- 1Department of Computer Science, University of Central Florida, Orlando, FL, United States
- 2Department of Computer and Information Sciences and Engineering, University of Florida, Orlando, FL, United States
- 3Speech Science, The University of Auckland, Auckland, New Zealand
Increasingly, virtual environments are being used in educational and training applications. As with other types of applications that use virtual environments, these scenarios must be evaluated in terms of user experience. However, they also should be evaluated on the efficacy of the training or learning provided, so as to ensure learning transfer. Frameworks, such as the Kirkpatrick Model, exist to evaluate training scenarios, but application of these frameworks has not been fully utilized in development of virtual environment-based education and training. To address this gap and to also share our process with other virtual environment developers, we discuss our experience applying the Kirkpatrick Model to an existing virtual human (VH) application for medical communication skills training. The Kirkpatrick Model provides different levels of evaluation for training programs that include learners’ reactions to the training, the knowledge acquired from the training, behaviors indicating the training was applied, and the degree high-level results were impacted as a result of the training. While we discuss all of the Model’s levels, our focus for this work is Level 3 Behavior. The Kirkpatrick Model currently recommends that behavioral change may only be measured while a trainee is working in a real-world context. However, given existing evidence that VH applications have been shown to elicit real-world behaviors from participants, we suggest that VH training scenarios may be a method of measuring Behavior level metrics before trainees are evaluated in situ. Initial support for this suggestion is provided by our study examining whether VHs can elicit changes in communication skills learners’ message production behavior over time. This study indicates that learners displayed changes in several metrics over the course of the semester. Based on this finding, we suggest a direction for future research: observing learner behavior in a virtual environment as a pre-cursor to behavioral measures while in a real-world scenario.
1 Introduction
Increasingly, virtual environments are being used in educational and training applications in a variety of scenarios, such as medical training and interpersonal communication skill building (Xie et al., 2021). Thus, in addition to general concerns regarding the user experience of these applications, virtual environment developers must often also evaluate the training provided by these scenarios. However, evaluating this training aspect of virtual environments can be challenging: in a recent review of virtual reality (VR) applications for skills training, Xie et al. note that identifying the particular factors of training that should be targeted to ensure learning transfer is difficult (Xie et al., 2021). The authors also note the existence of specific concerns regarding learning transfer between virtual training and the real world (Xie et al., 2021).
In the medical domain, researchers have applied the Kirkpatrick Model to address this question of how to evaluate educational virtual environments to target learning transfer (see Zaveri et al. (2016); Kundhal and Grantcharov (2009); Beal et al. (2017); Delisle et al. (2019) for examples). The Kirkpatrick Model is often considered the gold standard for evaluation of training but has not yet been widely applied in virtual environment-based training. The Kirkpatrick Model has four different “levels” by which an training program may be evaluated: 1) Reaction, 2) Learning, 3) Behavior, and 4) Results. The Kirkpatrick Model is suitable for evaluating virtual environment-based education and training across a range of criteria: the Model includes typical user experience measures at the first (Reaction) level, such as engagement or satisfaction, while also providing guidance to identify measures focused on the learning outcomes of the training program. To identify these outcome-focused measures, the Kirkpatrick Model recommends beginning with the final level, the Results level, to ensure a training program meets an organization’s larger mission or purpose. Once potential measures for the results level are identified, training program developers can work backward from this larger vision to identify behaviors and skills that should be targeted in the training program itself.
In this paper, we recommend the application of the Kirkpatrick Model to educational and training applications using virtual environments and explain our process of applying the Kirkpatrick Model to virtual human (VH) healthcare communication skills training. While other medical education research has applied the Kirkpatrick Model for training program evaluation, we detail our process here so that developers of non-medical virtual environments or simulations may benefit from the best practices of the medical education community. Our work here describes the process of applying the Kirkpatrick Model to a desktop virtual human (VH) application for healthcare students’ communication skills. By using this process, we were able to identify relevant metrics that allowed us to evaluate whether VHs can elicit changes in healthcare students’ communication skills over time.
A brief overview of our process is as follows: we began by identifying an existing problem in healthcare communication, patient adherence. Patient adherence refers to the level patients follow the medical instructions given to them by their healthcare providers. While we did not measure patient adherence directly, identifying patient adherence as a Results level measure directly informed our lower-level measures, as suggested by the Kirkpatrick Model. By aiming to improve patient adherence, we were then able to identify healthcare provider behaviors to target in our application–those that promote higher patient adherence. We then identified six metrics related to a healthcare provider’s message production behavior, or how one transforms one’s thoughts into messages to communicate with others. While our focus for this work is primarily discussing the Behavior level of the Kirkpatrick Model, we also detail potential level two and level one measures for our communication skills training application.
In addition to discussing our application of the Kirkpatrick Model, we also suggest that educational VH scenarios may be applicable to several levels of the Kirkpatrick Model. The latest version of the Model states that Behavior measures may only be evaluated when learners apply training in real-world settings. However, as VHs can elicit real-world behaviors from participants (Cassell et al., 2009; Kleinsmith et al., 2015), we suggest that evaluations of behaviors may begin to be examined with VHs by using behavioral measures that can be used in both the virtual and real worlds. In other words, developers may be able to gain insight regarding the efficacy of the virtual environment training by incorporating behavioral measures in the VH training itself, potentially lessening the gap between the Learning and Behavior levels of the Model.
To add to the existing literature that suggests that VHs can elicit real-world behaviors, we present our study examining whether VHs can elicit changes in communication skills learners’ message production over time. For this study, we invited speech-language pathology students to interview two virtual patients (VPs) over the course of their academic semester. Using the Kirkpatrick Model, we identified six message production metrics that to target patient adherence, or the degree to which a patient follows their providers’ healthcare instructions. Using the VP interview data, we compared students’ message production at different points in their academic semester using these message production metrics. This study indicates that learners displayed changes in several metrics over the course of the semester, thus suggesting the potential for VHs to capture trainee behavioral data.
2 Related Work
In this section, we discuss previous applications of the Kirkpatrick Model to virtual environments for education and/or training. We briefly introduce the model in Section 2.1 for discussion purposes, but a fuller description of the Model and each level is provided in Section 3.2. We note that for brevity, when we discuss the Model, we refer to the New World Kirkpatrick Model, as presented in Kirkpatrick and Kirkpatrick’s 2016 book (Kirkpatrick and Kirkpatrick, 2016). This New World Model is the latest iteration of the Kirkpatrick Model first presented in Dr. Kirkpatrick’s dissertation in 1954 (Kirkpatrick, 1954). In Section 2.2, we also discuss existing methods for evaluating healthcare communication skills training while in virtual environments, as medical communication skills training is the educational domain of interest for our VH scenario.
It is important to note that systems deploying virtual environments may cover systems with a variety of characteristics, ranging from low-to high-tech and from fully immersive environments that require the use of head-mounted displays (HMDs) to non-fully immersive 2D VR systems administered without HMDs (Li et al., 2011). While our work with VHs is focused on non-fully immersive systems, we believe that the Kirkpatrick concepts discussed are applicable to both fully- and non-fully immersive systems, as the Kirkpatrick Model is not reliant on any particular type of training in order to be used. Consequently, in this section, we discuss a number of systems that range in terms of immersiveness.
2.1 Existing Applications of the Kirkpatrick Model to Training in Virtual Environments
The Kirkpatrick Model has four levels for evaluating training (Kirkpatrick and Craig, 1970):
• Level 4 Results—the degree targeted outcomes occur
• Level 3 Behavior—the degree participants apply concepts learned in training
• Level 2 Learning—the degree participants acquire intended knowledge in training
• Level 1 Reaction—the degree participants find the training favorable
Results–the highest level and the “ultimate” outcome of the training scenario–measure the impact of the training on the organization level (productivity gains, cost savings, employee attitude/morale) (Brogden and Taylor, 1950). The Kirkpatrick Model advocates evaluating training scenarios with the results level in mind first, so that the impact of these results may inform the lower levels. Behavior, Level 3, measures the degree individuals actually use what they learned in training when they are on the job (Alliger et al., 1997). The next level, Learning (Level 2), is defined in this context as knowledge, skills and feelings acquired in the short term at the end of training (the simplest and most commonly used measurement) and in the long term to assess retention of what was learned. Reaction (Level 1) is a measurement of trainees’ feelings toward the training program in terms of utility and enjoyment, and it is the most commonly collected type of evaluation data (Bassi et al., 1996).
Given the popularity of the Kirkpatrick Model, several applications using virtual environments have applied the Model, but the use of the full-breadth of evaluation levels appears to be rare. The majority of studies on virtual environment-based training have reported positive results regarding users’ reactions (Level 1) (Schmidt and Stewart, 2009; Alaraj et al., 2011; Loukas et al., 2011; Kidd et al., 2012; Cohen et al., 2013). However, fewer studies have attempted to reach Levels 3 and 4. For example, Suàrez et al. applied the Kirkpatrick Model as a framework to compare learning with virtual human role-players and a variety of other training methods, including real human role-players (Suárez et al., 2021). The authors note that they only focused on Levels 1 and 2 of the Model explicitly because the higher levels can only be evaluated “once a long period of time has elapsed after training” (Suárez et al., 2021). While certain aspects of Levels 3 and 4, such as monitoring learners’ on-the-job behavior, do require some time to pass, the important behaviors to target in Level 3 can potentially be incorporated into educational virtual environments to begin understanding the impact of the training, as we will discuss in Section 3.2.
Similarly, Grabowski et al. developed a virtual reality-based pilot training simulation for underground coal miners (Grabowski and Jankowski, 2015). Work in the mining industry has been described as dirty, dark, wet, noisy, hot, uncomfortable and as being one of the most dangerous industries (Van Wyk and De Villiers, 2009), supporting the idea that the Kirkpatrick Model is a suitable evaluation method toward reducing the gap between theoretical training and practice. In this context, Grabowski et al. applied a training questionnaire based on the Kirkpatrick Model. Similar to many other educational applications using virtual environments, they focused on evaluating lower levels—in this case Level 1 (Reactions)—with less emphasis on Levels 2, 3, and 4.
As another example from the healthcare context, Zaveri et al. compared an online learning platform and a virtual human-based module (on Second Life) simulating pediatric sedation procedures (Zaveri et al., 2016). In contrast to the previously described research applying the Kirkpatrick Model, the authors attempted to evaluate their work regarding the first three of Kirkpatrick’s levels. The results showed positive findings for Kirkpatrick’s Level 1 (participants had a positive reaction to the experience). However, no statistically significant differences were found regarding Levels 2 and 3 when comparing the virtual-human module and the baseline web-based module. In another noteworthy example, Kundhal, et al. compared performance in a virtual environment to actual operating room performance by applying a checklist, effectively evaluating Levels 3 and 4 of the Model (Kundhal and Grantcharov, 2009). This work demonstrated that training in virtual environments can impact those two levels when simulating real environments.
Taken together, these efforts suggest a trend toward applying the Kirkpatrick Model to educational and training virtual environments, with more frequent application of the full Model in the healthcare education context. However, little is mentioned for these applications about how the Model was applied and to what extent it was used beyond questionnaires for the evaluation phase of those studies. Our work contributes to the field of virtual environments for education training by describing how we adapted the Model to virtual environment training, specifically in the context of a healthcare scenario, and how the Kirkpatrick Model can help virtual environment developers and researchers plan the overall goals and metrics for their proposed systems.
2.2 Educational Measures in Applications for Healthcare Communication Skills Training in Virtual Environments
Given the importance of doctor-patient communication, researchers in healthcare education have developed approaches to measure students’ communication competency in real environments. One such approach is the Control, Explaining, Listening and Influencing (CELI) model (Wouda et al., 2011), which aims to promote patient-centered communication. Given the complex nature of patient-centered communication, the developers of this model note that many healthcare communications skill training scenarios suffer from a mismatch between the learning objectives and skills taught in the training. To better address this mismatch, the CELI model was developed.
The existence of the CELI model for real world competency measurement would suggest that a simple method to address communication skills measurement in virtual environments is to use the CELI model in a virtual environment itself. However, follow-up research using the CELI model in the real world revealed that healthcare students require deliberate practice in order to improve communication skills past a “satisfactory” level (Wouda and van de Wiel, 2012). Deliberate practice involves a learner engaging in activities with explicit learning goals that can allow the learner to challenge any behaviors that are unconscious and sub-optimal. According to Wouda and van de Wiel, the key components of deliberate practice for healthcare communication skills training are as follows (Wouda and van de Wiel, 2013):
• “Learning tasks with well-defined goals”
• “Stimulating learning tasks of short duration with opportunities for immediate feedback, reflection, and corrections”
• “Having ample opportunities for repetition, gradual refinements, and practice in challenging situations”
• “Being motivated to improve”
Characteristics such as the “well-defined goals” and the need for tasks with “short duration” and “immediate feedback” suggest a narrower scope than a holistic view of patient-centered communication, which is the aim of the CELI model. Thus, from the existing literature on measuring healthcare communication competency, we see two important goals that should be addressed by healthcare communication skills scenarios: 1) alignment between a scenario’s stated learning objectives and the skills being taught 2) a narrower scope than broadly improving patient-centered communication.
Evidence of the latter goal is present in many healthcare communication skills training scenarios, as many of these scenarios focus on specific skills or types of communication. For example, several virtual patient scenarios focus on developing student empathy (Halan et al., 2015; Foster et al., 2016). Other applications have focused on information discovery, notably the Virtual People Factory system (discussed further in Section 3.1), the existing system to which we applied the Kirkpatrick Model in this work. Still other non-fully immersive systems, such as SIDNIE, targeted specific communication skills needed for working with a particular group of patients. In the case of SIDNIE, the system targeted learners’ unbiased and age-appropriate language when interacting with pediatric patients (Dukes et al., 2013).
These systems use a variety of methods specific to the communication skill of interest to measure learners’ performance. For example, information discovery in VPF2 is measured by students’ discovery of pre-defined pieces of important diagnostic information. Similarly, choosing the more unbiased and age-appropriate questions built into SIDNIE yields better performance. On the other hand, the empathy systems have used simulations to collect learner communication skills behavior that is then later evaluated by an expert grader using an existing framework, such as the Empathic Communication Coding System for empathy. While there are clearly a variety of methods to measure communications skills in virtual environments, common to many of these methods is the incorporation of an expert in healthcare communication to provide guidance on metric development. However, the process for working with these experts is often not explicitly discussed, especially in terms of ensuring alignment between a scenario’s learning objectives and the skills being taught. The Kirkpatrick Model is a good candidate for a framework to address these concerns and may provide a common perspective by which to discuss and compare these different metrics for healthcare communication skills training, despite originating from different skills and being applied to different virtual environments.
3 The Kirkpatrick Model and Its Application to an Educational Virtual Human Healthcare Scenario
We now discuss our application of the Kirkpatrick Model to a specific educational context: medical communication skills training. Since we were applying the Model to an existing training scenario, we begin this section with details of Virtual People Factory, a desktop-based system that features conversational VHs (see Section 3.1). Then, as the Model proposes addressing the highest level (Level 4 Results) first in order to address the learning-practice gap, we describe our process in Section 3.2 with details of Level 4 and work downward.
3.1 Virtual People Factory 2.0
VPF2 is a non-fully immersive application accessible online that enables creation of and interaction with VHs. It is an iteration of the conversational modeling system, Virtual People Factory, developed by Brent Rossen in (Rossen, 2011). VPF2 was designed to allow individuals without technical expertise but with a particular domain expertise, such as a healthcare instructor, to author a VH that can then be interviewed in the same application. The VPF2 authoring process mostly focuses on the creation of the VH script, which contains the dialogue responses a VH can provide, as well as the corresponding questions that can elicit those dialogue responses.
Script authors can also define various meta-data, such as discoveries and topics, for a VH script. A discovery is an important piece of information that should be uncovered by the learner over the course of a VH interview. Example discoveries from a medical VH include “Difficulty with tough foods” and “Coughs while eating.” In the context of a medical interview, these discoveries may be important for making a diagnosis. Another meta-data option provided by VPF2 is topics. Topics can be used to group question and response pairs. For instance, a virtual patient’s script could contain the question “How does your swallowing problem affect your social life?” under the topic of “Chief Complaint.”
In addition to the virtual human authoring capabilities provided by VPF2, the application also enables the interviewing of VHs in an online interface. This interface allows remote VH interviewers to ask a VH questions while using a personal desktop or laptop device. Typically, the interview is conducted in a chat interaction style, as shown in Figure 1: interviewers can type questions into an input box, and VPF2 will match the typed question to the available phrasings in the virtual human script. If a matching phrasing is found, the VH responds with the corresponding script response. If no matching phrasing is found, a standard exception response (“Sorry, I don’t understand what you just said. Can you say it another way?”) is returned instead. If an interviewer asks a question that is mapped to a discovery, that discovery is considered “uncovered” and is counted toward an interviewer’s discovery score. A discovery score is calculated by dividing the total number of uncovered discoveries by the total discoveries in a VH scenario.
We place our work on the reality-virtuality continuum (?) by describing VPF2 as a system to create and interact with conversational VHs. Conversational VHs combine the virtual components of a conversation partner, such as speech, gestures, animations, virtual characters, and varying capabilities to understand the user’s verbal and nonverbal inputs. The conversational virtual humans exist on a continuum of levels of immersion from displays such as on mobile phones and laptops to immersive displays such as head-mounted displays and CAVE-like systems. The conversational VHs discussed in this paper included VHs capable of conversational dialogue (either typed or spoken) restricted to the topic domain and deployed on lower-immersion laptop and desktop displays. This form factor was chosen to enable an educational experience that could be integrated into an existing curriculum and accessible via the resources available to the enrolled students.
3.2 The Kirkpatrick Model Applied
Our stakeholder for this work (listed as the third author, AM) was interested in integrating existing virtual patients (VPs) into a clinical practicum course for speech-language therapy (SLT) students. The clinical practicum course is part of the students’ clinical training, and the VP interviews were integrated into the course to provide support for the students’ final clinical exam. An overview of our process for improving the impact of VP training and the takeaways we identified from each step is as follows:
• Work with educators to determine and prioritize the most important results to target → patient adherence to healthcare recommendations and patient-centered communication
• Work with educators to determine and prioritize the most important behaviors learners need to exhibit to impact the above outcomes → SLTs should use language that promotes patient adherence and patient-centered communication
• Work with educators to clearly map VP learning objectives to the expected behaviors → VPs should recognize and reward learners’ language that promotes patient adherence and patient-centered communication
• Work with learners to understand their reaction to the training → learners should find the VP scenario useful in practicing using language that promotes patient adherence and patient-centered communication
3.2.1 Results
First, we began by discussing with our stakeholder the planned high-level results we wished to target using the existing VPs. Our discussion centered on which problems in healthcare might be impacted by a healthcare providers’ communication skills. (In our case, as we had an existing VPs focused on communication skills, this topic framed our initial results discussion, but if one is creating entirely new educational virtual environment application, this discussion will likely be more open-ended.) One pressing issue that arose in these initial discussions was that of patient adherence. Patient adherence, or the ability to follow a provider’s instructions for care, has been linked to successful patient outcomes. For example, for patients at risk of heart disease, patient non-adherence can greatly influence survival rates (Martin et al., 2005). In addition to the health risks associate with non-adherence, there is also a great economic cost. A 2004 survey estimated the “monetary waste” in the United States associated with non-adherence could be as great as $300 billion per year (DiMatteo, 2004). In 2005, the cost of medical non-adherence alone was calculated to approximately $100 billion annually.
Also of interest to our stakeholder was the applications’s cultivation of students’ holistic interviewing skills, an important aspect of patient-centered communication. Patient-centered communication is a method of communicating with patients that promotes a holistic understanding of patients rather than a sole focus on the patient’s medical problem, so an important skill healthcare students should cultivate is asking questions on biomedical topics and social topics. While holistic interviewing is often targeted as a result on its own, a lack of patient-centered communication may contribute to a lack of patient adherence. Take as an example the medical case of focus for our VH simulation, dysphagia. Dysphagia is characterized by difficulty swallowing, so an important factor to discuss is the patient’s diet: what types of food they eat, the hardness/softness of these foods, and so on. The importance of food in dysphagia management makes gathering a holistic perspective of the patient especially critical, as dysphagia patients’ cultures and food can have a large impact on their medical condition (Dikeman and Riquelme, 2002). However, such details about patients’ culture and food practices may not arise without the provider attempting to uncover a holistic view of the patient.
3.2.2 Behaviors
After identifying improved patient adherence and holistic interviewing as important outcomes to target, our next step was to identify healthcare providers’ communication behaviors that could affect these outcomes. The Kirkpatrick Model states that Level 3 Behaviors can only truly be evaluated when learners apply their training in the corresponding real-world scenario (Kirkpatrick and Kirkpatrick, 2016). Additionally, these behaviors should also be evaluated over the course of weeks or months after the training to ensure that the training is effective.
However, VHs have been to shown to elicit real-world behaviors from humans in a variety of situations, such as the presence of public speaking anxiety (Slater et al., 2006), context-switching in child peer-to-peer communication (Cassell et al., 2009), and display of empathy with virtual patients (Kleinsmith et al., 2015). These behaviors are often demonstrated despite “low representational and behavioral fidelity” (Slater et al., 2006) or even acknowledgement from participants that the VH was “less authentic” than an interaction with a real patient (Raij et al., 2006; Kleinsmith et al., 2015). Thus, we see that even less immersive systems can elicit real world behaviors with VHs. Similarly, VR has also been used to study psychological phenomenon previously only studied in physical settings (Fox et al., 2009).
Based on VHs’ abilities to elicit realistic behaviors from users, we suggest that the Kirkpatrick Model’s Level 3 Behaviors may also be observable in our application as well. In other words, we may begin to observe learners’ behavior during training (the VH simulation) itself and may use the training as a method to measure learner behavior over time. Being able to observe such measures early while learners are still interacting with VHs may give developers insight as to whether the proper behaviors are being learned from the training.
For our particular application, we should therefore identify behaviors related to patient adherence that are meaningful and measurable in both the real world and the virtual world that are observable over time. Research in doctor-patient communication indicates that cognitive factors, mostly the patient’s ability to understand medical information, are central to issues of patient adherence (Martin et al., 2005). Two popular recommendations for communication behaviors that promote patient understanding are reducing the use of medical jargon (Martin et al., 2005; Graham and Brookey, 2008; Oates and Paasche-Orlow, 2009; Green et al., 2014; Speer, 2015) and using simple language (Oates and Paasche-Orlow, 2009; Green et al., 2014; Speer, 2015). While these recommendations may seem simple, failure to follow them can have severe consequences. Patients have expressed concerns about providers who fail to use these recommended behaviors (Waisman et al., 2003; Shaw et al., 2009). In some cases, failure to practice these strategies has also led to malpractice lawsuits (Gordon, 1996).
A VH training scenario for medical communication skills can therefore use measures related to reduced medical jargon and simple language to promote patient adherence, but exactly how these how these measures should be calculated is not necessarily obvious. For example, easily calculable measures exist to calculate language complexity, such as the Flesch Reading Ease (Flesch, 1948), but how should these measures be applied to a learner’s communication behavior in a VH scenario? Should the goal be simply to promote that language complexity should be as low as possible? Additionally, we should also consider how these measures might relate to measures for patient-centered communication, such as asking the patient questions about relevant social or cultural topics.
At this stage, we recommend working with stakeholders to identify a framework to unify the behaviors of interest for the virtual environment scenario, as the framework can assist in further refining how the measures ought to be defined. In our work, our focus on specific communication behaviors of healthcare providers–using simple language, reducing jargon use, asking questions across a variety of biomedical and social topics–led us to identify a unifying concept in the communication literature. This concept, which encompasses all of these communication behaviors, is known as message production, or the process by which a communicator transforms a feeling or thought into a message to share it with other people. While the framework provided by message production did not come directly from the Kirkpatrick Model, we were able to identify it through our focus on patient adherence and holistic interviewing. This identification of message production then allowed us to determine a number of behavioral measures relevant to patient adherence and holistic interviewing. These measures are discussed in further detail in Section 4.2.
3.2.3 Learning Objectives
The Kirkpatrick Model includes several components as part of this level: knowledge, skills, attitude, confidence, and commitment (Kirkpatrick and Kirkpatrick, 2016). While all of these components are important to consider when developing training simulations, a focus on skills is likely of most interest for instructional designers, given its potential to overlap with the targeted behaviors from Level 3. Additionally, Kirkpatrick and Kirkpatrick also advise that many of these components can be evaluated simultaneously, so we choose to focus on skill evaluation with the plan to add evaluation of other the components in the future.
Given that we were iterating upon an existing VH training scenario, our first question related to the learning objectives was the degree to which learners were displaying these skills with our VPs currently. We therefore analyzed transcripts from existing interviews using the six metrics we identified from Level 3 Behavior. We analyzed transcripts from real healthcare students to determine if there was any change in these behaviors over time, with the hope that since these learners were enrolled in a clinical practicum course at the time of the interviews, a change in these behaviors would be displayed with the VPs. This analysis is discussed in detail in Section 4.
3.2.4 Reactions
The lowest level of the Kirkpatrick Model is the Reactions level. As with many existing training scenarios, we evaluated the Reactions level to some degree before applying the Kirkpatrick Model. This evaluation was done primarily through post-interview survey questions. These questions included items on the medical accuracy of the patient and aspects of the patient the learners found interesting or challenging. We chose to continue this method even after applying the Kirkpatrick Model to keep this level simple, as we felt this best aligned with the Kirkpatrick philosophy to place the higher levels at a greater importance. However, as we continue to develop the VP training scenarios for target patient adherence, questions that explicitly address the communication skills aspect of the training would be helpful. For example, future questions could include asking the learners about the impact of the training on their medical interviewing skills.
4 Materials and Methods
After identifying the six message production metrics relevant to patient adherence and holistic interviewing, we then used these metrics to examine retroactively medical communication skills learners’ message production behaviors with VPs. We gathered VP interviews from 66 real healthcare students from four previous years to identify any trends in students’ message production with VPs over time. The interviews were collected from several cohorts of learners (from the years 2015, 2016, 2018, and 2019) who had had VP interviews integrated into their academic coursework. For each course integration, at the beginning of the semester, students were given an introduction to virtual patient interviewing in VPF2. This introduction covered best practices when using VPF2, including tips such as avoiding the use of pronouns to better match the system’s natural language processing or how to track one’s progress in an interview. Also at the beginning of the semester, students were asked to complete a background survey with information on their previous experiences interacting with patients and with relevant technology, such as online messaging and videos game use.
After the system introduction and background survey, students began interviewing VPs. The number of VPs interviewed for each course integration, depending on the wishes of the instructors and the goals of the larger studies being conducted, but all students interviewed at least 3 VPs. Of these virtual patients, this work considers the first two interviews, as they are have the most similarities across the different course integrations. Firstly, for all of the cohorts, the first two interviews occurred approximately 1 month apart, and the first and second interviews had no other VP-related tasks between them. A diagram of the course integration tasks represented in this work is provided in Figure 2.
The virtual patients interviewed by the students differed, in efforts to coordinate with the instructors what patients they would find most useful to their classes. To investigate whether there were any effects on message production due to the students interacting with different virtual patients, students were grouped into two groups based on which patients they interviewed: Group A included the students who interviewed the virtual patients Lilly Smith and Vinny Devito. Group B included the students who interviewed Lilly Smith and Monica Roberts. Group A included students from the University of Auckland in 2015 and 2016, while Group B included students from the University of Auckland in 2018 and Kent State University in 2019.
4.1 Population
Information from the background survey for students in each interview group and across all students is provided in Table 1. For this work, 66 students completed the first two virtual patient interviews, but only 65 students completed the background survey. The survey data for the 65 respondents is reported here.
Across both groups, students’ average age was 26.9 ± 6.16 years. The majority of the students in both groups were female, (93.8%). This gender distribution is consistent with real-world speech language pathologists (ASHA, 2020). Students reported interacting with an average of approximately 32 patients with a standard deviation of 17 patients, and a slight majority reported no previous communication skills training (56.9%).
4.2 Metrics for Message Production in Virtual Human Scenarios
Using the framework provided by message production, we identified six measures relevant to patient adherence and holistic interviewing. These six metrics correspond to message production behaviors healthcare students should exhibit when interacting with patients. According to communication research, message production has three different categories of assessment: 1) goal attainment, 2) efficiency, and 3) social appropriateness. In the following subsections, we discuss the metrics identified for each category.
4.2.1 Goal Attainment
Key to message production is the role of language as a tool to achieve a goal. Humans do not engage in language use or social interaction as ends themselves but do so to accomplish a goal, such as building rapport (Berger, 2003). Thus, because message production is a goal-driven activity, the degree to which a speaker achieves his or her goal is an important measure. As discussed in Section 3.2, an important outcome for our stakeholder and a potential contributor to patient non-adherence was ensuring learners pursue a holistic view of their patients. So, one way we should measure learners’ goal attainment is by assessing whether their message production behavior promotes a holistic view of the patient. The measure we identified for this category of message production assessment is the number of unique ICF codes.
A message production behavior that fits this criterion is asking questions on both medical and social topics, and further consultation with our stakeholder introduced us to a systematic set of labels for classifying medical information. This set was the World Health Organization’s International Classification of Functioning, Disability, and Health (ICF). The WHO ICF is a framework used to “describe and measure health and disability” (Üstün et al., 2003). As part of this framework, the ICF includes a coding scheme to support a common vocabulary of health topics across disciplines and languages.
Our stakeholder identified a subset of codes from the ICF that related to dysphagia. This subset included a total of ninety-four ICF codes across different categories within the ICF, such as Body Functions and Structures, Environmental Factors, and Personal Factors. Using the subset, we may tag every question asked by learners to determine their coverage of different health topics. Examples of the ICF codes used can be found in Table 2. For each learner, the total number of unique ICF codes used in each interview was normalized by ninety-four, the total number of ICF codes being reviewed. A larger number of ICF codes used in a single interview likely indicates a more holistic view of the VP was pursued, as a wider range of topics would have been covered.

TABLE 2. Examples of ICF codes, Flesch Reading Ease scores, and medical words identified for measures in the Message Production Trends study.
4.2.2 Efficiency
The second category of message production assessment is efficiency; speakers can potentially enact multiple strategies to achieve their communication goals, but these strategies may vary in the amount of time and effort needed to enact them (Berger, 2003). To measure efficiency, we identified two metrics: questions per discovery and median question latency.
The questions per discovery metric originates from previous virtual patient literature (Halan et al., 2018) and is the ratio of the number of questions asked by the learner in the interview to the number of discoveries uncovered by the student. This metric reveals how efficiently a learner can uncover the important information in a virtual patient interview. Higher values for questions per discovery indicate less efficient interviewing, as the student had to ask a greater number of questions to uncover discoveries.
Our second efficiency metric, median question latency, is an adaptation of speech latency, a measure that has been used in existing communication literature (Greene and Geddes, 1993). Median question latency is measured in VP interviews by measuring the time interval in seconds between each of a learner’s questions to the VP and then taking the median of these intervals. While median question latency is inspired by speech latency in the communication literature, it must be noted that median question latency cannot be compared directly to speech latency, as median question latency contains the additional confound of typing time. Since learners must type their questions to the virtual patient in VPF2, examining the time between each question will also include the time needed to type each question.
4.2.3 Social Appropriateness
The final category of message production assessment is social appropriateness. In general contexts, examples of social appropriateness may include producing messages with the appropriate level of politeness, but as discussed previously in Section 3.2, the ability of a healthcare provider to adapt his or her language to promote patient adherence is also important. The two suggestions often given to providers to communicate in a manner that promotes patient adherence are 1) to speak in simple language (Graham and Brookey, 2008; Green et al., 2014; Speer, 2015) and 2) to use less medical jargon (Graham and Brookey, 2008; Oates and Paasche-Orlow, 2009; Green et al., 2014).
To target simple language, we propose two measures: 1) the percentage of learner utterances below the standard reading ease and 2) the percentage of student utterances similar to the virtual patient’s. Both of these measures use the Flesch Reading Ease formula (FRE). The FRE has been used in past research to evaluate patient-targeted documents (Williamson and Martin, 2010; Agarwal et al., 2013) and oral health advice (Bradshaw et al., 1975). The FRE uses a text’s words per sentence and syllables per word to calculate an overall score (Flesch, 1948). As the score increases, text difficulty decreases. Scores ranging from 60 to 70 are considered “standard” and correspond to an American eighth or ninth grade reading level (Flesch, 1949). The percentage of learner utterances below the standard reading ease (Percent Below Standard) addresses the general difficulty of a learner’s utterances by calculating the percentage of utterances that scored below 60, the lower end of the standard range of the FRE.
The percentage of learner utterances similar to the virtual patient’s (Percent Similar) was used to measure learner’ language difficulty in relation to the virtual patient’s. While general recommendations are to use simple language in medical communication, oversimplifying may also be problematic; for example, younger health care providers have been shown to engage in elderspeak with elderly patients (Kemper, 1994). Elderspeak involves changes in lexical complexity, speaking rate, and number of other factors of one’s communication and has been associated with inverse health outcomes of the elderly patients it is used with (Williams et al., 2009). Thus, while simple language is important, health care providers should also adapt accordingly to the patient they are currently interacting with. To measure learner adaptability, the reading ease of learners’ utterances were compared to the mean of the virtual patient’s reading ease. If a learner’s utterance was within one standard deviation of the virtual patient’s mean reading ease, this utterance was considered “similar” to the virtual patient’s. For each learner, the number of similar utterances was normalized by the count of all the learner’s utterances to calculate the final metric.
For our final metric, we used the percentage of medical words used by learners to target learners’ use of medical jargon. First, a medical word list was created to label the students’ transcripts. The medical word list included an 819-word long list created by Lei and Liu in efforts to create an updated academic medical word list (Lei and Liu, 2016). This list was augmented by words pulled from hospital glossaries focused on speech language pathology to ensure coverage of dysphagia-related terms (Cincinnati Childrens, 2021). To determine whether a student’s word was a medical word, student utterances were tagged with part-of-speech information using the Python NLTK library (Bird et al., 2009). Since the medical word list only contained nouns, adjectives, and adverbs, the student utterances were filtered down to words of these three parts-of-speech. The remaining words were lemmatized using the lemmatizer provided in the Python NLTK library and then compared against words of the same part-of-speech in the medical word list. For each student, the number of words that matched the medical word list was divided by the total number of words used by the student to produce the final measure.
5 Results
Transcripts from the VP interviews were downloaded from the VPF2 application for processing. While learners may have interacted with each VP multiple times, data was only pulled from a learner’s longest transcript to compute the six metrics to prevent artificial inflation of the metrics. For example, when calculating a learner’s unique ICF codes, using all of a learner’s transcripts may decrease this metric artificially, as the total number of utterances by a learner has no upper limit.
To identify any changes in message production behavior, we ran a mixed-design ANOVA on the six interview metrics. The within-subjects factor was VP interview (Interview 1 and Interview 2) and the between-subjects factor was interview group (Group A or Group B). VP interview was the main effect of interest in this analysis, as any significant effects of VP interview would indicate that there was a change in learners’ message production from Interview 1 to Interview 2. Such a finding would suggest that the VP interviews were able to elicit changes in learners’ message production. The between-subjects factor of interview group was included to determine if there were any group differences. While group differences were not the main focus of this analysis, we included the between-subjects factor because students came from different institutions and interviewed different virtual patients during their second interview.
Outliers were reviewed for each metric visually using boxplots. Any outliers and their treatment are noted below. Normality, homogeneity of variances, and homogeneity of covariances were assessed by Shapiro-Wilk test, Levene’s test of homogeneity of variances, and Box’s M test. Any instances in which these assumptions were not met are noted below. A summary of the ANOVA results is provided in Table 3.
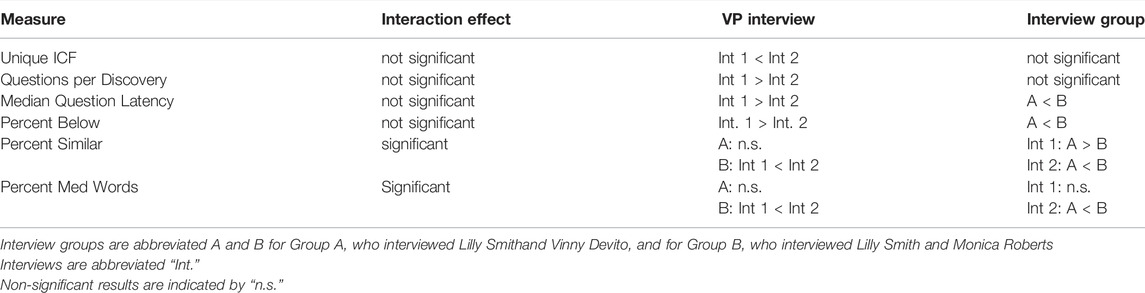
TABLE 3. A summary of the ANOVA results (interaction and main effects) for the Message Production Trends study.
5.1 Goal Attainment
The assumption of normality was not met for the unique ICF codes metric for the second virtual patient interview, p < 0.05, but the mixed-design ANOVA was still performed, as ANOVAs have been shown to be robust to violations to normality (Blanca et al., 2017). There was no significant interaction effect of virtual patient interview and interview group for the unique ICF codes used, F(1, 64) = 0.506, p = 0.479, partial η2 = 0.008. There was not a significant main effect of interview group, F(1, 64) = 1.78, p = 0.18, partial η2 = 0.027, but there was a significant main effect of virtual patient interview, F(1, 64) = 5.32, p = 0.024, partial η2 = 0.077. For students in both interview groups, the percent of unique ICF codes increased from Interview 1 (19.1 ± 8.84%) to Interview 2 (21.2 ± 6.45%). The means and standard deviations of the unique ICF codes used in Interview 1 and 2 by both interview groups are available in Figure 3.
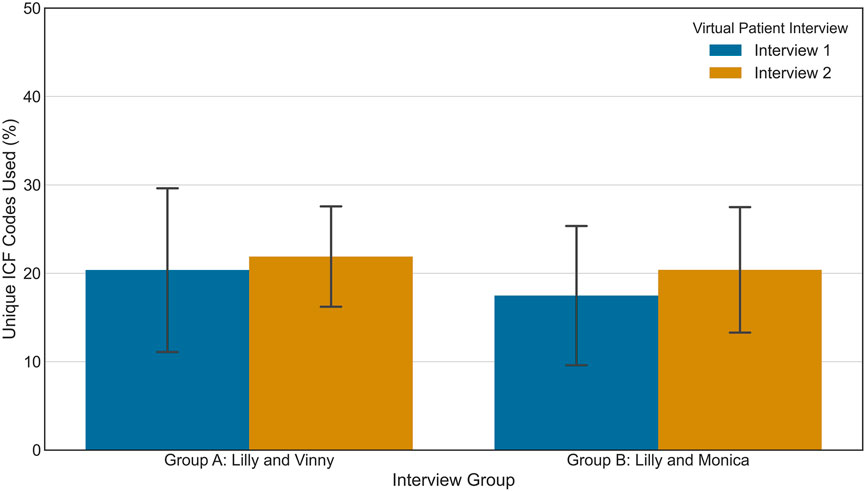
FIGURE 3. The means and standard deviations of unique ICF codes used for students in the Message Production Trends study.
5.2 Efficiency
For the questions per discovery metric, there was one extreme outlier, as identified by inspection of the SPSS version 26 boxplot. However, exclusion of this outlier did not change the results of the mixed-design ANOVA, so results including this point are presented here. Normality was violated, p < 0.05, but the mixed-design ANOVA was still performed. There was no significant interaction effect of virtual patient interview and interview group on questions per discovery, F(1, 64) = 2.91, p = 0.093, partial η2 = 0.043. There was neither a significant main effect of the interview groups, F(1, 64) = 1.25, p = 0.267, partial η2 = 0.0190, but there was a significant main effect of virtual patient interview, F(1, 64) = 35.7, p < 0.005, partial η2 = 0.358. Question per discovery decreased significantly from Interview 1 (5.60 ± 2.71) to Interview 2 (3.65 ± 1.58). The means and standard deviations of questions per discovery for each interview group for Interview 1 and Interview 2 are shown in Figure 4.
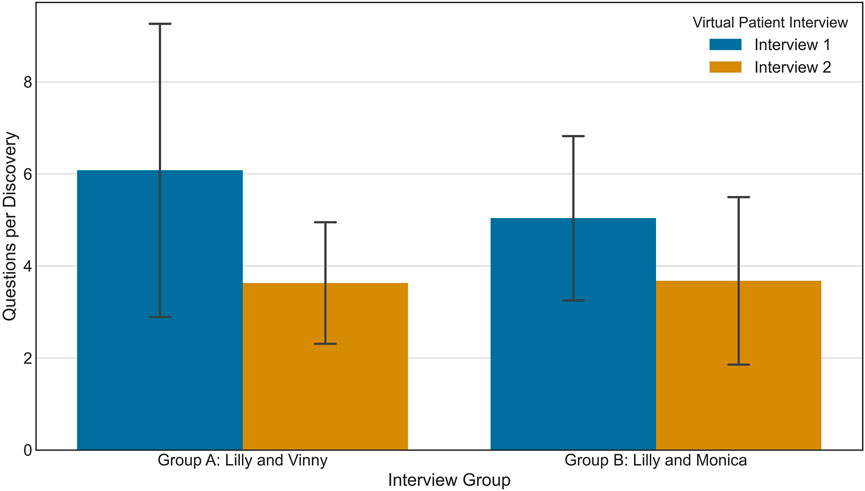
FIGURE 4. The means and standard deviations of questions per discovery for students in the Message Production Trends study.
For the median question latency, shown in Figure 5, there was one extreme outlier as identified by inspection of the SPSS version 26 boxplot. Unlike the previous metric, however, inclusion of this outlier did affect the significance results of the interaction effect of mixed-design ANOVA. Analysis reported here therefore excludes the participant with the outlying value, user BK19_08, a member of Group B.
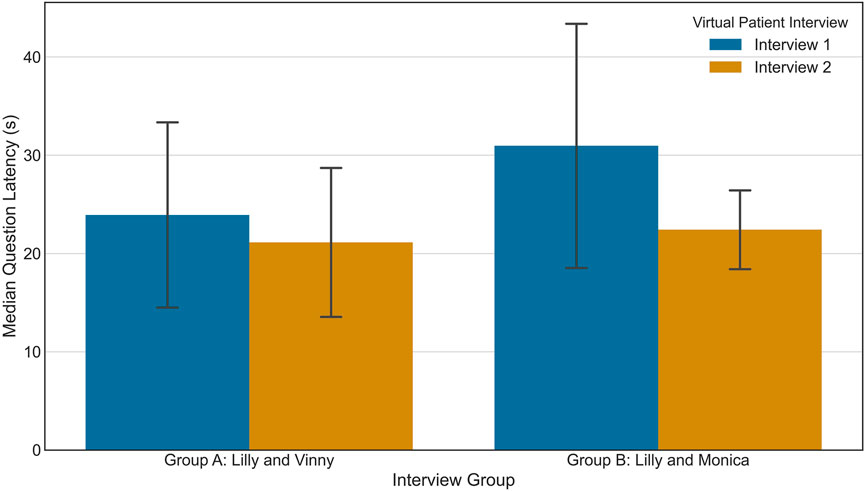
FIGURE 5. The means and standard deviations of median question latency for students in the Message Production Trends study.
Normality was violated, p < 0.05, but the mixed-design ANOVA was still run. There was no significant interaction effect of interview group and virtual patient interview, F(1, 63) = 3.621, p = 0.0616, partial η2 = 0.0543. There was, however, a significant effect of virtual patient interview, F(1, 63) = 51.5, p < 0.005, partial η2 = 0.450. Median question latency significantly decreased from Interview 1 (27.8 ± 11.2 s) to Interview 2 (20.5 ± 6.47 s). Similarly, there was also a significant main effect of interview group, F(1, 63) = 10.5, p = 0.002, partial η2 = 0.143. Group B had a significantly higher median question latency (27.5 ± 10.0 s) than Group A (21.4 ± 8.81 s).
5.3 Social Appropriateness
For percent of learner utterances below the standard reading ease (Percent Below), the assumption of normality was not met for students in Group B during Interview 2, p < 0.05. The mixed design ANOVA was still performed. There was no significant interaction effect of virtual patient interview and interview group on Percent Below, F(1, 64) = 0.203, p = 0.654, partial η2 = 0.003. There was a significant main effect of virtual patient interview, F(1, 64) = 5.30, p < 0.025, partial η2 = 0.076. For students in both groups, the average of Percent Below for Interview 1, 22.8 ± 8.78%, was significantly higher than the average for Interview 2, 20.3 ± 7.67%. There was also a significant main effect of interview group, F(1, 64) = 15.4, p < 0.005, partial η2 = 0.194. Averaged across both interviews, Group B’s Percent Below measure was significantly greater than Group A’s. This trend may be observed in Figure 6.
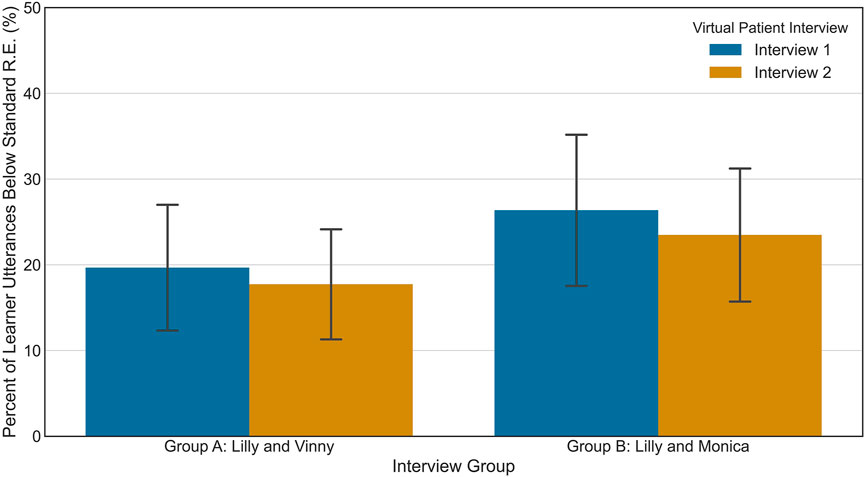
FIGURE 6. The means and standard deviations of percent of learner utterances below standard reading ease for students in the Message Production Trends study.
For percent of learner utterances similar to the virtual patient’s (Percent Similar), the assumption of normality was not met for students in Group a during Interview 1, p < 0.05. The mixed design ANOVA was still performed. There was a significant interaction effect of virtual patient interview and interview group on Percent Similar, F(1, 64) = 27.7, p < 0.005, partial η2 = 0.302.
Follow-up analysis for the main effect of interview group revealed that there was a significant difference in Percent Similar between the groups during Interview 1, F(1, 64) = 8.952, p = 0.004, partial η2 = 0.123. For Interview 1, Group A’s Percent Similar measure was significantly greater (58.2 ± 12.4%) than Group B’s (49.9 ± 9.59%). A significant difference was also at present at Interview 2, F(1, 64) = 5.36, p = 0.024, partial η2 = 0.077, but in the opposite direction. Group B’s Percent Similar metric (62.4 ± 10.7%) was significantly greater than Group A’s (55.8 ± 12.3%).
Follow-up analysis for the main effect of virtual patient interview shows that only Group B displayed a significant change in Percent Similar over the two interview, F(1, 29) = 30.2, p < 0.005, partial η2 = 0.510. Group B’s Percent Similar measures increased from an average of 49.9 ± 9.59% in Interview 1 to 62.4 ± 10.7% in Interview 2. There was no significant change for Group A over Interview 1 and Interview 2, F(1, 35) = 1.82, p = 0.186, partial η2 = 0.049. These trends may be observed in Figure 7.
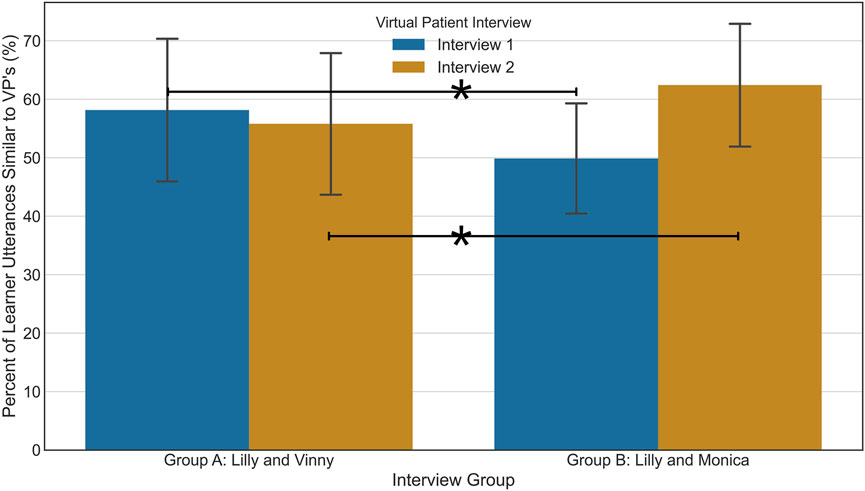
FIGURE 7. The means and standard deviations of percent of learner utterances similar to the virtual patient’s for students in the Message Production Trends study.
Finally for the percent of medical words used (Percent Medical), shown in Figure 8, the assumptions of normality and homogeneity of covariances were not met, p < 0.05, but the mixed design ANOVA was still performed. There was a significant interaction effect of virtual patient interview and interview group on the percent of medical words used, F(1, 64) = 4.13, p = 0.046, partial η2 = 0.061.
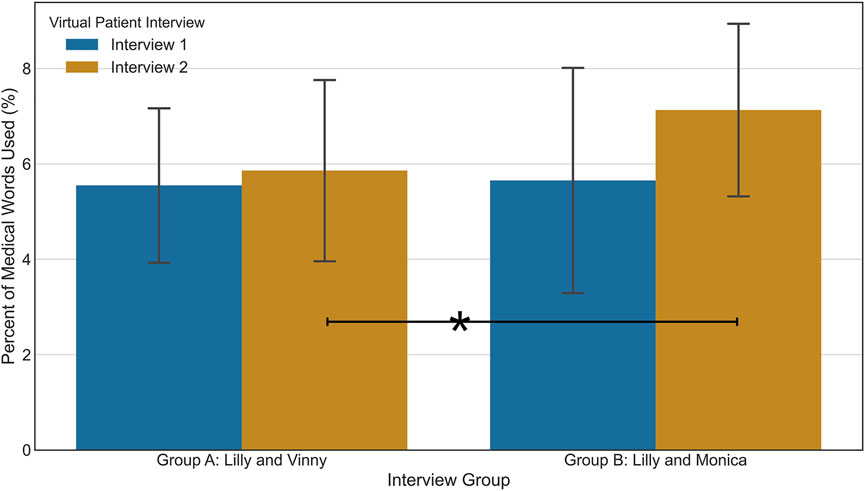
FIGURE 8. The means and standard deviations of percent of medical words used for students in the Message Production Trends study.
Follow-up analysis for the main effect of interview group reveals there was a significant difference between the two groups at Interview 2, F(1, 64) = 7.40, p = 0.008, partial η2 = 0.104. Group B’s average for percent of medical words used was significantly higher (7.13 ± 1.84%) than Group A (5.86 ± 1.93%). This difference was not present in Interview 1, F(1, 64) = 0.045, p = 0.833, partial η2 = 0.001.
Follow-up analysis for the main effect of virtual patient interview revealed only a significant change for Group B, F(1, 29) = 7.79, p = 0.009, partial η2 = 0.212. The percent of medical words used by Group A did not change significantly over the course of the interviews, F(1, 35) = 1.23, p = 0.276, partial η2 = 0.034.
6 Discussion
Our discussion of our results is broken into two subsections Section 6.1, discusses potential trends in learners’ message production and how they relate to known patterns in message production, while Section 6.2 discusses what the results of this work suggest for the application of the Kirkpatrick Model to other learning scenarios based in virtual environments.
6.1 Discussion of Learners’ Message Production With Virtual Patients
Students’ message production in the VP interviews demonstrated changes in some measures, as there were several significant main effects of virtual patient interview. The main effects of virtual patient interview indicated that students’ goal attainment and efficiency metrics changed significantly from Interview 1 to Interview 2. A main effect of virtual patient interview was also found for the Percent Below metric, one of the social appropriateness measures. These changes in students’ message production suggest that students ask questions on more topics, ask these questions more efficiently, and use less complicated language in Interview 2 than Interview 1. Based on these findings, virtual human interviews elicited changes in a variety of message production behaviors and may be useful in measuring students’ message production behavior throughout a semester.
Interestingly, for both the median question latency and the Percent Below metric, in addition to significant effect of virtual patient interview, there was also a significant difference between interview groups. As stated previously, a between-subjects factor was included in this analysis because students were required to interview different virtual patients in Interview 2 and because students came from different academic institutions. Group B (Lilly and Monica) included some students from Kent State University in the United States while Group A (Lilly and Vinny) only contained students from the University of Auckland in New Zealand. Cultural or environmental differences may have prompted some of the Group B students to produce messages in a manner different than those in Group A. However, further analysis with more students from different institutions would be needed to investigate this properly, as the majority of the students in this analysis came from the same institution, the University of Auckland.
The results for the social appropriateness metrics revealed additional differences in message production. For the remaining two metrics–Percent Similar and Percent Med Words–there were significant interaction effects. For both metrics, Group B experienced a significant increase from Interview 1 to Interview 2. At Interview 2, Group A’s values are also significantly less than Group B’s. In contrast to the Percent Below measure, in which we saw an overall difference in Group B (Lilly and Monica) compared to Group A (Lilly and Vinny), the influencing factor here seems to be isolated to Interview 2, suggesting that the changes in these metrics may be due to speaking to a different VP.
One potential reason that students in Group B spoke in a more similar language complexity to the VP during the second interview may be due to the ages and genders of the virtual patients interviewed. Previous research in linguistics shows that speakers “align” their speaking more closely to their speaking partners’ if the partner is considered an “in-group” member (Unger, 2010). In other words, in conversation, one speaker may mimic another speaker more if the second speaker is perceived to be similar. This perception of in-group versus out-group may have been present when students interviewed the virtual patients. Lilly Smith (Interview 1) is depicted as a 65 year-old female, while Monica Roberts (Group B, Interview 2) is depicted as a 38 year-old female. Since Monica Roberts is closer in age to the participants, the participants may have tried to match Monica more than Lilly in terms of language complexity. Such a perception may have affected the percentage of medical words used as well.
6.2 Overall Discussion
Using the metrics identified from the Kirkpatrick Model related to holistic interviewing and patient adherence, we demonstrated that the VPs elicited changes in students’ message production behavior over time. From this finding, we identify two contributions. Firstly, our work adds to the existing ability of VHs to elicit real-world behaviors from participants, as demonstrated in the works discussed previously (Slater et al., 2006; Cassell et al., 2009). Secondly, based on our application of the Kirkpatrick Model to identify how these behavioral measures were made, we find support for our suggestion to introduce educational VH simulations at the Behavioral level in the Kirkpatrick Model.
The ability to include educational VH simulations at later stages in the Kirkpatrick Model could have a great impact for developers of these applications. Because the metrics derived using the Kirkpatrick Model originate from important objectives in the educational context itself, this process provides some assurance that the measures are meaningful to what is being learned. Further, by incorporating behavioral measures into the VH scenario, there is the potential to lessen the gap between the Learning and Behavior levels in the Model, as learners will be able to engage in the critical behaviors while still interacting with the VH itself. The VH-based training may also be used as a Behavior level monitoring solution, which is critical to ensure trainees continue to apply training in real world settings. While future work will be needed to evaluate the general ability of virtual environments to blend aspects of the Learning and Behavior levels, our work provides initial support for this line of inquiry.
7 Conclusion
In this work, we recommend the use of the Kirkpatrick Model as a framework to evaluate educational and training applications using virtual environments. Specifically, we investigated the use of non-fully immersive, conversational VHs to evaluate learner behavior change during training by using the Kirkpatrick Model to identify behavioral measures that can be evaluated both in virtual environments and in the real-world. By incorporating behavioral measures into our VP-based desktop application, we hope to lessen the potential gap between the virtual simulations and the behaviors learners should perform in the real world. Our work provides a new perspective on measuring behavior as compared to the standard Kirkpatrick Model, which advises that learners’ behaviors may only be observed while in real-world scenarios.
In our application of the Kirkpatrick Model, we derived six metrics related to healthcare students’ real-world behaviors (Level 3) that promote holistic interviewing and patient adherence (Level 4 Results). These six metrics were then used to evaluate healthcare students’ message production with VPs over the course of an academic semester. We found significant changes in three of the six metrics. While follow-up research would be needed to confirm that these changes reflect students’ message production trends with real patients, we view this finding as encouraging: the behavior metrics motivated by the Kirkpatrick Model have some sensitivity to students’ language behavior and can be also be reused to evaluate students’ language behavior with real patients later on. Additional work will be needed to validate this approach, but we find support for our new perspective of the Kirkpatrick Model to observe behavior level measures with non-fully immersive VH technology. Additional work is needed to further validate our approach in fully-immersive simulations. Future work can also investigate the effects of measuring behavior level measures in simulation by comparing learner behaviors across virtual environments and reality, as well by tracking larger metrics such as those found in the Model’s results level.
Data Availability Statement
The raw data supporting the conclusion of this article will be made available by the authors, without undue reservation.
Ethics Statement
The studies involving human participants were reviewed and approved by the Institutional Review Board, University of Florida and The University of Auckland Human Participants Ethics Committee (UAHPEC 016700). The patients/participants provided their written informed consent to participate in this study.
Author Contributions
SC conducted the user studies and carried out the data analysis. BL and AM contributed to the design of the user studies. AM was the instructor of record for the University of Auckland students and the stakeholder in the Kirkpatrick Model process. All authors contributed to the authoring and conceptualization of the manuscript.
Conflict of Interest
The authors declare that the research was conducted in the absence of any commercial or financial relationships that could be construed as a potential conflict of interest.
Publisher’s Note
All claims expressed in this article are solely those of the authors and do not necessarily represent those of their affiliated organizations, or those of the publisher, the editors and the reviewers. Any product that may be evaluated in this article, or claim that may be made by its manufacturer, is not guaranteed or endorsed by the publisher.
Acknowledgments
The authors thank Heng Yao for his role in running the user study and data collection in 2018, as well as the Virtual Experiences Research Group for their feedback and expertise in both VPF2 development and manuscript feedback. Finally, the authors thank Dr. Ali Barikroo for his guidance in integrating the virtual patient interviews into his course.
References
Agarwal, N., Hansberry, D. R., Sabourin, V., Tomei, K. L., and Prestigiacomo, C. J. (2013). A Comparative Analysis of the Quality of Patient Education Materials from Medical Specialties. JAMA Intern. Med. 173, 1257–1259. doi:10.1001/jamainternmed.2013.6060
Alaraj, A., Lemole, M. G., Finkle, J. H., Yudkowsky, R., Wallace, A., Luciano, C., et al. (2011). Virtual Reality Training in Neurosurgery: Review of Current Status and Future Applications. Surg. Neurol. Int. 2, 52. doi:10.4103/2152-7806.80117
Alliger, G. M., Tannenbaum, S. I., Bennett, W., Traver, H., and Shotland, A. (1997). A Meta-Analysis of the Relations Among Training Criteria. Personnel Psychol. 50, 341–358. doi:10.1111/j.1744-6570.1997.tb00911.x
Asha, A. S.-L.-H. A. (2020). Profile of ASHA Members and Affiliates, Year-End 2019. Tech. rep. Available at: https://www.asha.org/siteassets/surveys/2001-2021-member-and-affiliate-profile-trends.pdf.
Bassi, L., Benson, G., and Cheney, S. (1996). Trends: Position Yourself for the Future. Alexandria, VA: American Society for Training and Development.
Beal, M. D., Kinnear, J., Anderson, C. R., Martin, T. D., Wamboldt, R., and Hooper, L. (2017). The Effectiveness of Medical Simulation in Teaching Medical Students Critical Care Medicine. Sim Healthc. 12, 104–116. doi:10.1097/SIH.0000000000000189
Berger, C. R. (2003). “Message Production Skill in Social Interaction,” in Handbook of Communication and Social Interaction Skills (Mahwah, NJ, US: Lawrence Erlbaum Associates Publishers).
Bird, S., Klein, E., and Loper, E. (2009). Natural Language Processing with Python(Beijing:Cambridge [Mass.]: O’Reilly)OCLC. 1st ed edn. ocn301885973.
Blanca, M. J., Alarcón, R., Arnau, J., Bono, R., and Bendayan, R. (2017). Non-normal Data: Is ANOVA Still a Valid Option? Psicothema 29, 552–557. doi:10.7334/psicothema2016.383
Bradshaw, P. W., Ley, P., Kincey, J. A., and Bradshaw, J. (1975). Recall of Medical Advice: Comprehensibility and Specificity. Br. J. Soc. Clin. Psychol. 14, 55–62. doi:10.1111/j.2044-8260.1975.tb00149.x
Brogden, H. E., and Taylor, E. K. (1950). The Theory and Classification of Criterion Bias. Educ. Psychol. Meas. 10, 159–183. doi:10.1177/001316445001000201
Cassell, J., Geraghty, K., Gonzalez, B., and Borland, J. (2009). Modeling Culturally Authentic Style Shifting with Virtual Peers.”in Proceedings of the 2009 international conference on Multimodal interface (Cambridge, MA: ICMI-MLMI ’09) 135. doi:10.1145/1647314
Cohen, D., Sevdalis, N., Taylor, D., Kerr, K., Heys, M., Willett, K., et al. (2013). Emergency Preparedness in the 21st century: Training and Preparation Modules in Virtual Environments. Resuscitation 84, 78–84. doi:10.1016/j.resuscitation.2012.05.014
Delisle, M., Ward, M. A. R., Pradarelli, J. C., Panda, N., Howard, J. D., and Hannenberg, A. A. (2019). Comparing the Learning Effectiveness of Healthcare Simulation in the Observer versus Active Role: Systematic Review and Meta-Analysis. Sim Healthc. 14, 318–332. doi:10.1097/SIH.0000000000000377
Dikeman, K. J., and Riquelme, L. F. (2002). Food for Thought. Perspect. Swal Swal Dis. (Dysph) 11, 31–35. doi:10.1044/sasd11.3.31
DiMatteo, M. R. (2004). Evidence-based Strategies to foster Adherence and Improve Patient Outcomes. JAAPA 17, 18
Dukes, L. C., Pence, T. B., Hodges, L. F., Meehan, N., and Johnson, A. (2013). “Sidnie,” in Proceedings of the 2013 International Conference on Intelligent User Interfaces (New York, NY, USA: Association for Computing Machinery)), 395–406. doi:10.1145/2449396.2449447
Foster, A., Chaudhary, N., Kim, T., Waller, J. L., Wong, J., Borish, M., et al. (2016). Using Virtual Patients to Teach Empathy. Sim Healthc. 11, 181–189. doi:10.1097/sih.0000000000000142
Fox, J., Arena, D., and Bailenson, J. N. (2009). Virtual Reality. J. Media Psychol. 21, 95–113. doi:10.1027/1864-1105.21.3.95
Grabowski, A., and Jankowski, J. (2015)., 72. Publisher: Elsevier, 310–314. doi:10.1016/j.ssci.2014.09.017Virtual Reality-Based Pilot Training for Underground Coal MinersSaf. Sci.
Graham, S., and Brookey, J. (2008). Do Patients Understand? Perm J. 12, 67–69. doi:10.7812/tpp/07-144
Green, J. A., Gonzaga, A. M., Cohen, E. D., and Spagnoletti, C. L. (2014). Addressing Health Literacy through clear Health Communication: A Training Program for Internal Medicine Residents. Patient Educ. Couns. 95, 76–82. doi:10.1016/j.pec.2014.01.004
Greene, J. O., and Geddes, D. (1993). An Action Assembly Perspective on Social Skill. Commun. Theor. 3, 26–49. doi:10.1111/j.1468-2885.1993.tb00054.x
Halan, S., Sia, I., Crary, M., and Lok, B. (2015). “Exploring the Effects of Healthcare Students Creating Virtual Patients for Empathy Training,” in International Conference on Intelligent Virtual Agents (Springer), 239–249. doi:10.1007/978-3-319-21996-7_24
Halan, S., Sia, I., Miles, A., Crary, M., and Lok, B. (2018). “Engineering Social Agent Creation into an Opportunity for Interviewing and Interpersonal Skills Training,” in Proceedings of the 17th International Conference on Autonomous Agents and MultiAgent Systems (Stockholm, Sweden).
Kemper, S. (1994). Elderspeak: Speech Accommodations to Older Adults. Aging Neuropsychol. Cogn. 1, 17–28. doi:10.1080/09289919408251447
Kidd, L. I., Knisley, S. J., and Morgan, K. I. (2012). Effectiveness of a Second Life Simulation as a Teaching Strategy for Undergraduate Mental Health Nursing Students. J. Psychosoc Nurs. Ment. Health Serv. 50, 28–37. doi:10.3928/02793695-20120605-04
Kirkpatrick, D. L., and Craig, R. (1970). Evaluation of Training. Evaluation Of Short-Term Training in Rehabilitation , 35Publisher. New York: ERIC.
Kirkpatrick, D. L. (1954). Evaluating Human Relations Programs for Industrial Foremen and Supervisors. Madison, Wisconsin: Doctoral, University of Wisconsin–Madison.
Kirkpatrick, J. D., and Kirkpatrick, W. K. (2016). Kirkpatrick’s Four Levels of Training Evaluation. Alexandria, VA: Association for Talent Development.
Kleinsmith, A., Rivera-Gutierrez, D., Finney, G., Cendan, J., and Lok, B. (2015). Understanding Empathy Training with Virtual Patients. Comput. Hum. Behav. 52, 151–158. doi:10.1016/j.chb.2015.05.033
Kundhal, P. S., and Grantcharov, T. P. (2009). Psychomotor Performance Measured in a Virtual Environment Correlates with Technical Skills in the Operating Room. Surg. Endosc. 23, 645–649. doi:10.1007/s00464-008-0043-5
Lei, L., and Liu, D. (2016). A New Medical Academic Word List: A Corpus-Based Study with Enhanced Methodology. J. English Acad. Purposes 22, 42–53. doi:10.1016/j.jeap.2016.01.008
Li, A., Montaño, Z., Chen, V. J., and Gold, J. I. (2011). Virtual Reality and Pain Management: Current Trends and Future Directions. Pain Manag. 1, 147–157. doi:10.2217/pmt.10.15
Loukas, C., Nikiteas, N., Kanakis, M., and Georgiou, E. (2011). Evaluating the Effectiveness of Virtual Reality Simulation Training in Intravenous Cannulation. Simulation Healthc. 6, 213–217. doi:10.1097/sih.0b013e31821d08a9
Martin, L. R., Williams, S. L., Haskard, K. B., and DiMatteo, M. R. (2005). The challenge of Patient Adherence. Ther. Clin. Risk Manag. 1, 189
Oates, D. J., and Paasche-Orlow, M. K. (2009). Health Literacy. Circulation 119, 1049–1051. doi:10.1161/CIRCULATIONAHA.108.818468
Raij, A., Johnsen, K., Dickerson, R., Lok, B., Cohen, M., Bernard, T., et al. (2006). Interpersonal Scenarios: Virtual\\approx Real?” in IEEE Virtual Reality Conference (VR 2006). IEEE, 59–66.
Rossen, B. H. (2011). Design and Evaluation of Conversational Modeling Methods for Interpersonal Simulation. Ph.D. thesis, Gainesville, FL: University of Florida.
Schmidt, B., and Stewart, S. (2009). Implementing the Virtual Reality Learning Environment. Nurse Educator 34, 152–155. doi:10.1097/nne.0b013e3181aabbe8
Shaw, A., Ibrahim, S., Reid, F., Ussher, M., and Rowlands, G. (2009). Patients' Perspectives of the Doctor-Patient Relationship and Information Giving across a Range of Literacy Levels. Patient Educ. Couns. 75, 114–120. doi:10.1016/j.pec.2008.09.026
Slater, M., Pertaub, D.-P., Barker, C., and Clark, D. M. (2006). An Experimental Study on Fear of Public Speaking Using a Virtual Environment. CyberPsychology Behav. 9, 627–633. doi:10.1089/cpb.2006.9.627
Speer, M. (2015). “Using Communication to Improve Patient Adherence,” in Communicating with Pediatric Patients and Their Families: The Texas Children’s Hospital Guide for Physicians, Nurses and Other Healthcare Professionals (Houston, USA: Texas Children’s Hospital), 221–227.
Suárez, G., Jung, S., and Lindeman, R. W. (2021). Evaluating Virtual Human Role-Players for the Practice and Development of Leadership Skills. Front. Virtual Real. 2, 31. doi:10.3389/frvir.2021.658561
Unger, L. (2010). The Social Role of Linguistic Alignment with In-Group and Out-Group Members Publisher. Edinburgh: The University of Edinburgh.
Ustün, T. B., Chatterji, S., Bickenbach, J., Kostanjsek, N., and Schneider, M. (2003). The International Classification of Functioning, Disability and Health: a New Tool for Understanding Disability and Health. Disabil. Rehabil. 25, 565–571. doi:10.1080/0963828031000137063
Van Wyk, E., and De Villiers, R. (2009). Virtual Reality Training Applications for the Mining Industry. Proc. 6th Int. Conf. Comput. graphics, virtual reality, visualisation interaction Africa, 53–63. doi:10.1145/1503454.1503465
Waisman, Y., Siegal, N., Chemo, M., Siegal, G., Amir, L., Blachar, Y., et al. (2003). Do Parents Understand Emergency Department Discharge Instructions? A Survey Analysis. Isr. Med. Assoc. J. 5, 567
Williams, K. N., Herman, R., Gajewski, B., and Wilson, K. (2009). Elderspeak Communication: Impact on Dementia Care. Am. J. Alzheimers Dis. Other Demen. 24, 11–20. doi:10.1177/1533317508318472
Williamson, J. M. L., and Martin, A. G. (2010). Analysis of Patient Information Leaflets provided by a District General Hospital by the Flesch and Flesch-Kincaid Method. Int. J. Clin. Pract. 64, 1824–1831. doi:10.1111/j.1742-1241.2010.02408.x
Wouda, J. C., and van de Wiel, H. B. M. (2013). How to Attain Expertise in Clinical Communication? Paediatric Respir. Rev. 14, 213–218. doi:10.1016/j.prrv.2013.04.005
Wouda, J. C., and van de Wiel, H. B. M. (2012). The Communication Competency of Medical Students, Residents and Consultants. Patient Educ. Couns. 86, 57–62. doi:10.1016/j.pec.2011.03.011
Wouda, J. C., Zandbelt, L. C., Smets, E. M. A., and van de Wiel, H. B. M. (2011). Assessment of Physician Competency in Patient Education: Reliability and Validity of a Model-Based Instrument. Patient Educ. Couns. 85, 92–98. doi:10.1016/j.pec.2010.09.007
Xie, B., Liu, H., Alghofaili, R., Zhang, Y., Jiang, Y., Lobo, F. D., et al. (2021). A Review on Virtual Reality Skill Training Applications. Front. Virtual Real. 2, 49. doi:10.3389/frvir.2021.645153
Keywords: virtual reality, educational technology, communication skills, kirkpatrick model, virtual humans, virtual patients
Citation: Carnell S, Gomes De Siqueira A, Miles A and Lok B (2022) Informing and Evaluating Educational Applications With the Kirkpatrick Model in Virtual Environments: Using a Virtual Human Scenario to Measure Communication Skills Behavior Change. Front. Virtual Real. 3:810797. doi: 10.3389/frvir.2022.810797
Received: 07 November 2021; Accepted: 28 March 2022;
Published: 14 April 2022.
Edited by:
Missie Smith, Independent researcher, Detroit, Michigan, United StatesReviewed by:
Simon Hoermann, University of Canterbury, New ZealandMeredith Carroll, Florida Institute of Technology, United States
Copyright © 2022 Carnell , Gomes De Siqueira , Miles and Lok . This is an open-access article distributed under the terms of the Creative Commons Attribution License (CC BY). The use, distribution or reproduction in other forums is permitted, provided the original author(s) and the copyright owner(s) are credited and that the original publication in this journal is cited, in accordance with accepted academic practice. No use, distribution or reproduction is permitted which does not comply with these terms.
*Correspondence: Stephanie Carnell , c3RlcGhhbmllY2FybmVsbEBnbWFpbC5jb20=