- 1James Watt School of Engineering, University of Glasgow, Glasgow, United Kingdom
- 2Transport for London (TfL), London, United Kingdom
- 3Electrical Engineering, University of Engineering and Technology, Lahore, Pakistan
- 4Electrical and Computer Engineering, Ajman University, Ajman, United Arab Emirates
- 5Artificial Intelligence Research Center (AIRC), Ajman University, Ajman, United Arab Emirates
The integration of Private Mobile Networks (PMN) with edge intelligence is expected to play an instrumental role in realizing the next generation of industry applications. This combination collectively termed Intelligent Private Networks (IPN) deployed within the scope of specific industries such as transport systems can unlock several use cases and critical applications that in turn can address rising business demands. This article presents a conceptual IPN that hosts intelligence at the network edge employing emerging technologies that satisfy a number of Next-Generation Railway System (NGRS) applications. NGRS use cases along with their applications and respective beyond 5G (B5G) enabling technologies have been discussed along with possible future research and development directions that will allow these promising technologies to be used and implemented widely.
1 Introduction
Connectivity is now found in almost all facets of modern lives. What started with the simple provision of connecting humans paved the way for connected processes and developments that changed the outlook of what now is a global economy. Over the past two decades, the number of connected devices and subsequent processes have risen immensely. The advantages this connectivity brings into our lives are not only limited to convenience and globalization but also provide the basis of use cases and applications that substantially contribute toward the socioeconomic welfare of our society.
Due to the rise in capacity, security, and critical communication needs Asad et al. (2019), major industries are now seeking to establish their own Private Mobile Networks (PMN). These PMN are private wide-area multiple access wireless networks that are highly scalable, provide an adequate range, are and consistent Quality of Service (QoS) in comparison to license-exempt solutions such as WiFi. The privatization of mobile networks was made possible with the advent of Long-Term Evolution (LTE) due to its simplicity and an all-IP nature. Such networks consist of an Evolved Packet Core (EPC) usually integrated with IP Multimedia System (IPMS) for handling voice calls and a typical Radio Access Network (RAN) (Katsalis et al., 2017). Another key enabler for PMN is the virtualization of computing and network resources where an entire core network and parts of access network can be hosted in the cloud while the cognitive radio at the edge of the network can make efficient use of unused spectrum to provide seamless end-to-end connectivity. Improvements in the LTE technologies in terms of added intelligence and improved capacity, both in core and access networks, have paved the way for 5G mobile networks (Zhang, 2020). We perceive that these improvements along with application-centric intelligence can further shape PMN for individual industries. Current railway systems are facing many challenges due to the deficiency of advanced 5G functions and capabilities to support various use cases, services, and scenarios (Dat et al., 2016). The 5G networking model use cases that support railway systems architecture are amazing speed; great service in a crowd; ubiquitous device communications; super real-time and reliable connections; and the best user experience. All of these use cases are challenges to railway networks which in turn become a necessity due to high rise data rates and capacity needs.
In this article, we present IPN for the Next-Generation Railway System (NGRS) and outline enabling technologies and frameworks that will be the key enablers in the scope of intelligent mobility. This article is structured in such a way that we start by outlining edge intelligence in the context of mobile networks in Section 2 and then a discussion on key challenges in NGRS is included in Section 3. Section 4 then includes mobile edge technologies and use cases that can potentially address the challenges outlined in Section 3. Section 5 presents the IPN architecture used to integrate edge intelligence and PMN in the context of addressing NGRS challenges via 5G or B5G technologies. Finally, Section 6 presents the conclusion and some future research directions.
2 Edge Intelligence in Mobile Networks
The exponential increase in computing resources and substantial improvements in Artificial Intelligence (AI) algorithms over the past decade has paved the way for intelligence to be integrated into the day-to-day operations of mobile networks. Several studies, Niyato (2020), Li et al. (2017), Zhang (2020), Zoha et al. (2020), and Hussain et al. (2020), have presented the use of AI-based frameworks to enable the imminent and future demands of ever-expanding mobile networks. For instance, in Niyato (2020), artificial intelligence- (AI-) based applications and services and their challenges in a comprehensive overview have been provided. It also highlights AI services and their mechanism as to how they are being applied to the network edge near the data sources and demonstrates how AI and edge computing can be mutually beneficial. To do so, it introduces and discusses edge intelligence and intelligent edge and their implementation methods and enabling technologies, namely, AI training and inference in the customized edge-computing framework. In Li et al. (2017), the standardization of 5G cellular networks is being expedited to provide insight into the candidate techniques as a whole and examine the design philosophy behind them. It highlights one of the most fundamental features among the revolutionary techniques in the 5G era; i.e., there emerges initial intelligence in nearly every important aspect of cellular networks, including radio resource management, mobility management, and service provisioning management. In Zhang (2020), several Internet-of-Things (IoT) and 5G sensor networks in railway communication have been exploited along with the previous industry challenges and their forthcoming resolutions. In Zoha et al. (2020), a summary of self-healing block in self-organizing 5G networks which presents a framework for autonomous outage detection and coverage optimization in an LTE has been presented. Hussain et al. (2020) presented a split in the 5G network into several 100-cell regions, each monitored by an edge server, and proposed a framework that preprocesses raw call detail records having user activities to create an image-like volume, fed to a machine learning (ML) model. Yang et al. (2019) presented the application of tactile Internet in healthcare by considering the diabetic ketoacidosis (DKA) as a 5G use case to monitor life-threatening complications of diabetes mellitus by using the C-Band sensing technique. Reducing the computational distribution and load in a dense deployment will require edge intelligence. With edge intelligence and dense deployment of base stations, the applications will be numerous. To explain the intelligence presented in future IPN for NGRS, we classify these operations into user-centric, network-centric, and application-centric edge intelligence. Although these will operate hand in hand in utter harmony, it is important to understand the perspective of intelligence from these different angles as shown in Figure 1.
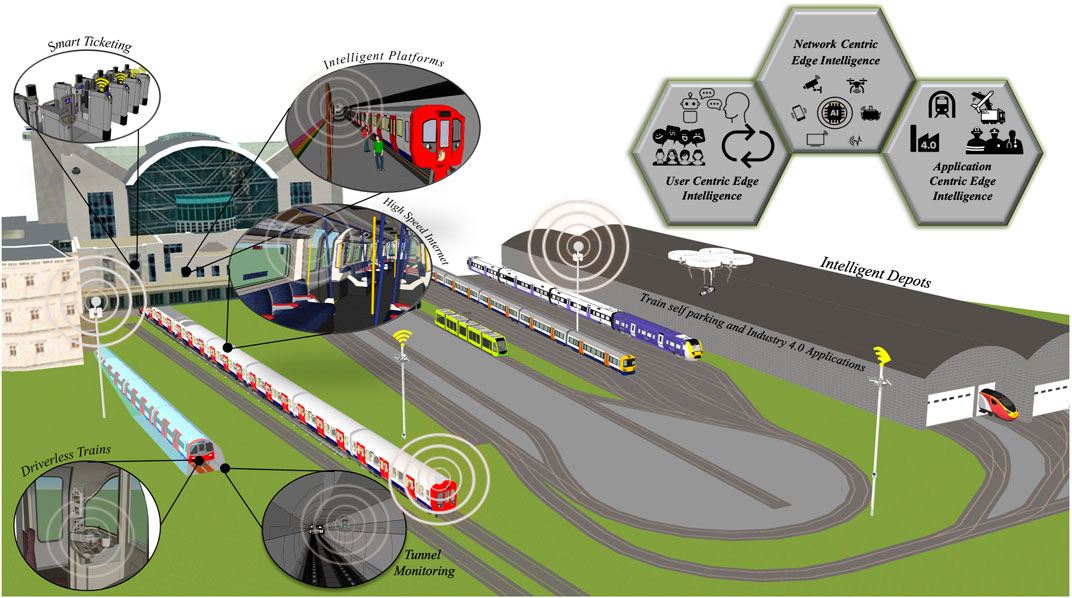
FIGURE 1. Intelligent Private Network (IPN) with user, network, and application-centric edge intelligence for Next-Generation Railway Systems (NGRSs).
2.1 User-Centric Edge Intelligence
The QoS of any network is a key metric in evaluating its performance as it correlates to the users’ level of satisfaction. In a similar pursuit, added intelligence in the network make them more user-centric by including users’ experience in the feedback loop. Beyond 5G (B5G) networks will be able to assess the users’ demands by running various distributed federated learning algorithms at the edge of the network. With the inference capability derived from federated learning in hand, the edge nodes performing user sentiment analysis can subsequently inform the network of required changes (Wang et al., 2019).
2.2 Network-Centric Edge Intelligence
The efficient utilization of network resources can make the network more sustainable in terms of lower Operational and Capital Expenditure (OPEX/CAPEX). With that in mind, self-organizing and self-optimizing algorithms deployed at the network edge can enable timely inference and quick decisions for effective radio resource management, user mobility management, network orchestration, and service provisioning.
2.3 Application-Centric Edge Intelligence
In B5G networks, several applications are expected to be hosted at the edge of the network. This will require virtualization of application containers and related computational resources at the base station. Since applications have varying demands, the application intelligence employed along with the deployment strategy will vary from case to case; for example, AI algorithms used in train automation will have a different deployment strategy compared to AI campaigns that elevate passenger travel experience.
3 Anticipated Challenges in NGRS
The advancement in wireless networks along with the technical developments in the transport sector has acquainted the industry with an entirely new approach to public safety, travel efficiency, and information dissemination. Intelligent Transport Systems (ITS) refer to a new set of information and communication technologies that allow transport entities to exchange data with each other or the infrastructure to support several transport-related applications. However, predominantly, the focus within ITS has been toward road users; hence in this article, we move that focus to intelligent railway systems. In the context of IPN, we discuss the key challenges and their use cases enabled by edge intelligence in railway transport networks.
Currently, the underground transportation system is facing key challenges with the passengers’ movement information and pathways, bidirectional communication between passenger flows on transit and information centers, and collection and processing of “big data” coming from a range of Internet-of-Things (IoT) devices. These challenges are mainly due to the maintenance, reliability, connectivity, and logistics issues while innovation management, cost management, deployment of IoT devices, durability, and sustainability of these devices also pose concerns. The methodologies prevailing in ITS where railway networks are concerned have not attained the level of research that would address all the outstanding issues. This lack of literature motivates this article where we have gathered evidence to identify the challenges and opportunities faced within the current railway transport networks. This will help us understand how the edge intelligence in B5G private networks holds significant importance while prioritizing relevant solutions.
3.1 Safety and Security
Public panic situations in the current underground train network can become severely adverse if prompt monitoring and response are not provided. Safety and security measures are highly essential for diffusing such situations in the interest of public safety. There are also concerns about crime and disorder that act as a barrier to passengers’ travel. An evolving focus on such issues is critical to reduce crime and improve passengers’ confidence. Public safety applications utilizing intelligent solutions at the network edge can help in the predicament of daily travel against the threat of terrorism, financial constraints, and monitoring passenger commutes.
3.2 Traffic and Crowd Management
Congestion due to traffic rise causes impatience, anger, and frustration within passengers which in turn influence their travel behavior causing the journeys to become slow, unpredictable, and stagnant (Asad et al., 2019). For transport businesses, any form of disruption or congestion in their services directly or indirectly affects their CAPEX and OPEX while causing harm not only to the business operations but also to the reputation of the company. If these travel delay issues are not addressed, on average, a passenger can waste up to two and a half days per year waiting for a congested train. Identification of passenger movement in underground train networks is also a persisting challenge that contributes to high energy consumption and subsequent failure of effective optimization measures for mobile networks (Asad et al., 2020a; Asad et al., 2020b). AI can fuel numerous traffic management applications residing at the edge of the PMN while keeping a closer look at the traffic patterns and inferring decisions in smarter ways.
3.3 Proactive Operations
Common transport systems are designed based on the principle of “reaction for any action.” While this has proven to work in many cases, with the current increase in quick response requirements, other modes of reactive operations are now being sought. With the reactive approach, actions operate after an event has occurred. For time-critical applications, this results in a waste of resources and user QoS. Therefore, there is a need to address these issues with proactiveness that can administer the dynamics of the transport specifically in an underground train network where the conditions are rapidly changing. This proactive nature can be achieved by having intelligence deployed at the edge of the network where the application can predict the future network state well in advance.
3.4 Latency
Real-time mission-critical applications and quick emergency response operations require the satisfaction of specific latency criteria without considering existing operational technology for train networks. However, the current wideband radio spectrum shared among various services pose challenges and obstacles in effectively serving the critical transport entity operations. Similarly, anomalies from existing passenger traffic flows are difficult to predict for such a demand critical consortium. For instance, if a criminal has to be put into custody by chasing them within a massive traffic flow, the real-time crowd information can be highly vital in providing the emergency services (ES) a safe and less disrupting passage to the criminal.
3.5 Planning and Costing
Existing technologies within underground train networks are extremely beneficial; however, they bring in deployment and sustainability-related costs. Several strategies and techniques have been developed and deployed for the planning and costing of densely populated networks and energy-efficient systems, but they fail to address many challenges. Planning regulations are required to be in compliance with the active development procedures and regulations. We believe that network intelligence at the edge can provide a scalable and cost-effective solution in the shape of a real-time informed decision-making framework suitable for planning and costing related activities of underground train networks.
3.6 Resilience
The impact of weather on the transport network is another main challenge that requires daily/hourly weather forecasting and understanding of the anticipated impact of change in the climate. The transport system must be resilient to extreme weather conditions and must have the capability of self-healing against climate adversities. Disruption to the trains and overall transport network could be caused by either rainfall, heatwaves, high winds, or snow. With a distributed private network employing intelligence at many facets, it can provide useful information to the control center in identifying weather patterns with future predictions allowing informed decision-making.
4 Addressing NGRS Challenges With IPN Edge Intelligence
In the following subsections, we first discuss the use cases of NGRS, and then we describe IPN technologies that we believe will play an instrumental role in the realization of NGRS.
4.1 Use Cases and Applications
Evolution toward B5G networks for transport systems aims to address challenges in essentially all businesses that come under the umbrella of ITS. Edge intelligence is expected to remodel the existing trends in terrestrial networks to accommodate the mobile nature of sensor networks, train signaling, security, and overall logistics within underground train networks. Although there are numerous use cases, we enlist a few that we believe will address the current challenges around underground railway environments.
4.1.1 Intelligent Train-to-Train (IT2T) Communications
The wireless industry is developing processes and methods that will achieve sustainable solutions to support IT2T Communications. Most of the approaches and state-of-the-art works regarding direct communications fall short in determining the low-level requirements, use cases, and their applications to the best of our knowledge. IT2T communications will be able to offer low-latency intelligent train networks, autonomous trains, fast adaptive management of congestion control, onboard Internet and infotainment services, broadcasting of real-time fault incidents, real-time fault detection and recovery, and teleoperated trains ensuring fast and safe control against hazards. Some of these use cases are now possible by utilizing small-sized antenna arrays within underground trains and tunnels operating in the sub-6 GHz and/or mmWave band. The vulnerability due to shadowing and poor isotropic propagation loss in the underground environment would need to be controlled and managed effectively within these use cases (Ford et al., 2017).
4.1.2 Contact-Tracing System
The novel Coronavirus 2019 (COVID-19) proved its viral infectious nature and consequently forced the entire world into a lockdown. For transport operators, such an outbreak placed an enormous challenge in continuing their services in a safe manner (Asad et al., 2020c). Train passengers are liable to face viral health issues while traveling in congested trains where all the travelers are in proximity to one another without social distancing measures. Contact-tracing and passenger distancing will be highly necessary and will act as one of the crucial types of mediation to avoid further spread. In such circumstances, an intelligent Contact-Tracing technique potentially hosted at the edge of the network integrated within the transport infrastructure will become an essential need for transport operators.
4.1.3 Intelligent Drones System
Encouraged by the promising 5G capabilities, we envisage surveying, information capturing, and live surveillance streaming through Intelligent Drones System (IDS). The functional application of the IDS will be a key enabler to address overcrowding situations in places such as train terminals. The other two functions for IDS can be the provisioning of communication services through network surveillance using low bandwidth control signals and/or streaming live camera surveillance using high bandwidth data signals. The operation of IDS is expected to be a mixture of multiple technologies to achieve highly versatile services. Drones within IDS will be able to communicate with the IPN utilizing their respective network slice in order to collate multiple communication services into one back-end cloud network (Solomitckii et al., 2018). This is achieved by integrating a Centralized Intelligent Multiplexer (CIM) at the edge of the network that receives multiple signals from different drones and combines them to establish the accurate content (multipath propagation) for data analytics (Solomitckii et al., 2018; Paulo Klaine, 2018).
4.1.4 Transport Hub Crowd Management
Predicting and managing major transport hubs’ capacity in both businesses as usual (BAU) and exceptional circumstances, e.g., rush hour, travel disruption, and planned events by using Machine Learning (ML), is one of the challenging aspects for Transport Hub Crowd Management (THCM). Inspired by fixed monitoring systems, THCM could employ fixed sensors (Asad et al., 2020a; Ansari et al., 2018) or 5G mobile networks (Li et al., 2017) to cope with the limitations it currently faces. This is stressed out by the exponential spread of uncertainties enabled by a high passenger density and frequent footprint in transport hubs. Sensors in the hubs would use a triangulation method to detect the location of passengers that would be echoed from their 5G handsets, called echolocation. Digital tools such as Real-Time Location Systems (RTLS) or Intelligent Positioning Systems (IPS) hosted at the network edge will be important for precise echolocation gathering of real-time crowd information.
4.1.5 Disaster Scenario Response
Edge intelligence within B5G networks will introduce an assortment of services that will operate on high throughput and low latency to address critical events that require a timely response. In the same way, the underground train network is a highly deserving environment to adopt such technology into portable devices leveraging the benefits of Massive Machine-Type Communications (mMTC) (Zhang, 2020). New portable lightweight devices equipped with intelligent computing applications and storage enabled via the IPN cloud would be able to provide robust remote connectivity from the location of the fault within underground ecology to where the experts are. In this case, a central control center with highly skilled workers would be available to support multiple remote operations simultaneously without physically going to the fault location. In other words, within the NGRS, the staff will be empowered to complete tasks by remotely connecting with skilled specialists while supporting a “paper-free” maintenance log, moving to fully digital infrastructure for secure instructions. The use of Intelligent Extended Reality (IXR) will be able to enhance a mixture of Augmented Reality (AR), Virtual Reality (VR), and Tactile Services (TS) for enhanced remote operations.
4.1.6 Intelligent Monitoring Services
The rapid growth of connected wireless devices drives the purpose of the Internet of Things (IoT) through mMTC within 5G and beyond networks. IoT within the underground environment requires the capability of interconnecting diverse services via the wireless network utilizing narrow bandwidth and consuming low power while providing maximum penetration in the train tunnels. In a complex underground ecology, the number of applications for such a use case suited to Narrow Band IoT (NB-IoT) (Li et al., 2017) is, but not limited to, underground tunnels gas and water metering, pressurized fuel detection, smoke alarms detection, fire alarms monitoring, and event detectors. AI within IPN is a key enabler for many isolated and distributed devices to function under one centralized cloud. Additionally, with such an IPN framework, broadcasting to and from all the connected devices at one time is also an essential component. For the implementation of broadcasting, it would require Intelligent B5G-Xcast (IBX) by leveraging the findings of point to point (PTP), point to multipoint (PTM), and 5G-Xcast project (Banchs et al., 2019). The use of laser-based IoT devices to monitor and subsequently recommend safe distances from the toxic gases found in underground confined areas also has utmost importance for safety purposes.
4.1.7 Intelligent Wireless Audio
Today, emergency services in the underground environment use wireless audio links through existing technologies that offer highly specialized RF communication solutions. Due to the scarce spectrum and shared resources, in-use emergency audio devices are facing challenges within the dynamic underground environment. Besides this fact, the multioperational emergency services are demanding an improvement in the quality (increment in quantity) of their radio spectrum in order to provide effective safety to their staff and passengers against antisocial behaviors. An innovative edge intelligence agent employed within the IPN can address such needs with the use of ultrareliable low-latency communications (URLLC). Integration of URLLC in IWA can be seen in current automation industries (Pilz et al., 2018) where there are high demands on communication for automation-related use cases but it has scarcely been used as a use case in the application of the transport industry specifically underground train networks where high reliability and low-latency services would benefit the IWA infrastructure.
4.1.8 Emergency Services
One of the great achievements the Third-Generation Partnership Project (3GPP) has obtained is standardizing the Mission-Critical Push-To-Talk (MCPTT) (Sanchoyerto et al., 2019) service as a use case. This ES use case can be used to act against anything abnormal such as fare evasion, suicide attempts, and incidents. MCPTT empowered with Enhanced Mobile Broadband (eMBB) can be an essential focus in ITS when considering the underground train environment. This will require an application that employs high bandwidth internet access, video streaming, and virtual reality with latency-sensitive devices. At the same time, utilizing network and radio slicing techniques, several high bandwidth sessions for separate emergency departments such as ambulance, firefighters, first-aid staff, mission-critical fault detection teams, or other paramedic services can be established within the IPN.
4.1.9 Intelligent Depots 4.0 (ID 4.0)
AI-based industrial automation powered by IPN is making a tremendous revolution toward B5G technologies. In the field of supply chain, this transposes toward the edge intelligence of logistics 4.0 that advances beyond process automation by using intelligent collaboration and interconnection between connected systems providing transformative applications (Wang et al., 2017). ID in the train network is an important use case that will push communications to another level where train depots will use automated coordination between objects and autonomous self-optimizing logistics. Furthermore, train parking, monitoring trains movement by Train Access Controller (TAC) through Radio-frequency identification (RFID) sensors, identification of electrified and nonelectrified tracks by using AR devices, self-automated track components between the running rails, use of Conversational AI (CAI) between moving trains and TAC when accessing live tracks, intelligence in infringing over the platform edge, automation in stopping a train in an emergency through the use of specialized low-latency devices, and real-time visibility of occupied and unoccupied train depot tracks are the hallmarks of Industry 4.0 that will feed into the ID 4.0.
4.2 IPN Technologies for NGRS
ITS-focused AI is a key enabler that can offer a solution to the complex train network in the form of an IPN in proximity to an operational environment. The demand for ubiquity, privacy, low latency, low-cost computing, and resource management requires an integration of heterogeneous systems and edge compatible applications into one smart network, presented as an IPN. With such a use case, isolated and disparate edge services will provide sufficient security by having multiple Virtual Private Networks (VPN) to safeguard against malicious attacks. The motivation of IPN comes from a combination of private mobile networks and capabilities of B5G networks in lieu of ITS and more specifically future railway systems which are summarized in Table 1 along with their applications, enabling technologies, and challenges. The idea of running several applications within a single network environment consolidates with IPN’s scalability, security, flexibility, and coverage expansion. This will evolve the existing private mobile networks toward smart transport digital facilities with the use of Machine Learning (ML) (Asad et al., 2020a; Zhang, 2020; Klaine et al., 2017; Liu et al., 2018; Ren et al., 2020; Haider et al., 2019; Fioranelli et al., 2019), AI, and cognitive analytics that is fully primed for existence at the edge establishing ground toward B5G networks. IPN main features are as follows:
• Scalability: massive connectivity and capacity in a cost-effective manner for business expansion and growth.
• Security: block unauthorized access, maintain end-to-end data confidentiality, and provide encryption with a complete on-demand solution.
• Flexibility: adaptive tethering and real-time adjustment with the environmental processes.
• Reliability: stable continuous connectivity and safe service continuity for business-critical operations.
• Evolution to B5G: future proof intelligence to support existing deployed solutions with investment opportunities to support additional use cases with stricter requirements.
Following are the components that we envisage will enable the IPN framework.
4.2.1 Artificially Intelligent Anyhaul
Due to the overwhelming data growth experienced by mobile network operators, they are compelled to further densify, centralize, and/or cloudify their networks. This has led to the provisioning of centralized streams and edge intelligent IoT/M2M devices used in the NGRS which are the key drivers to provide quick and robust responses. Through the use of AIA, transport network would be cost-effectively driven by reaching edge intelligent devices in no time from the centralized core1. Centralized IPN would only be consulted for control signaling when required, leaving edge devices to perform faster responses on their own in their locally present intelligent compute storage. With this approach, the IPN architecture presented would be exploited for delivering high capacity, ultralow latency, and highly accurate synchronization distribution needed to boost the overall performance of IPN while taking advantage of virtualized edge intelligent resources (Hilt, 2019).
4.2.2 New Radio
The 3GPP standard for the 5G radio access technologies (RAT) known as 5G New Radio (NR) supports a substantial expansion unlike traditional technologies with two new ranges of frequency bands, FR1 and FR2 (Pilz et al., 2018). The first range includes sub-6 GHz in addition to bandwidth offerings from 410 to 7,125 MHz. The latter one introduces frequency bands from 24.25 to 52.6 GHz with offerings of even higher bandwidth than the first ranges. An important aim in the development of the 5G NR within NGRS has been to ensure dynamic bandwidth communications depending on the requirement. In the presented IPN, NR is introduced at the interface of trains communication to the centralized smart architecture ensuring a high degree of forward compatibility in the radio interface design. In the context of NGRS, either IT2T communication or Train Signaling radio interface allows for substantial future evolution in terms of introducing new technology and enabling new services. For IT2T communication or Train Signaling radio interface, NR would support a massive number (e.g., ≥64) of steerable antenna elements for both transmission and reception. At higher frequency bands, the large number of antenna elements are primarily used for beamforming to achieve coverage, while at lower frequency bands they enable full-dimensional multiple-input multiple-output (MIMO) and interference avoidance by spatial filtering (Parkvall et al., 2017).
4.2.3 Mission-Critical Push to Talk
MCPTT originates from old-fashioned Push-to-talk Over Cellular (POC) services that integrate with mobile phone networks with a world of opportunities. The service supports pairs and group users for communication in the specific time slotting procedure. Each user will be able to converse both with audio (using low bandwidth) and with video (using higher bandwidth). MCPTT system allows participants to request a token to speak. A priority mechanism to arbitrate the participation of the users is provided in the communication of critical services (Sanchoyerto et al., 2019). Traditionally, the choice of which user requests to depend on their token time frames and numbers when using the service simultaneously. IPN presents intelligent MCPTT on the edge devices where simultaneous users on their critical missions would be addressed without delays. IPN-MCPTT connectivity works on a principle of separated Control and Data Architecture (CDSA) where mission-critical intelligence is handled on the edge without consulting IPN in every communication leaving IPN to monitor activities that each MCPTT device performs.
4.2.4 Intelligent CCTVs
Intelligent CCTVs integrated with edge intelligence will guard assets and processes in real-time by employing AI and ML algorithms for quick detection (Akbar and Azhar, 2018). The high-quality video stream from the CCTV multiplexed with edge intelligence will even allow the prediction of hazardous or emergency situations. The system can also grant access, monitor, and log people’s entry/exit into a building allowing a much-centralized control of railway systems. ICCTVs hold the local cache to record real-time events on the edge without the need of IPN core which makes the system intelligent, locally administrative, and less overhead intensive with only required frames per second to be sent to the centralized IPN in every recording.
4.2.5 Unmanned Aerial Vehicle
UAVs, more commonly known as drones, have a controller, usually ground-based with a strong communication link between the two. UAVs flight have various degrees of autonomy operation (Solomitckii et al., 2018; Sambo et al., 2019) either under remote control by a human operator or autonomously by onboard computers. Drones can extend network coverage and capacity to reach remote or highly crowded areas. Either individually or as a swarm controlled by IDS, they can also function as robotic equipment that offers detection, traction, interference mitigation, and/or optimization of systems avoiding costly human interventions. UAVs can be utilized to extend network coverage in highly crowded areas such as train terminus. In addition, UAVs can also be utilized in emergency coverage in situations of local infrastructure malfunction or disaster. Intelligent UAVs have local automation to take important decisions at the edge with control functions to be monitored from IPN. This network-centric intelligence provides a bridge that integrates UAVs into the IPN infrastructure as access points.
4.2.6 Intelligent Network Management
The automation technology designed for the management of automated planning, automated configuration, automated healing, and automated optimization is called Intelligent Network Management (INM). The functionality and behavior of the INM are standardized by the 3GPP and NGMNs (Next-Generation Mobile Networks) consortium while undergoing continuous improvements. AI will play an instrumental role in making the private networks sustainable as it requires almost none physical or remote interventions. INM supports a real-time reconfigurable managed ecosystem with virtualization for fast and ultrafast services between centralized IPN and devices on the edge through AIA.
4.2.7 Critical Machine-Type Communication
The cMTC is the interaction of machines for the actuation of data collection for necessary and time-critical actions (Zhang, 2020). The network of machines such as sensors, RFID tags, and collection of smart antennas in massive numbers with no intervention of humans would be secure, robust, reliable, and consistently scalable to cope with high capacity and low-latency demands. The cMTC can also function by using multiple varying frequency bands where NGRS have been postulated as a potential solution offering a single IPN platform for the “ecosystem” to several connected IoT devices. cMTC provides coverage in challenging environments such as underground train tunnels where coverage penetration is difficult. It also employs faster responses through the use of faster data throughput, without limitations on the usage. The presented architecture has cMTC driven connected devices employing hybrid configuration with a control center to provide centralized control through IPN with edge intelligence for the connected devices.
4.2.8 Conversational Artificial Intelligence
CAI is an AI variant that enables people to communicate with machines such as applications and devices with the functionality of human-like natural language via voice, text, touch, or gesture. It helps in achieving tasks with proactiveness and personalized engagement (Dashtipour, 2016). CAI integrated with the rest of the network entities will feature the IPN as a living entity that interacts with all its users employing several social AI features hosted at the network edge. At first, when a user tries to say something, Natural Language Understanding (NLU) deciphers its meaning in the user’s understandable words regardless of how words have been stated. Even among user’s grammatical mistakes, the CAI is able to understand the user’s intent with NLU. It even works with user’s idiosyncrasies and shortcuts and remembers the context from one statement to another, comprehending what is being said throughout the conversation. At the second stage, CAI determines the right response based on its intelligence using ML and by understanding the user’s intent. Finally, using natural language generation, the CAI generates a user-friendly response for easy comprehension.
4.2.9 Ultrawideband
UWB is a radio technology that uses a wide frequency spectrum to locate and track people in real-time when short-range links are paramount. UWB has an unlicensed frequency range from 3.1 to 10.6 GHz. Private networks utilizing these bands within their premises can reduce the risk of interference among their applications or technologies. UWB is a potential alternative to other local area wireless technologies, such as WiFi, Bluetooth, and other WLAN technologies. The principal advantage of UWB which distinguishes its features from others is its offering of faster data transfer rates (100 Mbits/s up to 1 Gbits/s) in comparison. Extended battery and durability give UWB an edge over prominent wireless technologies deployed to date2.
5 IPN-Driven Edge Intelligent NGRS Architecture
In the following subsections, we discuss IPN-driven edge intelligence architecture comprised of centralized IPN connectivity and local edge decisions for NGRS (Niyato, 2020; Deng et al., 2020; Zhou et al., 2019; Dey et al., 2019; Dai et al., 2020). This integrates our use cases with the technologies provided at the edge under the light of network, application, and user-centric intelligence as shown in Figure 2. The presented architecture would also exploit service continuity as a PMN where an edge-computing solution will handle passenger’s mobility. IPN link is used for backup and restore operation and for major reporting purposes. Intelligent actions are done at the edge with locally captured data.
5.1 IPN Connectivity
The presented IPN is envisaged to have numerous edge devices in its network with profound connectivity links. It integrates edge devices and edge compatible applications into one smart network, thus forming a heterogeneous system environment (Niyato, 2020). IPN controls the functions of all connected links and devices by preprocessing through software-defined tools. The training of edge devices is a lightweight task that mainly relies on the edge servers performed by IPN in the initial setup. Once edge devices are trained, they take their intelligent decisions locally without IPN consultation on every step which reduces the overhead, time, and cost. IPN also allows direct connectivity to local cloud resource and offload major tasks to an on-premise server. The on-premise server then takes care of synchronization with the centralized cloud for reporting purposes.
5.2 Edge Caching
Under edge intelligence, edge caching is a simplistic method to effectively improve the performance of the IPN where it administers the incoming distributed data coming toward the edge devices from end-users and its surrounding environment (Zhang et al., 2018; Deng et al., 2020). This property exploits edge caching with virtually no setup overhead apart from sending control signals in the form of reporting to the IPN. Along with this property, the data generated by edge devices itself fall under edge caching. In our presented IPN, the data generated in IT2T communication, CTS, IDS, gadgets used in DSR, IMS, IWA, and MCPTT in ES is stored locally without the need to send every instance data back to centralized IPN. Also, the sensors-based cMTC such as RFID, NB-IoT, CAI, and mobile (accelerometer, gyroscope, and magnetometer) gather environment data which is processed and stored at reasonable places and is used by AI algorithms to provide services to users.
5.3 Edge Training
Traditionally the training of AI models works on a principle of centralized deployment architecture which carries heavy traffic in both communication directions (Kukreja et al., 2019; Zhou et al., 2019), eventually creating overhead. In our presented IPN, ML-driven federated learning technique also known as collaborative learning is used to train the AI model with an aim to port onto edge devices. The AI model runs on a powerful central server using its compatible edge software tools and runtime environment. With this approach, controlling, retraining, and deployment of models to edge devices using IPN cloud connectivity proves to be the most reliable and effective method for edge training. Once all the edge devices are intelligently trained, the communication link to the IPN would only be used for backup and restore operation or for reporting purposes where edge devices take their own decisions locally.
5.4 Edge Inference
Edge Inference is a process that holds responsibility for evaluating performance once the ML model is trained by datasets based on learnt computational outputs on edge devices (Niyato, 2020). The ML model is constructed and trained by powerful Central Processing Units (CPUs) and Graphical Processing Units (GPUs). These units support the fast processing of numerous functions such as face image recognition and remote assistance with data-hungry devices. Once deployed on the edge devices, they maintain their performance and resilience when intelligently designed to accelerate edge inference.
5.5 Edge Offloading
Under edge intelligence, edge offloading is another critical feature that actively offloads its tasks to other edge devices in a network (Dey et al., 2019). Similarly, the presented IPN uses edge offloading characteristics in its edge devices for offloading necessary tasks like edge training, edge caching, or edge inference in a distributed computing paradigm. This property exploits an intelligent ecosystem created by edge devices. Edge offloading is of utmost importance due to its resilient-like strategy by independent edge devices compared to edge caching, edge training, and edge inference. Effective implementation of IPN gives edge intelligence a structured way to available resources in the edge environment.
6 Conclusion and Future Research Directions
The NGRS will play an active role with edge intelligence integrated within the IPN domain. NGRS should be constructed using a variety of technologies and network configurations discussed in this article and other related researches and will also depend on geographic areas, deployment models, and application scenarios in the underground train environments. We presented several use cases, applications, required technologies, capacity needs, and measures in this article that we believe will provide opportunities for transport businesses to put B5G mobile networks on their private trail. Most of the modern researches have addressed or are currently investigating the traditional challenges of ITS. However, there seems to be a gap between 5G/B5G use cases and their applications for ITS. It is believed that these research gaps will drive future research to unlock the true potentials of ITS through edge intelligence. Below we list few critical parameters that will be important research themes in the context of NGRS realization through edge intelligent IPN.
• Low-latency communication: an important parameter for use cases like IT2T communication, Intelligent Drone Systems, and Intelligent Wireless Audio. The role of URLLC and mmWave will be critical toward the realization of low-latency communications.
• Communication with Diverse Bandwidth Requirements: to meet the challenges of variable data rate services like in the case of Transport Hub Crowd Management, Emergency Services, and Intelligent Depots, it will be important to develop systems that are capable of providing diverse data rates while meeting task completion deadlines.
• Low-power communication: for use cases like Contact-Tracing Systems and Intelligent Monitoring Services (approx. 15 years of battery life3), it will be crucial to establish low-power communications for sustainable NGRS solutions.
• Reliability: to ensure communication reliability in scenarios like Disaster Scenario Response and Emergency Services, there would be an important future research direction in the context of NGRS.
Furthermore, as we move toward a net zero carbon footprint, research on energy-efficient Heterogeneous Networks (HetNets) will also be required to address the energy consumption of NGRS IPN. Other challenges that would need to be addressed in order to make IPN open up for general public use will be to enable neutral hosting, security measures through blockchain, and dynamic spectrum sharing among mobile network operators for adaptive capacity protocols.
Data Availability Statement
The original contributions presented in the study are included in the article/Supplementary Material; further inquiries can be directed to the corresponding author.
Author Contributions
Conceptualization, SMA, SA, and SH; data curation, SMA and SA; formal analysis, SMA, SA, RR, and AA; funding acquisition, RR and MI; methodology, SMA and SH; project administration, SH, RR, QA, and MI; software, SA and SMA; supervision, SH, RR, QA, and MI; validation, QA and MI; visualization, SMA, SA, and QA; writing–original draft, SMA; writing–review and editing, SA and AA.
Funding
This work is supported in parts by Engineering and Physical Sciences Council (EPSRC) grant number EP/R511705/1 along with partial funds by Deanship of Graduate Studies and Research (DGSR), Ajman University, under the grant number 2020-IRG-ENIT-10.
Conflict of Interest
The author SMA is employed by the company Transport for London (TfL) as a Senior Network and Telecoms Architect.
The remaining authors declare that the research was conducted in the absence of any commercial or financial relationships that could be construed as a potential conflict of interest.
Publisher’s Note
All claims expressed in this article are solely those of the authors and do not necessarily represent those of their affiliated organizations or those of the publisher, the editors, and the reviewers. Any product that may be evaluated in this article or claim that may be made by its manufacturer is not guaranteed or endorsed by the publisher.
Footnotes
1https://www.nokia.com/networks/portfolio/mobile-transport/.
2Ultra-Wideband (UWB) Ofcom document can be found at: https://www.ofcom.org.uk/__data/assets/pdf_file/0015/25152/uwb.pdf.
3Narrowband-IoT Vodafone document can be found at: https://www.vodafone.com/business/iot/managed-iot-connectivity/nb-iot.
References
Akbar, M. A., and Azhar, T. N. (2018). “Concept of Cost Efficient Smart Cctv Network for Cities in Developing Country,” in 2018 International Conference on ICT for Smart Society (ICISS), Semarang, Indonesia, 10-11 Oct. 2018 (IEEE), 1–4. doi:10.1109/ICTSS.2018.8550016
Ansari, S., Boutaleb, T., Sinanovic, S., Gamio, C., and Krikidis, I. (2018). “On the Design and Deployment of Multitier Heterogeneous and Adaptive Vehicular Networks,” in 2018 11th International Symposium on Communication Systems, Networks Digital Signal Processing (CSNDSP), Budapest, Hungary, 18-20 July 2018 (IEEE), 1–6. doi:10.1109/CSNDSP.2018.8471807
Asad, S. M., Ahmad, J., Hussain, S., Zoha, A., Abbasi, Q. H., and Imran, M. A. (2020a). Mobility Prediction-Based Optimisation and Encryption of Passenger Traffic-Flows Using Machine Learning. Sensors 20, 2629. doi:10.3390/s20092629
Asad, S. M., Ansari, S., Ozturk, M., Rais, R. N. B., Dashtipour, K., Hussain, S., et al. (2020b). Mobility Management-Based Autonomous Energy-Aware Framework Using Machine Learning Approach in Dense mobile Networks. Signals 1, 170–187. doi:10.3390/signals1020010
Asad, S. M., Dashtipour, K., Hussain, S., Abbasi, Q. H., and Imran, M. A. (2020c). “Travelers-tracing and Mobility Profiling Using Machine Learning in Railway Systems,” in 2020 International Conference on UK-China Emerging Technologies (UCET), Glasgow, UK, 20-21 Aug. 2020 (IEEE), 1–4. doi:10.1109/UCET51115.2020.9205456
Asad, S. M., Ozturk, M., Bin Rais, R. N., Zoha, A., Hussain, S., Abbasi, Q. H., and Imran, M. A. (2019). “Reinforcement Learning Driven Energy Efficient Mobile Communication and Applications,” in Reinforcement learning driven energy efficient mobile communication and applications, Ajman, United Arab Emirates, 10-12 Dec. 2019 (IEEE), 1–7. doi:10.1109/ISSPIT47144.2019.9001888
Banchs, A., Gutierrez-Estevez, D. M., Fuentes, M., Boldi, M., and Provvedi, S. (2019). A 5g mobile Network Architecture to Support Vertical Industries. IEEE Commun. Mag. 57, 38–44. doi:10.1109/MCOM.001.1900258
Dai, Y., Zhang, K., Maharjan, S., and Zhang, Y. (2020). Edge Intelligence for Energy-Efficient Computation Offloading and Resource Allocation in 5g beyond. IEEE Trans. Veh. Technol. 69, 12175–12186. doi:10.1109/tvt.2020.3013990
Dashtipour, K., HussainCambria, A. E., Hawalah, A. Y. A., Gelbukh, A., and Zhou, Q. (2016). Multilingual Sentiment Analysis: State of the Art and Independent Comparison of Techniques. Cogn. Comput. 8, 757–771. doi:10.1007/s12559-016-9415-7
Dat, P. T., Kanno, A., Yamamoto, N., and Kawanishi, T. (2016). 5g Transport Networks: the Need for New Technologies and Standards. IEEE Commun. Mag. 54, 18–26. doi:10.1109/MCOM.2016.7565268
Deng, S., Zhao, H., Fang, W., Yin, J., Dustdar, S., and Zomaya, A. Y. (2020). Edge Intelligence: The confluence of Edge Computing and Artificial Intelligence. IEEE Internet Things J. 7, 7457–7469. doi:10.1109/JIOT.2020.2984887
Dey, S., Mondal, J., and Mukherjee, A. (2019). “Offloaded Execution of Deep Learning Inference at Edge: Challenges and Insights,” in 2019 IEEE International Conference on Pervasive Computing and Communications Workshops (PerCom Workshops), Kyoto, Japan, 11-15 March 2019 (IEEE), 855–861. doi:10.1109/PERCOMW.2019.8730817
Fioranelli, F., Le Kernec, J., and Shah, S. A. (2019). Radar for Health Care: Recognizing Human Activities and Monitoring Vital Signs. IEEE Potentials 38, 16–23. doi:10.1109/MPOT.2019.2906977
Ford, R., Zhang, M., Mezzavilla, M., Dutta, S., Rangan, S., and Zorzi, M. (2017). Achieving Ultra-low Latency in 5g Millimeter Wave Cellular Networks. IEEE Commun. Mag. 55, 196–203. doi:10.1109/MCOM.2017.1600407CM
Gang Wang, G., Xiaoqing Zeng, X., and Tengfei Yuan, T. (2017). “Study on the Influence of Train Control System on Service Quality of Rail Transit,” in 2017 International Conference on Service Systems and Service Management, Dalian, China, 16-18 June 2017 (IEEE), 1–4. doi:10.1109/ICSSSM.2017.7996124
Haider, D., Ren, A., Fan, D., Zhao, N., Yang, X., Shah, S. A., et al. (2019). An Efficient Monitoring of Eclamptic Seizures in Wireless Sensors Networks. Comput. Electr. Eng. 75, 16–30. doi:10.1016/j.compeleceng.2019.02.011
Hilt, A. (2019). Availability and Fade Margin Calculations for 5g Microwave and Millimeter-Wave Anyhaul Links. Appl. Sci. 9, 5240. doi:10.3390/app9235240
Hussain, B., Du, Q., Imran, A., and Imran, M. A. (2020). Artificial Intelligence-Powered mobile Edge Computing-Based Anomaly Detection in Cellular Networks. IEEE Trans. Ind. Inf. 16, 4986–4996. doi:10.1109/tii.2019.2953201
Katsalis, K., Nikaein, N., Schiller, E., Ksentini, A., and Braun, T. (2017). Network Slices toward 5g Communications: Slicing the Lte Network. IEEE Commun. Mag. 55, 146–154. doi:10.1109/MCOM.2017.1600936
Klaine, P. V., Imran, M. A., Onireti, O., and Souza, R. D. (2017). A Survey of Machine Learning Techniques Applied to Self-Organizing Cellular Networks. IEEE Commun. Surv. Tutorials 19, 2392–2431. doi:10.1109/comst.2017.2727878
Klaine, P. V., Nadas, J. P. B., Souza, R. D., and Imran, M. A. 2018). Distributed Drone Base Station Positioning for Emergency Cellular Networks Using Reinforcement Learning. Cogn. Comput. , 10, 790, 804. doi:10.1007/s12559-018-9559-8
Kukreja, N., Shilova, A., Beaumont, O., Huckelheim, J., Ferrier, N., Hovland, P., and Gorman, G. (2019). “Training on the Edge: The Why and the How,” in 2019 IEEE International Parallel and Distributed Processing Symposium Workshops (IPDPSW), Rio de Janeiro, Brazil20-24 May 2019 (IEEE), 899–903. doi:10.1109/IPDPSW.2019.00148
Li, R., Zhao, Z., Zhou, X., Ding, G., Chen, Y., Wang, Z., et al. (2017). Intelligent 5g: When Cellular Networks Meet Artificial Intelligence. IEEE Wireless Commun. 24, 175–183. doi:10.1109/MWC.2017.1600304WC
Liu, L., Shah, S., Zhao, G., and Yang, X. (2018). Respiration Symptoms Monitoring in Body Area Networks. Appl. Sci. 8, 568. doi:10.3390/app8040568
Niyato, D., and Yan, X. (2020). Edge AI: Convergence of Edge Computing and Artificial Intelligence. Singapore: Springer.
Parkvall, S., Dahlman, E., Furuskar, A., and Frenne, M. (2017). Nr: The New 5g Radio Access Technology. IEEE Comm. Stand. Mag. 1, 24–30. doi:10.1109/MCOMSTD.2017.1700042
Pilz, J., Holfeld, B., Schmidt, A., and Septinus, K. (2018). Professional Live Audio Production: A Highly Synchronized Use Case for 5g Urllc Systems. IEEE Netw. 32, 85–91. doi:10.1109/MNET.2018.1700230
Ren, A., Zahid, A., Zoha, A., Shah, S. A., Imran, M. A., Alomainy, A., et al. (2020). Machine Learning Driven Approach towards the Quality Assessment of Fresh Fruits Using Non-invasive Sensing. IEEE Sensors J. 20, 2075–2083. doi:10.1109/jsen.2019.2949528
Sambo, Y. A., Klaine, P. V., Nadas, J. P. B., and Imran, M. A. (2019). “Energy Minimization Uav Trajectory Design for Delay-Tolerant Emergency Communication,” in 2019 IEEE International Conference on Communications Workshops (ICC Workshops), Shanghai, China, 20-24 May 2019 (IEEE), 1–6. doi:10.1109/ICCW.2019.8757127
Sanchoyerto, A., Solozabal, R., Blanco, B., and Liberal, F. (2019). Analysis of the Impact of the Evolution toward 5g Architectures on mission Critical Push-To-Talk Services. IEEE Access 7, 115052–115061. doi:10.1109/ACCESS.2019.2930936
Solomitckii, D., Gapeyenko, M., Semkin, V., Andreev, S., and Koucheryavy, Y. (2018). Technologies for Efficient Amateur Drone Detection in 5g Millimeter-Wave Cellular Infrastructure. IEEE Commun. Mag. 56, 43–50. doi:10.1109/mcom.2017.1700450
Wang, X., Han, Y., Wang, C., Zhao, Q., Chen, X., and Chen, M. (2019). In-edge Ai: Intelligentizing mobile Edge Computing, Caching and Communication by Federated Learning. IEEE Netw. 33, 156–165. doi:10.1109/MNET.2019.1800286
Yang, X., Fan, D., Ren, A., Zhao, N., and Alam, M. (2019). 5g-based User-Centric Sensing at C-Band. IEEE Trans. Ind. Inf. 15, 3040–3047. doi:10.1109/TII.2019.2891738
Zhang, K., Leng, S., He, Y., Maharjan, S., and Zhang, Y. (2018). Cooperative Content Caching in 5g Networks with mobile Edge Computing. IEEE Wireless Commun. 25, 80–87. doi:10.1109/MWC.2018.1700303
Zhang, L. (2020). Internet of Things and Sensors Networks in 5G Wireless Communications. doi:10.3390/books978-3-03928-149-7
Zhou, Z., Chen, X., Li, E., Zeng, L., Luo, K., and Zhang, J. (2019). Edge Intelligence: Paving the Last Mile of Artificial Intelligence with Edge Computing. Proc. IEEE 107, 1738–1762. doi:10.1109/JPROC.2019.2918951
Keywords: B5G, edge intelligence, NB-IoT, intelligent transport systems, intelligent private network, next generation transport systems, 6G
Citation: Asad S, Tahir A, Rais R, Ansari S, Abubakar A, Hussain S, Abbasi Q and Imran M (2021) Edge Intelligence in Private Mobile Networks for Next-Generation Railway Systems. Front. Comms. Net 2:769299. doi: 10.3389/frcmn.2021.769299
Received: 01 September 2021; Accepted: 20 September 2021;
Published: 14 October 2021.
Edited by:
Akram Alomainy, Queen Mary University of London, United KingdomReviewed by:
Syed Aziz Shah, Coventry University, United KingdomXiaodong Yang, Xidian University, China
Aifeng Ren, Xidian University, China
Copyright © 2021 Asad, Tahir, Rais, Ansari, Abubakar, Hussain, Abbasi and Imran. This is an open-access article distributed under the terms of the Creative Commons Attribution License (CC BY). The use, distribution or reproduction in other forums is permitted, provided the original author(s) and the copyright owner(s) are credited and that the original publication in this journal is cited, in accordance with accepted academic practice. No use, distribution or reproduction is permitted which does not comply with these terms.
*Correspondence: Syed Muhammad Asad, cy5hc2FkLjFAcmVzZWFyY2guZ2xhLmFjLnVr