- Julius Maximilian University of Wurzburg, Human-Technique-Systems, Wurzburg, Germany
The design and evaluation of assisting technologies to support behavior change processes have become an essential topic within the field of human-computer interaction research in general and the field of immersive intervention technologies in particular. The mechanisms and success of behavior change techniques and interventions are broadly investigated in the field of psychology. However, it is not always easy to adapt these psychological findings to the context of immersive technologies. The lack of theoretical foundation also leads to a lack of explanation as to why and how immersive interventions support behavior change processes. The Behavioral Framework for immersive Technologies (BehaveFIT) addresses this lack by 1) presenting an intelligible categorization and condensation of psychological barriers and immersive features, by 2) suggesting a mapping that shows why and how immersive technologies can help to overcome barriers and finally by 3) proposing a generic prediction path that enables a structured, theory-based approach to the development and evaluation of immersive interventions. These three steps explain how BehaveFIT can be used, and include guiding questions for each step. Further, two use cases illustrate the usage of BehaveFIT. Thus, the present paper contributes to guidance for immersive intervention design and evaluation, showing that immersive interventions support behavior change processes and explain and predict 'why' and 'how' immersive interventions can bridge the intention-behavior-gap.
Introduction
The road to hell is paved with good intentions. We all know that the translation from intending to change a behavior to successfully changing it often fails. We all have experienced what researchers call the intention-behavior-gap, value-behavior-gap, attitude-behavior-gap, or the knowledge-behavior-gap (Gollwitzer and Bargh, 1996; Sheeran, 2002; Whitmarsh, 2009; Carrington et al., 2010; Ajzen, 2011) (first row of Figure 1).
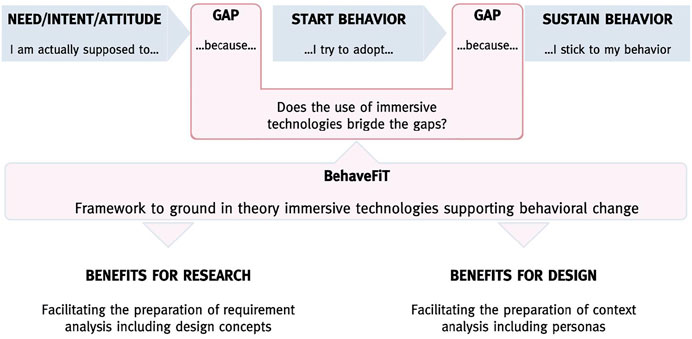
FIGURE 1. The teaser figures shows the contribution of the Behavioral Framework of Immersive Technologies - BehaveFIT.
While intentions may be the best predictor of behavior, on average they account only for 28% of the variance in future behavior, connoting that there may be other factors that predict or rather inhibit a successful behavior change (Sheeran, 2002).
Indeed, the field of psychology has a long tradition of explaining “why” the gap occurs, including a wide range of behavior determinants (e.g., Kollmuss and Agyeman, 2002; Davis et al., 2014; Gifford et al., 2018). However, the extensive quantity of determinants operating on different levels may impede the design, the evaluation, or the comparison of intervention applications, particularly for researchers from other fields. Since behavior change is challenging, assisting technologies that strive to support behavior change, have become an essential line of research within the human-computer interaction (HCI) research in general (Klasnja et al., 2011; Stawarz et al., 2015), and within the field of immersive interventions (mainly virtual reality applications) in particular (e.g., Kuo, 2015; Ahn et al., 2016; Sherman and Craig, 2018; Riva et al., 2019). After referring to the user's perceptions, the immersive technologies' features reveal many possibilities that could support behavior change. However, the description of why these features and possibilities may facilitate behavior change processes and how they are related to the determinants known from psychology often remains phenomenological (Stawarz and Cox, 2015; Riva et al., 2019). Accordingly, the lack of transfer between the disciplines results in a lack of predicting models between the features and possibilities of immersive technologies and the psychological behavior determinants, which further results in a lack of theoretical based prediction for the design and evaluation of immersive interventions.
In the present paper, we aim to use research from other disciplines to explain why and how immersive interventions support behavior change processes. According to the claim for theoretical research contributions raised by Wobbrock and Kientz (2016), we present a framework that targets 1) the summation of a variety of models describing and predicting behavior change from a psychological perspective. The summation results in an intelligible categorization of determinants and barriers. It further targets 2) the summation of the most common features and possibilities of immersive technologies from the human-computer interaction (HCI) perspective. This summation focuses on features of immersive technologies that might be relevant to support behavior change processes. While the first two aims contribute to the why, our final scope is 3) to form generic predicting paths that show how the immersive features and possibilities affect the psychological determinants and how they impact behavior change processes. Thus, both summation results will be mapped on to each other - the features and possibilities of immersive technologies will be mapped on to the categorization of the psychological determinants and barriers.
Consequently, the framework enables theory-driven predictions for the designing and evaluating of immersive intervention technologies designed to support behavioral changes. Figure 1 illustrates the value of the framework when immersive technology has been applied to bridge the intention-behavior-gap.
To achieve these aims, we first provide an overview of the psychological research that explains why the intention-behavior-gap occurs by introducing barriers that impede the adoption of new behavior. In the next step, we introduce intervention mechanisms based on so-called behavior change models that aim to explain how the intention-behavior-gap can be overcome. The psychological introduction is followed by the summation of research from the field of HCI, focusing on immersive interventions. Hence, thirdly we discuss the potentials of different features of immersive technologies that indicate “why” they can support behavior change. Finally, we illustrate the currently available empirical indicators of 'how' immersive technologies can support behavior change. After the theoretical overview (i.e., summations), we introduce the framework BehaveFIT, including the developing methods and results referring to the theoretical section. The benefits and limitations of the framework are discussed by considering the design and evaluation of immersive interventions supporting behavioral changes. Finally, two use cases illustrate the use of the framework.
Related Work
The Psychology of Why the Intention-Behavior-Gap Occurs
While addressing slightly different barriers, the intention-, value-, attitude- or the knowledge-behavior-gap are roughly summarized as the intention-behavior-gap as follows. The intention-behavior-gap describes the failure of the translation from intending to change a behavior to successfully changing it (Gollwitzer and Bargh, 1996; Kollmuss and Agyeman, 2002; Sheeran, 2002; Whitmarsh, 2009; Carrington et al., 2010; Ajzen, 2011; Schwarzer, 2014). The focus of psychological research on the intention-behavior-gap depends on the respective behavioral domain. Nonetheless, some generally valid barriers explaining this failure have been identified. The current section gives an overview of them but raises no claim of completeness. For a comprehensive presentation of the variety of the psychological barriers, we further refer to the work of Blake (1999), Kollmuss and Agyeman (2002), Cane et al. (2012), and Gifford et al. (2018).
Blake (1999) has identified three barriers; individuality, responsibility, and practicality. Individuality refers to barriers lying within the person such as attitudes, personality traits, or predispositions. Responsibility, or rather irresponsibility, refers to the lack of control or perceived influence on the situation. It correlates to distrust or disbelief in the need for change or a low expectancy of self-efficacy. Practicality refers to constraints lying outside the person, including limited resources or facilities, for example.
Other researchers have identified demographic factors, external factors, and internal factors that prevent people from changing their behavior (Kollmuss and Agyeman 2002). Demographic factors include gender and education. External factors entail institutional (e.g., infrastructure to use public transport to change one’s environmental behavior), economic (e.g., monetary benefit), social, and cultural factors (e.g., social norms). Internal factors include motivation (primary motives like altruism or immediate selective motives like comfort), value (that are shaped by, e.g., experiences, role models, education), knowledge, attitude (positive or negative valence toward the behavior), awareness (that underlies cognitive limitations, for example negative consequences are often slow and gradual, and thus not immediately tangible), emotional involvement (e.g., reactance, reduction of dissonance, feeling of helplessness), locus of control (perceived ability to influence the behavior), and responsibility and priorities (e.g., conflicts between contradicting wishes).
More recently (Gifford et al., 2018), have identified six categories of psychological barriers, including most of the categories mentioned above: no need to change, conflicting goals and aspirations, interpersonal relations, government and industry, tokenism, and lacking knowledge. No need to change describes the conviction that a behavior change is unnecessary due to denying the problem or believing it will resolve itself. Gifford et al. (2018) subsumed several barriers in this category: disbeliefs include mistrust, denial, and reactance. Broader belief systems (ideologies) include technosalvation, suprahuman control, and system justification. Limited cognition includes a lack of self-efficacy, optimism bias, and confirmation bias. Conflicting goals and aspirations represent conflicts between goals related to the intended behavior change and other goals, and encompasses costs, including financial investment or behavioral momentum, and perceived risks like unprofitable time investment. Interpersonal relations include the fear that significant others will disapprove of the changed behavior and subsume barriers related to social norms, social comparison, and social risks. Government and industry represent the lack of responsibility of individuals due to the belief that the industry or the government should take charge and include, among others, the perceived program inadequacy barrier. Tokenism refers to the beliefs of people who already adopted some steps of the intended behavior change and believe that they have done enough. While tokenism itself describes that easy changes tend to be chosen over action with higher effort, the rebound effect involves diminished or erased actions by subsequent actions. Lacking knowledge describes when people are paralyzed by their lack of knowledge about selecting the correct action, how to undertake the action, and the relative benefits of different actions. Gifford et al. (2018) subsumed barriers stemming from limited cognition like ignorance or numbness; and perceived risks like functional risks.
Desideratum 1 for the framework development. The psychological barriers explain “why” the intention-behavior-gap occurs. Perceived distances to approval or role models, and especially to tangible consequences, prevent people from starting or sustaining behavior change processes. However, although we already presented a condensed overview, it is hard to find starting points to design or evaluate immersive interventions from existing classifications and descriptions. Consequently, we identify the need for an intelligible categorization of those barriers to provide a guidance, particularly for non-psychologists.
The Psychology of How the Intention-Behavior-Gap Can Be Overcome
The mere description of why does not allow for predicting how the interventions deploy their effectiveness. Hence, this section sums up the psychological models and taxonomies that include the relations between the barriers, their occurrences within the change process, or milestones that accompany a successful change process. Theory-driven approaches based on so-called behavior change models and structured taxonomies contribute to improved research on behavioral interventions (see also guidelines of Byrne, 2020). Because of this, those approaches seem to be a promising starting point for improvement of immersive interventions. Note again, our selection raises no claim to completeness.
Behavior Change Models
Davis et al. (2014) provided a review of different theories of behavior change. Among the most frequently cited theories are The Health Belief Model HBM, (Becker and Maiman, 1975), the Theory of Planned Behavior (TPB, Ajzen, 1980), the Transtheoretical Model TTM, (Prochaska and Velicer, 1997), the Social Cognitive Theory (SCT, Bandura, 1977), and the Health Action Progress Approach HAPA, (Schwarzer, 2014).
Fear Appeal Models
Fear appeal determines behavior change mainly by the perceived threat of consequences of the original behavior linked directly to a behavior change. One of the essential models in fear appeal models is the HBM (Becker and Maiman, 1975). Here, the fear of consequences is linked with the weighing up of costs and benefits of a potential behavior change and some demographic variables.
Motivational Models
The area of motivational models goes beyond the idea of fear as a single motivator for behavior change. They include a variety of the barriers mentioned in section above and link them to behavior change. For example, the TPB (Ajzen, 1980) introduced the perceived behavior control, i.e., the perceived difficulty to perform the new behavior, the perceived behavior norms, and personal behavior attitudes as motivators and barriers in behavior change. Similarly, the SCT (Bandura, 1977) introduced motivators that contribute to behavior change initiation. The SCT emphasizes the social context and their self-efficacy expectations for implementing the behavior change. Motivational models focus on the intention to change behavior and the initiation of new behavior. They mainly illustrate a connection of motivators to behavior change rather than offering mechanisms for long-term implementation of change or the development of coping strategies with relapses.
Stage models
Addressing the problem of initiation and maintaining new behavior, stage models divide the behavior change process into a pre-defined number of behavioral changes. The stages contain specific mechanisms contributing to the transition between stage and classifications of persons regarding their progress. For example, the TTM (Prochaska and Velicer, 1997) describes six stages: precontemplation (awareness-raising), contemplation (decision-oriented), preparation (preparing the initiation of the target behavior), action (initiation of the target behavior), maintenance (maintaining the target behavior over time) and termination (automatic execution of the behavior). A drawback of these stage models is that the strict division into stages suggests a linear temporal course that does not reflect real behavior changes. Additionally, the length of the stages (e.g., maintenance = 6 months) seems arbitrary and could not be verified empirically (Bridle et al., 2005).
Integrative Models
Finally, some models go beyond pure motivation, which overcomes the fixation on development stages. Besides the expectation of results and risk perception, the HAPA (Schwarzer, 2014), for example, emphasized the role of self-efficacy expectation (similar to the SCT). However, the model goes beyond the formation of intentions. It also includes different types of self-efficacy during action and coping, planning and behavior implementation, and evaluation. Besides, the barriers mentioned above and behavior change are included as risk factors in the transition from planning to action maintenance. Like the stage models, a definition of user groups is possible (Non-Intender, Intender and Active). However, the change between the stages is less arbitrarily defined and instead depends on various personal and situational variables forming the motivation within each stage and allowing for relapses.
Taxonomy of Behavior Change Techniques
Additional support in the development of behavioral evaluations is provided by the “Taxonomy of Behavior Change Techniques” by Michie et al. (2013). This taxonomy comprises a summary of 93 types of behavior change techniques, which are grouped into 16 categories. The categories of behavior change techniques proposed by Michie et al. (2013) are scheduled consequences, reward and threat, repetition and substitution, antecedents, associations, covert learning, natural consequences, feedback and monitoring, goals and planning, social support, comparison of behavior, self-belief, comparison of outcomes, identity, shaping knowledge, and regulation.
In addition to the psychological barriers describing the why, this classification gives an overview of how existing interventions work. Hence, it offers the possibility for immersive interventions to refer to already empirically tested behavioral techniques. For example, to address a psychological barrier in the context of interpersonal relations, a comparison between interventions with a focus on social support or comparison of behavior might be appropriate to refer to. To overcome the barrier of the lack of self-efficacy expectation, surveying interventions in the category of self-belief or identity could be supportive.
Identification of Interactions and Moderators
To correctly interpret the outcome of an intervention, it is necessary to consider the role of confounding variables moderating intervention effects. Byrne (2020) roughly groups such moderators into three categories: temporal intervention context, mode of delivery, and individual characteristics. Inspired by a commentary by Scholz (2019), the temporal context of an intervention has been addressed by several researchers (e.g., Bolger and Zee, 2019; Rothman, 2019) in recent years. Discussions include, among others, the time of day, the time of year or time within the process of behavior change that the intervention is applied, or the cycle of repetitions. Additionally, the mode of delivery (Wilson et al., 2020) or the spatial context can also influence the outcome of a behavior change intervention. These variables include the choice of medium compared to in-person interventions that might be particularly relevant for immersive interventions. The third group of moderators is the characteristics of the target person (Byrne, 2020). These include both individual personality traits and the socio-ecological characteristics of a person. They can be controlled by carefully defining the intervention’s target group (see also Wienrich and Gramlich, 2020).
Desideratum 2 for the framework development. Several models predict behavior change processes, thus guiding the design of interventions, identifying influencing factors, controlling moderators and confounding variables, and defining target groups. Taxonomies of behavior change techniques offer additional classification and orientation. However, immersive interventions are rarely based on those models, and taxonomies doubting their quality (Byrne, 2020). Consequently, we identified the need for a predictive framework linking immersive interventions to the theoretical base of behavior change.
The Potentials of Immersive Technologies - Why Can They Support Behavior Change?
Immersive technologies have many potential ways to support behavior change processes since they refer to perceptions of users. The following section summarizes the most promising features considering behavior change and the corresponding perceptional links (see Table 1).
Immersion and Presence
Beyond doubt, the terms immersion and presence are inextricably linked with immersive technologies. In contrast to other fields of research (e.g., media communication), a long debate in the field of HCI ended in the agreement that immersion stands for what the technology delivers in all sensory and tracking modalities and that it can be objectively assessed. In contrast, presence can be defined as a human reaction to a system of a certain level of immersion and thus describes a subjective state (Slater, 1999, Slater, 2003). Further research identified different aspects of presence such as social presence (explained below), telepresence, spatial presence or being there, physical presence, self-presence, or place illusion, and plausibility illusion (for a systematic review, see Skarbez et al., 2017). Immersion and (spatial) presence could probably be considered as so-called “hygiene factors” that are necessary to a certain extent to allow other potentials of immersive technologies (e.g., self-presentation) to become effective, but whose occurrence alone does not lead to the intended behavioral effects. It might be conceptualized similarly to the role of pragmatic quality within the field of user experience (Hassenzahl et al., 2010).
Self-Representation and the Illusion of Virtual Body Ownership
One exclusive feature of VR is the sense of embodiment elicited by the appearance of a virtual alter ego–an avatar. Much research has shown that the virtual embodied representation can influence the user’s self-perception (see 2.3.1). The corresponding perceptions are mainly based on the illusion that the virtual representation is part of one’s physical body, called the sense of embodiment (Kilteni et al., 2012) or the illusion of virtual body ownership (IVBO). The sense of embodiment occurs with the corresponding phenomenological senses of self-location: the spatial experience of being inside a body, agency: the experience of controlling a body, and ownership: self-attribution of a body (Kilteni et al., 2012). Recently, the sense of change was added to describe further the IVBO (Rothman, 2019). The IVBO depends on anthropomorphism and the predictability and synchronicity of the avatar’s movements regarding the user’s physical movements (Maister et al., 2015). One behavior-relevant phenomenon that is closely related to the effects of virtual self-representation is the Proteus effect. It describes a change in behavior according to behavior users attributed to their virtual representation, even after they left the virtual simulation (Ratan et al., 2020; Yee and Bailenson, 2007).
The potential of self-representation and particularly the corresponding sense of embodiment or rather the illusion of virtual body ownership refers to personalizing of any (immersive) experience or making any (immersive) experience literally tangible.
Other- and Context-Representation
Similarly to self-representation, other users can be virtually embodied and varied in their appearance and behavior as social partners. The representation of real or artificial others gains in relevance when users start to interact with virtual others. Consequently, not only the appearance and behavior of interaction partners are crucial for a possible behavior change, but also the representation of interaction or social context (e.g., number of interaction partners, presenting similarities or differences, features of group processes, social status, social interdependence) (Wienrich et al., 2018). Similarly to the definition of spatial presence as a consequence of immersion, others’ inclusion and representation can lead up to a sense of social presence or co-presence. Social presence can be defined as the “sense of being with another” (Biocca and Harms, 2002, p. 456). In the context of assessing the social presence, the sub-dimensions of empathy, involvement, and feelings have been described (De Kort et al., 2007). The sense of social presence mainly depends on sensory factors (e.g., visual representation of others, interactivity), or on the social context (e.g., physical proximity, social cues) (Oh et al., 2018) as well as on the task-related social interdependence between the actors (e.g., avatars) (Wienrich et al., 2018). The representation of others refers to the potential of regulating and simulating social aspects within a behavior change process.
Virtual Objects and Environmental Cues
The design space of the situational context in VR is almost limitless. Any object, any feature of objects and any relation between objects and between objects and persons are modifiable. In particular, decoupling time and space from natural physical laws has been identified as a supportive aspect of behavioral change (Ahn et al., 2015; Sherman and Craig, 2018). For example, consequences can be directly elucidated, and users can be transported to any location or into (or out of) any context.
Potentials referring to manipulating the object-representation and the environment’s representation are promising features to influence spatial or temporal aspects and factors of context on behavior change.
Desideratum 3 for the framework development. Immersive technologies (mainly investigated in VR) show features with a high potential to support the behavioral change process. The corresponding perceptions occurring during or after an immersive experience seem promising to design personalized and socially relevant interventions overcoming psychological barriers preventing people from starting or sustaining behavior change processes. However, these features are rarely related to the psychological barriers leading up to a lack of clarity about whether, why, and how they affect human behavior change. Consequently, we identified the need for relating the features and the corresponding perceptions of immersive technologies to the psychological barriers impeding behavior change.
Empirical Indicators of How Immersive Technologies Can Support Behavior Change
This section sums up empirical results indicating how immersive interventions support behavior change processes. Since no models or taxonomies exist in this area, our report relies on empirical results.
A large proportion of investigations on immersive technologies and behavior change address the use of virtual exposure therapy in the treatment of clinical phobias or use exposure therapy to treat addictions (for reviews see: Morina et al. (2015); Powers and Emmelkamp (2008). The main focus of these applications is on coping with negative emotions or addictive cravings that are triggered by specific stimulus situations. The further potential for immersive technologies to promote new behavioral patterns is not addressed in these therapeutic applications.
However, recent studies have examined how the potential of immersive technologies mentioned above can be used to induce behavior change in non-clinical settings.
The Effect of Immersion on Behavior Change
Some studies focus on the feature of immersion itself as a trigger for behavior change. For example, Ahn et al. (2014) examined the extent as to which the immersion of an intervention technology affects pro-environmental behavior intentions. The results revealed higher environmental behavior intentions in a highly immersive VR condition than a lower immersive printed or 2D video condition. A follow-up study demonstrated that the environmental intentions correlated with the degree of interactivity within an immersive application (Ahn et al., 2015). To further identify how immersion impacts behavior change, some scientists consider the mediating role of corresponding perceptions mentioned above. One example of such an approach is the third series of experiments by Ahn et al. (2016). The studies embodied people in different virtual animals and revealed that presence did not have a mediating effect between immersion and behavior change, but the illusion of virtual body ownership. Similar results have been shown in other studies (Herrera et al., 2018; Ahn et al., 2019).
We can learn from these and similar studies that immersion is one crucial feature. However, other features and corresponding perceptions also explain important variance of the effects of immersive interventions on behavior change.
The Effect of Self- or Context-Presentation on Behavior Change
Other studies addressed the feature of self-presentation or context-representation on behavior change. Fox and Bailenson (2009), Kim et al. (2014), and Kuo (2015) provided feedback on behavioral consequences presented on one's virtual self-representation. Fox and Bailenson (2009) showed that an embodied avatar could increase behavioral intentions in exercising behavior when it is modified according to the user's current exercising performance. Also, Kim et al. (2014) and Kuo (2015) showed that modified virtual self-representation could significantly influence the motivation to change one's health behavior.
Additional to feedback on their avatar, the virtual environment can impact behavioral change. In an experiment by Soliman et al. (2017), the characteristics of the presented environment, either as natural or artificial, impacted pro-environmental attitudes. In the natural environment, the users felt more connected to nature and pro-environmental behavior than in a virtual urban environment. Likewise, Ahn et al. (2015) showed that behavior is influenced by the environmental framing of an immersive message. Mediated by increased self-efficacy, using positive or negative environmental cues significantly impacted behavioral intentions, with positive framing leading to the higher intention of paper saving behavior than negative. Other experiments, like the work of Hsu et al. (2018) showed that feedback on the impact of behavior patterns on the environment can help to motivate behavioral intentions.
The research on one’s self- or context-representation shows that besides the degree of immersion, other modifiable features such as the type of feedback or the framing of the situation are significant modifiers to support behavior change.
Transfer Between Virtual and Real Behavior
A frequently addressed problem in research on the effectiveness of clinical VR exposure therapy is whether the results of virtual intervention can be transferred to perceptions and behavior in the non-virtual world (i.e., the real world). The field of exposure therapy revealed several results that show a transfer of these effects, both in the short-term and the long-term after leaving the immersive environment (Morina et al., 2015; Powers and Emmelkamp, 2008). In addition to exposure therapy, the Proteus effect (described above) indicates that behavioral experiences during the immersive experience are also transferred to real behavior in both the short term and the long term after the experience (see for a recent review: Ratan et al., 2020).
However, in line with Stawarz and Cox (2015), some results also show the limitation of being more motivated than truly exhibiting changed behavior. For example, Kim et al. (2014) found that the positive effects of immersive interventions rapidly declined when the immersive system was not available for further exercises. The authors drew the conclusion that immersive interventions had an initial motivating effect but not a sustaining effect on real behavior.
Desideratum 4 for the framework development. Many studies from different behavioral domains (e.g., therapy, environment, health) show that immersive interventions can support behavior change. The empirical results revealed that different features manipulating immersion can influence the behavior change, that besides immersion, other features take effect on behavior change, and immersive interventions can be transferred to real behavior after the intervention. However, some studies presented conflicting results (despite the fact, we are not considering the publication bias). Further, only few of them provide detailed information of the why and how by linking the features with corresponding perceptions and psychological determinants and barriers. Consequently, we identified a need for predicting more precisely why and how immersive interventions help to overcome psychological barriers and support behavior change.
Outline of Present Work
The related work revealed plenty of research on psychological barriers, behavioral models, and the potentials of immersive interventions to support behavior change processes. Four desiderata corroborate the need for an intelligible summation and linkage between the psychology of why and how and the why and how of immersive interventions. To address the desiderata, we present a re-categorization of common barriers of behavior change from psychological research on why the intention-behavior-gap occurs. The result is an intelligible categorization of determinants and barriers (refer to desideratum 1). The mapping between behavior-relevant features of immersive technologies on to these categories provides an overview of possible immersive interventions (refer to desideratum 3). We then address the summation of psychological models on how behavior change techniques operate and research how immersive technologies affect behavior change (refer to desideratum 2). Finally, our framework provides a generic prediction path that enables a structured, theory-based approach to develop and evaluate immersive interventions (refer to desideratum 4). Two use cases illustrate the prediction path at the end of the paper.
Methods
The framework was developed in an iterative process, including literature reviews and expert feedback. A short outline of this process follows.
Literature Review
We reviewed literature stemming from the field of psychology and HCI. The procedure followed the guidelines of Baumeister and Leary (1997) and Moher et al. (2015). The result was 1) an overview of behavior change barriers (refer to the psychology of why) and 2) psychological behavior change models and taxonomies (refer to the psychology of how). Furthermore, it resulted in 3) an overview of immersive features (refer to the potentials, the why, of immersive technologies) and 4) their effects on behavior change (refer to the empirical indicators, the how, of immersive technologies). The analysis started with the summation of results stemming from the literature by applying the Affinity Diagram method (Holtzblatt and Beyer, 2017) in an internal workshop. By using the Affinity Diagram method, data can be classified based on their relationships. Our analysis followed the usual Affinity Diagram process, which includes five steps: 1) Collecting abstract data onto post-it notes; 2) Clustering post-its by answering the question: Is the post-it similar or different to the existing groups of post-its? 3) Naming the clusters; 4) Ranking the clusters; 5) Finding relationships between clusters and naming them. After the summation of literature review data, we incorporated additional aspects stemming from the discussion in the affinity workshop. The affinity workshop led to the first draft of the framework that has been evaluated and improved by one focus group and two expert interviews.
Focus Group and Expert Interview
A focus group comprising ten researchers and practitioners working in different fields, including psychology, media sciences, and HCI (Kuniavsky, 2003) evaluated the first draft. The main aim was the evaluation of the intelligibility of the framework. Without prior explanation, participants were given a visualization of the framework. After 15 min of discussion in sub-groups of four, we introduced the main ideas of the framework. The final group discussion revealed parts that were coherent and parts that were barely intelligible. To capture diverse expertize on the theoretical foundation, completeness, and value of the framework, we conducted two expert interviews. We interviewed a professor of social and behavioral psychology who had been working as a psychological researcher since 1990 and one professor of HCI research with a focus on VR and immersive technologies who had been working in this field since 1996. Both rated their expertize in the respective field as high 4) or very high 5) on a 5-pt Likert scale. At the beginning of each interview, we introduced the main ideas of the framework. In the following 45-min interview, the psychological expert was mainly interviewed about the incorporation of the psychological models and determinants within the framework. The interview with the VR/HCI expert focused on the inclusion of the features and mechanisms of immersive technologies.
The focus group's statements and the answers from the two experts were categorized via a structured content analysis into different thematic areas: labeling, framework structure, expansion, and tangibility. Based on the focus group and expert interviews, we revised the first draft leading up to the version presented in the following section.
BehaveFIT
BehaveFIT: Why Can Immersive Technologies Support Behavior Change
This section addresses desiderata one and three by categorizing the psychological barriers and by mapping the features and corresponding perceptions of immersive technologies on to this categorization. The categorization and mapping contribute to the question: Why can immersive technologies support behavior change?
BehaveFIT: Intelligible Categorization of Psychological Barriers
Section The Psychology of Why the Intention-Behavior-Gap Occur introduces psychological barriers explaining why the intention-behavior-gap occurs. Desideratum one identified the need for an intelligible categorization of those barriers to provide guidance for intervention design and evaluation. BehaveFIT suggests a categorization that includes six distances impeding old behavior detachment or the adoption of new behavior. In the following, the distances are introduced. Figure 2 (first column) shows which psychological barriers (2.1) from the literature belong to the categories.
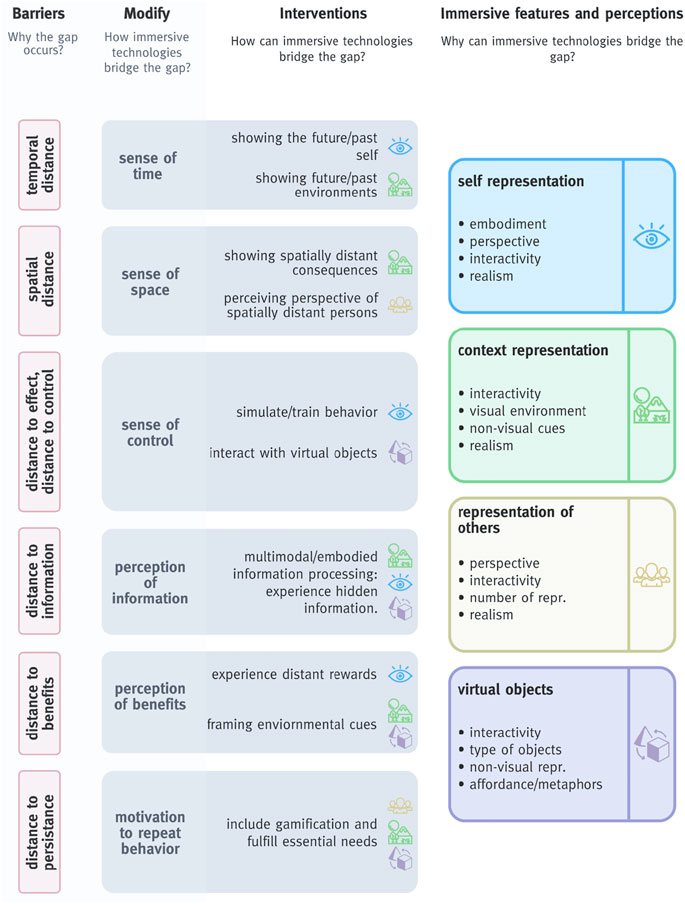
FIGURE 2. Sums up the framework BehaveFIT explaining why and how immersive technologies support behavior change processes.
Temporal distance
Describes barriers referring to the tendency to place a higher subjective value on rewards received immediately than those received in the future. Hence, the temporal distance of risks or benefits that often occur far in the future can lead to a failure to change behavior.
Spatial distance
Describes barriers referring to consequences that are geographically far away from the target person. Far away consequences are perceived as subjective, less affecting, consequential, or present, and thus, they are easier to ignore.
Distance from benefits
Describes barriers referring to the required effort in relation to the expected benefit of the change. It is related to the lack of immediate or near consequences but focuses on individual costs and risks associated with the change behavior.
Distance from effect/from control
Describes barriers referring to the lack of perceived control and self-efficacy. This distance can occur due to individual constraints or the expectation that other (more powerful) forces should solve the problem.
Distance from information
Describes barriers referring to a lack of information or to biased information processing. The lack of information interferes with problem discernment, and biased information processing correlates with justification behavior - both important for a successful change process.
Distance from persistence
Describes barriers referring to the way people are reluctant to change their behavior, particularly their habits. Behaviors, easy to adopt, tend to be chosen over action with higher effort cost, although the impact is relatively small.
BehaveFIT: Mapping of Features and Corresponding Perceptions to the Psychological Barrier Categories
Section Outline of Present Work introduces features and corresponding perceptions of immersive technologies, indicating why they can support behavior change processes. Desideratum three identified the need for relating the features and the corresponding perceptions to the psychological barriers impeding behavior change. BehaveFIT suggests a mapping on to the six barrier categories described above. The mapping shows where immersive technologies can help overcome these barriers. In the following, the mapping is introduced. Figure 2 (second, third, and fourth column) shows which features from the literature belong to the mapping.
Several features of immersive technologies manipulate the sense of time and corresponding effects and thus approach barriers referring to the category of temporal distance. Far away consequences (benefits and risks) can be brought nearer to the current behavior by showing the future self or future environments. Vice versa, users can experience events from the past to gain a better understanding. The corresponding modified self-or context perceptions can facilitate behavior change processes.
One crucial feature of immersive technologies is the ability to modify the sense of space, addressing barriers referring to the category of spatial distance. Again, far away consequences can be brought nearer to the current behavior. Particularly, other places and other persons can be experienced tangibly. The teleportation of oneself to other places or into other bodies or vice versa - bringing other places or persons into their own backyard–can facilitate behavior change processes.
Several features of immersive technologies increase the perception of benefits and thus approach barriers referring to the category of distance to benefits. Similarly to change processes themselves, rewarding effects often occur far in the future while costs occur immediately, fostering the focus on an over-proportional perception of effort. Modified immersive self or environments and corresponding perceptions can change the focus to the benefits and facilitate behavior change processes.
Particularly, modifying self-representation and the corresponding self-perception addresses barriers referring to the category of distance from effect/control by increasing the feeling of control and self-efficacy. The Proteus effect has proven that the effects go beyond mere exposure situations and manifest in real behavior habits, thus facilitating behavior change processes.
Augmenting or explaining complex information can facilitate information processing or the unmasking of biased information processing. Modifiable virtual objects and environments can support the information presented in addition to the behavior change processes.
Motivating repetitions by modified environments and gamification elements (e.g., a beach instead of the hospital), and increasing the feeling of relatedness by virtual companions are examples of approaching the distance to persistence by immersive technologies. Particularly, mapping on to the lack of motivation can facilitate the maintenance of behavior change processes.
BehaveFIT: How Do Immersive Technologies Support Behavior Change
Section The Potentials of Immersive Technologies - Why Can They Support Behavior Change? introduces psychological models and taxonomies, explaining how interventions should be designed to overcome the psychological barriers and support behavior change. This section addresses desideratum two and four that identified the need for a predictive framework linking immersive interventions to the theoretical base of behavior change. The linkage can guide the design and evaluation of immersive interventions. Consequently, this section introduces three steps explaining how BehaveFIT can be used, including guiding questions. Figure 3 shows the impact paths that can be derived from the framework, including the immersive features and corresponding perception, the psychological barrier as well as individual characteristics and intervention settings as moderators.
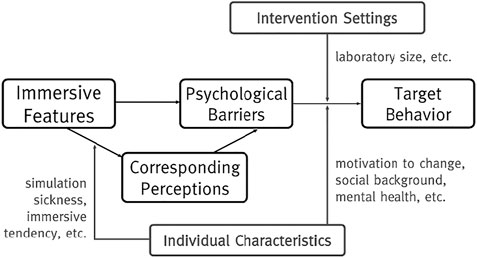
FIGURE 3. Overview of the BehaveFIT impact paths showing the expected impact of immersive technologies, moderators, and situational factors on behavior change.
Step 1: Defining the Pre-Conditions
The need for behavior change can occur in various domains such as environmental behavior or health behavior and in various stages of the change process such as initiation or maintenance of new behavior. Following Wienrich and Gramlich (2020), the behavior domain (1, “Target Behavior”) and the momentum within the change process (2, 3, “Intervention Settings”) should be determined at the beginning of the intervention planning process. Further intervention settings should be specified, including the selection of technical setup or the need for mobility, for example. Then, the addressed target group should be defined (4, “Individual Characteristics”). Individual characteristics could be linked to the technology, such as the vulnerability to simulator sickness or the target behavior, including previous behavior change attempts (see Figure 3).
Since fully defining all pre-conditions are out of the scope of the current contribution, please see Wienrich and Gramlich (2020) or Michie et al. (2014) for detailed information about the appropriate selection of pre-conditions to design and evaluate immersive interventions. However, the following guiding questions could support the definition of pre-conditions:
1. Target Behavior: What kind of behavior does the intervention address?
2. Intervention Setting: At what moment in the behavior change process is the intervention applied?
3. Intervention Setting: In which context, how often, and when are the target persons confronted with the intervention?
4. Individual Characteristics: What are the target persons' backgrounds and characteristics, and which persons are excluded?
Step 2: Defining the Psychological Barriers and Corresponding Immersive Features
Depending on the pre-conditions, the next step focuses on the selection of psychological barriers that target persons have to overcome. The six categories introduced in 5.1.1 guide the selection. Users should thoroughly analyze which barrier seems the most dominant and select one for a valuable intervention design (Byrne, 2020). reveals which features and corresponding perceptions of immersive technologies can help to overcome the barriers. In most cases, various features and their corresponding perceptions can be used to address one barrier. However, for a high-quality intervention design (Byrne, 2020), developers should choose one promising immersive potential to predict concrete impact paths. To define the psychological barriers and their corresponding immersive features properly, one can use the following guiding questions (see also Figure 2):
1. Analyze: Why do target persons show the intention-behavior-gap?
2. Select: Which barrier category is most likely to impede the change process, and which concrete psychological barrier within the category needs to be overcome?
3. Select: What kind of immersive feature and corresponding perception are linked to the selected barrier, and which concrete characteristic of the feature is supposed to induce the behavior change?
Step 3: Defining and Evaluating the Impact Path
To control the intervention's expected impact on behavior change, users should define the impact path of their intervention. The immersive intervention should impact the corresponding perceptions (1, “Corresponding Perceptions”) that in turn take effect on the psychological barrier. In the case of a successful intervention, the impact path would predict a successful behavior change or at least a facilitation of some aspects of the new behavior. In contrast, negative results show which expected impact does not occur, leading to a targeted intervention adaptation. An overview of the influence path from immersive features to behavior is given in Figure 3. It includes the immersive features, the corresponding perceptions as mediators, the psychological barriers, and the target behavior/behavior change. Additionally, it illustrates how individual characteristics can moderate the processing of immersive content and, together with the intervention settings, how they can moderate the relation between the barriers and behavior/behavior change. To define concrete impact paths, one can use the following guiding questions:
1. Select: How should immersive features affect the corresponding perceptions?
2. Select: How should the corresponding perceptions affect the psychological barrier?
3. Select: How should the barrier affect behavior/behavior change?
4. Check: Which other variables can confound the expected relations?
BehaveFIT: Use Cases
This section presents two paradigmatic use cases illustrating the framework's usefulness and the guiding function for intervention design and evaluation. The first use case aims to support people to engage in environmental behavior. The example illustrates how the questions, mentioned above, can guide the intervention and evaluation design. Note, we focus on demonstrating the usefulness of BehaveFIT. Thus presenting empirical data was out of scope. The second use case illustrates how students evaluate the framework's usage for design an immersive anti-discrimination intervention. Here, we focus on the evaluation of the students. Again, presenting empirical data regarding the intervention effect was out of scope.
Use Case 1: Environmental Intervention
Step 1 - Defining the Pre-Conditions
The researchers in our first example wanted to support people to engage in environmental behavior. Recently, the trend sport “plogging” (Swedish for plocka + jogging) has gained popularity. People clean their environment by collecting garbage as they run. Thus, the researchers decided to design an immersive plogging environment to further engage people in environmentally friendly behavior. In our example, the target behavior belongs to the domain of pro-environmental behavior in general and specifically to the domain of active cleaning behavior of the local environment (refer to question 1). Target persons are already aware of environmental problems but are not actively engaged in pro-environmental behavior besides recycling (refer to question 2). The intervention will be presented in a local environment festival and people will be confronted with the intervention only once (refer to question 3). The intervention includes active physical movements as walking in place and bending over to simulate plogging, and people with physical disabilities are excluded from the intervention (refer to question 4).
Step 2 - Defining the Psychological Barriers and Corresponding Immersive Features
In our example, the researchers analyzed that people who are aware but do not start engaging actively often perceive a lack of control: “What can I do?” (refer to question 1). Hence, they decided to address the barrier distance from control. Particularly, the researchers wanted to enhance the self-efficacy and the perception that one’s engagement matters (refer to question 2). Following the results, mentioned above, of a successful manipulation of the self-representation, the corresponding sense of embodiment, and the Proteus effect, the researchers decided to evaluate the impact of control over one’s self-representations within two different conditions. They developed two settings where the users either embodied and actively manipulated an athletic avatar or observed the avatars actions from first-person perspective without actively engaging in the virtual plogging activity (refer to question 3).
Step 3 - Defining and Evaluating the Impact Path
In our example, the selected immersive feature is self-representation. Thus, the researchers decided to modify the feature of self-representation (two avatar-conditions) that should enhance the sense of embodiment (see Figure 4), particularly the sense of control in VR (perceived agency) (refer to question 1). Furthermore, they assumed a relationship between the perceived agency and the psychological feeling of self-efficacy of environmental cleaning behavior (refer to question 2). Finally, they expected an increase of participants' pro-environmental behavior after the immersive intervention and increases active engagement in pro-environmental activities (refer to question 3). They took into account confounding variables such as openness to new experiences (refer to question 4).
Use Case 2: Student Evaluation and Anti-discrimination Intervention
The second use case illustrates how students evaluated the framework’s usage for designing an immersive anti-discrimination intervention in the context of a seminar. Four students (1 female, two males, one diverse; age: M = 21.75 SD = 0.10) got a short oral introduction to the framework and an explaining worksheet (Figure 5). The task was to develop a 360° video intervention concept to raise awareness for discriminating behavior and to plan a corresponding evaluation study. Figure 6 shows the student’s path model.
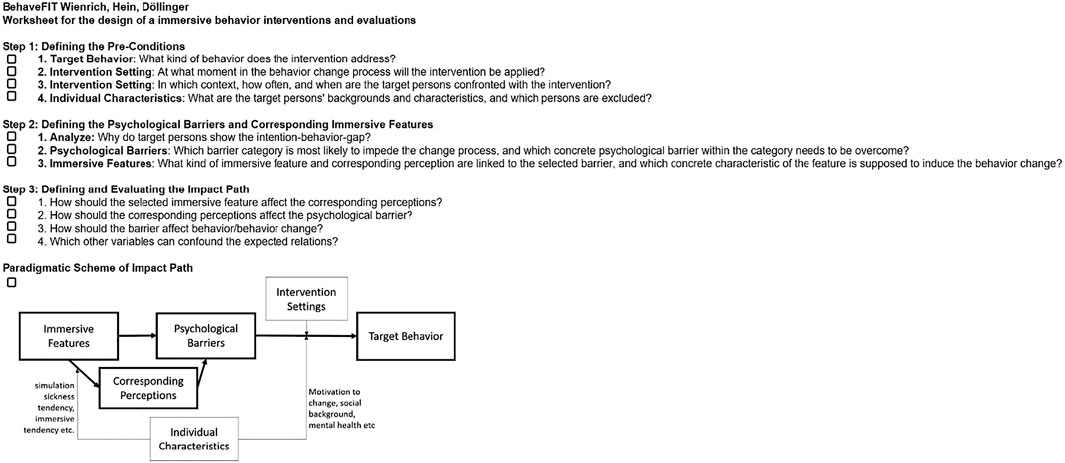
FIGURE 5. The worksheet illustrates how BehaveFIT can be used for intervention and evaluation design.
From the authors’ point of view, it is apparent that the students executed steps 1 and 2. The pre-conditions were defined clearly, and the psychological barrier and the corresponding perception have been selected from the BehaveFIT synopsis (see Figure 2). However, step 3 was incorporated only superficially. The students built the path model but did not define operationalized variables measuring the psychological barrier or the corresponding perception. The result might not be surprising as they depict the beginning of the seminar where the intervention concept’s development had priority. During the second half of the course, the students added information about the operationalization of the variables which are more crucial for the study design than for concept development. To get an impression of how the framework was used and whether BehaveFIT was comprehensive, we assessed on a five-point likert scale how comprehensive, helpful, structured, informative, and encompassing the framework was perceived. In an open question format, we further asked how the framework was used and which positive and limiting aspects were perceived. The results revealed a positive evaluation (comprehensive: M = 4.00 SD = 0.00, helpful: M = 3.75 SD = 0.50, structured: M = 4.5 SD = 0.58, informative: M = 4 SD = 0.00, and encompassing: M = 4.25 SD = 0.50). Further, the students used it for understanding, concept development and planning, and presentation and documentation. Giving structure, increasing understanding, getting a step-by-step process, and helpful visualizations were mentioned as positive aspects. Concrete examples provided on the worksheet and more support to discriminate the barriers were mentioned as limiting aspects.
In sum, second use case demonstrated that a relatively inexperienced user group could use BehaveFIT to design an immersive intervention and evaluation. Besides, preliminary results indicated that the framework is comprehensive and helpful.
Discussion
Review of Present Work
The intention-behavior-gap describes the failure of the translation from intending to change a behavior to successfully changing it (Gollwitzer and Bargh, 1996; Kollmuss and Agyeman, 2002; Sheeran, 2002; Whitmarsh, 2009; Carrington et al., 2010; Ajzen, 2011; Schwarzer, 2014). The related work revealed plenty of research on psychological barriers describing why the gap occurs and behavioral models explaining how behavioral interventions can overcome the gap. Furthermore, features of immersive interventions explain why they have a lot of potential to support behavior change processes and empirical findings indicating how they impact human behavior. However, the literature review also revealed four desiderata corroborating the need for an intelligible integration of psychological barriers, models, and immersive features. The current lack of transfer between the disciplines results in a lack of theoretical links between the features and possibilities of immersive technologies and psychological behavior determinants, which further results in a lack of theoretical-based predictions for the design and evaluation of immersive interventions. The framework BehaveFIT addresses the desiderata by (see also Figure 2):
• presenting an intelligible categorization of psychological determinants and barriers. The psychological barriers are described in six distances impeding the detachment of old behavior or the adoption of new behavior (desideratum 1).
• suggesting a mapping of immersive features and corresponding perceptions on to the six distances (barrier categories). The mapping shows why and where immersive technologies can help overcome the barriers (desideratum 3).
• addressing the summation of psychological models on how behavior change techniques operate and research how immersive technologies affect behavior change (desiderata 2).
• proposing a generic prediction path that enables a structured, theory-based approach to develop and evaluate immersive interventions (desideratum 4). There are three steps, which explain how BehaveFIT can be used, including guiding questions and one example for each step.
Benefits and Contribution of the BehaveFIT
Byrne (2020) identifies the most critical point in his review about gaps and priorities in advancing methods for health behavior change research is the consideration of behavioral change theories and models. Further, and in accordance with Wobbrock and Kientz (2016), a theory-driven approach has the effect of finding out not only if an intervention works but also why and how it works. This leads to evaluation results that are more generally applicable to further developments and new interventions in other areas. In addition, it supports the development of more targeted interventions by drawing on mechanisms already known about from psychological research. The present paper seized on this challenge by suggesting an interdisciplinary cross transfer to provide why and how immersive interventions support behavior change processes. BehaveFIT goes beyond existing suggestions as to why and how immersive intervention technologies might support behavioral change. The proposed relations between the psychological barriers and the immersive features serve as a starting point for predicting concrete paths of impact on immersive interventions. The summation, categorization, and intelligible mapping offer systematic guidance for design effectual immersive interventions. Concerning human-centered design, the pre-conditions of the interventions can be analyzed (step 1), including the context, target group, and confounding variables. Then, the psychological barriers and the corresponding immersive features can be defined in a structured and theory-driven way (step 2).
Concerning evaluation, Klasnja et al. (2011) suggested that assessing whether a technology accomplished behavioral change often leads to limited results. Due to the complexity of behavior change, evaluations “[...] often do not reveal why the technology that is being evaluated worked or did not work” (Klasnja et al., 2011, p. 3064). The authors stated further: "This knowledge, however, is of central importance to HCI researchers as they attempt to develop novel, yet effective systems" (Klasnja et al., 2011, p. 3064). BehaveFIT can contribute here in various ways. The predictions resulting from the concrete impact paths reduce the corresponding evaluation's complexity and thus are able to reveal why the technology worked or did not work (step 3). In line with Klasnja et al. (2011), we believe that knowing why is crucial to HCI researchers as they attempt to develop effective systems. Furthermore, since the framework includes established factors (psychological and technological), we recommend researchers following Wienrich and Gramlich (2020) to consider measurements that account for corresponding measurements assessing the impact or rather the manifestations of these effects (behavioral effects: e.g., stage of change questionnaire (Marcus et al., 1992) or index of habit strength (Verplanken and Orbell, 2003); immersive features and perceptions: e.g., virtual embodiment questionnaire (Roth and Latoschik, 2020), group presence questionnaire (Schubert et al., 2001) or networked minds measure of social presence (Biocca and Harms, 2003).
In sum, BehaveFIT provides guidance for immersive intervention design and evaluation to show that immersive interventions support behavior change processes and explain and predict why and how immersive interventions can bridge the intention-behavior-gap.
Limitations and Future Work
This framework is the first attempt at a structured integration of potentials from immersive technologies and psychological barriers determining behavioral change processes. The current framework can give a broad overview of the connection between these two fields. Consequently, the framework comes with certain restrictions concerning levels of detail of the framework's components, the impact paths, and application areas.
Further Components
Due to summation and comprehensibility, we accepted some restrictions regarding the complexity of mental processes and determinants during the behavioral change. Only a selection of mental inhibitors and facilitators have been included, although all of them can be divided into various sub-facets or sub-sub-facets (for a review, see Cane et al., 2012). The same limitations pertain to the factors of the immersive features and corresponding perceptions. Here, too, we would like to point out that the framework is intended as a selection to provide intelligible summation and guidance, and it does not claim completeness.
Besides including further barriers and features, future work might focus on identifying and weighing the most critical determinants influencing behavior change progress. Weighting the barriers and features might help to get further insights into the relevance or irrelevance of the determinants, which in turn might facilitate the guidance for the design and evaluation of immersive interventions.
Further Paths of Impact
Even though users of the current framework can extract specific paths of impact by mapping immersive features and psychological barriers, we do not define one-to-one relations between the features and barriers. As shown in the literature review, the current state of research does not yet allow such an exact assignment. Thus, the framework instead serves as a reference guide in HCI research for the design and evaluation of immersive intervention technologies. However, by investigating specific impact paths and interrelations between the barriers and features in future work, it will gradually be possible to include them in the framework structure.
Further Fields of Application
Since the majority of research activity has been done on virtual reality applications, the included immersive features and corresponding perceptions are based on VR. Hence, the features might not be necessarily transferable to other (immersive) technologies such as augmented reality (AR) or 360°-videos. Future work could examine the immersive dimensions in the context of non-VR-related technologies.
In sum, further development of BehaveFIT 1.0 might include both other therapeutic application areas and other immersive or partly immersive technologies. Further research might lead to a respective enlargement of the current framework and the addressed areas of application and use cases.
Conclusion
When choosing to change a specific behavior, people often cannot attain their goals, leading up to a gap between behavioral intention and actual behavior. Within HCI research, the development and evaluation of immersive technologies that target supporting the behavior change process and bridging this gap have gained importance. However, a significant part of these developments still lacks the theoretical background or remain limited to the initiation of a behavior or to short-term effects. This paper presented a first attempt to combine two research areas–the field of psychology examining behavior change and the field of HCI investigating immersive technologies and the corresponding human sensations and perceptions–in a comprehensive framework. BehaveFIT wants to guide the design and evaluation process of immersive intervention tools by assisting in the contextual analysis, including personas.
Additionally, it may provide guidance in identifying, inhibiting or facilitating mediators between immersive technologies and behavioral measures. A review of HCI research revealed that only a few determinants or paths of impact had been attended so far. Hence, we believe that the present work is an essential contribution to the encouragement of researchers from both fields to use BehaveFIT to bridge the gaps.
Data Availability Statement
The original contributions presented in the study are included in the article/supplementary material, further inquiries can be directed to the corresponding author.
Author Contributions
CW had the main idea for the article topic, guided the theoretical contribution, particularly the summation of psychological barriers, the summation of the immersive features, and the core of the BehaveFIT Model (Figure 2). She was also responsible for the method section. Further, she draws connections to the field of human-computer interaction. ND incorporated the behavioral models and the empirical studies on how immersive features impact on behavior (Figure 3). Further, she was responsible for the method section and she was responsible for formulating the guiding steps for the user-centered design process and contributed to the discussion. RH incorporated the example and the concrete path of impact. (Figure 4), contributed to the method and designed the figures.
Funding
This publication was supported by the Open Access Publication Fund of the University of Wuerzburg. This research has been funded by the German Federal Ministry of Education and Research in the project ViTraS (project number 16SV8219).
Conflict of Interest
The authors declare that the research was conducted in the absence of any commercial or financial relationships that could be construed as a potential conflict of interest.
References
Ahn, S. J., Bailenson, J. N., and Park, D. (2014). Short- and Long-Term Effects of Embodied Experiences in Immersive Virtual Environments on Environmental Locus of Control and Behavior. Comput. Hum. Behav. 39, 235–245. doi:10.1016/j.chb.2014.07.025
Ahn, S. J., Fox, J., Dale, K. R., and Avant, J. A. (2015). Framing Virtual Experiences. Commun. Res. 42 (6), 839–863. doi:10.1177/0093650214534973
Ahn, S. J. G., Bostick, J., Ogle, E., Nowak, K. L., McGillicuddy, K. T., and Bailenson, J. N. (2016). Experiencing Nature: Embodying Animals in Immersive Virtual Environments Increases Inclusion of Nature in Self and Involvement with Nature. J. Comput-mediat Comm. 21 (6), 399–419. doi:10.1111/jcc4.12173
Ahn, S. J., Hahm, J. M., and Johnsen, K. (2019). Feeling the Weight of Calories: Using Haptic Feedback as Virtual Exemplars to Promote Risk Perception Among Young Females on Unhealthy Snack Choices. Media Psychol. 22 (4), 626–652. doi:10.1080/15213269.2018.1492939
Ajzen, I. (2011). The theory of planned behaviour: reactions and reflections. Psychol. Health 26 (9), 1113–1127.
Ajzen, I. (1980). Understanding Attitudes and Predicting Social Behavior. Englewood Cliffs: Prentice Hall.
Bandura, A. (1977). Self-efficacy: toward a Unifying Theory of Behavioral Change. Psychol. Rev. 84 (2), 191–215. doi:10.1037/0033-295x.84.2.191
Baumeister, R. F., and Leary, M. R. (1997). Writing Narrative Literature Reviews. Rev. Gen. Psychol. 1 (3), 311–320. doi:10.1037/1089-2680.1.3.311
Becker, M. H., and Maiman, L. A. (1975). Sociobehavioral Determinants of Compliance with Health and Medical Care Recommendations. Med. Care 13, 10–24. doi:10.1097/00005650-197501000-00002
Biocca, F., and Harms, C. (2002). “Defining and Measuring Social Presence: Contribution to the Networked Minds Theory and Measure,” in Proceedings of PRESENCE, Porto Portogal, October 2002, 1–36.
Biocca, F., and Harms, C. (2003). Guide to the Networked Minds Social Presence Inventory V. 1.2: Measures of Co-presence, Social Presence, Subjective Symmetry, and Intersubjective Symmetry. East Lansing: Michigan State University.
Blake, J. (1999). Overcoming the 'value‐action gap' in Environmental Policy: Tensions between National Policy and Local Experience. Local Environ. 4 (3), 257–278. doi:10.1080/13549839908725599
Bolger, N., and Zee, K. S. (2019). Heterogeneity in Temporal Processes: Implications for Theories in Health Psychology. Appl. Psychol. Health Well‐being 11 (2), 198–201. doi:10.1111/aphw.12159
Bridle, C., Riemsma, R. P., Pattenden, J., Sowden, A. J., Mather, L., Watt, I. S., et al. (2005). Systematic Review of the Effectiveness of Health Behavior Interventions Based on the Transtheoretical Model. Psychol. Health 20 (3), 283–301. doi:10.1080/08870440512331333997
Byrne, M. (2020). Gaps and Priorities in Advancing Methods for Health Behaviour Change Research. Health Psychol. Rev. 14 (1), 165–175. doi:10.1080/17437199.2019.1707106
Cane, J., O'Connor, D., and Michie, S. (2012). Validation of the Theoretical Domains Framework for Use in Behaviour Change and Implementation Research. Implementation Sci. 7 (1), 37. doi:10.1186/1748-5908-7-37
Carrington, M. J., Neville, B. A., and Whitwell, G. J. (2010). Why Ethical Consumers Don't Walk Their Talk: Towards a Framework for Understanding the gap between the Ethical purchase Intentions and Actual Buying Behaviour of Ethically Minded Consumers. J. Bus Ethics 97 (1), 139–158. doi:10.1007/s10551-010-0501-6
Davis, R., Campbell, R., Hildon, Z., Hobbs, L., and Michie, S. (2014). Theories of Behaviour and Behaviour Change across the Social and Behavioural Sciences: a Scoping Review. Health Psychol. Rev. 9 (3), 323-344. doi:10.1080/17437199.2014.941722
De Kort, Y., IJsselsteijn, W., and Poels, K. (2007). “Digital Games as Social Presence Technology: Development of the Social Presence in Gaming Questionnaire (SPGQ),” in Proceedings of PRESENCE, Barcelona, Spain, October 2007, 195203.
Fox, J., and Bailenson, J. N. (2009). Virtual Self-Modeling: The Effects of Vicarious Reinforcement and Identification on Exercise Behaviors. Media Psychol. 12 (1), 1–25. doi:10.1080/15213260802669474
Gifford, R., Lacroix, K., and Chen, A. (2018). “Understanding Responses to Climate Change,” in Psychology and Climate Change (New York: Elsevier Academic Press), 161–183. doi:10.1016/b978-0-12-813130-5.00006-0
Gollwitzer, P., and Bargh, J. (1996). The Psychology of Action: Linking Cognition and Motivation to Behavior. New York: Guilford Press.
Hassenzahl, M., Diefenbach, S., and Göritz, A. (2010). Needs, Affect, and Interactive Products - Facets of User Experience. Interacting Comput. 22 (5), 353–362. doi:10.1016/j.intcom.2010.04.002
Herrera, F., Bailenson, J., Weisz, E., Ogle, E., and Zaki, J. (2018). Building Long-Term Empathy: A Large-Scale Comparison of Traditional and Virtual Reality Perspective-Taking. PloS one 13 (10), e0204494. doi:10.1371/journal.pone.0204494
Holtzblatt, K., and Beyer, H. (2017). Principles of Contextual Inquiry. Oxford: Contextual Design, 43–80. doi:10.1016/b978-0-12-800894-2.00003-x
Hsu, W.-C., Tseng, C.-M., and Kang, S.-C. (2018). Using Exaggerated Feedback in a Virtual Reality Environment to Enhance Behavior Intention of Water-Conservation. Educ. Technol. Soc. 21, 187–203.
Kilteni, K., Groten, R., and Slater, M. (2012). The Sense of Embodiment in Virtual Reality. Presence: Teleoperators and Virtual Environments 21 (4), 373–387. doi:10.1162/pres_a_00124
Kim, S. Y., Prestopnik, N., and Biocca, F. A. (2014). Body in the Interactive Game: How Interface Embodiment Affects Physical Activity and Health Behavior Change. Comput. Hum. Behav. 36 (2014), 376–384. doi:10.1016/j.chb.2014.03.067
Klasnja, P., Consolvo, S., and Pratt, W. (2011). “How to Evaluate Technologies for Health Behavior Change in HCI Research,” in Proceedings of the SIGCHI conference on human factors in computing systems, Vancouver, Canada, May 2011 (Vancouver: ACM), 3063–3072.
Kollmuss, A., and Agyeman, J. (2002). Mind the gap: Why Do People Act Environmentally and what Are the Barriers to Pro-environmental Behavior?. Environ. Educ. Res. 8 (3), 239–260. doi:10.1080/13504620220145401
Kuniavsky, M. (2003). Observing the User Experience: A Practitioner's Guide to User Research. Burlington, MA: Elsevier.
Kuo, P.-Y. (2015). “Engage People in Pro-environmental Behaviors through Online Prosocial Interaction and Pro-health Intervention,” in Proceedings of the 33rd Annual ACM Conference Extended Abstracts on Human Factors in Computing Systems - CHI EA '15, 207–210.
Maister, L., Slater, M., Sanchez-Vives, M. V., and Tsakiris, M. (2015). Changing Bodies Changes Minds: Owning Another Body Affects Social Cognition. Trends Cognitive Sciences 19 (1), 6–12. doi:10.1016/j.tics.2014.11.001
Marcus, B. H., Selby, V. C., Niaura, R. S., and Rossi, J. S. (1992). Self-efficacy and the Stages of Exercise Behavior Change. Res. Q. Exerc. Sport 63 (1), 60–66. doi:10.1080/02701367.1992.10607557
Michie, S., Atkins, L., and West, R. (2014). The Behaviour Change Wheel. A Guide to Designing Interventions. 1st ed. Great Britain: Silverback Publishing, 1003–1010.
Michie, S., Richardson, M., Johnston, M., Abraham, C., Francis, J., Hardeman, W., et al. (2013). The Behavior Change Technique Taxonomy (V1) of 93 Hierarchically Clustered Techniques: Building an International Consensus for the Reporting of Behavior Change Interventions. Ann. Behav. Med. 46 (1), 81–95. doi:10.1007/s12160-013-9486-6
Moher, D., Shamseer, L., Clarke, M., Ghersi, D., Liberati, A., Petticrew, M., et al. (2015). Preferred Reporting Items for Systematic Review and Meta-Analysis Protocols (PRISMA-P) 2015 Statement. Syst. Rev. 4 (1), 1. doi:10.1186/2046-4053-4-1
Morina, N., Ijntema, H., Meyerbröker, K., and Emmelkamp, P. M. G. (2015). Can Virtual Reality Exposure Therapy Gains Be Generalized to Real-Life? A Meta-Analysis of Studies Applying Behavioral Assessments. Behav. Res. Ther. 74, 18–24. doi:10.1016/j.brat.2015.08.010
Oh, C. S., Bailenson, J. N., and Welch, G. F. (2018). A Systematic Review of Social Presence: Definition, Antecedents, and Implications. Front. Robot AI 5 (5), 114. doi:10.3389/frobt.2018.00114
Powers, M. B., and Emmelkamp, P. M. G. (2008). Virtual Reality Exposure Therapy for Anxiety Disorders: A Meta-Analysis. J. anxiety Disord. 22 (3), 561–569. doi:10.1016/j.janxdis.2007.04.006
Prochaska, J. O., and Velicer, W. F. (1997). The Transtheoretical Model of Health Behavior Change. Am. J. Health Promot. 12 (1), 38–48. doi:10.4278/0890-1171-12.1.38
Ratan, R., Beyea, D., Li, B. J., and Graciano, L. (2020). Avatar Characteristics Induce Users' Behavioral Conformity with Small-To-Medium Effect Sizes: a Meta-Analysis of the proteus Effect. Media Psychol. 23 (5), 651–675. doi:10.1080/15213269.2019.1623698
Riva, G., Wiederhold, B. K., and Mantovani, F. (2019). Neuroscience of Virtual Reality: from Virtual Exposure to Embodied Medicine. Cyberpsychology, Behav. Soc. Networking 22 (1), 82–96. doi:10.1089/cyber.2017.29099.gri
Roth, D., and Latoschik, M. (2020). Construction of the Virtual Embodiment Questionnaire (VEQ). IEEE Trans. Visualization Comput. Graphics 26, 3546–3556. doi:10.1109/TVCG.2020.3023603
Rothman, A. J. (2019). It's about Time: Answering the Call for Greater Precision in Research and Practice. Appl. Psychol. Health Well‐being 11 (2), 191–197. doi:10.1111/aphw.12158
Scholz, U. (2019). It's Time to Think about Time in Health Psychology. Appl. Psychol. Health Well‐being 11 (2), 173–186. doi:10.1111/aphw.12156
Schubert, T., Friedmann, F., and Regenbrecht, H. (2001). The Experience of Presence: Factor Analytic Insights. Presence: Teleoperators & Virtual Environments 10 (3), 266–281. doi:10.1162/105474601300343603
Sheeran, P. (2002). Intention-behavior Relations: a Conceptual and Empirical Review. Eur. Rev. Soc. Psychol. 12 (1), 1–36. doi:10.1080/14792772143000003
Sherman, W., and Craig, A. (2018). Understanding Virtual Reality: Interface, Application, and Design. Burlington, MA: Morgan Kaufmann.
Skarbez, R., Brooks, J., and Whitton, M. (2017). A Survey of Presence and Related Concepts. ACM Comput. Surv. (Csur) 50 (6), 1–39.doi:10.1145/3134301
Slater, M. (1999). Measuring Presence: A Response to the Witmer and Singer Presence Questionnaire. Presence 8 (5), 560–565. doi:10.1162/105474699566477
Soliman, M., Peetz, J., and Davydenko, M. (2017). The Impact of Immersive Technology on Nature Relatedness and Pro-environmental Behavior. J. Media Psychol. 29 (1), 8–17. doi:10.1027/1864-1105/a000213
Stawarz, K., Cox, A., and Blandford, A. (2015). “Beyond Self-Tracking and Reminders: Designing Smartphone Apps that Support Habit Formation,” in Proceedings of the 33rd annual ACM conference on human factors in computing systems, Seoul, Korea, May 2015, 2653–2662.
Stawarz, K., and Cox, A. (2015). “Designing for Health Behavior Change: HCI Research Alone Is Not Enough,” in CHI'15 workshop: Crossing HCI and Health: Advancing Health and Wellness Technology Research in Home and Community Settings, Seoul, Korea, May 2015. doi:10.1145/2702123.2702230
Verplanken, B., and Orbell, S. (2003). Reflections on Past Behavior: A Self-Report Index of Habit Strength1. J. Appl. Soc. Psychol. 33 (6), 1313–1330. doi:10.1111/j.1559-1816.2003.tb01951.x
Whitmarsh, L. (2009). Behavioural Responses to Climate Change: Asymmetry of Intentions and Impacts. J. Environ. Psychol. 29 (1), 13–23. doi:10.1016/j.jenvp.2008.05.003
Wienrich, C., and Gramlich, J. (2020). appRaiseVR - an Evaluation Framework for Immersive Experiences. i-com 19 (2), 103–121. doi:10.1515/icom-2020-0008
Wienrich, C., Schindler, K., Döllinger, N., Kock, S., and Traupe, O. (2018). “Social Presence and Cooperation in Large-Scale Multi-User Virtual Reality-The Relevance of Social Interdependence for Location-Based Environments,” in 2018 IEEE Conference on Virtual Reality and 3D User Interfaces (VR) (IEEE), 207–214.
Wilson, T. E., Hennessy, E. A., Falzon, L., Boyd, R., Kronish, I. M., and Birk, J. L. (2020). Effectiveness of Interventions Targeting Self-Regulation to Improve Adherence to Chronic Disease Medications: A Meta-Review of Meta-Analyses. Health Psychol. Rev. 14 (1), 66–85. doi:10.1080/17437199.2019.1706615
Wobbrock, J. O., and Kientz, J. A. (2016). Research Contributions in Human-Computer Interaction. interactions 23 (3), 38–44. doi:10.1145/2907069
Keywords: immersive technologies, behavior change, intervention design, intervention evaluation, framework, virtual reality, intention-behavior-gap, human-computer interaction
Citation: Wienrich C, Döllinger N and Hein R (2021) Behavioral Framework of Immersive Technologies (BehaveFIT): How and Why Virtual Reality can Support Behavioral Change Processes. Front. Virtual Real. 2:627194. doi: 10.3389/frvir.2021.627194
Received: 08 November 2020; Accepted: 10 June 2021;
Published: 24 June 2021.
Edited by:
Amir Dirin, Haaga-Helia University of Applied Sciences, FinlandReviewed by:
Weiya Chen, Huazhong University of Science and Technology, ChinaDominique Bechmann, Université de Strasbourg, France
Copyright © 2021 Wienrich, Döllinger and Hein. This is an open-access article distributed under the terms of the Creative Commons Attribution License (CC BY). The use, distribution or reproduction in other forums is permitted, provided the original author(s) and the copyright owner(s) are credited and that the original publication in this journal is cited, in accordance with accepted academic practice. No use, distribution or reproduction is permitted which does not comply with these terms.
*Correspondence: Carolin Wienrich, Y2Fyb2xpbi53aWVucmljaEB1bmktd3VlcnpidXJnLmRl