- 1Bioengineering Department, Institute for Systems and Robotics (ISR-Lisboa), Instituto Superior Técnico, Lisbon, Portugal
- 2Department of Integrative Neurophysiology, Center for Neurogenomics and Cognitive Research (CNCR), Amsterdam Neuroscience, VU University Amsterdam, Amsterdam, Netherlands
Motor-imagery brain-computer interfaces (MI-BCIs) have the potential to improve motor function in individuals with neurological disorders. Their effectiveness relies on patients’ ability to generate reliable MI-related electroencephalography (EEG) patterns, which can be influenced by the quality of neurofeedback. Virtual Reality (VR) has emerged as a promising tool for enhancing proprioceptive feedback due to its ability to induce a sense of embodiment (SoE), where individuals perceive a virtual body as their own. Although prior research has highlighted the importance of SoE in enhancing MI skills and BCI performance, to date, no study has successfully isolated nor manipulated the SoE in VR before MI training, creating a gap in our understanding of the precise role of the priming effect of embodiment in MI-BCIs. In this study, we aimed to examine whether the virtual SoE when induced, as priming of avatar embodiment, and assessed before MI training, could enhance MI-induced EEG patterns. To achieve this, we divided 26 healthy participants into two groups: the embodied group, which experienced SoE with an avatar before undergoing VR-based MI training, and the non-embodied group, which underwent the same MI training without a prior embodiment phase, serving as a control. We analyzed subjective measures of embodiment, the event-related desynchronization (ERD) power of the sensorimotor rhythms, lateralization of ERD, and offline classification BCI accuracy. Although the embodiment phase effectively induced SoE in the embodied group, both groups exhibited similar MI-induced ERD patterns and BCI classification accuracy. This suggests that the induction of SoE prior to MI training may not significantly influence the training outcomes. Instead, it appears that the integration of embodied VR feedback during MI training itself is sufficient to induce appropriate ERD, as evidenced by previous research.
1 Introduction
Brain-Computer Interfaces (BCIs) constitute a cutting-edge area of research facilitating a direct communication channel between the human brain and external devices. This technological paradigm operates by capturing the electrical signals of the brain, primarily leveraging electroencephalography (EEG), and subsequently translating these signals into actionable commands capable of controlling a diverse array of devices, including but not limited to prosthetics, computers, and virtual avatars (Wolpaw et al., 2002). In recent years, motor-imagery (MI)-based BCIs have gained increasing attention in neurorehabilitation due to their potential to assist in the recovery of motor functions in individuals suffering from neurological conditions such as stroke, spinal cord injury, or other motor disorders (Pfurtscheller et al., 2008; Ang et al., 2011; Khan et al., 2020). Within the MI-BCI paradigm, users are instructed to imagine performing a particular movement, such as moving their left or right hand, and the subsequent alterations in brain activity are utilized to control the associated device. Notably, the MI task elicits significant power changes in μ (8–12 Hz) and β (18–26 Hz) EEG rhythms over the sensorimotor cortices. This phenomenon, known as event-related synchronization and desynchronization (ERS/ERD), intriguingly mirrors the modulation of EEG rhythms observed during the planning and execution of actual movements (Pfurtscheller and Lopes da Silva, 1999; Pfurtscheller and Neuper, 2001). Therefore, by repeatedly practicing MI, patients can stimulate motor areas of the brain, fostering the reorganization of neural circuits and aiding in the restoration of motor functions (Pichiorri et al., 2015).
Despite these promising aspects, the effective utilization of MI-BCIs in neurorehabilitation still faces several challenges. These include the high variability in users’ ability to generate robust and distinguishable ERD patterns, along with the requirement for extensive and rigorous training sessions, which can lead to high drop-out rates among users (Lotte et al., 2013). Moreover, the traditional neurofeedback paradigm, a central component of MI-BCI training, often employs abstract and arbitrary visual feedback, limiting the intuitiveness and efficacy of the training (Neuper et al., 2009). Virtual Reality (VR)-based MI-BCIs offer a solution to these challenges by providing immersive and engaging training environments that can enhance user motivation and increase BCI performance (Vourvopoulos et al., 2016; Vourvopoulos et al., 2019). Crucially, VR can deliver immediate and more naturalistic visual feedback to the user, helping them understand whether they are doing the MI task correctly, and leading to better training outcomes (Lotte et al., 2013). For instance, MI training with realistic visual feedback using virtual or robotic hands has been shown to elicit enhanced ERD patterns and improve BCI performance compared to standard screen-based feedback (Alimardani et al., 2014; Penaloza et al., 2018; Skola and Liarokapis, 2018; Choi et al., 2020b; Vourvopoulos et al., 2022). This implies that by observing a virtual body carrying out the expected motor actions, users learn to produce the appropriate signals more consistently and accurately as a form of priming, which can be used to enhance the overall performance of the MI-BCI system.
Specifically, priming is a type of implicit learning wherein a stimulus prompts a change in behavior (Stoykov and Madhavan, 2015). Prior research have shown that physical activity prior to a MI task (motor priming) facilitates the improvement in MI-BCI training in VR, helping also to maximize the engagement of sensory-motor networks (Vourvopoulos and Bermúdez i Badia, 2016; Amini Gougeh and Falk, 2023). Moreover, behavior can be also influenced by the characteristics of the virtual avatar or the digital representations, known as the “Proteus effect” (Yee and Bailenson, 2007). Latest research has shown an impact of the “Proteus effect” in MI, and specifically the impact of the age of the virtual avatar on MI execution time (Beaudoin et al., 2020). Thus, priming in VR can provide an additional path for driving behavior change and possibly accelerate learning in a rehabilitation setting. Nonetheless, when utilizing VR for priming-induced learning, a crucial aspect of feedback lies in the induction of the sense of embodiment (SoE).
Specifically, SoE refers to the illusory experience that a virtual body and hands are one’s own body and hands. Several conceptualizations of SoE exist, and for the purposes of our study, we adopt the framework proposed by (Kilteni et al., 2012). Concretely, they define SoE as a collection of sensations associated with being situated within, possessing, and exercising control over a body, specifically in the VR context. This sensation comprises three interrelated subcomponents: the sense of self-location (i.e., perceiving oneself as situated within a body), the sense of ownership (i.e., attributing experienced sensations to the body), and the sense of agency (i.e., identifying oneself as the initiator of bodily movements). When these subcomponents align, an individual can attain a SoE towards a body or body part, interpreting its characteristics as if they belonged to their own physical body. The rubber hand illusion serves as a classic instance of SoE where individuals experience illusory ownership over a rubber hand placed in a plausible anatomical position and synchronously stroked along with the individual’s real hand (Botvinick and Cohen, 1998). This pioneering experiment has been successfully replicated in VR and extended toward an entire virtual body through the use of not only visuotactile stimuli (Petkova and Ehrsson, 2008) but also visuomotor stimuli, where the virtual body’s movements align both spatially and temporally with the real body movements (Peck et al., 2013), and visuoproprioceptive stimuli, where the virtual body merely overlaps with the real body’s position (Maselli and Slater, 2014). Furthermore, Perez-Marcos et al. (2009) were first to show that sense of ownership of a virtual hand (a.k.a., virtual hand illusion) can be induced through the use of MI-BCI, where neurofeedback is presented through virtual hands performing the imagined motor action in synchrony. A later study found that both virtual hand illusion and hand MI share similar electrophysiological correlates, specifically the μ-band desynchronization, in fronto-parietal areas of the brain (Evans and Blanke, 2013), giving rise to the idea that SoE could potentially enhance ERD patterns during MI training. By developing a sense of ownership over the virtual hands during MI-BCI training, participants may more intuitively and comfortably accept the feedback conveyed through the movements of these virtual hands, potentially improving the MI-BCI performance. Several studies have since investigated the relationship between SoE and MI-BCIs with varying results. Choi et al. (2020b) showed that the process of action observation–observing a bimodal hand movement in VR while simultaneously engaging in MI of the same movement–led to enhanced ERD power and higher classification accuracy when compared to action observation provided via a conventional monitor display. Similarly, Du et al. (2021) found that a 3-min synchronous visuo-tactile stimulation of a virtual hand preceding a MI task led to greater ERD phenomena compared to the same stimulation of a rubber hand. Although both studies credited the enhanced MI abilities to the immersive nature of VR headsets and the illusion of embodiment they create, neither delved into quantifying embodiment levels nor investigated their direct influence on MI skills.
From studies that directly explored the relationship between SoE (or its sub-components) and BCI performance, some found positive correlations (Alimardani et al., 2014; Penaloza et al., 2018; Choi et al., 2020a; Juliano et al., 2020), whereas others found no relationships at all (Braun et al., 2016; Skola and Liarokapis, 2018; Skola et al., 2019). In terms of the relationship between SoE and ERD features, two studies found positive correlations (Braun et al., 2016; Penaloza et al., 2018), one study found no relationship (Skola and Liarokapis, 2018), and two studies found mixed effects. Namely, Skola et al. (2019) found a positive correlation between sense of ownership and ERD features, but a negative correlation between sense of agency and ERD features, whereas Nierula et al. (2021) found the opposite effects. Therefore, there seems to be no conclusive evidence that (virtual) SoE can increase MI-BCI performance, nor it improves the modulation of brain patterns associated with MI. In addition, existing studies have investigated SoE in conjunction with MI training, making it difficult to isolate the specific effects of embodiment on MI skills and BCI performance. As a result, no study up to date has successfully isolated and manipulated the SoE variable before MI training nor studied the priming effect of avatar embodiment, creating a gap in our understanding of the precise role of embodiment in MI-BCIs.
In the present study, we aimed to examine whether the virtual SoE, when induced and assessed before MI training as a form of priming, could enhance MI-induced ERD patterns. To achieve this, we established a between-subjects design in which the experimental group was subjected to a 5-min embodiment induction phase in VR, while the control group was intentionally deprived of embodiment cues. Subsequently, both groups underwent identical MI training phase with action observation of bimodal hand grasp movement in VR. From the acquired MI-EEG data we analyzed ERD power of the sensorimotor rhythms, lateralization of ERD, and offline classification BCI accuracy. We hypothesized that inducing SoE prior to MI training, as a form of avatar embodiment priming, might allow participants to better engage with their virtual bodies, fostering improved MI skills. Therefore, in the embodied group we expected to find 1) enhanced ERD patterns during MI, specifically a greater and lateralized power reduction in the μ rhythm, and 2) increased discriminability between the two classes of MI, i.e., increased offline BCI accuracy. In addition, we expected that a stronger SoE would be associated with a more potent ERD and a higher BCI accuracy. Finally, this study resulted into the production of freely and publicly available labeled datasets of electrophysiological signals (EEG, EMG, Temperature and Head Accelerometer data) during embodiment in VR (Vagaja and Vourvopoulos, 2023).
2 Methods
2.1 Participants
A total of 32 healthy participants were recruited for this study, most of whom were university students. Four participants were excluded due to technical issues during the EEG recording (either extensive artifacts in the data or faulty electrodes), and two participants failed to follow correctly the instructions. Thus, a total of 26 participants were eligible for the analysis, consisting of 10 males (mean age 25.4 ± 7.4) and 16 females (mean age 23 ± 3.2). All participants were right-handed, reported normal or corrected-to-normal vision, and had no motor impairments. Three participants had previous experience with BCIs, and five participants used VR more than twice. None of the participants were aware that the study aimed to examine the effect of virtual embodiment on MI skills. Participants were randomly assigned to either the embodied group (N = 13; 7 Female/6 Male) or the non-embodied group (N = 13; 8 Female/5 Male), which served as a control. All participants signed an informed consent before participating in the study in accordance with the 1964 Declaration of Helsinki.
2.2 Experimental design
A between-subject design was used to investigate the effect of the virtual embodiment priming phase on the subsequent motor-imagery training phase in VR. The embodiment phase involved immersing the experimental group of participants in a virtual laboratory room where they could perceive a virtual body moving in synchrony with their own movements from a first-person perspective. This was supplemented with a virtual hand illusion to enhance the SoE. On the other hand, the control group participants observed the virtual body from a third-person perspective, where the movements of the virtual body were independent of their own. This approach, adapted and modified from Wolf et al. (2021), was used to negate SoE while maintaining equivalent levels of presence (i.e., the sense of being physically present in the VR environment), appearance (i.e., the feeling that the virtual body resembles one’s real body), and duration of VR exposure in both groups. Watch the video for an overview of the whole experiment. The experiment comprised four main blocks (Figure 1A): 1) equipment setup and instructions (45–60 min), 2) resting-state EEG recording (4 min), 3) inducing or breaking the sense of embodiment in VR (5 min), and 4) MI training in VR (15 min). The entire experiment lasted approximately 90–120 min. Directly after embodiment manipulation, participants answered a questionnaire that measured their subjective sense of embodiment and physical presence (see Section 2.3).
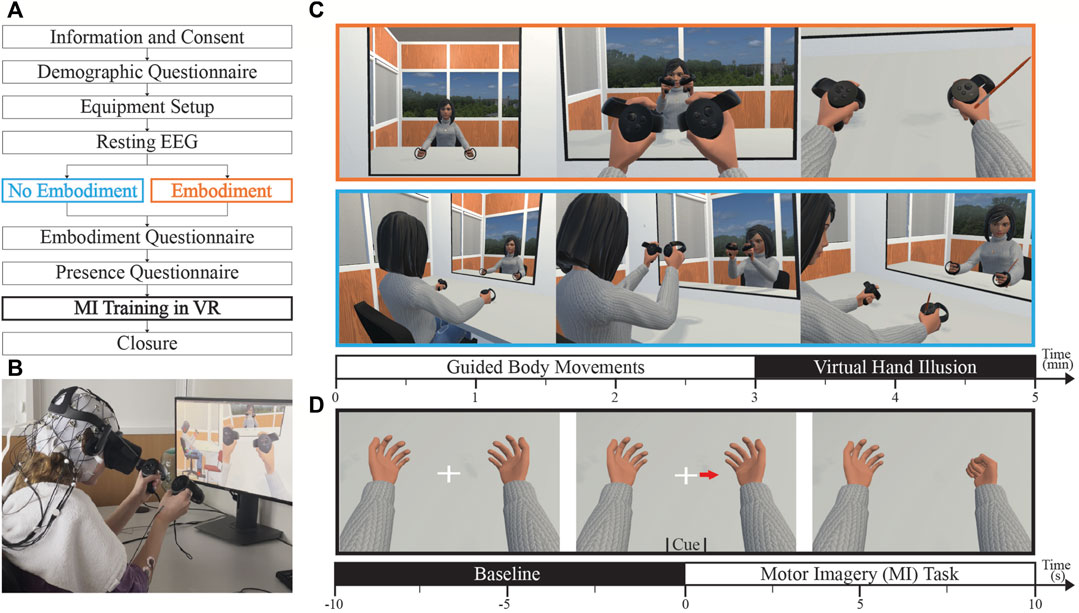
FIGURE 1. Experimental design. (A) The flowchart visualizes the controlled experimental procedure and gives an overview of the performed measurements; (B) A participant wearing the 32 active electrodes EEG system, HMD VR, and controllers; (C) Virtual scene during the embodiment priming phase for the embodied group (orange) and non-embodied group (blue); (D) Virtual scene during the MI training session for both groups.
2.2.1 Virtual embodiment priming phase
Participants sat at a desk in a 2 m × 4 m laboratory room. In their hands, they held VR controllers. After entering the virtual environment, they found themselves in a very similar reproduction of the room, but instead of a computer, there was a mirror on the desk. During the first 3 min they were guided to look around the environment, look in the mirror, explore and move their virtual body, and describe their surroundings and body (Peck et al., 2013). Then a brush appeared in the virtual environment and participants were asked to observe the brush stroking the hand of the virtual body for 2 min straight. Three types of embodiment induction triggers were used and manipulated between the two groups of participants: embodied and non-embodied group (Figure 1C).
1. Visuoproprioceptive triggers–In the embodied group, participants viewed a virtual body that matched their gender from a first-person perspective. This virtual body was positioned in the same location as their real body, and they could see it when looking directly down at themselves and in the virtual mirror. In contrast, participants in the non-embodied group viewed the gender-matched virtual body from a third-person perspective and did not have a virtual body that replaced their own body. When looking in the mirror, they could not see themselves, but only the reflection of the virtual body sitting in front of them.
2. Visuomotor triggers–Participants were guided to move their hands, head, and upper body to explore the virtual environment for 3 min continuously. In the embodied group, participants experienced the virtual body moving in synchrony with their own body movements, inducing a strong sense of body ownership and agency over the virtual body (Kilteni et al., 2015), whereas in the non-embodied group, the virtual body moved independently of participants’ movements.
3. Visuotactile triggers–To induce the virtual hand illusion in the embodied group, the experimenter used a soft paintbrush to stroke the participant’s real right hand in synchrony with an animation of a virtual brush stroking the corresponding virtual hand. The strokes were applied 24 times in a smooth and continuous motion, lasting on average 5 s, from the thumb proximal phalanx to the index metacarpal. To ensure a fair comparison, the 2-min virtual hand illusion was also implemented in the non-embodied group. However, participants in this group were asked to observe the brush stroking the right hand of the virtual body in front of them while the experimenter asynchronously stroked the participant’s real left hand.
2.2.2 MI training phase with action observation in VR
All participants performed the hand grasp MI training task in the same virtual environment as used during the embodiment phase (Figure 1D). However, in contrast to the embodiment phase, a mirror was not present on the desk. This allowed participants to focus solely on their virtual hands viewed from a first-person perspective. The training consisted of 40 randomly presented trials, with 20 trials per class (left/right hand grasp). Each trial comprised a 10-s resting period and a subsequent 10-s MI period. During the resting period, participants were instructed to focus on the cross positioned between the two virtual hands. After 10 s, an arrow appeared, pointing either to the left or right direction, and participants were instructed to repeatedly imagine grasping the indicated hand while observing the corresponding virtual hand executing the movement. Before the experiment, all participants were taught how to perform kinesthetic MI by imagining the sensation of making a fist in their hand. The participants clenched their fists a few times to indicate their understanding of the task. The MI-BCI training protocol was approved by the Ethics Committee of CHULN and CAML (Faculty of Medicine, University of Lisbon) with reference number: 245/19.
2.3 Questionnaires
Pre-experimental questionnaire surveyed the demographics and participants’ experience with VR, BCIs, and neurofeedback. Immediately after the embodiment phase, participants received two questionnaires surveying their sense of embodiment and physical presence (Table 1). They received the following instructions: “Please select your level of agreement with the following statements. There are no right or wrong answers. Statements refer to the experience you just had in VR. Virtual body refers to the avatar you saw in VR. The following phrases are used interchangeably and carry the same meaning: my body, my own body, my real body.” All questionnaire items were displayed in random order and were answered on a 7-point Likert scale, ranging from 1 (“strongly disagree”) to 7 (“strongly agree”). SoE was assessed using questions adapted from a standardized embodiment questionnaire proposed by Peck and Gonzalez-Franco (2021). This questionnaire consists of 16 items that measure SoE on the following subscales: appearance (i.e., the extent to which participants feel that the virtual body looks like their real body), response (i.e., the extent to which participants feel that the virtual body responds in a way that is consistent with their own movements and actions), ownership (i.e., the extent to which participants feel that the virtual body is their own body or a body that they have control over), multisensory (i.e., the extent to which participants feel that the virtual body is integrated with their own sensory experiences), and agency (i.e., the extent to which participants feel as the initiators of bodily movements). Physical presence, which refers to the sense of being physically located in a virtual environment, was assessed using the Multimodal Presence Scale (MPS) developed by Makransky et al. (2017). The MPS is a 15-item questionnaire that measures the three-dimensional theoretical model of presence: physical, social, and self-presence as described in Lee (2004). The physical presence subscale includes five items that assess the extent to which participants: 1) experience the virtual environment as mimicking the physical appearance of the real world; 2) are completely captivated by the virtual world and, therefore, become less aware of the real world in which they actually exist; 3) experience a general and intuitive sense of being in the virtual environment; 4) are unaware of the process by which the physical environment is mediated. This subscale was used as a control measure, since the virtual environment was maintained between the two groups.
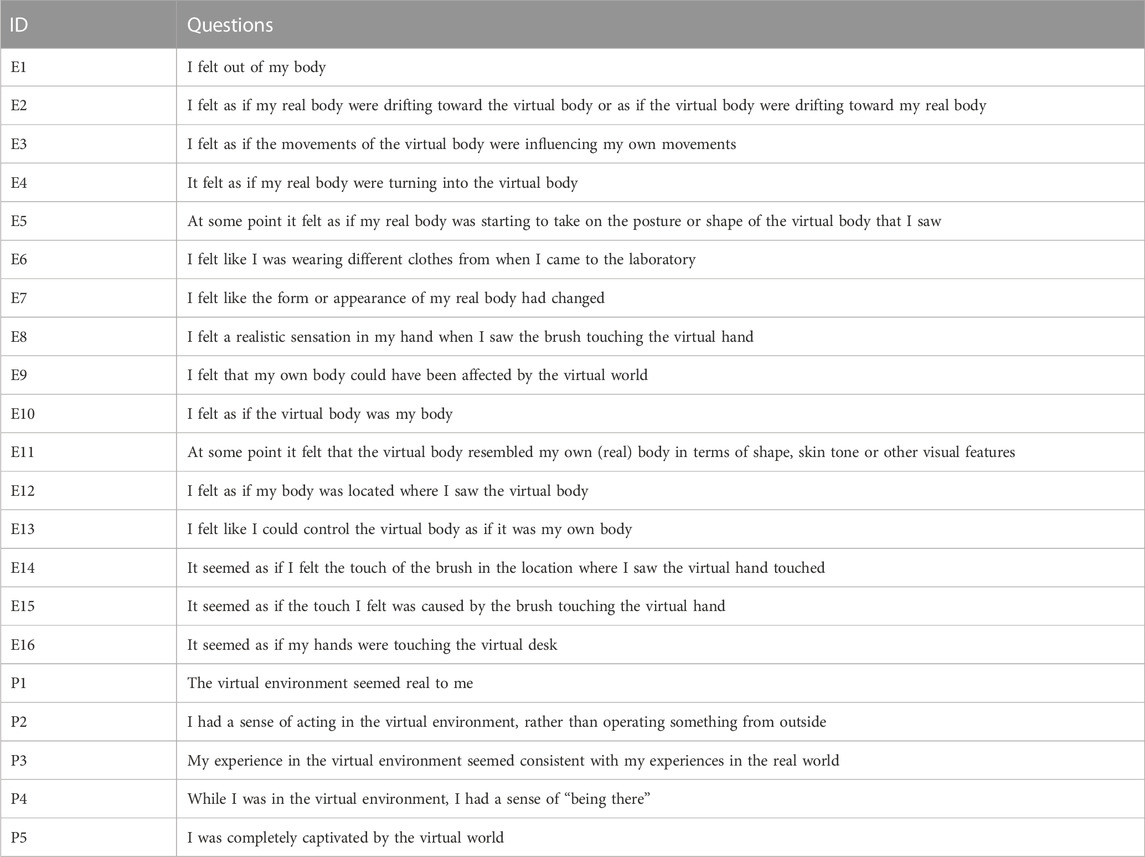
TABLE 1. Questionnaire assessing the sense of embodiment and physical presence. Adapted from Peck and Gonzalez-Franco (2021); Makransky et al. (2017).
To validate the internal consistency of our composite scores, derived from several Likert-scale items, we utilized Cronbach’s alpha (Cronbach, 1951). This measure assesses how closely related a set of items are as a group and is a commonly used measure of internal consistency in social and psychological research. Scores approaching or exceeding 0.7 are typically considered reliable, indicating that items cohesively measure the same underlying construct. Using the adapted SoE questionnaire from Peck and Gonzalez-Franco (2021), we determined Cronbach’s alpha for embodiment sub-scales. Specifically, appearance, response, ownership, multisensory, and agency yielded values of α = 0.75, α = 0.77, α = 0.76, α = 0.79, and α = 0.64 respectively. The physical presence subscale from the multimodal presence scale, by Makransky et al. (2017), achieved an alpha of α = 0.84. These results underscore the reliability of the questionnaires used, with the agency subscale suggesting potential areas for further exploration.
2.4 Experimental setup
2.4.1 EEG acquisition
For EEG data acquisition, a wearable wireless EEG amplifier was used (LiveAmp; Brain Products GmbH, Gilching, Germany) with 32 active electrodes (+3 ACC) at a sampling rate of 500 Hz. The spatial distribution of the electrodes followed the 10–20 EEG system. Ground and reference electrodes were located at the central and forehead lobes, respectively. The EEG amplifier was interfaced wirelessly through 2.4 GHz ISM band to the desktop computer, responsible for the EEG signal processing. The electrodes were carefully placed under the Oculus Rift CV1 such that slight head movements could not cause significant noise in the data (Figure 1B).
2.4.2 VR scene and equipment
The virtual scene was developed and implemented using the Unity 3D game engine (Unity Technologies, San Francisco, United States). The project files and the source code are available on GitHub1. Virtual bodies were created in Ready Player Me2 (a free modelling software used to create customizable 3D human avatars). The equipment used for viewing the virtual environment was Oculus Rift CV1 head-mounted-display developed and manufactured by Oculus VR, a subsidiary of Facebook, Inc., United States (Figure 1B). The head-mounted-display consists of a 2 x AMOLED binocular display, with a 1080 × 1200 resolution per-eye, 87° horizontal Field of View, and 6 degrees-of-freedom tracking. In the embodiment scenario, hand movements were tracked with Oculus Touch controllers and two Constellation sensors standing on each side of the desk. Tracking data was then applied to the virtual body using QuickVR, an open-source Unity library for achieving virtual embodiment (Oliva et al., 2022). Participants could therefore experience visuomotor synchronization between their upper body real movements and the movements of their virtual body.
2.5 Data analysis
2.5.1 EEG pre-processing
EEG signals were pre-processed and analyzed using MATLAB R2023a (The MathWorks, MA, United States) and EEGLAB toolbox v2022.1 (Delorme and Makeig, 2004). After down-sampling to 125 Hz, the signals were band-pass filtered between 1 and 40 Hz, re-referenced to common average, and epoched between −10 and 10 s for left and right MI trials. Next, Independent Component Analysis (ICA) was performed to identify artifactual components of non-brain origin. For the rejection of these components, the IClabel tool was used. IClabel uses a trained classifier for EEG independent components (Makeig et al., 1995; Pion-Tonachini et al., 2019), and provides a set of probability values for each component. For our datasets, we selected to automatically reject artifacts of “eye” and “muscle” origin with a probability value above 90%. Moreover, each dataset was manually inspected and bad epochs, as well as additional IC components that had not been detected by the automated method, were removed.
2.5.2 Time-frequency analysis
Following pre-processing, the event-related spectral perturbation (ERSP) was extracted from the epoched EEG signals. ERSP values were calculated for each channel, time point, and frequency band within the μ (8–12 Hz) range, and were then converted to ERD according to (Pfurtscheller and Aranibar, 1979) (Eq. 1). The resulting ERD values represent the percentage decrease in μ-power during the MI task compared to baseline. The sensorimotor area composed of the electrodes C3 and C4 was used to display the time-frequency ERSP maps. Additionally, the spatial distribution of the ERD over the scalp was computed by averaging ERD values in each channel within the μ band and the 10 s MI period. The μ band was chosen as it is the most reactive feature during MI (Pfurtscheller and Lopes da Silva, 1999).
2.5.3 Lateralization index
Lateralization between hemispheres is generally assessed by a lateralization index (LI), a measure commonly used to calculate the imbalance of neural activation intensity in brain imaging studies (Doyle et al., 2005). In this research, LI was determined using the ERD over the C3 and C4 electrodes, as these electrodes are believed to capture the most significant desynchronization near the sensorimotor area (Pfurtscheller and Lopes da Silva, 1999). Lateralization was assessed by subtracting the ERDs of electrodes contralateral to the movement side (C3 for right-hand MI and C4 for left-hand MI) from ERDs of electrodes ipsilateral to the movement side (Eq. 2). If the ERD value on the opposite side (contralateral) is lower than on the same side (ipsilateral), the LI value is positive, which suggests a higher level of contralateral desynchronization in the elicited ERD. Finally, LI was calculated as the average of the ERD differences on the right and left sides, using the following formula.
2.5.4 Offline BCI performance
To assess the discriminability of MI features (left vs. right hand) within each group, we trained a linear classifier using a standard BCI feature extraction and classification method. Specifically, for feature extraction, we computed 6 Common Spatial Patterns filters between the α and β bands (8–28 Hz). Common Spatial Patterns is a recognized and efficient algorithm in BCI design that can create spatial filters to optimize the discriminability between two distinct classes (Ramoser et al., 2000; Lotte, 2014). Next, we trained a Shrinkage Linear Discriminant Analysis classifier and performed a Monte Carlo cross-validation with a test set size of 20% of the data. The score for each fold of the cross-validation was computed, and the mean score was compared against the chance level, i.e., the accuracy expected from a random prediction. The final classification accuracy (%) reported is the average of these accuracies across all ten folds.
2.5.5 Statistical analysis
All statistical analyses were performed using R version 4.3.0 (R Core Team, 2018). Our dependent variables were mean ERD (%) of the C3 and C4 electrodes, lateralization index, and offline BCI performance (%). Our independent variables were composite questionnaire scores, each one being an average across multiple Likert scale items (see Table 1).
• Appearance = (E1 + E2 + E3 + E4 + E5 + E6 + E9 + E16)/8
• Response = (E4 + E6 + E7 + E8 + E9 + E15)/6
• Ownership = (E5 + E10 + E11 + E12 + E13 + E14)/6
• Multi-sensory = (E3 + E12 + E13 + E14 + E15 + E16)/6
• Agency = (E3 + E13)/2
• Embodiment = (Appearance + Response + Ownership + Multi-sensory)/4
• Physical Presence = (P1 +P2 +P3 +P4 +P5)/5
The normality of the distribution of all variables was assessed using the Shapiro-Wilk normality test. For the ERD, LI, accuracy, and questionnaire measures (appearance, response, agency, embodiment), a series of Welch Two Sample t-tests were performed. For the variables multisensory, ownership, and presence, Wilcoxon Rank Sum tests with a false discovery rate (FDR) correction (Benjamini and Hochberg, 1995) were performed because the assumptions of the t-test were not met for these variables. Pearson’s correlations were performed between all dependent and independent variables and were corrected for multiple comparisons using a 5% FDR. The significance level was set at 5% (p
3 Results
3.1 Sense of embodiment and presence
Our first goal was to verify if the embodiment phase was effective in inducing the sense of embodiment over the virtual body. As expected, participants in the embodied group reported higher levels of overall SoE than the participants in the non-embodied group (t (18.39) = 3.42, p = 0.01, d = −1.34) (Figure 2). In addition, embodied group showed significant differences in the questionnaire scores: response (t (20.22) = 2.51, p = 0.03, d = −0.98), agency (t (19.99) = 2.54, p = 0.03, d = −1.00), multisensory (W = 27.50, p = 0.01) and ownership (W = 23.00, p = 0.01). The effect sizes (Cohen’s d) were moderate to large. No significant differences were found for the variables’ appearance (t (22.91) = 1.20, p = 0.28, d = −0.48) and presence (W = 74.50, p = 0.87). This was expected since both the virtual environment and the virtual body were kept the same in both groups. Therefore, our manipulation was specific to inducing (or breaking) the sense of embodiment.
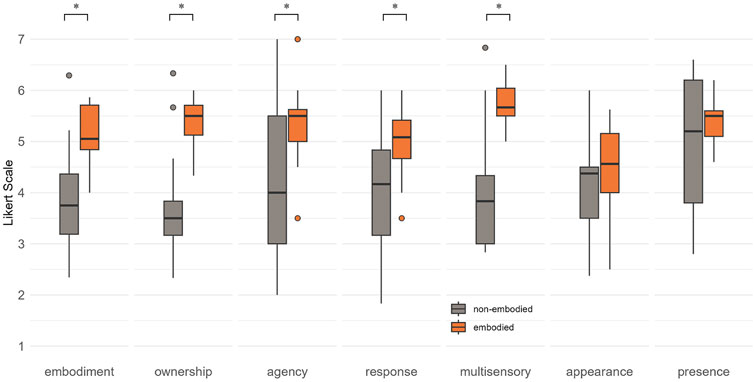
FIGURE 2. Differences in questionnaire scores between the embodied (orange) and non-embodied (gray) groups. Y-axis: Responses on the 7-point Likert scale, ranging from 1 (strongly disagree) to 7 (strongly agree). Scores above 4 indicate a sense of embodiment and presence. * indicates significant differences (i.e., p <0.05).
3.2 Participants’ ability to induce ERD during MI in VR
Before analyzing between-group differences in MI-induced brain patterns, we first assessed participants’ ability to induce sufficient ERD during MI training. The Single Sample Wilcoxon Signed-Rank test was used to compare the ERD power over C3 and C4 electrodes during the MI period with no ERD (0% ERD) for both left and right trials. In the non-embodied group, 12 out of 13 participants showed a statistically significant decrease in ERD power for both left and right trials over both electrodes. However, Subject 20 showed statistically non-significant decrease in ERD power for right trials over the ipsilateral C4 electrode (Z = −0.88, p = 0.38|Mdn = −2.00, IQR = 22.68). In the embodied group, 9 out of 13 participants demonstrated statistically significant decrease in ERD power for both left and right trials over both electrodes. Nonetheless, Subject 3 exhibited statistically non-significant decrease in ERD power for left trials over the contralateral C4 electrode (Z = −1.84, p = 0.07|Mdn = −8.22, IQR = 29.85). Subjects 3 and 7 displayed statistically significant decrease in ERD power over the ipsilateral C4 electrode for right trials (Z = 6.81, p < 0.001|Mdn = 8.15, IQR = 13.43 and Z = 7.06, p < 0.001|Mdn = 23.17, IQR = 29.08, respectively). Furthermore, Subject 14 showed statistically non-significant decrease in ERD power over the contralateral C3 electrode for right trials (Z = 2.15, p = 0.03|Mdn = 2.98, IQR = 10.10). These results suggest that 92% of participants in the non-embodied group were able to produce distinct ERD patterns during the MI session, compared to 69% of participants in the embodied group. Notably, 3 subjects in the embodied group did not show statistically significant decreased ERD power drop during right MI trials.
3.3 Between-group differences in ERD, lateralization index, and BCI performance
Our statistical analysis using Welch Two Sample t-tests did not reveal any significant differences (p > 0.05) between the groups in terms of μ-band ERDs around the sensorimotor area, LI, and BCI performance (Table 2). However, the non-embodied group exhibited small differences in terms of mean ERD power over both electrodes in both trials compared to the embodied group (Figure 3B). Furthermore, the temporal evolution of ERD was similar across two groups: the μ-rhythm power gradually changed relative to resting state and remained at the reduced level until the end of the trial (Figure 3A). In terms of lateralization, the non-embodied group showed small differences with a slightly higher lateralization of ERD during MI (M = 11.50, SD = 6.00) than the embodied group (M = 9.07, SD = 9.56), but the difference was not statistically significant (t = 0.78, p = 0.45) (Figure 4). The positive LI values observed in both groups indicate a predominantly contralateral decrease in ERD power during left- and right-hand MI (Figure 3C). In terms of BCI performance, although we observed a difference in the Linear Discriminant Analysis classification score in favor of the embodied group (embodied: M = 77.40%, SD = 20.53%; non-embodied: M = 75.19%, SD = 12.52%) (Figure 5), no statistically significant differences were found between the two groups (t = 0.33, p = 0.74). Altogether, these results suggest that there were no significant group-based variations in ERD features and BCI accuracy, indicating comparable outcomes between the non-embodied and embodied conditions.
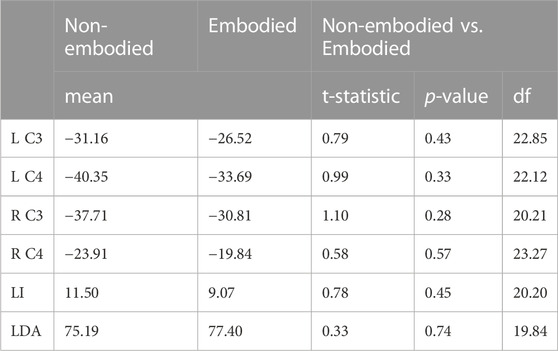
TABLE 2. t-test results from the group differences of ERD power (%) over contralateral (R C3, L C4) and ipsilateral (R C4, L C3) electrodes, lateralization index (LI), and offline BCI accuracy (LDA, Linear Discriminant Analysis).
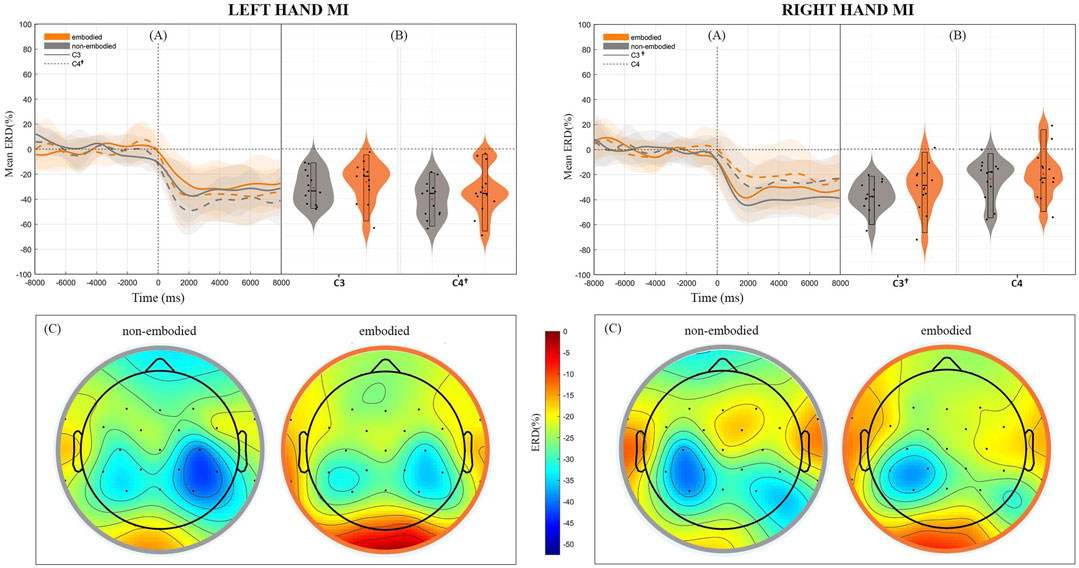
FIGURE 3. ERD grand averages across embodied (orange) and non-embodied (gray) groups during left and right motor imagery trials. (A) ERD time course. Lines represent mean ERD values (mean values were calculated within each subject across the μ frequency range, and then a grand mean value was obtained). Color shapes show the corresponding 25th and 75th percentiles. The vertical dashed lines represent the time limit of 10-s trial, while the horizontal line indicates the resting state; (B) ERD distributions for C3 and C4 electrodes between 0 and 10000 ms. Red dot and black line represent the group mean and median ERD value, respectively; (C) Topographic distribution patterns of mean ERD. The colors on the topographic maps indicate ERD magnitudes (blue color indicates strong ERD). In all graphs, ERD is expressed as a percentage and represents the decrease in μ-power during the MI task compared to the baseline (0% ERD). † indicates contralateral electrode.
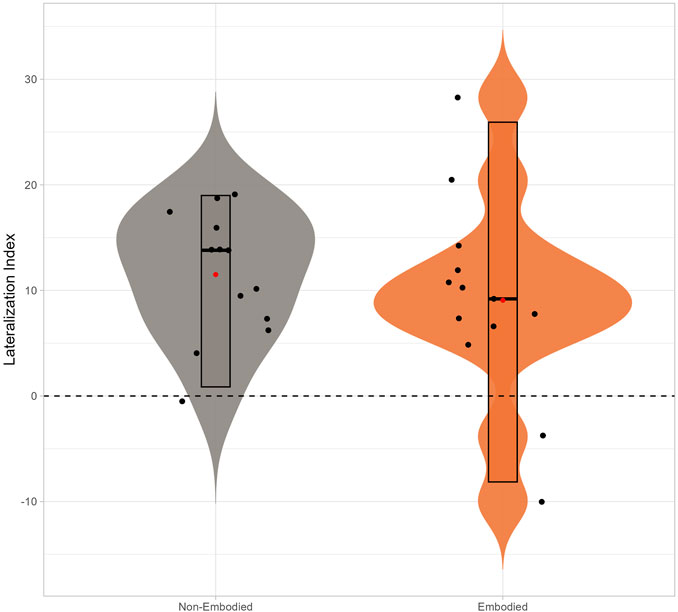
FIGURE 4. Differences in lateralization index between the embodied (orange) and non-embodied (gray) groups. The red dot and black line represent the group mean and median LI, respectively.
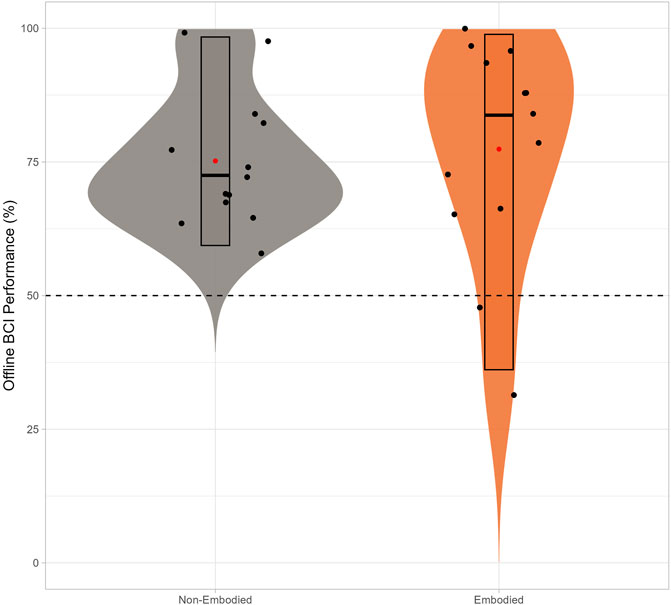
FIGURE 5. Differences in offline BCI accuracy between the embodied (orange) and non-embodied (gray) groups. The red dot and black line represent the group mean and median accuracy, respectively.
3.4 Relationship between ERD, BCI performance and subjective embodiment measures
Investigation into the relationship between embodiment and the ability to modulate μ-rhythms across all subjects showed no correlation between SoE score and ERD power over C3 and C4 electrodes in left trials (r = −0.06, p = 0.79 and r = 0.08, p = 0.72 respectively) as well as ERD power over C3 and C4 electrodes in right trials (r = 0.21, p = 0.31 and r = 0.21, p = 0.32 respectively) (Figure 6A). Similarly, no correlations were found between SoE and LI (r = −0.13, p = 0.54) (Figure 6B), and BCI accuracy (r = −0.13, p = 0.55) (Figure 6C). Regarding the remaining questionnaire scores (appearance, response, ownership, multisensory, agency, physical presence), no significant correlations were found with the ERD features (ERD power of contralateral and ipsilateral electrodes, LI) nor BCI performance. These results suggest that the induction of SoE and physical presence prior to MI training does not have a substantial or statistically significant influence on ERD modulation at the sensorimotor area during the MI task.
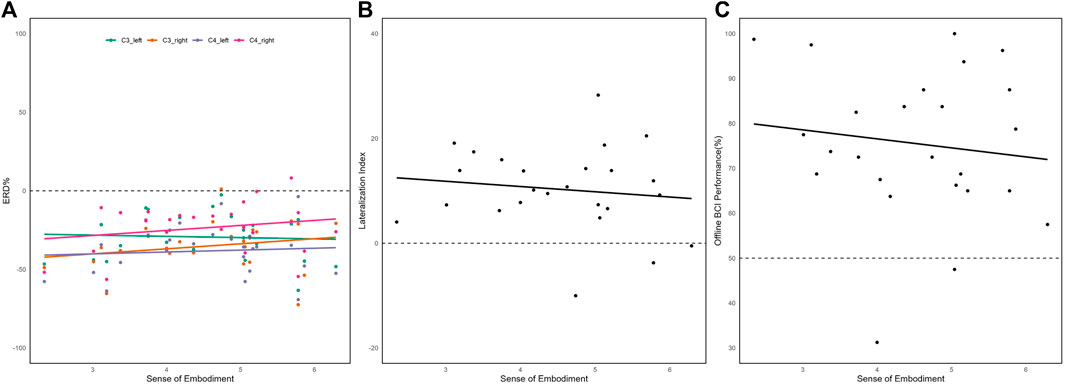
FIGURE 6. Correlations between reported sense of embodiment and (A) ERD (%) of contralateral and ipsilateral C3 and C4 electrodes; (B) lateralization indices across all subjects, and (C) offline BCI performance.
4 Discussion
To the best of our knowledge, the present study is the first to explore the priming effect of avatar embodiment, on MI-induced brain patterns. This study draws on previous research that suggests shared electrophysiological correlates between virtual hand illusion and hand MI (Evans and Blanke, 2013), VR priming (Vourvopoulos and Bermúdez i Badia (2016); Amini Gougeh and Falk (2023), the impact of “Proteus effect” in MI (Beaudoin et al., 2020), as well as the potential to enhance ERD patterns using VR during MI training (Braun et al., 2016; Penaloza et al., 2018). Our hypothesis was that inducing virtual SoE prior to MI training in VR could act as a form of priming that could further enhance MI-induced ERD patterns and increase offline BCI accuracy. While our results confirmed that a 5-min embodiment phase successfully induced a stronger SoE over the virtual body in the embodied group than in the non-embodied group, the subsequent MI-induced decrease in μ-power and the lateralization of ERD were similar across both groups. In addition, no differences were found in offline BCI accuracy between the two groups. Together, these results suggest that induction of embodiment prior to a MI training session in VR, does not impact MI skills nor seems to matter for an online MI-BCI session.
In our methodology, we employed subjective embodiment measures to quantify the strength of embodiment illusion manipulation between the two groups. As anticipated, participants in the embodied group reported significantly higher SoE than those in the non-embodied group. Importantly, feelings of VR presence and avatar appearance were reported similarly across groups, indicating that our manipulation specifically altered the SoE, without unintended effects on other aspects of the virtual experience. Additionally, we observed that the average embodiment scores for the non-embodied group were close to the neutral point of 4 on the 7-point Likert scale, suggesting that participants in this group neither strongly agreed nor disagreed with feeling embodied in the virtual avatar. Together, these findings suggest that our embodiment illusion manipulation was effective: a stronger sense of embodiment was created in the embodied group, while the non-embodied group experienced more neutral feelings towards embodiment. Notably, we observed great variability in the experiences of embodiment among participants in the non-embodied group, particularly in the subcomponents of agency, response, and multisensory perception. The participants’ verbal feedback at the end of the experiment could shed light on this finding. Despite being in the no-embodiment illusion condition, some participants experienced moments of identification with the avatar by actively attempting to synchronize their own movements with those of the avatar. This spontaneous SoE was further underscored by reports of ‘connecting’ with the avatar during asynchronous stroking of the incorrect hand. These subjective experiences potentially led to the formation of certain expectations about the experiment, thereby blurring the distinction between the embodied and non-embodied conditions. Therefore, the use of asynchronous visuomotor and visuotactile correlations in the non-embodied group could be a contributing factor to the observed variability in the reporting of embodiment. To minimize this variability and ensure a more consistent participant experience in future studies, it may be necessary to refine or reconsider the use of these parameters when designing no-embodiment conditions. Moreover, apart from instances of spontaneous embodiment reports, it is highly probable that as the MI-BCI session commenced and participants began to observe the virtual reality hands, the sense of embodiment emerged after a few trials, even for those who initially felt “disembodiment” in the non-embodied groups. In essence, it is quite likely that both groups experienced a sense of embodiment during the MI-BCI training session.
Furthermore, our study highlighted the variability in MI skills among participants, with some individuals exhibiting successful engagement in the MI task and while others experiencing challenges in evoking consistent ERD patterns. While 12 out of 13 participants in the non-embodied group successfully produced significant ERD, only 9 out of 13 participants in the embodied group achieved the same level of ERD. Additionally, three individuals in the embodied group exhibited no ERD, which may explain why we observed a slightly stronger mean ERD power as well as higher lateralization of ERD in the non-embodied group. Nevertheless, these findings align with previous research on MI proficiency (McKelvie and Demers, 1979; Isaac and Marks, 1994) and the prevalence of BCI inefficiency, where individuals may struggle to achieve satisfactory control or fail to elicit the required EEG patterns during BCI tasks (Allison and Neuper, 2010). The potential imbalance in MI proficiency levels between the groups is an important consideration when interpreting the results. It is plausible that the non-embodied group contained a higher ratio of participants with robust MI skills, which may have influenced the marginally superior mean ERD values observed in that group compared to the embodied group. Specifically, numerous recent research studies have explored how individual variances, such as personality traits, cognitive capabilities (Jeunet et al., 2015; Leeuwis et al., 2021), and gender (Wriessnegger et al., 2020) influence the performance of MI-BCI in the context of user training and learning. Nonetheless, in terms of gender differences, a recent meta-analysis of large EEG datasets consisting of 248 subjects, showed no significant differences in μ-ERD during MI (Alimardani et al., 2023).
Finally, despite our study not finding statistically significant differences in ERD modulation and offline BCI accuracy between groups, or significant correlations with reported embodiment scores, the high classification accuracy of around 70% achieved by both groups underscores that MI training with embodied VR feedback can lead to satisfactory BCI performance without necessarily requiring a prior embodiment phase. This beneficial impact of VR feedback and the embodiment it induces on MI training and BCI performance has been well documented. Research has shown that MI training paired with embodied VR feedback increases ERD power and BCI accuracy, outperforming training that uses standard screen-based feedback (Penaloza et al., 2018; Juliano et al., 2020; Vourvopoulos et al., 2022). Furthermore, a positive correlation has been observed between the SoE induced during VR-based MI training and ERD power (Braun et al., 2016; Penaloza et al., 2018), as well as BCI accuracy (Alimardani et al., 2014; Penaloza et al., 2018; Choi et al., 2020a; Juliano et al., 2020). Our findings contribute to this body of research, suggesting the timing and context of embodiment could be crucial. It appears that when SoE is induced during MI training, it sufficiently enhances both MI skills and BCI performance. However, inducing SoE prior to MI training, as done in our study, does not yield significant positive outcomes in terms of ERD modulation. These observations underscore the importance of exploring various facets of VR experiences, including timing, duration, and specific characteristics of embodiment, to further enhance MI training outcomes and BCI performance. Doing so will allow us to fully leverage the therapeutic potential of VR in neurorehabilitation.
5 Limitations and future work
Our study had several limitations related to our sample and experimental design. Primarily, our relatively small sample size of only 13 participants per group limits both the statistical power of our findings and their generalizability. Our future aim is to improve the statistical power by increasing the sample size and in doing so, we aim to delve into Linear Mixed Effects (LME) modeling, recognizing its versatility as a powerful statistical tool as opposed to correlations. We anticipate that employing LME modeling will offer a more sophisticated and flexible approach by handling both mixed and random effects, make efficient use of available data by accounting for missing or unbalanced data, while allowing for the inclusion of covariates, controlling of potential confounding factors.
Moreover, our between-study design requires careful matching of groups based on multiple variables such as gender, age, BCI, and VR experience to avoid potential confounding influences. Future research could implement measures like the Kinesthetic and Visual Imagery Questionnaire (KVIQ) (Malouin et al., 2007) or conduct an initial BCI session to evenly distribute BCI proficiency levels (or pre-existing MI abilities) across groups. Alternatively, adopting a within-subjects design, where participants undergo MI training twice, once with and once without an embodiment induction phase, could eliminate these issues. In this case, a sufficient time gap, such as 1–2 weeks, between sessions should be considered to counteract potential learning effects. Furthermore, future studies should focus on establishing a robust embodiment priming phase on an online MI-BCI session.
Furthermore, in the future we are planning to use additional questionnaires for measuring the perceived sense of embodiment (Roth and Latoschik, 2020; Eubanks et al., 2021) given that current questionnaire from Peck and Gonzalez-Franco (2021) is relatively new and the proposed measures of embodiment have not been tested through time for their reliability. In terms of future work, in order to establish a robust no-embodiment condition, future studies could consider removing the avatar entirely from the VR scene or replacing it with non-humanoid entities. Notably, the former approach could ensure total no-embodiment, while the latter would allow the administration of an embodiment questionnaire to explore possible correlations with MI skills. Finally, certain areas could be further explored. The 5-min embodiment phase in our study may have been too short to induce a robust sense of embodiment and influence subsequent MI skills. Drawing from the work of Kocur et al. (2020), future studies could investigate whether a longer embodiment phase, might influence MI-BCI performance. Most importantly, to unambiguously clarify the influence of the embodiment priming phase on MI skills, our experiment would benefit from replication with a more comprehensive no-embodiment condition.
6 Conclusion
In conclusion, our study provides insights into the role of embodiment in VR-based MI-BCIs. Though our 5-min embodiment phase effectively heightened the SoE in the embodied group, as evidenced by subjective measures, both groups demonstrated similar MI-induced ERD patterns and offline BCI accuracy. This suggests that the induction of embodiment prior to MI training may not significantly influence the training outcomes. Instead, it appears that the integration of embodied VR feedback during the MI training itself may be enough to induce appropriate ERD. These findings underscore the necessity of understanding the role of timing and context of embodiment in shaping MI skills. Future research might consider how manipulating different aspects of VR experiences, such as the duration and intensity of embodiment or the design of the VR feedback, could optimize MI training outcomes. This would further leverage the therapeutic potential of VR in neurorehabilitation therapies. Finally, this study resulted into the production of more than 78 publicly available labeled MI EEG datasets during embodiment in VR, including EMG and hand temperature (Vagaja and Vourvopoulos, 2023).
Data availability statement
The datasets presented in this study can be found in online repositories. The names of the repository/repositories and accession number(s) can be found below: https://doi.org/10.5281/zenodo.8086086.
Ethics statement
The studies involving humans were approved by Ethics Committee of CHULN and CAML (Faculty of Medicine, University of Lisbon). The studies were conducted in accordance with the local legislation and institutional requirements. The participants provided their written informed consent to participate in this study. Written informed consent was obtained from the individual(s) for the publication of any potentially identifiable images or data included in this article.
Author contributions
KV: Conceptualization, Data curation, Formal Analysis, Investigation, Methodology, Software, Visualization, Writing–original draft, Writing–review and editing. KL-H: Methodology, Supervision, Writing–review and editing. AV: Conceptualization, Funding acquisition, Project administration, Resources, Supervision, Validation, Writing–review and editing.
Funding
The author(s) declare financial support was received for the research, authorship, and/or publication of this article. This research is funded by the Fundação para a Ciência e Tecnologia (FCT) through CEECIND/01073/2018, the LARSyS–FCT Project (UIDB/50009/2020), and the NOISyS project (2022.02283.PTDC).
Acknowledgments
We would like to thank all the volunteers that participated in this study, the Evolutionary Systems and Biomedical Engineering Lab (LaSEEB) of the Institute for Systems and Robotics (ISR-Lisboa) for the availability of the experimentation space. Finally, we would like to acknowledge the support of the Erasmus + EU program, and the Portuguese Foundation for Science and Technology (FCT).
Conflict of interest
The authors declare that the research was conducted in the absence of any commercial or financial relationships that could be construed as a potential conflict of interest.
The author(s) declared that they were an editorial board member of Frontiers, at the time of submission. This had no impact on the peer review process and the final decision.
Publisher’s note
All claims expressed in this article are solely those of the authors and do not necessarily represent those of their affiliated organizations, or those of the publisher, the editors and the reviewers. Any product that may be evaluated in this article, or claim that may be made by its manufacturer, is not guaranteed or endorsed by the publisher.
Footnotes
1https://github.com/kvagaja/Virtual-Embodiment-and-Motor-Imagery-BCIs
References
Alimardani, M., Nishio, S., and Ishiguro, H. (2014). Effect of biased feedback on motor imagery learning in bci-teleoperation system. Front. Syst. Neurosci. 8, 52. doi:10.3389/fnsys.2014.00052
Alimardani, M., von Groll, V. G., Leeuwis, N., Rimbert, S., Roc, A., Pillette, L., et al. (2023). “Does gender matter in motor imagery bcis?,” in 10th international BCI meeting.
Allison, B., and Neuper, C. (2010). “Could anyone use a bci?,” in Brain-computer interfaces: human-computer interaction series, 35–54. doi:10.1007/978-1-84996-272-8_3
Amini Gougeh, R., and Falk, T. H. (2023). Enhancing motor imagery detection efficacy using multisensory virtual reality priming. Front. Neuroergonomics 4, 1080200. doi:10.3389/fnrgo.2023.1080200
Ang, K. K., Guan, C., Chua, K. S. G., Ang, B. T., Kuah, C. W. K., Wang, C., et al. (2011). A large clinical study on the ability of stroke patients to use an eeg-based motor imagery brain-computer interface. Clin. EEG Neurosci. 42, 253–258. doi:10.1177/155005941104200411
Beaudoin, M., Barra, J., Dupraz, L., Mollier-Sabet, P., and Guerraz, M. (2020). The impact of embodying an “elderly” body avatar on motor imagery. Exp. Brain Res. 238, 1467–1478. doi:10.1007/s00221-020-05828-5
Benjamini, Y., and Hochberg, Y. (1995). Controlling the false discovery rate: a practical and powerful approach to multiple testing. J. R. Stat. Soc. Ser. B Methodol. 57, 289–300. doi:10.1111/j.2517-6161.1995.tb02031.x
Botvinick, M., and Cohen, J. (1998). Rubber hands ‘feel’ touch that eyes see. Nature 391, 756. doi:10.1038/35784
Braun, N., Emkes, R., Thorne, J. D., and Debener, S. (2016). Embodied neurofeedback with an anthropomorphic robotic hand. Sci. Rep. 6, 37696. doi:10.1038/srep37696
Choi, J. W., Huh, S., and Jo, S. (2020a). Improving performance in motor imagery bci-based control applications via virtually embodied feedback. Comput. Biol. Med. 127, 104079. doi:10.1016/j.compbiomed.2020.104079
Choi, J. W., Kim, B. H., Huh, S., and Jo, S. (2020b). Observing actions through immersive virtual reality enhances motor imagery training. IEEE Trans. Neural Syst. Rehabilitation Eng. 28, 1614–1622. doi:10.1109/TNSRE.2020.2998123
Cronbach, L. J. (1951). Coefficient alpha and the internal structure of tests. psychometrika 16, 297–334. doi:10.1007/bf02310555
Delorme, A., and Makeig, S. (2004). Eeglab: an open source toolbox for analysis of single-trial eeg dynamics including independent component analysis. J. Neurosci. Methods 134, 9–21. doi:10.1016/j.jneumeth.2003.10.009
Doyle, L. M. F., Yarrow, K., and Brown, P. (2005). Lateralization of event-related beta desynchronization in the eeg during pre-cued reaction time tasks. Clin. Neurophysiol. 116, 1879–1888. doi:10.1016/j.clinph.2005.03.017
Du, B., Yue, K., Hu, H., and Liu, Y. (2021). A paradigm to enhance motor imagery through immersive virtual reality with visuo-tactile stimulus. Melbourne, Australia: IEEE, 703–708. doi:10.1109/SMC52423.2021.9658878
Eubanks, J. C., Moore, A. G., Fishwick, P. A., and McMahan, R. P. (2021). A preliminary embodiment short questionnaire. Front. Virtual Real. 2, 647896. doi:10.3389/frvir.2021.647896
Evans, N., and Blanke, O. (2013). Shared electrophysiology mechanisms of body ownership and motor imagery. NeuroImage 64, 216–228. doi:10.1016/j.neuroimage.2012.09.027
Isaac, A. R., and Marks, D. F. (1994). Individual differences in mental imagery experience: developmental changes and specialization. Br. J. Psychol. 85 (4), 479–500. (London, England: 1953). doi:10.1111/j.2044-8295.1994.tb02536.x
Jeunet, C., N’Kaoua, B., Subramanian, S., Hachet, M., and Lotte, F. (2015). Predicting mental imagery-based bci performance from personality, cognitive profile and neurophysiological patterns. PloS one 10, e0143962. doi:10.1371/journal.pone.0143962
Juliano, J. M., Spicer, R. P., Vourvopoulos, A., Lefebvre, S., Jann, K., Ard, T., et al. (2020). Embodiment is related to better performance on a brain–computer interface in immersive virtual reality: a pilot study. Sensors 20, 1204. doi:10.3390/s20041204
Khan, M. A., Das, R., Iversen, H. K., and Puthusserypady, S. (2020). Review on motor imagery based bci systems for upper limb post-stroke neurorehabilitation: from designing to application. Comput. Biol. Med. 123, 103843. doi:10.1016/j.compbiomed.2020.103843
Kilteni, K., Groten, R., and Slater, M. (2012). The sense of embodiment in virtual reality. Presence Teleoperators Virtual Environ. 21, 373–387. doi:10.1162/PRES_a_00124
Kilteni, K., Maselli, A., Kording, K. P., and Slater, M. (2015). Over my fake body: body ownership illusions for studying the multisensory basis of own-body perception. Front. Hum. Neurosci. 9, 141. doi:10.3389/fnhum.2015.00141
Kocur, M., Roth, D., and Schwind, V. (2020). Towards an investigation of embodiment time in virtual reality. Accepted: 2020-08-18T15:20:01Z publisher: Gesellschaft für Informatik e.V. doi:10.18420/muc2020-ws134-339
Lee, K. M. (2004). Presence, explicated. Commun. Theory 14, 27–50. doi:10.1111/j.1468-2885.2004.tb00302.x
Leeuwis, N., Paas, A., and Alimardani, M. (2021). Vividness of visual imagery and personality impact motor-imagery brain computer interfaces. Front. Hum. Neurosci. 15, 634748. doi:10.3389/fnhum.2021.634748
Lotte, F. (2014). “A tutorial on eeg signal-processing techniques for mental-state recognition in brain–computer interfaces,” in Guide to brain-computer music interfacing. Editors E. R. Miranda, and J. Castet (London: Springer London), 133–161. doi:10.1007/978-1-4471-6584-2_7
Lotte, F., Larrue, F., and Mühl, C. (2013). Flaws in current human training protocols for spontaneous brain-computer interfaces: lessons learned from instructional design. Front. Hum. Neurosci. 7, 568. doi:10.3389/fnhum.2013.00568
Makeig, S., Bell, A., Jung, T.-P., and Sejnowski, T. J. (1995). Independent component analysis of electroencephalographic data, 8. MIT Press.
Makransky, G., Lilleholt, L., and Aaby, A. (2017). Development and validation of the multimodal presence scale for virtual reality environments: a confirmatory factor analysis and item response theory approach. Comput. Hum. Behav. 72, 276–285. doi:10.1016/j.chb.2017.02.066
Malouin, F., Richards, C. L., Jackson, P. L., Lafleur, M. F., Durand, A., and Doyon, J. (2007). The kinesthetic and visual imagery questionnaire (kviq) for assessing motor imagery in persons with physical disabilities: a reliability and construct validity study. J. neurologic Phys. Ther. JNPT 31, 20–29. doi:10.1097/01.npt.0000260567.24122.64
Maselli, A., and Slater, M. (2014). Sliding perspectives: dissociating ownership from self-location during full body illusions in virtual reality. Front. Hum. Neurosci. 8, 693. doi:10.3389/fnhum.2014.00693
McKelvie, S. J., and Demers, E. G. (1979). Individual differences in reported visual imagery and memory performance. Br. J. Psychol. 70, 51–57. (London, England: 1953). doi:10.1111/j.2044-8295.1979.tb02142.x
Neuper, C., Scherer, R., Wriessnegger, S., and Pfurtscheller, G. (2009). Motor imagery and action observation: modulation of sensorimotor brain rhythms during mental control of a brain–computer interface. Clin. Neurophysiol. 120, 239–247. doi:10.1016/j.clinph.2008.11.015
Nierula, B., Spanlang, B., Martini, M., Borrell, M., Nikulin, V. V., and Sanchez-Vives, M. V. (2021). Agency and responsibility over virtual movements controlled through different paradigms of brain-computer interface. J. Physiology 599, 2419–2434. doi:10.1113/JP278167
Oliva, R., Beacco, A., Navarro, X., and Slater, M. (2022). Quickvr: a standard library for virtual embodiment in unity. Front. Virtual Real. 3. doi:10.3389/frvir.2022.937191
Peck, T. C., and Gonzalez-Franco, M. (2021). Avatar embodiment. a standardized questionnaire. Front. Virtual Real. 1. doi:10.3389/frvir.2020.575943
Peck, T. C., Seinfeld, S., Aglioti, S. M., and Slater, M. (2013). Putting yourself in the skin of a black avatar reduces implicit racial bias. Conscious. Cognition 22, 779–787. doi:10.1016/j.concog.2013.04.016
Penaloza, C. I., Alimardani, M., and Nishio, S. (2018). Android feedback-based training modulates sensorimotor rhythms during motor imagery. IEEE Trans. Neural Syst. Rehabilitation Eng. 26, 666–674. doi:10.1109/TNSRE.2018.2792481
Perez-Marcos, D., Slater, M., and Sanchez-Vives, M. V. (2009). Inducing a virtual hand ownership illusion through a brain–computer interface. NeuroReport 20, 589–594. doi:10.1097/WNR.0b013e32832a0a2a
Petkova, V. I., and Ehrsson, H. H. (2008). If i were you: perceptual illusion of body swapping. PLOS ONE 3, e3832. doi:10.1371/journal.pone.0003832
Pfurtscheller, G., and Aranibar, A. (1979). Evaluation of event-related desynchronization (erd) preceding and following voluntary self-paced movement. Electroencephalogr. Clin. Neurophysiology 46, 138–146. doi:10.1016/0013-4694(79)90063-4
Pfurtscheller, G., and Lopes da Silva, F. H. (1999). Event-related eeg/meg synchronization and desynchronization: basic principles. Clin. Neurophysiol. 110, 1842–1857. doi:10.1016/S1388-2457(99)00141-8
Pfurtscheller, G., Müller-Putz, G. R., Scherer, R., and Neuper, C. (2008). Rehabilitation with brain-computer interface systems. Computer 41, 58–65. doi:10.1109/mc.2008.432
Pfurtscheller, G., and Neuper, C. (2001). Motor imagery and direct brain-computer communication. Proc. IEEE 89, 1123–1134. doi:10.1109/5.939829
Pichiorri, F., Morone, G., Petti, M., Toppi, J., Pisotta, I., Molinari, M., et al. (2015). Brain–computer interface boosts motor imagery practice during stroke recovery. Ann. Neurology 77, 851–865. doi:10.1002/ana.24390
Pion-Tonachini, L., Kreutz-Delgado, K., and Makeig, S. (2019). Iclabel: an automated electroencephalographic independent component classifier, dataset, and website. NeuroImage 198, 181–197. doi:10.1016/j.neuroimage.2019.05.026
Ramoser, H., Muller-Gerking, J., and Pfurtscheller, G. (2000). Optimal spatial filtering of single trial eeg during imagined hand movement. IEEE Trans. Rehabilitation Eng. 8, 441–446. doi:10.1109/86.895946
R Core Team (2018). R: a language and environment for statistical computing. Vienna, Austria: R Foundation for Statistical Computing.
Roth, D., and Latoschik, M. E. (2020). Construction of the virtual embodiment questionnaire (veq). IEEE Trans. Vis. Comput. Graph. 26, 3546–3556. doi:10.1109/tvcg.2020.3023603
Skola, F., and Liarokapis, F. (2018). Embodied vr environment facilitates motor imagery brain–computer interface training. Comput. Graph. 75, 59–71. doi:10.1016/j.cag.2018.05.024
Skola, F., Tinková, S., and Liarokapis, F. (2019). Progressive training for motor imagery brain-computer interfaces using gamification and virtual reality embodiment. Front. Hum. Neurosci. 13, 329. doi:10.3389/fnhum.2019.00329
Stoykov, M. E., and Madhavan, S. (2015). Motor priming in neurorehabilitation. J. neurologic Phys. Ther. JNPT 39, 33–42. doi:10.1097/npt.0000000000000065
Vagaja, K., and Vourvopoulos, A. (2023). Electrophysiological signals of embodiment and mi-bci training in vr. doi:10.5281/zenodo.8086086
Vourvopoulos, A., and Bermúdez i Badia, S. (2016). Motor priming in virtual reality can augment motor-imagery training efficacy in restorative brain-computer interaction: a within-subject analysis. J. NeuroEngineering Rehabilitation 13, 69. doi:10.1186/s12984-016-0173-2
Vourvopoulos, A., Blanco-Mora, D. A., Aldridge, A., Jorge, C., Figueiredo, P., and Badia, S. B. I. (2022). Enhancing motor-imagery brain-computer interface training with embodied virtual reality: a pilot study with older adults. Rome, Italy: IEEE, 157–162. doi:10.1109/MetroXRAINE54828.2022.9967664
Vourvopoulos, A., Ferreira, A., and Badia, i. S. B. (2016). Neurow: an immersive vr environment for motor-imagery training with the use of brain-computer interfaces and vibrotactile feedback. Lisbon, Portugal: SCITEPRESS - Science and Technology Publications, 43–53. doi:10.5220/0005939400430053
Vourvopoulos, A., Jorge, C., Abreu, R., Figueiredo, P., Fernandes, J.-C., and Bermúdez I Badia, S. (2019). Efficacy and brain imaging correlates of an immersive motor imagery bci-driven vr system for upper limb motor rehabilitation: a clinical case report. Front. Hum. Neurosci. 13, 244. doi:10.3389/fnhum.2019.00244
Wolf, E., Merdan, N., Dolinger, N., Mal, D., Wienrich, C., Botsch, M., et al. (2021). The embodiment of photorealistic avatars influences female body weight perception in virtual reality. Lisboa, Portugal: IEEE, 65–74. doi:10.1109/VR50410.2021.00027
Wolpaw, J. R., Birbaumer, N., McFarland, D. J., Pfurtscheller, G., and Vaughan, T. M. (2002). Brain–computer interfaces for communication and control. Clin. Neurophysiol. 113, 767–791. doi:10.1016/S1388-2457(02)00057-3
Wriessnegger, S. C., Müller-Putz, G. R., Brunner, C., and Sburlea, A. I. (2020). Inter-and intra-individual variability in brain oscillations during sports motor imagery. Front. Hum. Neurosci. 14, 576241. doi:10.3389/fnhum.2020.576241
Keywords: embodiment, priming, virtual hand illusion, virtual reality, EEG, motor imagery, event-related desynchronization, brain-computer interfaces
Citation: Vagaja K, Linkenkaer-Hansen K and Vourvopoulos A (2024) Avatar embodiment prior to motor imagery training in VR does not affect the induced event-related desynchronization: a pilot study. Front. Virtual Real. 4:1265010. doi: 10.3389/frvir.2023.1265010
Received: 21 July 2023; Accepted: 04 December 2023;
Published: 08 January 2024.
Edited by:
Giacinto Barresi, Italian Institute of Technology (IIT), ItalyReviewed by:
Maryam Alimardani, Tilburg University, NetherlandsSelina C. Wriessnegger, Graz University of Technology, Austria
Copyright © 2024 Vagaja, Linkenkaer-Hansen and Vourvopoulos. This is an open-access article distributed under the terms of the Creative Commons Attribution License (CC BY). The use, distribution or reproduction in other forums is permitted, provided the original author(s) and the copyright owner(s) are credited and that the original publication in this journal is cited, in accordance with accepted academic practice. No use, distribution or reproduction is permitted which does not comply with these terms.
*Correspondence: Athanasios Vourvopoulos, athanasios.vourvopoulos@tecnico.ulisboa.pt