliated organizations, or those of the publisher, the editors and the reviewers. Any product that may be evaluated in this article, or claim that may be made by its manufacturer, is not guaranteed or endorsed by the publisher.
References
Abubshait, A., and Wiese, E. (2017). You look human, but act like a machine: Agent appearance and behavior modulate different aspects of human–robot interaction. Front. Psychol. 8, 1393. doi:10.3389/fpsyg.2017.01393
Alvarez, A. (2020). Human-autonomy teaming effects on workload, situation, awareness and trust. Long Beach: California State University.
Bainbridge, L. (1983). Ironies of automation. Automatica 19 (6), 129–135. doi:10.1016/0005-1098(83)90046-8
Barkouki, T., Deng, Z., Karasinski, J., Kong, Z., and Robinson, S. (2023). “XAI design goals and evaluation metrics for space exploration: A survey of human spaceflight domain experts,” in AIAA SCITECH 2023 Forum, Harbor, MD, January 23-27, 2023, 1828.
Barnes, M., Chen, J., and Jentsch, F. (2015). “Designing for mixed-initiative interactions between human and autonomous systems in complex environments,” in Systems, Man, and Cybernetics (SMC), 2015 IEEE International Conference, Hong Kong, China, October 9-12, 2015.
Chan, T., Kim, S. Y., Ramaswamy, B., Davidoff, S., Carrillo, J., Pena, M., et al. (2021). Human-computer interaction glow up: Examining operational trust and intention towards Mars autonomous systems. Available at: https://arxiv.org/abs/2110.15460 (Accessed October 28, 2021).
Chen, J., and Barnes, M. (2014). Human-agent teaming for multirobot control: A review of human factors issues. IEEE Trans. Human-Machine Syst. 44, 13–29. doi:10.1109/thms.2013.2293535
Clancey, W. J. (2003). “Agent interaction with human systems in complex environments: Requirements for automating the function of capcom in Apollo 17,” in AAAI Spring Symposium on Human Interaction with Autonomous Systems in Complex Environments, Palo Alto, CA, March 24–26, 2003.
Comerford, D., Brandt, S. L., Lachter, J. B., Wu, S.-C., Mogford, R. H., Battiste, V., et al. (2013). NASA's Single-pilot operations technical interchange meeting: Proceedings and findings. Available at: https://ntrs.nasa.gov/citations/20140008907 (Accessed April 1, 2013).
Conlon, N., Szafir, D., and Ahmed, N. (2022). “"I'm confident this will end poorly": Robot proficiency self-assessment in human-robot teaming,” in IEEE/RSJ International Conference on Intelligent Robots and Systems (IROS), Kyoto, Japan, October 23–27, 2022 (IEEE), 2127–2134.
Cummings, M. (2014). Man versus machine or man + machine? IEEE Intell. Syst. 29, 62–69. doi:10.1109/mis.2014.87
de Graaf, M. M., Ben Allouch, S., and Van Dijk, J. A. (2015). “What makes robots social?: A user’s perspective on characteristics for social human-robot interaction,” in Social Robotics: 7th International Conference, ICSR 2015, Paris, France, October 26-30, 2015 (Springer International Publishing), 184–193.
Degani, A., Heymann, M., Meyer, G., and Shafto, M. (2000). Some formal aspects of human-automation interaction. NASA Tech. Memorandum. Moffett Field, CA: NASA Ames Research Center, 209600.
Demir, M., McNeese, N. J., and Cooke, N. J. (2017a). Team situation awareness within the context of human-autonomy teaming. Cognitive Syst. Res. 46, 3–12. doi:10.1016/j.cogsys.2016.11.003
Demir, M., McNeese, N. J., and Cooke, N. J. (2017b). “Team synchrony in human-autonomy teaming,” in Nternational conference on applied human factors and ergonomics (Cham: Springer), 303–312.
Diehl, J. J., Crowell, C. R., Villano, M., Wier, K., Tang, K., and Riek, L. D. (2014). Clinical applications of robots in autism spectrum disorder diagnosis and treatment. Compr. guide autism, 411–422. doi:10.1007/978-1-4614-4788-7_14
Dorneich, M. C., Whitlow, S. D., Ververs, P. M., and Rogers, W. H. (2003). “Mitigating cognitive bottlenecks via an augmented cognition adaptive system,” in IEEE International Conference on Systems, Man and Cybernetics. Conference Theme-System Security and Assurance, Washington, DC, October 8, 2003, 937–944.
Dorneich, M., Dudley, R., Letsu-Dake, E., Rogers, W., Whitlow, S., Dillard, M., et al. (2017). Interaction of automation visibility and information quality in flight deck information automation. IEEE Trans. Human-Machine Syst. 47, 915–926. doi:10.1109/THMS.2017.2717939
Dorneich, M., Rogers, W., Whitlow, S., and DeMers, R. (2016). Human performance risks and benefits of adaptive systems on the flight deck. Int. J. Aviat. Psychol. 26, 15–35. doi:10.1080/10508414.2016.1226834
Faulhaber, A. K., and Friedrich, M. (2019). “Eye-tracking metrics as an indicator of workload in commercial single-pilot operations,” in Human Mental Workload: Models and Applications: Thirds International Symposium, H-WORKLOAD, Rome, Italy, November 14–15, 2019, 231–225.
Faulhaber, A., Friedrich, M., and Kapol, T. (2022). Absence of pilot monitoring affects scanning behavior of pilot flying: Implications for the design of single-pilot cockpits. Hum. Factors 64 (2), 278–290. doi:10.1177/0018720820939691
Feigh, K., Dorneich, M., and Hayes, C. (2012). Toward a characterization of adaptive systems A framework for researchers and system designers. Hum. Factors J. Hum. Factors Ergonomics Soc. 54, 1008–1024. doi:10.1177/0018720812443983
Fischer, U., and Mosier, K. (2014). “The impact of communication delay and medium on team performance and communication in distributed teams,” in Proceedings of the Human Factors and Ergonomics Society Annual Meeting, Chicago, IL, March 16 - 19, 2014 (Sage CA), 115–119.
Fleishman, E. A., Mumford, M. D., Zaccaro, S. J., Levin, K. Y., Korotkin, A. L., and Hein, M. B. (1991). Taxonomic efforts in the description of leader behavior: A synthesis and functional interpretation. Leadersh. Q. 2 (4), 245–287. doi:10.1016/1048-9843(91)90016-u
Gockley, R., Bruce, A., Forlizzi, J., Michalowski, M., Mundell, A., Rosenthal, S., et al. (2005). “Designing robots for long-term social interaction,” in 2005 IEEE/RSJ International Conference on Intelligent Robots and Systems, Edmonton, AB, Canada, August 2-6, 2005 (IEEE), 1338–1343.
Goodrich, K., Nickolaou, J., and Moore, M. (2016). “Transformational autonomy and personal transportation: Synergies and differences between cars and planes,” in 16th AIAA Aviation Technology, Integration, and Operations Conference, Washington, DC, June 13-17, 2016, 1–12.
Goodrich, M. (2013). “Multitasking and multi-robot,” in The Oxford handbook of cognitive engineering (Oxford, UK: Oxford University Press), 379.
Goodrich, M. (2010). “On maximizing fan-out: Towards controlling multiple unmanned vehicles,” in Human-robot Interactions in future military operations (Farnham, UK: Ashgate Publishing).
Hackman, J. R., and Wageman, R. (2005). A theory of team coaching. Acad. Manag. Rev. 30 (2), 269–287. doi:10.5465/amr.2005.16387885
Hardin, B., and Goodrich, M. (2009). “On using mixed-initiative control: A perspective for managing large-scale robotic teams,” in Proceedings of the 4th ACM/IEEE international conference on Human robot interaction, La Jolla, CA, March 11-13, 2009.
Haring, K. S., Satterfield, K. M., Tossell, C. C., De Visser, E. J., Lyons, J. R., Mancuso, V. F., et al. (2021). Robot authority in human-robot teaming: Effects of human-likeness and physical embodiment on compliance. Front. Psychol. 12, 625713. doi:10.3389/fpsyg.2021.625713
Hayashi, K., Shiomi, M., Kanda, T., and Hagita, N. (2010). “Who is appropriate? A robot, human and mascot perform three troublesome tasks,” in 19th international symposium in robot and human interactive communication, Viareggio, Italia, September 13-15, 2010 (IEEE), 348–354.
Ho, N., Johnson, W., Panesar, K., Wakeland, K., Sadler, G., Wilson, N., et al. (2017). “Application of human-autonomy teaming to an advanced ground station for reduced crew operations,” in AIAA/IEEE Digital Avionics Systems Conference - Proceedings, 2017, St. Petersburg, FL, September 17-21, 2017.
Hugues, O., Weistroffer, V., Paljic, A., Fuchs, P., Karim, A. A., Gaudin, T., et al. (2016). Determining the important subjective criteria in the perception of human-like robot movements using virtual reality. Int. J. humanoid robotics 13 (2), 1550033. doi:10.1142/s0219843615500334
International Space Exploration Coordination Group (2017). Scientific opportunities enabled by human exploration beyond low Earth orbit.
Iroju, O., Ojerinde, O. A., and Ikono, R. (2017). State of the art: A study of human-robot interaction in healthcare. Int. J. Inf. Eng. Electron. Bus. 3 (3), 43–55. doi:10.5815/ijieeb.2017.03.06
Johnson, E. (2010). Methodology to support dynamic function allocation policies between humans and flight deck automation. Available at: https://ntrs.nasa.gov/citations/20120009645 (Accessed June 1, 2012).
Johnson, W., Comerford, D., Lachter, J., Battiste, V., Feary, M., and Mogford, R. (2012). “HCI aero 2012 task allocation for single pilot operations: A role for the ground,” in HCI Aero 2012, Brussels, September 12-14, 2012.
Kaber, D., Omal, E., and Endsley, M. (1999). Level of automation effects on telerobot performance and human operator situation awareness and subjective workload. Automation Technol. Hum. Perform. doi:10.1080/001401399185595
Kaliardos, W. N. (2022). “Enough fluff: Returning to meaningful perspectives on automation,” in 2022 IEEE/AIAA 41st Digital Avionics Systems Conference (DASC), Portsmouth, VA, September 18-22, 2022 (IEEE), 1–9.
Karasinski, J., Holder, S., Robinson, S., and Marquez, J. (2020). Deep space human-systems research recommendations for future human-automation/robotic integration. Available at: https://ntrs.nasa.gov/citations/20205004361 (Accessed June 1, 2020).
Kidd, C. D., and Breazeal, C. (2008). “Robots at home: Understanding long-term human-robot interaction,” in 2008 IEEE/RSJ International Conference on Intelligent Robots and Systems, Nice, France, September 22-26, 2008 (IEEE), 3230–3235.
Locomation, Inc. (2022). Voluntary safety self-assessment (VSSA). Available at: https://locomation.ai/wp-content/uploads/2022/05/Locomation_VSSA_Spring_2022.pdf.
Le Vie, L., Barrows, B. A., Allen, B. D., and Alexandrov, N. (2021). “Exploring multimodal interactions in human-autonomy teaming using a natural user interface,” in AIAA scitech 2021 forum (AIAA), 1685. doi:10.2514/6.2021-1685
Lee, J. D., and See, K. A. (2004). Trust in automation: Designing for appropriate reliance. Hum. Factors 46 (1), 50–80. doi:10.1518/hfes.46.1.50_30392
Leite, I., Martinho, C., and Paiva, A. (2013). Social robots for long-term interaction: A survey. Int. J. Soc. Robotics 5, 291–308. doi:10.1007/s12369-013-0178-y
Lohse, M., Hegel, F., and Wrede, B. (2008). Domestic applications for social robots: An online survey on the influence of appearance and capabilities. J. Phys. Agents 2 (2), 21–32. doi:10.14198/JoPha.2008.2.2.04
Love, S. G., and Reagan, M. L. (2013). Delayed voice communication. Acta Astronaut. 91, 89–95. doi:10.1016/j.actaastro.2013.05.003
Lyons, J., Sycara, K., Lewis, M., and Capiola, A. (2021). Human-autonomy teaming: Definitions, debates, and directions. Front. Psychol. 12, 589585. doi:10.3389/fpsyg.2021.589585
Martini, M. C., Gonzalez, C. A., and Wiese, E. (2016). Seeing minds in others–Can agents with robotic appearance have human-like preferences? PLOS ONE 11 (2), e0149766. doi:10.1371/journal.pone.0146310
Matessa, M., Strybel, T., Vu, K., Battiste, V., and Schnell, T. (2017). Concept of operations for RCO/SPO. Available at: https://ntrs.nasa.gov/citations/20170007262 (Accessed July 10, 2017).
Matessa, M. (2018). “Using a crew resource management framework to develop human-autonomy teaming measures,” in Advances in Neuroergonomics and Cognitive Engineering, AHFE 2017. Adv. Intelligent Syst. Comput. (Cham, CH: Springer International) 586, 46–57. doi:10.1007/978-3-319-60642-2_5
McCann, R., McCandless, J., and Hilty, B. (2005). “Automating vehicle operations in next-generation spacecraft: human factors issues,” in Space 2005, Long Beach, CA, August 30–September 1, 2005, 1–14.
McDermott, P., Dominguez, C., Kasdaglis, N., Ryan, M., Trahan, I., and Nelson, A. (2018). Human-machine teaming systems engineering guide. Available at: https://www.mitre.org/news-insights/publication/human-machine-teaming-systems-engineering-guide(Accessed December 13, 2018).
McNeese, N. J., Demir, M., Cooke, N. J., and Myers, C. (2018). Teaming with a synthetic teammate: Insights into human-autonomy teaming. Hum. Factors 60 (2), 262–273. doi:10.1177/0018720817743223
Miller, C. A., and Funk, H. B. (2001). “Associates with etiquette: meta-communication to make human-automation interaction more natural, productive and polite,” in Proceedings of the 8th european conference on cognitive science approaches to process control, Munich, Germany, September 24–26, 2001, 24–26.
Miller, C. A., Funk, H., Wu, P., Goldman, R., Meisner, J., and Chapman, M. (2005). “The playbook approach to adaptive automation,” in Proceedings of the Human Factors and Ergonomics Society Annual Meeting, Orlando, FL, September 1, 2005 (Sage Publications), 15–19.
Miller, C. A., Goldman, R., Funk, H., Wu, P., and Pate, B. (2004). “A playbook approach to variable autonomy control: Application for control of multiple, heterogeneous unmanned air vehicles,” in Proceedings of FORUM 60, the Annual Meeting of the American Helicopter Society, Baltimore, MD, June 7-10, 2004, 7–10.
Miller, C. A., and Parasuraman, R. (2007). Designing for flexible interaction between humans and automation: Delegation interfaces for supervisory control. Hum. Factors 49 (1), 57–75. doi:10.1518/001872007779598037
Miller, C., Pelican, M., and Goldman, R. (1999). “"Tasking" interfaces for flexible interaction with automation: Keeping the operator in control,” in International Conference on Intelligent User Interfaces, Los Angeles CA, January 5 - 8, 1999.
National Business Aviation Association (2023). Crew resource management. Available at: https://nbaa.org/aircraft-operations/safety/human-factors/crew-resource-management/.
Nahavandi, S., Mohamed, S., Hossain, I., Nahavandi, D., Salaken, S. M., Rokonuzzaman, M., et al. (2022). Autonomous convoying: A survey on current research and development. IEEE Access 10, 13663–13683. doi:10.1109/access.2022.3147251
Neis, S. M. (2020). Evaluation of pilot vigilance during c ruise towards the implementation of reduced crew operations. Darmstadt: Technische Universität Darmstadt.
Neogi, N. A. (2016). “Capturing safety requirements to enable effective task allocation between humans and automaton in increasingly autonomous systems,” in 16th AIAA Aviation Technology, Integration, and Operations Conference, Washington, DC, 13-17 June 2016, 3594.
O’Neill, T., McNeese, N., Barron, A., and Schelble, B. (2022). Human–autonomy teaming: A review and analysis of the empirical literature. Hum. factors 64 (5), 904–938. doi:10.1177/0018720820960865
Park, H. S. (2013). “From automation to autonomy—a new trend for smart manufacturing,” in DAAAM International Scientific Book. Vienna, Austria: DAAAM International, 75–110.
Patel, J., Dorneich, M. C., Mott, D., Bahrami, A., and Giammanco, C. (2013). Improving coalition planning by making plans alive. IEEE Trans. Intelligent Syst. 28 (1), 17–25. doi:10.1109/mis.2012.88
Rasmussen, J. (1986). Information processing and human-machine interaction: An approach to cognitive engineering. New York, NY: Elsevier Science.
Sabelli, A., Kanda, T., and Hagita, N. (2011). “A conversational robot in an elderly care center: An ethnographic study,” in Proceedings of the 6th international conference on human-robot interaction, Lausanne Switzerland, March 6 - 9, 2011 (ACM), 37–44.
Salas, E., Cooke, N. J., and Rosen, M. A. (2008). On teams, teamwork, and team performance: Discoveries and developments. Hum. Factors 50 (3), 540–547. doi:10.1518/001872008x288457
Salas, E., Rico, R., and Passmore, J. (2017). “The psychology of teamwork and collaborative processes,” in The wiley blackwell handbook of the psychology of team working and collaborative processes. Editors E. Salas, R. Rico, and J. Passmore (Hoboken, NJ: Wiley Blackwell), 1.
Salas, E., Shuffler, M. L., Thayer, A. L., Bedwell, W. L., and Lazzara, E. H. (2015). Understanding and improving teamwork in organizations: A scientifically based practical guide. Hum. Resour. Manag. 54 (4), 599–622. doi:10.1002/hrm.21628
Salas, E., Wilson, K. A., Burke, C. S., and Wightman, D. C. (2006). Does crew resource management training work? An update, an extension, and some critical needs. Hum. factors 48 (2), 392–412. doi:10.1518/001872006777724444
Shively, J. (2017). Remotely piloted aircraft systems panel (RPASP) working paper: Autonomy and automation. Available at: https://ntrs.nasa.gov/citations/20170011326 (Accessed June 19, 2017).
Shively, R. J., Brandt, S. L., Lachter, J., Matessa, M., Sadler, G., and Battiste, H. (2016). “Application of human-autonomy teaming (HAT) patterns to reduced crew operations (RCO),” in International Conference on Engineering Psychology and Cognitive Ergonomics, Las Vegas, NV, 15-20 July, 2016, 244–255.
Shively, R. J., Lachter, J., Brandt, S. L., Matessa, M., Battiste, V., and Johnson, W. W. (2017). “Why human-autonomy teaming?,” in International conference on applied human factors and ergonomics (Cham: Springer), 3–11.
Stanton, N. A., Salmon, P. M., Walker, G. H., Baber, C., and Jenkins, D. P. (2005). “Process charting methods: Decision action Diagram (DAD),” in Human factors methods: A practical guide for engineering and design (Farnham, UK: Ashgate), 127–130.
The MITRE Corporation (2014). Systems engineering guide. McLean, VA: MITRE Corporate Communications and Public Affairs.
Tokadlı, G., and Dorneich, M. C. (2022). Autonomy as a teammate: Evaluation of teammate-likeness. J. Cognitive Eng. Decis. Mak. 16 (4), 282–300. doi:10.1177/15553434221108002
Tokadlı, G., and Dorneich, M. C. (2018). Development of design requirements for a cognitive assistant in space missions beyond low Earth orbit. J. Cognitive Eng. Decis. Mak. 12 (2), 131–152. doi:10.1177/1555343417733159
Tokadlı, G., Dorneich, M. C., and Matessa, M. (2021a). Toward human–autonomy teaming in single-pilot operations: Domain analysis and requirements. J. Air Transp. 29 (4), 142–152. doi:10.2514/1.d0240
Tokadlı, G., Dorneich, M., and Matessa, M. (2021b). Evaluation of playbook delegation approach in human-autonomy teaming for single pilot operations. Int. J. Human-Computer Interact. 37 (7), 703–716. doi:10.1080/10447318.2021.1890485
Turk, W. (2006). Writing Requirements for Engineers [good requirement writing]. Eng. Manag. 16 (3), 20–23. doi:10.1049/em:20060304
Ulusoy, U., and Reisman, G. (2022). Human-autonomy teaming for task execution support in next generation deep space habitats. Available at: https://custom.cvent.com/5802E71AA4E24C3FA9A1B3F099BBD52D/files/a79b1e1318854cb4894aebdc39632740.pdf.
Vagia, M., Transeth, A., and Fjerdingen, S. (2016). A literature review on the levels of automation during the years. What are the different taxonomies that have been proposed? Appl. Ergon. 53, 190–202. doi:10.1016/j.apergo.2015.09.013
Vicente, K. (1999). Cognitive Work Analysis: Toward safe, productive, and healthy computer-based work. Available at: http://www.ncbi.nlm.nih.gov/pubmed/21862397.
Wada, K., and Shibata, T. (2007). Living with seal robots—Its sociopsychological and physiological influences on the elderly at a care house. IEEE Trans. robotics 23 (5), 972–980. doi:10.1109/tro.2007.906261
Weiss, H., Patel, A., Romano, M., Apodoca, B., Kuevor, P., Atkins, , et al. (2022). “Methods for evaluation of human-in-the-loop inspection of a space station mockup using a quadcopter,” in IEEE Aerospace Conference (AERO), Big Sky, MT, March 05-12, 2022 (IEEE), 1–12.
Wiese, E., Buzzell, G. A., Abubshait, A., and Beatty, P. J. (2018). Seeing minds in others: Mind perception modulates low-level social-cognitive performance and relates to ventromedial prefrontal structures. Cognitive, Affect. Behav. Neurosci. 18 (5), 837–856. doi:10.3758/s13415-018-0608-2
Woods, D. D. (2016). The risks of autonomy. J. Cognitive Eng. Decis. Mak. 10, 131–133. doi:10.1177/1555343416653562
Keywords: human-machine teaming, cognitive assistant, playbook, single pilot operations, reduced crew operations, space operations
Citation: Tokadlı G and Dorneich MC (2023) Comparison and synthesis of two aerospace case studies to develop human-autonomy teaming requirements. Front. Aerosp. Eng. 2:1214115. doi: 10.3389/fpace.2023.1214115
Received: 28 April 2023; Accepted: 06 July 2023;
Published: 18 July 2023.
Edited by:
Tejas Puranik, National Aeronautics and Space Administration, United StatesReviewed by:
Max Mulder, Delft University of Technology, NetherlandsRaunak Bhattacharyya, University of Oxford, United Kingdom
Ankit Shah, Brown University, United States
Copyright © 2023 Tokadlı and Dorneich. This is an open-access article distributed under the terms of the Creative Commons Attribution License (CC BY). The use, distribution or reproduction in other forums is permitted, provided the original author(s) and the copyright owner(s) are credited and that the original publication in this journal is cited, in accordance with accepted academic practice. No use, distribution or reproduction is permitted which does not comply with these terms.
*Correspondence: Michael C. Dorneich, ZG9ybmVpY2hAaWFzdGF0ZS5lZHU=