- Arts et Métiers, Institute of Technology, LISPEN, HESAM Université, UBFC, Paris, France
Augmented Reality (AR) enhances the comprehension of complex situations by making the handling of contextual information easier. Maintenance activities in aeronautics consist of complex tasks carried out on various high-technology products under severe constraints from the sector and work environment. AR tools appear to be a potential solution to improve interactions between workers and technical data to increase the productivity and the quality of aeronautical maintenance activities. However, assessments of the actual impact of AR on industrial processes are limited due to a lack of methods and tools to assist in the integration and evaluation of AR tools in the field. This paper presents a method for deploying AR tools adapted to maintenance workers and for selecting relevant evaluation criteria of the impact in an industrial context. This method is applied to design an AR tool for the maintenance workshop, to experiment on real use cases, and to observe the impact of AR on productivity and user satisfaction for all worker profiles. Further work aims to generalize the results to the whole maintenance process in the aeronautical industry. The use of the collected data should enable the prediction of the impact of AR for related maintenance activities.
Introduction
Context
The aeronautic industry is faced with problems related to the complexity of maintenance documentation. Workers are overloaded with all the information necessary to perform each maintenance task on each equipment configuration while needing to apply the right procedures according to the regulatory structure (EASA, 2019). Aeronautics is a demanding sector because the safety of millions of passengers is at stake every day (IATA, 2018). Aircrafts are made up of advanced products and equipment optimized to ensure essential advanced technological functions while best meeting numerous constraints. Rigorous procedures must be followed to achieve the right quality standards. Aeronautical maintenance workers have to cope with the complexity and the variety of equipment, as well as the quality requirements that must be met.
This complexity is currently a challenge for the sector, especially when considering the recruitment of new employees. Today's experienced workers have developed their skills and knowledge over time with the evolution of the industry. They have had over 30 years to learn how to work effectively with the existing tools and instruction manuals. However, aeronautical maintenance activities are now constrained by a constantly expanding aeronautics sector (IATA, 2018) and the renewal of the aging workforce (MRO Survey, 2017). This increases the need to quickly recruit and train new novice operators who cannot assimilate all the required knowledge quickly enough. Support for maintenance instructions must evolve to make it easier to find and understand the right information for each task. The objective is for the new operators to be operational at the best possible level and to support them in improving their skills. The aim is to improve the overall productivity of beginners from the start.
AR seems to be a tool well suited to the current needs of aeronautical maintenance activities (Figure 1). AR tools improve situational awareness by superimposing digital information on the real world, in the right place and at the right time (Azuma, 1997). A user with a well-adapted AR tool has access to a better and faster understanding of situations. It is becoming an essential tool in various professional fields, such as culture, entertainment, medicine, retail, and industry (Van Krevelen and Poelman, 2010). Relevant AR use cases are demonstrated over a wide range of specific conditions and there is a drive to expand AR applications to more common and widespread tasks (Fuchs, 2019).
Scientific and Technical Problem
It is necessary to assess the impact of using AR to assist operators in aeronautical maintenance under real working conditions in order to determine the impact on these activities and to support subsequent deployment. AR tools can simplify access to useful information and instructions by providing filtered items based on current working tasks. It facilitates understanding by displaying data and models of equipment, tools, and manipulations directly on the physical work environment (Caudell and Mizell, 1992). Digital mediums make interactions between users and data more efficient (Mura et al., 2016). This explains why the implementation of AR tools to help workers on aeronautical maintenance activities seems interesting.
However, there is little feedback on the real extent of what this technology could concretely bring to aeronautical maintenance activities. The existing methods and criteria for evaluating AR are not suitable for the aeronautical maintenance environment. There is no real use case to experiment with and no current method for developing AR tools for aeronautical maintenance activities. Thus, the scientific and technical problem studied in this article is:
• How to set up and conduct field evaluations of the impact of AR tools for workers in aeronautical maintenance activities?
The first step is to acquire a clear vision of the criteria needed to evaluate AR and to understand the aeronautical maintenance environment to build the right criteria, taking into account the context of use. The next step is to develop AR tools based on real tasks for evaluation purposes. The final step is to conduct experiments with workers on the selected tasks to assess the impact of the AR tools on those tasks with the selected criteria. The three questions we investigate in this article are:
• Q1: How should we evaluate the impact of AR on aeronautical maintenance tasks?
• Q2: How should we deploy an AR tool to assist in performing aeronautical maintenance tasks?
• Q3: What is the added value of AR on aeronautical maintenance tasks?
This paper is structured as follows. State of the Art – AR Evaluation Criteria for Aeronautical Maintenance presents a state-of-the-art study on the evaluation of AR tools and discusses aeronautical maintenance constraints and needs to identify the evaluation criteria. Materials and Equipment details the materials and equipment used to deploy the AR tool and conduct the evaluation of AR impact. Methods describes the method for selecting the evaluation criteria suitable for aeronautical maintenance, taking into account the constraints in the field, the method for selecting the maintenance use cases to be evaluated, the method for deploying the AR tools on these tasks, and discusses the evaluation of the impact of AR with the selected criteria. Results presents the results of the implementation of an experiment conducted to evaluate the impact of AR compared to current practices on field tasks, the results obtained, and the associated analysis. Finally, Discussion ends the paper with a discussion of the work and an opening to further research on the subject.
State of the Art – AR Evaluation Criteria for Aeronautical Maintenance
AR is defined by Azuma (1997) as a technology that combines interactive virtual elements with the real world both spatially, in three dimensions, and temporally, in real time. This technology brings digital data directly to users in context, allowing them to focus more on physical tasks and gain a better understanding of situations (Caudell and Mizell, 1992). AR relies on software capable of recognizing and tracking elements of the real environment (2D, 3D, plan, and geolocation) to place and maintain virtual elements in the correct physical position (Kin and Dey, 2009). This AR software works on different types of equipment capable of acquiring the data necessary for localization, and of displaying digital elements directly (projectors or see-trough glasses) or indirectly (screen) (PWC, 2019).
In industrial fields, AR can be used throughout the lifecycle of a product for design, planning, manufacturing, inspection, and maintenance activities (Fite-Georgel, 2011). Reviewing recent studies on the subject, Quandt et al. (2018) identified general requirements for the development of industrial applications. These tasks include product and plant design, operator training, production assistance, logistical support, and remote maintenance. The requirements relate to all elements impacted by the applications, from cost and security in integration to installation, accuracy, and reliability in use. Palmarini et al. (Palmarini et al., 2017) ranked the different areas identified for the application of AR in industry. They detected a great interest in AR applications in the aviation industry and for maintenance activities such as assembly, repair, inspection, and training. Eschen et al. (2018) investigated the potential use of VR and AR to support operators in the aviation industry. They highlight that the most suitable technology for process guidance in maintenance is AR due to the high number of manual work interactions between the operators and the real parts.
Evaluation of AR Tools
Studies presenting evaluations of AR tools approach the evaluation from different perspectives. The topic can be divided into three types of assessments, which relate to technology, the use of AR instead of other tools, and the perspective of users.
The first theme on AR assessment concerns comparisons on the technology itself. With a systematic review of the literature, Palmarini et al. (Palmarini et al., 2018) identified a set of main characteristics that are compared in most studies. It includes hardware, the development platform, tracking techniques, and interaction methods. Baumeister et al. (2017) compared the possibility offered by the hardware for procedural tasks by evaluating the average response time to AR indications. Renner and Pfeiffer (2017) compared visualization methods for guiding purposes by measuring mean time to action and mean head movement during tasks.
The second research theme is the evaluation against other tools in a controlled environment (Werrlich et al., 2017). Webel et al. (2013) compared AR to video for training. Rios et al. (2013) compared AR to paper instructions for troubleshooting. Likewise, Syberfelt (2015) evaluates the AR guidance for assembly. Fiorentino et al. (2014), testing large-screen AR devices vs. paper instructions, distinguished time from tasks where AR may or may not be useful. Blaga et al. (2017) used a virtual environment to measure gesture accuracy with freehand gesture interactions in terms of reaction time, action time, and accuracy. Gavish et al. (2015) observed a reduction of unsolved errors on industrial maintenance tasks after AR training. In most cases, these experiments are conducted under laboratory conditions and on specific tasks where the conditions facilitate the identification and measurement of the comparison criteria to highlight the differences between the solutions.
The third theme related to AR assessment research is the impact on the users. The tools used have an impact on the way in which people work on the task and therefore the level of commitment necessary for the work and felt by the user through the task. This concept of cognitive load (Paas, 1992) can be assessed using quantitative or qualitative observations. For quantitative observations, direct solutions are to measure users' physiological values before and during the task, such as brain activation, or to perform dual task measurements by adding another task to do alongside the original task (Brunken et al., 2003). The evolution between the two situations indicates the cognitive load induced by the task.
For qualitative observations, Hornbaek (2006) evaluated different solutions and concluded that standard questionnaires are better than homemade questionnaires. Rubio et al. (2004) compared subjective methods of assessing mental workload and identified the NASA-TLX questionnaire for predicting user performance on tasks. Hart and Staveland (1988) defined the NASA-TLX (task load index) through years of workload assessment research. Users are asked to rate the six elements defining workload on a rating scale after the task. The task’s average workload score can be used for performance forecasting; a low value is related to better performance. Brooke et al. (1996) worked on usability to define the System Usability Scale questionnaires (SUS), focused on how the user felt about the tools during the task with ten questions scored on a five-point Likert scale. This score can be used to compare the usability of different types of solutions. Bangor et al. (2009) analyzed hundreds of studies using the SUS and linked the SUS score to the acceptability rating of a system through percentile rank comparison. The validity of the NASA-TLX and SUS subjective questionnaires in predicting objective impacts on performance has been advanced by the compilation of hundreds of retrospective studies more than 20 years after their construction (Hart, 2006; Brooke, 2013).
Constraints, Needs, and Evaluation in Aeronautical Maintenance
The objective of aeronautical maintenance is to meet maintenance needs adapted to the aeronautical sector through processes such as MSG-3 (Lugan, 2011). This reliability-driven process is designed to ensure that aircraft safety and reliability levels are maintained or to restore them to an optimum level after deterioration and obtain the data necessary to improve the design at minimal cost. To achieve these objectives, maintenance activities encompass numerous procedures which punctuate the life of all aeronautical equipment and are adapted to the service life, flight cycles, and direct observations. Certain circumstances only occur a few times during the life of the equipment, resulting in a low frequency of performing certain maintenance tasks on the product. The changes create a new configuration of the equipment and therefore different procedures to follow, which has an impact on the complexity of maintenance manuals. Workers have little time and opportunity to learn work instructions for all possible equipment configurations.
Regarding the needs of the aeronautical maintenance activities, Martinetti et al. (2017) specify that it is necessary to collect and use a significant amount of information on standard procedures, specific both to the different tasks required and to each piece of equipment to perform maintenance tasks. As each product may go through a maintenance process at a different time in its life, each product may be different from the other, making them even more difficult to work with than in production where each component is the same. The needs are to identify the products, to collect the right information necessary for the accomplishment of the task, to interpret the documentation, to understand the instructions, and possibly to verify the result and to validate the good execution of the task. These needs are not yet met with current tools, but AR tools seem to provide solutions.
In addition to these needs, many new constraints are applied to aeronautical maintenance activities with the growth of the aeronautical industry worldwide (MRO Survey, 2017). This growth accelerates the need to find solutions to maintain quality and increase productivity on tasks while improving the efficiency of operator training in various working conditions. In addition, the variability in the type and configurations of the equipment maintained makes it impossible to memorize information relating to each task, and the requirements of the aeronautical industry make it necessary to be certain that the correct information has been used. Various indicators are used to assess aeronautical maintenance at different scales. On a large scale, the most important factor is reliability, measured by metrics such as the mean time to failure. However, these elements drive maintenance globally and are unrelated to tasks performed during maintenance, which are monitored by other criteria. Certain criteria related to operator safety are considered in these assessments.
The major criterion linked to maintenance activities is the level of quality. Improper execution of the maintenance instructions can affect the product, meaning it must be discarded that part of the maintenance cycle must be redone. Parts can even be damaged during tasks, which is considered as non-quality. This leads to significant financial impacts related to the cost and time of repairing or repurchasing parts and impacts the overall maintenance cycle through the time required to resume maintenance on the parts concerned and the delay of subsequent maintenance steps. The second main criterion related to maintenance activity is the time required to perform the maintenance tasks. Equipment maintenance deadlines are contractually defined between the owners of the equipment and the maintenance facility. These times must be respected as best as possible to avoid penalties and maximize flight time. The delays that can accumulate at each stage of maintenance impact the delivery date. All these requirements will be considered when selecting and assessing the impact of AR in aeronautical maintenance.
In addition, aeronautical maintenance activities are also assessed in terms of productivity. From this point of view, the main aim for the evaluation is to observe the evolution of performance due to the use of AR. The criteria used must highlight the direct impact of AR on quality indicators and time to complete tasks. However, this would overlook the other benefits of AR on operators. Jetter et al. (2018) identified other KPIs (Key Performance Indicators) on the integration of AR systems in automotive maintenance through subjective analysis and questionnaires. They found that the perceived ease of use of AR solutions is as important as reducing the time and errors to conclude on the usefulness of AR.
Materials and Equipment
The material and equipment used in this study were selected according to the methodology detailed in Methods. They are divided between the aeronautical maintenance equipment used to perform a selected maintenance task and the AR equipment.
The selected maintenance task is an assembly task with many steps that can be improved with current AR tools. The environment study and the interview with the maintenance operators permitted the selection of eight complex sub-tasks, from which a need for a different instruction format has been identified. The equipment used to perform the task (Figure 2) consists of a list of mechanical parts to be installed, the axle to be positioned on the assembly, and bolts to be tightened. This list is completed with the tools necessary to install the parts, the corresponding wrenches to tighten the bolts, and the protective grease to be applied to the parts. Without AR, work instructions are provided through standard work documents in a paper format containing the information needed for assembly. It consists of an overview of the equipment, standardized textual instructions, and detailed images for some sub-tasks.
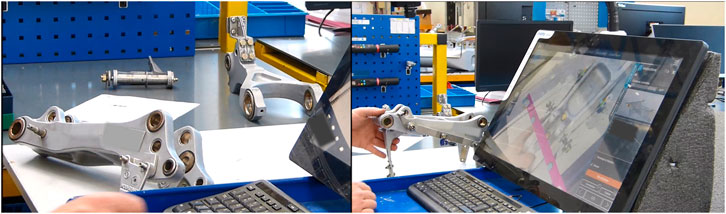
FIGURE 2. Pictures of the equipment, AR app, and AR workstation used in the study for experimentation in the work environment.
The AR tool was deployed with the industrial AR software solution Diota V2.3.0 (Diota, 2019). Diota is connected to the Catia Composer R2017X 3D creation software for the creation of content for the AR application. Diota Player software is used to play content created on selected hardware. The hardware is a shop floor workstation running the Windows 10 operating system equipped with a 27-inch touchscreen and an industrial HD camera. This configuration is installed on a standard mobile desk to facilitate integration into the workshop (Figure 2). This mobile workstation can be moved for installation on the assembly line to test the AR application in the real environment without disrupting the workflow. AR instructions are provided through the industrial AR application. For each subtask, the main part is tracked in 3D, and an AR work card overlays models of other parts in position, part reference numbers, and standardized text instructions.
Methods
The methodology that drives our work on the use of AR on aeronautical maintenance tasks is summarized in Figure 3. The main question on the impact of AR can be solved by experiments on the aeronautical maintenance task to evaluate the added value of AR. Setting up the experiments requires two previous steps to be carried out. One step is to select the evaluation criteria according to the aeronautical maintenance task (left side of the figure). The other step is to deploy an AR tool on a use case in the real work environment, similar to current practices (right side of the figure).
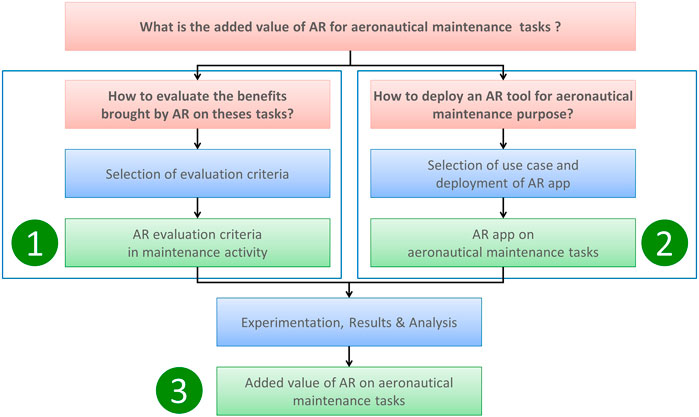
FIGURE 3. Methodology to set up and conduct field evaluation of the impact of AR tools for workers for aeronautical maintenance activities.
Selection of Evaluation Criteria
The criteria best suited to our study are selected by applying the constraints of industrial activity to the list of criteria for evaluating AR previously established, as summarized in Figure 4. The impact on the productivity of the activities of maintenance is assessed by comparing the time taken to understand instructions, task action time, and overall time required for the task. To fully assess the integration of the AR tool, this observation is supplemented by the evaluation of the impact for the workers with two questionnaires: NASA-TLX for the measurement of the cognitive load and SUS for the usability of the AR solution.
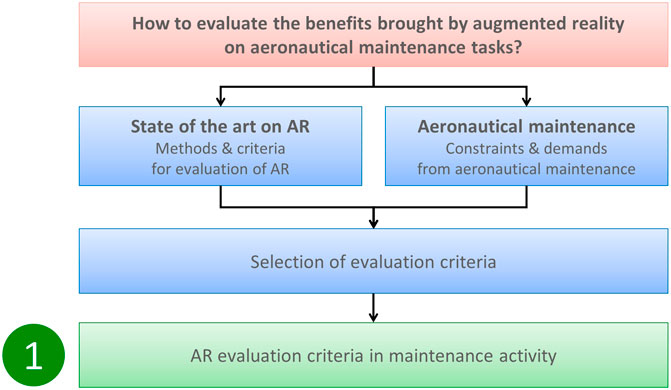
FIGURE 4. Methodology to select criteria for evaluation of the impact of AR for aeronautical maintenance.
Proposition for AR Evaluation
Table 1 summarizes the observation methods (col.2) for the evaluation of AR from different points of view (col.1). These are applicable and relevant for comparing AR to other solutions under specific and controlled conditions (col. 3), but not all are suitable for use in the field on real maintenance tasks. However, the needs and constraints of aeronautical maintenance require the selection of criteria observable in industrial conditions and relevant for the deployment of AR in this activity.
Selection in Accordance with Constraints
AR aims to save time and reduce quality issues on maintenance tasks, as it makes it easier to understand and access the right information. Regarding maintenance tasks, it is necessary to measure the impact on current tasks with evaluation criteria linked to performance measurements on these activities. Productivity is evaluated in terms of the quality and the time required to complete the task. Regarding AR, intrusive measurements, which can be done in the laboratory, cannot be carried out in the field. Workers must remain free to perform tasks without additional constraints.
The solution chosen is to use criteria without any particular installation constraints, suitable for use in a real workplace (see Table 1). This concerns questionnaires on the impact on the user and the criteria for comparing tools through the average time to complete a task and the average quality of the result of the task. This makes it possible to collect results both on the process side via productivity indicators and on the user appropriation side with questionnaires on cognitive load and usability.
Summary of the Selected Criteria
According to the indicators identified in the previous sub-steps, the most suitable structure for the classification of criteria is based on the concept of usability described with the three elements of the ISO 9241–11 (ISO, 1998) standard:
• Effectiveness, the ability of users to accomplish tasks using the system that matches the selection of tasks where AR is applicable with potential benefit.
• Efficiency, which relates to the level of resources consumed in the performance of tasks. It is linked to performance indicators on maintenance tasks which include measuring task execution time and evaluating the error rate on tasks.
• Satisfaction, which includes subjective feedback on the use of the system through the measurement of user acceptance and cognitive load with SUS and TLX questionnaires.
The vision through the notion of usability highlights two essential elements for the evaluation: the impact on the maintenance operation through efficiency criteria and the acceptance of AR by users measured with satisfaction indicators.
Time Measurement Values
AR has a different impact on the stages of understanding information and the stages of working on parts. The duration of each sub-task has been divided into “Understanding Time” (TU) and “Action Time” (TA). TU is the time used by the participant to research, understand, and translate instructions from support to actual parts. TA is the time taken by the participant to carry out the instructions on the equipment.
Calculation of Total Time Savings
We define the “Time Gain” (TG) quantity to assess the added value of AR on performance on sub-tasks, with TAR the recorded time “with AR” and TCS the recorded time “without AR.” TG is the comparison of the time used for each phase calculated with the following formula:
Understanding/Action Ratio Calculation
We define the “U/A ratio” (or RUA) quantity to assess the distribution of the time needed to find and understand the instructions (TU) and the time needed to perform the actions (TA) against the total time (TT) needed to accomplish the task or sub-task. It is calculated with the following formula:
Implementation of an AR Tool to Assist Aeronautical Maintenance Tasks
Details on Aeronautical Maintenance
The first element of the deployment method (Figure 5) is to work on maintenance activities. These activities occur at different points in the life cycle of the equipment and can be light unplanned interventions for the replacement of sub-equipment or heavy planned interventions requiring specific resources and skills, such as overhauls or modifications. The second type, identified as depot level, is more suitable for AR tools due to the complexity of the tasks and the variety of equipment covered. This level of maintenance can only be performed by accredited Maintenance, Repair, & Overhaul (or MRO) workshops and relates to major repairs and a wide variety of tasks leading to a complete overhaul of the equipment (Figure 6).
The fundamentals of these tasks are different and require different skills and resources, but there are also similarities in the overall sequence of action associated with each task. Each task requires a reference to standard instructions describing the elements required to perform the task (tools, grease, etc.), the task itself, and the way to validate the execution. This study focuses on the use of AR content on the shop floor and the help it provides operators on MRO tasks. It could be extended to other industrial maintenance activities dealing with the same constraints and needs as MRO tasks (low frequency, high quality requirements, many configurations of complex products). It does not explore the part of creating and managing maintenance data, which is also impacted by the use of AR tools, even if the use of digital and identified content should bring benefits to this type of task, in particular in terms of content updates.
Selecting the Maintenance Task for Creating AR Content
The deployment process of the AR application is divided into seven stages, presented in Figure 7. The study of user needs concerns the elements that make the use of AR interesting and refers to the selection of Maintenance, Repair, and Overhaul activities previously detailed. These are activities that involve quantity, complexity, and a variety of tasks. Then, the deployment of AR is impacted by certain elements of the work environment, such as the need to be hands-free, to be mobile, to use specific tools, or to work in specific areas, that induce choices for hardware and software. These elements allow selecting a use case to deploy AR among all the MRO activity tasks. Then software and hardware can be selected for our AR applications and content can be created. Each step includes reviews of the deployment with users to detect errors or missing information until a final review validates the latest version of the application.
Selection of Activities
For the same task, the complexity varies according to the type of equipment and the sub-tasks. As tasks involve practical work and knowledge, it is essential to work with the experts to select the right use cases. Demonstrating AR on an operation well known to users helps them to extrapolate to their daily activities. It makes it possible to collect feedback from the field, identify specific complex tasks, and select use cases according to needs. The assembly tasks on the sub-equipment before final assembly were selected because they consist of multiple sub-tasks and therefore require a significant amount of information to be found, understood, and translated into actions for each step. Once the use case has been selected, it is necessary to observe the current working environment to identify which AR solution is the most relevant and to choose a suitable one according to the constraints detailed in the previous chapter (Figure 8).
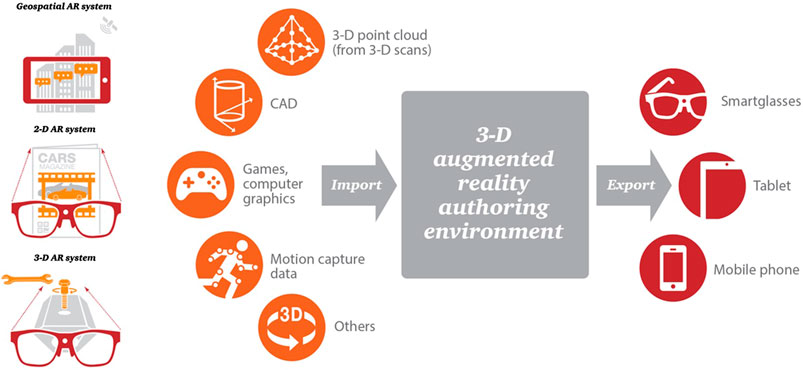
FIGURE 8. AR solutions (hardware and software possibilities) (PWC, 2019).
Software Selection
Regarding the software, workshop conditions and the aeronautical environment advise against the use of marker recognition. This would require the development of specific tools and procedures to install it. The maintenance tasks consist of working on mechanical parts that qualify the recognition based on 3D models. The Diota software solution (Diota, 2019) was selected because it uses 3D model-based recognition that is able to accurately track mechanical parts to overlay 2D or 3D data on work cards. It avoids the installation constraints imposed by other types of AR recognition and makes the solution relevant for industrial use in aeronautics. The authoring solution enables the use of existing 3D models of design parts for the creation of static or animated work cards that facilitate application deployment.
Hardware Selection
Hardware takes many forms, from computers or tablets to the more unusual like glasses or projectors, each with their own advantages and disadvantages. Deciding between hardware forms is affected by the necessary mobility, the possibilities of manual handling, the software available, and the availability of the equipment for use in an industrial environment. The selected AR software can be used on hardware running the Windows 10 operating system such as a PC, tablet, HoloLens V1, or specific Diota projector. The projector was rejected because the way the information was displayed was not relevant to the use case and its volume. HoloLens have a small field of view, low autonomy, and require time for learning interactions. The tablet requires handling the device with one or both hands, which is problematic when it is necessary to interact with parts. A PC equipped with a touch screen and an industrial mobile camera was selected and installed on a standard mobile work desk used in the workshop.
Implementation Process for AR Application
The final step is to create the content for the AR application itself using data from the current process, AR development tools, and feedback from maintenance operators. Current documentation provides regulatory information and key points to consider. AR development tools help organize each step around reference pieces that connect the virtual and physical world. Existing 3D models from the design are used for visual instructions and implemented into the 3D working environment to make using AR natural.
Reviews and Validation With the Users
The review of the first version of the application with the maintenance operators makes it possible to detect errors due to a misinterpretation of the current documentation or to the identification of missing information necessary for the task. This also makes it possible to verify the correct arrangement of the elements. The authoring process continues by applying the changes and iterating with the operators until the final version fixes the content of the AR application for industrial use. It can be introduced into the maintenance process to train operators, conduct experiments, and evaluate AR.
Results
Measurement Protocol
The experiment was carried out on an assembly task divided into eight sub-tasks of similar complexities to observe the impact of AR on this type of task under industrial conditions. Participants completed the task in the factory workflow under two conditions, one with current paper media used by workers and one with AR media available on a mobile workstation. Completion time was recorded, and participants’ comments and feedbacks were assessed through questionnaires immediately after the task. The material and equipment have been detailed previously in Materials and Equipment.
Participants
Nine participants were recruited from among the workshop operators to participate in the study. Six participants completed the eight sub-tasks under the two conditions (“without AR” and “with AR”) and three participants completed them only under the condition “with AR” before completing the two qualitative questionnaires. None of them had prior knowledge of AR technology. The participants were divided into three groups (“Beginner”, “Advanced”, or “Expert”) according to their knowledge of the task and of maintenance (Table 2).
Conditions
Two conditions were evaluated on the assembly line in the actual work area. For the condition “without AR,”, there were few adaptations compared to the current process. For the “with AR” condition, workers used the mobile workstation shown in Materials and Equipment. The “without AR” instructions consisted of standard working documents containing the information needed for assembly. It consisted of an overview, standardized textual instructions, and detailed images for each sub-task.
Procedure
A demonstration application unrelated to the observed task was presented to explain the possibilities of AR and how it works. The experimental conditions, the questionnaires, and the measurements were presented to the participants before volunteering. Before the experiment, the observer prepares the parts needed for assembly, the current support for the task, the AR application, questionnaires, and support for time measurements. Participants are reminded of the conditions of the experiment. Under “with AR” conditions, the participant goes through a brief overview of the controls. The mobile workstation is installed in the workspace and calibrated for part recognition.
The experience begins when the observer, participant, support, and equipment are ready. The participant navigates through the medium to find the information and perform the eight assembly sub-tasks. The observer writes down the understanding and action time required for the participant to accomplish each sub-task. After the experiment, the participant fills out the NASA-TLX questionnaire to assess the cognitive load induced on him by performing the task with the associated support. He also completes the SUS questionnaire to assess the usability of the AR media.
Raw Results
The results of the experiment are divided into two types. The quantitative results concern the evaluation of the impact of AR on the performance of workers on the job. The qualitative results concern the evaluation of the cognitive load induced on the users using each instructional medium and the subjective evaluation of the usability of the AR application by the workers. The results are divided by experience conditions (“with AR” and “without AR”) and by participant profile (“Beginner,” “Advanced,” or “Expert”) for each sub-task.
Quantitative Performance Measures – Process Side
The results are summarized in Table 3 and presented in Figure 9 for “total time saved” and in Figure 10 for the RUA calculated with Eqs. 1, 2 of Selection of Evaluation Criteria.
Considering all the user profiles together, five of the nine subjects on each of the eight subtasks, we get 40 measurements under both conditions. Per sub-task, workers spent an average of 30% less time on the understanding phase and 16% more time on the action phase, which leads to 9% saved in time total per sub-task. RUA shows a gain of 14 points between the current support and the AR tool.
By separately considering the beginner, advanced, and expert profiles on each of the eight sub-tasks, we obtain respectively 16, 16, and 8 measurements under the two conditions. By observing the average value per sub-task, workers spend less time on the understanding phase (25, 39, and 15%) and more time on the action phase (16, 27, and 38%) which leads to a total time saving per sub-task (7, 11, and 2%) for all worker profiles. RUA shows a gain for each profile of respectively 11 points, 17 points, and 14 points between the current support and the AR tool.
Qualitative User Related Measures – User Side
The results of the NASA-TLX and SUS questionnaires are presented in Figure 11, respectively on the left side and on the right side. The results of the advanced and expert users are grouped together. Due to major differences, the results for beginners are separated from the results for advanced and expert users. The results of the questionnaires show a reduction in cognitive load and an increase in worker satisfaction.
Analysis of Results
The distribution of the worker profiles for experience corresponds to the overall distribution of skills in the workshop. The goal of AR is to help workers find and understand instructions to make it easier to perform maintenance tasks. According to their knowledge, workers are not at the same level of ease in the tasks. It is important to observe all the profiles to measure the differences between them and to determine the impact of the AR on the workshop with different skill distributions.
Quantitative Performance Measures
Through total time saving and RUA, we observe an overall positive impact of AR (Figures 9, 10). Average results across all users show a total time saving of 9%. This is of high value in aeronautical processes. By taking all profiles independently, we also observe a saving on the average total time for the sub-tasks for each profile. Thus, when the distribution of profiles changes in the future, with more advanced workers, AR will continue to save total time on the overall workshop. Even with a low impact on expert users (2% of total time savings), there is little risk of wasting time with AR.
By comparing the beginner and advanced profiles, the impact of AR is greater on the advanced profiles (respectively, 7 and 11%), which was not expected. The assumption was that AR will be of less benefit to a user with more knowledge. This difference can be explained by the fact that beginners are not familiar with the process and take more time to link the instructions together, while the advanced instructions they are looking for and AR gives them easier and intuitive access to this information. Future beginners will directly benefit from AR and with practice they will become advanced, which will amplify the added value of AR. It could also promote versatility in workshops where advanced workers could move more easily between many tasks.
The distinction between understanding and action phases highlights important elements. We observe a positive impact of AR on understanding time: a gain of 30% considering all profiles. This confirms that AR has added value on the task by facilitating the task of processing instructions for aeronautical maintenance. However, a negative impact of AR is perceived on action time: 16% loss on the total time considering all the profiles. The hypothesis is that the use of a new, unknown device slows down actions and could induce a change in behavior where the user tends to verify more information thanks to the proximity of instructions and actions in AR. It could provide more benefits by anticipating the detection of errors before the end of the job, thus reducing the need for rework and increasing the quality throughout the process.
The added value of AR is also visible through the Understanding/Action ratio which is increased by 14 points considering all users. This means that workers around the world are spending less time processing instructions before every action using AR. The comparison of the evolution of the ratio for the different user profiles shows that the impact is greater for advanced than for beginner users.
Qualitative User-Related Measures
The SUS and TLX scores show positive feedback on the use of AR (Figure 11). Considering all the profiles, the usability of the AR media was rated 7.5 points better than the current media. Transposing these results into percentile rank (PR) means that the support of the task went from a good tool to an excellent tool (67.8–84.6 SUS PR). The impact of AR is also visible on cognitive load. It decreased between current support and AR support (TLX score 34.2–26.8). This means that less cognitive load is required to process AR instructions, allowing users to focus on performing tasks with greater efficiency.
To analyze in more detail the impact of AR on users, we have combined the measurements on advanced and expert users. Both profiles have knowledge and practice on the current instruction medium, as beginners still need to learn about the current instruction medium. The usability scores given by advanced and expert users are similar under both conditions and correspond to the best evaluation (over 85 points). For them, AR media is as usable as the current media that they are familiar with.
Beginners, on the other hand, gave a lower score under both conditions. This is because they are less comfortable with the maintenance process. However, we see a significant difference between the current support, which is just OK (SUS score of 57.5), and the AR application (SUS score of 72.5). This indicates a good usability of the application when converting to percentile rank. For beginners, there is a visible improvement in the usability of the support with AR medium.
This trend is the same for TLX measurements. Advanced and expert users tend to have a lower cognitive load on tasks than beginners, and the reduction of TLX score due to AR is greater for beginners. From a user perspective, AR has a greater impact for workers new to the maintenance process than for workers already familiar with current support.
Discussion
Method Development
In this work, we investigated the impact of AR tools on the performance of aircraft maintenance tasks from a process and worker perspective. The preparation of this evaluation was divided into three questions to be studied.
The first question (Q1) was to determine how to evaluate the advantages brought by AR on aeronautical maintenance tasks. The objective was to identify criteria not only suitable for evaluating the use of AR to assist a user in performing a task, but also adapted to the constraints of the aeronautical maintenance environment. Among the criteria and the AR evaluation methods, we selected those applicable in the workshop without disrupting the execution of maintenance tasks (Table 1). During the process, we selected performance indicators related to tasks, such as the non-quality detected or the overall time of the tasks, but also highlighted the specificities of the tasks that should be affected differently by the AR. For user feedback, we selected two questionnaires, one evaluating the cognitive load felt by workers while performing the task and the other focusing on the usability of the AR solution compared to the current medium.
The second question (Q2) was to determine how to deploy an AR tool for assistance with maintenance tasks dealing with quantity and a variety of complex products with high quality requirements and low frequency, such as aeronautical activities. To apply the criteria selected in an experiment, we had to deploy an AR solution covering the same information as the current media for the same task. We established a process (Figure 7) based on knowledge of the available AR technology (hardware and software), when it is useful, and the knowledge of experienced workers on aeronautical maintenance (activities needs and work environment constraints). This made it possible to focus on specific maintenance tasks and to deploy an AR application with appropriate content according to the instructions currently used by the workers, along with their comments on the tasks. 3D-model based recognition software and screen-based AR hardware were identified as most suited for an AR app used in aeronautical maintenance context.
The third question (Q3) was to measure the added value of AR on aeronautical maintenance tasks through field experiences, based on the elements selected and prepared with the two previous questions. Thanks to the criteria and the AR application, we were able to conduct experiments with AR in real conditions in the maintenance process line without disturbing the workers. The experiment was carried out on the workshop population, grouping together three different profiles (Table 2, 3), and over a long period. The results of the experiment provide answers on the added value of AR for all worker profiles on the tasks.
Conclusion
The creation of the AR application for experimentation following the deployment process highlighted the most relevant design choices for an AR application in this industrial context. Guidance tasks involving a high number of parts and specific information were identified for AR deployment. The use of 3D models-based recognition allows the use of AR directly on parts without complicating the task preparation. A mobile workstation equipped with a touch screen and a camera is sufficient for AR interaction and can be effectively integrated with operators in the work environment.
Considering all the user profiles, the experience highlights a gain brought by AR through each criterion. Compared to current support, the AR application received a better usability score from users, which echoes a better relationship between understanding phases and action phases for each sub-task. AR makes it easier to access and understand instructions so users can more easily focus on the action phase. A parallel can be drawn with the cognitive task load score which decreases with AR. Likewise, the total time required to complete the task is reduced with the AR tool. This confirms the hypothesis that the impact of AR is visible from the workers’ point of view and that AR is useful for aeronautical maintenance from a process point of view.
The comparison between the profiles highlights different impacts of AR according to the users. At first, beginners will benefit more from the AR solution (due to its good usability and reduction of cognitive load), which could facilitate their training. Then, the observation that emerges is that the advantage will increase in terms of productivity with AR tools in the hands of advanced workers familiar with the maintenance process. And it does not interfere with the work of experts who are as comfortable with AR as they are with the current media. However, the negative impact of the application of AR on the action phase should be reduced by better adapting the content and devices of AR to the needs of the workers. This observation on user profiles will be further investigated through additional experiments with more users per profile to confirm the results.
Limitations
Some limitations need to be addressed. One is the constraints on the evaluation criteria; the criteria had to be usable in the workshop without disturbing the workers and easily deployable so as to carry out experiments on the maintenance lines. It is closer to the actual impact of AR on tasks, but it limits observations. Another is the unsystematic selection of use cases based on current opportunities with AR tools and on the experience of shop floor workers to relate AR functionality to complex tasks with which they need assistance. There were also constraints on the feasibility and frequency of the experiments because aeronautics works on long cycles. The low availability of equipment for the chosen task, as well as the small number of subjects in the workshop, reduced the number of observations even over a long period. Due to this limitation, the potential long-term impact of AR on the level of general maintenance knowledge could not yet be investigated in this study. However, the nature of the activity requires users to rely on instructions for specific information about products with each support.
Perspectives
The added value of AR has been identified on selected tasks and this result should be extended to other tasks not yet addressed by this work using the established methodology. Improved interactions between the user and the AR (data capture and processing) could have an impact on other stages of the maintenance process. It is necessary to be able to generalize the choice of activities. Further work will consist of using these elements to extend the results to the AR impact assessment over the entire aeronautical maintenance process and to surpass the limits of feasibility and low frequency of experiments. The goal is to identify the features of the tasks which affect the impact of AR to generalize the results of limited on-field experiments. A classification of maintenance activities related to the specific needs of workers will lead to prediction of the value that AR would bring to each activity.
Data Availability Statement
The original contributions presented in the study are included in the article/Supplementary Material, further inquiries can be directed to the corresponding author.
Ethics Statement
Ethical review and approval was not required for the study on human participants in accordance with the local legislation and institutional requirements. Written informed consent for participation was not required for this study in accordance with the national legislation and the institutional requirements.
Author Contributions
QL, FD, FA, and FM contributed to the conceptualization of the research goals and aims. QL, FD, FA, and FM contributed to the design of the methodology of the study. FD, FA, and FM provided resources for the study and supervision of the research activity. QL deployed the elements used to perform the experiments, collected the data and performed the analysis. QL, FD, FA, and FM contributed to the interpretation of the analysis. QL wrote the first draft of the manuscript. All authors contributed to manuscript revision, read, and approved the submitted version.
Conflict of Interest
The authors declare that the research was conducted in the absence of any commercial or financial relationships that could be construed as a potential conflict of interest.
Acknowledgments
The content of this manuscript has been presented in part at the 16th EuroVR International Conference on Virtual Reality and Augmented Reality, EuroVR 2019, Tallinn, Estonia, October 23–25, 2019. Loizeau Q., Danglade F., Ababsa F., Merienne F. (2019) Evaluating Added Value of Augmented Reality to Assist Aeronautical Maintenance Workers—Experimentation on On-field Use Case. In: Bourdot P., Interrante V., Nedel L., Magnenat-Thalmann N., Zachmann G. (eds) Virtual Reality and Augmented Reality. EuroVR 2019. Lecture Notes in Computer Science, vol 11883. Springer, Cham. https://doi.org/10.1007/978-3-030-31908-3_10.
References
Bangor, A., Kortum, P., and Miller, J. (2009). Determining what individual SUS scores mean: adding an adjective rating scale. Journal of Usability Studies 4, 114–123. doi:10.5555/2835587.2835589
Baumeister, J., Ssin, S. Y., ElSayed, N. A. M., Dorrian, J., Webb, D. P., Walsh, J. A., et al. (2017). Cognitive cost of using augmented reality displays. IEEE Trans. Visual. Comput. Graph. 23, 2378–2388. doi:10.1109/TVCG.2017.2735098
Blaga, A. D., Frutos-Pascual, M., Al-Kalbani, M., and Williams, I. (2017). “Usability analysis of an off-the-shelf hand posture estimation sensor for freehand physical interaction in egocentric mixed reality,” in Adjunct proceedings of the 2017 IEEE international symposium on mixed and augmented reality, ISMAR-adjunct 2017, Nantes, France, October 9–13, 2017.
Brooke, J. (1996). SUS - A quick and dirty usability scale. Usabil. Eval. Indus. 194, 189–194. doi:10.1201/9781498710411-35
Brooke, J. (2013). SUS: a retrospective. Journal of Usability Studies. 8, 29–40. doi:10.5555/2817912.2817913
Brunken, R., Plass, J. L., and Leutner, D. (2003). Direct measurement of cognitive load in multimedia learning. Educ. Psychol. 38 (1), 53–61. doi:10.1207/S15326985EP3801_7
Caudell, T., and Mizell, D. (1992). “Augmented reality: an application of heads-up display technology to manual manufacturing processes,” in Proceedings of the Twenty-Fifth Hawaii International Conference on System Sciences, Kauai, HI, January 7–10, 1992.
Diota, (2019). Solutions 4.0 for Industry. Available at: http://diota.com/index.php/en/(Accessed June 14, 2019).
EASA Regulations. Available at: https://www.easa.europa.eu/regulations (Accessed June 14, 2019).
Eschen, H., Kötter, T., Rodeck, R., Harnisch, M., and Schüppstuhl, T. (2018). Augmented and virtual reality for inspection and maintenance processes in the aviation industry. Procedia Manufacturing 19, 156–163. doi:10.1016/j.promfg.2018.01.022
Fiorentino, M., Uva, A. E., Gattullo, M., Debernardis, S., and Monno, G. (2014). Augmented reality on large screen for interactive maintenance instructions. Comput. Ind. 65, 270–278. doi:10.1016/j.compind.2013.11.004
Fite-Georgel, P. (2011). “Is there a reality in industrial augmented reality?,” in 10th IEEE International Symposium on Mixed and Augmented Reality, ISMAR 2011, October 26, 2011 (IEEE).
Fuchs, H. (2019). The XR Future - the coming utopia or a gamer's plaything. Keynote. Available at: https://www.youtube.com/watch?v=lyX_0gcixf4 (Accessed January 05, 2019).
Gavish, N., Gutiérrez, T., Webel, S., Rodríguez, J., Peveri, M., Bockholt, U., et al. (2015). Evaluating virtual reality and augmented reality training for industrial maintenance and assembly tasks. Interact. Learn. Environ. 23 (6), 778–798. doi:10.1080/10494820.2013.815221
Hart, S. G. (2006). “NASA-task load index (NASA-TLX); 20 years later.” in Proceedings of the human factors and ergonomics society 50th annual meeting—2006, Moffett Field, CA, October 1, 2006.
Hart, S. G., and Staveland, L. E. (1988). Development of NASA-TLX (task load index): results of empirical and theoretical research. Adv. Psych. 52, 139–183. doi:10.1016/S0166-4115(08)62386-9
Hornbaek, K. (2006). Current practice in measuring usability: challenges to usability studies and research. Int. J. Hum. Comput. Stud. 64, 79–102. doi:10.1016/j.ijhcs.2005.06.002
IATA (2018). Press Release No.: 62. Available at: https://www.iata.org/pressroom/pr/Pages/2018-10-24-02.aspx (Accessed June 14, 2019).
ISO. (1998). Exigences ergonomiques pour travail de bureau avec terminaux à écrans de visualisation (TEV) - partie 11: lignes directrices relatives à l'utilisabilité. ISO. (11), 9241.
Jetter, J., Eimecke, J., and Rese, A. (2018). Augmented reality tools for industrial applications: what are potential key performance indicators and who benefits?. Comput. Hum. Behav. 87, 18–33. doi:10.1016/j.chb.2018.04.054
Kin, S. J., and Dey, A. K. (2009). Simulated Augmented reality windshield display as a cognitive mapping aid for elder driver navigation. Boston, MA: CHI 2009 – Navigation.
Lugan, G. (2011). L’élaboration de la maintenance aéronautique à travers la méthodologie MSG-3. Master Thesis. Dumas.
Martinetti, A., Rajabalinejad, M., and Dongen, L. V. (2017). Shaping the future maintenance operations: reflections on the adoptions of augmented reality through problems and opportunities. Procedia CIRP. 59, 14–17. doi:10.1016/j.procir.2016.10.130
MRO Survey (2017). When growth outpaces capacity. Available at: https://www.oliverwyman.com/our-expertise/insights/2017/apr/mro-survey-2017.html (Accessed June 14, 2019).
Mura, M. D., Dini, G., and Failli, F. (2016). An integrated environment based on augmented reality and sensing device for manual assembly workstations. Procedia CIRP. 41, 340–345. doi:10.1016/j.procir.2015.12.128
Paas, F. G. W. C. (1992). Training strategies for attaining transfer of problem-solving skill in statistics: a cognitive-load approach. J. Educ. Psychol. 84, 429–434. doi:10.1037/0022-0663.84.4.429
Palmarini, R., Erkoyuncu, J. A., and Roy, R. (2017). An innovative process to select augmented reality (AR) technology for maintenance. Procedia CIRP. 59, 23–28. doi:10.1016/j.procir.2016.10.001
Palmarini, R., Erkoyuncu, J. A., Roy, R., and Torabmostaedi, H. (2018). A systematic review of augmented reality applications in maintenance. Robot. Comput. Integrated Manuf. 49, 215–228. doi:10.1016/j.rcim.2017.06.002
PWC (2019). How will people create content for augmented reality?. Available at: https://usblogs.pwc.com/emerging-technology/how-will-people-create-content-for-augmented-reality/ (Accessed June 14, 2019).
Quandt, M., Knoke, B., Gorldt, C., Freitag, M., and Thoben, K. D. (2018). General requirements for industrial augmented reality applications. Procedia CIRP. 72, 1130–1135. doi:10.1016/j.procir.2018.03.061
Renner, P., and Pfeiffer, T. (2017). “Augmented reality assistance in the central field-of-view outperforms peripheral displays for order picking: results from a virtual reality simulation study,” in Adjunct Proceedings of the 2017 IEEE International Symposium on Mixed and Augmented Reality, ISMAR-Adjunct 2017, Nantes, France, October 9–13, 2017.
Rios, H., González, E., Rodriguez, C., Siller, H. R., and Contero, M. (2013). A mobile solution to enhance training and execution of troubleshooting techniques of the engine air bleed system on boeing 737. Procedia Computer Science 25, 161–170. doi:10.1016/j.procs.2013.11.020
Rubio, S., Diaz, E., Martin, J., and Puente, J. M. (2004). Evaluation of subjective mental workload: a comparison of swat, NASA-TLX, and workload profile methods. Appl. Psychol.: An International Review. 53 (1), 61–86. doi:10.1111/j.1464-0597.2004.00161.x
Syberfeldt, A., Danielsson, O., Holm, M., and Wang, L. (2015). Visual assembling guidance using augmented reality. Procedia Manufacturing. 1, 98–109. doi:10.1016/j.promfg.2015.09.068
Van Krevelen, D. W. F., and Poelman, R. (2010). A survey of augmented reality technologies, applications and limitations. Int. J. Virtual Real. 9 (2), 1–20. doi:10.20870/IJVR.2010.9.2.2767
Webel, S., Bockholt, U., Engelke, T., Gavish, N., Olbrich, M., and Preusche, C. (2013). An augmented reality training platform for assembly and maintenance skills. Robot. Autonom. Syst. 61, 398–403. doi:10.1016/j.robot.2012.09.013
Keywords: augmented reality, field evaluation, criteria, use-case selection, maintenance, aeronautic, industry, deploy
Citation: Loizeau Q, Danglade F, Ababsa F and Merienne F (2021) Methodology for the Field Evaluation of the Impact of Augmented Reality Tools for Maintenance Workers in the Aeronautic Industry. Front. Virtual Real. 1:603189. doi: 10.3389/frvir.2020.603189
Received: 05 September 2020; Accepted: 14 December 2020;
Published: 25 January 2021.
Edited by:
Hirokazu Kato, Nara Institute of Science and Technology (NAIST), JapanReviewed by:
Frank Guan, Singapore Institute of Technology, SingaporeGershon Dublon, Massachusetts Institute of Technology, United States
Copyright © 2021 Loizeau, Danglade, Ababsa and Merienne. This is an open-access article distributed under the terms of the Creative Commons Attribution License (CC BY). The use, distribution or reproduction in other forums is permitted, provided the original author(s) and the copyright owner(s) are credited and that the original publication in this journal is cited, in accordance with accepted academic practice. No use, distribution or reproduction is permitted which does not comply with these terms.
*Correspondence: Quentin Loizeau, cXVlbnRpbi5sb2l6ZWF1LnByb0BnbWFpbC5jb20=