- Peking University HuiLongGuan Clinical Medical School, Beijing HuiLongGuan Hospital, Beijing, China
Background: The high prevalence of Internet gaming disorder among children and adolescents and its severe psychological, health, and social consequences have become a public emergency. A high efficiency and cost-effective early recognition method are urgently needed.
Objective: We aim to develop and internally validate a nomogram model for predicting Internet gaming disorder (IGD) risk in Chinese adolescents and children.
Methods: Through an online survey, 780 children and adolescents aged 7–18 years who participated in the survey from June to August 2021 were selected. The least absolute shrinkage and selection operator regression model was used to filter the factors. Multivariate logistic regression analysis was used to establish the prediction model and generate nomograms and a website calculator. The area under the receiver operating characteristic curve, calibration plot, and decision curve analysis were used to evaluate the model's discrimination, calibration, and clinical utility. Bootstrapping validation was used to verify the model internally.
Results: Male sex and experience of game consumption were the two most important predictors. Both models exhibited good discrimination, with an area under the curve >0.80. The calibration plots were both close to the diagonal line (45°). Decision curve analyses revealed that two nomograms were clinically useful when the threshold probability for the intervention was set to 5–75%.
Conclusion: Two prediction models appear to be reliable tools for Internet gaming disorder screening in children and adolescents, which can also help clinicians to personalize treatment plans. Moreover, from the standpoint of simplification and cost, Model 2 appears to be a better alternative.
Introduction
Internet gaming disorder (IGD) was listed as a clinical phenomenon requiring further research by the Diagnostic Statistical Manual of Mental Disorders (Fifth Edition, DSM-5) in 2013 (1). Compared with adults, children and adolescents have a higher prevalence of IGD, by more than 20%, in many regions (2, 3). At present, the diagnosis of IGD is usually determined by doctors There have been many studies on the predictors of IGD. For example, Rho (4) found that greater amounts of time gaming, video game consumption experience, and single marital status were important predictors of IGD. Estévez (5) pointed out that difficulty in emotion regulation and lower peer attachment have predictive effects on problematic Internet gaming. In a survey conducted in a Korean population, Young Choi found that high impulsivity, high game cost, and long gaming time during workdays were significant risk factors for IGD (6). Jeong et al. (7) found that longer average daily gaming time, playing multiplayer games, depressive symptoms, and hyperactivity symptoms were independent risk factors for IGD. A longitudinal study conducted by Wartberg et al. (8) found that male sex, severe self-esteem problems, and severe hyperactivity symptoms are predictors of problematic online gaming behavior. In addition to the above, several other studies focusing on risk factors for IGD support that many variables are significantly associated with the development of IGD, including video game cost (9, 10), gaming time (11, 12), difficulty in emotion regulation (13), higher impulsivity (11, 14, 15), hyperactivity symptoms (16–19), self-esteem problems (19–21), playing multiplayer games (22, 23), emotional symptoms (24, 25), peer relationship problems (26–28), family relationship problems (29, 30), and male sex (2, 12, 31–33), among others. Therefore, we collected information on these variables, with age and lifestyle factors (which we believe may be related to family support) as candidate predictors.
To our knowledge, no prediction model has been developed to assess the risk of IGD in children and adolescents. Therefore, this study established a prediction model and conducted an internal validation to identify individuals at high risk of IGD, which is helpful in implementing the targeted intervention promptly.
Materials and Methods
Participants
From June 2021 to August 2021, we recruited participants through convenient sampling and online survey. The inclusion criteria were as follows: (1) Chinese adolescents who voluntarily participated in the study; (2) Age 7–18 years old; (3) Could use mobile phones independently (IOS or Android system); (4) Participants and guardians were required to submit informed consent online. The exclusion criteria were: (1) not completing all questionnaires; (2) exceeding the response duration (mean ± three standard deviations). This study was approved by the ethics committee of Beijing Huilongguan Hospital. All subjects were informed of the study plan before enrollment and submitted informed consent online.
Measures
Self-Administered Video Game Habit Questionnaire
We used a self-administered questionnaire on gaming habits to identify predictors related to video gaming among participants. The questionnaire included seven items: the age at the start of contact with video games, the age at the start of habitually playing video games, the average daily video game time in the preceding 6 months, the number of frequently played games (1–2 / 3 and above), the main ways of playing games (team games/single-player games), whether there was an experience of video game consumption (no/yes), and percentage of monthly game consumption in total expenditure (<20% / ≥20%).
IGD Scale
The outcome variable in the model is IGD, which is self-rated by the IGD scale. The scale was compiled by Pontes et al. (34), according to the diagnostic criteria of IGD proposed in the DSM-V. After being translated and appropriately modified by Chinese scholar Hong et al. (35) a Chinese version of the IGD Scale was developed The scale has good reliability and validity, with a Cronbach's α coefficient of 0.90 and a split-half reliability coefficient of 0.88 (35). There are nine items rated on a 5-point Likert-type scale ranging from 1 (never) to 5 (always). Those with a total score >21 (36) were defined as having IGD.
Strength and Difficulties Questionnaire
The questionnaire was compiled by American psychologist Robert Goodman in 1997 (37), and later translated into Chinese by the Shanghai Mental Health Center (38). The subscales of emotional problems, hyperactivity, and peer problems were used to evaluate the subjects. Each subscale had five items, with a score of 0–2 (non-conforming, slightly conforming, and fully conforming), ranging from 0 to 10. Higher scores indicated more severe difficulties. The Cronbach's α coefficient of the questionnaire was 0.79 (38).
The Self-Esteem Scale
The Self-Esteem Scale was originally created by Rosenberg (39). After being translated and revised by Ji et al. (40), a Chinese version was developed. This scale was used to evaluate the degree of self-esteem. The scale has 10 items, which are rated from 1 to 4 (very inconsistent, inconsistent, consistent, and very consistent) for a total score ranging from 10–40. A higher score indicates a higher degree of self-esteem. The split-half reliability coefficient of the scale was 0.96, the test-retest reliability coefficient was 0.78, and the criterion validity coefficient was 0.52 (41).
The Difficulties in Emotion Regulation Scale
DERS was compiled by Gratz and Roemer (42) and later translated into Chinese by Wang et al. (43). The scale includes six dimensions: lack of emotional clarity (clarity, five items), impulse control difficulties (impulse, six items), difficulties in goal-directed behavior when emotionally present (goals, five items), difficulty in accepting emotional responses (non-acceptance, six items), limited access to emotion regulation strategies (strategies, eight items), and lack of emotional awareness (awareness, five items). Items are rated on a 5-point Likert scale ranging from 1 (almost never) to 5 (almost always). Higher scores indicate more severe difficulties of emotion regulation. Cronbach's α coefficient of the scale was between 0.88 and 0.96, and the test-retest reliability was between 0.52 and 0.77 (44).
Brief Sensation Seeking Scale–Chinese Version
The BSSS was developed by Hoyle (45) and later translated into Chinese by Chen et al. (46). The scale is used to evaluate an individual's sensory seeking traits, including four dimensions: experience seeking, boredom susceptibility, thrill and adventure seeking, and disinhibition. Each dimension has two items, which are rated using a 5-point scale (1 = completely inconsistent, 5 = completely consistent) for a total score of 2–10. Higher scores indicate more pronounced traits. The Cronbach's α coefficient was 0.82, and the test-retest reliability coefficient was 0.69 (46).
Family Environment Scale-Chinese Version
In 1981, the Family Environment Scale was compiled by Moss and Moss (47). Fei et al. (48) translated it into Chinese in 1991. The subscales of cohesion, expressiveness, and conflict were selected to evaluate the participants. Each subscale had nine true-false questions. The subscale scores ranged from 0–9, with higher scores indicating more noticeable family's environmental characteristics. The reliability of each scale dimension was approximately 0.6 (49).
Statistical Analysis
Statistical analysis was performed using Empowerstats software and R software 4.1.1. Relevant software packages include rms, rmda, pROC, DynNom, and pmsampsize. Continuous variables are expressed as median (range) and were compared using the Mann–Whitney U test. In addition, the χ2 test was used to calculate statistical differences in categorical variables.
The least absolute shrinkage and selection operator (LASSO) regression was used to screen the predictors. The idea is to construct a penalty function to compress the regression coefficients of variables and shrink some variable coefficients to zero to achieve the screening of variables. Lambda is the penalty coefficient filtered through a 10-fold cross-validation (Figure 1A). Lambda.min refers to the penalty coefficient that minimizes the average error of the model. Log (lambda.min) = −4.15, draws the vertical line, and the number of variables screened was 14. Lambda.1se refers to the maximum penalty coefficient that makes the average error of the model within one standard deviation of the minimum value. Log (lambda.1se) = −3.04, draw a vertical line, and the number of variables screened is 8. To make the model error within the acceptable range and thus make the model concise and convenient for clinical use, lambda.1se was selected as the optimal penalty coefficient (Figure 1B).
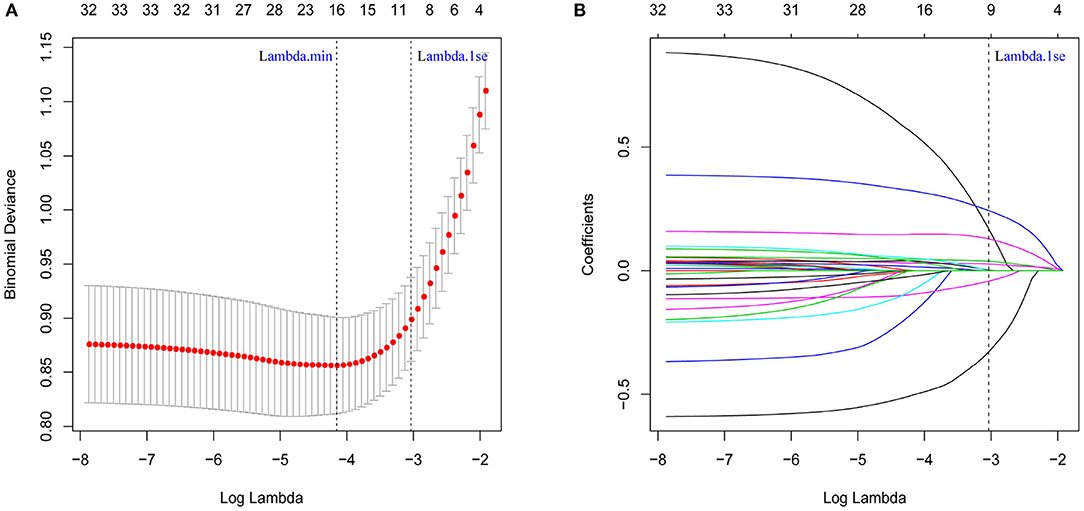
Figure 1. Determination of Internet Gaming disorder risk factors in children and adolescents by least absolute shrinkage and selection operator (LASSO) regression analysis. (A) The cross-validation for LASSO regression, where the parameter lambda was adjusted to find the best function set, is shown. The vertical dotted line on the left panel represents the log (lambda) corresponding to the optimal lambda. (B) The coefficients of predictors changed with lambda. The vertical dotted line in the right panel corresponds to the eight features selected with non-zero LASSO cross-validation coefficients.
The predictors screened by LASSO regression were included in the multivariate logistic regression analysis. Model 1 (complete) and Model 2 (simplified) were established. A logistic regression model was used to create a nomogram to predict the risk of IGD. The model's performance was internally validated using the bootstrap self-sampling method (k = 1,000). The area under the receiver operating characteristic curve (AUC) was used to evaluate the discrimination of the model. The closer the AUC is to 1, the better the model's discrimination. The Delong method was used to compare the difference between the two models. A calibration plot was used to evaluate the calibration of the model (50). Ideally, the calibration plot was a straight line with a slope of 1 and an intercept of 0. The more consistent the calibration plot of the model is with the reference line, the higher the calibration degree of the model. Decision curve analysis (51) was used to evaluate the clinical usefulness of the nomogram by calculating the net benefit under different threshold probabilities. The net benefit equals the true-positive rate multiplied by the gain of receiving treatment minus the false-positive rate multiplied by the loss of receiving treatment. Statistical significance was set at P < 0.05.
Results
Participant Characteristics
According to the IGD Scale scores, participants were divided into the IGD (n = 190, 24.3%) and non IGD (n = 590, 75.5%) groups. The IGD group included 124 boys and 66 girls, aged 7–18 years, with an average age of 14.4 years and an average length of education of 8.3 years. The non-IGD group included 265 boys and 325 girls, aged 7 to 18 years, with an average age of 14.3 years and an average length of education of 8.3 years. Table 1 lists the detailed demographics, video game use, and psychological characteristics.
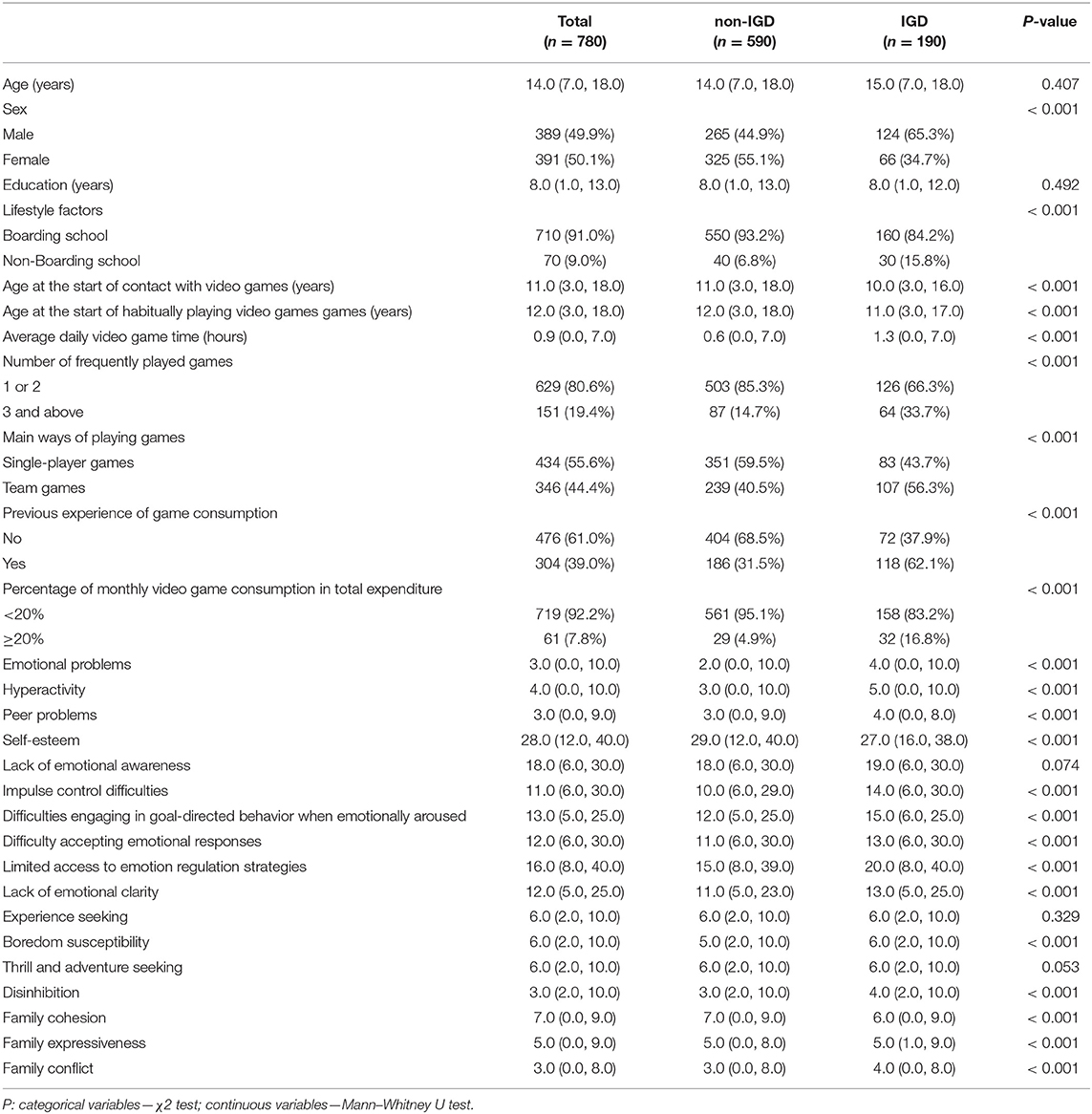
Table 1. Comparison of clinical characteristics between participants with and without IGD [n (%), median (range)].
Development of Nomograms for IGD Risk Prediction
Based on lasso regression analysis, the following eight predictors were selected: male sex, average daily video game time, experience of video game consumption, hyperactivity, age at the start of contact with video games (years), impulse control difficulties, limited access to emotion regulation strategies, and disinhibition.
Logistic regression was used to establish prediction models. Model 1 includes the above eight predictors, as presented in Table 2. Among them, male sex (OR = 2.17; 95%CI 1.42–3.32) and having video game consumption experience (OR = 1.93; 95% CI1.29–2.90) were the two most important predictors. To further simplify the model and keep the AUC value above 0.8, after constructing the model using different combinations of predictors, Model 2 was selected. Model 2 includes only four predictors: male sex, average daily video game time, having video game consumption experience, and hyperactivity, as presented in Table 2. Male sex (OR = 2.06; 95%CI 1.39–3.04) and having video game consumption experience (OR = 2.10; 95%CI 1.42–3.08) remained the two most important predictors.
Nomograms were established for Models 1 and 2 to calculate IGD risk, as presented in Figure 2. At the same time, based on model 2, an IGD risk website calculator was developed, as shown in Figure 3. Scan the QR code in Figure 3A or visit the website to gain free access to the calculator: http://www.empowerstats.net/pmodel/?m=22029_IGDpredictionmodel. The interactive user interface is shown in Figure 3B.
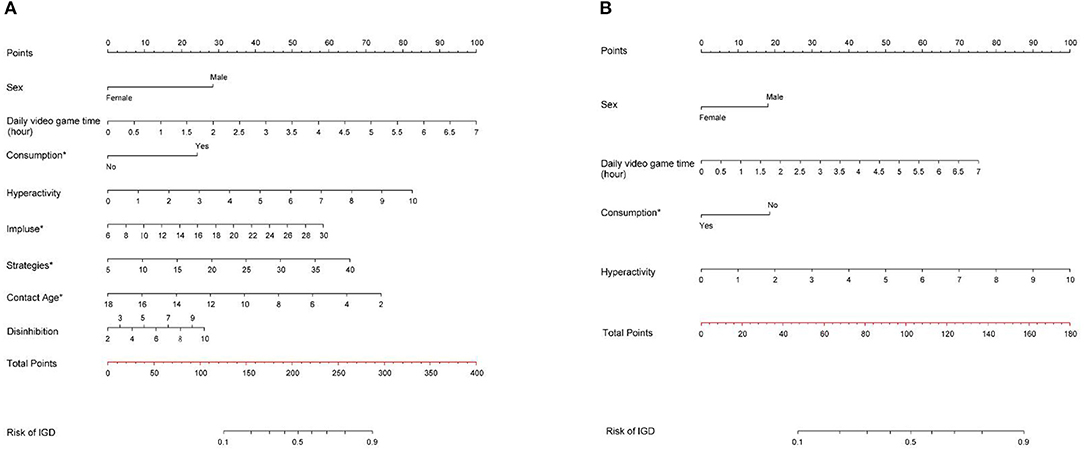
Figure 2. Nomograms for predicting the risk of Internet gaming disorder in children and adolescents. (A) Model 1; (B) Model 2. Consumption*: Experience of video game consumption; Impulse*: Impulse control difficulties; Strategies*: limited access to emotion regulation strategies; Contact age*: Age at the start of contact with video games.
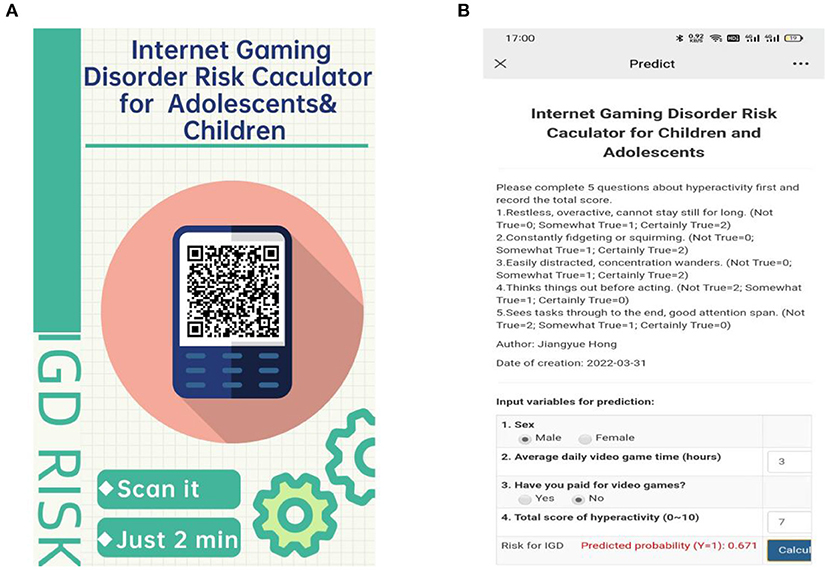
Figure 3. Internet gaming disorder risk calculator for adolescents and children. (A) QR (quick response) code poster of website calculator. (B) Interactive user interface.
Validation of Nomograms for IGD Risk Prediction
The internal bootstrap validation demonstrated that the Model 1-derived curve fits well with the ideal curve with a probability of 0 and 0.70. However, when the probability was set to >0.70, Model 1 could overestimate the probability of IGD (Figure 4A). Model 2 had a similar tendency; however, the start point of overestimation at the predicted probability was slightly lower than 0.70 (Figure 4B). Both models 1 and 2 showed good fitting and calibration.
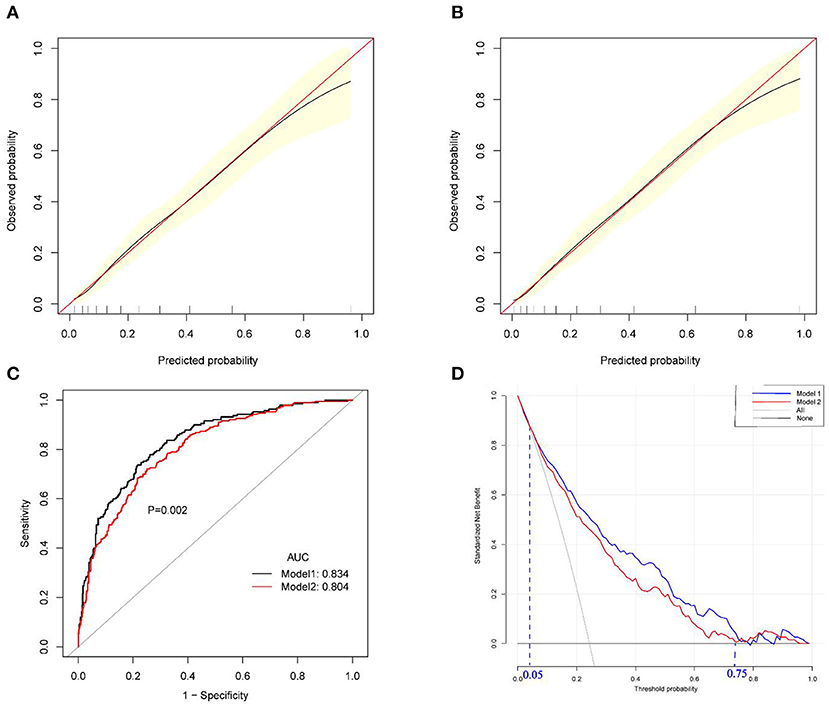
Figure 4. Validation and decision curve analysis of Model 1 and Model 2. (A) Calibration curve of Model 1; (B) calibration curve of Model 2; (C) Receiver operating characteristics curves of Model 1 and Model 2; (D) Decision curve analysis of Model 1 and Model 2. AUC: area under the curve.
Furthermore, Models 1 and 2 were further validated internally using receiver operating characteristic curve. The AUC for Model 1 was 0.834 (95% CI 0.801–0.867), yielding a sensitivity of 73.7% and a specificity of 78.5% at the optimal cutoff value that maximized the Youden's index. At the optimal corresponding cutoff values, Model 2 yielded a sensitivity of 72.1% and a specificity of 74.9%, and the AUC was 0.804 (95% CI: 0.770–0.839). The two nomograms have a good ability to discriminate whether an individual suffers from IGD (Figure 4C), although the discrimination of Model 1 was better than that of Model 2 (P = 0.002).
Clinical Use of Nomograms for IGD Risk Prediction
The decision curve analysis comparing the clinical usefulness of Models 1 and 2 is presented in Figure 4D. The threshold probability for IGD is plotted on the x-axis, and the model's standard net benefit is plotted on the y-axis. The area among the treat all lines, treat none line, and the model curve represent the clinical usefulness of each model. Both models 1 and 2 showed better cost-effectiveness than treating none and treating all. A comparison of the two nomogram models with two extreme schemes (“treat none” and “treat all ”) revealed a net benefit of >0 with a threshold probability of between 5 and 75%. Moreover, in this range, a smaller threshold probability led to a higher net benefit. Only when the threshold probability is <5% is there no difference between using our model and the scheme that considers treating all participants (i.e., regardless of whether they have IGD or not). On the other hand, when the threshold probability is above 75%, there is no difference between using our model and not treating any participant (i.e., no intervention regardless of IGD). Because Model 1 is located at the upper right, its clinical usefulness surpasses that of Model 2.
Discussion
In this study, the proportion of IGD in children and adolescents was 24.3%, which is similar to that reported by Chinese scholar Zheng-Chuan (52). As children and adolescents' IGD has become a shared interest, the assessment of the risk of IGD in this population has acquired clinical significance, which will encourage the early identification of high-risk children and adolescents to carry out targeted prevention or intervention. To the best of our knowledge, no nomogram has been developed to evaluate the risk of IGD. In this study, two models were developed to predict the risk of IGD in children and adolescents, and both were verified internally. Both exhibited good discrimination, calibration, and clinical usefulness.
In the final model, male sex, and experience of video game consumption were the two most important predictors, followed by daily video game time and hyperactivity, and the weight of the remaining predictors was small. For video game consumption experience, a South Korean study reported that video game consumption was a predictor of IGD in adults aged 20–40 years (4). Our result also aligns with several studies of adolescents that suggest that in-game purchases are associated with the development of behavioral addictions, such as IGD (9) and compulsive gambling (53, 54). For example, in a study of Japanese adolescents, Soichiro Ide found that teenagers who bought loot boxes were significantly more likely to have problems with online gaming. Loot boxes, also known as “loot cases” or “loot chests,” are a central feature of many video games. Players purchase loot boxes to obtain valuable in-game items that enhance their gameplay, which in turn may intensify their involvement in video gaming. In addition, this study found that boys have a higher risk of IGD than girls, which is consistent with the results of a longitudinal study in Germany (8). The reason appears to be related to the different ways of using the Internet, as males prefer games, while females prefer social media (55). Therefore, boys are more likely to suffer from IGD, which suggests the need for greater focus on boys to identify the problematic use of video games. Previous studies have indicated that daily video game time plays an important role in predicting the occurrence of IGD (4, 6, 7). A longer daily playtime was found to increase the likelihood for an individual to suffer from IGD, which is in line with clinical findings. This suggests that strict restrictions on children's and adolescents' game time are required to reduce the possibility of IGD and reduce the time spent playing video games in favor of other beneficial activities, such as physical exercise, parent-child interaction, and sleep. Hyperactivity is closely associated with IGDs. A cross-lagged panel design study among German adolescents found that higher levels of hyperactivity could predict the occurrence of IGD 1 year later. Moreover, hyperactivity was found to be an independent risk factor for the occurrence of IGD and a predictor of the persistence of IGD. IGD patients with higher hyperactivity levels are more likely to continue suffering from IGD 2 years later (7). Therefore, from a clinical perspective, the prevention and relief of IGD may require appropriate hyperactivity treatment.
Among other predictors that could be targeted for clinical intervention, the first is the age of exposure to video games. This study suggests that an earlier exposure to video games leads to a greater likelihood of developing IGD. This may be due to the long developmental experience and late maturity of the prefrontal cortex and to the fact that the executive control ability of children and adolescents is still not mature (56). Therefore, early exposure to video games may make it difficult for children to control their gaming behavior. The executive function is not mature until late adolescence (57, 58); therefore, we suggest that parents should control their children's exposure to video games after puberty. Furthermore, impulse control difficulties and limited access to emotion regulation strategies predict IGD. Some studies have shown that people with difficulty in emotion regulation engage in addictive behaviors to escape or regulate negative feelings (59). It is also plausible that if individuals exhibit poor control over impulsive reactions when feeling negative emotions or lack emotion regulation strategies, they might more likely get involved in behaviors that prolong their positive emotional state, such as playing video games. Video games might be a means for them to elevate their mood, which increases the likelihood of indulging in virtual video games. From the perspective of interventions, in addition to providing children and adolescents with more resources and tools to help them better regulate their emotions, parents and teachers also need to pay timely attention and provide appropriate guidance.
Overall, the performances of the two models were found to be similar, but Model 1 was more complex than Model 2. Impulse control difficulties, limited access to emotion regulation strategies, and disinhibition require an evaluation with the corresponding scale and scores for incorporation into the model. This may increase the utility cost of Model 1 and reduce its convenience as a screening tool. Model 2 contained only four predictors. Although the hyperactivity score also needs to be evaluated by the scale, only five items significantly reduce the utility cost. Considering that the two models have a similar overall performance, Model 2 may be more cost effective.
This study had some limitations. First, there is a lack of heterogeneous data to externally validate the models. Further studies should externally validate the models and evaluate their stability (60). Second, the identification of IGD in this study was based on the self-rated IGD Scale, which may be less accurate than face-to-face interviews. If possible, future research can use the results of these interviews as a diagnostic criterion of IGD in a large population. Moreover, subsequent researchers should consider including more game-related variables as predictors, such as game genres (61), and conducting dynamic follow-up observations to further verify and optimize the model's clinical utility.
Conclusion
In summary, the two nomograms developed in this study can be considered economic and effective tools for IGD risk assessment in Chinese children and adolescents. The creation of Nomograms and website calculator can objectively and accurately predict the probability of Internet gaming disorder and can help clinicians to personalize treatment plans. Moreover, from the standpoint of simplification and cost, Model 2 appears to be a better alternative.
Data Availability Statement
The raw data supporting the conclusions of this article will be made available by the authors, without undue reservation.
Ethics Statement
The studies involving human participants were reviewed and approved by the Ethics Committee of Beijing Huilongguan Hospital. Written informed consent to participate in this study was provided by the participants' legal guardian/next of kin.
Author Contributions
JH prepared the first draft of the paper. JW conducted the analysis. WQ designed a mobile phone program for the online survey. HC, JS, MZ, and YZ contributed to the data cleaning and manuscript interpretation. ST supervised this research and revised the manuscript. All authors read and approved the manuscript.
Funding
This work was funded by the Beijing Natural Science Foundation Grant (No. 7202072) and the Beijing Municipal Science & Technology Commission Grant (Z191100006619104).
Conflict of Interest
The authors declare that the research was conducted in the absence of any commercial or financial relationships that could be construed as a potential conflict of interest.
Publisher's Note
All claims expressed in this article are solely those of the authors and do not necessarily represent those of their affiliated organizations, or those of the publisher, the editors and the reviewers. Any product that may be evaluated in this article, or claim that may be made by its manufacturer, is not guaranteed or endorsed by the publisher.
Acknowledgments
We would like to thank Editage (www.editage.cn) for English language editing.
Supplementary Material
The Supplementary Material for this article can be found online at: https://www.frontiersin.org/articles/10.3389/fpsyt.2022.873033/full#supplementary-material
References
1. King DL, Delfabbro PH, Wu A, Doh YY, Kuss DJ, Pallesen S, et al. Treatment of Internet gaming disorder: an international systematic review and CONSORT evaluation. Clin Psychol Rev. (2017) 54:123–33. doi: 10.1016/j.cpr.2017.04.002
2. Mihara S, Higuchi S. Cross-sectional and longitudinal epidemiological studies of Internet gaming disorder: a systematic review of the literature. Psychiatry Clin Neurosci. (2017) 71:425–44. doi: 10.1111/pcn.12532
3. Paulus FW, Ohmann S, von Gontard A, Popow C. Internet gaming disorder in children and adolescents: a systematic review. Dev Med Child Neurol. (2018) 60:645–59. doi: 10.1111/dmcn.13754
4. Rho MJ, Jeong JE, Chun JW, Cho H, Jung DJ, Choi IY, et al. Predictors and patterns of problematic Internet game use using a decision tree model. J Behav Addict. (2016) 5:500–9. doi: 10.1556/2006.5.2016.051
5. Estévez A, Jáuregui P, Sánchez-Marcos I, López-González H, Griffiths MD. Attachment and emotion regulation in substance addictions and behavioral addictions. J Behav Addict. (2017) 6:534–44. doi: 10.1556/2006.6.2017.086
6. Rho MJ, Lee H, Lee TH, Cho H, Jung DJ, Kim DJ, et al. risk factors for internet gaming disorder: psychological factors and internet gaming characteristics. Int J Environ Res Public Health. (2017) 15:40. doi: 10.3390/ijerph15010040
7. Jeong H, Yim HW, Lee SY, Lee HK, Potenza MN, Lee H. Factors associated with severity, incidence or persistence of internet gaming disorder in children and adolescents: a 2-year longitudinal study. Addiction. (2021) 116:1828–38. doi: 10.1111/add.15366
8. Wartberg L, Zieglmeier M, Kammerl R. An empirical exploration of longitudinal predictors for problematic internet use and problematic gaming behavior. Psychol Rep. (2021) 124:543–54. doi: 10.1177/0033294120913488
9. Li W, Mills D, Nower L. The relationship of loot box purchases to problem video gaming and problem gambling. Addict Behav. (2019) 97:27–34. doi: 10.1016/j.addbeh.2019.05.016
10. Ide S, Nakanishi M, Yamasaki S, Ikeda K, Ando S, Hiraiwa-Hasegawa M, et al. Adolescent problem gaming and loot box purchasing in video games: cross-sectional observational study using population-based cohort data. JMIR Serious Games. (2021) 9:e23886. doi: 10.2196/23886
11. Gentile DA, Choo H, Liau A, Sim T, Li D, Fung D, et al. Pathological video game use among youths: a two-year longitudinal study. Pediatrics. (2011) 127:e319–29. doi: 10.1542/peds.2010-1353
12. Siste K, Hanafi E, Sen LT, Wahjoepramono P, Kurniawan A, Yudistiro R. Potential correlates of internet gaming disorder among indonesian medical students: cross-sectional study. J Med Internet Res. (2021) 23:e25468. doi: 10.2196/25468
13. Kun B, Demetrovics Z. Emotional intelligence and addictions: a systematic review. Subst Use Misuse. (2010) 45:1131–60. doi: 10.3109/10826080903567855
14. Ryu H, Lee JY, Choi A, Park S, Kim DJ, Choi JS. The relationship between impulsivity and internet gaming disorder in young adults: mediating effects of interpersonal relationships and depression. Int J Environ Res Public Health. (2018) 15:458. doi: 10.3390/ijerph15030458
15. Kräplin A, Scherbaum S, Kraft EM, Rehbein F, Bühringer G, Goschke T, et al. The role of inhibitory control and decision-making in the course of internet gaming disorder. J Behav Addict. (2020) 9:990–1001. doi: 10.1556/2006.2020.00076
16. Berloffa S, Salvati A, D'Acunto G, Fantozzi P, Inguaggiato E, Lenzi F, et al. Internet gaming disorder in children and adolescents with attention deficit hyperactivity disorder. Children. (2022) 9:428. doi: 10.3390/children9030428
17. Lee J, Bae S, Kim BN, Han DH. Impact of attention-deficit/hyperactivity disorder comorbidity on longitudinal course in internet gaming disorder: a 3-year clinical cohort study. J Child Psychol Psychiatry. (2021) 62:1110–9. doi: 10.1111/jcpp.13380
18. Concerto C, Rodolico A, Avanzato C, Fusar-Poli L, Signorelli MS, Battaglia F, et al. Autistic traits and attention-deficit hyperactivity disorder symptoms predict the severity of internet gaming disorder in an italian adult population. Brain Sci. (2021) 11:774. doi: 10.3390/brainsci11060774
19. Wartberg L, Kriston L, Zieglmeier M, Lincoln T, Kammerl R. A longitudinal study on psychosocial causes and consequences of Internet gaming disorder in adolescence. Psychol Med. (2019) 49:287–94. doi: 10.1017/S003329171800082X
20. Niemz K, Griffiths M, Banyard P. Prevalence of pathological internet use among university students and correlations with self-esteem, the general health questionnaire (GHQ), and disinhibition. Cyberpsychol Behav. (2005) 8:562–70. doi: 10.1089/cpb.2005.8.562
21. Stieger S, Burger C. Implicit and explicit self-esteem in the context of internet addiction. Cyberpsychol Behav Soc Netw. (2010) 13:681–8. doi: 10.1089/cyber.2009.0426
22. Triberti S, Milani L, Villani D, Grumi S, Peracchia S, Curcio G, et al. What matters is when you play: investigating the relationship between online video games addiction and time spent playing over specific day phases. Addict Behav Rep. (2018) 8:185–8. doi: 10.1016/j.abrep.2018.06.003
23. Müller KW, Janikian M, Dreier M, Wölfling K, Beutel ME, Tzavara C, et al. Regular gaming behavior and internet gaming disorder in European adolescents: results from a cross-national representative survey of prevalence, predictors, and psychopathological correlates. Eur Child Adolesc Psychiatry. (2015) 24:565–74. doi: 10.1007/s00787-014-0611-2
24. Wang Y, Liu B, Zhang L, Zhang P. Anxiety, depression, and stress are associated with internet gaming disorder during COVID-19: fear of missing out as a mediator. Front Psychiatry. (2022) 13:827519. doi: 10.3389/fpsyt.2022.827519
25. Wartberg L, Kriston L, Kramer M, Schwedler A, Lincoln TM, Kammerl R. Internet gaming disorder in early adolescence: associations with parental and adolescent mental health. Eur Psychiatry. (2017) 43:14–8. doi: 10.1016/j.eurpsy.2016.12.013
26. Lin S, Yu C, Chen J, Sheng J, Hu Y, Zhong L. The association between parental psychological control, deviant peer affiliation, and internet gaming disorder among chinese adolescents: a two-year longitudinal study. Int J Environ Res Public Health. (2020) 17:8197. doi: 10.3390/ijerph17218197
27. Zhang D, Wang S. The impact of peer attachment on left-behind children's pathological internet use: a moderated mediating effect model. Int J Environ Res Public Health. (2021) 18:9775. doi: 10.3390/ijerph18189775
28. Teng Z, Griffiths MD, Nie Q, Xiang G, Guo C. Parent-adolescent attachment and peer attachment associated with internet gaming disorder: a longitudinal study of first-year undergraduate students. J Behav Addict. (2020) 9:116–28. doi: 10.1556/2006.2020.00011
29. Schneider LA, King DL, Delfabbro PH. Family factors in adolescent problematic Internet gaming: a systematic review. J Behav Addict. (2017) 6:321–33. doi: 10.1556/2006.6.2017.035
30. Bonnaire C, Phan O. Relationships between parental attitudes, family functioning and Internet gaming disorder in adolescents attending school. Psychiatry Res. (2017) 255:104–10. doi: 10.1016/j.psychres.2017.05.030
31. Yu Y, Mo P, Zhang J, Li J, Lau J. Why is Internet gaming disorder more prevalent among Chinese male than female adolescents? the role of cognitive mediators. Addict Behav. (2021) 112:106637. doi: 10.1016/j.addbeh.2020.106637
32. Kiraly O, Griffiths MD, Urban R, Farkas J, Koekoenyei G, Elekes Z, et al. Problematic internet use and problematic online gaming are not the same: findings from a large nationally representative adolescent sample. Cyberpsychol Behav Soc Netw. (2014) 17:749–54. doi: 10.1089/cyber.2014.0475
33. Marraudino M, Bonaldo B, Vitiello B, Bergui GC, Panzica G. Sexual differences in internet gaming disorder (IGD): from psychological features to neuroanatomical networks. J Clin Med. (2022) 11:1018. doi: 10.3390/jcm11041018
34. Pontes HM, Griffiths MD. Measuring DSM-5 internet gaming disorder: development and validation of a short psychometric scale. Comput Human Behav. (2015) 45:137–43. doi: 10.1016/j.chb.2014.12.006
35. Hong Z, Xing J. Validity and reliability of two Chinese versions of Internet addiction subtypes scales in primary and middle school students. Chin Ment Health J. (2016) 30:936–8. doi: 10.3969/j.issn.1000-6729.2016.12.011
36. Monacis L, Palo V, Griffiths MD, Sinatra M. Validation of the internet gaming disorder scale - short-form (IGDS9-SF) in an Italian-speaking sample. J Behav Addict. (2016) 5:683–90. doi: 10.1556/2006.5.2016.083
37. Bourdon KH, Goodman R, Rae DS, Simpson G, Koretz DS. The strengths and difficulties questionnaire: US normative data and psychometric properties. J Am Acad Child Adolesc Psychiatry. (2005) 44:557–64. doi: 10.1097/01.chi.0000159157.57075.c8
38. Kou JH, Du YS, Xia LM. Formulation of children strengths and difficulties questionnaire (the edition for students) for Shanghai norm. China J Health Psychol. (2007) 15:3–5.
39. Rosenberg M. Society and the adolescent self-image. Social Forces. (1965) 3:305–7. doi: 10.1515/9781400876136
40. Ji YF, Yu X. The self-esteem scale. In: Wang XD, Wang XL, Ma Hong, editor. Rating Scales For Mental Health. Beijing: Chinese Mental Health Journal Supplements. (1999). p. 319–20.
41. Yang Y, Wang D. Retest of the bidimensional model of Rosenberg self-esteem scale. Chinese Ment Health J. (2007) 21:603–9.
42. Gratz KL, Roemer L. Multidimensional assessment of emotion regulation and dysregulation: development, factor structure, and initial validation of the difficulties in emotion regulation scale. J Psychopathol Behav Assess. (2004) 26:41–54. doi: 10.1023/B:JOBA.0000007455.08539.94
43. Wang L, Liu H, Du W, Li Z. Test of difficulties in emotion regulation scale in chinese people. China J Health Psychol. (2007) 16:846–8.
44. Ling D, Nan Z, Ding X, Psychology DO University NN. Reliability and validity of difficulties in emotion regulation normal scale in Chinese adolescent. China J Health Psychol. (2014).
45. Hoyle RH, Stephenson MT, Palmgreen P, Lorch EP, Donohew RL. Reliability and validity of a brief measure of sensation seeking. Pers Individ Dif . (2002) 32:401–14. doi: 10.1016/S0191-8869(01)00032-0
46. Chen X, Li F, Nydegger L, Gong J, Ren Y, Dinaj-Koci V, et al. Brief sensation seeking scale for Chinese - cultural adaptation and psychometric assessment. Pers Individ Dif. (2013) 54:604–9. doi: 10.1016/j.paid.2012.11.007
47. Moos RH, Moos BS. Family Environment Scale Manual 2nd ed. Palo Alto, CA: Consulting Psychologists Press (1986).
48. Fei LP, Shen QJ, Zheng YP, Zhao JP, Jiang SA, Wang LW. Preliminary evaluation of Chinese version of FACES II and FES: comparison of normal families and families of schizophrenic patients. Chinese Ment Health J. (1991) 5:198–202.
49. Tao JH, Jin FX, Zhang MR, Cheng ZH. Reliability and validity of FES-CV in the adolescent population. Chinese J Clin Psychol. (2015) 23:1024–7. doi: 10.16128/j.cnki.1005-3611.2015.06.015
50. Moons K, Wolff RF, Riley RD, Whiting PF, Westwood M, Collins GS, et al. PROBAST: a tool to assess risk of bias and applicability of prediction model studies: explanation and elaboration. Ann Intern Med. (2019) 170:W1–33. doi: 10.7326/M18-1377
51. Vickers AJ, Cronin AM, Elkin EB, Gonen M. Extensions to decision curve analysis, a novel method for evaluating diagnostic tests, prediction models and molecular markers. BMC Med Inform Decis Mak. (2008) 8:53. doi: 10.1186/1472-6947-8-53
52. Zheng-Chuan XU. An empirical study on the impact of motivation and prevention factors on online game addiction. J Fudan Univ. (2009) 48:308–14.
53. Rockloff M, Russell A, Greer N, Lole L, Hing N, Browne M. Young people who purchase loot boxes are more likely to have gambling problems: an online survey of adolescents and young adults living in NSW Australia. J Behav Addict. (2021) 10:35–41. doi: 10.1556/2006.2021.00007
54. Kristiansen S, Severin MC. Loot box engagement and problem gambling among adolescent gamers: findings from a national survey. Addict Behav. (2020) 103:106254. doi: 10.1016/j.addbeh.2019.106254
55. Durkee T, Kaess M, Carli V, Parzer P, Wasserman C, Floderus B, et al. Prevalence of pathological internet use among adolescents in Europe: demographic and social factors. Addiction. (2012) 107:2210–22. doi: 10.1111/j.1360-0443.2012.03946.x
56. Gazzaniga MS, Ivry RB, Mangun GR. Cognitive control. In: Gazzaniga MS, Ivry RB, Mangun GR, editors. Cognitive Neuroscience: the Biology of the Mind. Fifth edition. New York, NY: W.W. Norton & Company (2019). p. 517.
57. Diamond A. Executive functions. Annu Rev Psychol. (2013) 64:135–68. doi: 10.1146/annurev-psych-113011-143750
58. Prencipe A, Kesek A, Cohen J, Lamm C, Lewis MD, Zelazo PD. Development of hot and cool executive function during the transition to adolescence. J Exp Child Psychol. (2011) 108:621–37. doi: 10.1016/j.jecp.2010.09.008
59. Aldao A, Nolen-Hoeksema S, Schweizer S. Emotion-regulation strategies across psychopathology: a meta-analytic review. Clin Psychol Rev. (2010) 30:217–37. doi: 10.1016/j.cpr.2009.11.004
60. Collins GS, Reitsma JB, Altman DG, Moons KG. Transparent Reporting of a multivariable prediction model for Individual Prognosis Or Diagnosis (TRIPOD): the TRIPOD Statement. Br J Surg. (2015) 102:148–58. doi: 10.1002/bjs.9736
Keywords: Internet gaming disorder, children, prediction model, adolescents, nomogram
Citation: Hong J, Wang J, Qu W, Chen H, Song J, Zhang M, Zhao Y and Tan S (2022) Development and Internal Validation of a Model for Predicting Internet Gaming Disorder Risk in Adolescents and Children. Front. Psychiatry 13:873033. doi: 10.3389/fpsyt.2022.873033
Received: 10 February 2022; Accepted: 16 May 2022;
Published: 09 June 2022.
Edited by:
Zhiri Tang, City University of Hong Kong, Hong Kong SAR, ChinaReviewed by:
Maria Pietronilla Penna, Università di Cagliari, ItalyLan Xiao Cao, Shenzhen Children's Hospital, China
Wenle Li, Xian Yang Central Hospital, China
Copyright © 2022 Hong, Wang, Qu, Chen, Song, Zhang, Zhao and Tan. This is an open-access article distributed under the terms of the Creative Commons Attribution License (CC BY). The use, distribution or reproduction in other forums is permitted, provided the original author(s) and the copyright owner(s) are credited and that the original publication in this journal is cited, in accordance with accepted academic practice. No use, distribution or reproduction is permitted which does not comply with these terms.
*Correspondence: Shuping Tan, shupingtan@126.com