- 1Department of Psychology, University of Copenhagen, Copenhagen, Denmark
- 2Department of Computer Science, University of Copenhagen, Copenhagen, Denmark
Conducting user studies online and unsupervised instead of in laboratories gives quick access to a large and inexpensive participant pool. It is however unclear if data sourced this way is valid, and what the best practices for conducting unsupervised VR studies are. The restrictions on laboratory access experienced during COVID-19 further necessitate the development of valid procedures for remote data collection, especially for research fields such as VR that heavily rely on laboratory studies. In this paper we report our experiences with conducting two unsupervised VR studies amidst the pandemic, by recruiting participants online on relevant fora and employing participants’ own standalone VR equipment. We investigate whether it is feasible to collect valid data across in-VR survey responses and hand tracking. We report a good reliability of collected data, which requires only slightly more sanitation than a comparable laboratory study. We synthesize our experiences into practical recommendations for conducting unsupervised VR user studies using online recruitment, which can greatly reduce barriers to conducting empirical VR research and improve the quantity of VR user studies, regardless of laboratory availability.
Introduction
Experimental studies involving human subjects are increasingly conducted unsupervised and online. These are collectively referred to as crowdsourcing (Estellés-Arolas and González-Ladrón-De-Guevara, 2012; Goodman and Paolacci, 2017). Crowdsourcing brings with it a range of benefits such as participant diversity and flexibility (Goodman and Paolacci, 2017), thereby making up for some of the weaknesses associated with common laboratory practices, such as reliance on university students as research participants. Although crowdsourcing research also brings disadvantages, such as self-selection and reduced internal validity, studies generally indicate that it is a reliable protocol for research with human subjects (Behrend et al., 2011). A wealth of research covers practices about crowdsourcing experimentation, such as experimental design, data sanitation, and participant qualification tests (Crump et al., 2013; Daniel et al., 2018). These works together show the breadth of crowdsourcing research applications and point toward practical recommendations for conducting research this way.
Several types of online platforms facilitate unsupervised remote studies. Most popular are micro-task markets that provide researchers with infrastructure to post micro tasks, so-called Human Intelligence Tasks (HITs). Participants can then agree to conduct a HIT, usually, for quite modest pay. Amazon Mechanical Turk1 (AMT), Appen2 and Prolific3 are prominent examples of such systems.
Crowdsourcing is increasingly employed for research purposes, and notably the fields of social and personality psychology have experienced a recent increase in papers sourcing data this way (Anderson et al., 2019). Moreover, practical guidelines for crowdsourcing research have emerged (Sheehan, 2018).
The vast majority of the crowdsourced studies are conducted using desktop computers or mobile devices, and little is therefore known about conducting unsupervised user studies for immersive devices such as virtual reality. In recent years, some examples of conducting unsupervised VR research have been presented (Steed et al., 2016; Mottelson and Hornbæk, 2017; Ma et al., 2018). These works show that VR studies can be conducted without supervision, yet do not provide real-world insights from conducting unsupervised VR research with recruitment online. Recently, Rivu et al. (2021) presented a framework for running VR studies remotely. The authors provide organizational recommendations for approaches to conducting remote VR studies, including considering participants’ physical constraints and lessons of conducting remote and supervised experiments. Questions regarding subjective and objective data validity and quality sourced this way nonetheless remain.
During the COVID-19 pandemic, the need for conducting valid and unsupervised experiments has escalated further. Research in VR is, in particular, dominated by laboratory experiments (Cipresso et al., 2018). Due to health concerns and the wearable nature of HMDs, VR research is challenged. Clear evidence of the efficacy of crowdsourcing VR experiments could therefore alleviate some of the contemporary issues by accommodating safety concerns and also positively disrupt the statistical power, price, and duration of many VR user studies.
A recent scientometric analysis indicates that VR research spans many areas, most notably computer science, engineering, education, and neuroscience (Cipresso et al., 2018). Across disciplines, the vast majority of empirical VR research is done using controlled laboratory settings. Laboratory studies are time-consuming, often conducted with participants unfamiliar with the technology; one at a time. Furthermore, a noteworthy criticism of VR studies is their low sample sizes. Wu et al. (2020) report a mean sample size of 54 in a meta-analysis of VR research in education. Commonly, t-tests are used in VR research (Radianti et al., 2020), and with independent samples, two tails, an alpha level of 0.05, N = 27 in each group, and a medium effect size (d = 0.5), this would yield a power level of 0.4, which is considered low.
Despite an apparent lack of unifying guidelines for crowdsourcing VR research, the potential is vast. Some rough estimates state more than half a million Oculus Quest HMDs sold in 2020 (AR Insider, 2020), and the Oculus Quest subreddit4, for instance, has approximately 240,000 members, at the time of writing. Furthermore, there are several advantages associated with conducting unsupervised VR research online with participants who own their own VR devices. These include flexibility when circumstances do not allow for real-life experiments (such as the lockdown during COVID-19); reducing the potential influence of novelty effects (i.e., when results are influenced by the newness of the medium (Clark, 1983); the potential for usability studies with expert users; making research less time-consuming; and the potential of conducting experimentation in the context of participants’ homes.
This paper reports evidence from two studies that were conducted amidst a global pandemic. Both studies were conducted unsupervised, by participants themselves, at their own discretion. These studies had independent scientific purposes, and were therefore not conducted solely to verify the data collection method. In this paper, however, we summarize findings related to data collection. The data provides evidence for the feasibility of conducting unsupervised VR user studies, and the subsequent analysis and comparison to similar in-lab experimentation shows promising data quality. Specifically, we contribute with a qualification of the reliability of the data collected, we estimate the number of aberrant data, we compare results from an unsupervised study to a laboratory study, and we report on the demographics when recruiting participants through the biggest online VR community.
Research Questions
We structure our investigation of online and unsupervised VR research based on the following research questions:
1. Is data from VR studies collected from unsupervised participants reliable?
2. What are the percentage of aberrant responses from studies conducted this way?
3. Are findings from unsupervised VR studies comparable to lab studies?
4. How do we collect quality unsupervised hand/head tracking data?
5. What are the demographics of participants sourced this way?
Crowdsourcing
The literature is abundant with examples of crowdsourced human experiments since the idea was proposed more than a decade ago (Kittur et al., 2008; Paolacci et al., 2010). Since then, a host of papers have described methods for designing crowdsourcing studies, optimizing participant effort, and how to avoid invalid participation [e.g. (Heer and Bostock, 2010; Cheng et al., 2015; Gadiraju et al., 2015)]. There are similarly numerous ideas for designing novel interfaces and practices to optimize output from crowdworkers, such as gamification (Feyisetan et al., 2015) or conversational interfaces (Bradeško et al., 2017). Some highly influential research has been conducted using these techniques, among others, predicting protein structures (Cooper et al., 2010), creating a gold standard for image data sets (Deng et al., 2009), and transcribing books via web security measures (von Ahn et al., 2008).
Crowdsourcing is evidently an important driver for large-scale human experimentation. As this experimental paradigm has shown to be effective for, among others, social science studies, it raises the question to what extent VR studies can be run this way. Consumer VR has in 2020 reached a maturity with a critical mass of privately owned HMDs, enabling subjects to participate in research using their own equipment. Concurrently, COVID-19 has forced VR researchers to rethink research practices to accommodate the contemporary health safety concerns. We therefore investigate whether crowdsourcing methods, that have been highly influential for research practices in other fields, can be used for VR studies that have other physical and cognitive conditions than many other laboratory studies.
Steed and colleagues (Steed et al., 2016) conducted an “in-the-wild” study using smartphone-based consumer VR equipment. In the study, participants were self-embodied with a virtual avatar, and effects on presence and embodiment were reported, even in uncontrolled settings with relatively low-fidelity VR equipment. Since then, consumer-oriented VR equipment has both increased in adoption and in fidelity, making more complex VR applications with higher participant counts feasible.
More recent examples of non-laboratory VR research have also emerged. Ma et al. (2018) created a list of validated crowdworkers who own HMDs, by asking them to take a photograph of their devices together with a unique identification code. A study involving handout of Google cardboard VR equipment showed evidence for online and unsupervised VR research (Mottelson and Hornbæk, 2017). The popular application VRchat has also been used to conduct supervised VR user studies (Saffo et al., 2020). The crowdsourcing platform LabInTheWild has also featured a desktop-VR study (Huber and Gajos, 2020).
Together these works find that it is possible to conduct VR experiments without a laboratory. In this work, we build upon this literature and mitigate a range of shortcomings. In particular, we present data from two crowdsourced VR studies with participants’ own high-immersion equipment, using Oculus Quest HMDs, including both subjective data (survey responses) and objective data (hand tracking), and with a high number of participants sourced during the course of relatively few days. The first study collected within-VR survey data. The second study collected hand tracking data. Together they provide breadth in the recommendations on data quality assurance for unsupervised VR experiments.
Materials and Equipment
VR experiments designed for online participation and without presence of experimenters pose a number of resource requirements. Our methodology could potentially work with any mixed reality technology. Here, we focus on Oculus Quest, as it has widespread adoptance, and since it is a standalone HMD that does not require a PC, HMD, and a tracking setup. This HMD also has built-in hand tracking, which we utilize and validate in Study II. Any VR development engine would work for the purpose of conducting unsupervised VR user studies; we validate our proposed experimental designs using environments developed in Unity 2020. As our experimental applications do not have raison d’être beyond experimental research, it is not feasible to deploy these to the official Oculus app store; but rather, we distribute it using the unofficial app store SideQuest5. We see this, however, as an opportunity for larger scale empirical VR research [e.g., in line with Henze et al. (2011)].
Methods
Our methodology allows for arbitrary research designs. In Study I we employ a between-subjects design where a pedagogical avatar is independently manipulated; in Study II we employ a within-subjects with a 3D guidance technique as independent variable. We assign the condition (or order of) during run-time on the device. Consequently, we cannot guarantee a perfect distribution of participants across conditions (which would be possible with an API assignment of condition, which could however have implications for validity if certain conditions lead to higher drop-out rate). The procedure for conducting an online and unsupervised VR study using our proposed methodology follows three groups of steps which are described below. We validate this practice through Study I and II.
Pre study steps:
1. Develop simple backend API (POST user data, GET all data)
2. Implement VR application, build binary APK, send data to API upon completion/during runtime
3. Include a pre-study data qualification step (e.g., for tracking movement)
4. If needed, open an in-VR browser-based survey upon completion to collect relevant data (e.g., participant emails for reimbursement)
5. Pilot test app, align hypotheses, pre-registration, outlier critera
6. Submit APK to SideQuest, and await approval
Study steps:
1. Advertise study on relevant social media
2. Run study (suggested duration of two weeks)
3. Reimburse participants
Post study steps:
1. Extract all responses from API
2. Verify close-to even distribution of conditions
3. Conduct x2 tests on demographic data
4. Perform statistical analyses documented during pre-registration
Study I: Survey Responses
The purpose of this study was to investigate learning outcomes of a virtual lesson with a virtual guide (Petersen et al., 2021). Using a multiple-choice questionnaire (MCQ), we tested participants’ factual and conceptual knowledge of the topic of viruses within VR. This was done both before and after the simulation to estimate knowledge acquisition. Subjective measures about the virtual guide (e.g., uncanny valley from Ho and MacDorman (2017) and social presence from Makransky et al. (2017)) were also collected.
The study was conducted online and unsupervised. An identical follow-up supervised laboratory study was conducted, permitting comparison of data between study places. The duration of the study was approximately 60 min (from installation to completion). The laboratory study took relevant COVID-19 precautionary measures, such as cleaning all equipment after use, requiring participants to use hand disinfectant, and using a Cleanbox CX16 for decontaminating headsets with UVC light.
Participants
This study was conducted both as an unsupervised online study and as a supervised laboratory study. The first study had a total of 161 participants (83% male/15% female/2% non-binary; age M = 23.5, SD = 10.5), recruited on social media, who participated in the experiment using their own VR headset over the course of 11 days. They were reimbursed with a gift certificate worth $15 USD (or the equivalent in their preferred currency). The second study was conducted in our laboratory with 185 s year university students (79% female/21% male/0% non-binary; age M = 27.5, SD = 12.6), who participated for course credits.
Apparatus
The environment was developed in Unity 2020, deployed to Oculus Quest (1st gen). We used 3D models from the Unity Asset Store. Participants installed the experimental application using SideQuest7. A Python-based backend application running at Google App Engine stored user data and provided unique within-VR confirmation codes that participants entered into a survey at Google Forms to confirm participation and subsequent reimbursement.
Survey Data
We collected two types of survey data (see Table 1): objective (two learning outcomes measured twice) and subjective (three variables about avatars and one about enjoyment). We analyze the reliability of these variables to investigate the feasibility of collecting in-VR questionnaire responses.
Multiple-choice Questionnaire
We administered an objective MCQ both before and after the virtual lesson to measure knowledge acquisition. The test had 20 questions using the distinction from Anderson et al. (2001) between factual (e.g., numbers, places, years) and conceptual knowledge (e.g., explaining, connecting, transferring). Each question had four available answers. Answers were selected using the hand-held controller (see Figure 1).
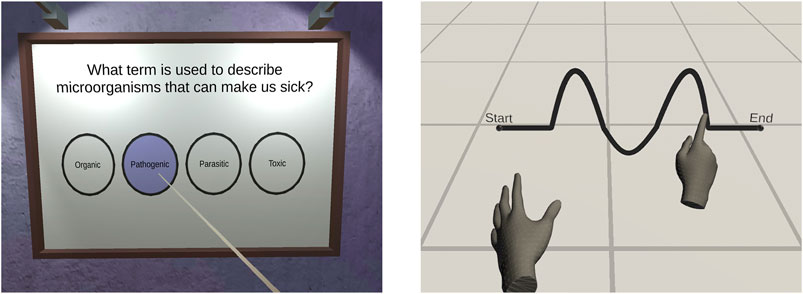
FIGURE 1. In this paper we report the experiences with conducting two fundamentally different unsupervised VR studies: Study I (left) collected subjective and objective in-VR survey responses related to learning; Study II (right) collected hand tracking data for mid-air gesture guidance.
Eight participants in the online study had a negative pre-to post score. Thirteen participants had identical scores before and after the lesson, which were not due to ceiling effects, with a mean score of 12.7 (SD = 2.8). We can therefore assume that about 13% of the cohort did not pay an ideal amount of attention to the study. For the laboratory study we identify 3% who had negative or zero increase in knowledge scores. The fraction of aberrant responses in the unsupervised study is thus higher, which warrants an objective exclusion criteria defined before data collection for unsupervised VR experimentation (for instance, as measured in a post test). Nevertheless, the vast majority of participants completed the study with the expected attention.
Participants improved their test scores as a result of participating in the study (see Figure 2). Comparing the online and laboratory scores, we observe a more cohesive distribution of test scores in the laboratory study. Regardless, the data shows that conducting an unsupervised VR study online is feasible, and that objective survey responses (and improvement thereof) are obtainable through this paradigm.
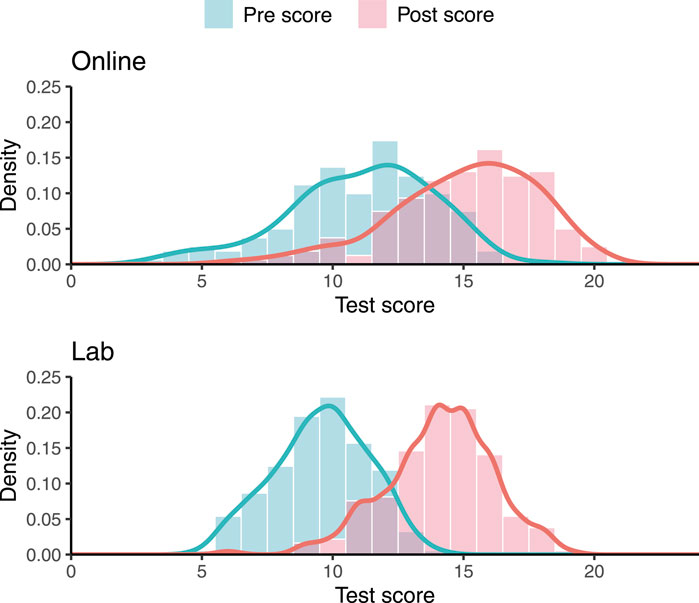
FIGURE 2. Histograms of correct answers to the MCQ (factual and conceptual scores combined). Online N = 161, Lab N = 185. Participants in both studies significantly increased their test scores.
The effect size for factual knowledge acquisition was large in both studies (d = 1.6 and 2.6, respectively; see Table 2). For conceptual knowledge acquisition we observe medium-to-large effect sizes (d = 0.7 and 0.9, respectively; see Table 2). We observe that the laboratory study resulted in the largest effect sizes, presumably because of a reduced number of aberrant responses, and because of demographic differences.
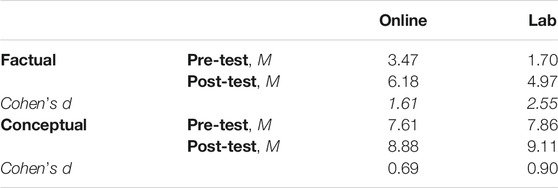
TABLE 2. Mean scores from a 20 item multiple-choice test (10 factual and 10 conceptual questions). The lab study shows larger effect sizes for pre-to post score, yet with same trend.
Subjective Data
We collected three validated subjective measures, each a mean of five items (see Table 1). To estimate the reliability of responses we compare the Cronbach’s α of the responses from the online study, the laboratory study, and with the original scale validation. Note that the Cronbach’s α for the Uncanny Valley questionnaire (Ho and MacDorman, 2017) is a mean across three dimensions; we, however, excluded Eeriness to reduce the number of questions.
Table 3 shows the reliability measures for our VR studies conducted unsupervised and supervised, respectively; the last column shows the α as reported by the scale validation study. Depending on the source, the values all fall into the category “Good consistency” (
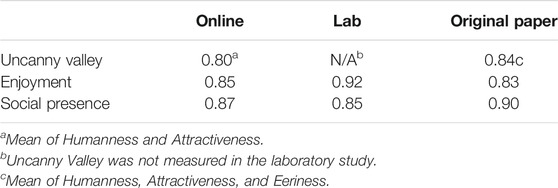
TABLE 3. Cronbach’s α for subjective measures. We report similar reliability measures compared between online and lab studies, and in comparison to the original scale.
Summary of Study I
The participants significantly increased knowledge from the virtual lesson and found it highly enjoyable. The main empirical contributions are that virtual guides impact factual and conceptual learning differently. As for factual information, a virtual guide risks diminishing one’s learning compared to learning from just a narration; this is not the case when learning about conceptual information (here, a virtual guide may aid learning). Both the subjective and objective survey data collected in-VR as part of the study showed good reliability, and comparable to a laboratory study. The online study had an increased number of inattentive participants; this issue is however possible to address with data sanitation protocols.
Study II: Hand Tracking
In this study participants learned mid-air gestures with help of a novel guidance technique that corrected the avatar hand movements during training. The purpose of the study was to acquire evidence showing that correcting the avatar movements can be used to support motor learning (Lilija et al., 2021). The investigated technique was compared to a conventional hand guidance technique (ghosted hand) and a control condition (no guidance) in a within-subject study design. The target gestures required a smooth movement from left to right, tracing an invisible path in mid-air with the index finger.
Despite the fact that hand tracking for VR has seen increased research attention and support in consumer-oriented VR technology in recent years, built-in HMD solutions require line-of-sight and optimal lighting conditions to work properly. Collecting hand tracking data unsupervised therefore required an additional effort in securing stable tracking data. The study was conducted online and unsupervised. The collected data shows that conducting valid hand tracking is feasible by involving only a few steps for data qualification. The study took approximately 20 min from installation to completion.
Participants
A pilot study had 30 participants; 1 female, 29 male, 0 non-binary; ages 18–50 (
Apparatus
The virtual environment was developed in Unity 2020 and deployed to Oculus Quest (1st and 2nd gen). Participants installed the experimental application using SideQuest. The application tracked finger and head movements. Upon completing the study, a unique participant ID was generated and participants entered it into a Google Forms survey to match collected demographics data. Log files of about 2 MB per participant were posted to Amazon Web Services.
Collecting Motor Data
The purpose of the study was to investigate a novel technique’s ability to support gesture training in VR, and hence motor learning. To evaluate the navigation techniques, quality tracking data is a necessity (e.g., to speculate whether certain interventions lead to more accurate movements). A pilot study revealed, however, that sub-optimal hand tracking hampered such analyses. Out of 30 data sets from the pilot study, eight of them (27%) had to be removed from the analysis due to low quality hand tracking. We determined the quality of hand tracking by measuring the jitter experienced during the experiment. The jitter was defined as an abnormal distance between the index finger in two adjacent rendered frames. In the main study, we added a screening test to encourage participants to improve their environmental conditions. The screening test consisted of a simple selection task forcing the participants to move their hand in the relevant interaction space. During this task we measured the jitter of the index finger, frames with low-confidence tracking and head location. The participants could only proceed to the experiment if their tracking quality was satisfactory and if they followed the instructions (e.g., standing at the marked location). If the tracking quality was deemed unsatisfactory, the participants were encouraged to move to a brighter location, stand in the marked area, and keep their hand within line-of-sight. Additionally, we evaluated the hand tracking quality post-study. Once again, we computed the number of jitters and low-confidence frames experienced during the experiment. We excluded three participants (8%) that passed the screening test and still experienced poor hand tracking. The screening test notably lowered the data rejection rate from 27 to 8%.
Findings
The collected data contains participant finger and head movement in 3D. During training, the participants performed as many gestures as possible within 40 s. Later they were given a short-term retention test in which they performed the same gesture as many times as possible within 20 s. The training and short-term retention test was administered within-subject for each of the three conditions (correction of movement, control, and ghosted hand). To provide an overview of said data, we plot participant’s movements in 2D (see Figure 3), collected from the interface shown in Figure 1.
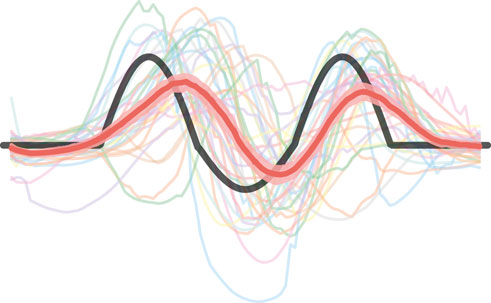
FIGURE 3. Line plots of finger movements (X,Y) for a particular gesture. The thick black line denotes the target gesture, the red line shows the mean gesture performed across all participants with the error band showing the 0.99 CI. The thin colored lines each represent the mean gesture across one participant’s trials. Together the plot shows valid finger tracking data from unsupervised VR study.
The thin colored curves show individual participant movement data, averaged. The red curve shows the mean across all participants, with the error band showing 0.99 confidence interval, with 100 equidistant samples on the x-axis. The resulting plot reveals little variation in the continuity of participants’ gestures, and hence supports the validity of the entailed data collection and cleaning techniques. The collected data allowed us to compare the number of repetitions, movement accuracy, and learning effect between the conditions. We found out that training with the correction technique significantly outperformed the control condition and performed equally well as a popular hand guidance technique (ghosted hand). Both the pilot study and the main study corroborated this conclusion, giving confidence in the collected data.
Summary of Study II
Study II investigated a novel interaction technique for supporting hand gesture learning. We conducted two remote unsupervised experiments (pilot and main) with a total of 69 participants in which we tracked the participants’ finger and head movement in 3D. We found out that the investigated technique significantly improved the short-term retention of the trained movement when compared to the control condition. Furthermore, the technique performed equally well as a conventional technique for supporting hand gesture learning (ghosted hand).
The issue of collecting quality hand tracking data for our study forced us to introduce interventions to the study procedure as well as post-hoc data analysis to reduce the amount of unfit data. We greatly reduced the problematic data collection using the described methods, and hence conclude that unsupervised VR studies concerning motor learning are indeed feasible.
Demographics
The data presented in this paper is mainly sourced from unsupervised VR user studies, recruited online. The participants hence privately own a VR headset (specifically Oculus Quest) and were willing to install a custom application onto their device to participate in our research. This undeniably places some technical burden onto the participant, which may limit generalizability of findings. To understand the demographics of the population these studies have been sampling from, we summarise unique participant records (N = 226).
The population mostly identifies as male (86%), some female (12%), and few as non-binary (2%). The median age was 26 years (SD = 10.0). Figure 4 shows the breakdown of gender and age (non-binary omitted for too few data points). The homogeneity of age, and especially gender, should be taken into account when recruiting for user studies; for experiments requiring a heterogeneous sample, other solutions could probably yield a more representative sample.
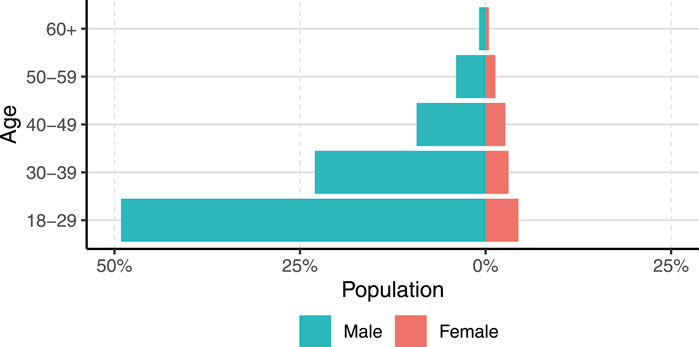
FIGURE 4. Our records show a majority young and male population, with 86% male and approximately half of the sample being males between 18 and 29 years.
As with age and gender, we observe a fairly homogeneous population with regards to prior experience with VR (see Figure 5). Not surprisingly, we observe a majority VR expert population. The experience of the population might limit the generalizability of usability studies conducted this way; but conversely support other types of VR user studies such as expert evaluations (Klas, 2012) while also mitigating novelty effects (Koch et al., 2018).
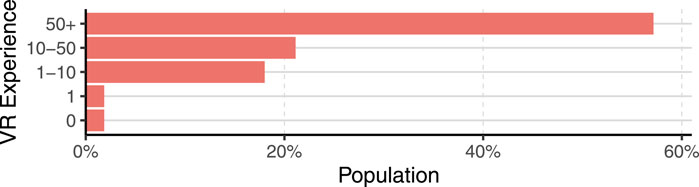
FIGURE 5. VR experience by number of times a participant has previously been immersed. The majority of the population are expert users with more than fifty previous uses. This limits risks of novelty-effects and enables expert evaluations, but has less generalizability.
Approximately half of the population came from the United States (based on location, not country of origin). The list of countries from where participants were situated includes countries from Northern and Southern America, Europe, Middle East, and Asia (see Figure 6).
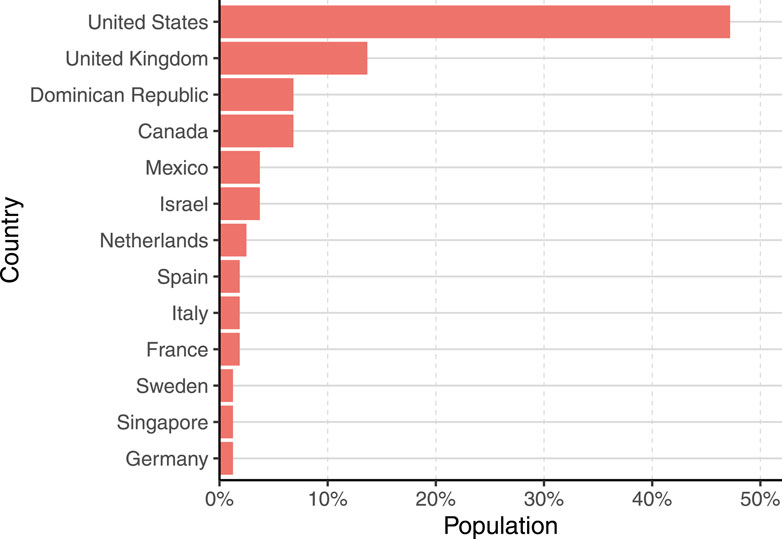
FIGURE 6. List of countries participants were situated in while conducting the studies. The list omits countries with less than 1% representation.
The population was relatively well educated (see Figure 7). We speculate the educational level reported is also a function of the relative young age of the participants.
Size of Population
In this paper we report data from three unsupervised studies (
Discussion
Based on our experiences we here discuss practical as well as methodological considerations with regards to conducting unsupervised VR studies with online recruitment.
Recruitment and Reimbursement
We advertised our studies across social media (Facebook, Twitter, Reddit) with regular posts. The vast majority of participants came from Reddit; specifically from relevant VR communities at the subreddits r/OculusQuest, r/oculus, r/sidequest, and r/virtualreality. We reimbursed participation with Amazon and Steam gift cards. Amazon gift cards showed as non-ideal as their use are limited to one country only, requiring manual registration across many Amazon stores; also Amazon vouchers cannot easily be bought in bulk. Steam vouchers are also not ideal for reimbursement as they involve stating the receiver’s username upon purchase, and because they cannot be bought in bulk. For future studies, we encourage researchers who are interested in reimbursing unsupervised VR participants recruited online to look for a service that delivers world-wide vouchers in bulk.
Consent
We acquired informed consent in participants’ desktop browser before they installed the experimental application (using the World Health Organization, 2021) standard for human experimental participation). We do not see any technical reason why informed consent could not be prompted upon opening the application, if researchers prefer to have the entire study process limited to in-VR only.
Data Quality
We have reported some techniques for quality assurance of data depending on the type of data collected. We estimate an approximate 10% ill-intended user participation which can be mitigated with appropriate practices. We recommend having prior objective procedures for excluding participants (e.g., duration, tracking quality, verifiable questions), in addition to post-hoc data cleaning techniques (e.g., outlier detection, inconsistency in survey responses, identical responses across questions). These should be prepared as part of the pre-registration. Additionally, we recommend logging the number of times a participant took off their HMD to measure interruptions (e.g., using OVRManager.HMDUnmounted). We also recommend storing the unique device ID to limit participation to once per device (e.g., through SystemInfo.deviceUniqueIdentifier); note however, that this also prohibits acquaintances of the HMD owner to participate using the same device. To respect privacy, a hash of the identifier could be used instead as to avoid storing of personal identifiable information. In summary, in assuring data quality we recommend the following procedures:
• Check for internet connectivity upon initialization
• Check if HMD was taken off using OVRManager.HMDUnmounted
• Store SystemInfo.deviceUniqueIdentifier (or hash of) to avoid multiple participation
• Report the number of drop-outs (e.g., as number of downloads, installs, and completions), and ensure these are not skewed by experimental manipulation
• Measure duration and flag outliers, e.g., M ± 3 SD
• Check for inconsistency in survey responses
• Perform height calibration
• For hand tracking, have a data quality screening phase
• For hand tracking, count low confidence frames and jitter
• Define objective exclusion criteria before collecting data based on the pilot study
• Ask about tracking issues, distractions, or completion strategies to reveal invalid responses
• Estimate about 10% aberrant responses
Sample
As noted above, the sample subjected to analyses in this paper primarily consists of males in their mid twenties who are well-educated and can be described as VR-experts. Comparing our sample to the subset of crowdworkers who are HMD-owners shows a similar biased representation. Kelly et al. (2021) recently conducted a survey of headset owners and non-owners in online platforms (AMT and Prolific), comparing an undergraduate participant pool, also showed a negligible proportion of women and elders among HMD owners. Naturally, this imposes some limitations on studies conducted this way; specifically with regard to generalizability of findings.
Peck et al. (2020) showed how underrepresentation of females in VR research causes systematic bias of findings. Specifically, the authors show how simulator sickness increases with higher proportion of female participants. Studies conducted unsupervised and online will likely be exposed to the same bias issues. With few options of immediately extending the demographics of consumer-oriented VR owners, the limited diversity in the potential sample should be considered early for these studies. As advised by Peck et al. (2020), demographics of participants, including age, gender, and race/ethnicity, should be reported. Also, claims about generalizability should be avoided. Finally to mitigate the gender bias concerns, the sample bias should be considered during preregistration, and could entail a commitment to reporting differences in effects across genders.
The sample consisted predominately of VR experts. While this imposes restrictions for some study purposes (e.g., usability), we mainly see this as an opportunity to conduct VR studies with reduced risk of novelty effects. While a sample of enthusiast VR users mostly with considerable VR experience poses certain limitations to studies regarding for instance usability, it severely limits first-time effects that are widespread across immersive media studies (Hopp and Gangadharbatla, 2016), which are oftentimes a result of an overwhelming experience of immersion.
Implications for VR Studies
This paper has a number of implications for future VR user study designs. Overall, the results suggest that online VR studies are viable, and that data sourced this way is only modestly harder to quality assure compared to traditional laboratory studies. This way, researchers can conduct unsupervised VR studies with large numbers of participants from diverse countries in a relatively short amount of time, without compromising validity of conclusions drawn from the data. The issues revolving practical matters usually associated with traditional VR studies, such as facilitating technical equipment and allocating physical infrastructure, could be greatly reduced. Certain measures must, however, be taken to ensure quality data; we have listed practical recommendations to this end.
The fact that Study I included both an online and a laboratory study gave important insights into the feasibility of conducting both types of experiments during a global health crisis. While the latter is possible if one takes relevant precautionary safety measures, online experiments are an easier and safer alternative as human contact is eliminated altogether.
Study II showed that conducting unsupervised VR studies with a focus on hand tracking is viable, with important quality assurance steps imposed on the study procedure. We foresee this could have a great impact on VR embodiment research, such as cognitive or behavioral aspects of body ownership. As studies in this domain typically involve relatively few subjects due to the complex nature of these studies, this could positively affect generalizability of findings with regards to embodiment.
Limitations
Conducting remote and unsupervised user studies impose certain limitations. For instance, over-recruitment is necessary because of the number of aberrant responses when conducting studies this way. Compared to physical studies with higher internal experimental control, a larger number of people will fail to pay attention, or go through the procedures quickly, without the presence of an evaluation. Methods for mitigating these issues known from the crowdsourcing literature apply to VR studies too, such as defining outlier criteria and including verifiable questions [e.g., Kittur et al. (2008)]. As an additional step, especially relevant when quality movement tracking is necessary, we recommend including a pre-study tracking confidence test that tests the physical space, potentially asking the participant to move to a more suitable location (e.g., for better lighting or extended space). During this phase it should also be underlined whether the study requires a sitting or standing VR setup.
Conducting unsupervised studies in the confines of participants’ own homes reduces opportunities for studies requiring large movement space. VR studies conducted unsupervised should ideally communicate physical requirements (e.g., standing, sitting, moving) before study participation. Certain types of studies are therefore less ideal considering participants’ surroundings, such as redirected walking or other locomotion techniques. Conversely, the home surroundings of participants pose design opportunities for VR research, such as self-therapy, or ecological system evaluations.
Number of quantitative insights are the prime argument for conducting VR studies unsupervised. Conversely, collecting qualitative data this way is harder. Some research suggests the use of VR platforms such as VRChat to facilitate supervised and online VR experimentation (Saffo et al., 2020; Rivu et al., 2021). Our studies have mostly focused on quantitative findings, and the qualitative feedback we received was therefore limited. Further studies could cultivate methodologies for remote studies with a qualitative focus.
Conclusion
Through two case studies we report the experiences with conducting unsupervised VR experiments online during the COVID-19 pandemic. We collected subjective questionnaire responses in-VR in an educational study, and we collected hand tracking data for a gesture elicitation study. We provide a wealth of suggestions for ensuring data quality from experiments run this way, including experimental design considerations, implementation strategies, practical considerations regarding recruitment and reimbursement, and data analysis and quality assurance. Regardless of the availability of laboratory, VR user studies conducted enable diverse and high number of participants at lower costs, at limited and manageable risks for data quality.
Data Availability Statement
The raw data supporting the conclusions of this article will be made available by the authors, without undue reservation.
Ethics Statement
The studies involving human participants were reviewed and approved by Department of Pscyhology, University of Copenhagen. The patients/participants provided their written informed consent to participate in this study.
Author Contributions
GP, AM, and GM designed and conducted Study I; KL designed and conducted Study II; AM, GP, and KL conducted the statistical analyses; AM made figures; AM, GM, KL, and GM wrote the manuscript.
Funding
This research was supported by Innovation fund Denmark under the agreement “SIPROS”, UCPHs Data+ pool under the agreement “Quantifying Body Ownership”.
Conflict of Interest
The authors declare that the research was conducted in the absence of any commercial or financial relationships that could be construed as a potential conflict of interest.
Footnotes
4https://www.reddit.com/r/OculusQuest
References
Anderson, C. A., Allen, J. J., Plante, C., Quigley-McBride, A., Lovett, A., and Rokkum, J. N. (2019). The Mturkification of Social and Personality Psychology. Pers Soc. Psychol. Bull. 45, 842–850. doi:10.1177/0146167218798821
Anderson, L. W., Krathwohl, D. R., Airasian, P. W., Cruikshank, K. A., Mayer, R. E., Pintrich, P. R., et al. (2001). A Taxonomy for Learning, Teaching, and Assessing: A Revision of Bloom’s Taxonomy of Educational Objectives. (New York: Addison Wesley Longman).
Behrend, T. S., Sharek, D. J., Meade, A. W., and Wiebe, E. N. (2011). The Viability of Crowdsourcing for Survey Research. Behav. Res. 43, 800–813. doi:10.3758/s13428-011-0081-0
Bradeško, L., Witbrock, M., Starc, J., Herga, Z., Grobelnik, M., and Mladenić, D. (2017). Curious cat–mobile, Context-Aware Conversational Crowdsourcing Knowledge Acquisition. ACM Trans. Inf. Syst. 35, 1-46. doi:10.1145/3086686
Cheng, J., Teevan, J., and Bernstein, M. S. (2015). Measuring Crowdsourcing Effort with Error-Time Curves. In Proceedings of the 33rd Annual ACM Conference on Human Factors in Computing Systems, 1365–1374. doi:10.1145/2702123.2702145
Cipresso, P., Giglioli, I. A. C., Raya, M. A., and Riva, G. (2018). The Past, Present, and Future of Virtual and Augmented Reality Research: a Network and Cluster Analysis of the Literature. Front. Psychol. 9, 2086. doi:10.3389/fpsyg.2018.02086
Clark, R. E. (1983). Reconsidering Research on Learning from media. Rev. Educ. Res. 53, 445–459. doi:10.3102/00346543053004445
Cooper, S., Khatib, F., Treuille, A., Barbero, J., Lee, J., Beenen, M., et al. (2010). Predicting Protein Structures with a Multiplayer Online Game. Nature. 466, 756–760. doi:10.1038/nature09304
Crump, M. J. C., McDonnell, J. V., and Gureckis, T. M. (2013). Evaluating Amazon's Mechanical Turk as a Tool for Experimental Behavioral Research. PLOS ONE. 8, e57410–18. doi:10.1371/journal.pone.0057410
Daniel, F., Kucherbaev, P., Cappiello, C., Benatallah, B., and Allahbakhsh, M. (2018). Quality Control in Crowdsourcing. ACM Comput. Surv. 51, 1–40. doi:10.1145/3148148
Deng, J., Dong, W., Socher, R., Li, L.-J., Kai Li, Kai., and Li Fei-Fei, Li. (2009). Imagenet: A Large-Scale Hierarchical Image Database. In 2009 IEEE Conference on Computer Vision and Pattern Recognition, 248–255. doi:10.1109/CVPR.2009.5206848
Estellés-Arolas, E., and González-Ladrón-de-Guevara, F. (2012). Towards an Integrated Crowdsourcing Definition. J. Inf. Sci. 38, 189–200. doi:10.1177/0165551512437638
Feyisetan, O., Simperl, E., Van Kleek, M., and Shadbolt, N. (2015). Improving Paid Microtasks through Gamification and Adaptive Furtherance Incentives. In Proceedings Of the 24th International Conference On World Wide Web (Republic and Canton of Geneva, CHE: International World Wide Web Conferences Steering Committee)WWW ’15, Florence, Italy, 333–343. doi:10.1145/2736277.2741639
Gadiraju, U., Kawase, R., Dietze, S., and Demartini, G. (2015). Understanding Malicious Behavior in Crowdsourcing Platforms. In Proceedings of the 33rd Annual ACM Conference on Human Factors in Computing Systems. (New York, NY, USA: Association for Computing Machinery), 1631–1640. doi:10.1145/2702123.2702443
Goodman, J. K., and Paolacci, G. (2017). Crowdsourcing Consumer Research. J. Consumer Res. 44, 196–210. doi:10.1093/jcr/ucx047
Heer, J., and Bostock, M. (2010). Crowdsourcing Graphical Perception. In Proceedings of the SIGCHI Conference on Human Factors in Computing Systems. (New York, NY, USA: Association for Computing Machinery), 203–212. doi:10.1145/1753326.1753357
Henze, N., Pielot, M., Poppinga, B., Schinke, T., and Boll, S. (2011). My App Is an Experiment. Int. J. Mobile Hum. Computer Interaction (Ijmhci). 3, 71–91. doi:10.4018/jmhci.2011100105
Ho, C.-C., and MacDorman, K. F. (2017). Measuring the Uncanny Valley Effect. Int. J. Soc. Robotics 9, 129–139. doi:10.1007/s12369-016-0380-9
Hopp, T., and Gangadharbatla, H. (2016). Novelty Effects in Augmented Reality Advertising Environments: The Influence of Exposure Time and Self-Efficacy. J. Curr. Issues Res. Advertising. 37, 113–130. doi:10.1080/10641734.2016.1171179
Huber, B., and Gajos, K. Z. (2020). Conducting Online Virtual Environment Experiments with Uncompensated, Unsupervised Samples. PLOS ONE. 15, e0227629–17. doi:10.1371/journal.pone.0227629
Insider, A. R. (2020). Has Oculus Quest Sold One-Million Lifetime Units? Available at: https://arinsider.co/2020/09/21/has-oculus-quest-sold-one-million-units/ (Online; Accessed November 1, 2020).
Kelly, J. W., Cherep, L. A., Lim, A., Doty, T., and Gilbert, S. B. (2021). Who Are Virtual Reality Headset Owners? a Survey and Comparison of Headset Owners and Non-owners. PsyArXiv. doi:10.1109/vr50410.2021.00095
Kittur, A., Chi, E. H., and Suh, B. (2008). Crowdsourcing User Studies with Mechanical Turk. In Proceedings of the SIGCHI Conference on Human Factors in Computing Systems. (New York, NY, USA: Association for Computing Machinery), 453–456. doi:10.1145/1357054.1357127
Klas, C.-P. (2018). User Studies for Digital Library Development. Expert evaluation methods. (Cambridge, UK: Facet).
Koch, M., von Luck, K., Schwarzer, J., and Draheim, S. (2018). The novelty Effect in Large Display Deployments–Experiences and Lessons-Learned for Evaluating Prototypes. In Proceedings of 16th European Conference on Computer-Supported Cooperative Work-Exploratory PapersEuropean Society for Socially Embedded Technologies (EUSSET), Nancy, France.
Lilija, K., Kyllingsbaek, S., and Hornbaek, K. (2021). Correction of Avatar Hand Movements Supports Learning of a Motor Skill. In 2021 IEEE Virtual Reality ConferenceVR, Lisboa, Portugal. doi:10.1109/VR50410.2021.00069
Ma, X., Cackett, M., Park, L., Chien, E., and Naaman, M. (2018). Web-based Vr Experiments Powered by the Crowd. In Proceedings Of the 2018 World Wide Web Conference (Republic and Canton of Geneva, CHE: International World Wide Web Conferences Steering Committee)WWW ’18, Lyon, France, 33–43. doi:10.1145/3178876.3186034
Makransky, G., and Lilleholt, L. (2018). A Structural Equation Modeling Investigation of the Emotional Value of Immersive Virtual Reality in Education. Education Tech Res. Dev. 66, 1141–1164. doi:10.1007/s11423-018-9581-2
Makransky, G., Lilleholt, L., and Aaby, A. (2017). Development and Validation of the Multimodal Presence Scale for Virtual Reality Environments: A Confirmatory Factor Analysis and Item Response Theory Approach. Comput. Hum. Behav. 72, 276–285. doi:10.1016/j.chb.2017.02.06610.1016/j.chb.2017.02.066
Mottelson, A., and Hornbæk, K. (2017). Virtual Reality Studies outside the Laboratory. In Proceedings of the 23rd ACM Symposium on Virtual Reality Software and Technology. (New York, NY, USA: Association for Computing Machinery). doi:10.1145/3139131.3139141
Paolacci, G., Chandler, J., and Ipeirotis, P. (2010). Running Experiments Using Amazon Mechanical Turk. Judgment Decis. Making 5, 411–419.
Peck, T. C., Sockol, L. E., and Hancock, S. M. (2020). Mind the gap: The Underrepresentation of Female Participants and Authors in Virtual Reality Research. IEEE Trans. Vis. Comput. Graphics. 26, 1945–1954. doi:10.1109/TVCG.2020.2973498
Petersen, G. B., Mottelson, A., and Makransky, G. (2021). Pedagogical Agents in Educational Vr: An in the Wild Study. In Proceedings of the 2021 CHI Conference on Human Factors in Computing Systems. (New York, NY, USA: Association for Computing Machinery). doi:10.1145/3411764.3445760
Radianti, J., Majchrzak, T. A., Fromm, J., and Wohlgenannt, I. (2020). A Systematic Review of Immersive Virtual Reality Applications for Higher Education: Design Elements, Lessons Learned, and Research Agenda. Comput. Education. 147, 103778. doi:10.1016/j.compedu.2019.103778
Rivu, R., Mäkelä, V., Prange, S., Rodriguez, S. D., Piening, R., Zhou, Y., et al. (2021). Remote Vr Studies–A Framework for Running Virtual Reality Studies Remotely via Participant-Owned Hmds. arXiv preprint arXiv:2102.11207
Saffo, D., Yildirim, C., Di Bartolomeo, S., and Dunne, C. (2020). Crowdsourcing Virtual Reality Experiments Using Vrchat. In Extended Abstracts of the 2020 CHI Conference on Human Factors in Computing Systems. (New York, NY, USA: Association for Computing Machinery), 1–8. doi:10.1145/3334480.3382829
Sheehan, K. B. (2018). Crowdsourcing Research: Data Collection with Amazon's Mechanical Turk. Commun. Monogr. 85, 140–156. doi:10.1080/03637751.2017.1342043
Steed, A., Frlston, S., Lopez, M. M., Drummond, J., Pan, Y., and Swapp, D. (2016). An 'In the Wild' Experiment on Presence and Embodiment Using Consumer Virtual Reality Equipment. IEEE Trans. Vis. Comput. Graphics 22, 1406–1414. doi:10.1109/tvcg.2016.2518135
Stewart, N., Ungemach, C., Harris, A., Bartels, D., Newell, B., Paolacci, G., et al. (2015). The Average Laboratory Samples a Population of 7,300 Amazon Mechanical Turk Workers. Judgment And Decision Making X, 10, 5.
von Ahn, L., Maurer, B., McMillen, C., Abraham, D., and Blum, M. (2008). Recaptcha: Human-Based Character Recognition via Web Security Measures. Science. 321, 1465–1468. doi:10.1126/science.1160379
World Health Organization (2021). Templates for Informed Consent Forms. Available at: https://www.who.int/groups/research-ethics-review-committee/guidelines-on-submitting-research-proposals-for-ethics-review/templates-for-informed-consent-forms (Online; accessed May 1, 2021).
Keywords: virtual reality, COVID-19, user studies, crowdsourcing, online experiments
Citation: Mottelson A, Petersen GB, Lilija K and Makransky G (2021) Conducting Unsupervised Virtual Reality User Studies Online. Front. Virtual Real. 2:681482. doi: 10.3389/frvir.2021.681482
Received: 16 March 2021; Accepted: 14 May 2021;
Published: 28 May 2021.
Edited by:
Michael Madary, University of the Pacific, United StatesReviewed by:
Richard Skarbez, La Trobe University, AustraliaMary C. Whitton, University of North Carolina at Chapel Hill, United States
Copyright © 2021 Mottelson, Petersen, Lilija and Makransky. This is an open-access article distributed under the terms of the Creative Commons Attribution License (CC BY). The use, distribution or reproduction in other forums is permitted, provided the original author(s) and the copyright owner(s) are credited and that the original publication in this journal is cited, in accordance with accepted academic practice. No use, distribution or reproduction is permitted which does not comply with these terms.
*Correspondence: Aske Mottelson, amot@psy.ku.dk