- 1Cell and Molecular Biology Laboratory, Center for Nuclear Energy in Agriculture, University of São Paulo (USP), Piracicaba, Brazil
- 2Princeton Institute for International and Regional Studies (PIIRS), Princeton University, Princeton, NJ, United States
- 3Luiz de Queiroz College of Agriculture, University of São Paulo (USP), Piracicaba, Brazil
- 4Graduate Program in Biodiversity and Biotechnology, Federal University of Tocantins, Palmas, Brazil
- 5São Paulo's Agency for Agribusiness Technology, Piracicaba, Brazil
- 6Department of Microbial Ecology, Netherlands Institute of Ecology (NIOO-KNAW), Wageningen, Netherlands
- 7Ecology and Biodiversity Group, Utrecht University, Utrecht, Netherlands
- 8Graduate Programa in Environmental Sciences, University Brazil, Fernandópolis, Brazil
A common agricultural practice of combining organic fertilizer vinasse (a liquid residue from sugarcane ethanol production) with mineral nitrogen (N) fertilizer promotes N losses such as greenhouse gas emissions due to the effects of physicochemical changes in soil on the microbiota inhabiting this environment. In this study, we applied microarray GeoChip v.5.0M technology to obtain a better insight into the prokaryotic communities and identify and quantify the N functional gene families associated with the N processes in sugarcane soils without N fertilizer (N0), with urea at 60 kg ha−1 (N60), and with vinasse combined with urea (NV). Soil samples were collected at 7 (T7) and 150 (T150) days after N application, corresponding to maximum and minimum nitrous oxide (N2O) emissions, respectively, for molecular and physicochemical analysis. Additionally, the metagenomes of these DNA samples, previously deposited in the MG-RAST server, were accessed to investigate the functions and taxonomic groups associated with selected gene families. The results revealed that 87% of the select gene families were significantly responsive to the fertilizer combined treatment (NV) in the 7 days after the application. The most responsive genes and processes were nitrification [with the amoA gene from ammonia-oxidizing Bacteria (AOB) and Archaea (AOA) and hao from Bacteria], ammonification (with gdh and ureC genes from Bacteria and Archaea), and denitrification (with p450nor from Eukarya). The AOA, Nitrosopumilus, and AOB, Nitrosomonas, were the groups with the greatest functions associated with nitrification, as well as a pathogenic Mycobacterium, with denitrification. The results also revealed that under N fertilizers and decreased O2 in soil, the increases in K and P nutrients can promote the growth of the halophile Archaea Natronomonas and the Bacteria Anaeromyxobacter, which can reduce N2O. In conclusion, this typical agricultural fertilization management may favor functional genes and archaeal and bacterial groups associated with N processes that have the potential to reduce environmental damage in tropical sugarcane soils.
Introduction
Vinasse is a byproduct of ethanol from the sugarcane industry, generated during the distillation process (1, 2), with a production rate of 10–18 L while preparing each liter of ethanol (3). Its chemical composition varies depending on the mill plant used for the production of ethanol and the distillation process. In general, sugarcane vinasse is composed of water (93%) and organic solids and minerals (7%), such as potassium (K), calcium (Ca), and magnesium (Mg) (4, 5). This residue from ethanol production has high levels of organic matter and low C:N ratio (6) and low contents of nitrogen (N) (0.97–4.75 g L−1) and phosphorus (P) (1–190 mg L−1). The main non-aqueous components in vinasse are organic matter, such as glycerol, organic acids, and yeasts (5), and the volatile solids are present at high levels (6). When applied to the soil, the amount of organic material, nitrate (), ammonium (), potassium (K+), calcium (Ca+2), magnesium (Mg+2), sodium (Na+), and metals increase (5, 6). An acidic pH (3.9–5.1), high chemical oxygen demand (COD) (50.000–95.000 mg L−1), and biological oxygen demand (DBO) (18.900–78.300 mg L−1) also occur (6). If vinasse is poured into the environment in an unplanned way, it can cause pollution in the soil surface and underground due to the excessive accumulation of salts, which results in a toxic condition (6).
Vinasse use as a liquid fertilizer can be applied with mineral N sources in the sugarcane crops to minimize the ecological problem of its residue disposal into the environment (7, 8). However, even though this practice increases sugarcane productivity, it also causes physical, chemical, and biochemical changes in the soil environment (9, 10). There is a lack of information about the impacts of this agricultural management on the structure and functionality of soil microbial communities in tropical soils, but it is known that their changes are often correlated with soil chemical factors (11–16). This has been demonstrated for Bacteria (17–19) but not for Archaea.
Studies have shown that the addition of plant-derived organic residues commonly used in the fallow season, such as vinasse or sugarcane straw (20), increase the emissions of nitrous oxide (N2O), a greenhouse gas (GHG) (20, 21), especially when the vinasse is combined with N addition (20, 21). Lourenço et al. (21) concluded that the amoA gene from ammonia-oxidizing Bacteria (AOB), active in the nitrification process, was the most functional gene related to N2O emissions in sugarcane crops under vinasse fertilization. Regarding the ammonia-oxidizing Archaea (AOA), which also have amoA (22), some studies have demonstrated their relevant role in the N biogeochemical cycle (23, 24). However, experts warn about the imminent need to associate genomic data from molecular methods in microbial ecology with biochemical data to close the “gap” between physiological mechanisms by AOA and N metabolism (22).
Cloutier et al. (25) described the parallel quantification of N functional genes as one emerging technology that could be combined with high-throughput sequencing of microbial DNA to study N metabolism in soil. The microarray technology GeoChip v.5.0M (Agilent Technologies Inc., Santa Clara, CA, United States) has become a powerful and high-performance tool to monitor environmental processes with high-sensitivity detection of microbial functional genes (26–29). The chip contains 167,044 distinct probes belonging to different categories of genes involved in carbon (C) and N (ammonification, nitrification, fixation, among others) metabolisms (28). The probes were originated from gene sequences of microorganisms and correspond to a unique sequence of a gene family (Ex: aceA) and encode the same class of proteins. Several studies have concluded that this technique can be mainly used to link data from microbial communities to the processes and functions to which they are related (30) in a broad sense. Although recent studies have been able to obtain relevant information about the N metabolism genes in soil microbiota with molecular techniques (14, 16, 21, 31), GeoChip v.5.0M technology offers the advantage of analyzing many functional genes at once, providing a broader view of the functional response of the microbiota under a given condition (32).
In this sense, we would like to obtain a better insight into the archaeal and bacterial communities and microbially mediated environmental processes that play important role in the N cycle in sugarcane soil amended with vinasse and N mineral as fertilizers. We hypothesized that vinasse use combined with N fertilizer affects functional gene families and bacterial and archaeal taxonomic groups inhabiting sugarcane cultivated soils. Since the microbial N metabolism in soils is associated with N losses, such as GHGs (20), better understanding the functionality of Archaea and Bacteria can help mitigate this economic and environmental problems.
Materials and Methods
Experimental Design, Soil Treatments, and Soil Sampling
This study was carried out in greenhouse conditions with sugarcane seedlings grown for 150 days between April and December 2013 in nine 100-L pots, as described by Navarrete et al. (19). The sugarcane seedlings of Saccharum spp. CTC-02 were obtained from in vitro fertilization and tissue culture. The red clayey podzolic soil was obtained from the 0–20 cm layer in the Areão farm, belonging to the Luiz de Queiroz School of Agriculture, University of São Paulo (ESALQ-USP), in Piracicaba, São Paulo, Brazil (22° 42′ 30″ S e 47° 38′ 00″ W). All the pots received, in addition to the sugarcane seedlings, 90 kg of soil, and basic fertilization [150 kg ha−1 of triple P2O5 superphosphate and 80 kg ha−1 of potassium chloride (KCl)]. Three treatments were established containing three replicates each: the control treatment (N0) received only the basic fertilization as described above, without a nitrogen fertilizer; the second treatment (N60) received, in addition to the basic fertilization, inorganic (urea) nitrogen fertilizer (at a dose of 60 kg ha−1), which was immediately mixed with the soil to avoid losses due to volatilization; the third treatment (NV) received urea (60 kg ha−1) supplemented with vinasse [at a dose of 0.06 L kg−1 (120 m3 ha−1)]. The K content of the KCl added in the NV treatment was calculated, excluding the amount already present in the vinasse (defined above), to introduce into the system the same amount of this nutrient in all the mesocosms. Water was added in the same volume as the vinasse, and in the treatments that did not receive vinasse, the soil moisture content in the mesocosms was maintained at 80% of the field capacity throughout the experiment using a humidity sensor (Extech MO750, Nashua, NH, United States).
Ten sugarcane plants were grown in each mesocosm, and only four sugarcane plants were left in each mesocosm until the end of the experiment. Sugarcane plants were removed in pairs from each mesocosm at 50, 90, and 150 days after the first soil fertilization to maintain the root system under the limited capacity of the mesocosm.
The soil samples were collected at 7 and 150 days after the addition of the nitrogen fertilizer and vinasse to perform molecular analyses and for the determination of chemical factors. These collection periods were determined according to the maximum and minimum carbon dioxide (CO2) and N2O emissions from the soil, as shown in Navarrete et al. (19). Three bulk soil samples (~100 g in each one) were collected from the topsoil (0–10 cm) in equidistant positions within an equilateral triangle (radius equivalent to 1/3 of the circular surface of the mesocosm) and pooled together, forming only one sample with 300 g. For molecular analysis, soil subsamples with 250 mg were separated from the sample with 300 g obtained from each mesocosm. The subsamples were stored at −20°C until processing within 72 h.
Analysis of Soil Chemical Factors
Several soil chemical factors were analyzed according to the methodology used by Empresa Brasileira de Pesquisa Agropecuária (33). After the soil samples were sieved, to make a homogeneous mixture, a LECO CN 2000 elementary analyzer (Perkin Elmer, Waltham, MA, United States) was used to determine the C and total N by dry combustion. The pH was determined using a calcium chloride (CaCl2) 0.01 M solution. The content of B (boron) was obtained by extraction with hot water, while Al (aluminum), Ca, and Mg were extracted with KCl 1 M solution. After extraction, Ca and Mg were determined by atomic absorption spectrometry, and Al by acid-base titration. P and K were extracted with ion exchange resin, but P was determined using colorimetry and K with atomic emission spectrometry.
To calculate the sum of exchangeable bases (SB), the soil contents of Ca, Mg, and K were combined. The values of Ca, Mg, K, Al, and H were used to calculate the cation exchange capacity (CEC). Base saturation (BS%) was obtained from the ratio between SB and CEC. Finally, for the potential acidity (H + Al), the equation based on the Shoemaker-McLean-Pratt (SMP) buffer method was used.
DNA Extraction and Microarray Probes Analysis
Using the Power Lyzer Power Soil kit (MoBio Laboratories, Carlsbad, CA, United States), the genomic DNA was extracted from the subsamples of each mesocosm. After this procedure, the isolated DNA from the subsamples of each mesocosm was mixed, totaling 18 samples (3 treatments × 3 replicates × 2 collection periods). The quality and quantity of each one of the 18 DNA samples were evaluated by spectrophotometry in a NanoDrop device (NanoDrop RND-1000 NanoDrop Technologies, Inc., Wilmington, DE, United States). DNA was stored at −20°C until use.
To prepare the DNA for GeoChip v.5.0M analysis, each sample was purified and marked with the fluorescent dye Cy-3 (34). The DNA (600 ng each) was mixed with random primers (300 ng mL−1), denatured at 99.9°C for 5 min, and immediately cooled on ice. A solution containing 5 mM of dAGC-TP, 2.5 mM of dTTP, 40 U of the Klenow fragment, and 25 nM of Cy-3 dUTP was added to the denatured DNA, and the reaction volume was adjusted to 50 mL with distilled H2O. The labeling solution was incubated at 37°C for 6 h, followed by 3 min at 95°C. The labeled DNA was purified with the QIAquick Kit (Qiagen, Valencia, CA, United States), and dye incorporation was confirmed with a NanoDrop spectrophotometer (NanoDropR ND-1000 Technologies, Inc., Wilmington, DE, United States) using the absorption spectra of the standard solution for Cy-3. DNA samples were dried under vacuum and stored at −20°C until hybridization.
The tagged DNA was resuspended in DNase/RNase-free distilled water and subsequently mixed with the solution for hybridization of the GeoChip v.5.0M probes, according to the procedure described in de Chaves et al. (35). After hybridization, the slides containing the GeoChip microarrays were washed with a buffer solution (Agilent Wash Buffers 1 and 2—Agilent Technologies Inc., Santa Clara, CA, USA) following the manufacturer's protocol. Using a NimbleGen MS200 scanner (Roche NimbleGen, Madison, WI, United States), the microarrays were digitalized, and the image data were extracted using Agilent Feature Extraction v. 2.6 (Agilent Technologies Inc., Santa Clara, CA, United States).
To determine the luminous intensity resulting from the hybridization of the genes marked with the corresponding probes, the software Agilent Feature Extraction v.11.5 was used (Agilent Technologies Inc., Santa Clara, CA, United States). The data were submitted to the Microarray Data Manager, available from the Institute for Environmental Genomics (website http://www.ou.edu/content/ieg/tools/data-analysis-pipeline.html), and were analyzed according to the parameters described in de Chaves et al. (35).
Shotgun Sequencing of Total Soil Genomic DNA and Data Processing
Eighteen DNA sequencing libraries were prepared using the Illumina Nextera sample preparation kit (Illumina, San Diego, CA, USA) according to the manufacturer's instructions. Equal concentrations of libraries were loaded on the MiSeq Reagent v.2 sequencing reagent kit (Illumina, San Diego, CA, USA). The equipment used for shotgun metagenomic sequencing was a MiSeq Personal Sequencing System (Illumina, San Diego, CA, USA), operated in the Rapid Run Mode to generate 2 × 250 base pairs (bp) paired-end reads. In summary, the sequencing resulted in an average of 105.5 MB of sequences per sample.
Regarding data processing, first, paired-end reads were merged using FLASH v.1.2.5 (36) to produce consensus sequences. Quality control of the consensus sequences was performed using the Phred quality score (20) to each base call (37) with an executable default script in SeqClean (http://www.bioinformatics.org/), and low-quality bases were removed. Shotgun sequencing of the soil DNA samples resulted in ~547,415 merged reads and 375,429 unmerged reads after the quality-based filtering procedure. Merged and unmerged trimmed sequences were concatenated into a single file for the metagenomic dataset, which is available on the Metagenomics Rapid Annotation (MG-RAST) server (38) under the “Metagenome of sugarcane soil—CENA/USP” project with accession numbers 4582104.3–4582153.3.
Selection of Gene Families, Functions, and Groups Responsive to Treatments
To select the gene families responsive to the treatments and their associated functions, the values obtained from the hybridization of the genomic DNA marked with the microarray probes corresponding to the category “N metabolism” were submitted to statistical analysis of variance (ANOVA, followed by Tukey/LSD, p < 0.05) using the program Sisvar v.5.6 (39). Gene families that showed statistically significant changes in absolute abundance in relation to the treatment N0 were used as references for the functional and taxonomic investigation analysis in the MG-RAST server.
The curated databases RefSeq (40) and Subsystems (SEED base annotation) (41) were accessed for taxonomic and functional annotation, respectively. Functions investigated were selected according to the UniProt (42) (https://www.uniprot.org/uniprot/P00370) and its previous description in the literature: (i) each metagenome was analyzed in the RefSeq (40) database to determine the abundance of each group; and (ii) the sequences from Archaea or Bacteria were subjected to a functional filter, previously selected in the Subsystems.
Statistical Correlation Analyses
To verify which soil factors can affect the abundance of the N metabolism gene family, we performed a Spearman correlation using Sigma Plot software v.12.5 SYSTAT (Software Inc., CA, United States) and assumed a p < 0.05 threshold level for acceptance between two datasets: soil chemical parameters and each previously significant selected gene family. All dataset combinations (positive and negative) were used for the construction of network to illustrate the relationships between soil factors and gene families from N metabolism in soil with vinasse and urea fertilization. The constructed networks were analyzed using Gephi software version 0.9.2 (43), which has a graphical interface. The nodes in the network represent the soil factors and the selected gene families, and the edges represent significant (p < 0.05) positive or negative correlations between the nodes. Network graphs were based on a set of statistical measures and parameters, such as the numbers of nodes and edges, modularity, clusterization coefficient, degree and betweenness centrality distribution, training algorithm parameters, and activation functions (44, 45).
Spearman rank correlation coefficients were also calculated to generate a heatmap correlation graph to illustrate the connection degree of these datasets. This analysis was performed in the R environment v.4.0.2 with the “multtest” package (46): (i) the absolute abundance of the selected gene families among the GeoChip hybridization signals; and (ii) the soil physicochemical factors.
Results
Analysis of Soil Chemical Factors
The majority (~84%) of the soil chemical factors analyzed were significantly responsive (p < 0.05) to the addition of vinasse, regardless of the period of collection of the soil samples (T7 or T150) (Table 1). However, in the T150 period, an increase of 10% in the contents of these soil factors was observed. S and K (both with ~90% of increase in NV compared to N0) were highlighted, presenting the highest levels. Zn and Mn showed significant increases in the T7 period (25 and 65%, respectively), while Mg and SB increased significantly only in T150 (23 and 30%). C and N showed significant increases in both sample collection periods, though only in the treatment that received vinasse (15 and 5%, respectively, concerning treatment N0). However, these soil factors showed no significant differences between the two periods (Table 1). Corresponding to the increases in C and N in the NV treatment, the C:N ratio showed a significant reduction of 18% in this treatment compared with the N0 treatment, in both periods. Still, considering the soil factors responsive to the addition of vinasse, the pH increased by ~6% in the two periods in relation to the control treatment.
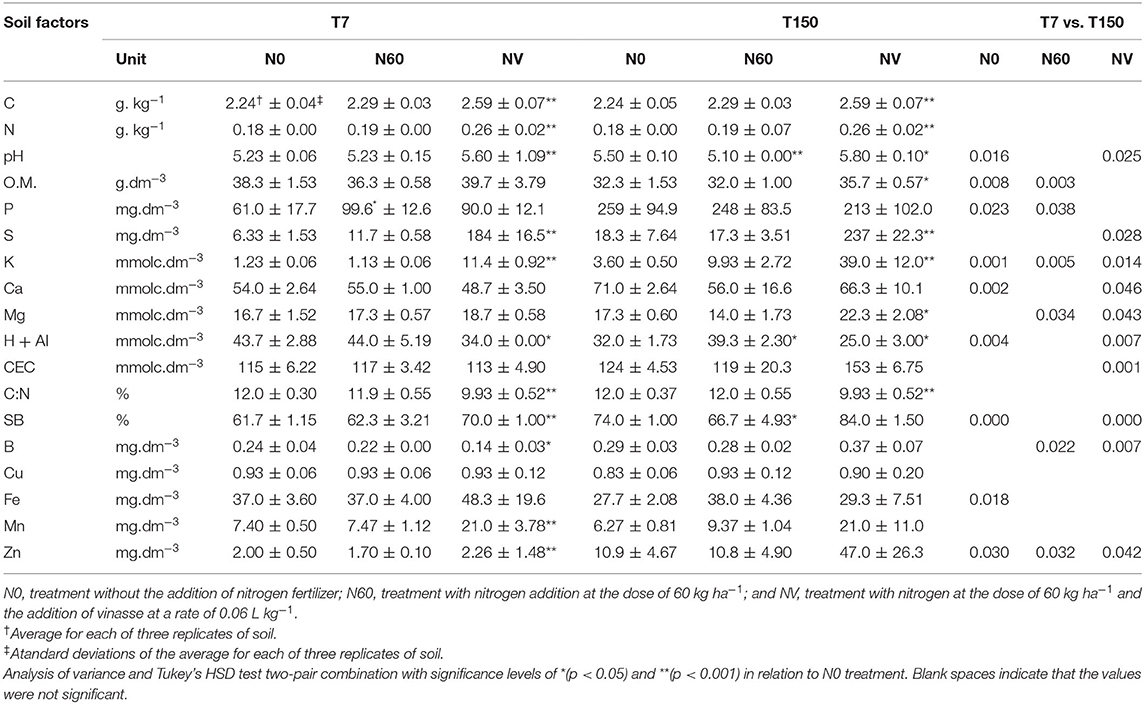
Table 1. Chemical compounds present in the soil treatments without nitrogen fertilization (N0), with nitrogen fertilization (N60), and with nitrogen fertilization combined with vinasse (NV) collected at seven (T7) and one hundred fifty (T150) days after the start of the experiment.
When only urea was added to the soil (N60 treatment), the pH decreased significantly (8% compared to the N0 control treatment), but only in the T150 period. P was the only soil factor that increased significantly in the N60 treatment (30% compared to N0), but only in T7. Considering the values of the soil factors between the two periods (T7 and T150), a smaller number of factors in the N60 treatment showed significant differences in their levels in relation to those observed in the NV treatment (6 in N60 and 10 in NV). In NV, Zn, and K showed the highest rates of increase, with 95 and 70%, respectively.
Selection of Gene Families Responsive to Treatments by GeoChip v.5.0M and Their Binning to Archaea and Bacteria
GeoChip v.5.0M has 30 gene families within the nitrogen category corresponding to the following processes or functions associated with the N metabolism: nitrification, denitrification, ammonification, anammox, dissimilatory N reduction, assimilatory N, assimilatory N reduction, and respiration. In all gene families available in this version of GeoChip v.5.0M, there were hybridizations between the corresponding probes and the DNA samples from the treatments.
For each replicate sample, the values resulting from the quantification of the hybridization between each gene from the N metabolism and the corresponding probes were added. These values were used to represent the replicates in the statistical analysis. In a total of 30 gene families in the GeoChip v.5.0M (nitrogen category), 10 (amoA, hao, gdh, ureC, hzsA, napA, nirK, nitrate/nitrite_transporter, and p450nor) (Table 2) showed significant values (p < 0.05) in the variance test. These gene families belong to the following N processes: amoA and hao to nitrification; gdh and ureC to ammonification; hzsA to anammox, napA to dissimilatory N; nirK to denitrification; nitrate and nitrite_transporter to assimilatory N; and p450nor to respiration in denitrification. In 90% of the gene families selected through statistical analysis, the highest number of hybridizations between the probes and the corresponding genes occurred in the T7 period. The highest number of hybridizations occurred at T150 only for the hao gene and its corresponding to probes from Archaea (45% of the total available in GeoChip v.5.0M) (Table 2). The gdh gene, involved in the ammonification process, presented 6% of the total probes available from Archaea and 83% from Bacteria. ureC, napA, and nirK presented 95 to 100% from Bacteria. The hao and hzsA genes had only probes from Bacteria available in GeoChip v.5.0M, as nitrate and nitrite transporter and p450nor, with 100% from Eukarya (Table 2).
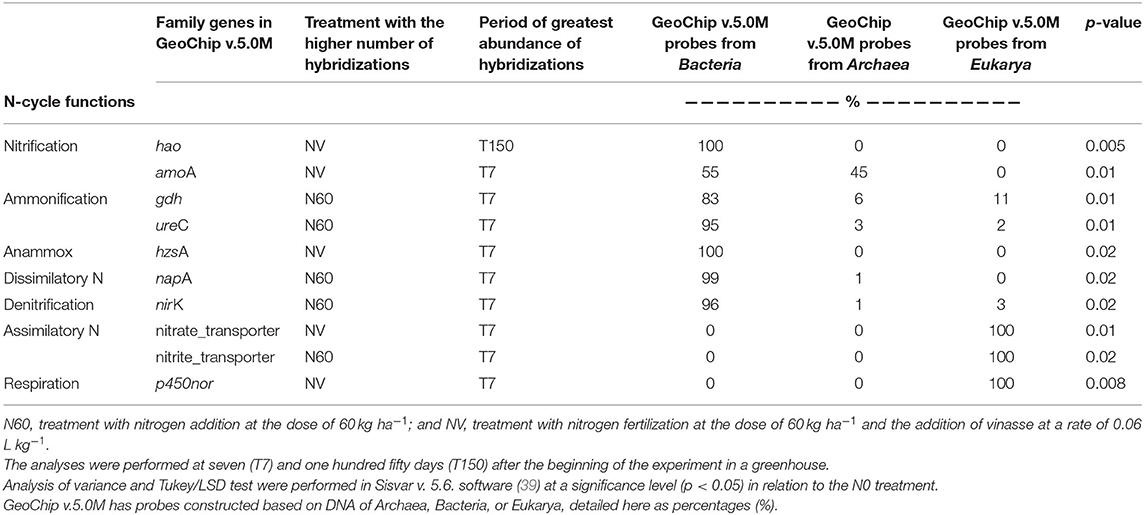
Table 2. GeoChip v.5.0M nitrogen family gene significance (p < 0.05) in the Tukey LSD test, N cycle functional correspondence, treatment (N60 or NV), and collection period (T7 or T150) with more potential occurrence and origin of probes (Bacteria, Archaea, and Eukarya).
The gdh and ureC genes, associated with the ammonification process, were more abundant in the N60 treatment and the T7 sampling period, as well as nirK (denitrification). The number of hybridizations by the p450nor gene, followed by the hao gene, presented the highest significant values in the statistical analysis (p = 0.008 and p = 0.005, respectively). Coincidentally, p450nor and hao also showed the highest hybridization values in the treatment that received vinasse.
Functional Gene Families and Archaeal and Bacterial Taxonomic Groups Associated With the N Metabolism Analyzed in the MG-RAST Server
Only three of the eight functions analyzed in MG-RAST were present in Archaea (Table 3). Ammonia monooxygenase, glutamate dehydrogenase, and urease are enzymes associated with amoA, gdh, and ureC genes (Tables 2, 3). These genes correspond to the nitrification (amoA) and ammonification (gdh and ureC) processes (Table 2). The genus Nitrosopumilus, from the phylum Thaumarchaeota, presented the greatest number of sequences of ammonia monooxygenase among Archaea in the NV treatment and T7 period (Table 3). Sulfolobus (from Crenarchaeota) presented the greatest number of sequences of glutamate dehydrogenase and Natronomonas (from Euryarchaeota) of urease, both in the T150 period. The glutamate dehydrogenase was highest in treatment NV and urease in N60. However, only the value from urease in Natronomonas was statistically significant (p < 0.05 in Tukey/LSD). Ammonia monooxygenase sequences were analyzed at domain and genus levels. From total Archaea, this function presented a 4-fold decrease between NVT7 and NV150 in numbers of sequences. From Bacteria, between NVT7 and NVT150, it increased twice.
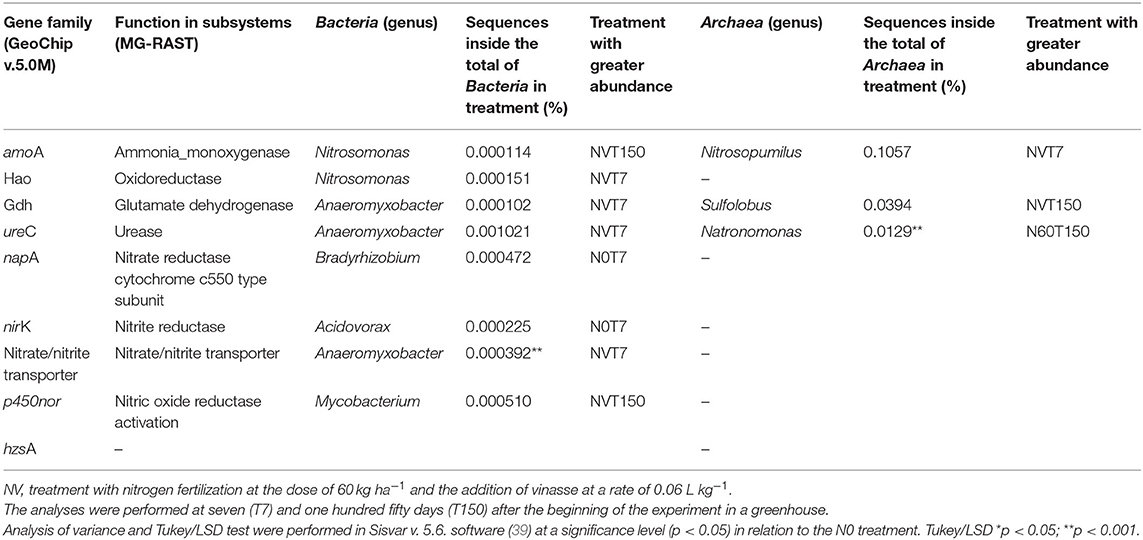
Table 3. Archaea and Bacteria frequencies of functions in metagenomes corresponding to N cycle gene families selected by GeoChip v.5.0M.
All functions investigated were found in Bacteria. Proteobacteria from the genus Anaeromyxobacter stood out because they exhibited the greatest number of sequences of glutamate dehydrogenases, urease, and nitrate/nitrite transporter. Anaeromyxobacter was the most frequent genus among Bacteria with the selected functions (40% of the total), and NVT7 included all these sequences (Table 3). The oxidoreductase enzyme, associated with the hao gene family, was found only in Bacteria, in which the treatment NVT7 had the greatest number of sequences. The abundances of functions were statistically significant for nitrate/nitrite transporter in Anaeromyxobacter (p < 0.001).
The treatment that received vinasse combined with urea, NV, presented the majority of groups with the selected functions (~70%). Conversely, N60 presented only one urease function in the Archaea Natronomonas. The gene family hzsA was not found in the subsystems by MG-RAST (Table 3).
Correlation Analyses Between Selected Gene Families and Physicochemical Soil Factors
Heatmaps and network correlation tests between GeoChip v.5.0M gene families responsive to N treatments and soil physicochemical factors (Figures 1, 2) revealed that T7 featured two times more correlations than T150, where the majority in T7 were positives (85% of the network) and in T150, negatives. The soil factor P was involved in 80% of the positive correlations in the heatmap for T7, and in the network T7, was the soil factor that was most correlated (12% of the total). Particularly in the network, all correlations involving P were positive. P was especially involved with the ammonification gene family in both analyses, but only in T7. In the heatmap for T7 (Figure 1), OM was involved in 75% of the negative correlations but, in the network T150, was the soil factor that established most correlations (1/4), with all being positive.
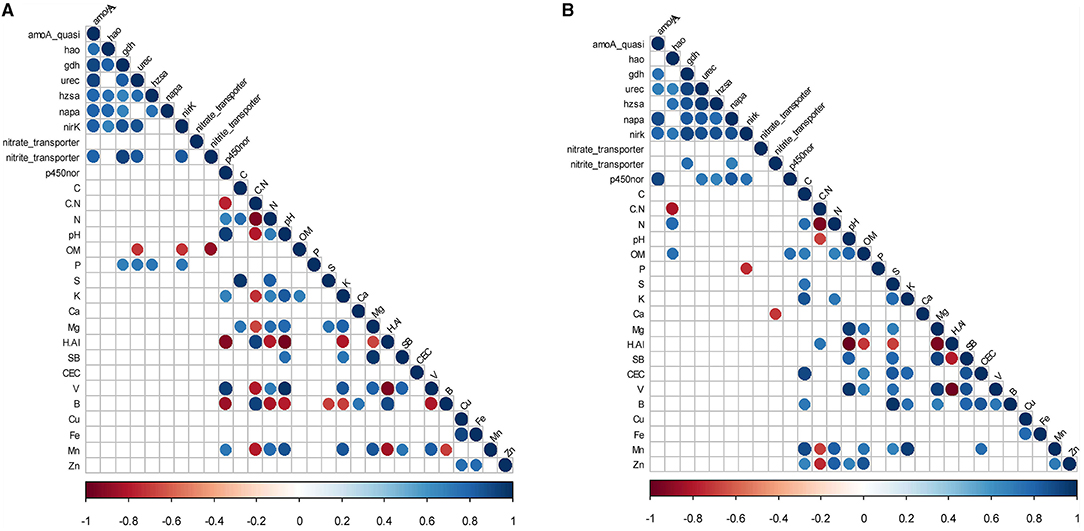
Figure 1. Heatmap correlation test between GeoChip v.5.0M gene families responsive to N treatments and physicochemical soil factors, according to significant values (p < 0.05) of Spearman rank correlation coefficients performed in the R environment with the “Hminsk and CorrPlot” package. (A) Sample collection 7 days after fertilizer addition; (B) Sample collection 150 days after fertilizer addition. Positive values (blue circles) represent positive correlations, and negative values (red circles) represent negative correlations.
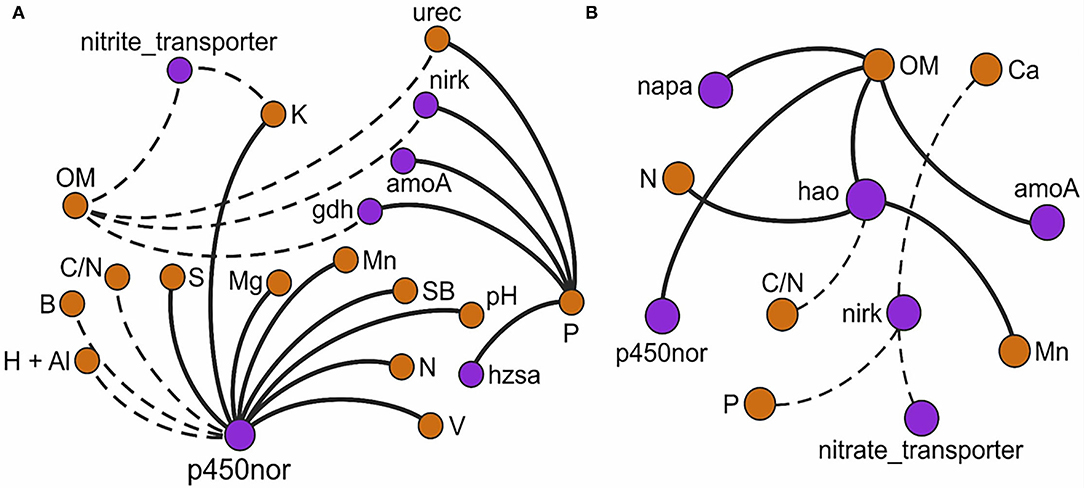
Figure 2. Network correlation analysis of the GeoChip v.5.0M gene families responsive to N treatments and physicochemical soil factors based on significant (p < 0.05) Spearman rank correlation coefficient values. (A) Sample collection 7 days after fertilizer addition; (B) Sample collection 150 days after fertilizer addition. The nodes represent the physicochemical soil factors (light brown) and gene families (purple). The thick edges represent positive correlations, and the dashed edges represent negative correlations.
The p450nor gene family established most of the correlations in the network T7 (1/4 of total), and the majority of them (85%) were positive (Figure 2). In T150, the hao gene family was the most present gene family in correlations, involved in 60% of them in the heatmap and 75% in the network, with all being positive, especially with N (Figures 1, 2). Curiously, in the heatmap T7 (Figure 1), N had positive correlations with the p450nor gene, and in T150, the correlations were positive with the hao gene. Instead, C:N had negative correlations with the p450nor gene in T7 and negative correlations in T150 with the hao gene. Another interesting result is that hao did not exhibit any correlations in the T7 network analysis (Figure 2).
Discussion
Although the use of urea as N fertilizer combined with vinasse is widely recommended, researchers and government agencies have carried out studies in the field and laboratories to evaluate their roles as emission sources of GHGs, such as N2O, which results from this practice. Understanding the microbial processes involved in the production of this gas has become relevant for the sugar and alcohol industry since N losses in the sugarcane systems have environmental and economic impacts on society. Our results using GeoChip v.5.0M technology showed that nearly 87% of the functions related to the N metabolism might have been active in the first days after the addition of fertilizers. Although the correlations between the two datasets encompassing soil chemical factors and gene families have been limited to P and various gene families in the T7 period, at least in part due to the low number of replicate samples in this study, they suggested the occurrence of specific microbial metabolic activities in this period. The rapid increases in the availability of N and C sources in the system resulting from the addition of fertilizers (Table 1) would have led the microbiota to quickly access these less complex sources of nutrients and perform the immobilization and mineralization of N (47). Consequently, the nitrification process must have been quickly activated, which justified the great abundance of amoA genes detected in the NV treatment (Table 2). Other evidence of the occurrence of nitrification on a large scale was the increase of the ammonia monooxygenase sequences from Nitrosopumilus (AOA) (Table 3) and the reduction of the C:N ratio (Table 1) in NVT7, which suggests the possibility losses of N in the form of NO3 besides de C (47, 48). Moreover, in the same treatment, greatest production of N2O was detected by Navarrete et al. (19). It is known that the addition of this organic residue results in the availability of nutrients in sugarcane soils that are able to promote changes in the structure and functionality of the microbiota associated with N and C cycles (11, 19, 35).
Three nutrients analyzed here deserve to be highlighted due to their relevance in response to treatments: Zn and K showed increases, mainly in the T150 period, while P increased in the control from T7 to T150 (Table 1). A study evaluating the number of copies of the amoA gene from Archaea and Bacteria in an Australian soil submitted to different doses of Zn concluded that high doses of this nutrient could have inhibited the nitrification process of ammonia in the short term (49). In the same study, after only two years, the population of AOB became resilient to high doses of Zn and was able to perform the process. The same inhibitory effect promoted by Zn on nitrification can be observed in other studies (50, 51). Zn is one of the most abundant micronutrients and heavy metals commonly found in vinasse (52, 53) and, in our study, it was the soil chemical factor that showed the greatest increase between T7 and T150 periods in the NV treatment (Table 1). High levels of this micronutrient in the NV treatment could justify the reduction of ammonia monooxygenase in T150 associated with the amoA gene from Nitrosopumilus. In addition, it is possible that ammonia monooxygenase sequences from Nitrosomonas (AOB) increased only in T150 because of their resilience to the Zn inhibition effect.
K was another nutrient that vinasse residue supplies in large quantities (54, 55) and was already demonstrated to be a good nitrification reducer in alkaline soils (56). In an incubation experiment with potassium thiosulfate (KTS), N2O production decreased after 12 days of application (56). Regarding P, in agricultural alkaline soils, the high availability of this nutrient can reduce the nitrification process (57). Here, although the pH values remained between 5.2 and 5.8 (acidic), its increase in control and NV treatments in T150 in relation to T7 may favor nitrification reducer by K. In relation P, our results obtained from correlation analysis showed that the levels of this nutrient were strongly associated with gene families in the first days after the application of N fertilizers, suggesting its influence on the microbial N metabolism (Figures 1, 2). The gene family hzsA, associated with the anammox process, had the greatest abundance in NVT7 and showed positive correlations with P in this study and other agricultural soils (58). This result suggests an influence of this nutrient on the anammox process, besides that of nitrification. Once in this N process, the NO2 is used as an electron acceptor to oxidase NH3 due to anaerobic conditions (59), and it is possible that the higher consumption of O2 in the first days of application of the N fertilizer with vinasse may promote oxygen-limiting conditions.
In the hypothesis concerning to the most of the nutrient mobilized by the plants between 7 and 150 days after the addition of fertilizers, the high number of correlations between P and gene families in T7 would support the high N metabolism in this period. The p450nor gene family stood out in our analysis as the most correlated gene family with soil chemical factors (Figures 1, 2). It presented the greatest prevalence in NV (p = 0.008) in the T7 period according to the GeoChip analysis (Table 2). However, considering the nitric oxide reductase function from Bacteria associated with p450nor, its prevalence was in T150 (Table 3). The p450nor gene [cytochrome P450 nitric oxide (NO) reductase (NOR)] is related to the reduction of NO2 to N2O (60, 61). The system is located in the mitochondria and is activated during anaerobic respiration (62), which is carried out by denitrifying fungi in agricultural soils (62–64), causing plant diseases (62, 65). In Bacteria, this denitrification gene has been demonstrated to enhance virulence against the host because it uses an alternative electron acceptor, which is favorable to their lifestyle under anaerobic conditions (66). According to our results, there were significant increases in p450nor in the NV treatment and in the T7 period and, regarding its associated functions with Mycobacterium (Table 3), in the T150 period. Suleiman et al. (11) observed increases in the sporulation of fungi concomitantly with the highest volumes of N2O during the first days after the addition of vinasse in sugarcane soils. According to Higgins et al. (67), environments with regular N inputs impose the utilization of N-oxides by the microbiota. These observations suggest that fungi denitrification by p450nor could have occurred and increased under NVT7 conditions. Lourenço et al. (21) associated the production of N2O in sugarcane soils under vinasse with N fertilizers with the nirK gene from denitrifying fungi; however, this study did not analyze the p450nor denitrification gene. Instead, the nirK gene seems not to be assessed here on a large scale by the microbiota in NVT7 since it had the greatest abundance in N60 and sequences of nitrite reductase in N0.
The high S availability in NV may benefit from the increase of p450nor in this treatment (Table 1), which can also intensify the denitrification process, with a reduction in sulfate for the decomposition of organic matter (68) and an increase in N2O production (69). This observation could contribute to justify the positive correlations among the p450nor gene, OM, and S in our analyses (Figures 1, 2). According to Schlüter et al. (70), the lack of O2 and the availability of NO3 as an electron acceptor are basic requirements for heterotrophic denitrification in soils. It is possible that the accelerated immobilization of N is readily available to microorganisms in the first days after fertilization, while the sugarcane plantlets, which do not require large amounts of this nutrient (71), favored the nitrification processes related to amoA and denitrification related to p450nor in the NV treatment.
In this study, one of the genes that showed the greatest response to NV was hao, which encodes the oxidoreductase enzyme in the nitrification process (HAO) (72, 73). Zhao et al. (74) quantified the hao gene associated with nitrifying Bacteria in a lake that received domestic sewage. The lake regions with the highest amounts of NO3 and NH3 showed the highest abundance of hao, suggesting the association of this gene with the eutrophication process. Is it possible that the physicochemical conditions of the soil submitted to the application of vinasse in this period were similar to a eutrophication process such as that described in Zhao et al. (74)? Analyzing the oxidoreductase enzymes that correspond to the hao gene family, Nitrosomonas (AOB), which is related to N2O emissions by nitrification (75), had the greatest number of sequences in NVT7. Wu et al. (76) concluded that Nitrosomonas and Nitrosopumilus tended to dominate in moderately eutrophic sediment with a significant ammonium input (2.86 mM). In addition, the higher prevalence of ammonia monooxygenase and oxidoreductase sequences in NVT7 suggests a high rate of nitrification, which would also lead to the formation of NO3 and N2O. Smith et al. (31) concluded that the napA gene, associated with the reduction of nitrate to nitrite in denitrification, is more important in environments with lower concentrations of NO3. In our microarray analyses, napA was more abundant in the N60 treatment and, in the MG-RAST analysis, the nitrate reductase in N0 (Tables 2, 3), suggesting that NO3 could be found in higher concentrations in NV.
The gene families ureC and gdh are associated with the ammonification process, in which the formation of NH4 from the organic matter by microorganisms occurs (77). Since a significant number of correlations between ureC and gdh with P were identified, we also considered the potential effect of this element on the ammonification process. Previous studies revealed the inhibitory effect of KH2PO4 on ammonification (78). In our study, the contents of K increase in N60 and NV treatments at the T150 period (Table 1). This could justify the increases of ureC and gdh in N60T7 and urease and glutamate dehydrogenase from Bacteria in NVT7 and their decrease in NVT150.
The results also revealed the greatest number of nitrate and nitrite transporter functions (related to ammonification) from the genus Anaeromyxobacter, a Proteobacteria (Table 3). Due to its versatile metabolism, this genus is composed of ecologically competitive Bacteria that can survive in several ecosystems, microaerophilic or anaerobic, including agricultural soils (79). This genus can reduce NO3 to NH4 via NO2 as an electron acceptor and reduce N2O (80). A specific clade from the nosZ operon, A. dehalogenans, has a higher affinity for N2O, suggesting that they are more competitive under the environmental levels of N2O (80). Anaeromyxobacter type nosZ sequences were found to be the most abundant nosZ genes in agricultural soils from Illinois (USA) and appear to compose a significant portion of the N2O-reducing community (79, 81), suggesting an important role in the N cycling. The high prevalence of this Bacteria in our soils with N fertilizer, suggests favorable conditions to their development due to the microaerophilic environment and N2O presence. On the other hand, Archaea associated with the ammonification process was frequent in the T150 period, where the salt concentrations were higher than in T7. While the non-saline soil conditions of T7 may favor the AOA Nitrosopumilus, Archaea with organic matter recycling capabilities, such as Natronomonas (from order Halobacteriales), were found in the saline soil condition in T150 (82). Natronomonas is a haloalkaline Archaea with the capacity to survive in soils with higher salt contents (82). According to our results, the highest number of urease sequences in Archaea is from Natronomonas. Some studies have shown that urease is sensitive to low pH (83), which may justify the presence of halophiles in soils with more salts.
GeoChip v.5.0M is a highly specific, sensitive, and quantitative method based on both computational and experimental assays (28). This technology can reveal information about the functionality of the microbial community in several environments not yet analyzed through other molecular techniques (28, 32). In this study, the analyses of metagenomes combined with GeoChip v.5.0M allowed the identification of which gene families associated with the N cycle significantly responded to the physicochemical changes resulting from typical agricultural management in tropical soils. Moreover, it was possible to indicate groups of Archaea and Bacteria associated with the N process, with potential increases under higher salt contents and N2O levels, however, in low O2 sources. This information suggests their specific metabolic capabilities in restricted conditions. The results also call attention to the increase of the p450nor gene family in these conditions that were previously associated with the denitrification process by Fungi pathogenic to plants and Mycobacterium pathogenic to animals. Finally, the Bacteria and Archaea domains are certainly ubiquitous in soil but have developed very specialized functions depending on the physical and chemical characteristics of their environments (82).
Conclusion
N losses in sugarcane crop soils by management practices promote economic and environmental impacts that need attention. Our results suggest “key” genes and processes in the N cycle that may be changed due to the new composition of soil factors under vinasse and urea fertilization. Our DNA analysis from the soil samples using microarray technology and metagenome annotation revealed that the gene families related to ammonification (gdh and ureC from Bacteria and Archaea), nitrification (amoA from AOA and AOB), and denitrification (p450nor from Eukarya) are the main processes responsive to this typical fertilizer management in the first days after the application. Ammonia oxidizers and halophilic Archaea were responsive to the increases in K salts and nutrients such as P in soil under vinasse application. This common practice for cultivated sugarcane may favor an increase of Anaeromyxobacter, which is capable of reducing the GHG gas N2O.
Data Availability Statement
The datasets presented in this study can be found in online repositories. The names of the repository/repositories and accession number(s) can be found at: Metagenomics Rapid Annotation (MG-RAST) server (http://www.metagenomics.anl.gov) under project accession Metagenomes of sugarcane soils-CENA USP and accession numbers 4582104.3 to 4582153.3.
Author Contributions
All authors listed have made a substantial, direct and intellectual contribution to the work, and approved it for publication.
Funding
This study was supported by the Coordenação de Aperfeiçoamento de Pessoal de Nıivel Superior, CAPES, Brazil (Grant Number: 23038.006927/2014-35/Premium 116/2017) and Fundação de Amparo à Pesquisa do Estado de São Paulo, FAPESP, Brazil (Grant Numbers: 2011/51749-6 and 2012/13321-7). MC was supported by the Conselho Nacional de Desenvolvimento Cientıifico e Tecnológico, CNPq, Brazil (Grant Number: 142146/2017-0) and CAPES (Finance Code 001). AN was supported by the FAPESP (Grant Number: 2017/03575-5). AV was supported by FAPESP (Grant Number: 2019/25931-3). DB was supported by CAPES (Finance Code 001). ST was recipient of a CNPq (Grant Number: 311008/2016-0). Publication number 7301 of the Netherlands Institute of Ecology (NIOO-KNAW).
Conflict of Interest
The authors declare that the research was conducted in the absence of any commercial or financial relationships that could be construed as a potential conflict of interest.
Publisher's Note
All claims expressed in this article are solely those of the authors and do not necessarily represent those of their affiliated organizations, or those of the publisher, the editors and the reviewers. Any product that may be evaluated in this article, or claim that may be made by its manufacturer, is not guaranteed or endorsed by the publisher.
Acknowledgments
We wish to thank Tatiana Rosa Diniz for support during the greenhouse experiment.
References
1. Glória NA. Uso agronômico de resíduos. In: Reunião Brasileira de Fertilidade do Solo e Nutrição de Plantas, Vol. 20. Piracicaba (1992). p. 195–212.
2. Dias MOS, Ensinas AV, Nebra SA, Maciel Filho R, Rossell CEV, Maciel MRW. Production of bioethanol and other bio-based materials from sugarcane bagasse: integration to conventional bioethanol production process. Chem Eng Res Des. (2009) 87:1206–16. doi: 10.1016/j.cherd.2009.06.020
3. Gasparotto F, Rodrigues FS, Seratto CD, da Costa TR. Cadeias produtivas da cana-de-açúcar, do algodão e de frutas. Maringá: UniCesumar (2014).
4. Hidalgo K, Rodríguez B, Valdivié M, Febles M. Utilización de la vinaza de destilería como aditivo para pollos en ceba. Rev Cuba Cienc Agrícola. (2009) 43:281–4. Available online at: https://www.redalyc.org/articulo.oa?id=193015481011
5. Christofoletti CA, Escher JP, Correia JE, Marinho JFU, Fontanetti CS. Sugarcane vinasse: environmental implications of its use. Waste Manag. (2013) 33:2752–61. doi: 10.1016/j.wasman.2013.09.005
6. Moran-Salazar RG, Sanchez-Lizarraga AL, Rodriguez-Campos J, Davila-Vazquez G, Marino-Marmolejo EN, Dendooven L, et al. Utilization of vinasses as soil amendment: consequences and perspectives. Springerplus. (2016) 5:1007. doi: 10.1186/s40064-016-2410-3
7. Penatti CP, Cambria S, Boni PS, Arruda FCO, Manoel LA. Efeitos da aplicação de vinhaça e nitrogênio na soqueira da cana-de-açúcar - Boletim Técnico Copersucar, Forty-Fourth Volume. São Paulo: Copersucar (1988).
8. Moraes BS, Junqueira TL, Pavanello LG, Cavalett O, Mantelatto PE, Bonomi A, et al. Anaerobic digestion of vinasse from sugarcane biorefineries in Brazil from energy, environmental, and economic perspectives: profit or expense? Appl Energy. (2014) 113:825–35. doi: 10.1016/j.apenergy.2013.07.018
9. Madejón E, Burgos P, López R, Cabrera F. Soil enzymatic response to addition of heavy metals with organic residues. Biol Fertil Soils. (2001) 34:144–50. doi: 10.1007/s003740100379
10. Tejada M, Gonzalez JL. Effects of two beet vinasse forms on soil physical properties and soil loss. Catena. (2006) 68:41–50. doi: 10.1016/j.catena.2006.04.025
11. Suleiman AKA, Lourenço KS, Pitombo LM, Mendes LW, Roesch LFW, Pijl A, et al. Recycling organic residues in agriculture impacts soil-borne microbial community structure, function and N2O emissions. Sci Total Environ. (2018) 631–2:1089–99. doi: 10.1016/j.scitotenv.2018.03.116
12. Lourenço KS, Suleiman AKA, Pijl AS, van Veen JA, Cantarella H, Kuramae EE. Resilience of the resident soil microbiome to organic and inorganic amendment disturbances and to temporary bacterial invasion. Microbiome. (2018) 6:142. doi: 10.1186/s40168-018-0525-1
13. Lourenço KS, Suleiman AKA, Pijl A, Cantarella H, Kuramae E. Dynamics and resilience of soil mycobiome under multiple organic and inorganic pulse disturbances. Sci Total Environ. (2020) 733:139173. doi: 10.1016/j.scitotenv.2020.139173
14. Bossolani JW, Crusciol CAC, Merloti LF, Moretti LG, Costa N, Tsai SM, et al. Long-term lime and gypsum amendment increase nitrogen fixation and decrease nitrification and denitrification gene abundances in the rhizosphere and soil in a tropical no-till intercropping system. Geoderma. (2020) 375:114476. doi: 10.1016/j.geoderma.2020.114476
15. Bossolani JW, Crusciol CAC, Leite MFA, Merloti LF, Moretti LG, Pascoaloto IM, et al. Modulation of the soil microbiome by long-term Ca-based soil amendments boosts soil organic carbon and physicochemical quality in a tropical no-till crop rotation system. Soil Biol Biochem. (2021) 156:108188. doi: 10.1016/j.soilbio.2021.108188
16. Rocha KF, Kuramae E, Borges BMF, Leite MFA, Rosolem CA. Microbial N cycling gene abundance is affected by cover crop specie and development stage in an integrated cropping system. Arch Microbiol. (2020) 202:2005–12. doi: 10.1007/s00203-020-01910-2
17. Jesus EC, Marsh TL, Tiedje JM, Moreira FMS. Changes in land use alter the structure of bacterial communities in Western Amazon soils. ISME J. (2009) 3:1004–11. doi: 10.1038/ismej.2009.47
18. Faoro H, Alves AC, Souza EM, Rigo LU, Cruz LM, Al-Janabi SM, et al. Influence of soil characteristics on the diversity of Bacteria in the Southern Brazilian Atlantic Forest. Appl Environ Microbiol. (2010) 76:4744–9. doi: 10.1128/AEM.03025-09
19. Navarrete AA, Diniz TR, Braga LPP, Silva GGZ, Franchini JC, Rossetto R, et al. Multi-analytical approach reveals potential microbial indicators in soil for sugarcane model systems. PLoS ONE. (2015) 10:e0129765. doi: 10.1371/journal.pone.0129765
20. Wang J, Chen Z, Xiong Z, Chen C, Xu X, Zhou Q, et al. Effects of biochar amendment on greenhouse gas emissions, net ecosystem carbon budget and properties of an acidic soil under intensive vegetable production. Soil Use Manag. (2015) 31:375–83. doi: 10.1111/sum.12202
21. Lourenço KS, Dimitrov MR, Pijl A, Soares JR, do Carmo JB, van Veen JA, et al. Dominance of bacterial ammonium oxidizers and fungal denitrifiers in the complex nitrogen cycle pathways related to nitrous oxide emission. Glob Change Biol Bioenergy. (2018) 10:645–60. doi: 10.1111/gcbb.12519
22. Lehtovirta-Morley LE. Ammonia oxidation: ecology, physiology, biochemistry and why they must all come together. FEMS Microbiol Lett. (2018) 365:1–9. doi: 10.1093/femsle/fny058
23. Tourna M, Stieglmeier M, Spang A, Konneke M, Schintlmeister A, Urich T, et al. Nitrososphaera viennensis, an ammonia oxidizing archaeon from soil. Proc Natl Acad Sci USA. (2011) 108:8420–5. doi: 10.1073/pnas.1013488108
24. Sauder LA, Albertsen M, Engel K, Schwarz J, Nielsen PH, Wagner M, et al. Cultivation and characterization of Candidatus Nitrosocosmicus exaquare, an ammonia-oxidizing archaeon from a municipal wastewater treatment system. ISME J. (2017) 11:142–57. doi: 10.1038/ismej.2016.192
25. Cloutier ML, Bhowmik A, Bell TH, Bruns MA. Innovative technologies can improve understanding of microbial nitrogen dynamics in agricultural soils. Agric. Environ. Lett. (2019) 4:190032. doi: 10.2134/ael2019.08.0032
26. Varon-Lopez M, Dias ACF, Fasanella CC, Durrer A, Melo IS, Kuramae EE. Sulphur-oxidizing and sulphate-reducing communities in Brazilian mangrove sediments: sulphur cycling in mangroves. Environ Microbiol. (2014) 16:845–55. doi: 10.1111/1462-2920.12237
27. Cong J, Liu X, Lu H, Xu H, Li Y, Deng Y, et al. Analyses of the influencing factors of soil microbial functional gene diversity in tropical rainforest based on GeoChip 5.0. Genom Data. (2015) 5:397–8. doi: 10.1016/j.gdata.2015.07.010
28. Shi Z, Yin H, Van Nostrand JD, Voordeckers JW, Tu Q, Deng Y, et al. Functional gene array-based ultrasensitive and quantitative detection of microbial populations in complex communities. mSystems. (2019) 4:e00296–19. doi: 10.1128/mSystems.00296-19
29. Zhao L, Liu Y, Wang Z, Yuan S, Qi J, Zhang W, et al. Bacteria and fungi differentially contribute to carbon and nitrogen cycles during biological soil crust succession in arid ecosystems. Plant Soil. (2020) 447:379–92. doi: 10.1007/s11104-019-04391-5
30. Yu X, LaBaer J. High-throughput identification of proteins with AMPylation using self-assembled human protein (NAPPA) microarrays. Nat Protoc. (2015) 10:756–67. doi: 10.1038/nprot.2015.044
31. Smith CJ, Dong LF, Wilson J, Stott A, Osborn AM, Nedwell DB. Seasonal variation in denitrification and dissimilatory nitrate reduction to ammonia process rates and corresponding key functional genes along an estuarine nitrate gradient. Front Microbiol. (2015) 6:542. doi: 10.3389/fmicb.2015.00542
32. Carter MQ, Xue K, Brandl MT, Liu F, Wu L, Louie JW, et al. Functional metagenomics of Escherichia coli O157:H7 interactions with spinach indigenous microorganisms during biofilm formation. PLoS ONE. (2012) 7:e44186. doi: 10.1371/journal.pone.0044186
33. Empresa Brasileira de Pesquisa Agropecuária (EMBRAPA). Manual de Métodos de Análise do Solo. Rio de Janeiro: EMBRAPA (2011).
34. Wu L, Liu X, Schadt CW, Zhou J. Microarray-based analysis of subnanogram quantities of microbial community DNAs by using whole-community genome amplification. Appl Environ Microbiol. (2006) 72:4931–41. doi: 10.1128/AEM.02738-05
35. de Chaves MG, Silva GGZ, Rossetto R, Edwards RA, Tsai SM, Navarrete AA. Acidobacteria subgroups and their metabolic potential for carbon degradation in sugarcane soil amended with vinasse and nitrogen fertilizers. Front Microbiol. (2019) 10:1680. doi: 10.3389/fmicb.2019.01680
36. Magoč T, Salzberg SL. FLASH: fast length adjustment of short reads to improve genome assemblies. Bioinformatics. (2011) 27:2957–63. doi: 10.1093/bioinformatics/btr507
37. Ewing B, Green P. Base-calling of automated sequencer traces using Phred. II. Error probabilities. Genome Res. (1998) 8:186–94. doi: 10.1101/gr.8.3.186
38. Meyer F, Paarmann D, D'Souza M, Olson R, Glass E, Kubal M, et al. The metagenomics RAST server – a public resource for the automatic phylogenetic and functional analysis of metagenomes. BMC Bioinformatics. (2008) 9:386. doi: 10.1186/1471-2105-9-386
39. Ferreira DF. Sisvar: a computer analysis system to fixed effects split plot type designs. Rev Bras Biom. (2019) 37:529. doi: 10.28951/rbb.v37i4.450
40. O'Leary NA, Wright MW, Brister JR, Ciufo S, Haddad D, McVeigh R, et al. Reference sequence (RefSeq) database at NCBI: current status, taxonomic expansion, functional annotation. Nucl Acids Res. (2016) 44:D733–45. doi: 10.1093/nar/gkv1189
41. Overbeek R, Begley T, Butler RM, Choudhuri JV, Chuang HY, Cohoon M, et al. The subsystems approach to genome annotation and its use in the project to annotate 1000 genomes. Nucl. Acids Res. (2005) 33:5691–5702. doi: 10.1093/nar/gki866
42. The UniProt Consortium. UniProt: the universal protein knowledgebase in 2021. Nucleic Acids Res. (2021) 49:D480–9. doi: 10.1093/nar/gkaa1100
43. Bastian M, Heymann S, Jacomy M. Gephi: an open source software for exploring and manipulating networks. Proc Int AAAI Confer Web Soc Media. (2009) 3:361–2. Available online at: https://ojs.aaai.org/index.php/ICWSM/article/view/13937
44. Brandes U. A faster algorithm for betweenness centrality. J Math Sociol. (2001) 25:163–77. doi: 10.1080/0022250X.2001.9990249
45. Blondel VD, Guillaume J-L, Lambiotte R, Lefebvre E. Fast unfolding of communities in large networks. J Stat Mech. (2008) 2008:P10008. doi: 10.1088/1742-5468/2008/10/P10008
46. Pollard KS, Dudoit S, van der Laan MJ. Multiple testing procedures: the multtest package and applications to genomics. In: Gentleman R, Carey VJ, Huber W, Irizarry RA, Dudoit S, editors. Bioinformatics and Computational Biology Solutions Using R and Bioconductor. Statistics for Biology and Health. New York, NY: Springer New York (2005). p. 249–71.
47. Han K-H, Choi W-J, Han G-H, Yun SI, Yoo S-H, Ro H-M, et al. Urea-nitrogen transformation and compost-nitrogen mineralization in three different soils as affected by the interaction between both nitrogen inputs. Biol Fertil Soils. (2004) 39:193–9. doi: 10.1007/s00374-003-0704-4
48. Schroder JL, Zhang H, Girma K, Raun WR, Penn CJ, Payton ME. Soil acidification from long-term use of nitrogen fertilizers on winter wheat. Soil Sci Soc Am J. (2011) 75:957–64. doi: 10.2136/sssaj2010.0187
49. Mertens J, Broos K, Wakelin SA, Kowalchuk GA, Springael D, Smolders E. Bacteria, not archaea, restore nitrification in a zinc-contaminated soil. ISME J. (2009) 3:916–23. doi: 10.1038/ismej.2009.39
50. Mertens J, Degryse F, Springael D, Smolders E. Zinc toxicity to nitrification in soil and soilless culture can be predicted with the same biotic ligand model. Environ Sci Technol. (2007) 41:2992–7. doi: 10.1021/es061995+
51. Liu A, Fang D, Wang C, Li M, Young RB. Recovery of soil nitrification after long-term zinc exposure and its co-tolerance to Cu in different soils. Environ Sci Pollut Res. (2015) 22:314–9. doi: 10.1007/s11356-014-3338-1
52. Wilkie AC, Riedesel KJ, Owens JM. Stillage characterization and anaerobic treatment of ethanol stillage from conventional and cellulosic feedstocks. Biomass Bioenergy. (2000) 19:63–102. doi: 10.1016/S0961-9534(00)00017-9
53. Prasad MNV, Shih K, editors. Environmental Materials and Waste: Resource Recovery and Pollution Prevention. Amsterdam; Boston, MA: Elsevier; Academic Press (2016).
54. Moraes BS, Zaiat M, Bonomi A. Anaerobic digestion of vinasse from sugarcane ethanol production in Brazil: challenges and perspectives. Renew Sustain Energy Rev. (2015) 44:888–903. doi: 10.1016/j.rser.2015.01.023
55. Parsaee M, Kiani MKD, Karimi K. A review of biogas production from sugarcane vinasse. Biomass Bioenergy. (2019) 122:117–25. doi: 10.1016/j.biombioe.2019.01.034
56. Cai Z, Gao S, Xu M, Hanson BD. Evaluation of potassium thiosulfate as a nitrification inhibitor to reduce nitrous oxide emissions. Sci Total Environ. (2018) 618:243–9. doi: 10.1016/j.scitotenv.2017.10.274
57. Shi X, Hu HW, Müller C, He JZ, Chen D, Suter HC. Effects of the nitrification inhibitor 3,4-dimethylpyrazole phosphate on nitrification and nitrifiers in two contrasting agricultural soils. Appl Environ Microbiol. (2016) 82:5236–48. doi: 10.1128/AEM.01031-16
58. Zhou H, Zhang Q, Gu C, Jabeen S, Li J, Di H. Soil anammox community structure in different land use soils treatment with 13C urea as determined by analysis of phospholipid fatty acids. Appl Microbiol Biotechnol. (2017) 101:6659–9. doi: 10.1007/s00253-017-8404-4
59. Narita Y, Zhang L, Kimura Z, Ali M, Fujii T, Okabe S. Enrichment and physiological characterization of an anaerobic ammonium-oxidizing bacterium “Candidatus Brocadia sapporoensis.” Syst Appl Microbiol. (2017) 40:448–57. doi: 10.1016/j.syapm.2017.07.004
60. Novinscak A, Goyer C, Zebarth BJ, Burton DL, Chantigny MH, Filion M. Novel p450nor gene detection assay used to characterize the prevalence and diversity of soil fungal denitrifiers. Appl. Environ. Microbiol. (2016) 82:4560–4569. doi: 10.1128/AEM.00231-16
61. Higgins SA, Schadt CW, Matheny PB, Löffler FE. Phylogenomics reveal the dynamic evolution of fungal nitric oxide reductases and their relationship to secondary metabolism. Genome Biol Evol. (2018) 10:2474–89. doi: 10.1093/gbe/evy187
62. Shoun H, Fushinobu S, Jiang L, Kim S-W, Wakagi T. Fungal denitrification and nitric oxide reductase cytochrome p450nor. Philos Trans R Soc Lond B Biol Sci. (2012) 367:1186–94. doi: 10.1098/rstb.2011.0335
63. Mothapo N, Chen H, Cubeta MA, Grossman JM, Fuller F, Shi W. Phylogenetic, taxonomic and functional diversity of fungal denitrifiers and associated N2O production efficacy. Soil Biol Biochem. (2015) 83:160–75. doi: 10.1016/j.soilbio.2015.02.001
64. Wu D, Senbayram M, Well R, Brüggemann N, Pfeiffer B, Loick N, et al. Nitrification inhibitors mitigate N2O emissions more effectively under straw-induced conditions favoring denitrification. Soil Biol Biochem. (2017) 104:197–207. doi: 10.1016/j.soilbio.2016.10.022
65. Mendes LW, de Chaves MG, Fonseca MC, Mendes R, Raaijmakers JM, Tsai SM. Resistance breeding of common bean shapes the physiology of the rhizosphere microbiome. Front Microbiol. (2019) 10:2252. doi: 10.3389/fmicb.2019.02252
66. Fritz C, Maass S, Kreft A, Bange F-C. Dependence of Mycobacterium bovis BCG on anaerobic nitrate reductase for persistence is tissue specific. Infect Immun. (2002) 70:286–91. doi: 10.1128/IAI.70.1.286-291.2002
67. Higgins SA, Welsh A, Orellana LH, Konstantinidis KT, Chee-Sanford JC, Sanford RA, et al. Detection and diversity of fungal nitric oxide reductase genes (p450nor) in agricultural soils. Appl Environ Microbiol. (2016) 82:2919–28. doi: 10.1128/AEM.00243-16
68. Lau GN, Sharma KR, Chen GH, van Loosdrecht MCM. Integration of sulphate reduction, autotrophic denitrification and nitrification to achieve low-cost excess sludge minimisation for Hong Kong sewage. Water Sci Technol. (2006) 53:227–35. doi: 10.2166/wst.2006.101
69. Šimek M, Virtanen S, Krištufek V, Simojoki A. Yli-Halla M. Evidence of rich microbial communities in the subsoil of a boreal acid sulphate soil conducive to greenhouse gas emissions. Agric Ecosyst Environ. (2011) 140:113–22. doi: 10.1016/j.agee.2010.11.018
70. Schlüter S, Henjes S, Zawallich J, Bergaust L, Horn M, Ippisch O, et al. Denitrification in soil aggregate analogues-effect of aggregate size and oxygen diffusion. Front Environ Sci. (2018) 6:17. doi: 10.3389/fenvs.2018.00017
71. Mariano E, Leite JM, Vieira-Megda MX, Ciampitti IA, Vitti AC, Faroni CE, et al. Biomass and nutrient content by sugarcane as affected by fertilizer nitrogen sources. Crop Sci. (2016) 56:1234–44. doi: 10.2135/cropsci2015.06.0349
72. Whittaker M, Bergmann D, Arciero D, Hooper AB. Electron transfer during the oxidation of ammonia by the chemolithotrophic bacterium Nitrosomonas europaea. Biochim Biophys Acta Bioenerg. (2000) 1459:346–55. doi: 10.1016/S0005-2728(00)00171-7
73. Koch H, van Kessel MAHJ, Lücker S. Complete nitrification: insights into the ecophysiology of comammox Nitrospira. Appl Microbiol Biotechnol. (2019) 103:177–89. doi: 10.1007/s00253-018-9486-3
74. Zhao R, Chen Y, Qu J, Jin P, Zheng Z, Cui Z. Insights into the variations of hao-dependent nitrifying and nir-dependent denitrifying microbial communities in ammonium-graduated lake environments. Appl Sci. (2019) 9:3229. doi: 10.3390/app9163229
75. White CJ, Lehnert N. Is there a pathway for N2O production from hydroxylamine oxidoreductase in ammonia-oxidizing bacteria? Proc Natl Acad Sci USA. (2016) 113:14474–6. doi: 10.1073/pnas.1617953114
76. Wu L, Han C, Zhu G, Zhong W. Responses of active ammonia oxidizers and nitrification activity in eutrophic lake sediments to nitrogen and temperature. Appl Environ Microbiol. (2019) 85:e00258–19. doi: 10.1128/AEM.00258-19
77. Dannenmann M, Simon J, Gasche R, Holst J, Naumann PS, Kögel-Knabner I, et al. Tree girdling provides insight on the role of labile carbon in nitrogen partitioning between soil microorganisms and adult European beech. Soil Biol Biochem. (2009) 41:1622–31. doi: 10.1016/j.soilbio.2009.04.024
78. Ryan JA, Sims JL, Peaslee DE. Effect of phosphate and chloride salts on ammonification in waterlogged soils. Soil Sci Soc Am J. (1972) 36:915–7. doi: 10.2136/sssaj1972.03615995003600060023x
79. Sanford RA, Wagner DD, Wu Q, Chee-Sanford JC, Thomas SH, Cruz-Garcia C, et al. Unexpected nondenitrifier nitrous oxide reductase gene diversity and abundance in soils. Proc Natl Acad Sci USA. (2012) 109:19709–14. doi: 10.1073/pnas.1211238109
80. Yoon S, Nissen S, Park D, Sanford RA, Löffler FE. Nitrous oxide reduction kinetics distinguish bacteria harboring clade I nosZ from those harboring clade II nosZ. Appl Environ Microbiol. (2016) 82:3793–800. doi: 10.1128/AEM.00409-16
81. Orellana LH, Rodriguez-R LM, Higgins S, Chee-Sanford JC, Sanford RA, Ritalahti KM, et al. Detecting nitrous oxide reductase (nosZ) genes in soil metagenomes: method development and implications for the nitrogen cycle. MBio. (2014) 5:e01193–14. doi: 10.1128/mBio.01193-14
82. Navarro-Noya YE, Valenzuela-Encinas C, Sandoval-Yuriar A, Jiménez-Bueno NG, Marsch R, Dendooven L. Archaeal communities in a heterogeneous hypersaline-alkaline soil. Archaea. (2015) 2015:1–11. doi: 10.1155/2015/646820
Keywords: Anaeromyxobacter, nitrogen cycling, sugarcane soil, Natronomonas, p450nor
Citation: de Chaves MG, Venturini AM, Merloti LF, Barros DJ, Rossetto R, Kuramae EE, Tsai SM and Navarrete AA (2021) Combined Use of Vinasse and Nitrogen as Fertilizers Affects Nitrification, Ammonification, and Denitrification by Prokaryotes. Front. Soil Sci. 1:746745. doi: 10.3389/fsoil.2021.746745
Received: 24 July 2021; Accepted: 21 October 2021;
Published: 22 November 2021.
Edited by:
Annamaria Bevivino, Italian National Agency for New Technologies, Energy and Sustainable Economic Development (ENEA), ItalyReviewed by:
Armand W. Koné, Université Nangui Abrogoua, Côte d'IvoireGerardo Moreno, University of Extremadura, Spain
Copyright © 2021 de Chaves, Venturini, Merloti, Barros, Rossetto, Kuramae, Tsai and Navarrete. This is an open-access article distributed under the terms of the Creative Commons Attribution License (CC BY). The use, distribution or reproduction in other forums is permitted, provided the original author(s) and the copyright owner(s) are credited and that the original publication in this journal is cited, in accordance with accepted academic practice. No use, distribution or reproduction is permitted which does not comply with these terms.
*Correspondence: Acacio Aparecido Navarrete, YWNhY2lvbmF2YXJyZXRlQGdtYWlsLmNvbQ== orcid.org/0000-0001-6204-8702