Radio frequency sensing and its innovative applications in diverse sectors: A comprehensive study
- 1James Watt School of Engineering, University of Glasgow, Glasgow, United Kingdom
- 2Department of Telecommunication Engineering, Peshawar, Pakistan
- 3School of Engineering and Physical Science, Heriot-Watt University, Edinburgh, United Kingdom
- 4Electronics Science and Engineering, University of Electronic Science and Technology of China, Chengdu, China
- 5Department of Computer Science, King Fahad Naval Academy, Al Jubail, Saudi Arabia
Recent advancements in radio frequency (RF) sensing technology can be attributed to the development of the Internet of Things (IoT), healthcare, RF-identification, and communication applications. RF sensing is a multidisciplinary research field that requires expertise in computing, electronics, and electromagnetics to cover all system features, including protocol development, antenna design, sensor integration, algorithm formulation, interconnection, data, and analytics. The overarching aim of this work is to present detailed information about RF technologies and their innovations and application diversity from the novel work carried out at CSI Lab 1, together in one platform with an extensive survey. This study presents state-of-the art applications and RF sensing that include W-Fi, radar, and SDR and RFID-based sensing. A comprehensive survey and study of the advantages and limitations of each non-contact technology is discussed. Additionally, open research gaps have been identified as well. Decades of knowledge and experience have been put to use to meet new challenges and demands. The development and study of RF systems, IoT, RFID sensing, and research and deployment activities, are briefly discussed. The emerging research projects with industry, institutional research centers, and academic studies are also addressed. Finally, an outline of identified potential future research areas is provided, emphasizing opportunities and challenges.
1 Introduction
Due to rapid advancements in communication technology, multiple equipment and devices are now capable of communicating with one another inside a network, referred to as the Internet of “Things.” The growth of the IoT benefits several developing applications. In general, these applications are divided into three layers: sensing, gateway, and cloud. Studies have extensively used RF signals to collect events in the IoT context (i.e., RF sensing). While RF signals are transmitted, reflected, obstructed, and dispersed by things such as buildings, furnishings, automobiles, and living beings, it is possible to gather relevant data from received RF signals such as one’s position, moving directions, speed, and vitals. RF sensing, as opposed to traditional hardware sensors, provides consumers with low-cost and unobtrusive services. Furthermore, because RF signals are broadcast, they may be utilized not just to monitor multiple individuals but also to record changes in the environment across a vast region. With the use of RF technologies, end-users do not need to carry equipment or disrupt their daily routine. RF signals are used to monitor macro and micro activity and to track objects. This technology revolution enables many emerging applications and services to the market, driving innovation. The IoT and RF-identification (RFID) technologies for RF sensing play a significant part in the industrial revolution. RFID emerged as an identifying technology in industrial applications such as supply chain tracking; however, in recent years, RFID devices have gained popularity beyond identification due to their reliable and durable features in the field of healthcare, asset management, retail sales, tap-and-go payments, and many other industrial applications. RFID is considered an innovative sensing paradigm due to its ease of fabrication, low costs, miniaturized dimensions, flexibility, endurance for extreme environments, passive activation power requirements, effective read ranges, and beyond line-of-sight communications. From its conventional use in tracking and identification applications, RFID has a great significance in RF sensing, IoT industries, and smart healthcare advancements with enormous applications to smartly connect and monitor devices remotely. Novel antenna design, tag-sensing, biomedical and wearable sensors, chipless-RFID, enhanced localization, and tracking systems are hot subjects for researchers. Figure 1 shows some of the potential contactless sensing applications.
RF sensing is a multi-discipline research domain that requires significant knowledge of computing, electronics, and electromagnetics to address system features such as protocol creation, antenna design, sensor integration, algorithm development, communications, and data analysis. In parallel, by allowing devices to connect and exchange data, the IoT creates a nearly limitless number of opportunities for sensing-based technologies. The capacity to link physical equipment or assets, to improve service, efficiency, and performance is the underlying economic value of the Internet of Things domain. These attributes help the industrial sector businesses to meet their objectives that include cost reductions, enhanced operational efficiency, increased productivity, less business risk, and improved compliance. About every IoT application needs a data connection between the digital and physical world, and RFID is the effective key enabler for doing so (Nepa et al., 2021).
The communication, sensing, and imaging (CSI) research team has achieved great milestones in the research field ranging from novel RFID antenna designs and developing commercial IoT applications to continued work on non-invasive healthcare innovations using RF sensing (Imran et al., 2020a; Cui et al., 2020). This article provides an overview of CSI’s significant research projects in the field of RFID, IoT, and RF sensing. Some research initiatives are highlighted with a focus on industrial partners or other institutes. The diverse applications of these technologies with their quantifying measuring parameters and the technical challenges in each application are discussed wherever required. This survey will provide up-to-date knowledge on innovative techniques utilized in the field of radio frequency/wireless communication through the diverse use-cases from the CSI group, which is known to have the best 5G center in Scotland, United Kingdom, and a world-class nano-fabrication center. The group has identified potential novel research areas to be worked on in future while emphasizing the opportunities and challenges.
2 Contactless sensing: RFID and IoT
RFID and IoT are promising technologies to revolutionize the world beyond detection, environmental applications, transportation, and in the health and welfare sectors. The paradigm shift of RFID applications from the traditional market to modern applications is worth mentioning here, such as intelligent transportation systems (Sodhro et al., 2019), smart home applications (Sodhro et al., 2020), IoT applications for speech recognition (Ge et al., 2020), and so on. Figure 2 shows an overview of the RFID paradigm shift and promising application areas of IoT overlapping with RFID.
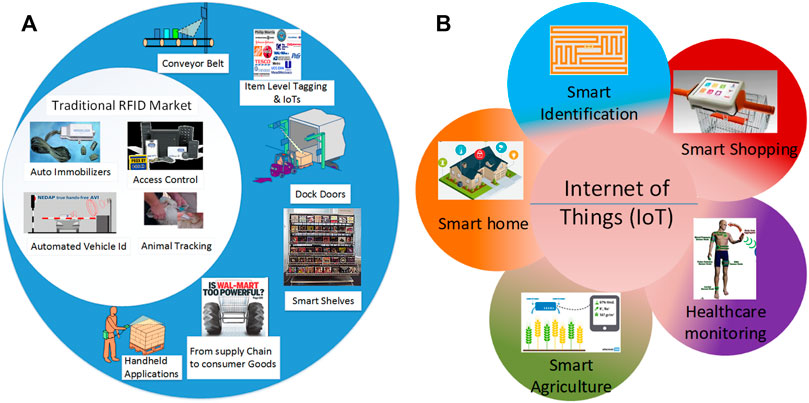
FIGURE 2. Paradigm shift of RFID applications. (A) From traditional to modern applications. (B) Promising application areas of IoT overlapping with RFID.
Though RFID tags can be designed in the low frequency (LF) and high frequency (HF) ranges, ultra-high frequency (UHF) is the most used band in RFID applications for its simple design structures and printing options. UHF-RFID tags are ideal for most applications due to their easy fabrication process, compact sizes, long read ranges, flexibility, and multiple optimization and form factors which are application-specific. The approved frequency bands for RFID operations are not similar worldwide. The European Telecommunications Standards Institute (ETSI/EU) approved a bandwidth of 865–868 MHz and 915–921 MHz, the Federal Communications Commission (FCC/US) approved a bandwidth of 902–928 MHz, most regions of Asia use 865–868 MHz, China uses 840–845 MHz and 920–925 MHz bands, Japan 915–928 MHz, Australia 920–926 MHz, whereas the global range is 860–960 MHz (GS1 (2022)). Therefore, the tag/readers need to be designed that can operate in accordance with the specified frequency ranges for various regions.
The practical problem encountered while testing the tags in real use-case applications is their inability to perform well in the vicinity of different surface environments such as metal, liquid, human body, and other high-permittivity materials. To explore solutions to the mentioned problems, Sharif et al. (2021b) designed a novel RFID tag antenna and developed a complete contactless delivery system for drinks, medicine, food, and similar items. It is a standalone tag antenna system with blockchain technology for safe, real-time supply chain management, traceability, and verification of hard-to-tag objects such as liquid bottles containing various densities of liquids such as oil, water, soft drinks, or wine. To achieve a good antenna-to-chip impedance match on high permittivity surfaces, the characteristic mode analysis (CMA) theory is implemented and a low-cost inkjet-printed folded dipole antenna is designed with a nested loop technique. The optimization and tuning of the tag is carried out by observing the characteristic modes of the object surface, which in the current case, is the plastic water bottles with different permittivity of ɛr = 3.4 and 3.1, respectively. The dielectric constant of water is taken as 79.5% for the simulation environment in a CST microwave studio. Figure 3 shows commercial retail deployment and successful testing of an automatic refrigerator system based on smart shelves using RFID. This system is billed using blockchain ledgers and its mining time is shown on the right in the figure. The indoor lab tests were carried out in the CSI-IoT lab using the Tagformance Pro device and the Tag Designer Suite (TDS) software. Tagformance is a complete measurement solution by Voyantic for evaluating the performance of RFID tags commercially. The setup of the lab experiments is shown in Figure 4.
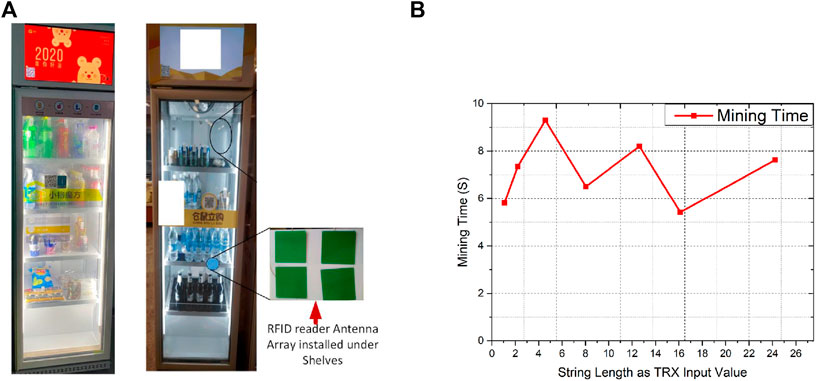
FIGURE 3. Commercial setup of a blockchain-based smart refrigerator for RFID-tagged liquid bottles in supply chain management. (A) Auto refrigerator system with RFID antenna shelves for billing tagged water bottles. (B) Mining time of blockchain transactions (Sharif et al., 2021b).
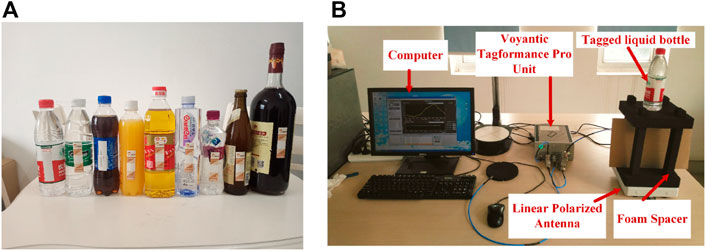
FIGURE 4. Lab setup of RFID-tagged liquid bottles using the Voyantic Tagformance Unit. (A) Fabricated tag antenna mounted on liquid bottles. (B) Tagformance Pro setup for testing (Sharif et al., 2021b).
The read range obtained after putting the tag on liquid-filled plastic bottles is 6.5 and 2.6 m on glass bottles. The suggested UHF RFID tag can be printed directly onto the bottles. The real-time billing app developed by the team for this application is implemented using blockchain smart contracts. It gives every single item a unique identification for real-time monitoring of liquid bottles. The smart contract can read and record information in the blockchain ledger. Using the suggested technology, a smart contract can be created for each item automatically to enhance consumer–supplier trust, and also, the tag can be printed directly onto the bottles.
By exploiting the significant modes using the concepts of the CM theory, Sharif et al. (2019f) proposed a novel RFID tunable flag-tag antenna design that works perfectly for metallic surfaces and dielectric materials. The concept is being applied for a retail application of metallic cans and a low-cost smart refrigerator system with automated payment and inventory restoration functions along with a smart can-trash bin for the IoT. The developed system is successfully tested and verified in a real use-case demonstration setup with 97.5% successful billing accuracy. The novelty of the design lies in the fact that it employed an existing metal-can structure as a radiator for its tag, utilizing the CM of the can structure. Figure 5 shows the mesh views of the metal can using 6230 flat triangles and “Rao Wilton Glisson” basis functions. The MATLAB code with mode tracking is created to perform CMA. Furthermore, a leaf-shaped radiator is proposed by Sharif et al. (2019a) which is fabricated on an FR4 substrate using the CM theory. The read range obtained is 3.5 m on non-metallic objects and 5 m on metallic objects. The tag is designed and chosen after experimenting with various radiating antenna structures and parametric analyses. The suggested tag is a folded patch with a feeding loop which is stacked on two substrate layers. The folded patch is tuned for resonance at 915 MHz using characteristic modes. The antenna is prototyped using a 2 mm thick FR4 substrate where the impedance matching with the chip is achieved using an inductive feeding loop. The tag works well in the FCC range with 10 dB return loss and can be tuned to the ETSI frequency range by introducing additional metallic tracks to the structure. Furthermore, the proposed design is low-cost since no vias or shorting pin is required. It can be read from a distance of 3.5 m in free space and 5 m on a 200 × 200 mm metal plate. Figure 6 shows the commercial setup of this project.
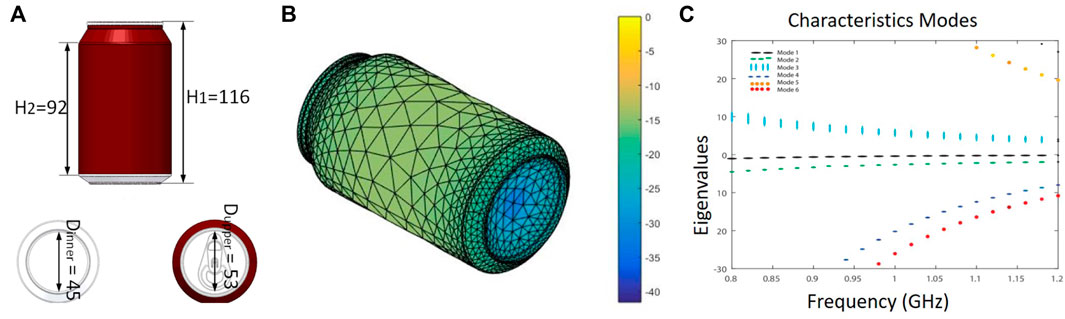
FIGURE 5. CMA of the metallic can. (A) Metallic can dimensions. (B) Mesh view using RWG functions. (C) Eigenvalues of characteristic modes as a function of frequency (Sharif et al., 2019e).
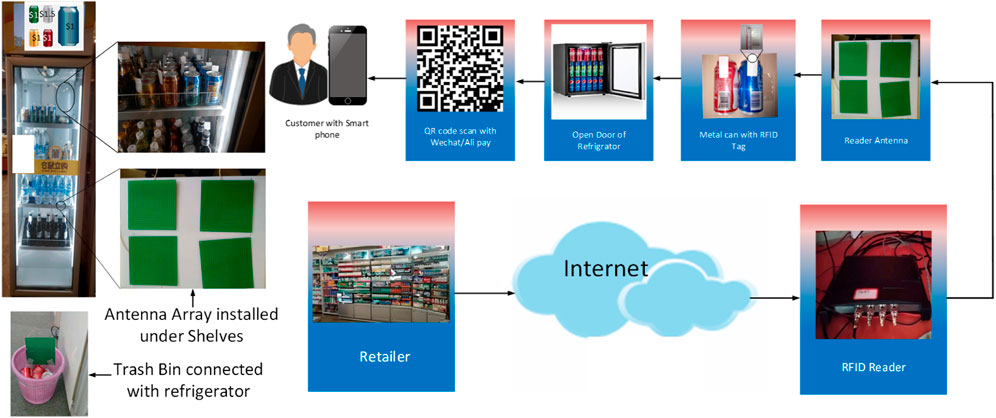
FIGURE 6. Commercial testing setup and block diagram of an RFID-based smart refrigerator (Sharif et al., 2019a).
Particle swarm optimization (PSO) and the theory of characteristic modes are exploited by Sharif et al. (2021c) to design a logarithmic spiral near-field RFID tag sensor based on uniform magnetic field characteristics. Using this tag in sensing applications such as human body temperature monitoring and commercial item-level labeling of high-end jewelry is an exciting prospect.
A novel dual-polarized planar pyramid UHF-RFID antenna is proposed by Althobaiti et al. (2020). This research is featuring polarization-frequency band diversity using characteristic mode analysis. The upper patch of the design wrapped around the FR4 substrate is shorted to the ground plane using stubs. Using CMA, the design is updated with slots placed diagonally to alter the resonance of corresponding modes for a lower operating band. However, at the lower band, the updated design exhibits inductive characteristics in the FCC band. The parallel slots can be utilized to evaluate the resonant modes in any required RFID band. For diversity mode analysis, the chip is placed at the current minima directly. With a very compact structure of just 50 × 50 × 2 mm dimension in-spite dual-polarized properties, it is low cost and easy to prototype, achieving a read range of 5.5 and 8.5 m on OFF/ON metallic surfaces, respectively. A similar technique is also utilized by Sharif et al. (2019c) to design an orientation-independent tag with polarization diversity.
A machine learning-based RFID system is developed by Sharif et al. (2021a) to detect contamination in food items. An overview of the methodology is shown in Figure 7. For contamination sensing, printed RFID stickers are used. The Tagformance Unit is used to measure RSSI while XGboost is used to train the model. Its accuracy is 90%, which lays the path for pervasive contamination monitoring utilizing RFID machine learning to inform people about health and food safety.
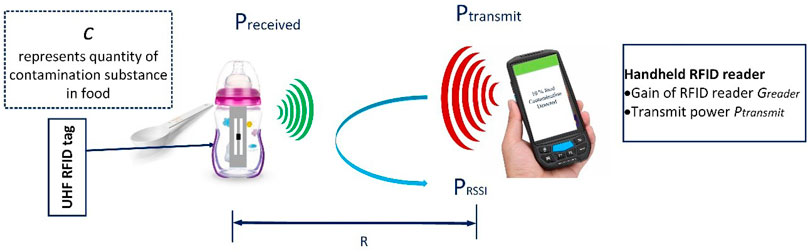
FIGURE 7. Machine learning-based food contamination detection using RFID (Sharif et al., 2021a).
Ouyang et al. (2019) tested an optimized low-profile RFID tag antenna for fruit labeling. The label was successfully tested for automatic billing in departmental stores’ setups. The labels have the capability to store useful information for customers related to the fruit’s farm location, picking date, price, expiry, and other useful data. Sharif et al. (2019d) successfully tested RFID sensors for salinity-sugar contents of water by measuring the back-scattered power mechanism. This research paves the path for portable device-based liquid analysis, which is beneficial for healthcare applications in future IoT. A wearable wide-band paper-printed RFID antenna is prototyped by Sharif et al. (2019b) for healthcare applications within a global frequency band of 860–960 MHz with a read range of 5.5 m on the human body while 8.5 m on metallic objects. The human body effects are mitigated by introducing the Hilbert fractal curves in the antenna design. A long-range tag antenna is proposed by Sharif et al. (2018) with a 9.5 m read range. This antenna platform is resilient and environment-independent due to its capacity to function on tough surfaces such as metal, wood, and the human body.
Sensing applications benefit from the UHF tag’s sensitivity capabilities. The integration of RFID-IoT tags in healthcare applications has recently resulted in improved patient convenience, cost savings, tracking, and more organized patient healthcare observations. Figure 8 shows the use of a novel tag by Sharif et al. (2019e) for healthcare applications that works well in the proximity of water, IV solution, and blood phantom bags and can detect wound heal conditions. The sensing potential of this tag makes it suitable for use in IV-solution level observations, blood-bags’ remote management, and other remote healthcare applications which can greatly reduce the workload of medical staff. Moreover, to track and tag medicines and pills, a spider web-shaped conformal tag is proposed by Sharif et al. (2020) that works in the near-field with uniform electrical field characteristics.
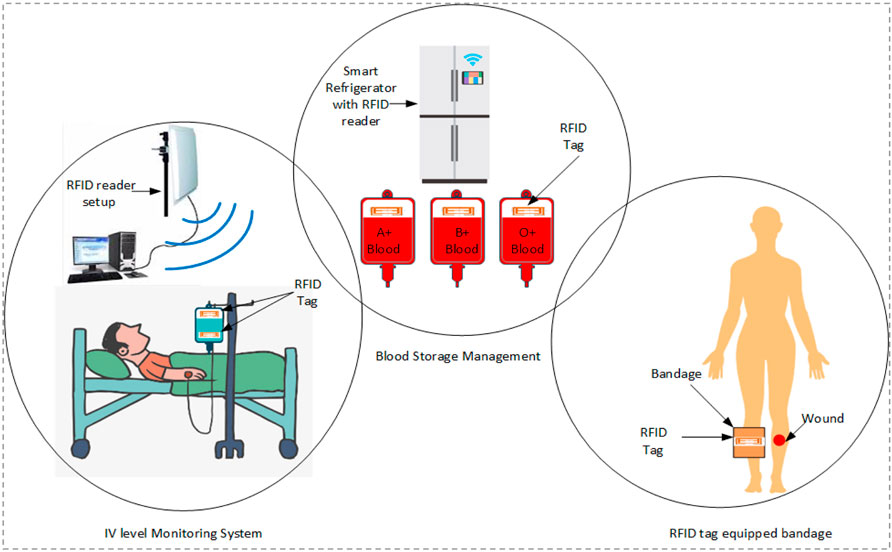
FIGURE 8. Schematic diagram for the potential use of the RFID-IoT tag in healthcare applications (Sharif et al., 2019e).
The IoT and its industrial applications will serve up the fourth industrial revolution since IoT-based connectivity is speculated to reach billions by 2021–22. The standardization and diligent research into technology have also resulted in significant advancements. As 3GPP provides a concise and unique visual description of the narrow band-IoT standardization process, Dangana et al. (2021) highlighted some of the aspects of a wireless sensor network, IoT, industrial-IoT, NB-IoT, and the technological issues they face. This work outlines the obstacles that must be overcome by wireless communication technology to be productive in an industrial setting. For the agriculture industry, a smart fertilization and irrigation assistance user-friendly smartphone app is presented by Sun et al. (2020). Using moisture sensors and optical transducers, the system is designed to monitor changes in soil moisture. It can detect the nitrogen, phosphorus, and potassium levels of houseplants and notify the owner about soil conditions via email. For care taking of people suffering from dementia and sleep disorders, a wearable monitoring system using an IoT platform is presented by Hu et al. (2020). The prototype is developed and tested using the MySignals HW Complete Kit, Arduino Uno microcontroller, LEDs, speakers, micro SD card, micro SD card reader, SPI interface, and esp8266 module, and real-time data transmission, processing, visualizing, and storage are technically realized. This device tracks sleep patterns, provides illumination while the user is awake at night, helps them fall asleep with an audio assistance function, and if required, triggers an emergency alert to caretakers if the person remains sitting or standing for a prolonged period at night. In future, machine learning and sleep-pattern recognition techniques can be analyzed using mass datasets with real biometric readings.
The idea for adopting IoT technology into smart homes is to address the smart living aspect of future smart homes and to assure comfort, safety, and security. The wide use cases of smart living seen in everyday lives include smart doors with a sense of automatic opening and closing, smart lighting systems, heating systems, ventilation systems, security systems, smart healthcare. Xu et al. (2020)prioritized the security aspects of physical devices using perspective and network layers while designing the smart home system with the help of Arduino and Raspberry Pi. The novelty of this work is the entrance system which connects the door lock and surveillance system with facial recognition algorithms, where face recognition is required before fingerprint or password unlocking. A successful prototype for smart home barriers and entry systems for kids and pets is presented by Zhang et al. (2020), showing access to the kitchen and other sensitive areas being controlled in real-time using an e-platform called Ubidots. Utilizing the same platform, the air quality index is measured in an indoor environment by employing humidity, temperature, and dust sensors and communicated frequently over text messages to improve the air quality (Zhou et al., 2020). Using the Alibaba Cloud IoT platform on STM32, Wu et al. (2020) presented a smart lighting system for smart cities that can save electricity with a remote controlling feature.
The IoT systems can interact with the environment and enhance processes by learning through interactions owing to developments in sensing and communication technologies. This will result in the development of intelligent environments and self-aware networked “things” for applications in the fields of healthcare, transportation, the digital society, agriculture, energy, and the ecosystem.
3 RF sensing, 5G, and beyond
With a vision of sustainable healthcare sector, the CSI Lab is actively working toward non-invasive, proactive, and predictive healthcare contactless techniques. Future use cases of intelligent contactless sensing are shown in Figure 9.
Software-defined radio-based Testbed for large-scale body movement using KNN-based machine learning classification with an accuracy of up to 90% is proposed by Ashleibta et al. (2020). This novel non-invasive approach will pave the way for the development of a sustainable framework for future healthcare systems. The fundamental aim was to analyze channel-state-information comprising two USRP platforms. Various body movements were recorded and analyzed in a furnished indoor lab environment while the data were classified using DT, KNN, NB, and DT machine learning algorithms. The CSI-based data are compared with the proposed platform, concluding that this framework comes with an advantage of frequency-carrier modification and transmitted/received power alteration. Similarly, in a non-invasive next-generation healthcare application, motion detection using artificial intelligence is performed using different NN, KNN, and SVM algorithms by Song et al. (2021). Various stages of wireless sensor body networks for next-generation health applications are shown in Figure 10 (Alkhayyat et al., 2019). An enhanced IoT system is presented in this research for stage-one efficiency.
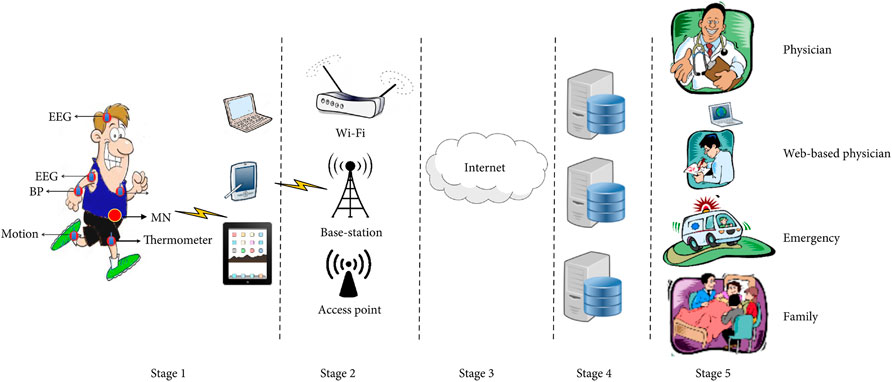
FIGURE 10. Wireless sensor body networks for IoT-based healthcare applications (Alkhayyat et al., 2019).
The serious consequences of falls in the elderly have prompted the creative use of technology to create systems sensitive enough to detect and notify fall incidents as and when they occur. Tahir et al. (2022) presented a detailed discussion on the essentials of IoT-based fall-detection system for elders. A complete overview of considerable deep learning and machine learning algorithms is discussed along with Edge, Fog, and Cloud-IoT layers and their performance metrics for result validation.
A wide-band body-centric wearable millimeter wave antenna prototype is proposed by Ur-Rehman et al. (2018) for the 5G IoT industry. Surface current distribution is used in the design concept at 60 GHz frequency with an antenna gain of 9.6 dBi. The current efficiency is 70% which needs to be improved further.
Ashleibta et al. (2021b)used a 5G-enabled system with an operating frequency of 3.75 GHz to detect single and multi-subject 16 movements which are shown in Figure 11. For this purpose, experiment data were recorded in the form of CSI amplitudes with 51 wireless sub-carriers analyzed and concatenated to record multi-subject motion. After collecting the data, they were fed into a deep learning algorithm and 83% accuracy was found in terms of multi-subject with 16 classification categories. Khan et al. (2022) proposed non-invasive indoor human activity detection for smart home applications which is shown in Figure 12. The main focus of this study was to break the limit of localization and find the location of patients using universal software-defined radio peripheral (USRP) devices. CSI samples are collected by observing a single individual do no activity, standing, sitting, and forward-leaning activities in various places within a room. After collecting the CSI amplitude data, calculating the feature from this, and then applying different machine learning algorithms, 96% accuracy was found from different locations in the room.
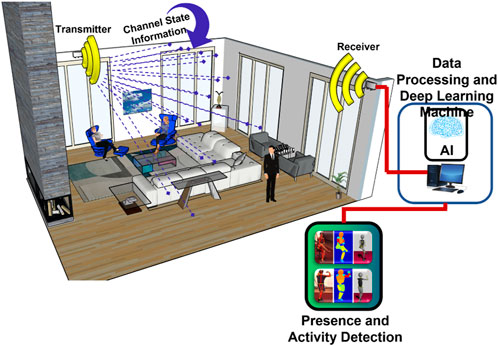
FIGURE 11. Schematic of multi-subject health monitoring using Wi-Fi signals (Ashleibta et al., 2021b).
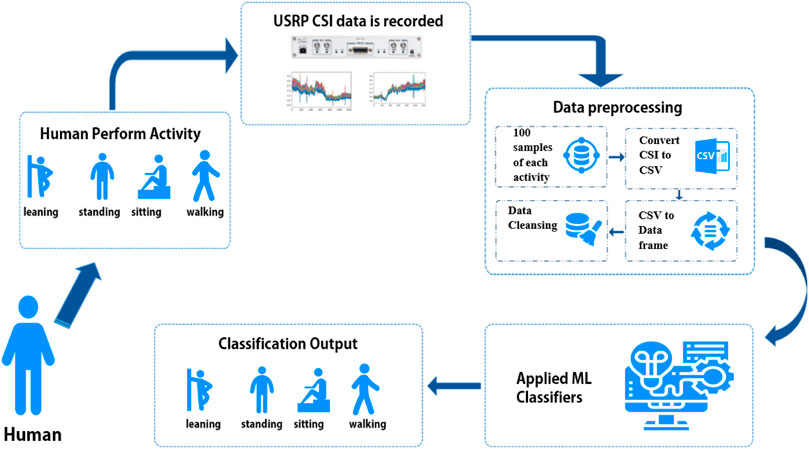
FIGURE 12. Block diagram demonstrating non-invasive indoor human activity detection for smart home applications using software-defined radios (Khan et al., 2022).
Freezing of gait (FOG) is the onset of disabilities in Parkinson’s disease (PD) patients due to an episodic lack of forward movement and disrupting daily activities. An overview of the concept is shown in Figure 13. In this work, a Wi-Fi-based system was proposed which used a 5G spectrum for the detection of daily routine FOG activity. A total of 225 events involving 45 FOG cases are taken from 15 patients using 30 sub-carriers, and the classification of a deep neural network with high accuracy of more than 90% was achieved (Tahir et al., 2019). Ashleibta et al. (2021a) proposed non-contact breathing detection and heart rate monitoring using Wi-Fi which is shown in Figure 14. Data were recorded in the form of channel state information (CSI). The author presented the comparison result of the proposed solution and wearable sensor (ground truth). After obtaining the proposed result, it was fed into machine learning algorithms and KNN was found to be the best model with the highest accuracy of 91%. Ashleibta et al. (2020) proposed human activity monitoring, namely, exercise, picking up an object, sitting down, standing up, and other activities as shown in Figure 15. In this experiment, using two universal software radio peripheral (USRP) models that function as SDR-based transceivers, the fluctuation of channel state information amplitude was retrieved from a continuous stream of various frequency sub-carriers. The data were trained, tested, and validated using time-domain CSI data statistical features and 89.73% accuracy was found from the K-nearest machine learning algorithm. Rehman et al. (2021) used COVID and non-COVID scenarios, in which real-time data were collected of abnormal breathing patterns during the pandemic using a non-contact SDR platform and applied an ML model for classifying the collected data with more than 90% accuracy.
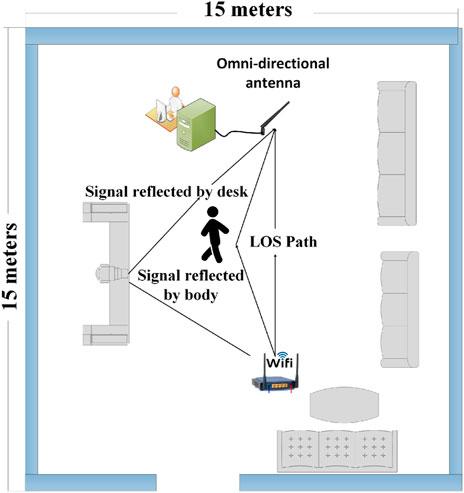
FIGURE 13. Schematic of FOG activity monitoring using Wi-Fi signals (Tahir et al., 2019).
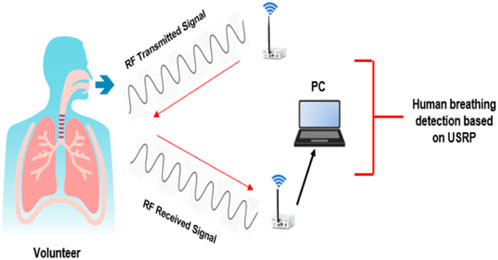
FIGURE 14. Schematic of non-contact breathing detection using Wi-Fi signals (Ashleibta et al., 2021a).
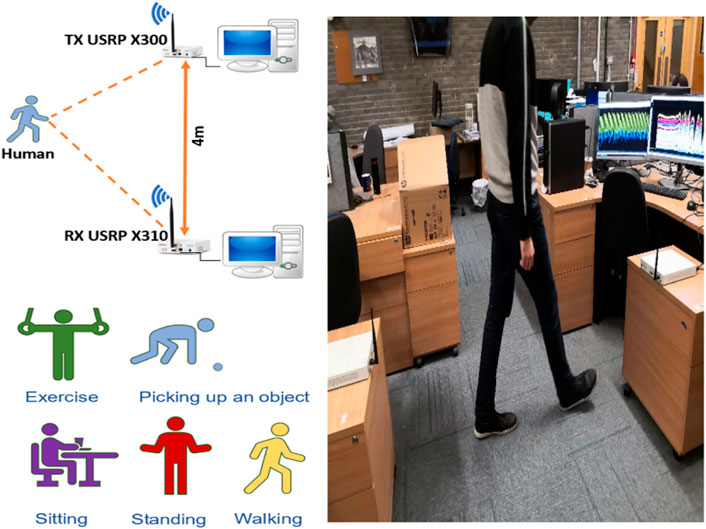
FIGURE 15. Experiment setup of activity monitoring using Wi-Fi signals (Ashleibta et al., 2020).
Taylor et al. (2020) proposed human motion detection. The data were in the form of channel state information and each activity was performed between the antennas using USRPs. The binary classification was performed on sitting and standing activities. The performance of machine learning is illustrated by the fact that the random forest algorithm provides an accuracy of 92.47%. A comparison was made between the outcome that was proposed and the benchmark dataset, in which the activity was monitored by using wearable devices. For a 6 GHz application, a 1-bit subwavelength metasurface unit cell is presented (Kazim et al., 2020). There are three layers to it: the bottom layer is used for the placement of PIN diodes and for DC biassing, the middle layer serves as a ground plane, and the top layer is used for wave reflection as shown in Figure 16. The reflection coefficient is larger than 90%, and a phase difference of 180 ± 20 was attained over a 200 MHz bandwidth.
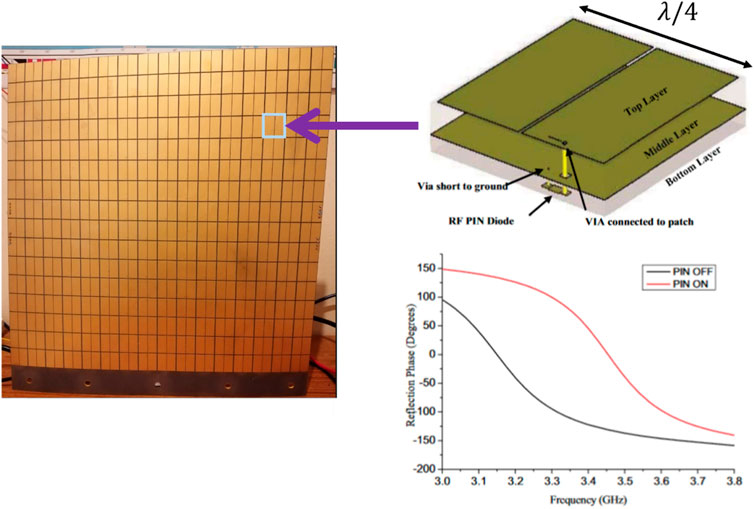
FIGURE 16. Overall proposed view of the unit cell with the diode (Kazim et al., 2020).
A wearable, compact, dual-polarized, multiple-input-multiple-output (MIMO) antenna is proposed by Mahmood et al. (2021). It has a bandwidth of 4.8–30 GHz with a maximum radiation efficiency and directional gain of 96% and 5.72 dBi, respectively. The antenna’s performance was assessed by near-field microwave imaging for both on-body (breast) and free space conditions. They achieved high fidelity, low SAR, and exact tumor localization results. Climate changes become a challenge due to negative energy usage behavior in recent years in the United Kingdom. Taha et al. (2021)monitored electricity consumption vs. occupancy during minimal consumption periods (i.e., bank weekends, holidays, and outside working hours). Usman et al. (2022) used intelligent wireless walls (IWW) to monitor activities in challenging locations where traditional microwave detection is ineffective. There were two complex environments as shown in Figure 17: the first is a corridor junction situation in which the transmitter and receiver are located in separate corridor sections, while the second is a multi-floor scenario in which the transmitter and receiver are located on two different floors of the building. Multiple subjects were involved in activity monitoring and achieved 100% classification accuracy.
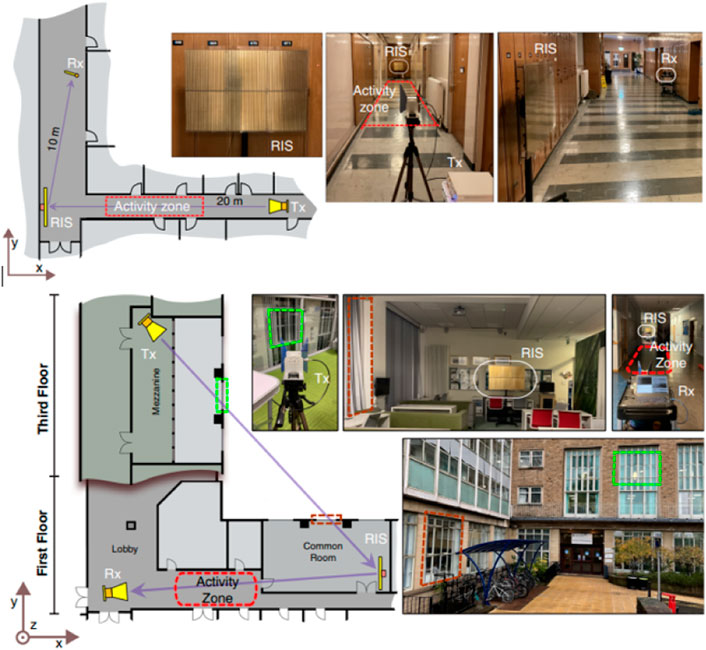
FIGURE 17. Two complex environments using intelligent wireless walls (IWW) (Usman et al., 2022).
Research especially carried out to assist patients in interacting with their surroundings using an electromagnetic brain-computer-metasurface, which is regulated by human brain signals directly, is proposed by Ma et al. (2022). The proposed EBCM platform converts P300-based EEG evoked potentials to electromagnetic digital coding information non-invasively. Two EBCM operators send text wirelessly. Using the same EBCM platform, several proof-of-concept mind control schemes are presented, including visual-beam scanning, wave modulations, and pattern encoding. Saeed et al. (2021) used 8 GHz frequency-modulated continuous-wave (FMCW) RADAR to observed 99 individuals’ daily activities, including walking, bending, sitting, drinking, and standing, which is shown in Figure 18. Spectrograms were generated after post-processing the data. The spectrogram was fed into the residual neural network and a classification accuracy of more than 90% was obtained. Taylor et al. (2021) used micro-doppler spectrograms and recorded various human activities such as standing, fall events, walking, picking up objects, drinking water, and sitting as shown in Figure 19. The convolution neural network algorithm was applied to the dataset with PCA and achieved 95.30% classification accuracy. Saeed et al. (2022)recorded human activity monitoring which includes bending, sitting, drinking, walking, and standing. Data were recorded in the form of micro-doppler signatures and pre-processing was carried out using the MATLAB tool. The spectrogram was divided into the train, validate, and test datasets. Using the residual neural network (ResNet) model it achieved 91% accuracy.
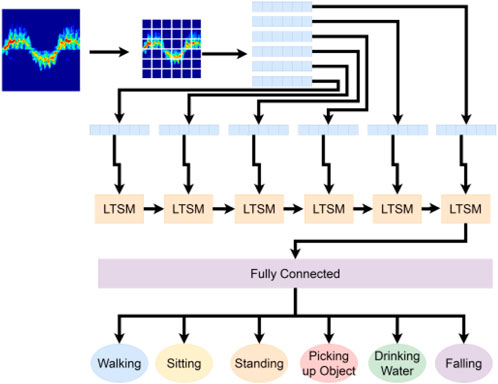
FIGURE 18. Overall framework for multiple activity monitoring using the FMCW radar (Saeed et al., 2021).
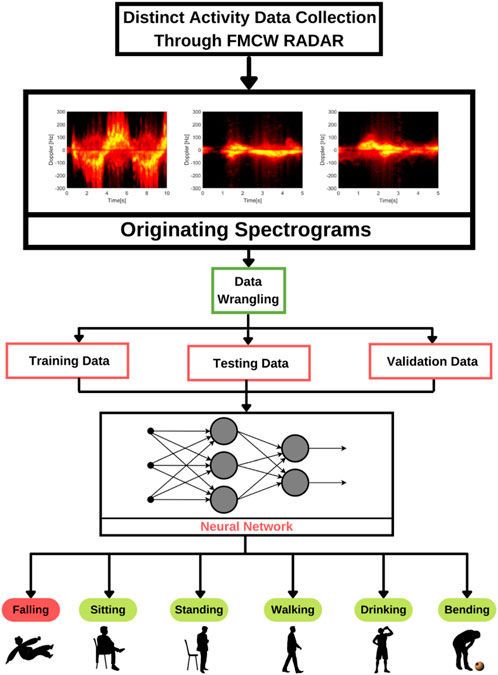
FIGURE 19. Overall methodology for activity monitoring using the FMCW radar (Taylor et al., 2021).
An ultra-wideband (UWB) radar sensor was used to detect gestures of smoking, vaping, or nothing, taken in an oil field or gas station. After pre-processing data in the form of spectrograms, the data were divided into the form of train and test and fed into deep learning algorithms VGG16, VGG19, and InceptionV3 and concluded that InceptionV3 have good classification accuracy of 90% (Hameed et al., 2022a). A UWB radar-based contactless privacy-preserving British sign language recognition system was designed to detect the six most common signs, namely, depressed, confused, hate, happy, sad, and lonely. The spectrogram divided into test and train was fed into a pre-trained deep learning algorithm. The results show that VGG16 outperformed VGG19 and InceptionV3 with around 93.33% accuracy (Hameed et al., 2022b).
4 Discussion and present/future challenges
With the rise of 5G breakthroughs across all industrial sectors, the IoT and artificial intelligence are reinforcing its adaptation in the everyday life applications. The International Data Corporation forecasts show that by 2025, there will be 42 billion devices linked to the Internet which is approximately 80 zettabytes of data. All of the applications covered in this study that use sensing technologies have demonstrated appropriate accuracy of the classification; however, the trials are only carried out in a controlled setup. The results demonstrate that the distance between the subject and the devices in use, many occupants within the region of interest, and behind-the-wall detection of movement all have a substantial impact on the overall accuracy of the system. In this context, sensing technology, in conjunction with other signal processing techniques, should be designed to deliver dependable and resilient performance in real-world circumstances while maintaining as much precision as practicable. There will be no way for the general public and healthcare systems throughout the world to trust and rely on these technologies unless they are properly evaluated. Imran et al. (2020b)discussed a few key obstacles in the IoT and sensing technologies along some major key concerns that still need to be addressed. Security and privacy, routing strategies, protocols and scalability still remain the most challenging aspects for contactless sensing systems. Identity attacks in back-scatter communication are another major concern. A physical layer authentication via a residual channel at the reader end could possibly be one of the solutions (Mehmood et al., 2020), but there is still room to improve the accuracy of such schemes when deployed in densely populated tag areas. Just like the RF sensing and IoT devices, RFID communication also poses several technical challenges and its use in daily life for assistive living. There is a great possibility of RFID signal interference with other electromagnetic indoor signals, which may significantly affect the performance of the whole system and may cause consequences if used in medical assistance applications without considering these open threats in RFID systems. In addition to these environmental conditions, state policies and organizational setup are still a challenge in such deployments. Also, these technologies are quite sensitive to the delays required to process data, which calls for new investigations around the trade-off between learning cost and performance. Keeping these limitations in consideration, the CSI group has come up with possible solutions for a contactless intelligent sensing domain. The group has so far worked in diverse areas including non-invasive healthcare where single-subject activity and vital sign monitoring is extensively researched and a roadmap for the projects are shown in Figure 20. These achievements include an intelligent non-invasive real-time human activity recognition system for next-generation healthcare, a flexible and scalable testbed for large-scale body movement, and RF sensing for detecting breathing abnormalities using software-defined radios. Considering the after-COVID situation where wearing face masks has become a norm, the strength of RF sensing has been utilized to its limits for cognitive impaired persons, who mostly rely on sign language and lip reading. Lip reading under masks has been extensively researched using UWB radar and Wi-Fi signals, as these waves can easily penetrate the mask and are able to read lips with appropriate machine learning and deep learning algorithms. Introducing wireless on walls with intelligent reflective surfaces being used for activity monitoring is another innovative work with great results and accuracy. Technologies such as IRS, radar, SDRs, Wi-Fi, lidar, RFID, and LoRa can be further utilized for localization of multi-subject activity and vital sign monitoring. The ongoing and future innovative research areas identified include body glucose level monitoring using non-invasive methods, lip reading under masks using UWB radar and Wi-Fi signals, IW-based sensing, and multi-subject activity and vitals’ monitoring using radio frequency.
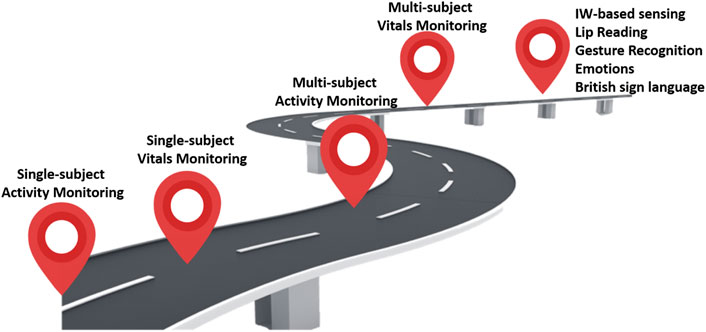
FIGURE 20. CSI progress road-map and identified future research projects in a contactless sensing domain.
We can conclude future directions and potential research areas as follows:
• Pushing limits of RF sensing to detect minor movements and read lips “under masks.”
• The intelligent reflective surfaces can be optimized and tested for RFID-based applications where non-line-of-sight communication is still a problem.
• The IRS, radar, SDR, RFID, and LoRa technologies need further investigation for solutions toward multi-subject activity and vitals monitoring.
• Glucose level monitoring using non-invasive techniques is still trending and demands further research.
• RFID in the context of the IoT has great potential in the field of healthcare for non-invasive applications.
5 Conclusion
This study provides a comprehensive overview of the University of Glasgow’s research and deployment activities pertaining to RFID systems, IoT for everyday applications, and RF sensing and development. The primary research initiatives in collaborations with industrial partners and institutional research centers are also presented. Furthermore, a detailed analysis of present and prospective research areas is also discussed. Through this study, it is established that contactless sensing has a promising potential to facilitate independent assisted living. Activity and vitals, such as respiration and heartbeat, for single subjects can be monitored with high accuracy by contactless sensing. However, due to certain limitations, extensive research is required for multi-subject detection and tracking in coordination with RFID. This study also highlighted substantial contributions achieved by CSI toward the 5G and 6G industries and has proposed novel works for these progressing sectors. The article also gives an insight into RF sensing and discovered that it could be considerably improved by applying machine learning (ML) methods to stronger represent data for new signals from the 5G spectrum. The study concluded that the proposed technique would not only be beneficial for personal IoT applications such as indoor localization, activity recognition, and healthcare but also for other applications in smart city management, etc.
Author contributions
All authors listed have made a substantial, direct, and intellectual contribution to the work and approved it for publication.
Funding
This work was supported by the Engineering and Physical Sciences Research Council, grant number EP/T021020/1.
Conflict of interest
The authors declare that the research was conducted in the absence of any commercial or financial relationships that could be construed as a potential conflict of interest.
Publisher’s note
All claims expressed in this article are solely those of the authors and do not necessarily represent those of their affiliated organizations, or those of the publisher, the editors, and the reviewers. Any product that may be evaluated in this article, or claim that may be made by its manufacturer, is not guaranteed or endorsed by the publisher.
Footnotes
1Communication, Sensing and Imaging (CSI) Lab, Electronic and Electrical Engineering, University of Glasgow, United Kingdom
References
Alkhayyat, A., Thabit, A. A., Al-Mayali, F. A., and Abbasi, Q. H. (2019). Wbsn in iot health-based application: Toward delay and energy consumption minimization. J. Sensors 2019, 1–14. doi:10.1155/2019/2508452
Althobaiti, T., Sharif, A., Ouyang, J., Ramzan, N., and Abbasi, Q. H. (2020). Planar pyramid shaped uhf rfid tag antenna with polarisation diversity for iot applications using characteristics mode analysis. IEEE Access 8, 103684–103696. doi:10.1109/access.2020.2999256
Ashleibta, A. M., Abbasi, Q. H., Shah, S. A., Khalid, M. A., AbuAli, N. A., and Imran, M. A. (2021a). Non-invasive rf sensing for detecting breathing abnormalities using software defined radios. IEEE Sens. J. 21, 5111–5118. doi:10.1109/JSEN.2020.3035960
Ashleibta, A. M., Taha, A., Khan, M. A., Taylor, W., Tahir, A., Zoha, A., et al. (2021b). 5g-enabled contactless multi-user presence and activity detection for independent assisted living. Sci. Rep. 11, 17590–17615. doi:10.1038/s41598-021-96689-7
Ashleibta, A. M., Zahid, A., Shah, S. A., Abbasi, Q. H., and Imran, M. A. (2020). Flexible and scalable software defined radio based testbed for large scale body movement. Electronics 9, 1354. doi:10.3390/electronics9091354
Cui, T. J., Zoha, A., Li, L., Shah, S. A., Alomainy, A., Imran, M. A., et al. (2020). Wireless on walls: Revolutionizing the future of health care. IEEE Antennas Propag. Mag. 63, 87–93. doi:10.1109/map.2020.3036063
Dangana, M., Ansari, S., Abbasi, Q. H., Hussain, S., and Imran, M. A. (2021). Suitability of nb-iot for indoor industrial environment: A survey and insights. Sensors 21, 5284. doi:10.3390/s21165284
Ge, Y., Ansari, S., Abdulghani, A., Imran, M. A., and Abbasi, Q. H. (2020). “Intelligent instruction-based iot framework for smart home applications using speech recognition,” in 2020 IEEE International Conference on Smart Internet of Things (SmartIoT), China, 19-21 Aug, 2022, 197.
GS1 (2022). Overview of UHF frequency allocations (860 to 960 MHz) for RAIN RFID, 2022 [Online]. Available: https://www.gs1.org/docs/epc/uhf_regulations.pdf.
Hameed, H., Azam, N., Usman, M., Abbas, H., Imran, M. A., and Abbasi, Q. H. (2022a). Rf sensing for smoking detection at oil fields.
Hameed, H., Usman, M., Khan, M. Z., Hussain, A., Abbas, H. T., Imran, M. A., et al. (2022b). Privacy-preserving British sign language recognition using deep learning. TechRxiv.
Hu, X., Abdulghani, A. M., Imran, M., and Abbasi, Q. H. (2020). “Internet of things (iot) for healthcare application: Wearable sleep body position monitoring system using iot platform,” in Proceedings of the 2020 International Conference on Computing, Networks and Internet of Things, Sanya China, April 24 - 26, 2020, 76–81.
Imran, M. A., Ghannam, R., and Abbasi, Q. H. (2020a). Engineering and technology for healthcare. United States: John Wiley & Sons.
Imran, M. A., Zoha, A., Zhang, L., and Abbasi, Q. H. (2020b). Grand challenges in iot and sensor networks. Sec. IoT Sens. Netw 1. doi:10.3389/frcmn.2020.619452
Kazim, J. u. R., Ur-Rehman, M., Al-Hasan, M., Mabrouk, I. B., Imran, M. A., and Abbasi, Q. H. (2020). “Design of 1-bit digital subwavelength metasurface element for sub-6 ghz applications,” in 2020 International Conference on UK-China Emerging Technologies (UCET), China, 20-21 Aug. 2020, 1. doi:10.1109/UCET51115.2020.9205382
Khan, M. Z., Taha, A., Taylor, W., Imran, M. A., and Abbasi, Q. H. (2022). Non-invasive localization using software-defined radios. IEEE Sens. J. 22, 9018–9026. doi:10.1109/JSEN.2022.3160796
Ma, Q., Gao, W., Xiao, Q., Ding, L., Gao, T., Zhou, Y., et al. (2022). Directly wireless communication of human minds via non-invasive brain-computer-metasurface platform. arXiv preprint arXiv:2205.00280.
Mahmood, S. N., Ishak, A. J., Jalal, A., Saeidi, T., Shafie, S., Soh, A. C., et al. (2021). A bra monitoring system using a miniaturized wearable ultra-wideband mimo antenna for breast cancer imaging. Electronics 10, 2563. doi:10.3390/electronics10212563
Mehmood, A., Aman, W., Rahman, M. M. U., Imran, M. A., and Abbasi, Q. H. (2020). “Preventing identity attacks in rfid backscatter communication systems: A physical-layer approach,” in 2020 International Conference on UK-China Emerging Technologies (UCET), China, 20-21 Aug. 2020, 1.
Nepa, P., Buffi, A., Michel, A., Motroni, A., Bernardini, F., Singh, R., et al. (2021). “Past, present and future rfid activities at the University of pisa,” in 2021 IEEE International Conference on RFID Technology and Applications (RFID-TA), Delhi, India, 6-8 Oct. 2021, 252–255.
Ouyang, J., Sharif, A., Yan, Y., and Abbasi, Q. H. (2019). “Small conformal uhf rfid tag antenna for labelling fruits,” in 2019 IEEE Asia-Pacific Microwave Conference (APMC), Singapore, December 10 to December 13, 2019, 33.
Rehman, M., Shah, R. A., Khan, M. B., Shah, S. A., AbuAli, N. A., Yang, X., et al. (2021). Improving machine learning classification accuracy for breathing abnormalities by enhancing dataset. Sensors 21, 6750. doi:10.3390/s21206750
Saeed, U., Alqahtani, F., Baothman, F., Shah, S. Y., Shah, S. I., Badshah, S. S., et al. (2022). “Monitoring discrete activities of daily living of young and older adults using 5.8 ghz frequency modulated continuous wave radar and resnet algorithm,” in Body area networks. Smart IoT and big data for intelligent health management. Editors M. Ur Rehman, and A. Zoha (Cham: Springer International Publishing), 28–38.
Saeed, U., Shah, S. Y., Shah, S. A., Ahmad, J., Alotaibi, A. A., Althobaiti, T., et al. (2021). Discrete human activity recognition and fall detection by combining fmcw radar data of heterogeneous environments for independent assistive living. Electronics 10, 2237. doi:10.3390/electronics10182237
Sharif, A., Abbasi, Q. H., Arshad, K., Ansari, S., Ali, M. Z., Kaur, J., et al. (2021a). Machine learning enabled food contamination detection using rfid and internet of things system. J. Sens. Actuator Netw. 10, 63. doi:10.3390/jsan10040063
Sharif, A., Imran, M. A., Ouyang, J., Abbasi, Q. H., and Yan, Y. (2019a). “Circular polarized rfid tag antenna design using characteristic mode analysis,” in 2019 international workshop on antenna technology (iWAT) (Manhattan, New York, U.S.: IEEE), 62–64.
Sharif, A., Kumar, R., Ouyang, J., Abbas, H. T., Alomainy, A., Arshad, K., et al. (2021b). Making assembly line in supply chain robust and secure using uhf rfid. Sci. Rep. 11, 18041–18117. doi:10.1038/s41598-021-97598-5
Sharif, A., Ouyang, J., Arshad, K., Imran, M. A., and Abbasi, Q. H. (2020). “Spider web shaped near-field uhf rfid reader antenna for healthcare and iot applications,” in 2020 IEEE International Symposium on Antennas and Propagation and North, Montreal, QC, Canada, 5-10 July 2020, 1535–1536.
Sharif, A., Ouyang, J., Chattha, H. T., Imran, M. A., and Abbasi, Q. H. (2019b). “Wearable uhf rfid tag antenna design using hilbert fractal structure,” in 2019 UK/China Emerging Technologies (UCET), China, 21-22 August 2019, 1.
Sharif, A., Ouyang, J., Chattha, H. T., Long, R., Raza, A., and Abbasi, Q. H. (2018). “Uhf rfid tag antenna design for challenging environment surfaces,” in 12th European Conference on Antennas and Propagation (EuCAP 2018), London, 09-13 April 2018.
Sharif, A., Ouyang, J., Imran, M. A., and Abbasi, Q. H. (2019c). “Orientation insensitive uhf rfid tag antenna with polarization diversity using characteristic mode analysis,” in 2019 IEEE International Symposium on Antennas and Propagation and USNC-URSI Radio Science Meeting, Atlanta, Georgia, USA - 7-12 July 2019, 651.
Sharif, A., Ouyang, J., Raza, A., Imran, M. A., and Abbasi, Q. H. (2019d). Inkjet-printed uhf rfid tag based system for salinity and sugar detection. Microw. Opt. Technol. Lett. 61, 2161–2168. doi:10.1002/mop.31863
Sharif, A., Ouyang, J., Yan, Y., Raza, A., Imran, M. A., and Abbasi, Q. H. (2019e). Low-cost inkjet-printed rfid tag antenna design for remote healthcare applications. IEEE J. Electromagn. RF Microw. Med. Biol. 3, 261–268. doi:10.1109/jerm.2019.2924823
Sharif, A., Ouyang, J., Yang, F., Chattha, H. T., Imran, M. A., Alomainy, A., et al. (2019f). Low-cost inkjet-printed uhf rfid tag-based system for internet of things applications using characteristic modes. IEEE Internet Things J. 6, 3962–3975. doi:10.1109/jiot.2019.2893677
Sharif, A., Yan, Y., Ouyang, J., Chattha, H. T., Arshad, K., Assaleh, K., et al. (2021c). Uniform magnetic field characteristics based uhf rfid tag for internet of things applications. Electronics 10, 1603. doi:10.3390/electronics10131603
Sodhro, A. H., Gurtov, A., Zahid, N., Pirbhulal, S., Wang, L., Rahman, M. M. U., et al. (2020). Toward convergence of ai and iot for energy-efficient communication in smart homes. IEEE Internet Things J. 8, 9664–9671. doi:10.1109/jiot.2020.3023667
Sodhro, A. H., Obaidat, M. S., Abbasi, Q. H., Pace, P., Pirbhulal, S., Fortino, G., et al. (2019). Quality of service optimization in an iot-driven intelligent transportation system. IEEE Wirel. Commun. 26, 10–17. doi:10.1109/mwc.001.1900085
Song, Y., Taylor, W., Ge, Y., Dashtipour, K., Imran, M. A., and Abbasi, Q. H. (2021). “Design and implementation of a contactless ai-enabled human motion detection system for next-generation healthcare,” in 2021 IEEE International Conference on Smart Internet of Things, Jeju, Korea, Republic of Korea, 13-15 Aug. 2021, 112–119.
Sun, J., Abdulghani, A. M., Imran, M. A., and Abbasi, Q. H. (2020). “Iot enabled smart fertilization and irrigation aid for agricultural purposes,” in Proceedings of the 2020 International Conference on Computing, Networks and Internet of Things, New York United States, April 24 - 26, 2020, 71–75.
Taha, A., Hopthrow, T., Wu, R., Adams, N., Brown, J., Zoha, A., et al. (2021). Identifying the lack of energy-conscious behaviour in clinical and non-clinical settings: An nhs case study. Electronics 10, 2468. doi:10.3390/electronics10202468
Tahir, A., Ahmad, J., Shah, S. A., Morison, G., Skelton, D. A., Larijani, H., et al. (2019). Wifreeze: Multiresolution scalograms for freezing of gait detection in Parkinson’s leveraging 5g spectrum with deep learning. Electronics 8, 1433. doi:10.3390/electronics8121433
Tahir, A., Taylor, W., Taha, A., Usman, M., Shah, S. A., Imran, M. A., et al. (2022). “Iot based fall detection system for elderly healthcare,” in Internet of things for human-centered design (Cham: Springer), 209–232.
Taylor, W., Dashtipour, K., Shah, S. A., Hussain, A., Abbasi, Q. H., and Imran, M. A. (2021). Radar sensing for activity classification in elderly people exploiting micro-Doppler signatures using machine learning. Sensors 21, 3881. doi:10.3390/s21113881
Taylor, W., Shah, S. A., Dashtipour, K., Zahid, A., Abbasi, Q. H., and Imran, M. A. (2020). An intelligent non-invasive real-time human activity recognition system for next-generation healthcare. Sensors 20, 2653. doi:10.3390/s20092653
Ur-Rehman, M., Kalsoom, T., Malik, N. A., Safdar, G. A., Chatha, H. T., Ramzan, N., et al. (2018). “A wearable antenna for mmwave iot applications,” in IEEE International Symposium on Antennas and Propagation & USNC/URSI National Radio Science Meeting, Singapore Asia, 04-10 December 2021, 1211.
Usman, M., Rains, J., Cui, T. J., Khan, M. Z., Imran, M. A., Abbasi, Q. H., et al. (2022). Intelligent wireless walls for contactless in-home monitoring. Light. Sci. Appl. 11, 212–213. doi:10.1038/s41377-022-00906-5
Wu, S., Abdulghani, A. M., Ansari, S., Imran, M. A., and Abbasi, Q. H. (2020). “Iot enabled smart lighting system using stm32 microcontroller with high performance arm® cortex®-m3 core,” in 2020 International Conference on UK-China Emerging Technologies (UCET) (IEEE), China, 20-21 Aug. 2020, 1.
Xu, Z., Ansari, S., Abdulghani, A. M., Imran, M. A., and Abbasi, Q. H. (2020). “Iot enabled smart security framework for 3d printed smart home,” in 2020 IEEE International Conference on Smart Internet of Things (SmartIoT) (IEEE), Beijing, China, 14 2020 to Aug. 16 2020, 117–123.
Zhang, W., Abdulghani, A. M., Imran, M. A., and Abbasi, Q. H. (2020). “Internet of things (iot) enabled smart home safety barrier system,” in Proceedings of the 2020 International Conference on Computing, Networks and Internet of Things, Sanya China, April 24 - 26, 2020, 82–88.
Keywords: RF sensing, IoT, RFID, radar, SDR, Wi-Fi, healthcare, antenna design
Citation: Lubna L, Hameed H, Ansari S, Zahid A, Sharif A, Abbas HT, Alqahtani F, Mufti N, Ullah S, Imran MA and Abbasi QH (2022) Radio frequency sensing and its innovative applications in diverse sectors: A comprehensive study. Front. Comms. Net 3:1010228. doi: 10.3389/frcmn.2022.1010228
Received: 02 August 2022; Accepted: 23 August 2022;
Published: 21 September 2022.
Edited by:
Akram Alomainy, Queen Mary University of London, United KingdomReviewed by:
Syed Aziz Shah, Coventry University, United KingdomVictor Hugo C. de Albuquerque, University of Fortaleza, Brazil
Copyright © 2022 Lubna, Hameed, Ansari, Zahid, Sharif, Abbas, Alqahtani, Mufti, Ullah, Imran and Abbasi. This is an open-access article distributed under the terms of the Creative Commons Attribution License (CC BY). The use, distribution or reproduction in other forums is permitted, provided the original author(s) and the copyright owner(s) are credited and that the original publication in this journal is cited, in accordance with accepted academic practice. No use, distribution or reproduction is permitted which does not comply with these terms.
*Correspondence: Qammer Hussain Abbasi, qammer.abbasi@glasgow.ac.uk
†ORCID: Fehaid Alqahtani, orcid.org/0000-0001-9564-6653