AgRIS: wind-adaptive wideband reconfigurable intelligent surfaces for resilient wireless agricultural networks at millimeter-wave spectrum
- School of Computing, University of Nebraska–Lincoln, Lincoln, NE, United States
Wireless networks in agricultural environments are unique in many ways. Recent measurements reveal that the dynamics of crop growth impact wireless propagation channels with a long-term seasonal pattern. Additionally, short-term environmental factors, such as strong wind, result in variations in channel statistics. Next-generation agricultural fields, populated by autonomous tractors, drones, and high-throughput sensing systems, require high-throughput connectivity infrastructure, resulting in the future deployment of high-frequency networks, where they have not been deployed before. More specifically, when millimeter-wave (mmWave) communication systems, a viable candidate for 5G and 6G high-throughput solutions, are deployed for higher throughput, these issues become more prominent due to the relatively small wavelength at this frequency band. To improve coverage in the mmWave spectrum in agricultural settings, reconfigurable intelligent surfaces (RISs) are a promising solution with low energy consumption and high cost efficiency when compared to half-duplex active relays with multiple antennas. To ensure link resiliency under dynamic channel behavior, an adaptive RIS for broadband wireless agricultural networks (AgRIS) at mmWave band is designed in this work. AgRIS relies on output from a time-series model that forecasts the short-term wind speed based on measured wind data, which is readily available in most farms. The temporal correlation between link reliability and wind speed is demonstrated through extensive field experiments. Our simulation results demonstrate that AgRIS with a small footprint of 11 × 11 elements can help mitigate the adversarial effects of wind-induced signal level drop by up to 8 dB and provides high energy efficiency of 1 Gbits/joule.
1 Introduction
Recent advances in wireless communication technologies have enabled many new services and applications, such as small cells with multiple-input–multiple-output (MIMO) communications operating at millimeter-wave (mmWave) frequencies. On one hand, these recent solutions are targeted to achieve high spectral efficiency in densely populated areas, including urban and indoor scenarios. On the other hand, agricultural operations in rural fields are undergoing a major transformation with the inclusion of autonomous tractors (John Deere, 2022), fleets of field robots (Atefi et al., 2021), drones, and high-throughput sensing systems for precision agriculture solutions. However, as more data are being generated and processed in agricultural wireless networks under the scope of precision agriculture or agricultural Internet of Things (Ag-IoT) (Vuran et al., 2018); (Chamara et al., 2022), a digital divide is created due to the lack of advanced communication infrastructure for agricultural fields. Such a communication bottleneck hinders the timely transmission of in-field data to edge or cloud computing centers and hampers the decision-making process in precision farming (Liu et al., 2022). A promising solution bridging this digital divide is mmWave communications with a wide bandwidth. In particular, a contiguous bandwidth of 7 GHz at the unlicensed 60 GHz band provides abundant spectral resources to high throughput and low latency (Federal Communications Commission, 2010).
To maximize the efficacy of mmWave wireless systems in agricultural settings, novel system hardware and software designs are needed based on the distinct features of wireless agricultural environments. In particular, the agricultural channels embody the dynamics of crop growth. They are subject to weather impacts, shown in our previous work, to cause noticeable channel attenuation due to partial line-of-sight (LoS) obstruction and scattering effect from crop canopies (Nie et al., 2022; Vuran et al., 2022). Therefore, new solutions are needed to maintain link stability and enable network resiliency.
Current solutions using active electronic components (e.g., power amplifiers and analog-to-digital converters) in hybrid beamforming are unsuitable in agricultural wireless networks because of their high energy consumption and cost (Wei et al., 2015). To achieve a balance in energy and spectral efficiency, an emerging solution of reconfigurable intelligent surfaces (RISs) is promising for improving link reliability in agricultural wireless networks. The RISs are based on two-dimensional metamaterials, namely, metasurfaces, to achieve controlled reflection and other engineered functionalities of electromagnetic waves (Liaskos et al., 2018; Fara et al., 2022). While most efforts on RIS design focus on static environments, an adaptive method will be fundamental to achieving reliable link performance in dynamic wireless channels.
In this work, we leverage the results of extensive mmWave communication experiments in agricultural fields (Nie et al., 2022; Vuran et al., 2022) to design and analyze a novel adaptive RIS for wireless agriculture networks (AgRIS) (shown in Figure 1). AgRIS accommodates the channel dynamics and provides wireless link resiliency for future precision agriculture applications. The contributions of our work are summarized as follows:
• We analyze the temporal correlation between gust speed and instantaneous signal-to-noise ratio (SNR) based on data collected from 5-month-long field experiments. The results demonstrate that sustained gust1 leads to a high fluctuation of the SNR of up to 7 dB in a 1-min interval, as well as continuous volatility of the average SNR across a wind duration of 8 min. In addition, we quantify the SNR variation using an exponentially modified Gaussian distribution, which is the first model that quantifies the wind effect on link reliability.
• We develop a short-term (i.e., several minutes ahead) wind speed prediction scheme based on the non-linear autoregressive exogenous model with measured wind speed and air pressure data. We show that this prediction scheme outperforms existing methods based on support vector machines.
• Recognizing the unique channel dynamics, we present a novel concentric square-shaped subarray design for AgRIS that is adaptive to different wind conditions.
• We analyze an AgRIS-aided point-to-point agricultural link that will be promising for future smart agriculture and compare the SNR improvement assisted by AgRIS with the baseline case without AgRIS. We also compare the energy efficiency between the proposed AgRIS and traditional RIS with a fixed dimension.
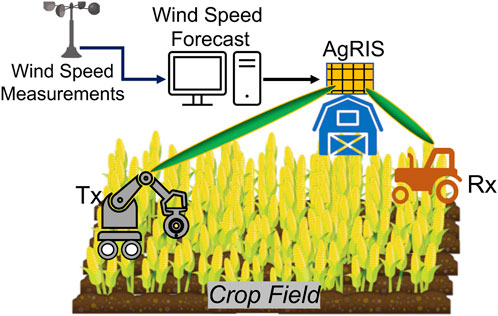
FIGURE 1. Illustration of agricultural wireless links with AgRIS. The wind-speed collection device is connected to a computer to predict the short-term wind speed, which will be fed to the controller of AgRIS. AgRIS can be mounted on farm infrastructure, such as a farmhouse. The data transmitted from a field robot (shown as “Tx”) are reflected from AgRIS toward a tractor (denoted as “Rx”).
The rest of this paper is organized as follows. The related work is presented in Section 2. A description of the agricultural field experiments and observation of channel dynamics is given in Section 3. The system model of the AgRIS is elaborated in Section 4. The short-term wind speed prediction model based on measurement data is described in Section 5. The performance analysis of the AgRIS is presented in Section 6. Finally, this paper is concluded in Section 7.
2 Related work
When electromagnetic waves propagate through the plant canopy, depolarization may occur. A previous study is focused on depolarization and attenuation when signals at 40 GHz penetrate through a single plant canopy (Alejos et al., 2007). In an anechoic chamber, two types of individual plants are analyzed for both slow and fast fading at different wind speeds. The experiment results show that different plant species scatter signals differently. However, the results based on a single plant may not apply to agricultural fields with row crops. In Vuran et al. (2022) and Nie et al. (2022), we present the results of the first mmWave experiments performed in agricultural fields. Based on experiments performed in three farm fields over 5 months during the growing season of two types of crops (corn and soybean), we show that during the growing season, the crop canopy surface acts as a “new ground,” creating multipath components and resulting in higher path loss. It is also shown that an increase of 4 m/s in gust speed results in a half-power decrease (3-dB SNR degradation) due to increased scattering, which motivates this work.
Most prior research effort concerning agricultural wireless networks are dedicated to wireless sensor networks at sub-GHz frequencies (Dong et al., 2013). The comprehensive data monitored in crop fields range from environmental factors, including soil nutrient levels and pH levels, to plant phenotyping that consists of high-resolution image data (Chamara et al., 2022). The long-range wide area network (LoRaWAN) has recently been utilized in the Ag-IoT for low-power applications (Vuran et al., 2018). However, when emerging needs arise for broader bandwidths to transmit high-speed data, the LoRaWAN is no longer sufficient. Other solutions, including satellite communications and optic fiber connectivity, are costly for stakeholders (e.g., farmers) in the agricultural industry. Therefore, the mmWave spectrum that offers a wider bandwidth is being studied in the rural scenario. A rural macrocellular propagation channel model at 73-GHz E-band is developed with the focus of coverage analysis (MacCartney and Rappaport, 2017). Based on field measurements, this channel model demonstrates an achievable range of
Among recent progress in the area of RIS design, only limited studies have focused on adaptive schemes, which include adaptive transmission (Lin et al., 2020), adaptive RIS pattern coding scheme to achieve a single-radio frequency MIMO for multiplexing (Li Q. et al., 2021; Karasik et al., 2021), and adaptive path deployment (Liaskos et al., 2020). Overall, existing adaptive schemes mainly depend on feedback from the wireless channel and algorithms in artificial intelligence (Wang et al., 2021). To the best of our knowledge, this is the first work focusing on adaptive RIS design based on environmental factors in general, and wind specifically. In addition to the RIS, but related to agricultural applications, adaptive antenna arrays have been investigated, and an active beamforming algorithm is designed for underground communication (Salam and Vuran, 2017).
3 Field experiments and observations
The AgRIS design is motivated by observations of data collected from extensive field experiments at the Field Phenotyping Facility in the Eastern Nebraska Research, Extension, and Education Center (ENREEC) (Figure 2) near Mead, Nebraska (Vuran et al., 2022)2, where a weather station is located. Since the focus of this work is the impact of wind on wireless link reliability and the measure to mitigate this impact, we highlight the related observations and experiments to provide readers with an overview of the relevant measurement scenarios and associated data. The comprehensive details of the experiments and corresponding channel models can be found in Vuran et al. (2022)and Nie et al. (2022).
3.1 Measurement setup
During the field experiments, we utilized a pair of TerraGraph (TG) channel sounders (Shkel et al., 2021). Operating at 60.48 GHz with a wide bandwidth of 2.16 GHz, the TG channel sounder can continuously listen to the channel, record channel impulse response, and compute the instantaneous SNR. More detailed parameters of the channel sounder are listed in Table 1.
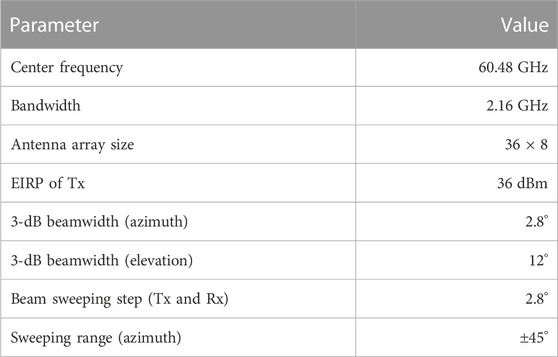
TABLE 1. Channel sounder parameters (Shkel et al., 2021).
3.2 Field experiments
The weather station at the ENREEC facility continuously collects data at a 1-min interval with a set of environmental statistics, including temperature, relative humidity, 1-min average wind speed, and 5-s gust speed. Regarding wind speed, the Beaufort wind force scale, as shown in Table 2, is an empirical model commonly used to classify the strength of wind (Fry, 1967). We focus on two experiment days during July 2021 when the crop was at its peak growing season. More specifically, measurements conducted on 7 July were performed under a gentle breeze (level 3 on the Beaufort scale), while measurements conducted on 16 July were under light air to light breeze (levels 1 and 2 on the Beaufort scale). In particular, the measured 5-s gust speed represents instant wind conditions, which is more indicative than the 1-min average wind speed in analyzing channel fluctuation. Based on the computed real-time SNR values, we obtain a one-on-one mapping between the SNR and gust speed statistics to characterize the impacts of wind on agricultural link stability at the mmWave spectrum.
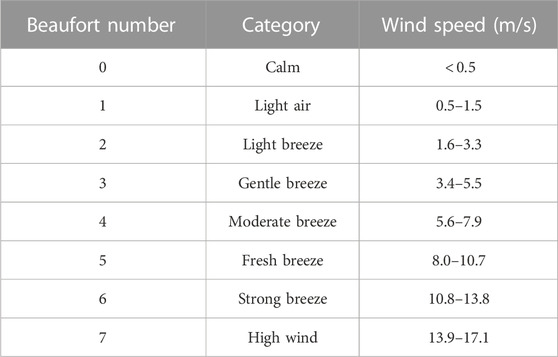
TABLE 2. Partial Beaufort scale (Fry, 1967).
3.3 Observation of wind effects on link stability
We obtain a temporal correlation between the received SNR and the 5-s gust speed. A total of 50 data samples were collected across the 8-min measurement duration on 7 July and 61 data samples from 16 July. As shown in the upper subfigures in Figures 3A, B, the x-axis shows time sample indices; the blue boxes are the SNR values measured in the 1-min interval, with the red lines inside indicating the average values; and the red crosses represent extreme cases. The lower subfigures are the corresponding gust speeds. Figure 3A is plotted for the experiments conducted on 7 July 2021, and Figure 3B presents the data collected from the experiments on 16 July 2021. This observation sets our baseline for the link resiliency scheme aided by AgRIS (detailed in Section 6). The rest of the channel and environment factors, including link distance (77 m), transmit power (36 dBm), measurement locations, transceiver height (8 ft/2.44 m), and polarization states (vertical polarization), are maintained unchanged.
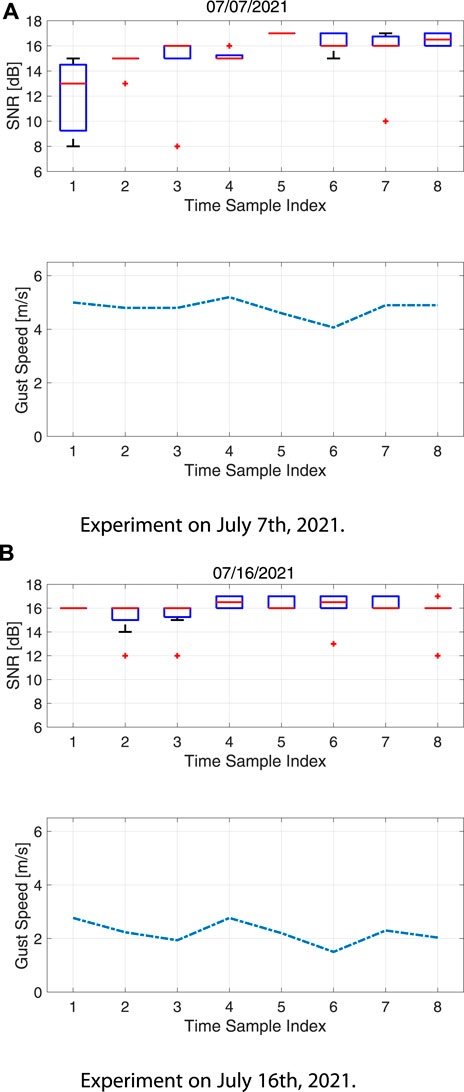
FIGURE 3. Correlation between the gust speed and SNR over time from agricultural field experiments. It is observable that a higher SNR fluctuation is correlated with strong gusts. (A) Experiment on 7 July 2021. (B) Experiment on 16 July 2021. The red crosses represent the SNR with maximum link distortion.
To quantify the SNR variation under different wind conditions, we fit an exponentially modified Gaussian distribution3 with the following probability distribution function (Grushka, 1972):
with a mean of μ + 1/λ and a variance of σ2 + 1/λ2. As shown in Figure 4, a longer left tail can be observed from the 7 July data, indicating a larger SNR variation. Correspondingly, the value of μ is smaller, but the value of σ is higher. These values from the exponentially modified Gaussian distribution provide a tool to quantitatively characterize the SNR variation due to wind. It is straightforward to distinguish the wind conditions of these 2 days from the fitted dashed curves. We can expect that under high wind conditions, μ as the dominating part of the average will decrease, while σ, which indicates the variance of the Gaussian distribution, will increase.
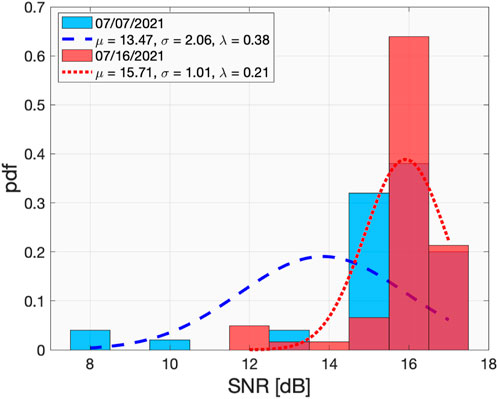
FIGURE 4. Probabilistic distributions of SNR values on the two experiment days and the best-fit exponentially modified Gaussian distributions.
4 System model
The traditional RISs are a type of two-dimensional artificial material (also known as metasurfaces) consisting of an array of patch elements that can control the wavefront of electromagnetic waves to achieve engineered functionalities, including controlled reflection, absorption, beam split, and beam collimation (Liaskos et al., 2018; Di Renzo et al., 2020). A control mechanism exists, including varactors and PIN diodes connected to the patch elements on the RIS, to achieve phase shift modulation of each patch element (Zhang et al., 2018). Hence, RISs can engineer the wavefront by applying different bias voltages to the varactors. AgRIS has an additional feature: to be adaptive to the external environment with various subarray dimensions, which can be activated or deactivated using the existing control mechanism. This feature makes AgRIS suitable for dynamic wireless channels, including the mmWave agricultural scenario, as illustrated in Figure 1.
Different from traditional RISs that are mostly mounted on exterior and/or interior walls of buildings in the urban scenario, our design leverages the existing vertical infrastructure in agricultural operations, such as farmhouses, water towers, grain bins, irrigation systems, and tractors, as surfaces for AgRIS. These infrastructure elements have exterior facades that are suitable for RIS deployment. To maintain link resiliency despite the wind, the AgRIS design controls the phase shift of each element under different subarray configurations. In this way, AgRIS can generate different beam patterns and rescatter the impinging wave in different directions with varying beam gains.
4.1 Received SNR model of AgRIS-aided links
The geometry of an AgRIS-aided link is shown in Figure 5, where a Tx, an Rx, and AgRIS are shown along with the links created among them. We assume that both transceivers and AgRIS are in each other’s far field. The power received by the Rx has two sources: the LoS path from the Tx (the black arrow) and the path reflected from AgRIS near the Rx (the blue arrows). The LoS path loss can be expressed as (Ju et al., 2019) follows:
where PL0(d0) represents the free-space path loss at a reference distance d0 = 1 m; nLoS is the path loss exponent (PLE); dTR is the distance between the transceivers in boresight; Aair is the attenuation caused by atmospheric gases, which are dominated by oxygen and water vapor molecules suspended in the air; and χσ is the shadowing factor with a standard deviation σ.
Based on the field experiment data, the PLE nLoS will vary with crop growth stages. For the ease of received power calculation, we rewrite (2) in the form of average path gain as
where g0(d0) is the path gain of the reference distance, which is the inverse of path loss. Since the atmospheric attenuation is shown to be insignificant, given the environmental data during our field experiments (Nie et al., 2022), we ignore the term Aair here. Following the Friis formula, the average path gain of the reflected path from AgRIS can be expressed as
where dT–S is the Euclidean distance from the Tx (denoted as “T” in subscript) to AgRIS (denoted as “S” in subscript), dS–R is the Euclidean distance from AgRIS to the Rx (denoted as “R” in subscript), λ is the wavelength, and lAgRIS is the reflection loss due to AgRIS. Notably, a free-space path loss exponent n0 = 2 is assumed in this path because AgRIS is assumed to be mounted at the infrastructure above the crop canopy, thus not obstructing the first Fresnel zone between transceivers. The reflection loss, which is caused by impedance mismatch at the boundary of two propagation media (i.e., the air and the metal patch element of AgRIS), can be modeled as the ratio between the power of the reflected power and that of the incident power, which is expressed as
where n0 = 1 is the refractive index of air and n1 is the refractive index of the material chosen for AgRIS’s metal patch element, which depends on the design choice.
With respect to the propagation channel, a cumulative time-domain channel response under noncoherent combining can be expressed as
where hLoS is the channel response of the LoS path, which can be expressed as
where Gt(θt, φt) and Gr(θr, φr) are the antenna/antenna array gains of Tx and Rx at the pointing angles in the elevation and azimuth planes, respectively. In Eq. 6 hAgRIS is the channel response from the AgRIS-reflected path, which is
where GAgRIS(θR, φR) is the gain of AgRIS in the direction of (θR, φR). The received signal-to-noise ratio (SNR) with a transmit power of Pt is
where B is the signal bandwidth and N0 = kT is the noise spectral density, with k being the Boltzmann constant and T being the temperature. Observing the susceptibility of the direct point-to-point path between transceivers during windy conditions, we design a scheme aided by AgRIS to prevent the sudden power level decrease. Next, we further elaborate on the design of AgRIS regarding GAgRIS.
4.2 AgRIS with subarrays in a concentric square shape
Different from conventional RIS design, where the surface patch elements are controlled on a row and/or column basis as a uniform array, AgRIS is controlled in a manner of subarrays in concentric square shapes. The reason for such a design is that, unlike other designs that achieve beam flattening (Lu et al., 2021), our design keeps the square shape of the RIS, thus maintaining a general beam shape profile in both azimuth and elevation planes, with only variations in beamwidth and array gains. As illustrated in Figure 6, the center array has a square shape, which has a size of n1 elements (with
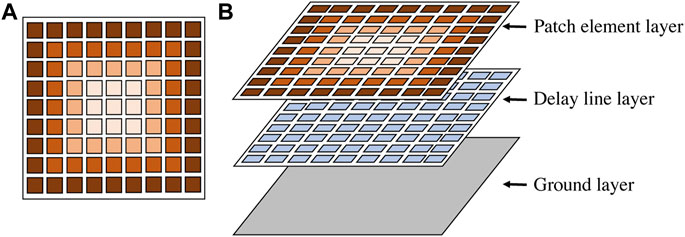
FIGURE 6. AgRIS design with subarrays following a concentric square shape. (A) Top layer of AgRIS with different colors indicating the boundaries of subarrays. (B) Structure of AgRIS with multiple layers.
More specifically, since this work focuses on the operation of AgRIS and the achievable performance metrics under dynamic agricultural environments, without loss of generality, we make the following assumptions: 1) for AgRIS elements, the phase shifts are continuous in the range of [0, 2π]. This wide range of continuous phase shifts has been shown to be achievable in the existing literature when applying an external bias voltage at different levels to control the impedance of the substrate based on liquid crystal (Li X. et al., 2021); 2) AgRIS has knowledge about the direction of the Rx (via links through low-rate wireless techniques, such as LoRaWAN); and 3) both the Tx and Rx remain static during data transmission4. The array gain of AgRIS, modeled in Eq. 8, can be further expressed as (Zhang et al., 2018):
where the second line represents the array factor, k = 2π/λ is the wavenumber, E(n,m)(θ, φ) is the far-field radiation pattern of a single element, and dx and dz represent the inter-element spacing along the y- and z-axes, respectively, when AgRIS is placed along the y–z plane following a three-dimensional Cartesian coordinate system. This relationship follows the pattern multiplication rule (Balanis, 2015). AgRIS will demonstrate different patterns and array gains depending on the activated subarray.
4.3 Environment-aware AgRIS link adaptation
In general, AgRIS is adaptive to environmental changes from predictions based on computations of measured environmental data through an external device, such as an anemometer for wind speed or a rain gauge for precipitation rate. This unique feature of AgRIS makes it more adaptive than the traditional RIS. The prediction results can be transmitted to AgRIS through low-rate communication links. The wind speed prediction model is detailed in Section 5. This scheme ensures that short-term wind speed prediction is sufficiently accurate and can be used in AgRIS configuration in near real-time.
5 Short-term gust speed prediction model
In this section, we present the wind speed prediction model, which will be used to select the appropriate AgRIS configuration. Prior work on wind speed prediction is mainly focused on its applications in wind power systems. Researchers mostly used support vector machines (He and Xu, 2019), deep neural networks (Khosravi et al., 2018), and other machine learning algorithms to forecast short-term wind speed. However, other closely correlated environmental factors, such as air pressure, have not been considered, which may affect the prediction accuracy.
Since wind speed is a time-series data, analysis methods in time series are applicable. The cause of wind is the difference in air pressure, which is an exogenous factor, which is also a time series. Typically, a time-series model relies on the current and previously observed values to predict future ones. Since wind speed constantly changes and varies geographically and seasonally, a data-based approach is more suitable for developing accurate prediction results.
The non-linear autoregressive exogenous (NARX) model, a non-linear extension of the time-series model, relies on not only the data of interest but also on the external series of data that influences the data under study (Li et al., 2011). The NARX model is defined as
where v is the variable of interest (e.g., wind speed in our case), p is the exogenous variable (e.g., air pressure in our case), and
6 Numerical results
In this section, we present a quantitative study of the AgRIS design and analyze the system performance in a wideband mmWave agricultural network. First, we study a baseline scenario without AgRIS on the basis of experimental data. Then, we analyze the performance when AgRIS is utilized to improve link resiliency. The SNR value is chosen as the metric that indicates potential performance improvement.
6.1 Baseline scenario
In the baseline scenario, the only viable path is the LoS path (i.e., the black line in Figure 5), where both beams of Tx and Rx are aligned and configured based on Table 1. We leveraged the data from our field experiments in 2021 (Vuran et al., 2022). The boresight link distance is 77 m, and both transceivers are at the same height of 8 ft (
6.2 AgRIS-aided link performance
In this scenario, we consider the link structure in Figure 5, where AgRIS will reflect signals from the Tx toward the Rx when activated to improve link performance. In addition, in situ weather data are collected by a weather station. A computer connected to the weather station will predict the wind speed based on recorded and/or measured data in real-time. We propose two operating modes of AgRIS: 1) under different wind speeds predicted, different subarrays will be activated (denoted as the subarray mode); 2) if a predicted wind speed exceeds a predefined threshold, we will activate and maintain the largest subarray of AgRIS (denoted as the ON/OFF mode), which is the same as a traditional RIS. We will compare the spectral efficiency and energy efficiency for both cases.
The AgRIS inter-element spacing is assumed to be λ/2 to minimize the effects of mutual coupling and grating lobes. The angle of controlled reflection is assumed to be 30° from the normal direction of AgRIS. When wind impacts the agricultural field, the weather station will record the 5-s gust speed and feed such data to the local computer. The short-time wind speed forecast model will compute the gust speed in the following minute, and the corresponding AgRIS configuration will be sent to the controller of AgRIS. Then, signals from both the LoS and AgRIS-reflected paths are combined at the Rx.
We perform a multistep prediction of wind speed based on the NARXNET model elaborated in Section 5 by setting a prediction interval of 8 min, representing the same duration of signal transmission as shown in our examples in Figure 2. This updating frequency ensures that AgRIS will be updated near real-time during each link period. As shown in Figure 8, the mean square error reaches its minimum value at epoch 11, yielding 0.099641, which outperforms the short-term prediction method using support vector machines in He and Xu (2019).
In the adaptive scheme, based on the Beaufort scale and the field experiment results shown in Figure 2, we design and map the following wind speed and AgRIS array gain:
with three subarray dimensions, n1 = 5 × 5, n2 = 7 × 7, and n3 = 11 × 11. These dimensions of AgRIS are set such that Tx, AgRIS, and Rx are in each other's electromagnetic far field while maintaining a cost-effective footprint to be suitable for agricultural applications. When the gust speed is less than 2 m/s, no AgRIS is activated because the LoS path is sufficiently stable, as indicated in Figure 2. In Figure 9, a comparison between the baseline scenario and AgRIS-aided link performance is provided. The black curve denotes the performance in the baseline scenario, where the minimum SNR observed in the 1-min interval (in Figure 2) is plotted, indicating the worst-case scenario. In the AgRIS-aided link, two operation modes are adopted: 1) ON or OFF and 2) subarrays according to (12), respectively. The blue dashed curve shows the performance of the first mode, which shows the best performance. This is because regardless of the gust speed predicted, as soon as one predicted value is greater than the predefined threshold, AgRIS is activated and maintained at its full dimension, which is identical to the traditional RIS operation. The red dashed curve demonstrates the performance when the proposed subarray structure is utilized. It is shown that the SNR shows less variation as compared to the baseline case, indicating a much more resilient link, with at most 8 dB increased from the worst-case scenario. The difference between the red and blue dashed curves shows that different subarrays are activated under different predicted gust speeds. The maximum difference is less than 2 dB. Next, we compare the energy efficiency of these two options to demonstrate that the subarray design is preferred in real-world applications.
This design with four levels of array gains can be quantized into a two-bit control signal in front of the actual AgRIS phase configuration stream. Based on the predicted gust speed in the adaptive link, AgRIS will be switched to different states with subarray gains specified in Eq. 12. Hence, the spectral efficiency is computed as
6.3 Energy efficiency
Since AgRIS is an adaptive mmWave RIS, phase control components are needed. The requirement of a large range of phase shifts corresponds to a large range of capacitance needed, which indicates that varactor diodes are preferred over PIN diodes. In particular, in operation at 60 GHz, fewer options are available for varactor diodes, and one of them is MACOM’s MAVR-000120-1411, which is a gallium arsenide (GaAs) hyperabrupt varactor diode and has a capacitance range from 0.14 pF at 10.5 V reverse bias voltage to 0.9 pF at 1 V reverse bias voltage (MACOM, 2022). We assume that each AgRIS surface element is connected to a varactor diode to realize phase shifts. In addition, other electronic components, including controllers, also consume energy in the AgRIS system. The energy efficiency at AgRIS can be expressed as
where Pctrl and Pdiode are the power consumed at the controller as the static power and each diode as the dynamic power, respectively, and N is the number of varactor diodes used for activating elements. We suppose that Pctrl = 15.75 W (Wang et al., 2022) and each diode operating at 60 GHz consumes 15 mW. Once activated, the average power consumption for the largest AgRIS subarray is 17.565 W. We can then compare the numerical result of energy efficiency for both AgRIS options. As shown in Figure 10, when both static and dynamic power consumption is considered, both operation options of AgRIS demonstrate comparable energy efficiency performance (shown in the blue and red dashed curves, respectively). The reason is that the dominant factor is the static power compared to the relatively small AgRIS size in our current design. When dynamic power consumption is the sole factor considered, the subarray design outperforms the ON/OFF design remarkably because fewer elements are activated to achieve sufficient resiliency. In general, as the dimension of AgRIS increases, especially when dynamic power becomes the dominant factor, the subarray design will be a preferable option with respect to throughput and energy efficiency.
7 Conclusion
In this work, we present the design of AgRIS, an adaptive RIS for agricultural wireless networks, which aims to improve link resiliency despite varying wind conditions. Using data from field experiments, we quantify the temporal correlation between link reliability and wind speed and fit such a variation using an exponentially modified Gaussian distribution. Accordingly, we utilize a time-series NARXNET model to predict short-term wind speeds and validate its performance through recorded data from an in situ weather station. Our prediction model is shown to outperform existing solutions. By mapping the AgRIS with various subarrays to different wind speed levels based on the prediction, we demonstrate through simulation results that the AgRIS can help mitigate the impact under wind-induced signal level decrease and strengthen link resiliency with up to 8-dB SNR improvement compared to the baseline case without AgRIS while doubling the energy efficiency as compared to a traditional RIS. Potential future directions include harvesting wind resources to power AgRIS for sustainable wireless agricultural networks, which will be our future work.
Data availability statement
Publicly available datasets were analyzed in this study. These data can be found at: https://ieee-dataport.org/documents/mmwave-farm-channel-modeling-wireless-agricultural-networks-broadband-millimeter-wave.
Author contributions
SN and MV contributed to the conception and design of the study. MV organized the database. SN performed the statistical analysis. All authors contributed to the article and approved the submitted version.
Funding
This work was partly supported by the U.S. National Science Foundation grants ECCS-2030272 and CNS-2212050.
Acknowledgments
The authors would like to thank Yufeng Ge, Frank Bai, Santosh Pitla, David Scoby, Stuart Hoff, and Paul Jasa for their support in conducting experiments at the corresponding sites. The authors also thank Mohammad M.R. Lunar, Prashant Subedi, David Besonen, and Sang Won Shin for their help in equipment setup and field measurements.
Conflict of interest
The authors declare that the research was conducted in the absence of any commercial or financial relationships that could be construed as a potential conflict of interest.
Publisher’s note
All claims expressed in this article are solely those of the authors and do not necessarily represent those of their affiliated organizations, or those of the publisher, the editors, and the reviewers. Any product that may be evaluated in this article, or claim that may be made by its manufacturer, is not guaranteed or endorsed by the publisher.
Footnotes
1Gusts are short term over 5 s.
2https://ard.unl.edu/phenotyping/field-phenotyping-facility
3This distribution describes the sum of two random variables that independently follow a Gaussian distribution and an exponential distribution, respectively.
4This assumption is to ensure that the only channel variation comes from the environment dynamics. Our future work will discuss the mobility of transceivers.
References
Alejos, A., Sanchez, M. G., Cuinas, I., and Gomez, P. (2007). Depolarization effect by wind incidence on vegetation at 40 GHz. In The second European conference on antennas and propagation, EuCAP 2007, Edinburgh Scotland: IEEE, 1–6. doi:10.1049/ic.2007.1554
Atefi, A., Ge, Y., Pitla, S., and Schnable, J. (2021). Robotic technologies for high-throughput plant phenotyping: Contemporary reviews and future perspectives. Front. Plant Sci. 12.
Chamara, N., Islam, M. D., Bai, G. F., Shi, Y., and Ge, Y. (2022). Ag-IoT for crop and environment monitoring: Past, present, and future. Agric. Syst. 203, 103497. doi:10.1016/j.agsy.2022.103497
Di Piazza, A., Di Piazza, M., and Vitale, G. (2014). Estimation and forecast of wind power generation by FTDNN and NARX-net based models for energy management purpose in smart grids. algorithms 8, 13.
Di Renzo, M., Zappone, A., Debbah, M., Alouini, M.-S., Yuen, C., De Rosny, J., et al. (2020). Smart radio environments empowered by reconfigurable intelligent surfaces: How it works, state of research, and the road ahead. IEEE J. Sel. areas Commun. 38, 2450–2525.
Dong, X., Vuran, M. C., and Irmak, S. (2013). Autonomous precision agriculture through integration of wireless underground sensor networks with center pivot irrigation systems. Ad Hoc Netw. 11, 1975–1987.
Fara, R., Ratajczak, P., Phan-Huy, D.-T., Ourir, A., Di Renzo, M., and De Rosny, J. (2022). A prototype of reconfigurable intelligent surface with continuous control of the reflection phase. IEEE Wirel. Commun. 29, 70–77.
Grushka, E. (1972). Characterization of exponentially modified Gaussian peaks in chromatography. Anal. Chem. 44, 1733–1738.
He, J., and Xu, J. (2019). Ultra-short-term wind speed forecasting based on support vector machine with combined kernel function and similar data. EURASIP J. Wirel. Commun. Netw. 2019, 1–7.
John Deere (2022). John deere advances automated farming at ces 2023. Available at: https://www.iotworldtoday.com/2023/01/06/john-deere-advances-automated-farming-at-ces-2023/.
Ju, S., Kanhere, O., Xing, Y., and Rappaport, T. S. (2019). “A millimeter-wave channel simulator nyusim with spatial consistency and human blockage,” in 2019 IEEE global communications conference (GLOBECOM), 1–6. doi:10.1109/GLOBECOM38437.2019.9013273
Karasik, R., Simeone, O., Di Renzo, M., and Shitz, S. S. (2021). Adaptive coding and channel shaping through reconfigurable intelligent surfaces: An information-theoretic analysis. IEEE Trans. Commun. 69, 7320–7334.
Khosravi, A., Koury, R., Machado, L., and Pabon, J. (2018). Prediction of wind speed and wind direction using artificial neural network, support vector regression and adaptive neuro-fuzzy inference system. Sustain. Energy Technol. Assessments 25, 146–160.
Lee, G., Jung, M., Kasgari, A. T. Z., Saad, W., and Bennis, M. (2020). “Deep reinforcement learning for energy-efficient networking with reconfigurable intelligent surfaces,” in ICC 2020-2020 IEEE international conference on communications (ICC) (IEEE), 1–6.
Li, G., Wen, C., Zheng, W. X., and Chen, Y. (2011). Identification of a class of nonlinear autoregressive models with exogenous inputs based on kernel machines. IEEE Trans. Signal Process. 59, 2146–2159. doi:10.1109/TSP.2011.2112355
Li, Q., Wen, M., and Di Renzo, M. (2021). Single-RF MIMO: From spatial modulation to metasurface-based modulation. IEEE Wirel. Commun. 28, 88–95.
Li, X., Wan, Y., Liu, J., Jiang, D., Bai, T., Zhu, K., et al. (2021). Broadband electronically scanned reflectarray antenna with liquid crystals. IEEE Antennas Wirel. Propag. Lett. 20, 396–400.
Liaskos, C., Nie, S., Tsioliaridou, A., Pitsillides, A., Ioannidis, S., and Akyildiz, I. (2018). A new wireless communication paradigm through software-controlled metasurfaces. IEEE Commun. Mag. 56, 162–169.
Liaskos, C., Nie, S., Tsioliaridou, A., Pitsillides, A., Ioannidis, S., and Akyildiz, I. (2020). End-to-End wireless path deployment with intelligent surfaces using interpretable neural networks. IEEE Trans. Commun. 68, 6792–6806. doi:10.1109/TCOMM.2020.3012577
Lin, S., Zheng, B., Alexandropoulos, G. C., Wen, M., Chen, F., and Smumtaz, S. (2020). Adaptive transmission for reconfigurable intelligent surface-assisted OFDM wireless communications. IEEE J. Sel. Areas Commun. 38, 2653–2665.
Liu, Y., Li, D., Du, B., Shu, L., and Han, G. (2022). Rethinking sustainable sensing in agricultural Internet of things: From power supply perspective. IEEE Wirel. Commun. 29, 102–109. doi:10.1109/MWC.004.2100426
Lu, H., Zeng, Y., Jin, S., and Zhang, R. (2021). Aerial intelligent reflecting surface: Joint placement and passive beamforming design with 3D beam flattening. IEEE Trans. Wirel. Commun. 20, 4128–4143.
MacCartney, G. R., and Rappaport, T. S. (2017). Rural macrocell path loss models for millimeter wave wireless communications. IEEE J. Sel. Areas Commun. 35, 1663–1677. doi:10.1109/JSAC.2017.2699359
MACOM (2022). Solderable GaAs Constant Gamma Flip-Chip Varactor Diode, MAVR-000120-1411. Available at: https://cdn.macom.com/datasheets/MAVR-000120-1411.pdf
Nie, S., Lunar, M. M., Bai, G., Ge, Y., Pitla, S., Koksal, C. E., et al. (2022). Mmwave on a farm: Channel modeling for wireless agricultural networks at broadband millimeter-wave frequency. 2022 19th Annu. IEEE Int. Conf. Sens. Commun. Netw. (SECON), 388–396. doi:10.1109/SECON55815.2022.9918595
Salam, A., and Vuran, M. C. (2017). “Smart underground antenna arrays: A soil moisture adaptive beamforming approach,” in Ieee infocom 2017 - IEEE conference on computer communications, 1–9. doi:10.1109/INFOCOM.2017.8056990
Shkel, A., Mehrabani, A., and Kusuma, J. (2021). “A configurable 60GHz phased array platform for multi-link mmWave channel characterization,” in 2021 IEEE ICC workshops, 1–6. doi:10.1109/ICCWorkshops50388.2021.9473724
Vuran, M. C., Lunar, M. M., Nie, S., Ge, Y., Pitla, S., Bai, G., et al. (2022). “Millimeter-wave agricultural channel measurements in corn and soybean fields at different growth stages,” in 2022 IEEE international symposium on antennas and propagation and USNC-ursi radio science meeting (AP-S/URSI) (Denver, CO, United States: IEEE), 1686–1687. doi:10.1109/AP-S/USNC-URSI47032.2022.9887346
Vuran, M. C., Salam, A., Wong, R., and Irmak, S. (2018). Internet of underground things in precision agriculture: Architecture and technology aspects. Ad Hoc Netw. 81, 160–173.
Wang, J., Tang, W., Han, Y., Jin, S., Li, X., Wen, C.-K., et al. (2021). Interplay between RIS and AI in wireless communications: Fundamentals, architectures, applications, and open research problems. IEEE J. Sel. Areas Commun. 39, 2271–2288. doi:10.1109/JSAC.2021.3087259
Wang, J., Tang, W., Liang, J. C., Zhang, L., Dai, J. Y., Li, X., et al. (2022). Reconfigurable intelligent surface: Power consumption modeling and practical measurement validation. arXiv preprint arXiv:2211.00323.
Wei, Z., Zhu, X., Sun, S., Huang, Y., Dong, L., and Jiang, Y. (2015). Full-duplex versus half-duplex amplify-and-forward relaying: Which is more energy efficient in 60-GHz dual-hop indoor wireless systems? IEEE J. Sel. Areas Commun. 33, 2936–2947. doi:10.1109/JSAC.2015.2481211
Zeinali, S., Dehghani, M., and Talebbeydokhti, N. (2021). Artificial neural network for the prediction of shoreline changes in Narrabeen, Australia. Appl. Ocean Res. 107, 102362.
Keywords: wireless networks, millimeter-wave communications, reconfigurable intelligent surfaces, rural broadband, agricultural networks
Citation: Nie S and Vuran MC (2023) AgRIS: wind-adaptive wideband reconfigurable intelligent surfaces for resilient wireless agricultural networks at millimeter-wave spectrum. Front. Comms. Net 4:1169266. doi: 10.3389/frcmn.2023.1169266
Received: 19 February 2023; Accepted: 22 May 2023;
Published: 19 June 2023.
Edited by:
Josep Miquel Jornet, Northeastern University, United StatesReviewed by:
Arjun Singh, SUNY Polytechnic Institute, United StatesChong Han, Shanghai Jiao Tong University, China
Copyright © 2023 Nie and Vuran. This is an open-access article distributed under the terms of the Creative Commons Attribution License (CC BY). The use, distribution or reproduction in other forums is permitted, provided the original author(s) and the copyright owner(s) are credited and that the original publication in this journal is cited, in accordance with accepted academic practice. No use, distribution or reproduction is permitted which does not comply with these terms.
*Correspondence: Shuai Nie, shuainie@unl.edu