- 1Data Science Initiative, University of California, Davis, Davis, CA, United States
- 2University Library, University of California, Davis, Davis, CA, United States
There is widespread interest in evaluating the social impacts of research and other scholarly activities. Conventional metrics for social impacts focus on economics or wealth creation, such as patents or technology transfer. These kinds of metrics are less appropriate for many scholarly fields, and miss the specific social concerns or needs that researchers aim to address. In this paper, drawing on ideas from ethics and development economics, we develop a conceptual framework for characterizing the social goals of research. We first distinguish resources—such as wealth and intellectual credit—from the goals of scholarship, and further distinguish inward- and outward-facing goals. Outward-facing goals refer to the intended social impacts of research. Next we introduce the Capabilities Approach, a conceptual framework for human well-being developed by ethicists and economists over the last 40 years. This Approach focuses on basic human needs, rather than wealth, and we draw specifically on a list of central human capabilities developed by philosopher Martha Nussbaum. We propose that the items on this list provide a useful starting point for articulating the specific social aims of research. We argue that the Capabilities Approach can facilitate research communication and improve the recognition of public engagement in academic and funding institutions. Familiar bibliographic data and text mining methods can be used in a capabilities-inspired portfolio analysis, and modest changes to existing data collection systems—for tenure and promotion, or research funding applications—could support the development of even richer capabilities-inspired metrics and incentive systems.
Introduction
Among researchers, university administrators, and science policymakers, there is widespread interest in understanding and measuring the social impact of research and other scholarly activities. In Science, the Endless Frontier, Vannevar Bush argued for the creation of the US National Science Foundation — an institution dedicated to funding “basic” rather than “applied” scientific research — on the grounds that “new scientific knowledge” was essential to treat disease and mental illness, protect national security, and promote “the public welfare” more generally (Bush, 1945–1960, pp. 5-6). Today, discussions of the social impact of research often focus on the economic impacts of that research (Narin et al., 1997; Toole, 2012; Fealing and Johnson, 2017; Li et al., 2017).
But the interests of society go well beyond an interest in wealth generation (Bozeman and Sarewitz, 2005). Policymakers and members of the general public frequently have specific concerns or needs that researchers aim to address. In this paper, we propose that a more specific conceptual framework would be useful for characterizing the aims of research and other scholarly activities. We first distinguish two kinds of goals for scholarly research — inward- and outward-facing — and discuss the relationship between these goals, the value of research, and conventional bibliometrics and economic metrics of research productivity. Next we introduce the Capabilities Approach, a conceptual framework for national well-being that was developed by economists and philosophers as an alternative to measures such as Gross Domestic Product. Specifically, we discuss a list of “central capabilities,” developed as a list of universal basic human needs by ethicist Martha Nussbaum (Nussbaum, 2000, 2006). We suggest that the social goals of scholarly research can often be described in terms of this list of central capabilities. To illustrate our argument, we examine in detail the variety of ways in which researchers from different fields might contribute to a central capability for adequate shelter.
We then turn to the development of metrics for tasks such as portfolio analysis and program evaluation. We first apply text mining and natural language processing methods to standard bibliographic data, showing how existing capabilities-oriented research can be discovered across conventional academic disciplines. Next we reflect on the full range of scholarly outputs and the need for institutionalized connections between researchers and the people who would benefit from their research. Finally we consider how internal institutional data, such as researcher curricula vitae and grant applications, can be used to identify and incentivize social engagement and outward-facing scholarly activities (that is, activities with outward-facing goals). We consider both the value of existing institutional data and how modest changes to data-collection systems might dramatically improve their value for capabilities-inspired incentives or program evaluation.
The Goals of Scholarly Research
Scholarly research can be understood as a social practice — that is, a complex, collaborative, goal-oriented, socially organized activity (Hicks and Stapleford, 2016). Many fields of research have both inward-facing and outward-facing goals. Inward-facing goals characterize the value of research for the relevant scholarly community, in terms of the further production of new knowledge (new conceptual frameworks, new findings, new instruments for data collection, new analytical methods). For example, establishing the existence of the Higgs boson leads to new research projects to more precisely measure its properties (Staley, 2017). Outward-facing goals characterize the value of research for other social practices. For example, conservation biology was originally defined in terms of contributing to broader social efforts to protect biodiversity and preserve natural or undeveloped spaces (Soulé, 1985). Similarly, agricultural scientists, for example — including plant and animal breeders, weed scientists, pest entomologists, and so on — generally see their work as contributing to efforts to feed the world. In some research fields, outward-facing goals may be limited to “dissemination” or “communication,” a one-way transmission of inward-facing achievements to various publics. But the examples of conservation biology and agricultural science show that inward- and outward-facing goals can also be deeply intertwined. The broader, outward-facing goals of these fields shape the kinds of inward-facing intellectual achievements that are valued within the community of researchers.
Both kinds of goals are distinct from resources. Resources are goods that are valuable because they are useful for other purposes — such as pursuing research goals — but are not valuable on their own. For example, external funding is essential in many fields; but is only valuable insofar as it is used to support further research. Latour and Woolgar have observed that credit is a vital resource in scientific practice: researchers, labs, or institutions are rewarded for their accomplishments (whether actual or merely apparent) in terms of “credit” or “credibility,” and this resource can be “invested” or “spent” on research funding, attracting prestigious (i.e., high-credit) faculty/collaborators, or in intellectual debates (Latour and Woolgar, 1979, ch 5). However, at the level of the intellectual community, the production and circulation of credit is neither an inward- nor outward-facing goal.
The distinction between goals and resources does not mean that resources are worthless or unimportant. Indeed, resources are extremely important. However, they are important only insofar as they are useful for the pursuit of the goals of inquiry. It is more than reasonable for researchers to pursue funding and scholarly credit, because these resources are essential for producing new knowledge or contributing to important social goods. But funding and credit are not valuable in themselves, and it is a conceptual mistake to evaluate researchers on the basis of funding and credit alone.
Citation-based bibliometrics — including citation counts, the h-index, and Journal Impact Factor — are often understood as attempts to generically measure the inward-facing value of academic research. The idea here is simple enough: researchers tend to cite more important or valuable research, and so papers with more citations contain more intrinsically valuable results. So, rather than relying on complicated expert judgments of how well a body of research promotes various field-specific aims, we can simply (and, supposedly, objectively) count citations (Garfield, 1979, p. 2). However, there are a number of familiar problems with this interpretation of citation-based metrics. There are, for example, documented “citation penalties” against women (Larivière et al., 2013; Laurance et al., 2013; Maliniak et al., 2013; Ghiasi et al., 2015; Mihaljević-Brandt et al., 2016) and uneven citation practices across disciplines complicates evaluation of interdisciplinary research (Wang et al., 2017). On average, life science, humanities, and social science papers contain more citations than physical science, mathematics, and computer science papers (Boyack et al., 2018; Sánchez-Gil et al., 2018), so raw citation counts must be “normalized” across fields. But there is no broad agreement about how this normalization should be carried out (Radicchi et al., 2008; Waltman et al., 2012; Hutchins et al., 2016, 2017; Janssens et al., 2017). Different fields also operate according to different publication timelines. It is common in the humanities, for example, where mongraphs remain a primary research output, for citation curves to span many years or even decades, whereas citation curves in the experimental sciences more frequently span significantly shorter time periods. On a more theoretical level, Hicks and Melkers (2012) have identified a taxonomy of citation types that identifies 13 different theories of researchers' citations, which percolate differently through citation networks. For these reasons, citation-based bibliometrics are at best a highly controversial proxy for inward-facing value.
Most importantly for our purposes here, understanding citation-based bibliometrics as measures of inward-facing value risks confusing resources with goals. High citation counts, as such, are never the primary goal of research. Sacrificing scientific quality for the sake of high citation counts in prestigious journals violates the norms of research integrity. Researchers who are caught engaging in this kind of behavior are subject to sharp criticism from their peers and, potentially, professional censure (Levelt Committee et al., 2012; Van der Zee, 2017; Wansink, 2017).
Instead of measures of inward-facing value, citation counts are a useful measure of credit, in Latour and Woolgar's sense. (Note that Latour and Woolgar use citations to measure credit; Latour and Woolgar, 1979, Figures 5.2, 5.3, pp. 220–2, 225.) So, while citation-based bibliometrics can be useful for tracking an important resource within a research community, we should not confuse these metrics with evaluations of researchers' inward-facing accomplishments.
Similarly, economically-oriented metrics—such as patent citations—can be understood as attempts to generically measure the outward-facing value of academic research. These kinds of metrics are biased against fields that generally do not produce patentable technologies, such as cultural studies or astrophysics. They also potentially confuse a resource—wealth—with the actual outward-facing value of research. This is not to say that researchers do not care about wealth or intellectual property. Agronomists often work to develop patentable technologies that will economically sustain family farms. But these are means to further goals, namely, ensuring food security (and perhaps preserving the specific culture associated with family farms). Similarly, willingness-to-pay for ecosystem services might be used as a proxy for the outward-facing value of conservation biological research; but the aim of the research is to conserve the ecosystem, not improve economic efficiency (Raymond et al., 2013).
A few studies have attempted to measure the contributions of research to outward-facing goals, rather than resources or distant proxies for goals. Thonon et al. (2015) conducted a review of 76 bibliometric/research evaluation articles on biomedicine, looking specifically at the kinds of metrics (“indicators,” in their terminology) used in these articles. They identified 9 “indicators of health service impact,” which seem to be more-or-less direct measurements of the contribution of research to health. Examples of these metrics include “citation of research in policy guidelines,” “patients [sic] outcomes,” and “changes in clinical practice” (Thonon et al., 2015, Table 3). However, the most popular of these indicators, “citation of research in clinical guidelines,” appeared in only 7 articles (9%). By comparison, the h-index (which is based on scholarly citations, an inward-facing resource) appeared in 31 articles (41%). In addition, 7 of the 9 “health service impact” indicators had no methodological details, and the other 2 had only “suggested” methods.
In the remainder of this essay, we focus on outward-facing value, and bracket further discussion of inward-facing value. This is not to say that all researchers do, or should, focus on outward-facing goals. Inward-facing goals are important for the progress of research. Institutions should not replace an overemphasis on publication counts and impact factors with an overemphasis on outward-facing metrics. At the same time, we believe that many researchers would prefer to pursue more outward-facing goals, but feel compelled to produce more publications and maximize citation metrics—to chase the proxies for inward-facing value, even when they would rather work toward outward-facing goals.
Our discussion of outward-facing goals may remind readers of the US National Science Foundation's [US NSF] “broader impacts” criterion. This criterion, which is used in the review of all funding proposals submitted to US NSF, requires researchers to identify how a potential project “encompasses the potential to benefit society and contribute to the achievement of specific, desired societal outcomes.” The broader impacts criterion is considered by US NSF to be just as important as the other, inward-facing, intellectual merit criterion (National Science Foundation, 2018, III-2).
In line with US NSF's language, much of the science policy literature on the criterion treats “broader impacts” as more-or-less synonymous with outward-facing goals for research. For example, Bozeman (2017) gloss broader impacts as “criteria [sic] related to socio-economic impacts” (1), and note that “terms such as “socio-economic” impacts, “social impacts,” “societal impacts,” and “broader impacts” [are] used, sometimes interchangeably” (2) (see also Intemann, 2009; Schienke et al., 2009; Holbrook, 2012).
But internal US NSF analyses of funding proposals have found that proposed broader impacts activities overwhelmingly focus on STEM education and research communication. A topic model analysis of approximately 100,000 proposals, conducted for the National Science Board, found that 60% of proposals discussed broader impacts in terms of “teach/train/learn”—that is, STEM education— and 20% each on “broaden participation”—recruiting underrepresented groups into STEM careers—and “dissemination”—research communication. Only 10% of proposals discussed “other societal benefit[s]”—which include, but are not necessarily limited to, outward-facing research goals (National Science Board, 2011, p. 260). Similarly, in an analysis of approximately 600 awarded and declined proposals submitted to the Division of Environmental Biology, Watts et al. (2015) found much more emphasis on “teaching” than “benefits to society” (which, they note, is “the least definitive category” of broader impacts activities). In short, despite the criterion's outward-facing language, “broader impacts” is typically operationalized as inward-facing.
Our interest in outward-facing goals is also similar to Bozeman and Sarewitz' notion of “public values” for scientific research and policy (Bozeman and Sarewitz, 2005, 2011). Quoting Bozeman (2007), they define “society's public values” as
those providing normative consensus about (1) the rights, benefits, and prerogatives to which citizens should (and should not) be entitled; (2) the obligations of citizens to society, the state and one another; (3) and the principles on which governments and policies should be based (Bozeman and Sarewitz, 2011, p. 4).
They go on to suggest four ways of identifying such public values, namely, as embedded in laws and policies, as revealed by public opinion surveys, in “a posited model,” and as found in public policy statements. Public values are intended to provide a normative background for policy deliberation and evaluation. This approach is primarily procedural: to put it too crudely, “public values” are whatever the majority of citizens can agree on. By contrast, the capabilities approach that we introduce below is primarily based on substantive or “outcome-oriented” (Nussbaum, 2006, 81ff) notions such as human flourishing, dignity, and human rights (Nussbaum, 2006, 284ff). (At the same time, note that Bozeman (2007), 154-6, discusses “threats to dignity and subsistence” as one kind of “public values failure,” while Nussbaum (2006), 388ff appeals to the procedural notion of an “overlapping consensus.”)
While the public values approach is analytically useful, our review of Bozeman's work did not turn up anything like a concrete list of general public values. Relevant public values are identified in discussions of particular cases; but there does not appear to be anything like the list of central capabilities that we present and discuss below. (Bozeman, 2007, Tables 8.1 and 8.2, 140-1 and 143, do present lists of public values for good governance and good civil servants; but few if any of these items would seem to be goals for academic research). We feel that a concrete, but open-ended and revisable, list of general social goods is especially useful when, for example, researchers are trying to articulate the outward-facing goals of their research to non-specialists.
The Capabilities Approach
The Capabilities Approach is a conceptual framework for human well-being developed in the 1980s in economics and ethics (Sen, 1985). In the twentieth century, econometricians had promoted the use of Gross National Product [GNP] and Gross Domestic Product [GDP] as measures of well-being: a richer country was better-off. However, these kinds of metrics have two theoretical limitations. First, they are aggregative rather than distributive. The total amount of wealth in a country does not tell us whether that wealth is distributed more equitably or primarily held by a small fraction of the population. Similarly, they cannot tell us whether or to what extent a fraction of the population is faced with grinding poverty, unable to satisfy their basic needs.
The second—and, for our purposes, more significant—problem with wealth-based measures of well-being is that wealth is a resource and not a goal. It is not valuable on its own, but rather only because it is useful for exchanging for other goods (Aristotle, 2014, 1096a5). Amartya Sen, the originator of the Capabilities Approach, pointed out that individuals have different “capabilities” to translate wealth into other goods, closer to what we take to constitute well-being (Sen, 1985, 6–7; see also Nussbaum, 2006, chs. 2–3). Consider two people, one who is capable of walking normally and one who uses a wheelchair. Suppose they otherwise enjoy equal health, are equally well-educated, and in particular have the same income. The individual who uses the wheelchair will generally need more time to navigate spaces—they have to make their way around to the ramp or elevator rather than just walking up the stairs—and may simply be unable to access some spaces that are readily accessible to the individual who is able to walk normally—such as a sidewalk without accessibility cuts. The individual in the wheelchair will also have greater expenses than the normally-abled individual, even given their equal health. The individual in the wheelchair will either need to travel by public transportation — which will impose serious time costs — or use a heavily customized car. The relative scarcity of accessible housing will generally increase both the time they spend searching for housing and what they pay for housing. And so on. For these reasons, even a perfectly equal distribution of wealth will not translate to equal well-being for these two individuals.
Given these problems with wealth, Sen proposed that metrics of well-being should focus directly on “what people can do or be” (Sen, 1985, ix, emphasis in original; compare Stiglitz et al., 2009, 11); that is, what they are capable of. This “Capabilities Approach” has been especially influential in international development settings. In 1990, Sen served on the panel of consultants who developed the first United Nations Human Development Report; the theoretical framework for human development in chapters 1 and 2 of that report frequently refer to “capabilities” (United Nations Development Programme, 1990). Anand and Sen (1994) developed the methodology for the Human Development Index, which remains widely used in international development. The Capabilities Approach has also been influential in the development of health policy (Kibel and Vanstone, 2017) and rethinking the social impacts of science and technology (Mormina, 2018).
In the next section, we use Martha Nussbaum's list of central capabilities (Nussbaum, 2000) to extend the capabilities approach into portfolio analysis and research program evaluation. Nussbaum's list articulates a set of basic human needs and values—goods that are necessary, to some degree, for a minimally decent human life, and are recognized as such across history and cultural differences (Nussbaum, 2000, ch. 1). Importantly, the items on the list are specific enough to suggest workable metrics while also allowing for flexibility or interpretation across cultures and between individuals. For example, “adequate nutrition” is easy to recognize as a basic human need, and for many development purposes can be measured more-or-less straightforwardly in terms of calories and other nutrients consumed per day. But culturally appropriate food can vary across cultures and among individuals, and the preservation of landraces—traditional crop varieties, highly adapted to particular locations and often cultivated by indigenous and sustenance farmers—and food traditions has been a major issue in global food politics (Alkon and Mares, 2012; Brandt, 2014). In addition, the list is not considered comprehensive or complete; additional capabilities can be added as other basic human needs and values come to our attention.
Research and Central Capabilities
The list of central capabilities is given in Figure 1. We propose that the combination of specificity and flexibility reflected in this list makes the central capabilities useful for characterizing the outward-facing goals of many scholarly research programs. Many researchers see themselves as working to address problems such as food insecurity, homelessness, or the diabetes epidemic. While addressing these problems would have effects on state or national GDP, this would be a side effect of the research; increasing GDP is not the primary goal.
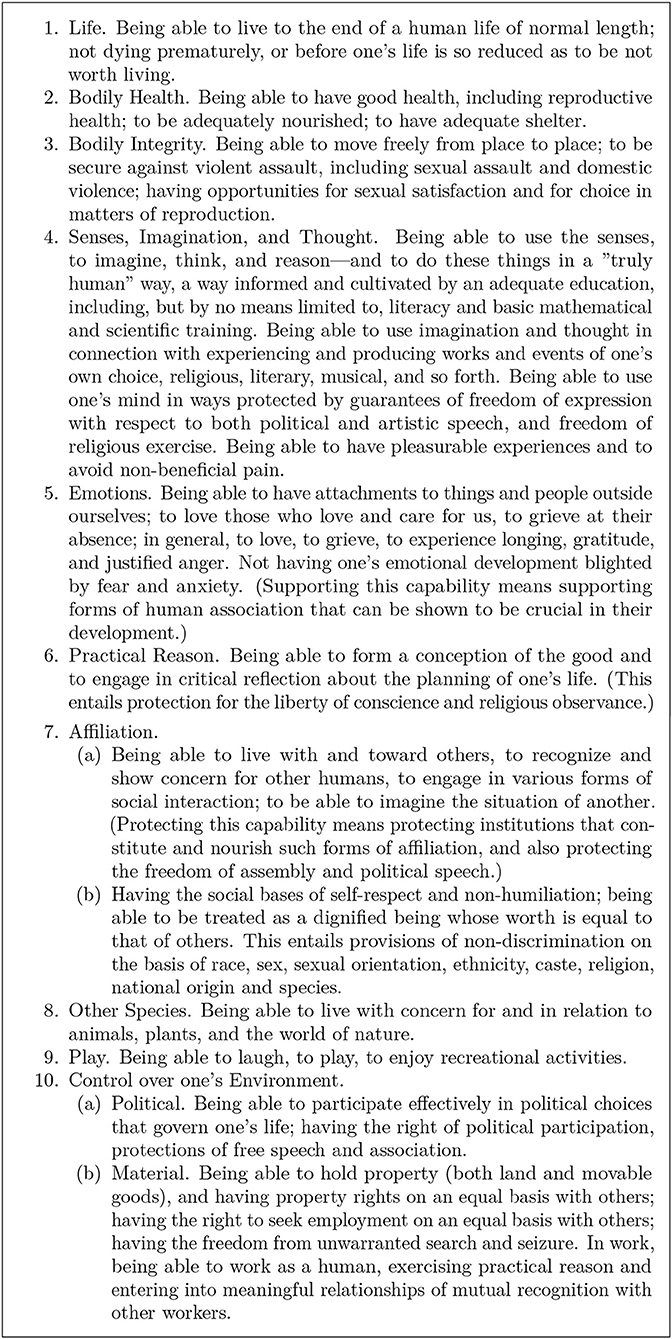
Figure 1. Nussbaum's list of central capabilities (Nussbaum, 2000, 78ff; Nussbaum, 2006, 76ff).
The fact that the central capabilities are easily recognized as important goods would also be useful in research communication contexts. But without a common language, researchers have difficulty explaining their aims to university administrators, external funders, other researchers, and the general public. In addition, the lack of a common language makes it difficult for institutions to recognize, support, and reward the pursuit of outward-facing goals. Nussbaum's list provides a common language that can be used to address these challenges. Legislators might not be in a good position to understand the complex jargon and mixed qualitative-quantitative methods used by geographers, for example; but they should be able to recognize the value of research on the way farmers' pesticide use interacts with political, economic, and ecological inequality (Galt, 2014), which speaks to the central capabilities for adequate nutrition, bodily health, healthy relations with other species, and political and material control over farmer's environments. Similarly, legislators will probably not be familiar with ethnography, but might be interested in research on the ways adult ESL teachers manage classrooms where students have different first languages (Mori, 2014). This research refers to the educational component of “senses, imagination, and thought,” but also affiliation and political control over one's environment. (Note also that, at the time this paper was written, Mori was a graduate student in UC Davis' linguistics program. This shows that, for fields that emphasize single-author publications, student research contributes to an institution's research profile, and can be overlooked if one considers only faculty-authored publications.)
Below, we focus on one specific capability—“adequate shelter”—and consider how research across the academy might contribute to it. We emphasize that there are different ways to understand “adequate shelter,” and that no one conceptualization is obviously correct. The central capabilities give researchers from different disciplines a broader, more flexible, and yet common language for describing the goals of their research compared to wealth-based metrics alone. This flexibility also opens possibilities for interdisciplinary collaboration, as researchers who approach “adequate shelter” in different ways might be able to identify complementary expertise and research questions (Star and Griesemer, 1989; Gorman, 2010).
We argue that this, and other features of the capabilities approach, can help address common concerns that the use of research metrics infringes on academic freedom or intellectual autonomy (Smith et al., 2011; Wilsdon et al., 2015, ch. 7; Statement on “Academic Analytics” Research Metrics1, Holland et al., 2016). We suggest that these concerns are, in part, responses to three limitations of many commonly-used research metrics. First, because research metrics typically measure resources rather than goals, their use can direct attention and incentives toward the acquisition of resources and away from the pursuit of goals (both inward- and outward-facing). Second, because research metrics are typically inward-facing, they similarly direct attention and incentives away from outward-facing activities. The pursuit of outward-facing goals is doubly penalized by these two aspects of research metrics. Third, when outward-facing metrics are used, the list of potential areas of impact can be fixed and limited (e.g., only economic impacts). Researchers who would prefer to pursue other outward-facing goals, not included on the list, may feel that their research is not adequately recognized. That is, researchers may feel like the metrics are imposed on them from above, and that they are required to conform their research practices to the metrics, rather than the metrics being adaptable to cover the full variety of research practices.
However, a sophisticated use of our conceptual framework for outward-facing goals can help address all three concerns, and thereby has the potential to expand, not restrict, academic freedom. First, we emphasize that it is a conceptual mistake to confuse resources and goals. Metrics for resources can be useful, because resources are useful; but resource metrics should not be the primary basis for evaluating research. Second, our framework suggests that, because researchers typically have both inward- and outward-facing goals, both inward- and outward-facing metrics should be used. The list of central capabilities can help researchers communicate the goals of their research, and identify areas where bibliometricians and evaluators need to develop new metrics. Third, the list of central capabilities is explicitly open to revision and extension, in two important respects. First, the listed capabilities are conceptually broad — again, the example we consider below is “adequate shelter.” Nussbaum stresses that the items on the list need specification to be applied in particular contexts (Nussbaum, 2006, pp. 78-9). We show below how different researchers can understand “adequate shelter” in different ways, applying a wide variety of different disciplinary tools and methods to the same central capability.
Second, Nussbaum stresses that the list of central capabilities should not be read as complete or exhaustive (Nussbaum, 2006, p. 78). Researchers in some fields contribute to distinct, generally recognized, socially valuable goods that do not appear in Figure 1. For example, Nussbaum's list does not include infrastructure—plumbing, electricity, internet access—or transportation, or clean water or air. But these are widely recognized as important basic needs, or even human rights (for example, Gleick, 1998). Especially when engaging with researchers in other fields, administrators, funders, and other stakeholders, researchers can use the language of “basic needs” and “human rights” to argue that the outward-facing goals of their research are central capabilities and should be included on the list. Because of its open-ended nature, when the list of central capabilities is used to inform research evaluation activities, evaluators need to work together with researchers to ensure the list includes appropriate capabilities operationalized in appropriate ways. That is, metrics based on the central capabilities need to be adapted to cover the full range of outward-facing goals pursued by researchers in the particular institution (funding portfolio, professional society) being evaluated.
When the central capabilities are used in these ways, we believe that many researchers will find them to be useful for articulating the outward-facing goals that they already have. In this way, we believe that the central capabilities will respect, and even promote, academic freedom.
We turn now to the capability for “adequate shelter.” This capability appears as one component of the second item in Nussbaum's list, “bodily health.” Placing shelter in this way suggests a public health perspective: homelessness increases the risk of injury and disease, and so improving access to housing can reduce these health burdens. This, in turn, suggests a economics-public policy perspective: what are the most efficient ways to improve access to housing, especially affordable housing in high-rent regions such as the San Francisco Bay Area? Specifically, what are the effects of rent control, or housing vouchers, or subsidizing developers on the housing stock?
Housing policy can also be considered from other social science perspectives. A sociologist might examine the social causes and effects of eviction/foreclosure, or the social networks that housing insecure people use to avoid or manage homelessness. Geographers might trace the spatial distribution of gentrification through changes in who owns property and the legacy of redlining. Legal scholarship might focus on the impacts or design of housing discrimination law and the rights of tenants. Humanistic social scientists might study the way home ownership acts as a class marker, or the ritualized interactions between police and homeless people. Similarly, cultural scholars might examine the ideological function of various cultural representations of homelessness, such as the happy-go-lucky hobo, the distraught and pitiful refugee, the lazy bum, and the menacing drifter, and relate these tropes to policy debates.
Alternatively, from an engineering or material science perspective, “adequate shelter” may be seen as a technical challenge, driven in part by climate change: how can we make housing more energy efficient—or better yet, a net producer of energy—while also using less water and more resistant to fire and earthquakes? From this perspective, the most important drivers may be regionally specific. For example, water efficiency, passive cooling, and fire resistance are more important in California; while flood and tornado resistance and efficient heating are more important in Indiana.
“The home” can also be an important site or location for research in various fields. Nursing or clinical medical research might compare home vs. hospital births, or home vs. hospital palliative care. Indoor environmental health researchers might examine home-based exposures to mold and other microbiota, allergens, lead in paint or water, or toxic chemicals from carpet and furniture. Broadening the scale of “adequate shelter” slightly, environmental justice researchers might examine toxic exposures at the neighborhood level, or compare the distribution of pollutants across cities or regions.
Last but certainly not least, architecture and other fields of design treat the home as an aesthetic experience and a vehicle for personal artistic expression. From this perspective, our built environment is something that we create and control, or perhaps even perform; we are not just passively influenced or directed by the home in which we live.
From Capabilities to Metrics
Given that the central capabilities provide a conceptual framework and common language for describing the social aims of research, the next challenge is to translate these capabilities into metrics. What existing data sources might be used to examine contributions to the capabilities across a department, funding program, or university? What new data infrastructure could be developed to provide a richer picture of research in terms of the central capabilities?
In this section, we first show how natural language processing [NLP] methods can be applied to familiar bibliographic data in a capabilities-inspired analysis. Next we consider ways of moving beyond publications, from research outputs to social outcomes. Finally, we discuss some potential uses and limitations of non-public data, including grant proposals and CVs.
(Text) Mining for Capabilities
Bibliometrics has traditionally focused on the citation-based proxies for inward-facing quality or research impact discussed above. However, text mining and NLP methods can be applied to bibliographic data to examine a research portfolio in terms of the central capabilities. In this section, we show how these methods can be used in an exploratory or discovery mode: What outward-facing goals do UC Davis researchers aim to promote with their research? Which of these goals can be characterized in terms of the list of central capabilities? How does the pursuit of these goals vary across different disciplines?
Data and Methods
To explore the use of text mining and NLP to answer these questions, we used the Scopus web interface and API2 to retrieve the text of 6,344 abstracts of scholarly publications by UC Davis-affiliated researchers in 2014.
To associate papers with different research fields, we used Scopus' All Science Journal Classification codes [ASJC], which assign all indexed journals to one or more of 27 different subject areas3. Because of different publishing rates across disciplines, as well as Scopus' emphasis on journals rather than books and quantitative rather than qualitative fields, this list is imbalanced, with many more papers in fields such as medicine and agriculture than in fields such as social science or humanities. We therefore used the ASJC codes to draw a sample of 150 papers from each field, with a total of 2,354 papers. The resulting sample is somewhat more balanced across fields; see Figure 2. However, because several subject areas had fewer than 150 papers, papers can appear in multiple subject areas, and there are more natural science and engineering subject areas than social science and humanities subject areas, the sample still overrepresents work in agriculture, biology, and medicine.
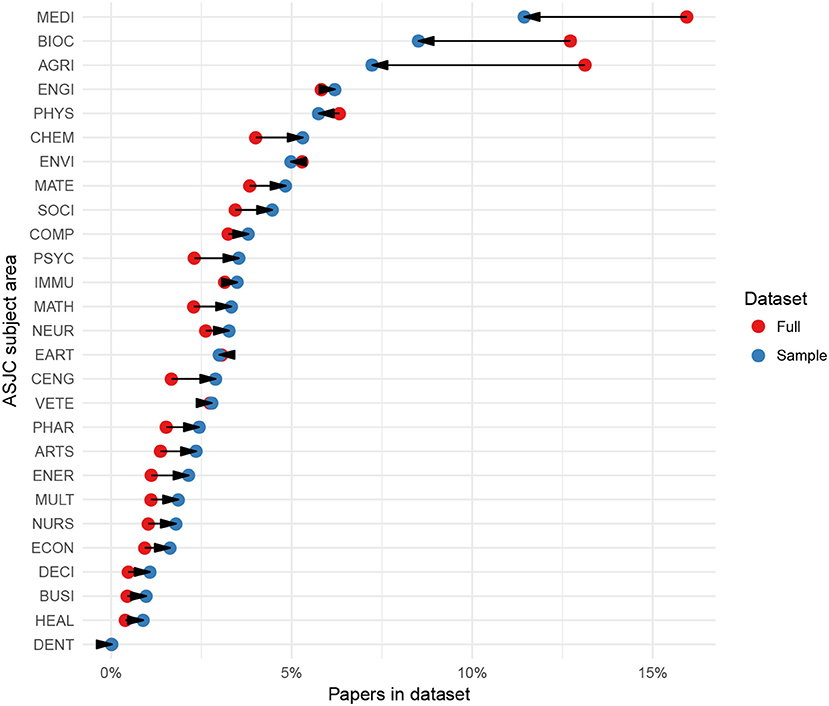
Figure 2. Composition of full and sample datasets for textual analysis. The full dataset (6,344 papers; red points) is dominated by papers in fields such as medical research [MEDI], sub-organismal biology [BIOC], and agriculture [AGRI]. Drawing samples from each subject area separately decreased the fraction of papers from these fields, and modestly increased the fraction of papers from fields such as chemistry [CHEM] and psychology [PSYC] (2,354 papers; blue points). Because a given paper can be classified in more than one subject area, values do not necessarily add to 100%.
A more sophisticated sampling method might incorporate faculty affiliations, sampling across departments rather than subject areas (Wang and Waltman, 2016). However, our exploratory attempts to use this approach produced a large number of false-positive name matches—especially to a few papers in physics and genomics with hundreds of authors. Due to limited resources, for this exploratory analysis we chose to use the simpler ASJC code-based sampling.
We next used NLP methods to extract and cluster 2,000 nouns from these abstracts. This involved three major steps: identifying nouns, constructing word embeddings of the nouns, and arranging the nouns into clusters. First, the Python spaCy NLP package (Arnold, 2017) was used to identify the part-of-speech for each individual word in the abstracts. This allowed us to extract only nouns. Each noun was given a “term frequency-inverse document frequency” (TF-IDF) score, which takes into account both how often the term appears across all abstracts as well whether it appears in many abstracts or just a few. A term like “the” has a high term frequency, but also appears in many documents, and so will have a low TF-IDF score. More specialized terms, such as “water,” appear in fewer documents but are used frequently in those documents, and so have a high TF-IDF score. Specifically, from 16,131 distinct unigram noun lemmas, we selected 2,000 with the highest TF-IDF scores, using each abstract as a distinct document and using counts of unigram noun tokens as document lengths.
We next constructed word embeddings for these 2,000 nouns. Word embeddings represent words in an arbitrary space; nouns that are close together in this space tend to occur in the same abstracts. Specifically, we used the singular value decomposition method described in Levy and Goldberg (2014), calculating positive pairwise mutual information using document-level bag-of-words co-occurrence counts (rather than, e.g., sentence-level skip-gram counts). We selected a 100-dimensional space, to achieve modest dimension reduction across just 2,000 terms.
Finally, the affinity propagation algorithm was used to organize the nouns into clusters (Frey and Dueck, 2007; Bodenhofer et al., 2018). This algorithm starts with randomly-assigned cluster membership, then iteratively refines the clusters to improve similarity. In this case, two nouns are similar to the extent that they are nearby in the word embedding space (high cosine similarity), which corresponds to appearing in the same abstracts. Using the default parameter values, affinity propagation resulted in 176 clusters, with most clusters containing approximately 15 terms. Manual inspection of these noun clusters suggested that they were adequate for exploratory purposes. In particular, 12 clusters appeared to have a notable connection to the central capabilities. Term lists for these selected clusters are given in Figure 3.
Instead of word embeddings and affinity propagation, topic models could also be used to organize nouns into clusters.
Findings and Discussion
Figure 4 shows the 5 most-prevalent terms for each of the 12 selected clusters, with token counts (number of term occurrences) across the paper abstracts. This figure indicates some noise in our simple text analysis—for example, “a” (used as a noun, as in “figure A”) appears in the list for climate change. However, the term lists taken as a whole are highly suggestive of moderately specific research topics.
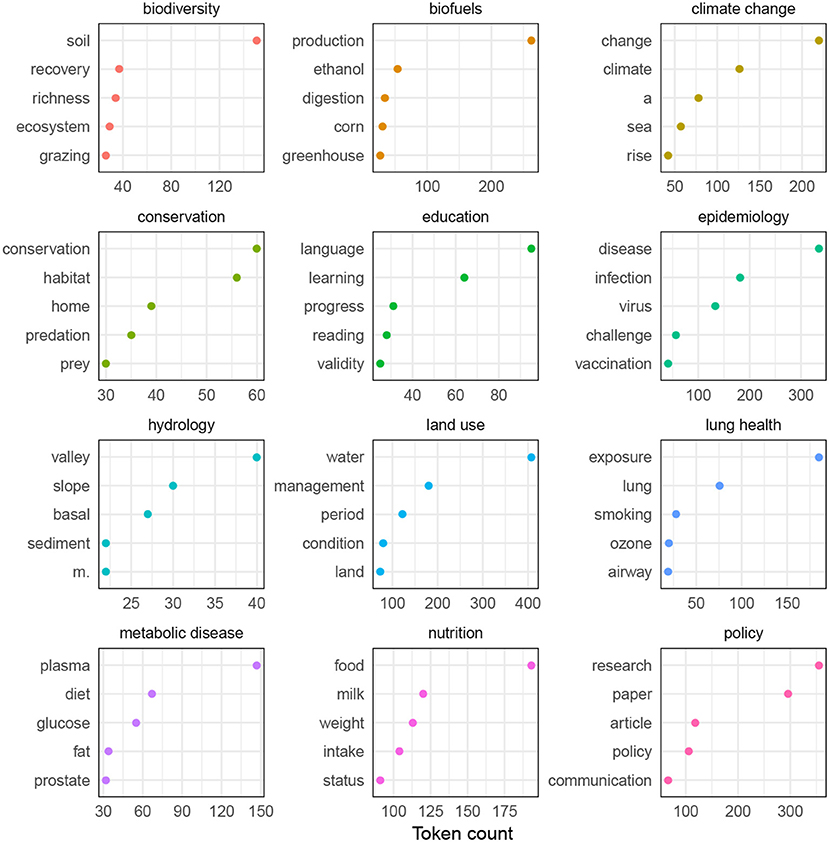
Figure 4. The 5 most-prevalent terms for each selected cluster. Token counts are across the sample dataset. Point colors are not meaningful.
Several of the selected clusters fall under the capabilities of life and bodily health. Notably, these clusters pick out specific areas of biomedical research. There is not one giant cluster for all health research; rather, there are distinct clusters for epidemiology and infectious disease, pulmonary health, metabolic disease, and nutrition. While biomedical researchers in general aim to promote health, specific areas of research address more specific kinds of illness or injury.
Other clusters address environmental concerns. Clusters such as biodiversity, conservation, and land use fall easily under the heading of “other species” (#8 on Nussbaum's list). And still other clusters—including biofuels, climate change, hydrology, and perhaps land use—are likely more anthropocentric, addressing human concerns and interests rather than those of wildlife or natural ecosystems. As discussed above, the list of central capabilities should not be considered complete or comprehensive, and any institution that takes a capabilities-inspired approach should ensure that the list they put into practice reflects the full range of social goals pursued by their researchers.
A few social science clusters appeared in this exploratory analysis. Education in the sense of basic literacy and knowledge falls under the central capability for senses, imagination, and thought (#4 in Figure 1). Higher or more critical education might fit better under the capability for practical reason (#6 in Figure 1), which includes the capability “to form a conception of the good and to engage in critical reflection about the planning of one's life.” Certain areas of policy research might be addressed to the capability for political control over one's environment (#10 in Figure 1), insofar as policy research promotes general engagement in processes of governance. However, many policy researchers focus on the governance of specific issues, e.g., education policy, environmental policy, defense policy. This kind of policy research might be conceived as combining other capabilities with the capability for political control over one's environment.
Figure 5 shows density distributions for the prevalence of cluster terms across different ASJC subject areas, for papers with at least 5 terms from the given cluster and subject areas with at least 3 papers satisfying this condition. This kind of analysis would be useful for examining the way research aims/capabilities are distributed across disciplines or departments. Clusters such as biodiversity, conservation, and lung health appear in only a few fields, at least according to the ASJC taxonomy; while clusters such as land use and nutrition appear in many fields. To some extent, this may be due to noise in the cluster terms; for example, “management” is a prominent term in the land use cluster, but might appear in medical research in contexts such as “pain management” or “disease management.” Further text analysis methods—such as bigram analysis—would help discriminate these different uses of terms. However, even given this noise, the right tails of the distributions indicate fields or disciplines that focus more on the cluster topic. For example, in land use, the longest right tails are found in agriculture, earth science [EART], energy science [ENER], and environmental science [ENVR]. These long tails mean that these fields have papers that use the cluster terms several times, suggesting that these papers are focused on the topic of land use. Similarly, the long tails in climate change, education, and nutrition suggest that these topics may be important research areas in both social science and humanities. A dataset that placed more emphasis on humanities and social science would help bring out topics that are specific to those fields.
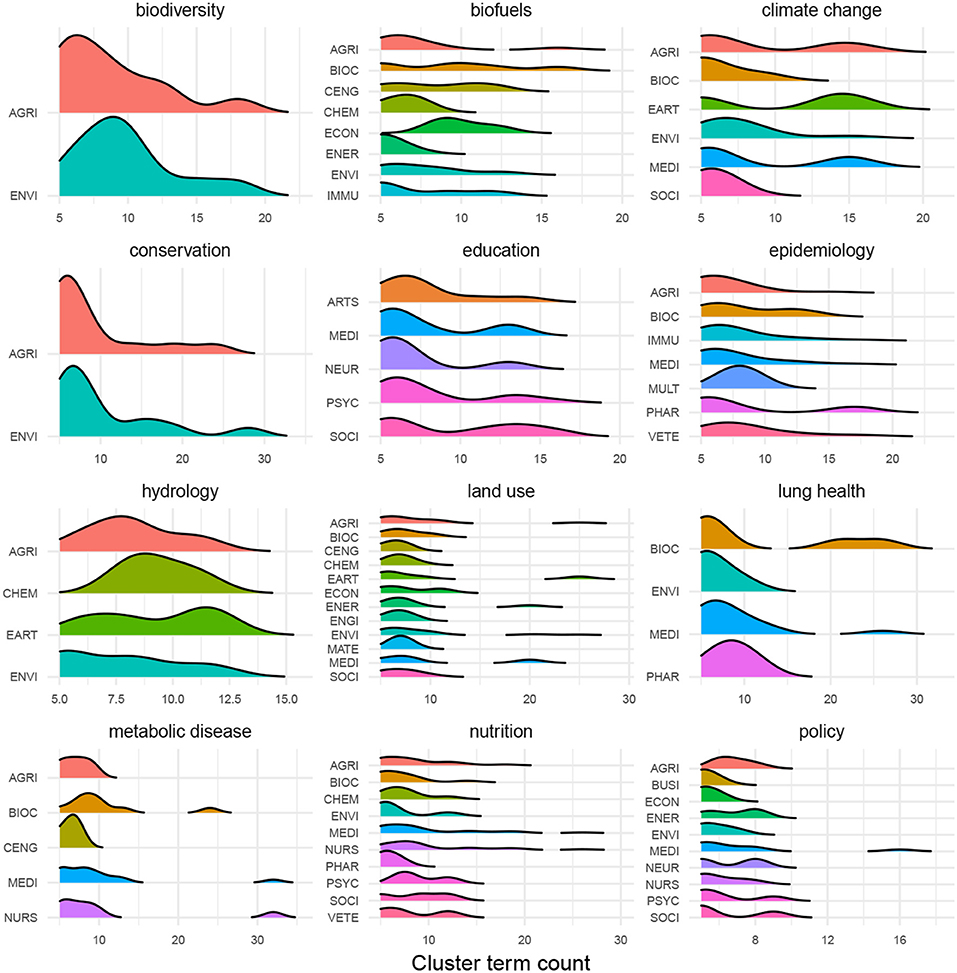
Figure 5. Density distributions for selected cluster term counts by ASJC subject areas. Only papers with at least 5 terms from the given cluster are included; only subject areas with at least 3 papers satisfying this condition are included. Colors correspond to ASJC subject areas across panels. Curve heights relative to gray baselines are comparable within panels, but not between panels.
In every cluster and subject area, the majority of papers contain fewer than 10 instances of the cluster terms; this reflects both the small number of terms in each cluster and the short length of abstracts. Analysis of the full text of papers using a more distributional clustering method—such as topic modeling—might better discriminate papers that focus on a given topic from papers that briefly mention it.
This exploratory analysis suggests that UC Davis researchers do pursue outward-facing goals with their research, and that these goals can be characterized in terms of central capabilities. There are also signs of both disciplinary differences and disciplinary overlap. At least in this dataset, biodiversity appears to be exclusive to biology, while climate research appears in multiple disciplines.
The fact that these clusters can be related to central capabilities supports our view that the capabilities approach would be a useful conceptual framework for activities such as research communication, research evaluation, and portfolio analysis. Researchers are already working “within” various central capabilities; the list in Figure 1 can therefore serve as a common language for characterizing the outward-facing goals of this research.
These text mining methods could also be used under other conceptual frameworks. For example, it might be used to identify the public values associated with research portfolios (Bozeman and Sarewitz, 2005).
Beyond Publications
Scholarly publication by itself will generally do little to promote capabilities. A sociological analysis of the structural causes of homelessness will not reduce homelessness; these findings must be put into practice.
This gap between research and social impact is frequently conceived in terms of communication or dissemination: how do we get research findings from scholars to the public? However, from an evaluation perspective, the question can be framed differently: what are the institutional or organizational links that connect research outputs to medium-term or mid-scale outcomes and longer-term or large-scale impacts (Centers for Disease Control Prevention, 2011, pp. 21–25)? From the evaluator's perspective, research outputs are not limited to scholarly journal articles or books, but also might include public talks, popular writing, testimony to policymakers and in legal settings, consultations, extension programs, writing handbooks or guidelines for use by professionals, and so on. Many of these outputs are more likely to influence medium-term outcomes, and thereby effect social impact, than are research publications. For example, an art historian might reach a thousand people by consulting for a museum on a particular exhibit, but might reach only a few dozen scholars with a book.
Some models of scholarship explicitly connect outputs to outcomes. In community-based participatory research [CBPR] or participatory action research [PAR], research activities are conducted in close partnership with community members, usually with an eye to understanding and redressing significant injustices. Similarly, extension programs are designed to provide professionals — often, but not only, agricultural specialists — with cutting-edge technical expertise. In both cases, the outward-facing goals of research can often be understood in terms of promoting central capabilities.
From a metrics perspective, the challenge is to identify sources of data that encompass the full range of outputs and outcomes. Because Scopus — and similar indexing services, such as Web of Science or Dimensions4 — focus exclusively on academic publications, less conventional data sources are needed.
Internal Sources of Data
We argue that internal data can help identify the variety of outputs that researchers use to pursue different outward-facing goals. Specifically, researchers' curricula vitae [CVs] and grant proposals could be especially valuable for understanding the range of researchers' social engagement activities. Traditionally, and in line with inward-focused incentive structures, academic CVs focus primarily on pedigree (education, faculty appointments) and publication outputs (scholarly journal articles, books, and conference presentations), and only secondarily on other kinds of research output (popular presentations and writing, consultation, community-based research outputs, policy work). But this does not mean that these kinds of outputs are not included. Many universities give (or even require) faculty the opportunity to report “public engagement,” “other scholarly output,” and “service” in tenure and promotion dossiers. Similarly, at funding agencies such as US NSF, annual report forms include space for public talks and broader impacts-type activities. Text mining methods could be applied to these kinds of records, to identify the variety of different kinds of public engagement. In addition, linking research output texts to CV data on public engagement could help us understand how researchers use different outputs to promote different central capabilities.
In “The Goals of Scholarly Research,” we noted that analyses of US NSF's broader impacts criterion find that it is typically operationalized as inward-facing, not outward-facing. However, these same analyses also find that roughly 10% of proposals discuss “other societal benefits,” some of which are likely to be outward-facing. Since 2013, US NSF has required all proposals to include an explicit discussion of anticipated broader impacts in the one-page proposal summary. Text mining methods—similar to those used in the two broader impacts reports that we discussed above—could be applied to these broader impacts statements to survey the variety of broader impacts activities pursued by an institution's researchers—that is, researchers' outputs beyond academic publications.
These kinds of internal data could be even more valuable if researchers had the opportunity to self-classify their work in terms of central capabilities. For example, at the beginning of the tenure or promotion process, simple check-box forms could be used to reveal associations between different disciplines/departments and central capabilities. However, check-box forms are limited to a fixed list of central capabilities. Richer kinds of data collection—such as narratives of researchers' work and accomplishments—when done at scale or over several years, could feed into a more open or reflexive textual analysis. More granular categories of public engagement on reporting forms or templates—distinguishing public talks, citizen science, and community-based participatory research, for example—would also be useful. These kinds of modest changes to data collection systems could support broader initiatives to change incentive structures and improve the cultural balance between inward- and outward-facing scholarly activities.
This is not to say that conceptual and technological developments are sufficient to drive institutional change. Campus politics, faculty suspicion of any use of research metrics, and changing leadership could be important challenges for any attempt to institutionalize the conceptual framework that we have presented here. We suggest that smaller academic units and low-stakes projects might be useful to explore the value of the central capabilities as a framework for evaluating the social impact of research. For example, departments might aggregate social engagement data from their members' CVs and include these prominently in annual reports to the administration. Or research offices might use analyses of broader impacts statements as a tool for “matchmaking” interdisciplinary collaborations. Individual researchers might experiment with the language of the central capabilities in grant applications, public engagement activities, and perhaps even tenure packages. The language of the central capabilities is intended to be accessible; this means that it can be used even when institutions do not formally recognize it as an important conceptual framework for characterizing the goals of research.
Conclusion
The Capabilities Approach complements conventional, economics-based approaches to the social impacts of research. The Capabilities Approach directs our attention to the specific outward-facing goals of scholarship, and is relevant even to fields that do not produce patentable or otherwise marketable technology. Nussbaum's list of central capabilities provides a concrete and specific starting place for characterizing the outward-facing goals of scholarship. The flexibility of Nussbaum's list means that scholars in different disciplines can recognize common goals even across different methods and conceptual frameworks. Text mining methods, applied to both existing and future data, can be used to discover capabilities-relevant work across the disciplines and the variety of forms of public engagement. Because the list is open-ended, scholars of topics that don't fit easily in the list as it stands—such as water and transportation—can and should be encouraged to articulate central capabilities that better characterize their work.
Author Contributions
DH conceived the overall approach of the essay in discussion with MS and CS. DH prepared the first draft of the manuscript. All authors contributed revisions, reviewed the final version for submission, and approved the submitted version.
Funding
DH's postdoctoral fellowship is funded by a grant from Elsevier.
Conflict of Interest Statement
The authors declare that the research was conducted in the absence of any commercial or financial relationships that could be construed as a potential conflict of interest.
Footnotes
1. ^Available online at: https://www.aaup.org/news/statement-urges-caution-toward-academic-analytics.
2. ^Available online at: https://www.scopus.com.
3. ^What Are Scopus What Are Scopus Subject Area Categories and ASJC Codes? (2018). Available online at: https://service.elsevier.com/app/answers/detail/a_id/12007/supporthub/scopus/.
4. ^Available online at: https://www.dimensions.ai/.
References
Alkon, A. H., and Mares, T.M. (2012). “Food Sovereignty in US food movements: radical visions and neoliberal constraints,” in Agriculture and Human Values. Available online at: http://www.springerlink.com/index/10.1007/s10460-012-9356-z
Anand, S., and Sen, A. (1994). Human Development Index: Methodology and Measurement. United Nations Human Development Report Office.
Aristotle (2014). “Nicomachean ethics,” in The Complete Works of Aristotle, ed J. Barnes (Princeton, NJ: Princeton University Press).
Arnold, T. (2017). A tidy data model for natural language processing using clean NLP. arXiv[Preprint]arXiv: 1703.09570 [cs, stat].
Bodenhofer, U., Palme, J., Melkonian, C., Kothmeier, A., and Kostic, N. (2018). Apcluster: Affinity Propagation Clustering. Version 1.4.5. Available online: https://CRAN.R-project.org/package=apcluster
Boyack, K.W., van Eck, N.J., Colavizza, G., and Waltman, L. (2018). Characterizing in-text citations in scientific articles: a large-scale analysis. J. Inform. 12, 59–73. doi: 10.1016/j.joi.2017.11.005
Bozeman, B. (2007). Public Values and Public Interest: Counterbalancing Economic Individualism. Public Management and Change Series. OCLC: ocm84903820. Washington, DC: Georgetown University Press, 214.
Bozeman, B., and Sarewitz, D. (2005). Public values and public failure in US science policy. Sci. Public Policy 32, 119–136. doi: 10.3152/147154305781779588
Bozeman, B., and Sarewitz, D. (2011). Public value mapping and science policy evaluation. Minerva 49, 1–23. doi: 10.1007/s11024-011-9161-7
Bozeman, B. and Youtie, J. (2017). Socio-economic impacts and public value of government-funded research: lessons from Four US National Science Foundation Initiatives. Res. Policy 46, 1387–1398. doi: 10.1016/j.respol.2017.06.003
Brandt, M. (2014). Zapatista corn: a case study in biocultural innovation. Soc. Stud. Sci. 44, 874–900. doi: 10.1177/0306312714540060
Bush, V. (1945–1960). Science, the Endless Frontier. National Science Foundation, 220. Available online at: http://books.google.com/books?id=KLuKnQEACAAJ&dq=intitle:science+the+endless+frontier&hl=&cd=4&source=gbs_api.
Centers for Disease Control Prevention (2011). Introduction to Program Evaluation for Public Health Programs: A Self-Study Guide. Available online at: https://www.cdc.gov/eval/guide/cdcevalmanual.pdf
Fealing, K. H. Lane, J. I. King, J., and Johnson, S. (2017). Measuring the Economic Value of Research: The Case of Food Safety. Cambridge University Press, 209.
Frey, B. J., and Dueck, D. (2007). Clustering by passing messages between data points. Science 315, 972–976. doi: 10.1126/science.1136800
Galt, R. E. (2014). Food Systems in an Unequal World: Pesticides, Vegetables, and Agrarian Capitalism in Costa Rica. Tucson: University of Arizona Press, 305.
Garfield, E. (1979). Citation Indexing: Its Theory and Application in Science, Technology, and Humanities. New York, NY: Isi Press, 304.
Ghiasi, G., Larivière, V., and Sugimoto, C. R. (2015). On the compliance of women engineers with a gendered scientific system. PLoS ONE 10:e0145931. doi: 10.1371/journal.pone.0145931
Gleick, P. H. (1998). The human right to water. Water Policy 1, 487–503. doi: 10.1016/S1366-7017(99)00008-2
Gorman, M. (2010). “Introduction: trading zones, interactional expertise, and collaboration,” in Trading Zones and Interactional Expertise, ed M. Gorman (Cambridge, MA; London: MIT Press), 1–4.
Hicks, D., and Melkers, J. (2012). “Bibliometrics as a Tool for Research Evaluation,” in Handbook on the Theory and Practice of Program Evaluation. ed A. Link and N. Vornatas (Cheltenham, UK; Northampton, MA: Edward Elgar), 323–349.
Hicks, D. J., and Stapleford, T. A. (2016). The virtues of scientific practice: macIntyre, virtue ethics, and the historiography of science. J. Hist. Sci. Soc. 107, 449–472. doi: 10.1086/688346
Holbrook, J. B. (2012). “Re-assessing the science–society relation: the case of the US National Science Foundation's Broader Impacts Merit Review Criterion (1997–2011),” in Peer Review, Research Integrity, and the Governance of Science. Beijing: People's Publishing House. Available online at: http://digital.library.unt.edu/ark:/67531/metadc77119/
Holland, C., Lorenzi, F., and Hall, T. (2016). Performance anxiety in academia: tensions within research assessment exercises in an age of austerity. Policy Futures Educ. 14, 1101–1116. doi: 10.1177/1478210316664263
Hutchins, B.I., Yuan, X., Anderson, J.M., and Santangelo, G.M. (2016). Relative citation ratio (RCR): a new metric that uses citation rates to measure influence at the Article Level. PLoS Biol. 14:e1002541. doi: 10.1371/journal.pbio.1002541
Hutchins, B. I., Hoppe, T. A., Meseroll, R. A., Anderson, J. M., and Santangelo, G. M. (2017). Additional support for RCR: a validated article-level measure of scientific influence. PLoS Biol. 15:e2003552. doi: 10.1371/journal.pbio.2003552
Intemann, K. (2009). Why diversity matters: understanding and applying the diversity component of the National Science Foundation's Broader Impacts Criterion. Soc. Epistemol. 23, 249–266. doi: 10.1080/02691720903364134
Janssens, A.C.J.W., Goodman, M., Powell, K.R., and Gwinn, M. (2017). A critical evaluation of the algorithm behind the relative citation ratio (RCR). PLoS Biol. 15:e2002536. doi: 10.1371/journal.pbio.2002536
Kibel, M., and Vanstone, M. (2017). Reconciling ethical and economic conceptions of value in health policy using the capabilities approach: a qualitative investigation of non-invasive prenatal testing. Soc. Sci. Med. 195, 97–104. doi: 10.1016/j.socscimed.2017.11.024
Larivière, V., Ni, C., Gingras, Y., Cronin, B., and Sugimoto, C. R. (2013). Bibliometrics: global gender disparities in science. Nat. News 504, 211–213. doi: 10.1038/504211a
Latour, B., and Woolgar, S. (1979). Laboratory Life: The Construction of Scientific Facts. Available online at: http://books.google.com/books?hl=en&lr=&id=XTcjm0flPdYC&oi=fnd&pg=PA7&dq=latour+(woolgar+laboratory+life)&ots=Vofpg3Cse3&sig=kfyLYBFDQ0iikV2X3fKKtMmiHu4
Laurance, W. F., Useche, D. C., Laurance, S.G., and Bradshaw, C.J. A. (2013). Predicting publication success for biologists. BioScience 63, 817–823. doi: 10.1525/bio.2013.63.10.9
Levelt Committee, Noort Committee, Drenth. (2012). Flawed Science: The Fraudulent Research Practices of Social Psychologist Diederik Stapel. Tilburg: Tilburg University. Available online at: https://www.tilburguniversity.edu/upload/3ff904d7-547b-40ae-85fe-bea38e05a34a_Final%20report%20Flawed%20Science.pdf
Levy, O., and Goldberg, Y. (2014). Neural word embedding as implicit matrix factorization. Adv. Neural Inform. Proc. Syst. 2, 2177–2185. Available online at: http://papers.nips.cc/paper/5477-neural-word-embedding-as-implicit-matrix-factorization (Accessed August 16, 2018).
Li, D., Azoulay, P., and Sampat, B. N. (2017). The applied value of public investments in biomedical research. Science 356, 78–81. doi: 10.1126/science.aal0010. pmid: 28360137
Maliniak, D., Powers, R., and Walter, B. F. (2013). The gender citation gap in international relations. Int. Organ. 67, 889–922. doi: 10.1017/S0020818313000209
Mihaljević-Brandt, H., Santamaría, L., and Tullney, M. (2016). The Effect of Gender in the Publication Patterns in Mathematics. PLoS ONE 11:e0165367. doi: 10.1371/journal.pone.0165367
Mori, M. (2014). Conflicting ideologies and language policy in adult ESL: complexities of language socialization in a majority-L1 classroom. J. Lang. Identity Educ. 13, 153–170. doi: 10.1080/15348458.2014.919810
Mormina, M. (2018). Science, technology and innovation as social goods for development: rethinking research capacity building from Sen's capabilities approach. Sci. Eng. Ethics. doi: 10.1007/s11948-018-0037-1. [Epub ahead of print].
Narin, F., Hamilton, K.S., and Olivastro, D. (1997). The increasing linkage between U.S. technology and public science. Res. Policy 26, 317–330. doi: 10.1016/S0048-7333(97)00013-9
National Science Board (2011). National Science Foundation's Merit Review Criteria: Review and Revisions. NSB/MR-11–22. Available online at: https://nsf.gov/nsb/publications/2011/nsb1211.pdf
National Science Foundation (2018). Proposal & Award Policies & Procedures Guide. NSF 18-1. Available online at: https://www.nsf.gov/pubs/policydocs/pappg18_1/pappg_3.jsp#IIIA2
Nussbaum, M. C. (2000). Women and Human Development: The Capabilities Approach. Cambridge; New York, NY: Cambridge University Press.
Nussbaum, M. C. (2006). Frontiers of Justice: Disability, Nationality, Species Membership. The Tanner lectures on human values. Cambridge, MA: The Belknap Press; Harvard University Press, 487.
Radicchi, F., Fortunato, S., and Castellano, C. (2008). Universality of citation distributions: toward an objective measure of scientific impact. Proc. Natl. Acad. Sci. U.S.A. 105, 17268–17272. doi: 10.1073/pnas.0806977105. pmid: 18978030
Raymond, C., Singh, G.G., Benessaiah, K., Bernhardt, J. R., Levine, J., Nelson, H., et al. (2013). Ecosystem services and beyond. BioScience 63, 536–546. doi: 10.1525/bio.2013.63.7.7. JSTOR: info/10. 1525/bio.2013.63.7.7
Sánchez-Gil, S., Gorraiz, J., and Melero-Fuentes, D. (2018). Reference density trends in the major disciplines. J. Inform. 12, 42–58. doi: 10.1016/j.joi.2017.11.003
Schienke, E. W. Brown, D.A., Davis, K.J., Keller, K., Shortle, J.S., Stickler, M. et al. (2009). The role of the National Science Foundation broader impacts criterion in enhancing research ethics pedagogy. Soc. Epistemol. 23, 317–336. doi: 10.1080/02691720903364282
Smith, S., Ward, V., and House, A. (2011). Impact' in the proposals for the UK's research excellence framework: shifting the boundaries of Academic Autonomy. Res. Policy 40, 1369–1379. doi: 10.1016/j.respol.2011.05.026
Soulé, M. E. (1985). What is conservation biology? BioScience 35, 727–734. doi: 10.2307/1310054. JSTOR: 1310054
Staley, K. (2017). “Decisions, decisions: inductive risk and the Higgs boson,” in Exploring Inductive Risk: Case Studies of Values in Science, eds K. Elliott and T. Richards (New York, NY: Oxford University Press), 37–58.
Star, S. L., and Griesemer, J. R. (1989). Institutional ecology, ‘Trans- lations' and Boundary Objects: Amateurs and Professionals in Berkeley's Museum of Vertebrate Zoology, 1907-39. Soc. Stud. Sci. 19, 387–420. doi: 10.1177/030631289019003001
Stiglitz, J., Sen, A., and Fitoussi, J.-P. (2009). Report of the Commission on the Measurement of Economic Performance and Social Progress. 1–292.
Thonon, F., Boulkedid, R., Delory, T., Rousseau, S., Saghatchian, M., van Harten, W., et al. (2015). Measuring the outcome of biomedical research: a systematic literature review. PLoS ONE 10:e0122239. doi: 10.1371/journal.pone.0122239
Toole, A. A. (2012). The impact of public basic research on industrial innovation: evidence from the pharmaceutical industry. Res. Policy 41, 1–12. doi: 10.1016/j.respol.2011.06.004
United Nations Development Programme (1990). Human Development Report 1990. United Nations Development Programme. Available online at: http://hdr.undp.org/en/reports/global/hdr1990
Van der Zee, T. (2017). The Wansink Dossier: An Overview. Available online at: http://www.timvanderzee.com/the-wansink-dossier-an-overview/
Waltman, L., van Eck, N. J., and van Raan, A. F. J. (2012). Universality of citation distributions revisited. J. Am. Soc. Inform. Sci. Technol. 63, 72–77. doi: 10.1002/asi.21671
Wang, J., Veugelers, R., and Stephan, P. (2017). Bias against novelty in science: a cautionary tale for users of bibliometric indicators. Res. Policy 46, 1416–1436. doi: 10.1016/j.respol.2017.06.006
Wang, Q., and Waltman, L. (2016). Large-scale analysis of the accuracy of the journal classification systems of web of science and scopus. J. Inform. 10, 347–364. doi: 10.1016/j.joi.2016.02.003
Wansink, B. (2017). Archive of “The Grad Student Who Never Said ‘No.”' Available online at: https://web.archive.org/web/20170312041524/http:/www.brianwansink.com/phd-advice/the-grad-student-who-never-said-no
Watts, S. M., George, M.D., and Levey, D.J. (2015). Achieving broader impacts in the national science foundation, division of environmental biology. BioScience 65, 397–407. doi: 10.1093/biosci/biv006
Wilsdon, J., Curry, S., Jones, R., Kerridge, S., Tinkler, J., Wouterset, P., et al. (2015). The Metric Tide: Report of the Independent Review of the Role of Metrics in Research Assessment and Management. Higher Education Funding Council for England. Available online at: http://www.hefce.ac.uk/pubs/rereports/Year/2015/metrictide/ (visited on 05/24/2018)
Keywords: social impact of research, broader impacts, capabilities approach, natural language processing, research portfolio analysis
Citation: Hicks DJ, Stahmer C and Smith M (2018) Impacting Capabilities: A Conceptual Framework for the Social Value of Research. Front. Res. Metr. Anal. 3:24. doi: 10.3389/frma.2018.00024
Received: 02 April 2018; Accepted: 06 August 2018;
Published: 28 August 2018.
Edited by:
George Chacko, NET eSolutions Corporation (NETE), United StatesReviewed by:
James Britt Holbrook, New Jersey Institute of Technology, United StatesBhaven Sampat, Columbia University, United States
Copyright © 2018 Hicks, Stahmer and Smith. This is an open-access article distributed under the terms of the Creative Commons Attribution License (CC BY). The use, distribution or reproduction in other forums is permitted, provided the original author(s) and the copyright owner(s) are credited and that the original publication in this journal is cited, in accordance with accepted academic practice. No use, distribution or reproduction is permitted which does not comply with these terms.
*Correspondence: Daniel J. Hicks, ZGpoaWNrc0B1Y2RhdmlzLmVkdQ==