- Department of Computational Media, Jack Baskin School of Engineering, University of California, Santa Cruz, Santa Cruz, CA, United States
Physical rehabilitation is often an intensive process that presents many challenges, including a lack of engagement, accessibility, and personalization. Immersive media systems enhanced with physical and emotional intelligence can address these challenges. This review paper links immersive virtual reality with the concepts of therapy, human behavior, and biofeedback to provide a high-level overview of health applications with a particular emphasis on physical rehabilitation. We examine each of these crucial areas by reviewing some of the most influential published case studies and theories while also considering their limitations. Lastly, we bridge our review by proposing a theoretical framework for future systems that utilizes various synergies between each of these fields.
1. Introduction
In 1968, Ivan Sutherland, one of the godfathers of computer graphics, demonstrated the first head-mounted display (HMD) immersive media system to the world: an immersive Virtual Reality (iVR) headset that enabled users to interactively gaze into a three dimensional (3D) virtual environment (Sutherland, 1968; Frenkel, 1989; Steinicke, 2016). Three years before the “Sword of Damocles,” Sutherland described his inspiration for the system in what became one of the most influential essays of immersive media: “The ultimate display would, of course, be a room within which the computer can control the existence of matter. A chair displayed in such a room would be good enough to sit in. Handcuffs displayed in such a room would be confining, and a bullet displayed in such a room would be fatal. With appropriate programming, such a display could literally be the Wonderland into which Alice walked” (Sutherland, 1965). Morbidness aside, this vision of an ultimate display asks if it is possible to create such a computationally adept medium that reality itself could be simulated with physical response. Sutherland's “Sword of Damocles” helped spark a new age of research aimed at answering this question for both academia and industry in the race to build the most immersive displays for interaction within the virtual world (Costello, 1997; Steinicke, 2016). However, this trend was short-lived due to hardware constraints and costs at the time (Costello, 1997).
The past decade has seen explosive growth in this field, with increases in computational power and affordability of digital systems effectively reducing barriers to technological manufacturing, consumer markets, required skills, and organizational needs (Westwood, 2002). In 2019, seven million commercial HMDs were sold and with sales projected to reach 30 million per year by 2023 (Statista, 2020). This mass consumer adoption has partly been due to a decrease in hardware cost and a corresponding increase in usability. These commercial systems provide a method for conveying 6-DoF information (position and rotation), while also learning from user behavior and movement. From these observations, we argue that the integration of iVR as a medium for guided physical healthcare may offer a cost-effective and more computationally adept option for exercise.
Reflecting back to Sutherland's vision of an ultimate display, we ask: what would be the ultimate iVR system for physical rehabilitation? If the sensation of physical reality can be simulated through computation, how might that reality best help the user with exercises and physical rehabilitation? From these questions, we posit that Sutherland's vision of the Ultimate Display requires augmentation to address a key area for healthcare: an intelligent perspective of how to best assist a user. In this paper, we explore these questions by reviewing immersive virtual reality as it intersects the fields of therapy, human behavior, and biofeedback. Immersive media affords a medium for enhancing the therapy and healthcare process. It establishes a mode for understanding human behavior, simulating perception, and providing physical assistance. Biofeedback provides a methodology for evaluating emotional response, a crucial element of mental health that is not often explored in healthcare. Given these key points, the rest of this introduction describes our motivation and goals in undertaking this study.
1.1. A Need for a More Efficacious Healthcare Medium With Physical Therapy
Physical inactivity leads to a decline of health, with significant motor degradation, a loss of coordination, movement speed, gait, balance, muscle mass, and cognition (Howden and Meyer, 2011; Sandler, 2012; Centers for Disease Control and Prevention, 2019). In contrast, the medical benefits of regular physical activity include prevention of motor degradation, stimulation of weight management, and reduction of the risk of heart disease and certain cancers (Pearce, 2008). While traditional rehabilitation has its merits, compliance in performing physical therapy may be limited due to high costs, lack of accessibility, and low education (Campbell et al., 2001; Burdea, 2003; Jack et al., 2010; Mousavi Hondori and Khademi, 2014; Centers for Disease Control and Prevention, 2019). These exercises also usually lack positive feedback, which is critical in improving compliance with physical therapy protocol (Sluijs et al., 1993). Taking these issues into consideration, some higher-tech initiatives associated with telemedicine, virtual reality, and robotics programs have been found to be more effective in promoting compliance than traditional paper-based and verbal instructions (Deutsch et al., 2007; Byl et al., 2013; Mellecker and McManus, 2014). These higher-tech exercise programs often use sensors to passively monitor a patient's status or to provide feedback so the action can be modified. They may also use actuators to assist the patient in completing the motion (Lindeman et al., 2006; Bamberg et al., 2008). Thus, technology may enable a patient to better follow their physical therapy program, aiding independent recovery and building on the progress made with the therapist. This raises the question of whether virtual environments in the form of iVR might be a suitable technology to address these issues.
Immersive virtual environments and the recent uptake of serious games have immense potential for addressing these issues. The ability to create stimulating programmable immersive environments has been shown to increase therapy compliance, accessibility, and data throughput (Mousavi Hondori and Khademi, 2014; Corbetta et al., 2015). Considerable success has been reported in using virtual environments for therapeutic intervention between psychological and physiological research. However, these systems have been mostly constrained due to cost and hardware limitations (Costello, 1997). For example, early 2000s head-mounted display systems had significant hardware constraints, such as low resolution and low refreshment rates, which led to non-realistic and non-immersive experiences that induced motion sickness (LaViola, 2000). Therefore, at that time, the potential use of immersive displays as a rehabilitation tool was quite limited.
These challenges are no longer as prevalent today: modern iVR systems have advanced technically and can now enhance user immersion through widening the field of view, increasing frame rate, leveraging low-latency motion capture, and providing realistic surround sound. These mediums are becoming ever more mobile and are now a part of the average consumer's entertainment experience (Beccue and Wheelock, 2016). As a result, we argue that now is the right time to consider these display mediums as a possible means of addressing the need for effective, cost-effective healthcare. It may be possible that for iVR to be used as a vehicle to augment healthcare to assist users in recovery by transforming the “fixing people” mentality (Seligman et al., 2002) of traditional rehabilitation into adventures in the virtual world that provide both meaningful enjoyable experiences and restorative exercise.
1.2. Review Goals
The goal of this paper is to survey the theory, application, and methodology of influential works in the field of immersive media for the purpose of exploring opportunities toward future research, with the ultimate aim of applying these technologies to engage physical rehabilitation. The subsequent sections of this paper provide a discussion of the following topics:
• the current state of academic research in utilizing iVR for physical rehabilitation and health;
• the behavioral theory behind the success of utilizing iVR;
• the applications of biofeedback and incorporating runtime user analysis in virtual environments;
• and bridging potential synergies between each of these areas toward applying them for future research.
This work will provide an overview of iVR for physical rehabilitation and health through an understanding of both past and current academic projects. We aim to provide an informative view on each of our goals as well as offering suggestions for how these concepts may be used to work toward an ultimate display of physical rehabilitation. We believe that this work will be of interest to interdisciplinary researchers at the intersection of immersive media, affective computing, and healthcare intervention.
1.3. Scope and Limitations
The term VR was coined long before the advent of recent immersive virtual reality (iVR) systems. This has led to differences in how the term “VR” is applied, and these differences can be seen within the existing literature. For the purposes of this review, we define niVR as non-immersive systems that utilize a monitor and allow user interaction through conventional means such as keyboards, mice, or custom controllers (Costello, 1997). VR systems that provide a head-mounted display (HMD) with a binocular omni-orientation monitor, along with appropriate three-dimensional spatialized sound, are categorized as iVR. Augmented reality (AR) systems employ virtual feedback by allowing the user to see themselves and their surroundings projected virtually onto a screen, usually in a mirror-like fashion (Assis et al., 2016). These systems are similar in how they present movement-based tasks with supplementary visual and auditory feedback, but differ in their interaction methods (Levac et al., 2016).
Our review focuses on iVR systems for physical rehabilitation, health, and games for health. We examine high-impact case studies, meta-reviews, and position papers from academia with an emphasis on research conducted in the past two decades. This paper provides a high-level overview of each of these areas and their implications for healthcare. However, we must acknowledge that immersive media, and many of the other concepts described in this paper, are rapidly changing fields. Many of the academic work and positions discussed in this paper are likely to change in the future as technology advances. With these considerations in mind, this paper provides a snapshot of these research areas from past to present and derives limitations and challenges from such to infer the need for future research in advancing an ultimate display for physical rehabilitation. We start by examining iVR for healthcare and rehabilitation.
2. Immersive Virtual Reality and Therapy
In the past two decades, there have been many publications and studies focusing on VR technologies for application in psychotherapy, physiotherapy, and telerehabilitation. Modern iVR technology is commonly known for its impact on enhancing the video gaming paradigm by deepening user involvement and leading to more dedicated interaction (Baldominos et al., 2015). The increased physical demands of these video gaming platforms have garnered interest for their potential in therapy through repetitive and quantifiable learning protocols (Salem et al., 2012). Early research suggests that the use of iVR systems is useful for psychological, physical, and telepresence therapy (Kandalaft et al., 2013; Straudi et al., 2017).
2.1. Psychological Therapy Applications
Psychological research has seen an increase in the use of iVR due to its ability to simulate realistic and complex situations that are critical to the success of laboratory-based human behavior investigations (Freeman et al., 2017). Some of these investigations include the successful reduction of pain through the use of stimuli in iVR. This has shown results equivalent to the effects of a powerful analgesic treatment, such as morphine, for burn victim wound treatment (Hoffman et al., 2011; Gromala et al., 2015). With the immersive capabilities of modern headsets, such as the HTC Vive and Oculus Rift, there has been an increase in studies reporting positive outcomes of iVR exposure therapies for post-traumatic stress disorder (Rothbaum et al., 2014; Morina et al., 2015), borderline personality disorder (Nararro-Haro et al., 2016), phobias (Grillon et al., 2006; Shiban et al., 2015), and schizophrenia (Rus-Calafell et al., 2014), as well as many other psychological therapies. This accelerated iVR use in psychological therapy is often attributed to the relationship between increased presence and emotion (Diemer et al., 2015; Morina et al., 2015). Increasing the number of meaningful stimuli that resonate with the users' engagement using iVR is a crucial factor in influencing user behavior and experience (Baños et al., 2004), and, with the price of computing devices and hardware decreasing, headsets are becoming more popular and immersive in doing so (Beccue and Wheelock, 2016; Statista, 2020). Thus, immersion through iVR can lead to greater emotional influence on the user and can incite the desired physiological responses by crafting a stimulating and engaging virtual environment (Chittaro et al., 2017). While this work shows great promise, the psychological application of iVR is still largely underdeveloped and lacking in terms of proven beneficial results. Similar results and benefits can also be seen with physical therapy interventions utilizing iVR.
2.2. Physiological Therapy Applications
Traditional forms of physical therapy and rehabilitation are based on therapist observation and judgment; this process can be inaccurate, expensive, and non-timely (Mousavi Hondori and Khademi, 2014). Many studies have indicated that iVR can be an effective tool in improving outcomes compared to conventional physical therapy (Lohse et al., 2014). Environments can be tailored to cue specific movements in real-time through sensory feedback via the vestibular system and mirror imagery to exemplify desired ranges of motion (Iruthayarajah et al., 2017). With the emergence of new immersive multimedia, iVR experiences with sight, sound, and touch can be integrated into rehabilitation. Studies have indicated that iVR intervention is useful in improving a variety of motor impairments, such as hemiparesis caused by Parkinson's disease, multiple sclerosis, cerebral palsy, and stroke (Iruthayarajah et al., 2017).
High repetitions of task-oriented exercises are critical for locomotive recovery, and user adherence to therapy protocol is imperative. iVR-based physical rehabilitation can induce adherence to therapy protocol as successfully as (and sometimes better than) human-supervised protocol due to the capabilities of multi-sensory real-time feedback (Corbetta et al., 2015). Games can be used to guide the user in their movements and provide mechanics to reward optimal exercises (Corbetta et al., 2015). Additionally, this multi-sensory, auditory, and visual feedback can further persuade users to exercise harder through increased stimuli. iVR-based physical rehabilitation also allows for increased quantitative feedback for both the user and the therapist. The capacity of modern iVR systems to implement three-dimensional motion tracking serves as an effective way to monitor progress during rehabilitation, allowing healthcare professionals to obtain a more in-depth view of each user's independent recovery (Baldominos et al., 2015).
Multiple reviews have been conducted consisting of hundreds of studies through the past decade, and have concluded that niVR is useful for motor rehabilitation (Cameirão et al., 2008; Saposnik et al., 2011; Mousavi Hondori and Khademi, 2014). Many of these studies have confirmed that the use of iVR results in significant improvements when compared to traditional forms of therapy (Corbetta et al., 2015; Iruthayarajah et al., 2017). These studies used Kinect, Nintendo Wii, IREX: Immersive Rehabilitation Exercise, Playstation EyeToy, and CAVE, as well as custom-designed systems. For a given treatment time, the majority of these studies suggested that video game-based rehabilitation is more effective than standard rehabilitation (Cruz-Neira et al., 1993; Lohse et al., 2014; Corbetta et al., 2015; Iruthayarajah et al., 2017). Subsequently, the physical rehabilitation communities have been enthusiastic about the potential to use gaming to motivate post-stroke individuals to perform intensive repetitive task-based therapy. Some games can combine motion capture as a way to track therapy adherence and progress. Despite these promising studies, technology at the time needed to improve in terms motion-tracking accuracy in order to become more effective, reliable, and accessible (Crosbie et al., 2007; Mousavi Hondori and Khademi, 2014). The existing research indicates that more work is needed to continue gaining a deeper understanding of the efficacy of iVR in rehabilitation (Cameirão et al., 2008; Dascal et al., 2017). These modern iVR headsets open up new opportunities for accessibility and affordability of treatment.
2.3. Telerehabilitation Applications
Telerehabilitation approaches provide decreased treatment cost, increased access for patients, and more quantifiable data for therapists (Lum et al., 2006). There have been various studies confirming the technical feasibility of in-home telerehabilitation, as well as an increase in the efficiency of these services (Kairy et al., 2013). In these studies, users generally achieve more significant results in rehabilitation due to the increased feedback from the telerehabilitation VR experience (Piron et al., 2009). Due to the mobile and computational nature of VR displays, these iVR telerehabilitation studies suggests that the usability and motivation of the rehabilitation treatment for the user can be sustained while reducing work for therapists and costs for patients (Lloréns et al., 2015).
2.4. Limitations of Current Studies for iVR Rehabilitation
While iVR has shown great promise from these studies, we must establish whether these HMDs and immersive displays are a truly beneficial medium. The cost of HMDs is reducing and commercial adoption is prevalent (Beccue and Wheelock, 2016). However, research into the effectiveness of iVR as a medium for rehabilitation is still inconsistent and is not often verified for reproducibility. An unfortunate commonality between these studies lies in a lack of reporting methodology, small or non-generalizable user sample sizes, not accounting for the novelty effect, and making blunt comparisons in terms of the effectiveness and usability of such systems. For example, in a review by Parsons et al., hundreds of studies addressing virtual reality exposure therapy for phobia and anxiety were reviewed in terms of affective functioning and behavior change. The biggest issue with Parsons's comparative review was a small sample size and a failure to account for the variety of factors that play into VR. The authors argue that Virtual Reality Exposure Therapy (VRET) is a powerful tool for reducing negative symptoms of anxiety, but could not directly calculate demographics, anxiety levels, phobia levels, presence, and immersion between these studies. While curating this review did provide an active snapshot into VRET usage in academia, it is arguable that the data from these studies may have been weak or biased due to the low sample sizes demonstrating positive results and the missing factors of usability for use beyond a single academic study (Parsons and Rizzo, 2008). A study by Jeffrey et al. examined twenty children who received distraction from IV treatment with two controls; iVR HMDs with a racing game as a distraction and a distraction-free treatment case. The results indicated that pain reduction was significant, with a four-fold decrease in facial pain scale responses in cases where iVR was used (Gold et al., 2006). This work positively supports the use of iVR HMDs as a medium for pain reduction, but also lacks a large sample size and provides a somewhat biased comparison of iVR. Is it not to be expected that any distraction of pediatric IV placement would reduce pain? Is iVR vs. no distraction a fair comparison to the general protocol for pediatric IV placement? What about the usage of a TV, or even an audiobook, against the iVR case? In another review by Rizzo et al., VRET was studied using an immersive display that showed veterans 14 different scenarios involving combat-related PTSD stimuli. In one trial, 45% of users were found to no longer test positive for PTSD after seven sessions of exposure. In another trial, more than 75% no longer tested positive for PTSD after 10 sessions. Most users reported liking the VR solution more than traditional exposure therapy (Rizzo et al., 2014). Again, this use of iVR for therapy focused on a small sample size and specific screening techniques, which must be taken into consideration when reviewing the results. Testing for PTSD change in this context only provides a snapshot of VR's effectiveness. Furthermore, the novelty effect (in the sense that the users are not acclimated to the system) may have a significant influence on the result. Given these points, what would happen when users have fully acclimated to this system—is the promise of VRET therapy demonstrated by Parsons et al. truly generalizable? Ultimately, the answer may lie in the direct need for more iVR rehabilitation studies to evaluate and transparently disseminate results between the iVR and niVR comparative norms. An ultimate display for physical rehabilitation with the ability to simulate almost any reality in instigating therapeutic goals may have much potential, but we must understand the behavioral theory behind iVR as a vehicle for healthcare.
3. Immersive Virtual Reality, Behavior, and Perception
As discussed in the previous section, immersive media systems hold vast potential for synergizing the healthcare process. Rehabilitation research, including physical and cognitive work incorporating iVR-based interventions, has been on the rise in recent years. There is now the ability to create programmable immersive experiences that can directly influence human behavior. Conducting conventional therapy in a iVR environment can enable high-fidelity motion capture, telepresence capabilities, and accessible experiences (Lohse et al., 2014; Elor et al., 2018). Through gamification, immersive environments with commercial iVR HMDs, such as the HTC Vive, can be programmed to increase therapy compliance, accessibility, and data throughput by crafting therapeutic goals as game mechanics (Elor et al., 2018). However, what drives the success of iVR healthcare intervention? What aspects of behavioral theory can inform an optimal virtual environment that will assist users during their healthcare experiences? This section aims to explore and understand the theory behind the success of using iVR in healthcare.
3.1. The Benefits of Immersion
iVR provides a means of flexible stimuli through immersion for understanding human behavior in controlled environments. Immersion in a virtual environment can be characterized by the sensorimotor contingencies, or the physical interaction capability of a system (Slater, 2009). It attributes to how well the system may connect a user in iVR through heightened perception and ability to take action, also known as perceptual immersion (Skarbez et al., 2017). This is dependent on the number of motor channels and the range of inputs provided by the system in order to achieve a high fidelity of sensory stimulation (Bohil et al., 2011). Subsequently, perceptual immersion also opens an opportunity for psychological immersion (Skarbez et al., 2017), enabling users to perceive themselves to be enveloped by and a part of the environment (Lombard et al., 2000).
The success of iVR therapeutic intervention is often attributed to the influence of immersion in terms the ability to enhance the relationship between presence and emotion in an engaging experience, and the influence of this on overcoming adversity in task-based objectives (Morina et al., 2015). Immersion can be continuously enhanced through improving graphics, multi-modality, and interaction (Slater, 2009). Strong immersive stimuli through a iVR system, and the ability to provide a feeling of presence and emotion engagement in a virtual world, are key to influencing user behavior (Baños et al., 2004; Morina et al., 2015; Chittaro et al., 2017). Because of this, iVR can play an essential role in augmenting the physical therapy process through the benefits of immersion as it corresponds to a greater spatial and peripheral awareness (Bowman and McMahan, 2007).
Higher-immersion virtual environments were found to be overwhelmingly positive in treatment response (Miller and Bugnariu, 2016). The detachment from reality that is induced by immersion in a virtual world can reduce discomfort for a user, even as far as minimizing pain when compared to clinical analgesic treatments (Hoffman et al., 2011; Gromala et al., 2015). For example, one study found that an iVR world of playful snowmen and snowballs may reduce pain as effectively as morphine during burn victims' wound treatment (Mertz, 2019). Increasing the number of stimuli using iVR is a crucial factor in influencing user experience (Baños et al., 2004). With iVR systems becoming ever more affordable and accessible, these immersive environments are becoming available to the average consumer (Beccue and Wheelock, 2016).
3.2. Presence in the Virtual Environment
Given the benefits of immersion, from task-based guidance in spatial awareness to enabling psychological engagement, it is critical to quantify the effects of presence through immersion. Diemer et al. (2015) have suggested that presence is derived from the technological capabilities of the iVR system and is strengthened by the sense of the immersion of a virtual environment. “Presence” can be defined as the state of existing, occurring, and being present in the virtual environment, and it has been extensively modeled and quantified through past research. Schubert et al. (2001) have argued that presence has three dimensions: spatial presence, involvement, and realness. These dimensions are often quantified through a preliminary survey and cognitive scenario evaluation. Witmer and Singer (1998) have argued that presence is cognitive and is manipulated through directing attention and creating a mental representation of an Immersive Virtual Environment (iVE). Furthermore, Seth et al. (2012) have argued for the introspective predictive coding model of presence, which posits that presence is not limited to iVR but is “a basic property of normal conscious experience.” This argument rests on a continuous prediction of emotional and introspective states, where the perceiver's reaction to the stimulus is used to identify success. For example, a fear stimulus as can be utilized during the prediction of emotional states, where the user compares the actual introspective state (fear and its systems) with the predicted emotional state (fear). A higher presence indicates successful suppression of the mismatch between the predicted emotional state vs. actual emotional state (Diemer et al., 2015). Thus, if the prediction of the fear stimuli is victorious over the mismatch of the user's actual reaction, this may indicate that they were happy, rather than in a state of fear (as was predicted). The idea that suppression of information in a VR experience is vital for presence and the inducing emotion is not new and was previously proposed by Schuemie et al. (2001). Seth et al. (2012) have emphasized that the prediction of emotional states from stimuli plays a crucial role in enabling an emotional experience. Parsons and Rizzo, (2008) research supports this claim; presence is regarded as a necessary mediator to allow “real emotions” to be activated by a virtual environment. However, Diemer et al. has cautioned that research has not yet clarified the relationship between presence and emotional experiences in iVR.
Moreover, quantifying presence is still primarily conceptualized through task-based methods (such as subjective ratings, questionnaires, or interviews), all of which are largely qualitative in nature. A debate between many of these presence theories is whether or not emotion is central to modeling presence. For example, Schubert et al.'s “spatial presence” or Slater's “place illusion” do not require emotion as a prerequisite for presence, which is unlike Diemer's hypothesis of emotion connecting presence and immersion. Given that physical health and recovery has been heavily linked to emotional states (Salovey et al., 2000; Richman et al., 2005), we consider Diemer at al.'s model of presence. Therefore, to effect presence in a virtual environment, there is a need of quantifying emotion. How does one model emotion in this regard, or even quantify it?
3.3. Emotion and Virtual Environments
Quantifying the human emotional response to media has been the topic of much debate in academia. Ekman (1992), a pioneer of emotion theory, argued that there are six basic emotions: anger, fear, sadness, enjoyment, disgust, and surprise. He argued that there are nine characteristics of emotions: they have universal signals, they are found between animals, they affect the physiological system (such as the nervous system), there are universal events which invoke emotion, there is coherence in emotional response, they have rapid onset, they have a brief duration, they are appraised automatically (subconsciously), and their occurrence is involuntary (Ekman, 1992). Ekman's theory does not dismiss any affective phenomena, but instead organizes them to highlight the distinction, based on previous research (in the fields of evolution, physiology, and psychology) between the field and his previous work. His theory also provides a means of quantifying emotions using these principals; it offers a theoretical framework for constructing empirical studies to understand affective states as well as basic emotions (Ekman, 1992). Ekman's basic emotions were found and identifiable in media such as music (Mohn et al., 2011) and photos (Collet et al., 1997).
Since the early 2000s, researchers have examined how technology can extend, emulate, and understand human emotion. Picard (2000), the pioneer of affective computing, has expanded upon theories such as Ekman's to build systems that understand emotion and can communicate with humans emotionally. This had lead numerous findings and demonstrations of systems that demonstrate discrete models (including Ekman's basic emotion model, appraisals models, dimensional models, circuit models, and component models) for quantifying emotional response (Picard, 2000; Kim, 2014). Moreover, numerous machine learning methods have been demonstrated as emotion inference algorithms, such as classification, artificial neural networks, support vector machines, k-nearest neighbor, decision trees, random forests, naive Bayes, deep learning, and various clustering algorithms (Kim, 2014).
With Diemer et al.'s model, emotional engagement may enhance presence to assist the user in an iVE task. Thus, it is useful to quantify a user's emotional response in an iVE. Many studies have examined sense signals and classified patterns as an emotional response from the Autonomic Nervous System. In relation to the basic emotions, Collet et al. (1997) have observed patterns in skin conductance, potential, resistance, blood flow, temperature, and instantaneous respiratory frequency through the use of six emotion-inducing slides presented to 30 users in random order. Through the use of questionnaires, Meuleman and Rudrauf (2018) found that appraisal theory induced the highest emotional response with the HTC Vive iVR System. Liu et al. have utilized real-time EEG-based emotion recognition by applying an arousal-valence emotion model with fractal dimension analysis (Liu et al., 2011) with 95% accuracy along with the National Institute of Mental Health's (NIMH) Center for Study of Emotion and Attention (CSEA) International Affective Picture System (IAPs) (Lang et al., 1997). One of the most widely used metrics for emotion evaluation is the NIMH CSEA Self-Assessment Manikin (SAM) (Bradley and Lang, 1994). Waltemate et al. (2018) used SAM to evaluate emotion concerning the sense of presence and immersion in embedded user avatars with 3D scans through an iVR social experience. SAM enables the evaluation of dimensional emotion (through quantifying valence, arousal, and dominance) by using a picture-matching survey to evaluate varying stimuli. It has been validated for pictures, audio, words, event-related potentials, functional magnetic resonance imaging, pupil dilation, and more (Bradley and Lang, 1994; Lang et al., 1997; Bynion and Feldner, 2017; Geethanjali et al., 2017).
In addressing emotional experiences that influence presence, or a user's sense of “being in” an iVE, we must consider what influences these experiences. Broadly, the majority of research turns to human perception to answer this question. Previous psychological research on threat perception, fear, and exposure therapy implies a relationship between perception and emotion. Perception influences emotion and presence in an iVE, which enables a controlled environment for identifying the most relevant aspects of each user's emotional experience (Baños et al., 2004). The association between perception and conceptual information in iVR must also be considered, as this can play a crucial role in eliciting emotional reactions. For behavior research focusing on areas such as fear, anxiety, and exposure effects, it is vital that iVR is able to induce emotional reactions leading presence and immersion (Diemer et al., 2015). This can be achieved by adjusting perceptual feedback of a user's actions through visual cues, sounds, touch, and smell to trigger an emotional reaction. This goes two ways, in the sense that iVR allows the consideration of how perception can be influenced by iVR itself while also enabling emotional engagement. Therefore, researchers can dissociate perceptual and informational processes as controlled conditions to manipulate their studies in unique ways using iVR (Baños et al., 2004). Given that researchers have found ways to model and influence perception for presence and emotion, what has been done in iVR?
3.4. Human Perception and Multi-Sensory Displays
Human perception appears to be the ultimate driver of user behavior. Yee and Bailenson's (2007) Proteus effect has demonstrated how both self-representation and context in a virtual environment can be successfully influenced via iVR HMDs. The way we perceive the world around us—through our expectations, self-representation, and situational context—may influence how we act and how we approach behavioral tasks. Human perception is reliant on multimedia sensing, such as processing sight, sound, feel, smell, and taste (Geldard et al., 1953). This is problematic because the majority of published research on iVR does not account for this; many studies focus on a singular modality such as a sight or sound, and only occasionally connect sight, sound, and feel. However, with modern advances in commercially available hardware, all senses except for taste have the potential to be controlled in a virtual environment.
3.4.1. Stimuli and Perception
Exploring new input modalities for iVR in physical rehabilitation may help discover new and effective approaches for treatment experience. For example, there have been many studies that have examined how haptic feedback can communicate, help recognize, and inform pattern design for emotions. Bailenson et al. (2007) examined how interpersonal touch may reflect emotional expression and recognition through a hand-based force-feedback haptic joystick. They found that users were able to both recognize and communicate emotions beyond chance through the haptic joystick. In a study by Mazzoni and Bryan-Kinns (2015), the design and evaluation of a haptic glove for mapping emotions evoked by music were found to reliably convey pleasure and arousal. Bonnet et al. (2011) found that facial expression emotion recognition was improved when utilizing a “visio-haptic” platform for virtual avatars and a haptic arm joystick. Salminen et al. (2008) examined the patterns of a friction-based horizontally rotating fingertip stimulator for pleasure, arousal, approachability, and dominance for hundreds of different stimuli pairs. Fingertip actuation indicated that a change in the direction and frequency of the haptic stimulation led to significantly different emotional information. Obrist et al. (2015) demonstrated that patterns in an array of mid-air haptic hand stimulators map onto emotions through varying spatial, directional, and haptic parameters. Miri et al. (2020) examined the design and evaluation of vibrotactile actuation patterns for breath pacing to reduce user anxiety. The authors found that frequency, position, and personalization are critical aspects of haptic interventions for social-emotional applications.
Many prior studies have also found that olfactory echoing principle of universal emotions. Fox (2009) has examined the human sense of smell and its relationship to taste, human variation, children, emotion, mood, perception, attraction, technology, and related research. Sense of smell is often dependent on age (younger people outperform older people), culture (western cultures differ from eastern cultures), and sex (women outperform men). However, other studies suggest that sense of smell mainly depends on a person's state of mental and physical health, regardless of other factors. Some 80-year-olds have the same olfactory prowess as 20-year-olds, and a study from the University of Pennsylvania showed that people who are blind do not necessarily have a keener sense of smell than sighted people (Fox, 2009). It appears to be possible to “train” one's sense of smell to be more sensitive. This poses a problem for researchers, as some subjects in repetitive experiments become skilled in this (i.e., the weight of scent differ for people depending on their sensitivity). Subsequently, Fox (2009) has argued that “the perception of smell consists not only of the sensation of the odors themselves but of the experiences and emotions associated with sensations.” These smells can evoke strong emotional reactions based on likes and dislikes determined by the emotional association. This occurs because the olfactory system is directly connected with an ancient and primitive part of the brain called the limbic system where only cognitive recognition occurs. Thus, a scent may be associated with the triggering of deeper emotional responses. Similar to the Proteus effect (Yee and Bailenson, 2007), our expectations of an odor influence our perception and mood when encountering the stimulus (Fox, 2009).
In terms of perception, positive emotions are indicated with pleasant fragrances and can affect the perception of other people (such as attractiveness of perfume and photographs). Unpleasant smells tend to lead to more negative emotions and task-based ratings (such as when viewing a picture or a completing survey of pleasant or unpleasant odors). General preferences for smells exist (i.e., that the smell of flowers is pleasant and that the smell of gasoline or body odor is unpleasant). Some fragrances, such as vanilla, are universally perceived as pleasant (which is why most perfumes use vanilla). Perfume makers have also shown that appropriate use of color can better identify our liking of fragrance (Fox, 2009). This is supported by the work of Hirsch and Gruss (1999), who explored how olfactory aromas can be quantified to demonstrate arousal. They explored 30 different scents via wearable odor masks with 31 male volunteers. By measuring penile blood flow, the authors found that every smell produced an increase of penile blood flow when compared to no odor, and that pumpkin pie and lavender (which, according to Fox, is considered a universally pleasant scent) produced the most blood flow, with a 40% increase (Hirsch and Gruss, 1999). There appear to be universal smells that are coherent across different demographics, similar to Ekman's argument for universal emotions shared by different races, animals, and sexes (Ekman, 1992; Fox, 2009). An ultimate display that could utilize these smells and adapt to each user's individual preferences by understanding their presence and emotion could be useful in both eliciting an engaging medium of therapy and discovering new universal stimuli.
3.4.2. On Multi-Modal Immersive Virtual Reality Environments
Many researchers have started to recognize and explore the potential of multi-modality iVR interfaces. In an exploratory study by Biocca et al. (2001), the authors concluded that presence may derive from multi-modal integration, such as haptic displays, to improve user experiences. Bernard et al. (2017) showcased an Arduino-driven haptic suit for astronauts to increase embodied situation awareness, but no evaluation was reported. Goedschalk et al. (2017) examined the potential of the commercially available KorFX vest to augment aggressive avatars, but found an insignificant difference between the haptic and non-haptic conditions. And, Krogmeier et al. (2019) demonstrated how a bHaptics Tactisuit vest can influence greater arousal, presence, and embodiment in iVR through a virtual avatar “bump.” The authors found significantly greater embodiment and arousal with full vest actuation compared to no actuation. However, this study only examined a singular pattern and one set stimuli.
Numerous examples can also be seen with thermal actuation, haptic retargeting, and olfactory input. For example, Wolf et al. (2019) and Peiris et al. (2017) explored thermal actuation embedded in iVR HMD facial masks and tangibles which increased enjoyment, presence, and immersion. Doukakis et al. (2019) evaluated a modern system for audio-visual-olfactory resource allocation with tri-modal virtual environments which suggested that visual stimuli is the most preferred for low resource scenarios and aural/olfacotry stimuli preference increases significantly when budgeting is available. Warnock et al. have found that multi-modality notifications through visual, auditory, tactile, and olfactory interfaces were significant in personalizing the needs and preferences of home-care tasks for older adults with and without disability (McGee-Lennon and Brewster, 2011; Warnock et al., 2011). Azmandian et al. (2016) used haptic virtual objects to “hack” real-world presence by shifting the coordinates of the virtual world, leading users to believe that three tangible cubes lay on a table when in reality there was only one cube. Olfactory inputs have been found to be incredibly powerful in increasing immersion and emotional response, such as in Ischer et al.'s (2014) Brain and Behavioral Laboratory Immersive Oder System, Aiken and Berry's (2015) review of olfaction for PTSD treatment, and Schweizer et al.'s (2018) application of iVR and olfactory input for training emergency response. Dinh et al. (1999) demonstrated that multi-sensory stimuli for an iVR virtual office space can increase both presence and spatial memory from a between-subjects factorial user study that varied level of visual, olfactory, auditory, and tactile information. These systems have shown great promise in personalizing systems with the capability to rapidly adapt to smells in an iVR environment. Beyond these theories and proposed systems, there are many limitations and challenges to keep in mind when translating these theories into applied environments.
3.5. Limitations of Current Studies for iVR Behavior and Perception
Immersion, presence, and emotion are critical in influencing an engaging, motivating, and beneficial iVR therapy. However, these themes are not analyzed in iVR therapy studies as standard. This may be primarily due to a lack of uniform quantification of these areas. However, there are many surveys and sensing techniques used to quantify biofeedback, such as the NIMH CSEA SAM and valence-arousal models. Even when studies incorporate such considerations, sample sizes are usually small and methodology is not always transparent. A gold standard can be seen with the NIMH CSEA Self-Assessment Manikin (Bynion and Feldner, 2017; Geethanjali et al., 2017), for which affect is validated using a stimuli database that has been pre-validated by hundreds of participants. There may be a clear benefit in releasing the iVR stimuli evaluated through the ultimate display to create an international affective database for cross-modal virtual reality stimuli.
The user's understanding of how to perform therapy exercises, as well as their commitment to performing them for the duration of the therapy, is critical to ensure effectiveness of rehabilitation. The emotional response generated by an immersive experience influences user engagement and may motivate patients to continue with the objectives of the virtual experience (Chittaro et al., 2017). Therefore, we ask: how might we quantify the success of iVR stimuli toward affecting a users emotional engagement? This leads us to the next section, in which we discuss how understanding the increasing availability of biometric sensors and biofeedback devices for public use may help us find answers to these questions (Soares et al., 2016).
4. Immersive Virtual Reality and Biofeedback
This section aims to identify the theory and usage of biofeedback through a variety of sensory modalities for immersive media and behavioral theory. Biofeedback devices have gained increasing popularity, as they use sensors to gather useful, quantifiable information about user response. For example, the impedance of the sweat glands, or galvanic skin response (GSR), has been correlated to physiological arousal (Critchley, 2002; Boucsein, 2012). This activity can be measured through readily available commercial GSR sensors, and has been explored by researchers to measure the arousal created by media such as television, music, and gaming (Rajae-Joordens, 2008; Salimpoor et al., 2009). Different types of iVR media may affect biofeedback performance. Cameiro et al. analyzed niVR-based physical therapy that uses biofeedback to adapt to stroke patients based on the Yerkes-Dodson law (Cameirao et al., 2009) or the optimal relationship between task-based performance and arousal (Cohen, 2011). By combining heart rate (HR) with GSR, game events and difficulty were quantitatively measured for each user to evaluate optimal performance. Another example can be seen in the work of (Liu et al., 2016), in which GSR alone achieved a 66% average emotion classification accuracy for users watching movies. Combined with GSR, HR can indicate the intensity of physical activity that has occurred. There is definite potential in evaluating the GSR and HR of each user to determine the intensity of the stimuli using different systems of iVR. However, GSR and HR are not the only biometric inputs that could be potentially leveraged when understanding an immersive experience.
In another biofeedback modality, commercially available electroencephalography (EEG) sensors have shown great promise in capturing brain activity and even in inferring emotional states (Ramirez and Vamvakousis, 2012). Brain-computer Interfaces (BCI) incorporating EEG devices have become ever more affordable and user-friendly, with computational techniques for understanding user engagement and intent in medical, entertainment, education, gaming, and more (Al-Nafjan et al., 2017). Based on a review of over 280 BCI-related articles, Al-Nafjan et al. (2017) have argued that EEG-based emotion detection is experiencing booming growth due to advances in wireless EEG devices and computational data analysis techniques such as machine learning. Accessible and low-cost BCIs are becoming more widely available and accurate in the context of both medical and non-medical applications. They can be used for emotion and intent recognition in entertainment, education, and gaming (Al-Nafjan et al., 2017). When compared with 12 other biofeedback experiments, studies that used EEG alone were able to reach 80% max recognition (Goshvarpour et al., 2017). Arguably, the most considerable challenges of BCI are costs, the impedance of sensors, data transfer errors or inconsistency, and ease of use (Al-Nafjan et al., 2017; Goshvarpour et al., 2017).
Even with these challenges, EEG has been successfully used to as a treatment tool for understanding conditions like attention deficit/hyperactivity disorder (ADHD), anxiety disorders, epilepsy, and autism (Marzbani et al., 2016). Brain signals that are characteristic of these conditions can be analyzed with EEG biofeedback to serve as a helpful diagnostic and training tool. Sensing apparatus can be coupled with interactive computer programs or wearables to monitor and provide feedback in many situations. By monitoring levels of alertness in terms of average spectral power, EEG can aid in diagnosing syndromes and conditions like ADHD, anxiety, and stroke (Lubar, 1991). Lubar et al. (1995) used the brainwave frequency power of game events to extract information about reactions to a repeated auditory stimulus, and have demonstrated significant differences between ADHD and non-ADHD groups. Through exploring different placements and brainwave frequencies of EEG sensors across a user's scalp, different wavebands can be used to infer the emotional state and effect of audio-visual stimuli (Deuschl et al., 1999). For example, Ramirez and Vamvakousis (2012) used the alpha and beta bands to infer arousal and valence, respectively, which are then mapped to a two-dimensional emotion estimation model. With these examples in mind, how does one quantify brainwaves for emotional inference?
4.1. Brainwaves as a Means of Studying Emotional Intelligence
Hans Berger, a founding father of EEG, was one of the first to analyze these frequency bands of brain activity and correlate them to human function (Haas, 2003; Llinás, 2014). The analysis of different brainwave frequencies has been correlated to different psychological functions, such as the 8–13 Hz Alpha band relating to stress (Foster et al., 2017), the 13–32 Hz Beta band relating to focus (Rangaswamy et al., 2002; Baumeister et al., 2008), the 0.5–4 Hz Delta band relating to awareness (Walker, 1999; Hobson and Pace-Schott, 2002; Iber and Iber, 2007; Brigo, 2011), the 4–8 Hz Theta band relating to sensorimotor processing (Green and Arduini, 1954; Whishaw and Vanderwolf, 1973; O'Keefe and Burgess, 1999; Hasselmo and Eichenbaum, 2005), and the Gamma band of 32–100 Hz related to cognition (Singer and Gray, 1995; Hughes, 2008; O'Nuallain, 2009). These different frequencies may prove fruitful in quantifying the effects of virtual stimuli during iVR based physical therapy, taking into account the fact that signals may be noisy due to other biological artifacts and must be handled carefully (Vanderwolf, 2000; Whitham et al., 2008; Yuval-Greenberg et al., 2008). For example, alpha activity is reduced with open eyes, drowsiness, and sleep (Foster et al., 2017); increases in beta waves have been suggesting for active, busy, or anxious thinking and concentration (Baumeister et al., 2008); delta activity spikes with memory foundation (Hobson and Pace-Schott, 2002) such as flashbacks and dreaming (Brigo, 2011); theta activity increases when planning motor behavior (Whishaw and Vanderwolf, 1973) path spatialization (O'Keefe and Burgess, 1999) memory, and learning (Hasselmo and Eichenbaum, 2005); and gamma shows patterns related to deep thought, consciousness, and meditation (Hughes, 2008).
Additionally, there are many methods for evaluating and classifying emotions with brainwaves. Eimer et al. (2003) used high-resolution EEG sensing to analyze the processing of Ekman's six basic emotions via facial expression during P300 event-related potential analysis (ERP). Emotional faces had significantly different reaction times from neutral faces (supporting the rapid onset of emotion Ekman's principle). The authors concluded that ERP facial expression effects gated by spatial attention appear inconsistent, however, ERP effects are directly due to Amygdala activation, they also conclude that ERP results demonstrate facial attention is strongly dependent on facial expression, and that the six basic facial expressions with emotions were strikingly similar (Eimer et al., 2003). ERPs are an effective way to quantify EEG brainwave readings for emotional analysis, but they are not always reliable. However, they can accurately gauge from an arousal response by looking at a P300 window of revealing stimuli. These techniques open opportunities for estimating emotion through multiple biofeedback modalities.
Researchers have combined these EEG interfaces with other forms of multi-modal biometric data collection such as GSR and HR to increase the inference of affective response. By combining GSR with HR and EEG, researchers have been able to increase the accuracy of emotion recognition (Liu et al., 2016; Goshvarpour et al., 2017). Other niVR based games have successfully incorporated the use of these biofeedback markers to determine physiological response (Cameirao et al., 2009; Soares et al., 2016). However, there is a lack of studies exploring these biometrics with iVR and physical therapy, such as the one described in this paper. This is particularly true in the case of examining long-term use beyond the novelty period and allowing for user acclimatization to the experimental environment. With such limitations in mind, it is possible that these effects and psychological responses could be quantitatively measured through combining active EEG sensing with the flexible stimuli of iVR gameplay. In the light of this, what has been done to bridge biofeedback to iVR?
4.2. Biofeedback Systems Utilized With Virtual Reality
The closest experience (albeit not immersive) to the proposed ultimate display augmentation for rehabilitation discussed in this paper can be seen in i Badia et al.'s work on a procedural biofeedback-driven nonlinear 3D-generated maze that utilized the NIMH CSEA International Affective Picture System. VR mental health treatment has seen extensive exploration and promising results over the past two decades. However, most of the experiences are not personalized for treatment, and more personalized treatment is likely to lead to more successful rehabilitation. i Badia et al. (2018) has argued for the use of biofeedback strategies to infer the internal state of the patient state. Users navigated a maze where the visuals and music were adapted according to emotional state (i Badia et al., 2018). The framework incorporated the Unity3D game engine in a procedural content generation through three modules of real-time affective state estimation, event trigger computation, and virtual procedural scenarios. These were connected in a closed-loop during runtime through biofeedback, emotion game events, and sensing trigger events. The software architecture uses any iVR medium and runs the Unity application with a separate process for data acquisition via UDP protocol, which was published and shared as a Unity plugin (i Badia et al., 2018). Overall results indicated significance for anger, fear, sadness, and neutral (in Friedman analysis), and a Self-Assessment Manikin Indicated significant feelings of pleasantness associated with the experience. However, the game was not explored using an immersive medium (instead, a Samsung TV was used), varying intensity was not explored, and control factors were random to each user, which may have influenced results (i Badia et al., 2018).
Immersive experiences exploring low-cost commercial biofeedback devices have been also been presented, although methodology has not been fully disseminated. Redd et al. (1994) found that cancer patients during Magnetic Resonance Imaging responded with a 63% decrease in anxiety with heliotropin (a vanillalike scent) with humidified air when compared to a odorless humidified air alone. Expanding upon this work, Amores et al. (2018) utilized a low-cost commercial EEG device, a brain-sensing headband named Muse 2 (InteraXon, 2019), with an olfactory necklace and immersive virtual reality for promoting relaxation. By programming odor to react to alpha and theta EEG activity within iVR, users demonstrated increases of 25% physiological response and reported relaxation when compared to no stimulus. This may validate the effectiveness of combining iVR with olfactory input, as well as the ability to quantify mental state through physiological changes through low-cost, low-resolution commercial EEG.
In another example, Abdessalem et al. compared mental activity of EEG recordings to the International Affective Picture System for a serious game named “AmbuRun.” Users entered an iVR game in which they had to carry a patient in an ambulance to the hospital and drive it through traffic. They evaluated the game with 20 participants, and the difficulty adapted to each user so that higher frustration led to more traffic (Abdessalem et al., 2018). The authors identified significant results; 70% of players reported that the game was harder when they were frustrated, while only 15% said they did not notice any change in difficulty. However, this study does not share baseline EEG activity results, nor does it explain the adaptive difficulty algorithms that were used (Abdessalem et al., 2018). Other examples relating biofeedback and iVR can be found in the work of Maŕın-Morales et al. (2018), who examined EEG and heart rate variability with portable iVR HMDs to elicit emotions by exploring 3D architectural worlds. Krönert et al. (2018) developed a custom headband that recorded BVP, PPG, and GSR while adults completed various games in learning environments. Van Rooij et al. (2016) developed a game that displayed diaphragmatic breathing patterns in children with the aim of reducing in-game anxiety, and was able to get users to reverse panic attacks. Again, while all these results were highly promising in incorporating biofeedback techniques to augment iVR user experiences, they were also lacking in many areas.
4.3. Limitations of Current Studies for iVR Biofeedback
A large amount of work has been done independently in the biofeedback field in terms of methods of sensing mental activity, and there is now a plethora of sensing methods. Some games have been created incorporating biofeedback with promising results. However, these studies are often vague and do not publish stimuli or demos beyond what is written in the paper. In this literature review, we have found that most of these biofeedback games are not multi-modal sensing and thus do not account for any low-resolution sensing or movement artifacts from gameplay through sensor fusion (i.e., HR and GSR could be used with in-game behavior to cross-validate physiological signal change during therapy with EEG sensing). Additionally, the majority of these studies do not incorporate runtime feedback from the user themselves (beyond pre- or post-test surveys). Quantifying emotion is usually done either solely through biofeedback and emotion estimation, or post-test surveys, but never both during runtime. It is possible that biofeedback emotional estimation combined with embedded gameplay surveys may be a way to better objectively measure presence, as long as immersion is not broken when queried for survey response.
Additionally, these studies are often not conducted with multi-modal stimuli. Human perception is inherently multi-modal, and perhaps emotional response may become more accurate when utilizing multiple human senses beyond audio and visual stimuli. What happens when we factor in smell and touch while collecting biofeedback measures within iVR? As with the other limitations discussed in the previous two sections, much of this work is not disseminated beyond the papers themselves [with the exception of i Badia et al.'s (2018) published biofeedback plugin]. Future researchers can address these limitations by fully disseminating their methodology and algorithms in their work, and such aspects should be transparent toward the design and evaluation of immersive media with biofeedback.
5. An Ultimate Display for Physical Rehabilitation
We dedicate this section to expand upon the current literature review and bridge the discussions in the previous sections on immersive virtual reality, rehabilitation, behavioral theory, and biofeedback. In the previous sections, we discussed how the newfound commercial adoption of iVR devices and the affordability of biofeedback devices may lead to new opportunities for adaptive experiences in healthcare that are feasible for the average consumer. iVR-based therapy from psychological, physiological, and telepresence applications have shown great promise and great potential. The theory and success behind iVR as a medium for healthcare intervention is driven by immersion and its relationship with presence and emotion. Because presence and emotion tend to be subjective, quantification of their measures is not always reproducible. However, many quantification methods exist, ranging from a sensing algorithmic approach to a variety of validated surveys. The current literature review has found that more work must be done to provide clear guidelines, universal iVR stimuli to evaluate affect, and an environment that factors multi-modal sensing and stimulation for presence and emotion. These items may address a need for a controllable multi-modal immersive display that can factor in physical and emotional intelligence through both qualitative and quantitative biofeedback.
5.1. Augmenting the Ultimate Display
To bridge the many academic works that we have surveyed, we consider a theoretical framework toward augmenting the ultimate display for rehabilitation. Such an augmentation would utilize the capabilities of a controlled iVE and quantifying emotion both through biofeedback (i.e., heart rate, sweat glands, and brainwaves) while also using in-game surveys to measure the user's self-perception and emotional state. The environment would factor in human perception and emotion through multiple co-dependent senses rather than a single sense. This could be achieved via olfactory modules, haptic feedback vests, and iVR HMDs. The system must account for pre-gameplay states and develop a baseline emotion profile for each user; this could be done by asking the user to relax for a set period of time while in the display in order to calibrate biofeedback sensors. With such a profile, we could examine how biofeedback changes occur when the user is presented with varying stimuli during exercise. The system may follow the effects of physical rehabilitation performance in comparison to biofeedback response and presented stimuli. By factoring in these metrics, we may be able to provide an iVR healthcare experience that adapts to each user's individual response and preferences.
This augmented display would equate to a sandbox controlled virtual environment to assist in the therapy process by enabling users to explore new attitudes, modulate cognitive biases, and examine behavioral responses. Through these multi-modal sensory and motor simulations, researchers could craft experiences to assist in therapeutic engagement, and quantify or adapt the experience through biofeedback during runtime. Our vision for this augmented ultimate display comes from the synergy of three components: immersive media, biofeedback, and wearable robotics. Figure 1 demonstrates these mediums as inputs to augment the therapy process and show how they bring about emotional intelligence, physical intelligence, and adaptability.
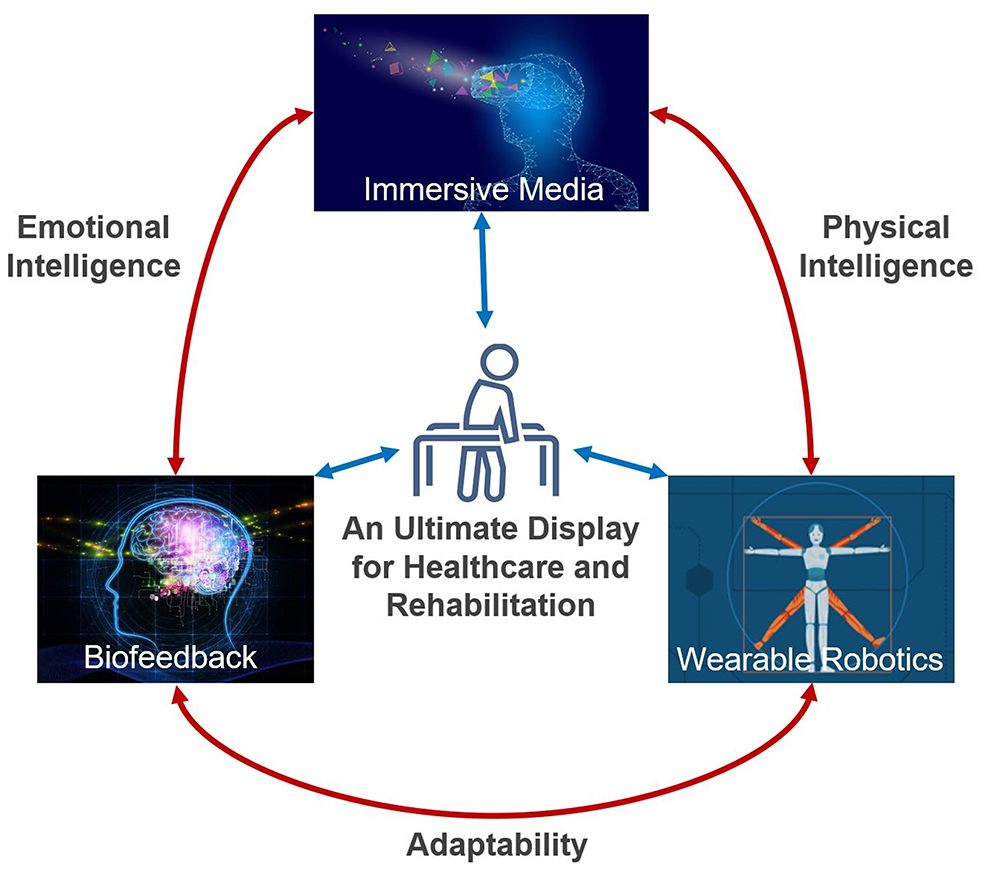
Figure 1. Components of the theoretical ultimate display augmented for rehabilitation. Areas and some of their synergies through immersive media, wearable robotics, and biofeedback. Elements of wearable robotics enable automated tracking of user progression. Physical intelligence examples include haptic stimulation, physical assistance, and positional sensing informed between the virtual experience and the wearable. Emotional intelligence examples include personalizing iVR stimuli by arousal response and calibrating the difficulty of iVR therapy based on heart rate. Adaptability examples include adjusting the physical assistance of wearable robotics and allowing for biometric input modalities to enable users of mixed ability to participate in the virtual experience.
As discussed in the previous sections, many components of this proposed augmentation have been rigorously researched independently within their respective fields. The synergies of these areas have the potential to produce emotional, physical, and adaptive intelligence from the interdisciplinary combination of these mediums. Nevertheless, these concepts are often not applied to healthcare. Some emergent research, as discussed in the previous sections [such as the work of i Badia et al.'s (2018)], has explored synergies between these areas, but these have not been fully demonstrated in healthcare or rehabilitation. Given the potential that immersive media has shown in therapy and rehabilitation, these fields and their synergies should be explored as one. This is necessary to advance the field of immersive media for healthcare and to fully understand how an ultimate display augmented for rehabilitation can be met. The center of Figure 1 represents this vision; a display in which the very world the user performs their rehabilitation in can adapt its difficulty and game mechanics to motivate and guide them through their emotional response through immersive computational media. Such a display would explore the limits of modeling a person's emotional reaction, mental perception, and physical ability, while also applying rehabilitation theory in a quantifiable and controlled environment. Just as the moon influences the tide, perhaps this display could influence our emotional “tides” to best perform rehabilitative tasks by influencing our perception for the better. The core elements of this biometric infused cyber-physical approach to immersive media in rehabilitation are illustrated in Figure 1.
This review examined how iVR can be a powerful tool in reducing discomfort and pain. As in the case of SnowWorld, created at University of Washington's HITLab, the experience demonstrated that iVR can be as effective as morphine in reducing pain for burn victims (Gromala et al., 2015). Much of this success can be attributed to the benefits and affordances of immersion (Bowman and McMahan, 2007; Slater, 2009; Diemer et al., 2015; Skarbez et al., 2017). Therefore, the augmented ultimate display would need to enable the crafting of virtual worlds with high levels of presence and emotional engagement to assist user perception in overcoming adversity experienced in rehabilitation (such as pain and discomfort). One example to explore this may be readily feasible by augmenting the NIMH International Affective Databases (IAD) (Lang et al., 1997). Researchers could extend these existing stimuli with multi-modality and evaluate user experience through biofeedback. Additionally, through utilizing the capabilities of a controlled iVE, emotion could be accurately quantified through both employing biofeedback while also using in-game surveys to measure the user's self-perception and emotional state. This data might be further explored to adapt both the immersive media stimuli and the level of assistance. For example, such an experience may allow researchers to build a baseline affective dataset for each user that could be applied to other immersive healthcare experiences with iVR. Similar emotional states from this baseline experience can be used to predict emotional response in order to adjust game difficulty and assist users with physical movement. Through this process, we may be able to create the ultimate behavioral sandbox for quantifying emotion during behavioral tasks and collect profiles to be applied to runtime physical therapy environments that can account for emotional intelligence during gameplay.
5.2. The Ultimate Display as a Rehabilitation Toolbox for Task-Based Experiences
The development of an augmented ultimate display for rehabilitation may have broader impacts in the field of healthcare research. To illustrate some of the many theories that this system could explore, we share the following for consideration:
• Perception theory indicates that human perception is the composition of parallel senses of sight, hear, smell, feel, and taste, all of which influence behavior presence (Chalmers and Ferko, 2008). Subsequently, a multi-sensory iVR experience should induce more significant immersion with affordances for presence and emotional response (Bowman and McMahan, 2007; Slater, 2009; Diemer et al., 2015; Skarbez et al., 2017). If this is true, perhaps we can create better iVR experiences for higher therapy engagement, compliance, and satisfaction.
• The Yerkes-Dodson Law states that, for any behavioral task, there is an optimal level of arousal to induce the optimal level of performance (Cohen, 2011). This law is one of the most frequently cited cognitive psychology theories but has never been verified (Teigen, 1994). If we can quantify arousal with the ultimate display by combining biofeedback sensing with in-game micro surveys, we may be able to verify the relationship between arousal and task-performance. If this is true, we may be able to create optimal stimuli to assist users in overcoming adversity within their therapy regimen.
• Csikszentmihalyi's Flow Theory suggests that total engagement in an activity can be achieved when perceived opportunities (challenges) are in balance with the action capabilities (skills) of an experience (Csikszentmihalyi, 1975, 1990). This concept has been extended in virtual environments with “Gameflow,” where user enjoyment is a result of balancing an environment's required concentration, challenge, skill, control, goals, feedback, immersion, and interaction of an environment (Sweetser and Wyeth, 2005). Similarly to the Yerkes-Dodson Law, augmenting the ultimate display for physical rehabilitation enables a controlled environment to develop and measure optimal models of user engagement with therapy tasks.
5.3. Limitations of This Review
There are many limitations to consider in this review. Firstly, the fields of rehabilitation, immersive media, and biofeedback are vast and ever-changing. However, we believe this review provides an adequate snapshot of the current potential that each of these literature review themes holds for assistive application. Additionally, this study primarily focused on iVR through head-mounted displays. Other extended reality mediums, such as spatial computing with augmented and mixed reality headsets, should be considered. With the advent of 5G edge computing and many extended reality devices exploring high-throughput streaming and social interaction, new paradigms for iVR-based therapy may emerge in the coming years. Yet, we believe that this review of iVR-based HMDs is still very relevant due to newfound consumer adoption and the necessity to drive and review the limitations of a field that is currently still maturing.
6. Conclusion
Immersive virtual reality paired with multi-modal stimuli and biofeedback for healthcare is an emerging field that is underexplored. Our bridging review of iVR contributes to the body of knowledge toward understanding immersive assistive technologies by reviewing the feasibility of a biometric-infused immersive media approach. We reviewed and discussed iVR therapy applications, the behavioral theory behind iVR, and quantification methods using biofeedback. Common limitations in all these fields include the need to develop a standard database for iVR-affective stimuli and the need for transparent dissemination of experimental methodology, tools, and user demographics in evaluating iVR for healthcare. We proposed an ultimate display augmented for rehabilitation that utilizes virtual reality by combining immersive media, biofeedback, and wearable robotics. Specific outcomes of such a system may include new algorithms and tools to integrate emotion feedback in iVR for researchers and therapists, discoveries of new relationships between emotion and action in physical therapy, and new methodologies to produce optimal therapy benefits for patients by incorporating immersive media and biometric feedback. These results may lead to deeper mediums for both clinical and at-home therapy. They may uncover novel approaches to rehabilitation and increase the affordability, accuracy, and accessibility of treatment. We believe that future of iVR healthcare may become a new field of therapy; a field that is centered on immersive physio-rehab that reacts, learns, and adapts its stimuli and difficulty to each individual user to establish a more engaging and impactful rehabilitation experience.
Author Contributions
AE wrote the first draft of the manuscript. SK assisted with the literature review search and provided further writing. AE iteratively revised the manuscript with SK. The ideas of the paper were formulated through a 4-year collaboration with local physical therapy centers in Santa Cruz, California, to which all authors contributed. All authors contributed to the article and approved the submitted version.
Funding
This material is based upon work supported by the National Science Foundation under Grant No. #1521532. Any opinions, findings, and conclusions or recommendations expressed in this material are those of the author(s) and do not necessarily reflect the views of the National Science Foundation. Additionally, AE was supported by the University of California Global Community Health Wellbeing 2020 Fellows program.
Conflict of Interest
The authors declare that the research was conducted in the absence of any commercial or financial relationships that could be construed as a potential conflict of interest.
Acknowledgments
The authors thank Noah Wardrip-Fruin for his advice, suggestions, and support during the literature review of this manuscript.
References
Abdessalem, H. B., Boukadida, M., and Frasson, C. (2018). “Virtual reality game adaptation using neurofeedback,” in The Thirty-First International Flairs Conference (Melbourne, FL).
Aiken, M. P., and Berry, M. J. (2015). Posttraumatic stress disorder: possibilities for olfaction and virtual reality exposure therapy. Virtual Real. 19, 95–109. doi: 10.1007/s10055-015-0260-x
Al-Nafjan, A., Hosny, M., Al-Ohali, Y., and Al-Wabil, A. (2017). Review and classification of emotion recognition based on EEG brain-computer interface system research: a systematic review. Appl. Sci. 7:1239. doi: 10.3390/app7121239
Amores, J., Richer, R., Zhao, N., Maes, P., and Eskofier, B. M. (2018). “Promoting relaxation using virtual reality, olfactory interfaces and wearable EEG,” in 2018 IEEE 15th International Conference on Wearable and Implantable Body Sensor Networks (BSN) (Las Vegas, NV), 98–101. doi: 10.1109/BSN.2018.8329668
Assis, G. A. d., Corrêa, A. G. D., Martins, M. B. R., Pedrozo, W. G., and Lopes, R. d. D. (2016). An augmented reality system for upper-limb post-stroke motor rehabilitation: a feasibility study. Disabil. Rehabil. 11, 521–528. doi: 10.3109/17483107.2014.979330
Azmandian, M., Hancock, M., Benko, H., Ofek, E., and Wilson, A. D. (2016). “Haptic retargeting: Dynamic repurposing of passive haptics for enhanced virtual reality experiences,” in Proceedings of the 2016 Chi Conference on Human Factors in Computing Systems (San Jose, CA), 1968–1979. doi: 10.1145/2858036.2858226
Ba nos, R. M., Botella, C., Alca niz, M., Lia no, V., Guerrero, B., and Rey, B. (2004). Immersion and emotion: their impact on the sense of presence. CyberPsychol. Behav. 7, 734–741. doi: 10.1089/cpb.2004.7.734
Bailenson, J. N., Yee, N., Brave, S., Merget, D., and Koslow, D. (2007). Virtual interpersonal touch: expressing and recognizing emotions through haptic devices. Hum. Comput. Interact. 22, 325–353. doi: 10.1080/07370020701493509
Baldominos, A., Saez, Y., and del Pozo, C. G. (2015). An approach to physical rehabilitation using state-of-the-art virtual reality and motion tracking technologies. Proc. Comput. Sci. 64, 10–16. doi: 10.1016/j.procs.2015.08.457
Bamberg, S. J. M., Benbasat, A. Y., Scarborough, D. M., Krebs, D. E., and Paradiso, J. A. (2008). Gait analysis using a shoe-integrated wireless sensor system. IEEE Trans. Inform. Technol. Biomed. 12, 413–423. doi: 10.1109/TITB.2007.899493
Baumeister, J., Barthel, T., Geiss, K.-R., and Weiss, M. (2008). Influence of phosphatidylserine on cognitive performance and cortical activity after induced stress. Nutr. Neurosci. 11, 103–110. doi: 10.1179/147683008X301478
Beccue, M., and Wheelock, C. (2016). Research Report: Virtual Reality for Consumer Markets. Technical report, Tractica Research.
Bernard, T., Gonzalez, A., Miale, V., Vangara, K., Stephane, L., and Scott, W. E. (2017). “Haptic feedback astronaut suit for mitigating extra-vehicular activity spatial disorientation,” in AIAA SPACE and Astronautics Forum and Exposition (Orlando, FL). doi: 10.2514/6.2017-5113
Biocca, F., Kim, J., and Choi, Y. (2001). Visual touch in virtual environments: an exploratory study of presence, multimodal interfaces, and cross-modal sensory illusions. Presence 10, 247–265. doi: 10.1162/105474601300343595
Bohil, C. J., Alicea, B., and Biocca, F. A. (2011). Virtual reality in neuroscience research and therapy. Nat. Rev. neurosci. 12, 752–762. doi: 10.1038/nrn3122
Bonnet, D., Ammi, M., and Martin, J.-C. (2011). “Improvement of the recognition of facial expressions with haptic feedback,” in 2011 IEEE International Workshop on Haptic Audio Visual Environments and Games (Nanchang), 81–87. doi: 10.1109/HAVE.2011.6088396
Boucsein, W. (2012). Electrodermal Activity. Boston, MA: Springer Science & Business Media. doi: 10.1007/978-1-4614-1126-0
Bowman, D. A., and McMahan, R. P. (2007). Virtual reality: how much immersion is enough? Computer 40, 36–43. doi: 10.1109/MC.2007.257
Bradley, M. M., and Lang, P. J. (1994). Measuring emotion: the self-assessment manikin and the semantic differential. J. Behav. Ther. Exp. Psychiatry 25, 49–59. doi: 10.1016/0005-7916(94)90063-9
Brigo, F. (2011). Intermittent rhythmic delta activity patterns. Epilepsy Behav. 20, 254–256. doi: 10.1016/j.yebeh.2010.11.009
Burdea, G. C. (2003). Virtual rehabilitation-benefits and challenges. Methods Inform. Med. 42, 519–523. doi: 10.1055/s-0038-1634378
Byl, N. N., Abrams, G. M., Pitsch, E., Fedulow, I., Kim, H., Simkins, M., et al. (2013). Chronic stroke survivors achieve comparable outcomes following virtual task specific repetitive training guided by a wearable robotic orthosis (ul-exo7) and actual task specific repetitive training guided by a physical therapist. J. Hand Ther. 26, 343–352. doi: 10.1016/j.jht.2013.06.001
Bynion, T. M., and Feldner, M. T. (2017). “Self-assessment manikin,” in Encyclopedia of Personality and Individual Differences, eds V. Zeigler-Hill, and T. Shackelford (Cham: Springer). doi: 10.1007/978-3-319-28099-8_77-1
Cameir ao, M. S., Bermúdez, S., and Verschure, P. (2008). Virtual reality based upper extremity rehabilitation following stroke: a review. J. CyberTher. Rehabil. 1, 63–74. Available online at: http://hdl.handle.net/10553/48154
Cameirao, M. S., Bermúdez, I. B. S., Duarte Oller, E., and Verschure, P. F. (2009). The rehabilitation gaming system: a review. Stud. Health Technol. Inform. 145, 65–83. doi: 10.3233/978-1-60750-018-6-65
Campbell, R., Evans, M., Tucker, M., Quilty, B., Dieppe, P., and Donovan, J. (2001). Why don't patients do their exercises? Understanding non-compliance with physiotherapy in patients with osteoarthritis of the knee. J. Epidemiol. Commun. Health 55, 132–138. doi: 10.1136/jech.55.2.132
Chalmers, A., and Ferko, A. (2008). “Levels of realism: from virtual reality to real virtuality,” in Proceedings of the 24th Spring Conference on Computer Graphics (Budmerice Castle: ACM), 19–25.
Chittaro, L., Sioni, R., Crescentini, C., and Fabbro, F. (2017). Mortality salience in virtual reality experiences and its effects on users' attitudes towards risk. Int. J. Hum. Comput. Stud. 101, 10–22. doi: 10.1016/j.ijhcs.2017.01.002
Cohen, R. A. (2011). “Yerkes-Dodson law,” in Encyclopedia of Clinical Neuropsychology, eds J. S. Kreutzer, J. DeLuca, and B. Caplan (New York, NY: Springer), 2737–2738. doi: 10.1007/978-0-387-79948-3_1340
Collet, C., Vernet-Maury, E., and Dittmar, A. (1997). Autonomic nervous system response pattern specificity to basic emotions. Int. J. Psychophysiol. 1, 53–54. doi: 10.1016/S0167-8760(97)85486-4
Corbetta, D., Imeri, F., and Gatti, R. (2015). Rehabilitation that incorporates virtual reality is more effective than standard rehabilitation for improving walking speed, balance and mobility after stroke: a systematic review. J. Physiother. 61, 117–124. doi: 10.1016/j.jphys.2015.05.017
Costello, P. J. (1997). Health and Safety Issues Associated With Virtual Reality: A Review of Current Literature. Advisory Group on Computer Graphics.
Critchley, H. D. (2002). Electrodermal responses: what happens in the brain. Neuroscientist 8, 132–142. doi: 10.1177/107385840200800209
Crosbie, J., Lennon, S., Basford, J., and McDonough, S. (2007). Virtual reality in stroke rehabilitation: still more virtual than real. Disabil. Rehabil. 29, 1139–1146. doi: 10.1080/09638280600960909
Cruz-Neira, C., Sandin, D. J., and DeFanti, T. A. (1993). “Surround-screen projection-based virtual reality: the design and implementation of the cave,” in Proceedings of the 20th Annual Conference on Computer Graphics and Interactive Techniques (New York, NY), 135–142. doi: 10.1145/166117.166134
Csikszentmihalyi, M. (1975). Beyond Boredom and Anxiety: The Experience of Play in Work and Leisure. San Fransico, CA: Jossey-Bass.
Csikszentmihalyi, M. (1990). FLOW: The Psychology of Optimal Experience, 1st Edn. New York, NY: Harper & Row; Harper Perennial Modern Classics. Available online at: https://www.amazon.com/Flow-Psychology-Experience-Perennial-Classics/dp/0061339202
Dascal, J., Reid, M., IsHak, W. W., Spiegel, B., Recacho, J., Rosen, B., and Danovitch, I. (2017). Virtual reality and medical inpatients: a systematic review of randomized, controlled trials. Innov. Clin. Neurosci. 14, 14–21.
Deuschl, G., and Eisen, A. (1999). Recommendations for the practice of clinical neurophysiology: guidelines of the international federation of clinical neurophysiology. Electroencephalogr. Clin. Neurophysiol. Suppl. 52, 1–304.
Deutsch, J. E., Lewis, J. A., and Burdea, G. (2007). Technical and patient performance using a virtual reality-integrated telerehabilitation system: preliminary finding. IEEE Trans. Neural Syst. Rehabil. Eng. 15, 30–35. doi: 10.1109/TNSRE.2007.891384
Diemer, J., Alpers, G. W., Peperkorn, H. M., Shiban, Y., and Mühlberger, A. (2015). The impact of perception and presence on emotional reactions: a review of research in virtual reality. Front. Psychol. 6:26. doi: 10.3389/fpsyg.2015.00026
Dinh, H. Q., Walker, N., Hodges, L. F., Song, C., and Kobayashi, A. (1999). “Evaluating the importance of multi-sensory input on memory and the sense of presence in virtual environments,” in Proceedings IEEE Virtual Reality (Cat. No. 99CB36316), 222–228. doi: 10.1109/VR.1999.756955
Doukakis, E., Debattista, K., Bashford-Rogers, T., Dhokia, A., Asadipour, A., Chalmers, A., and Harvey, C. (2019). Audio-visual-olfactory resource allocation for tri-modal virtual environments. IEEE Trans. Visual. Comput. Graph. 25, 1865–1875. doi: 10.1109/TVCG.2019.2898823
Eimer, M., Holmes, A., and McGlone, F. P. (2003). The role of spatial attention in the processing of facial expression: an ERP study of rapid brain responses to six basic emotions. Cogn. Affect. Behav. Neurosci. 3, 97–110. doi: 10.3758/CABN.3.2.97
Ekman, P. (1992). An argument for basic emotions. Cogn. Emot. 6, 169–200. doi: 10.1080/02699939208411068
Elor, A., Teodorescu, M., and Kurniawan, S. (2018). Project star catcher: A novel immersive virtual reality experience for upper limb rehabilitation. ACM Trans. Access. Comput. 11:20. doi: 10.1145/3265755
Foster, J. J., Sutterer, D. W., Serences, J. T., Vogel, E. K., and Awh, E. (2017). Alpha-band oscillations enable spatially and temporally resolved tracking of covert spatial attention. Psychol. Sci. 28, 929–941. doi: 10.1177/0956797617699167
Freeman, D., Reeve, S., Robinson, A., Ehlers, A., Clark, D., Spanlang, B., et al. (2017). Virtual reality in the assessment, understanding, and treatment of mental health disorders. Psychol. Med. 47, 2393–2400. doi: 10.1017/S003329171700040X
Frenkel, K. A. (1989). An interview with Ivan Sutherland. Commun. ACM 32, 712–714. doi: 10.1145/63526.63531
Geethanjali, B., Adalarasu, K., Hemapraba, A., Pravin Kumar, S., and Rajasekeran, R. (2017). Emotion analysis using SAM (self-assessment manikin) scale. Biomed. Res. 28, 18–24. Available online at: https://www.alliedacademies.org/articles/emotion-analysis-using-sam-selfassessment-manikin-scale.pdf
Goedschalk, L., Bosse, T., and Otte, M. (2017). “Get your virtual hands off me!-developing threatening IVAs using haptic feedback,” in Benelux Conference on Artificial Intelligence (Groningen: Springer), 61–75. doi: 10.1007/978-3-319-76892-2_5
Gold, J. I., Kim, S. H., Kant, A. J., Joseph, M. H., and Rizzo, A. S. (2006). Effectiveness of virtual reality for pediatric pain distraction during IV placement. CyberPsychol. Behav. 9, 207–212. doi: 10.1089/cpb.2006.9.207
Goshvarpour, A., Abbasi, A., and Goshvarpour, A. (2017). An accurate emotion recognition system using ECG and GSR signals and matching pursuit method. Biomed. J. 40, 355–368. doi: 10.1016/j.bj.2017.11.001
Green, J. D., and Arduini, A. A. (1954). Hippocampal electrical activity in arousal. J. Neurophysiol. 17, 533–557. doi: 10.1152/jn.1954.17.6.533
Grillon, H., Riquier, F., Herbelin, B., and Thalmann, D. (2006). Virtual reality as a therapeutic tool in the confines of social anxiety disorder treatment. Int. J. Disabil. Hum. Dev. 5, 243–250. doi: 10.1515/IJDHD.2006.5.3.243
Gromala, D., Tong, X., Choo, A., Karamnejad, M., and Shaw, C. D. (2015). “The virtual meditative walk: virtual reality therapy for chronic pain management,” in Proceedings of the 33rd Annual ACM Conference on Human Factors in Computing Systems (Seoul: ACM), 521–524. doi: 10.1145/2702123.2702344
Haas, L. F. (2003). Hans berger (1873-1941), richard caton (1842-1926), and electroencephalography. J. Neurol. Neurosurg. Psychiatry 74:9. doi: 10.1136/jnnp.74.1.9
Hasselmo, M. E., and Eichenbaum, H. (2005). Hippocampal mechanisms for the context-dependent retrieval of episodes. Neural Netw. 18, 1172–1190. doi: 10.1016/j.neunet.2005.08.007
Hirsch, A., and Gruss, J. (1999). Human male sexual response to olfactory stimuli. J. Neurol Orthop. Med. Surg. 19, 14–19.
Hobson, J. A., and Pace-Schott, E. F. (2002). The cognitive neuroscience of sleep: neuronal systems, consciousness and learning. Nat. Rev. Neurosci. 3:679. doi: 10.1038/nrn915
Hoffman, H. G., Chambers, G. T., Meyer, W. J., Arceneaux, L. L., Russell, W. J., Seibel, E. J., et al. (2011). Virtual reality as an adjunctive non-pharmacologic analgesic for acute burn pain during medical procedures. Ann. Behav. Med. 41, 183–191. doi: 10.1007/s12160-010-9248-7
Howden, L., and Meyer, J. (2011). Age and Sex Composition: 2010. US Census Bureau. Recuperado de: Available online at: http://www.census.gov/prod/cen2010/briefs/c2010br-03
Hughes, J. R. (2008). Gamma, fast, and ultrafast waves of the brain: their relationships with epilepsy and behavior. Epilepsy Behav. 13, 25–31. doi: 10.1016/j.yebeh.2008.01.011
i Badia, S. B., Quintero, L. V., Cameirao, M. S., Chirico, A., Triberti, S., Cipresso, P., et al. (2018). Towards emotionally-adaptive virtual reality for mental health applications. IEEE J. Biomed. Health Inform. 23, 1877–1887. doi: 10.1109/JBHI.2018.2878846
Iber, C., and Iber, C. (2007). The AASM Manual for the Scoring of Sleep and Associated Events: Rules, Terminology and Technical Specifications, Vol. 1. Westchester, IL: American Academy of Sleep Medicine.
Iruthayarajah, J., McIntyre, A., Cotoi, A., Macaluso, S., and Teasell, R. (2017). The use of virtual reality for balance among individuals with chronic stroke: a systematic review and meta-analysis. Top. Stroke Rehabil. 24, 68–79. doi: 10.1080/10749357.2016.1192361
Ischer, M., Baron, N., Mermoud, C., Cayeux, I., Porcherot, C., Sander, D., et al. (2014). How incorporation of scents could enhance immersive virtual experiences. Front. Psychol. 5:736. doi: 10.3389/fpsyg.2014.00736
Jack, K., McLean, S. M., Moffett, J. K., and Gardiner, E. (2010). Barriers to treatment adherence in physiotherapy outpatient clinics: a systematic review. Manual Ther. 15, 220–228. doi: 10.1016/j.math.2009.12.004
Kairy, D., Tousignant, M., Leclerc, N., Côté, A.-M., Levasseur, M., and the Telage Researchers (2013). The patient's perspective of in-home telerehabilitation physiotherapy services following total knee arthroplasty. Int. J. Environ. Res. Public Health 10, 3998–4011. doi: 10.3390/ijerph10093998
Kandalaft, M. R., Didehbani, N., Krawczyk, D. C., Allen, T. T., and Chapman, S. B. (2013). Virtual reality social cognition training for young adults with high-functioning autism. J. Autism Dev. Disord. 43, 34–44. doi: 10.1007/s10803-012-1544-6
Kim, K. (2014). Emotion Modeling and Machine Learning in Affective Computing. Available online at: https://api.semanticscholar.org/CorpusID:239954
Krogmeier, C., Mousas, C., and Whittinghill, D. (2019). “Human, virtual human, bump! A preliminary study on haptic feedback,” in 2019 IEEE Conference on Virtual Reality and 3D User Interfaces (VR) (Osaka: IEEE), 1032–1033. doi: 10.1109/VR.2019.8798139
Krönert, D., Grünewald, A., Li, F., Grzegorzek, M., and Brück, R. (2018). “Sensor headband for emotion recognition in a virtual reality environment,” in International Conference on Information Technologies in Biomedicine (Kamień Śląski: Springer), 539–548. doi: 10.1007/978-3-319-91211-0_47
Lang, P. J., Bradley, M. M., and Cuthbert, B. N. (1997). International affective picture system (IAPS): technical manual and affective ratings. NIMH Center Study Emot. Attent. 1, 39–58.
LaViola, J. J. Jr. (2000). A discussion of cybersickness in virtual environments. ACM Sigchi Bull. 32, 47–56. doi: 10.1145/333329.333344
Levac, D. E., Glegg, S. M., Sveistrup, H., Colquhoun, H., Miller, P., Finestone, H., et al. (2016). Promoting therapists' use of motor learning strategies within virtual reality-based stroke rehabilitation. PLoS ONE 11:e0168311. doi: 10.1371/journal.pone.0168311
Lindeman, R. W., Yanagida, Y., Hosaka, K., and Abe, S. (2006). “The tactapack: a wireless sensor/actuator package for physical therapy applications,” in 2006 14th Symposium on Haptic Interfaces for Virtual Environment and Teleoperator Systems (Alexandria, VA), 337–341. doi: 10.1109/HAPTIC.2006.1627117
Liu, M., Fan, D., Zhang, X., and Gong, X. (2016). “Human emotion recognition based on galvanic skin response signal feature selection and SVM,” in 2016 International Conference on Smart City and Systems Engineering (ICSCSE) (Hunan), 157–160. doi: 10.1109/ICSCSE.2016.0051
Liu, Y., Sourina, O., and Nguyen, M. K. (2011). “Real-time EEG-based emotion recognition and its applications,” in Transactions on Computational Science XII (Heidelberg: Springer), 256–277. doi: 10.1007/978-3-642-22336-5_13
Llinás, R. R. (2014). Intrinsic electrical properties of mammalian neurons and CNS function: a historical perspective. Front. Cell. Neurosci. 8:320. doi: 10.3389/fncel.2014.00320
Lloréns, R., Noé, E., Colomer, C., and Alca niz, M. (2015). Effectiveness, usability, and cost-benefit of a virtual reality-based telerehabilitation program for balance recovery after stroke: a randomized controlled trial. Arch. Phys. Med. Rehabil. 96, 418–425. doi: 10.1016/j.apmr.2014.10.019
Lohse, K. R., Hilderman, C. G., Cheung, K. L., Tatla, S., and Van der Loos, H. M. (2014). Virtual reality therapy for adults post-stroke: a systematic review and meta-analysis exploring virtual environments and commercial games in therapy. PLoS ONE 9:e93318. doi: 10.1371/journal.pone.0093318
Lombard, M., Ditton, T. B., Crane, D., Davis, B., Gil-Egui, G., Horvath, K., et al. (2000). “Measuring presence: a literature-based approach to the development of a standardized paper-and-pencil instrument,” in Third International Workshop on Presence, Vol. 240 (Delft), 2–4.
Lubar, J., Swartwood, M., Swartwood, J., and Timmermann, D. (1995). Quantitative EEG and auditory event-related potentials in the evaluation of attention-deficit/hyperactivity disorder: effects of methylphenidate and implications for neurofeedback training. J. Psychoeduc. Assess. 34, 143–160.
Lubar, J. F. (1991). Discourse on the development of EEG diagnostics and biofeedback for attention-deficit/hyperactivity disorders. Biofeedback Self-Regul. 16, 201–225. doi: 10.1007/BF01000016
Lum, P. S., Uswatte, G., Taub, E., Hardin, P., and Mark, V. W. (2006). A telerehabilitation approach to delivery of constraint-induced movement therapy. J. Rehabil. Res. Dev. 43:391. doi: 10.1682/JRRD.2005.02.0042
Maríin-Morales, J., Higuera-Trujillo, J. L., Greco, A., Guixeres, J., Llinares, C., Scilingo, E. P., et al. (2018). Affective computing in virtual reality: emotion recognition from brain and heartbeat dynamics using wearable sensors. Sci. Rep. 8:13657. doi: 10.1038/s41598-018-32063-4
Marzbani, H., Marateb, H. R., and Mansourian, M. (2016). Neurofeedback: a comprehensive review on system design, methodology and clinical applications. Basic Clin. Neurosci. 7:143. doi: 10.15412/J.BCN.03070208
Mazzoni, A., and Bryan-Kinns, N. (2015). “How does it feel like? An exploratory study of a prototype system to convey emotion through haptic wearable devices,” in 2015 7th International Conference on Intelligent Technologies for Interactive Entertainment (INTETAIN) (Torino), 64–68. doi: 10.4108/icst.intetain.2015.259625
McGee-Lennon, M. R., and Brewster, S. (2011). “Reminders that make sense: designing multimodal notifications for the home,” in 2011 5th International Conference on Pervasive Computing Technologies for Healthcare (PervasiveHealth) and Workshops (Dublin), 495–501. doi: 10.4108/icst.pervasivehealth.2011.246032
Mellecker, R., and McManus, A. (2014). Active video games and physical activity recommendations: a comparison of the Gamercize stepper, XBOX Kinect and XaviX J-Mat. J. Sci. Med. Sport 17, 288–292. doi: 10.1016/j.jsams.2013.05.008
Mertz, L. (2019). Virtual reality is taking the hurt out of pain. IEEE Pulse 10, 3–8. doi: 10.1109/MPULS.2019.2911819
Meuleman, B., and Rudrauf, D. (2018). Induction and profiling of strong multi-componential emotions in virtual reality. IEEE Trans. Affect. Comput. 1–15. doi: 10.1109/TAFFC.2018.2864730
Miller, H. L., and Bugnariu, N. L. (2016). Level of immersion in virtual environments impacts the ability to assess and teach social skills in autism spectrum disorder. Cyberpsychol. Behav. Soc. Network. 19, 246–256. doi: 10.1089/cyber.2014.0682
Miri, P., Flory, R., Uusberg, A., Culbertson, H., Harvey, R. H., Kelman, A., et al. (2020). PIV: Placement, pattern, and personalization of an inconspicuous vibrotactile breathing pacer. ACM Trans. Comput. Hum. Interact. 27, 1–44. doi: 10.1145/3365107
Mohn, C., Argstatter, H., and Wilker, F.-W. (2011). Perception of six basic emotions in music. Psychol.Mus. 39, 503–517. doi: 10.1177/0305735610378183
Morina, N., Ijntema, H., Meyerbröker, K., and Emmelkamp, P. M. (2015). Can virtual reality exposure therapy gains be generalized to real-life? A meta-analysis of studies applying behavioral assessments. Behav. Res. Ther. 74, 18–24. doi: 10.1016/j.brat.2015.08.010
Mousavi Hondori, H., and Khademi, M. (2014). A review on technical and clinical impact of microsoft kinect on physical therapy and rehabilitation. J. Med. Eng. 2014:846514. doi: 10.1155/2014/846514
Nararro-Haro, M. V., Hoffman, H. G., Garcia-Palacios, A., Sampaio, M., Alhalabi, W., Hall, K., et al. (2016). The use of virtual reality to facilitate mindfulness skills training in dialectical behavioral therapy for borderline personality disorder: a case study. Front. Psychol. 7:1573. doi: 10.3389/fpsyg.2016.01573
Obrist, M., Subramanian, S., Gatti, E., Long, B., and Carter, T. (2015). “Emotions mediated through mid-air haptics,” in Proceedings of the 33rd Annual ACM Conference on Human Factors in Computing Systems (Seoul), 2053–2062. doi: 10.1145/2702123.2702361
O'Keefe, J., and Burgess, N. (1999). Theta activity, virtual navigation and the human hippocampus. Trends Cogn. Sci. 3, 403–406. doi: 10.1016/S1364-6613(99)01396-0
O'Nuallain, S. (2009). Zero power and selflessness: what meditation and conscious perception have in common. J. Cogn. Sci. 4, 46–64.
Parsons, T. D., and Rizzo, A. A. (2008). Affective outcomes of virtual reality exposure therapy for anxiety and specific phobias: a meta-analysis. J. Behav. Ther. Exp. Psychiatry 39, 250–261. doi: 10.1016/j.jbtep.2007.07.007
Pearce, P. Z. (2008). Exercise is medicine. Curr. Sports Med. Rep. 7, 171–175. doi: 10.1097/01.CSMR.0000319712.63793.5f
Peiris, R. L., Peng, W., Chen, Z., Chan, L., and Minamizawa, K. (2017). “Thermovr: Exploring integrated thermal haptic feedback with head mounted displays,” in Proceedings of the 2017 CHI Conference on Human Factors in Computing Systems (Denver, CO: ACM), 5452–5456. doi: 10.1145/3025453.3025824
Picard, R. W. (2000). Affective Computing. Cambridge, MA: MIT Press. doi: 10.7551/mitpress/1140.001.0001
Piron, L., Turolla, A., Agostini, M., Zucconi, C., Cortese, F., Zampolini, M., et al. (2009). Exercises for paretic upper limb after stroke: a combined virtual-reality and telemedicine approach. J. Rehabil. Med. 41, 1016–1020. doi: 10.2340/16501977-0459
Rajae-Joordens, R. (2008). “Measuring experiences in gaming and TV applications,” in Probing Experience. Philips Research, Vol. 8, eds J. H. D. M. Westerink, M. Ouwerkerk, T. J. M. Overbeek, W. F. Pasveer, and B. de Ruyter (Dordrecht: Springer). doi: 10.1007/978-1-4020-6593-4_7
Ramirez, R., and Vamvakousis, Z. (2012). “Detecting emotion from EEG signals using the emotive EPOC device,” in International Conference on Brain Informatics (Macau: Springer), 175–184. doi: 10.1007/978-3-642-35139-6_17
Rangaswamy, M., Porjesz, B., Chorlian, D. B., Wang, K., Jones, K. A., Bauer, L. O., et al. (2002). Beta power in the EEG of alcoholics. Biol. Psychiatry 52, 831–842. doi: 10.1016/S0006-3223(02)01362-8
Redd, W. H., Manne, S. L., Peters, B., Jacobsen, P. B., and Schmidt, H. (1994). Fragrance administration to reduce anxiety during MR imaging. J. Magn. Reson. Imaging 4, 623–626. doi: 10.1002/jmri.1880040419
Richman, L. S., Kubzansky, L., Maselko, J., Kawachi, I., Choo, P., and Bauer, M. (2005). Positive emotion and health: going beyond the negative. Health Psychol. 24:422. doi: 10.1037/0278-6133.24.4.422
Rizzo, A., Hartholt, A., Grimani, M., Leeds, A., and Liewer, M. (2014). Virtual reality exposure therapy for combat-related posttraumatic stress disorder. Computer 47, 31–37. doi: 10.1109/MC.2014.199
Rothbaum, B. O., Price, M., Jovanovic, T., Norrholm, S. D., Gerardi, M., Dunlop, B., et al. (2014). A randomized, double-blind evaluation of d-cycloserine or alprazolam combined with virtual reality exposure therapy for posttraumatic stress disorder in Iraq and Afghanistan war veterans. Am. J. Psychiatry 171, 640–648. doi: 10.1176/appi.ajp.2014.13121625
Rus-Calafell, M., Gutiérrez-Maldonado, J., and Ribas-Sabaté, J. (2014). A virtual reality-integrated program for improving social skills in patients with schizophrenia: a pilot study. J. Behav. Ther. Exp. Psychiatry 45, 81–89. doi: 10.1016/j.jbtep.2013.09.002
Salem, Y., Gropack, S. J., Coffin, D., and Godwin, E. M. (2012). Effectiveness of a low-cost virtual reality system for children with developmental delay: a preliminary randomised single-blind controlled trial. Physiotherapy 98, 189–195. doi: 10.1016/j.physio.2012.06.003
Salimpoor, V. N., Benovoy, M., Longo, G., Cooperstock, J. R., and Zatorre, R. J. (2009). The rewarding aspects of music listening are related to degree of emotional arousal. PLoS ONE 4:e7487. doi: 10.1371/journal.pone.0007487
Salminen, K., Surakka, V., Lylykangas, J., Raisamo, J., Saarinen, R., Raisamo, R., et al. (2008). “Emotional and behavioral responses to haptic stimulation,” in Proceedings of the SIGCHI Conference on Human Factors in Computing Systems (Florence), 1555–1562. doi: 10.1145/1357054.1357298
Salovey, P., Rothman, A. J., Detweiler, J. B., and Steward, W. T. (2000). Emotional states and physical health. Am. Psychol. 55:110. doi: 10.1037/0003-066X.55.1.110
Saposnik, G., Levin, M., Group, S. O. R. C. S. W., et al. (2011). Virtual reality in stroke rehabilitation. Stroke 42, 1380–1386. doi: 10.1161/STROKEAHA.110.605451
Schubert, T., Friedmann, F., and Regenbrecht, H. (2001). The experience of presence: factor analytic insights. Presence 10, 266–281. doi: 10.1162/105474601300343603
Schuemie, M. J., Van Der Straaten, P., Krijn, M., and Van Der Mast, C. A. (2001). Research on presence in virtual reality: a survey. CyberPsychol. Behav. 4, 183–201. doi: 10.1089/109493101300117884
Schweizer, T., Renner, F., Sun, D., Kleim, B., Holmes, E. A., and Tuschen-Caffier, B. (2018). Psychophysiological reactivity, coping behaviour and intrusive memories upon multisensory virtual reality and script-driven imagery analogue trauma: a randomised controlled crossover study. J. Anxiety Disord. 59, 42–52. doi: 10.1016/j.janxdis.2018.08.005
Seligman, M. E. P. (2002). “Positive psychology, positive prevention, and positive therapy,” in Handbook of Positive Psychology, eds C. R. Snyder and S. J. Lopez (New York, NY: Oxford University Press), 3–12.
Seth, A. K., Suzuki, K., and Critchley, H. D. (2012). An interoceptive predictive coding model of conscious presence. Front. Psychol. 2:395. doi: 10.3389/fpsyg.2011.00395
Shiban, Y., Schelhorn, I., Pauli, P., and Mühlberger, A. (2015). Effect of combined multiple contexts and multiple stimuli exposure in spider phobia: a randomized clinical trial in virtual reality. Behav. Res. Ther. 71, 45–53. doi: 10.1016/j.brat.2015.05.014
Singer, W., and Gray, C. M. (1995). Visual feature integration and the temporal correlation hypothesis. Annu. Rev. Neurosci. 18, 555–586. doi: 10.1146/annurev.ne.18.030195.003011
Skarbez, R., Brooks, F. P. Jr., and Whitton, M. C. (2017). A survey of presence and related concepts. ACM Comput. Surv. 50, 1–39. doi: 10.1145/3134301
Slater, M. (2009). Place illusion and plausibility can lead to realistic behaviour in immersive virtual environments. Philos. Trans. R. Soc. B 364, 3549–3557. doi: 10.1098/rstb.2009.0138
Sluijs, E. M., Kok, G. J., and Van der Zee, J. (1993). Correlates of exercise compliance in physical therapy. Phys. Ther. 73, 771–782. doi: 10.1093/ptj/73.11.771
Soares, R., Siqueira, E., Miura, M., Silva, T., and Castanho, C. (2016). “Biofeedback sensors in game telemetry research,” in Simpósio Brasileiro de Jogos e Entretenimento Digital (São Paulo), 81–89.
Statista (2020). Forecast Unit Shipments of Augmented (AR) and Virtual Reality (VR) Headsets From 2019 to 2023 (In Millions). Technical report, Statista Research.
Steinicke, F. (2016). “The science and fiction of the ultimate display,” in Being Really Virtual (Cham: Springer). doi: 10.1007/978-3-319-43078-2_2
Straudi, S., Severini, G., Charabati, A. S., Pavarelli, C., Gamberini, G., Scotti, A., et al. (2017). The effects of video game therapy on balance and attention in chronic ambulatory traumatic brain injury: an exploratory study. BMC Neurol. 17:86. doi: 10.1186/s12883-017-0871-9
Sutherland, I. E. (1965). “The ultimate display,” in Multimedia: From Wagner to Virtual Reality, eds R. Packer and K. Jordan (New York, NY: W. W. Norton & Company, Inc.), 506–508.
Sutherland, I. E. (1968). “A head-mounted three dimensional display,” in Proceedings of the December 9-11, 1968, Fall Joint Computer Conference, Part I (ACM), 757–764. doi: 10.1145/1476589.1476686
Sweetser, P., and Wyeth, P. (2005). Gameflow: a model for evaluating player enjoyment in games. Comput. Entertain. 3:3. doi: 10.1145/1077246.1077253
Teigen, K. H. (1994). Yerkes-dodson: a law for all seasons. Theory Psychol. 4, 525–547. doi: 10.1177/0959354394044004
Van Rooij, M., Lobel, A., Harris, O., Smit, N., and Granic, I. (2016). “Deep: A biofeedback virtual reality game for children at-risk for anxiety,” in Proceedings of the 2016 CHI Conference Extended Abstracts on Human Factors in Computing Systems (San Jose, CA: ACM), 1989–1997. doi: 10.1145/2851581.2892452
Vanderwolf, C. (2000). Are neocortical gamma waves related to consciousness? Brain Res. 855, 217–224. doi: 10.1016/S0006-8993(99)02351-3
Waltemate, T., Gall, D., Roth, D., Botsch, M., and Latoschik, M. E. (2018). The impact of avatar personalization and immersion on virtual body ownership, presence, and emotional response. IEEE Trans. Visual. Comput. Graph. 24, 1643–1652. doi: 10.1109/TVCG.2018.2794629
Warnock, D., McGee-Lennon, M., and Brewster, S. (2011). “The role of modality in notification performance,” in IFIP Conference on Human-Computer Interaction (Lisbon: Springer), 572–588. doi: 10.1007/978-3-642-23771-3_43
Westwood, J. D. (2002). Medicine Meets Virtual Reality 02/10: Digital Upgrades, Applying Moore's Law to Health, Vol. 85. Clifton, VA: IOS Press.
Whishaw, I., and Vanderwolf, C. H. (1973). Hippocampal eeg and behavior: change in amplitude and frequency of RSA (theta rhythm) associated with spontaneous and learned movement patterns in rats and cats. Behav. Biol. 8, 461–484. doi: 10.1016/S0091-6773(73)80041-0
Whitham, E. M., Lewis, T., Pope, K. J., Fitzgibbon, S. P., Clark, C. R., Loveless, S., et al. (2008). Thinking activates EMG in scalp electrical recordings. Clin. Neurophysiol. 119, 1166–1175. doi: 10.1016/j.clinph.2008.01.024
Witmer, B. G., and Singer, M. J. (1998). Measuring presence in virtual environments: a presence questionnaire. Presence 7, 225–240. doi: 10.1162/105474698565686
Wolf, D., Rietzler, M., Hnatek, L., and Rukzio, E. (2019). Face/on: multi-modal haptic feedback for head-mounted displays in virtual reality. IEEE Trans. Visual. Comput. Graph. 25, 3169–3177. doi: 10.1109/TVCG.2019.2932215
Yee, N., and Bailenson, J. (2007). The proteus effect: the effect of transformed self-representation on behavior. Hum. Commun. Res. 33, 271–290. doi: 10.1111/j.1468-2958.2007.00299.x
Keywords: immersive virtual reality, virtual reality therapy, immersion, presence, emotion, perception, multimodal displays, biofeedback
Citation: Elor A and Kurniawan S (2020) The Ultimate Display for Physical Rehabilitation: A Bridging Review on Immersive Virtual Reality. Front. Virtual Real. 1:585993. doi: 10.3389/frvir.2020.585993
Received: 22 July 2020; Accepted: 08 October 2020;
Published: 12 November 2020.
Edited by:
Wendy A. Powell, Tilburg University, NetherlandsReviewed by:
Richard Skarbez, La Trobe University, AustraliaYuki Ban, The University of Tokyo, Japan
Copyright © 2020 Elor and Kurniawan. This is an open-access article distributed under the terms of the Creative Commons Attribution License (CC BY). The use, distribution or reproduction in other forums is permitted, provided the original author(s) and the copyright owner(s) are credited and that the original publication in this journal is cited, in accordance with accepted academic practice. No use, distribution or reproduction is permitted which does not comply with these terms.
*Correspondence: Aviv Elor, YWVsb3JAdWNzYy5lZHU=