Nitrogen fertilizer driven nitrous and nitric oxide production is decoupled from microbial genetic potential in low carbon, semi-arid soil
- 1Department of Soil and Crop Sciences, Texas A&M University, College Station, TX, United States
- 2Texas A&M AgriLife Research, Lubbock, TX, United States
- 3Environmental Sciences Division, Argonne National Laboratory, Lemont, IL, United States
- 4Texas A&M AgriLife Research, Vernon, TX, United States
- 5Department of Ecology and Conservation Biology, Texas A&M University, College Station, TX, United States
Introduction: Nitrous oxide (N2O) emission from soil is a major concern due to its contribution to global climate change and its function as a loss mechanism of plant-available nitrogen (N) from the soil. This is especially true in intensive agricultural soils with high rates of N fertilizer application such as those on the semi-arid Southern High Plains, USA.
Methods: This study examined emissions of N2O, pore-space concentrations of N2O and nitric oxide (NO), soil chemical properties, water content, and the genetic potential for N cycling five years after conservation system and N management implementation.
Results: For these semi-arid soils with low N, carbon, and water contents, large soil N2O emissions (up to 8 mL N2O-N m-2 day-1) are directly related to the application of N fertilizer which overwhelms the N2O reducing capacity of the soil. When this fertilizer N is depleted, N2O flux is either low, non-existent, or net-negative and has been observed as early as mid-season for preplant applied N fertilizer (-0.1 mL N2O-N m-2 day-1). Soil pore-space gas concentrations (N2O and NO) remained relatively constant across the growing season (average N2O: 0.78 µL N2O L-1 soil air; NO: 3.3 µL NO L-1 soil air, indicating a base-level of N-cycle activity, but was not directly related to surface emissions of N2O which decreased across the growing season. In addition, genetic potential for N cycle activities increased across the growing season simultaneously with stagnant/reduced N cycle activity. This reflects the difficulty in relating genetic potential to in-situ activity in field research.
Conclusion: It is likely that in a nutrient and carbon-poor soil, such as the semi-arid agricultural soil in this study, the microbial processes associated with N cycling are mostly limited by inorganic-N and less directly related to genetic potential at the time of sampling.
1 Introduction
Nitrous oxide (N2O) production is one of the major concerns in fertilized and irrigated agroecosystems due to its contribution to the greenhouse effect and its role in the destruction of stratospheric ozone, both of which contribute to its large global warming potential (1, 2). Microbial nitrification and denitrification in natural and managed soils are responsible for 70% of global N2O emissions (3–5). The controls over these two N cycling processes can be broken down into proximal (primary) and distal (secondary) effects based on their immediacy of impact on the process.
Primary controls over nitrogen gas cycling and N2O production include the amount of, and interactions between soil carbon (C) (6–8) soil nitrate () (9–12) and ammonium () (13), soil oxygen (O2) (14), and pH (7, 15) and have been well documented in the literature. Briefly, both -N and -N are the starting substrate for the production of N gases in soil ecosystems, where the oxidation (nitrification) and reduction (denitrification) is directly tied to soil O2 content with nitrification requiring an aerobic environment and denitrification requiring an anerobic environment. Furthermore, the use of carbon as an energy source in anaerobic environments enables denitrification to take place (6) while C respiration may create temporary anaerobic microsites which can harbor denitrification activity. Lastly, soil pH directly influences the completion of denitrification through the pH specificity of N2O reductase activity (16) and other nitrification and denitrification enzymes. Distal controls over N trace gas emissions mostly operate to affect the genetic potential for denitrification and nitrification and can include disturbance (frequency and intensity), soil pH, soil C content, soil moisture and O2 content, temperature, and predation (7, 15). Understanding genetic potential within a system as a distal control allows for better defining the mechanisms behind soil processes. For example, in the case of semi-arid soils, the genetic potential for specific processes such as denitrification may not be expected due to the low water content of the soil. However, previous studies have shown a strong potential for processes such as N2O reduction (via nosZ clade I and II abundance) in semi-arid lands which is a key step in denitrification (17).
These primary and secondary controls for soil N cycling can be significantly affected by producer decisions regarding soil tillage and nutrient management. Physical disturbances such as tillage can alter soil structure, introduce/bury organic inputs, and increase water infiltration which act to alter the O2 and C content of the soil while also affecting microbial abundance (18, 19). The application of fertilizer alters the soil chemical composition affecting the rate of nutrient cycling and soil pH in addition to altering the soil microbial community through selection and inhibition based on the added nutrients (20). Furthermore, the timing of these decisions can alter the potential for production and consumption of greenhouse gases (GHGs) and affect their concentration in the soil atmosphere (21–23) especially in semi-arid soils which are less well buffered against environmental change due to low organic matter, water, and nutrient content and thus naturally vary throughout the growing season.
Previous studies have highlighted the importance of N fertilizer application on N2O emissions from the soil surface in semi-arid agriculture (17) where it was determined that the timing of N fertilizer application altered the temporal pattern of N2O production. However, surface emissions alone may not fully indicate the level of N-cycle activity related to N fertilizer application. Pore-space, or soil air, concentrations of N gases have been studied in several environments including agricultural production (24) and forests (25) where N gas production was correlated with N fertilizer application and soil N content (respectively). In highly intensive agroecosystems such as those on the semi-arid Southern High Plains, pore-space concentrations will also likely be related to soil N content through N fertilizer application but will likely differ in concentration due to the low N, C, and water content in the semi-arid soils relative to previous studies (24, 25). Strong relationships between pore-space concentrations of N gases and emissions from the soil surface in forest soils have also been reported (26), but soil management decisions may alter this relationship due to alterations in pore-space, microsites, and genetic potential in the soil profile. In the case of semi-arid soils, this has already begun to be evaluated, where strong genetic potential for N2O consumption was determined in surface soils (23).
The study reported here evaluates the combined effects of conservation soil management (no-tillage and cover cropping) and altered N timing (pre-plant vs. split application) on N gas production in semi-arid soil under cotton (Gossypium hiristum L.) production. In addition, soil chemical parameters that drive N gas production and the genetic potential for N gas production are evaluated to begin to understand the role these proximal and distal controls play in producing N gases in fertilized semi-arid systems. We hypothesized that conservation management practices would alter both proximal and distal controls for N gas production in this system due to the known effects cover-cropping and no-tillage have on soil chemical characteristics and the activity and abundance of microorganisms. We further hypothesized that there will be a combined effect of genetic potential and soil -N on N gas production supporting previous determinations of N2O emissions being driven by denitrification in these soils. Lastly, we hypothesized that N gas production and the drivers of production will vary seasonally with peak activity during the early growing season for the pre-plant application of N fertilizer which should alleviate N limitation and jumpstart N cycling in this system.
2 Methods
2.1 Site description
Research was conducted in continuous cotton systems at the Texas A&M AgriLife Research Center in Lubbock, TX (33.687°, -101.827°) where conservation systems were implemented in Fall 2014 and sampling was conducted from 2018-2020. Annual precipitation is 481 mm and the 30-year mean annual temperature for Lubbock is 16.1°C (30-year average, 1991-2020) (27). The soil series at the research site is an Acuff loam described as a fine-loamy, mixed, superactive, thermic Aridic Paleustoll (28).
The study was designed as a split plot with the main plot consisting of different conservation practices (tillage systems), and the split plot consisting of N fertilizer treatments differing in their time of application. Conservation practices included no-tillage with a winter wheat cover crop (NTW) and no-tillage winter fallow (NT), compared to a conventional tillage winter fallow (CT) system. Both the NTW and NT systems were introduced in November 2015. The field was in conventional tillage for at least the previous 60 years. Split plot N fertilizer application timings include the following: no-added N (control); 100% of N applied pre-plant (PP); and 40% of N applied PP and 60% applied mid-season (SPLIT). The study consisted of 3 blocks (replicates) for a total of 27 plots, each measuring 15 m in length and four rows wide with 1 m row spacing. Full agronomic information for the three-year study and a timeline for management and sampling in 2020 is included in the supplemental material (Supplementary Material 1: Methods).
Agronomic information for the final year is briefly presented here. Furrow irrigation of the control plots and all treatment plots was conducted on 03 June, 30 June, 10 August, and 26 August with each event applying about 152 mm of water. Pre-plant N fertilizer was applied 6 May 2020. Mid-season N fertilizer applications were made 21 July 2020 near the initiation of reproductive growth. Application of N fertilizer was made at rate of 168 kg ha-1 as urea-ammonium-nitrate (UAN) and was conducted using a knife and coulter fertilizer applicator mounted to a tractor. Nitrogen fertilizer was applied 10 – 15 cm from the cotton row at a depth of 15 cm. Nitrate added in the irrigation water amounted to 47 kg N ha-1 in 2020, calculated from the concentration of nitrate-N in the irrigation water, the rate of irrigation applied, and the number of irrigation events (29). Cotton (DP 1820) was planted on 1 June 2020 and harvested on 20 November 2020. Cover crops were planted in November 2019 and terminated on 15 April 2020.
2.2 Soil sampling and analyses
Soil sampling was conducted at three key cotton growth periods: early-season (June, vegetative growth), mid-season (August, peak plant production), and late-season (October, reproductive growth) in 2018, 2019, and 2020. However, only 2020 is presented in the main text due to the inclusion of soil gas and microbial genetic potential measurements being made in conjunction with soil sampling in the final year. In 2020, samples were specifically collected on 24 June, 05 August, and 10 October. Soil samples were collected using 2.5 x 40 cm hand probes to a depth of 20 cm separated into 0-10 and 10-20 cm depths. Six soil cores per plot were collected, homogenized, and stored on ice until a ~25 g subsample could be collected and frozen at -80°C for DNA analysis. The remaining soil was weighed and then dried at 60°C for 7 days to determine gravimetric water content. Soil samples were extracted for soil nitrate-N (-N) and ammonium-N (-N) using a 1 M KCl (1:10 soil to extractant ratio, 4 g of soil) and analyzed for -N using flow injection spectrometry (FIAlab 2600, FIAlab Instruments Inc., Bellevue, WA) following cadmium reduction to and for -N by the Berthelot reaction involving salicylate (30). In addition, soil samples were analyzed for mineralizable C (Cmin) by a 3-day rewetting incubation with 40 g of air-dried soil sieved at 4 mm (31). Soil pH was determined by a 1:2 dilution of 10 g soil with DI water (32).
2.3 Microbial analyses
Microbial analyses for genetic potential were conducted by quantitative polymerase chain reaction (qPCR) of major bacterial nitrification and denitrification genes and the genetic marker for bacterial abundance (Table 1) for samples collected in the final year of the study (33–39). DNA was extracted using a Quick-DNA Fecal/Soil Microbe 96 Magbead Kit (Zymo Research, Irvine, CA, USA) with a 30-min, room-temperature drying period prior to final DNA elution. Quantitative polymerase chain reaction was conducted on a Thermo Fisher Quant Studio 5 (Thermo Fisher Scientific Inc., Waltham Mass, US) with gBlock gene fragments specific for each gene assayed (Supplementary Table 1, Integrated DNA Technologies, Inc., Coralville, IA, USA). In addition, quality control qPCR was conducted on the Quant Studio 5 by spiking a known concentration qPCR reaction with extracted DNA from this study and evaluating if any inhibition occurred in a process similar to Hartman et al. (40), where extracted DNA was added to a qPCR assay to quantify the abundance of Vibrio alginolyticus with gyrB as the gene target (41). A spiked sample with a threshold cycle (Ct) value within 3 standard deviations of the quality control Ct mean was determined to not contain inhibitors. A small number (<25) of samples were determined to inhibit PCR reactions and were diluted 1:10 with sterile water to reduce inhibition and re-analyzed with qPCR as described above. Initial extractions and dilutions were used to conduct qPCR evaluation of the soil for the 16S rRNA gene, nosZ clade I and clade II (N2O reductases). Due to elution volume limitations, additional extractions were conducted, and diluted 1:10 with sterile water, as described above for nirK (copper dependent nitrite reductase), nirS (cytochrome cd nitrite reductase), and amoA (ammonia monooxygenase) qPCR evaluations from the same soil subsamples as the previous extractions.
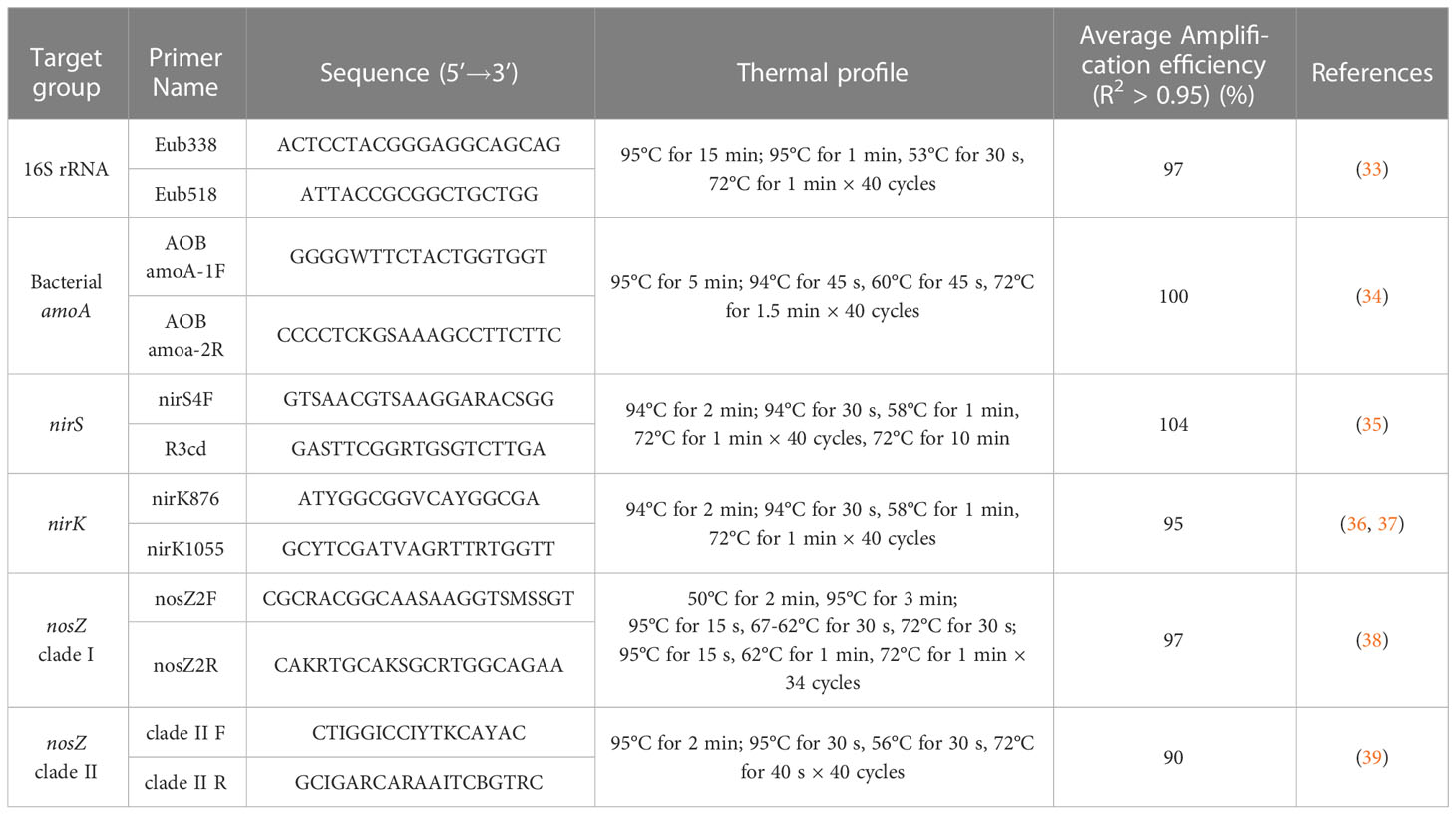
Table 1 Primer sequences and thermal profiles for total bacterial and bacterial N-cycle functional gene abundances.
2.4 Greenhouse gas emissions and pore-space concentrations
Nitrous oxide emissions and in-ground concentrations of N2O and NO were collected during the final year of the study using a Gasmet DX4040 portable FTIR gas analyzer (Gasmet Technologies, Helsinki, Finland) integrated with a LiCor 8100A opaque chamber for soil surface emissions. The Gasmet DX4040 was also connected to in-ground pore-space probes (PSPs) to measure in-ground concentrations (42). Soil N2O emissions and pore-space samplings were conducted within 48 hours of soil sample collection. Soil NO emissions were measured but were not determined to be significant (flux rate below measurable limit) and thus are not included. Pore-space concentration measurements were conducted according to Brummell et al. (43) and McDonald et al. (42). Briefly, PSPs were installed at the beginning of the growing season and then connected to reservoir bottles 24 hours before measurement. On the day(s) of sampling, PSPs with reservoir bottles were integrated into a closed loop system with the Gasmet analyzer for soil N2O and NO concentration determination. The reservoir bottle increased the system volume to greater than that of the sample cell within the Gasmet, thus eliminating any vacuum being placed on soil pores.
2.5 Statistical analyses
Analyses of variance (ANOVA) for soil chemical parameters was conducted across the three growing seasons within each depth using the lmer function within the lme4 package in R (44) where the initial analysis of variance model included sampling period, conservation system, and N treatment as fixed effects with evaluation of their main effects as well as their combined interactions. Random effects specified in the initial model were rep and the interaction of rep and sampling period to account for repeated measurement of the same research plots over time. The two sampling depths were parsed prior to ANOVA due to the well-known effects of depth on soil parameters, and the desire to evaluate the data in the context of the area from which soil pore-space gases will be measured. In addition, the application of N fertilizer at a depth of 15 cm created a gradient of inorganic-N between the two depths which may have affected the production of greenhouse gases at depth. Consistent over the evaluation of soil parameters was the significant effect of sampling period as a main effect, while only the repeated measure of -N was affected with an interaction of sampling period and N timing. Due to the strong observed effect of sampling period as a main factor, analyses for the final year of the study were split by depth and sampling period to understand within seasonal effects without the variability of the distinct climatic and soil conditions at each sampling period. Analyses of variance of soil chemical parameters for the three-year dataset and final year are presented in the supplementary material (Supplementary Material 2: Soil Chemical Results). Microbial genetic potential for N cycling was also evaluated by ANOVA within sampling period and depth for the final year of the study (Supplementary Material 3: Microbial abundance in the final year).
Principal components analysis of the soil parameters was conducted using the prcomp function in the stats package in R (45), where an initial evaluation of the relationship of soil parameters was conducted across all three years of the study, and then separately for the final year to support the use of the final year as a reasonable representative of the variability in soil parameters over the length of the study. Soil parameters were normalized to a range of 0-1 prior to analyses to evaluate the variability between samples without the vast differences in scale using the preProcess function of the caret package in R (46). As mentioned above, soil gas measurements were only collected in the final year of the study due to the use of the first two years as test periods for the soil pore-space probes and subsequent mechanical issues with the FTIR analyzer that prevented full-season evaluation of pore-space gases and surface emissions, further necessitating the final-year analyses. Microbial abundance data was subsequently limited to analysis during the final year of the study as well due to the research team’s decision to specifically focus on the final year for the holistic evaluation of the soil biochemical effects of the implemented treatments on gas production. Principal components analysis of microbial gene abundances was conducted using the same process as the analysis for soil chemical parameters detailed above. However, soil chemical parameter vectors were fit to the ordination of microbial gene data using the envfit function in the vegan package in R (47). Principal components ordinations and contributions to axes were plotted using ggplot2 (48)
LASSO regression analysis was conducted using the glmnet and cv.glmnet functions within the glmnet package in R (49). The data was split into training and testing datasets at random with a 75%, 25% split resulting in 114 samples for training and 38 samples for testing (46). The model for each gas was initially developed using leave-one-out cross validation with the cv.glmnet function, after which the best model was refined using the glmnet function and the lambda generated by the initial model. The model was then used to predict gas concentration for the testing dataset using the predict function, and the R2 of the fit was calculated to determine predictive capacity of the model for the new dataset. Lastly, the measured vs. the predicted concentrations for the testing dataset were plotted to visualize the fit of the data to the linear model using ggplot2 (48).
3 Results
3.1 Soil characteristics
Soil chemical analyses were conducted for the combined effect of the implemented treatments and time over the course of the growing season across all three years of the study and are presented in the supplementary material (Supplementary Material 2: Soil chemical results). Due to significant effects of sampling period as a main effect and minimal interaction with soil chemical parameters, soil chemical characteristics were evaluated within sampling period and depth for the final year of the study where soil chemical analyses were combined with microbial gene abundance and gas sampling. The results of ANOVA for the final year are also presented in the supplementary material (see Supplementary Material 2.2 Final Year Analyses).
The relationships between soil chemical parameters as they are affected by the implementation of conservation system and altered N timings was addressed using PCA across the entire dataset (Supplementary Figure 2) and within the final year of the study (Figure 1) across both depths. It is clear that the data in the final year are representative of the pattern of variance present in the three-year dataset due to the similar pattern in variance for the full dataset and the final year alone and the similar total variance explained by PCA of soil chemical parameters between the final year (66.8%, Figure 1) and the full three-year dataset (59.2%, Supplemental Figure 2). For the final year, PC1 explained 38.7% of the variance in the data where significant contributions to the variability explained included soil -N, pH, and -N which each contributed greater than 20% of the variability (Figure 1). PC2 explained 28.1% of the variance in soil chemical parameters where significant contributions included GWC and Cmin content (Figure 1), Lastly, PC3 also explained a moderate proportion of variability, accounting for 14.6% of the variability with both Cmin content and soil pH significantly contributing to the variance explained (Figure 1).
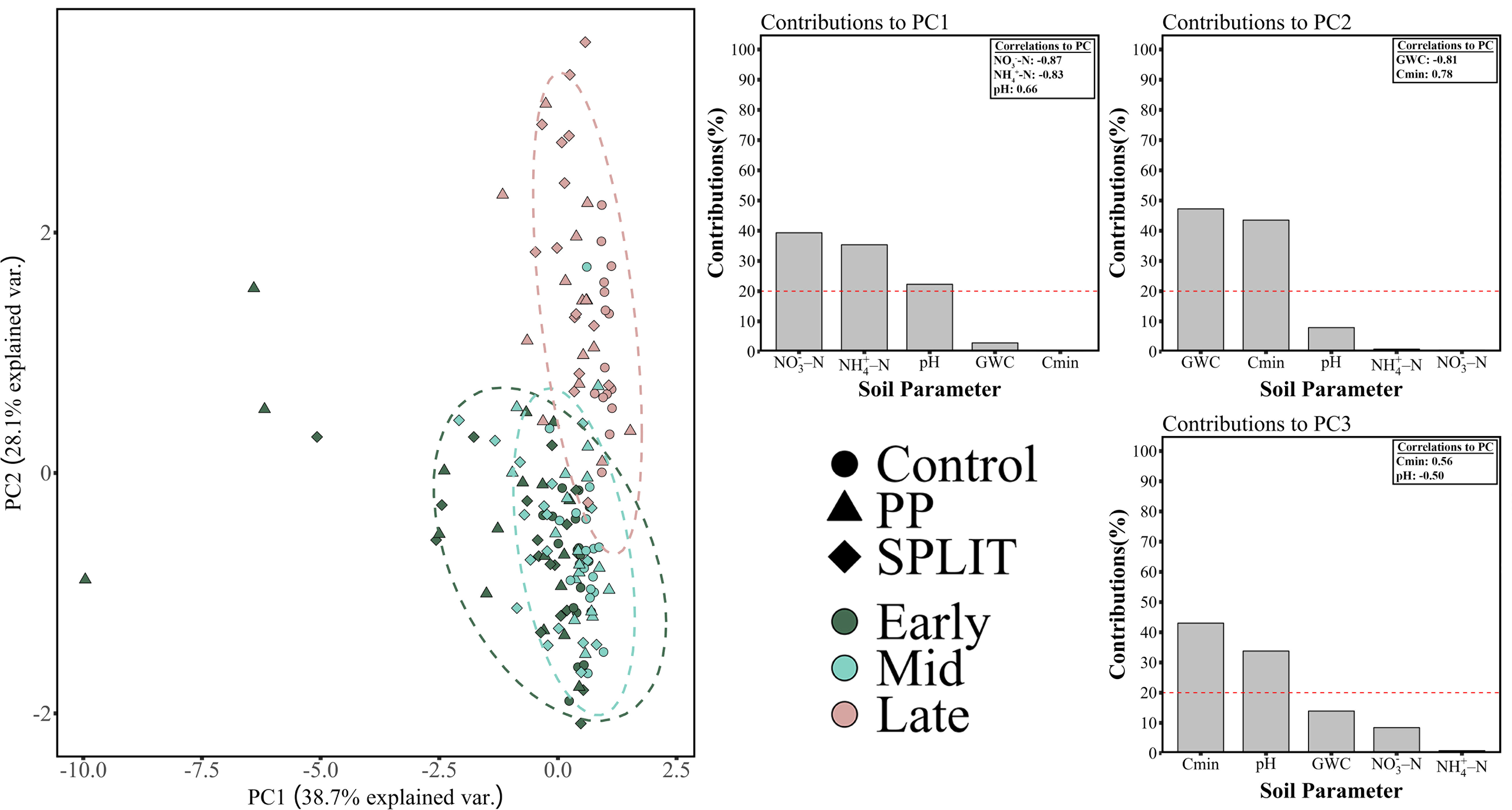
Figure 1 Principal components analysis of soil chemical characteristics in the final year of the study. Soil characteristic contributions to the top three principal components are presented with correlation coefficients for the significant contributors to each PC. Control, no-added nitrogen (N) fertilizer; PP, pre-plant fertilizer application at a rate of 168 kg N ha-1; SPLIT, 40% preplant, 60% mid-season application of N fertilizer at a total rate of 168 kg N ha-1. Early, early-season sampling (June); Mid, mid-season sampling (August); Late, late-season sampling (October). GWC, gravimetric water content; Cmin, mineralizable carbon content; -N, nitrate; -N ammonium.
Correlations between the significant contributors to variance explained for each PC are displayed in Figure 1. Both -N and -N were negatively correlated with PC1 and soil pH was positively correlated with PC1 (Figure 1); Cmin content was positive correlated with PC2 and GWC was negatively correlated with PC2; and both Cmin content and pH were negatively correlated with PC3. As mentioned above, PCA analyses were conducted across depths and depth was not determined to cluster within the ordination of either soil chemical dataset.
3.2 Microbial abundances
Analyses of variance for genetic abundances are reported in the supplemental material (see Supplemental Material 5: Microbial abundance in the final year). In brief, bacterial amoA (AOB) abundance was affected at all three sampling periods, while nirS and nirK abundances were unaffected at any of the three sampling periods. In general, bacterial N cycle gene abundances increased across the growing season, with a distinct increase in AOB abundance from the early- to mid-season sampling. As with soil chemical characteristics, microbial genetic abundances were evaluated by PCA to determine overall relationships of implemented treatments and sampling periods on the microbial genetic potential for N cycle activities (Figure 2). In addition, soil chemical characteristics were fit to the PCA ordination of the microbial gene abundances, and their vectors are presented on Figure 2. It should be noted that vector coordinates were multiplied by 4 for visualization on the figure, but the relationships to the ordination of the microbial gene abundances remain unchanged. In addition, some clustering was observed by depth for microbial gene abundances and ellipses are fit to these clusters. Principal components analysis of microbial gene abundances revealed no distinct clustering by sampling period, conservation system, or N timing. The data was moderately clustered by depth, where the 0-10 cm depth contained much more variability than the 10-20 cm depth (Figure 2). The total variability explained by PCA for gene abundances was 67.2 for PC1 and PC2 combined, where PC1 explained 50.4% of the variance and PC2 explained 16.8% of the variance. In addition, PC3 moderately contributed to variance explained (13.8%). Significant contributors to the variability explained by PC1 included the 16S gene, nosZ clade I, nirK, and nirS where all genes were negatively correlated with PC1 (Figure 2). Only nosZ clade II significantly contributed to the variance explained by PC2, where clade II was positive correlated with PC2 (Figure 2). Lastly, only AOB significantly contributed to the variance explained by PC3, where AOB was positively correlated with the axis (Figure 2).
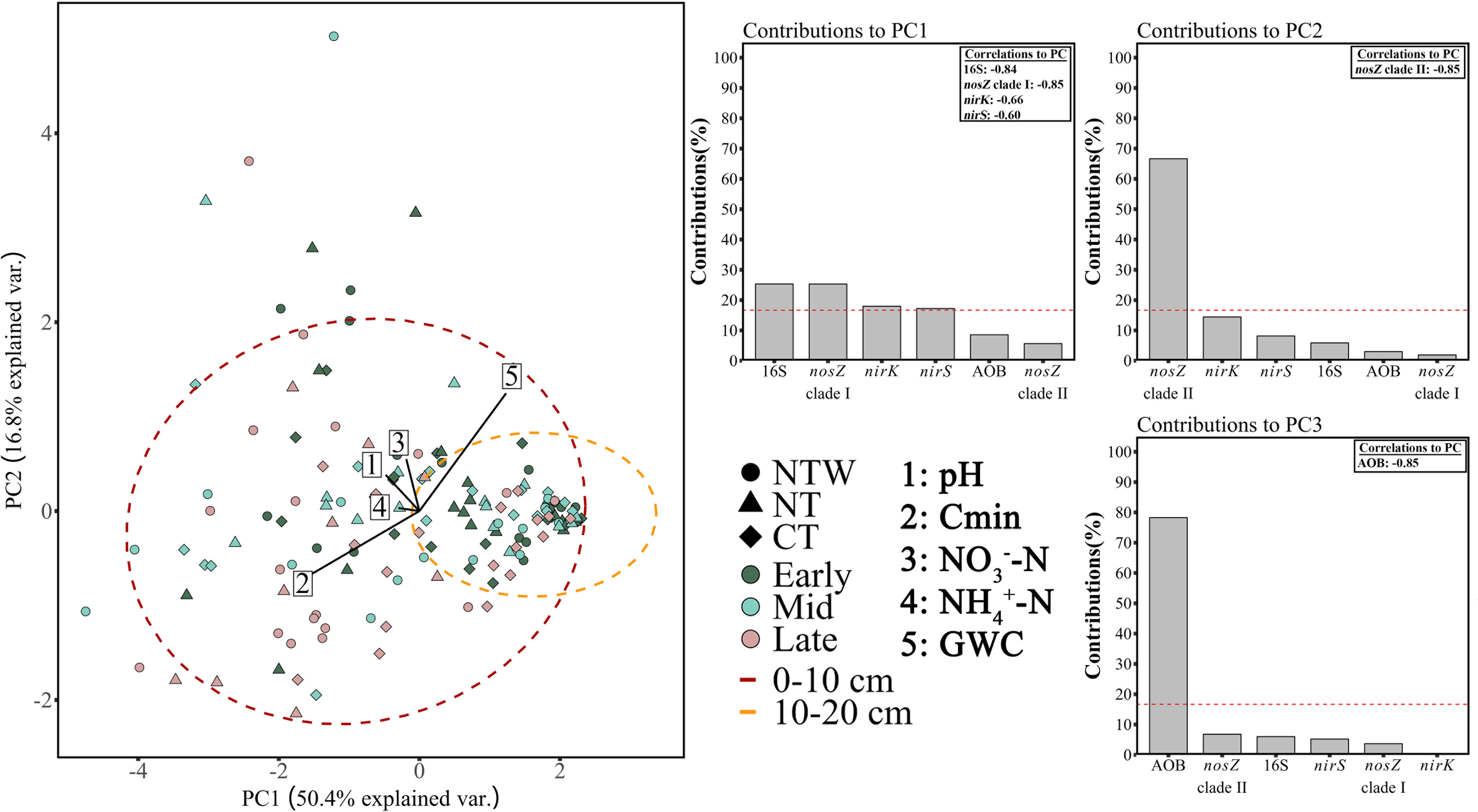
Figure 2 Principal components analysis of bacterial nitrogen cycle gene abundances. Gene abundance contributions to each PC are presented with correlations between significant contributors and each PC. Environmental variables were fit to the ordination and their associated vectors are displayed at 4× size for ease of visualization. Ellipses denote clusters of samples by depth. Control, no-added nitrogen (N) fertilizer; PP, pre-plant fertilizer application at a rate of 168 kg N ha-1; SPLIT, 40% preplant, 60% mid-season application of N fertilizer at a total rate of 168 kg N ha-1. Early, early-season sampling (June); Mid, mid-season sampling (August); Late, late-season sampling (October). GWC, gravimetric water content; Cmin, mineralizable carbon content; -N, nitrate; -N ammonium.
For the analysis fitting soil chemical parameters to the ordination of genes in Figure 2, only Cmin content and GWC were determined to be significant, with nearly antithetical relationships to the ordination data (Figure 2).
3.3 Nitrous oxide emissions
Nitrous oxide emissions were evaluated at each soil sampling event to assess the impacts of conservation system and N timing on production of N2O from the soil-atmosphere interface. At the early-season sampling, N timing significantly affected N2O emissions (p=0.002, Figure 3) where emissions from the PP treatment (8071 µg N2O-N m-2 d-1) were greater than from the SPLIT treatment (1697 µg N2O-N m-2 d-1) and the control (-218 µg N2O-N m-2 d-1).
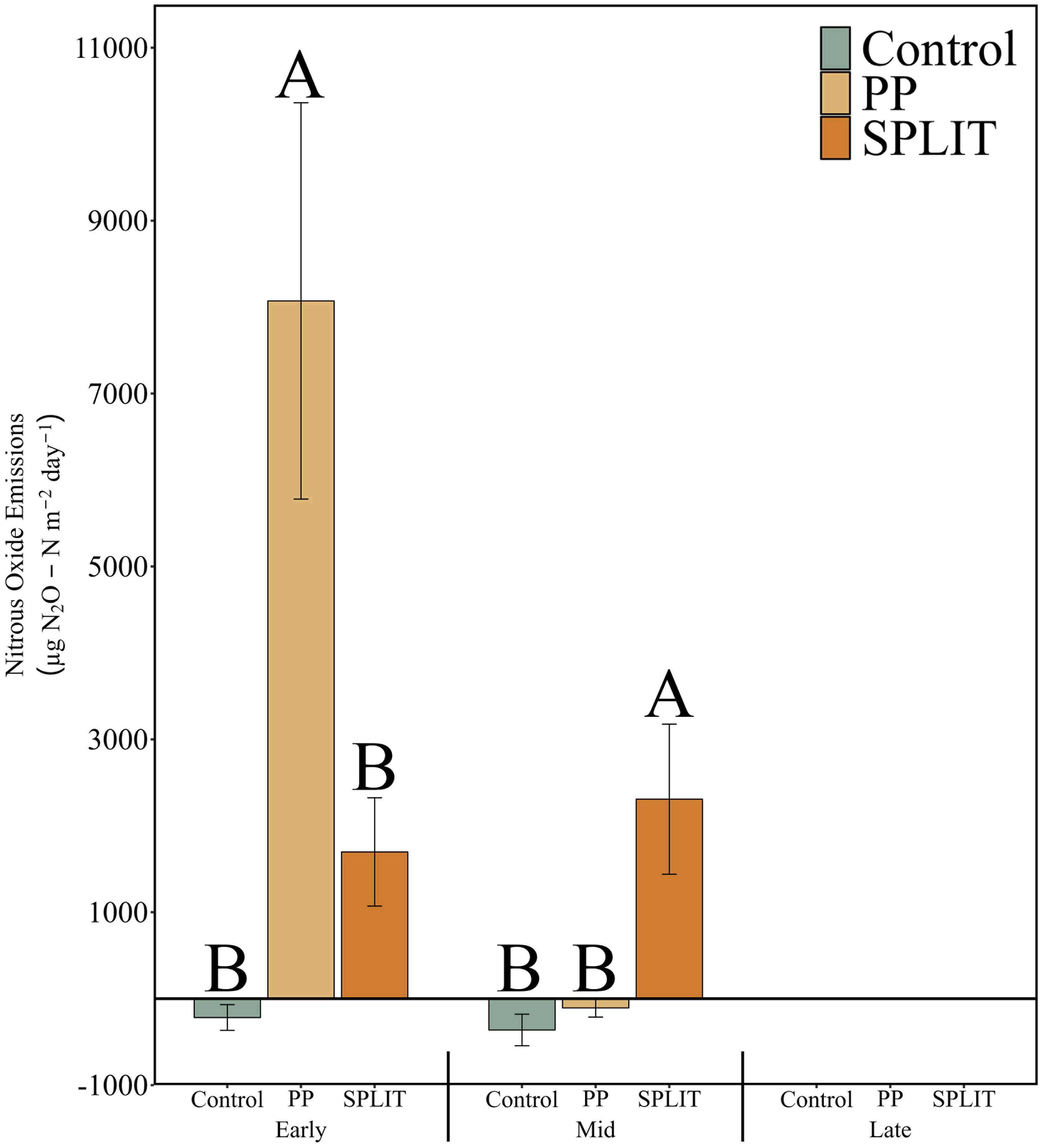
Figure 3 Nitrous oxide emissions (µg N2O-N m-2 day-1) measured at the soil-atmosphere interface in 2020 at the early growth period (June), mid-season growth period (August), and late growth period (October) of a cotton cropping system. Data was collected at a single timepoint within each growth stage in conjunction with soil sampling and pore-space gas concentrations. Control, no added N fertilizer; PP, 100% pre-plant application of N fertilizer (168 kg ha-1); SPLIT, 40% PP 60% mid-season application of N fertilizer. Error bars are standard error. LSD letters are compared within sampling period and are different at α=0.05.
At the mid-season sampling, N timing affected N2O emissions (p=0.002, Figure 3) where emissions from the SPLIT treatment (2307 µg N2O-N m-2 d-1) were greater than from the PP treatment (-106 µg N2O-N m-2 d-1) and the control (-362 µg N2O-N m-2 d-1).
No net production or consumption of N2O was determined at the late-season sampling (Figure 3 and Supplementary Table 12,13).
3.4 Soil pore-space concentrations of nitrogen
Pore-space concentrations of N2O and NO were analyzed for each sample date at a depth of 7.5 cm and 15 cm. At the early-season sampling at the 7.5 cm depth, pore-space concentration of N2O was affected by N timing (p=0.041, Figure 4 and Supplementary Table 13) where the PP treatment had greater N2O concentration (1.2 µL N2O L-1) than the control (0.88 µL N2O L-1), but not the SPLIT treatment (1.0 µL N2O L-1). No treatment effects were determined for NO at 7.5 cm or for either N2O or NO at 15 cm at the early season sampling (Figure 4 and Supplementary Table 12). Pore-space concentrations of NO and N2O were unaffected at both 7.5 cm or 15 cm depth for the mid- and late-season samplings (Figure 4 and Supplementary Tables 12, 13). Across the growing season, pore-space N2O concentrations consistently decreased at both the measured depths, while NO concentrations increased from the early- to mid-season samplings and then decreased at the late season sampling at the 7.5 cm depth and were unchanged from early- to mid-season, but decreased at the late-season sampling at the 15 cm depth.
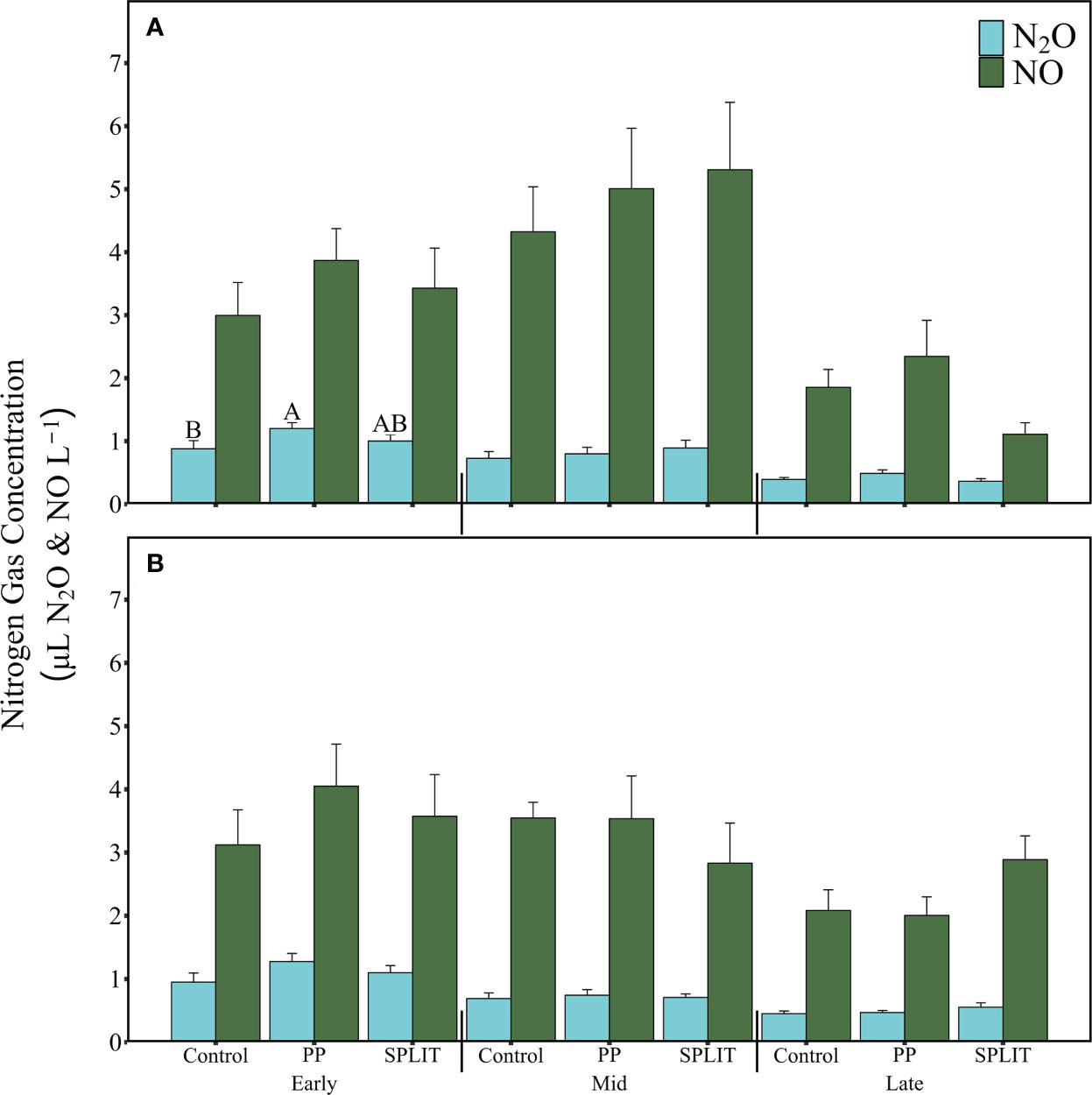
Figure 4 Average nitrogen (N) gas concentrations of soil pore-space N gases (N2O and NO, µL L-1) for N timing treatments at each sampling period. (A) 7.5 cm depth; (B) 15 cm depth. Control, no-added N; PP; 100% pre-plant N fertilizer application; SPLIT 40% pre-plant 60% mid-season application of N fertilizer. LSD letters are different at p< 0.05 within each sampling period and N gas, error bars represent standard error.
3.5 Regression modeling of soil gas production
Least absolute shrinkage and selection operator (LASSO) regression modeling was conducted for pore-space concentrations of N2O and NO to determine the best representation of the drivers of each gas’ production in these semi-arid soils. The models developed for each gas represent the most parsimonious combination of soil chemical parameters and microbial gene abundances that can predict the concentration of N2O and NO in the soil pore-space. Model coefficients for each gas within each sampling period are reported in Table 2, and predictive capacity of the model is presented in Figure 5.
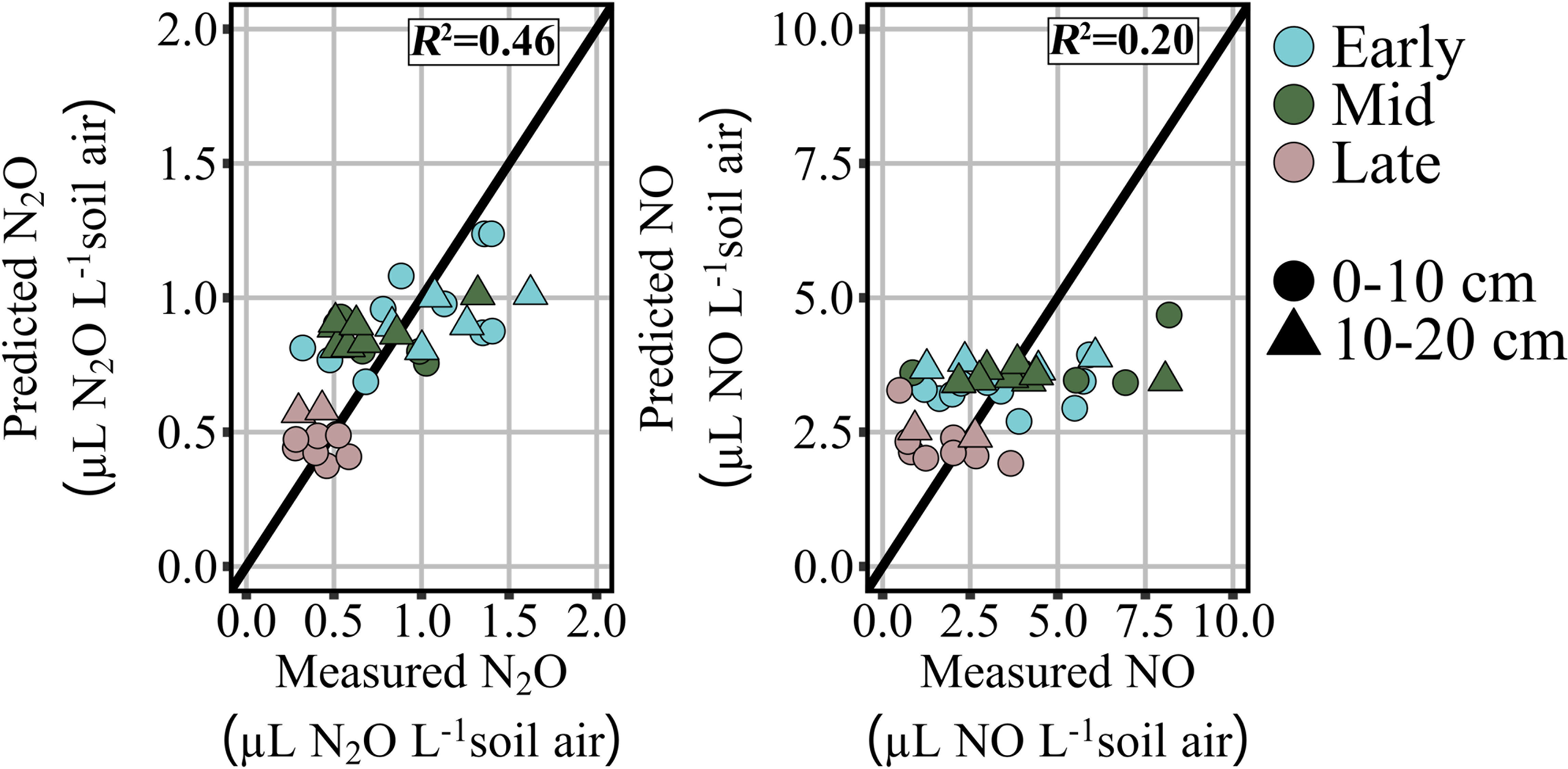
Figure 5 Least absolute shrinkage and selection operator (LASSO) regression model prediction of testing data for pore-space concentration of nitrous oxide (µL N2O L-1 soil air) and pore-space concentration of nitric oxide (µL NO L-1 soil air).
The model generated for the prediction of pore-space N2O concentration performed moderately, with an R2 of 0.46 for the measured vs. predicted concentration (Figure 5). The model coefficients for pore-space N2O included GWC, pH, and -N (Table 2). The model generated for prediction of pore-space NO performed poorly compared to N2O with an R2 of 0.20 (Figure 5). The model coefficients for pore-space NO included GWC and AOB abundance (Table 2).
4 Discussion
4.1 Variability in soil chemical composition and genetic potential
Soil chemical characteristics were analyzed across the 3-year study and separately within the final year, where variability in the final year was determined to be similar to that of the entire three-year dataset. Principal components analysis confirmed the strong effect of sampling period on soil chemical conditions, where the early- and mid-season samplings clustered together, with the late-season sampling period moderately separated. Sampling period separation was most apparent along PC2, where the extremely dry soil conditions separated the late-season sampling from the two samplings earlier in the growing season. There was no clear clustering by conservation system or N treatment, likely due to the strong effect of climate and treatment implementation which resulted in temporal clustering by sampling period. We hypothesized that soil management practices would impact soil chemical parameters and water content of the soil, which was confirmed through ANOVA, but did not result in clear patterns of separation with PCA, suggesting a larger overall effect of either climate, or another unmeasured factor.
Principal components analysis of genetic potential generally did not reveal any distinct clustering of implemented treatments or patterns in gene abundance through the growing season for the N cycling community as a whole. Interestingly, the contributions to each PC of the microbial gene abundance PCA were distinct. PC1 was associated with the overall bacterial population and the population of typical denitrifiers (nirS/K and nosZ clade II), PC2 was highly driven by the abundance of nosZ clade II which is known to be highly abundant in the soils in this study (23), and PC3 was affected by AOB abundance. The application of environmental variables to the ordination revealed a significant relationship between the variability in genetic potential and Cmin and GWC. Although no treatments clustered with these parameters, the significant relationships suggest some distal control of C and water on the genetic potential for N cycling in this semi-arid soil. However, there was clearly an additional unmeasured factor driving the variability in genetic potential observed in these semi-arid soils, although it must be noted that the relationship between soil chemical changes and microbial gene abundance may not be linear due to genetic potential including active, dormant, or even relic DNA.
4.2 Trends in nitrogen gas production in semi-arid agriculture
A strong relationship between pore-space N2O and NO and emissions from the soil surface was expected, as was previously reported in forest soils (26); however, no significant relationship was found in our study due to the sporadic nature of significant positive emissions of N2O. A reduction in N2O concentrations in the soil pore-space observed with the progression of the growing season at both depths was likely related to diminishing N resources. The same reduction was also oberved for N2O emissions but emissions and pore-space concentrations were not correlated (analysis not reported). Compared to previous measurements in agricultural fields (24) and forests (25), the concentration of NO in the soil pore-space was much greater for this semi-arid agricultural soil. We suspect that these high NO concentrations are a consequence of greater N fertilizer input than previous studies. Increases in gas production aligned with reported trends in N gas production in fertilized systems (10–12), and with seasonal variation in gas production (25). Increased NO concentrations at the mid-season sampling likely also reflect general increases in microbial activity due to increased temperatures where NO concentration wasn’t affected by the application of N fertilizer.
4.3 Relating soil chemistry and genetic potential to nitrogen gas production
It was hypothesized that denitrification was driving the production of N gas production in these semi-arid soils due to the large abundance of nosZ clade I and II genes previously observed (23). LASSO regression analysis produced a model supporting this hypothesis for N2O pore-space concentration where the model featured positive coefficients for GWC and -N, and a negative coefficient for pH. Both GWC and are known drivers of N2O production (9–12), and their inclusion in the model as positive coefficients supports the idea that the pore-space N2O measured is resulting from denitrification activities. When considering the timing of N2O emissions and the method of application (liquid UAN knifed into the soil), it is not surprising that denitrification is the main driver of excess gas production that translates to enrichment of N2O in the pore-space and non-directly to emission of N2O from the soil surface. Furthermore, the inclusion of pH in the model is key, as the effects of pH on N2O reduction capacity are well known (10). The regression model produced for N2O pore-space concentrations was moderately predictive, resulting in an R2 of 0.46 for the testing dataset. Basing the prediction of pore-space gas on such a small set of field measurements (and only five total soil chemical parameters) likely resulted in reduced predictive power. However, the moderate predictivity of the model does not dimmish the lack of genetic potential contribution to the model for N2O in the pore-space.
In contrast to the denitrification driven concentration of N2O in the soil pore-space, the LASSO regression model for NO concentration featured indicators of nitrification. Increased ammonia oxidation by AOB could have resulted in pooling of NO in the soil pore-space if it overwhelmed the oxidizing and reducing capacity of the N cycling population (13). The addition of GWC to this model indicates the controlling nature of water in this semi-arid soil. However, it must be noted that the model was poorly predictive, producing an R2 of 0.2 for the testing dataset comparison of measured and predicted pore-space NO concentrations. Due to the poor performance of the model, we cannot clearly define the production of NO as a nitrification activity, especially without the inclusion of in the model. Despite the lack of connection to genetic potential these models represent early indications of distinct N-cycle pathways producing each of these important GHGs and further represent the clear differences between N gas production in semi-arid lands and mesic systems where carbon plays a stronger role in N gas production (6, 7, 15).
4.4 Genetic potential is generally decoupled from gas production mechanisms
To further consider the interactions of soil chemistry, genetic potential, and N gas production we will examine the PP timing of N fertilizer application. Emissions and pore-space concentrations of N2O peak during the early-season sampling for the PP timing due to the recent application of N fertilizer (10–12) supporting our hypothesis that N fertilizer application would relieve the poor N content of the soil, jumpstart N cycling, and overwhelm the N2O reducing capacity of the soil. By the mid-season sampling, net consumption of N2O was recorded for the PP timing and was likely related to the loss of soil -N by plant and microbial consumption. Low inorganic-N concentrations are known to be associated with N2O consumption (50–52), and by mid-season, -N was greatly reduced compared to the early-season.
Despite the reduction in emissions, and pore-space concentrations, genetic potential for these pathways increased from the early- to mid-season samplings in the PP timing. We suspect there are two potential reasons for this seemingly antithetical outcome. First, we suspect the genetic abundance increase measured at the mid-season point may reflect the past increase in N cycle gene abundance and subsequent N cycle activity which resulted in the loss of from the soil, and reduced emissions at the mid-season sampling period. This is supported by the abundance of nosZ clade II, which increased from early- to mid-season reflecting a need for N2O-reductase in the preceding months where high N cycle activity produced more N2O and thus increased the need for this gene. The high level of N cycle activity would also thus be reflected in greater abundances of other N cycle organisms from the early- to mid-season sampling periods which we also observed.
A second potential reason for this outcome follows similar logic to the first where a significant loss of soil -N occurred between the early-and mid-season sampling, related to plant and microbial consumption. However, the loss of soil N would have selected for non-traditional N2O reducers as has been seen in several environments (26, 51, 52). In this scenario, the reduced is indicative of an active population of N cycling organisms and an active crop that is consuming soil inorganic-N. With continually reducing N resources, the plant may be selecting for organisms that can mineralize organic matter and supplement the N supply. For AOB, this would likely be a highly plausible reason for the increase in abundance as there would be fresh continually being released from the soil matrix. However, for the other genetic abundance increases, this is less plausible than the reflection of past activity and environmental selection resulting in the measured community abundance as explained above. Furthermore, due to environmental selection and plant selection, one would expect to see a decrease in genetic potential for N cycle activities between the mid- and late-season samplings, which was not reflected in this study.
The lack of reduction in genetic potential over the growing season further reflects the difficulty in using gene abundance to predict microbial activity. When evaluating microbial processes that aren’t consistently active in the study system, the stable nature of DNA in the environment and the potential for dormant or inactive microbial species, clouds the connection between genetic potential and actual environmental outcomes.
5 Conclusions
For semi-arid agricultural soils with low organic C and limited water, N cycle activity is likely exclusively driven by the application of N fertilizer, with N2O emissions peaking following application, and returning to no emissions or consumption of N2O once the fertilizer -N is depleted. This is in contrast to high concentrations of N2O and NO within the soil pore-space which indicated N cycle activity through the mid-season sampling. For producers considering the environmental impact of N fertilization and soil management, any steps taken to reduce bulk applications of N fertilizer may thus reduce gaseous N losses from the soil without negatively affecting N cycling activity within the soil matrix. Regression modeling of pore-space concentrations revealed distinct drivers of N2O and NO where N2O is associated with drivers for denitrification and NO is associated with the genetic potential for nitrification. The model for N2O was moderately predictive and would likely be greatly improved by the inclusion of measures of active microbial processes which better reflect the connection to active denitrifiers.
Consistent -N concentrations throughout the growing season in conjunction with the abundant ammonia oxidizing population indicate N mineralization as a key process to supplement low N conditions in these soils but it’s effect on N gas production is unclear. It is possible that under reduced inorganic-N conditions the crop selects for N mineralization activities which increase the concentration of NO in the pore-space through N mineralization and ammonia-oxidation. Furthermore, it was clear that genetic potential was not reflective of current microbial activity at this semi-arid study site but may indicate past N cycle activity. It would be interesting to apply this research approach in more consistent N2O producing conditions to evaluate the effectiveness of regression analyses and machine learning to predict N2O flux out of the soil. However, for semi-arid soils that often rely on microsites for anaerobic activity, there likely a host of conditions that would need further consideration to improve predictability of N2O emission.
Data availability statement
The original contributions presented in the study are included in the article/Supplementary Material. Further inquiries can be directed to the corresponding author.
Author contributions
MM: Data acquisition, data interpretation, and writing of the manuscript. KL: Conception and design, data interpretation, and writing of the manuscript. PD, TB: Writing of the manuscript. BH: Data acquisition and writing of the manuscript. TG: Data interpretation and writing of the manuscript. All authors contributed to the manuscript and approved the submitted version.
Funding
Funding for this work was provided through the Texas A&M AgriLife Research Air Quality Initiative.
Conflict of interest
The authors declare that the research was conducted in the absence of any commercial or financial relationships that could be construed as a potential conflict of interest.
Publisher’s note
All claims expressed in this article are solely those of the authors and do not necessarily represent those of their affiliated organizations, or those of the publisher, the editors and the reviewers. Any product that may be evaluated in this article, or claim that may be made by its manufacturer, is not guaranteed or endorsed by the publisher.
Supplementary material
The Supplementary Material for this article can be found online at: https://www.frontiersin.org/articles/10.3389/fsoil.2022.1050779/full#supplementary-material
References
1. Ravishankara AR, Daniel JS, Portmann RW. Nitrous oxide (N2O): The dominant ozone-depleting substance emitted in the 21st century. Science (2009) 326(5949):123–5. doi: 10.1126/science.1176985
2. IPCC. Climate change 2013: The physical science basis: Working group I contribution to the fifth assessment report of the intergovernmental panel on climate change. Stocker T, Qin D, editors. United Kingdon and New York, NY, USA: Cambridge University Press (2013).
3. Barnard R, Leadley P, Hungate B. Global change, nitrification, and denitrification: A review. Global Biogeochem Cycl (2005) 19:GB1007. doi: 10.1029/2004GB002282
4. Butterbach-Bahl K, Baggs EM, Dannenmann M, Kiese R, Zechmeister-Boltenstern S. Nitrous oxide emissions from soils: How well do we understand the processes and their controls? Philos Trans R Soc B: Biol Sci (2013) 368(1621):20130122. doi: 10.1098/rstb.2013.0122
5. Wrage-Mönnig N, Horn MA, Well R, Müller C, Velthof G, Oenema O. The role of nitrifier denitrification in the production of nitrous oxide revisited. Soil Biol Biochem (2018) 123:A3–A16. doi: 10.1016/j.soilbio.2018.03.020
6. Shah DB, Coulman GA. Kinetics of nitrification and denitrification reactions. Biotechnol Bioeng (1978) 20:43–72. doi: 10.1002/bit.260200105
7. Robertson G. Nitrification and denitrification in humid tropical ecosystems: Potential controls on nitrogen retention. Mineral nutr Trop For savanna Ecosyst (1989) 9:55–69.
8. Wu K, Chen D, Tu C, Qiu Y, Burkey KO, Reberg-Horton SC, et al. CO2 induced alterations in plant nitrate utilization and root exudation stimulate N2O emissions. Soil Biol Biochem (2017) 106:9–17. doi: 10.1016/j.soilbio.2016.11.018
9. Matson PA, McDowell WH, Townsend AR, Vitousek PM. The globalization of N deposition: Ecosystem consequences in tropical environments. Biogeochemistry (1999) 46:67–83. doi: 10.1023/A:1006152112852
10. Liu B, Frostegård Å, Bakken LR. Impaired reduction of N2O to N2 in acid soils is due to a posttranscriptional interference with the expression of nosZ. mBio (2014) 5(3):e01383–14. doi: 10.1128/mBio.01383-14
11. Samad MS, Bakken LR, Nadeem S, Clough TJ, de Klein CAM, Richards KG, et al. High-resolution denitrification kinetics in pasture soils link N2O emissions to pH, and denitrification to C mineralization. PloS One (2016) 11(3):e0151713. doi: 10.1371/journal.pone.0151713
12. Highton MP, Bakken LR, Dörsch P, Wakelin S, de Klein CAM, Molstad L, et al. Soil N2O emission potential falls along a denitrification phenotype gradient linked to differences in microbiome, rainfall and carbon availability. Soil Biol Biochem (2020) 150:108004. doi: 10.1016/j.soilbio.2020.108004
13. Caranto JD, Lancaster KM. Nitric oxide is an obligate bacterial nitrification intermediate produced by hydroxylamine oxidoreductase. Proc Natl Acad Sci (2017) 114(31):8217–22. doi: 10.1073/pnas.1704504114
14. Zumft WG. Cell biology and molecular basis of denitrification. Microbiol Mol Biol Rev (1997) 61(4):533–616. doi: 10.1128/mmbr.61.4.533-616.1997
15. Wallenstein MD, Myrold DD, Firestone M, Voytek M. Environmental controls on denitrfying communities and denitrification rates: Insights from molecular methods. Ecol Appl (2006) 16(6):2143–52. doi: 10.1890/1051-0761(2006)016[2143:ECODCA]2.0.CO;2
16. Mørkved PT, Dörsch P. And bakken, L.R. the (N2O) product ratio of nitrification and its dependence on long-term changes in soil pH. Soil Biol Biochem (2007) 39:2048–57. doi: 10.1016/j.soilbio.2007.03.006
17. Peterson GA, Halvorson AD, Havlin JL, Jones OR, Lyon DJ, Tanaka DL. Reduced tillage and increasing cropping intensity in the great plains conserves soil c. Soil tillage Res (1998) 47(3-4):207–18. doi: 10.1016/S0167-1987(98)00107-X
18. van Groenigen K-J, Bloem J, Bååth E, Boeckx P, Rousk J, Bodé S, et al. Abundance, production and stabilization of microbial biomass under conventional and reduced tillage. Soil Biol Biochem (2010) 42(1):48–55. doi: 10.1016/j.soilbio.2009.09.023
19. Geisseler D, Scow KM. Long-term effects of mineral fertilizers on soil microorganisms – a review. Soil Biol Biochem (2014) 75:54–63. doi: 10.1016/j.soilbio.2014.03.023
20. Malhi SS, Lemke R, Wang ZH, Chhabra BS. Tillage, nitrogen and crop residue effects on crop yield, nutrient uptake, soil quality, and greenhouse gas emissions. Soil Till Res (2006) 90(1-2):171–83. doi: 10.1016/j.still.2005.09.001
21. Halvorson AD, Del Grosso SJ, Reule CA. Nitrogen, tillage, and crop rotation effects on nitrous oxide emissions from irrigated cropping systems. J Environ Qual (2008) 37(4):1337–44. doi: 10.2134/jeq2007.0268
22. Smith P, Martino D, Cai Z, Gwary D, Janzen H, Kumar P, et al. Greenhouse gas mitigation in agriculture. Philos Trans R Soc Ser B Biol Sci (2008) 363(1492):789–813. doi: 10.1098/rstb.2007.2184
23. McDonald MD, Lewis KL, DeLaune PB, Boutton TW, Reed JD, Gentry TJ. Nitrous oxide consumption potential in a semi-arid agricultural system: Effects of conservation soil management and nitrogen timing on nosZ mediated N2O consumption. Front Environ Sci (2021) 9:702806. doi: 10.3389/fenvs.2021.702806
24. Gut A, Neftel A, Staffelbach T, Riedo M, Lehmann BE. Nitric oxide flux from soil during the growing season of wheat by continuous measurements of the NO soil–atmosphere concentration gradient: A process study. Plant Soil (1999) 216(1):165–80. doi: 10.1023/A:1004752104808
25. Dong F, Simon J, Rienks M, Schäffer J, von Wilpert K, Rennenberg H. Environmental effects on soil NO concentrations and root n uptake in beech and spruce forests. J Plant Nutr Soil Sci (2016) 179(2):244–56. doi: 10.1002/jpln.201500191
26. Medinets S, Gasche R, Kiese R, Rennenberg H, Butterbach-Bahl K. Seasonal dynamics and profiles of soil NO concentrations in a temperate forest. Plant Soil (2019) 445(1):335–48. doi: 10.1007/s11104-019-04305-5
27. NOAA. Noaa National Centers for Environmental Information. Climate at a glance Washington, D.C., USA: National oceanic and atmospheric administration (2021). Available at: https://www.ncdc.noaa.gov/cag/.
28. U.S. Department of Agriculture NRCS. Acuff soil series. Washington, DC: Unites States Department of Agriculture Natural Resources Conservation Service (2016). Available at: https://soilseries.sc.egov.usda.gov/OSD_Docs/A/ACUFF.html.
29. DeLaune PB, Sij JW, Park SC, Krutz LJ. Cotton production as affected by irrigation level and transitioning tillage systems. Agron J (2012) 104(4):991–5. doi: 10.2134/agronj2011.0420
30. Dorich RA, Nelson DW. Direct colorimetric measurement of ammonium in potassium chloride extracts of soils. Soil Sci Soc Am J (1983) 47(4):833–6. doi: 10.2136/sssaj1983.03615995004700040042x
31. Franzluebbers AJ. Should soil testing services measure soil biological activity? Agric Environ Lett (2016) 1(1):1–5. doi: 10.2134/ael2015.11.0009
32. Schofield RK, Taylor AW. The measurement of soil pH. Soil Sci Soc Am J (1955) 19:164–7. doi: 10.2136/sssaj1955.03615995001900020013x
33. Fierer N, Jackson JA, Vilgalys R, Jackson RB. Assessment of soil microbial community structure by use of taxon-specific quantitative PCR assays. Appl Environ Microbiol (2005) 71(7):4117–20. doi: 10.1128/AEM.71.7.4117-4120.2005
34. Rotthauwe JH, Witzel KP, Liesack W. The ammonia monooxygenase structural gene amoA as a functional marker: Molecular fine-scale analysis of natural ammonia-oxidizing populations. Appl Environ Microbiol (1997) 63(12):4704–12. doi: 10.1128/aem.63.12.4704-4712.1997
35. Throbäck IN, Enwall K, Jarvis A, Hallin S. Reassessing PCR primers targeting nirS, nirK and nosZ genes for community surveys of denitrifying bacteria with DGGE. FEMS Microbiol Ecol (2004) 49(3):401–17. doi: 10.1016/j.femsec.2004.04.011
36. Henry S, Baudoin E, López-Gutiérrez JC, Martin-Laurent F, Brauman A, Philippot L. Quantification of denitrifying bacteria in soils by nirK gene targeted real-time pcr. J Microbiol Methods (2004) 59(3):327–35. doi: 10.1016/j.mimet.2004.07.002
37. Chen Z, Luo X, Hu R, Wu M, Wu J, Wei W. Impact of long-term fertilization on the composition of denitrifier communities based on nitrite reductase analyses in a paddy soil. Microb Ecol (2010) 60(4):850–61. doi: 10.1007/s00248-010-9700-z
38. Henry S, Bru D, Stres B, Hallet S, Philippot L. Quantitative detection of the nosz; gene, encoding nitrous oxide reductase, and comparison of the abundances of 16S rRNA, narG, nirK, and nosZ genes in soils. Appl Environ Microbiol (2006) 72(8):5181. doi: 10.1128/AEM.00231-06
39. Jones CM, Graf DR, Bru D, Philippot L, Hallin S. The unaccounted yet abundant nitrous oxide-reducing microbial community: A potential nitrous oxide sink. ISME J (2013) 7(2):417. doi: 10.1038/ismej.2012.125
40. Hartman LJ, Coyne SR, Norwood DA. Development of a novel internal positive control for taqman® based assays. Mol Cell Probes (2005) 19(1):51–9. doi: 10.1016/j.mcp.2004.07.006
41. Zhou S, Hou Z, Li N, Qin Q. Development of a sybr green I real-time PCR for quantitative detection of vibrio alginolyticus in seawater and seafood. J Appl Microbiol (2007) 103(5):1897–906. doi: 10.1111/j.1365-2672.2007.03420.x
42. McDonald MD, Lewis KL, Gentry TJ, DeLaune PB. Soil pore space gas probes for use in agricultural research. Soil Secur (2021) 5:100015. doi: 10.1016/j.soisec.2021.100015
43. Brummell ME, Farrell RE, Siciliano SD. Greenhouse gas soil production and surface fluxes at a high Arctic polar oasis. Soil Biol Biochem (2012) 52:1–12. doi: 10.1016/j.soilbio.2012.03.019
44. Bates D, Maechler M, Bolker B, Walker S. Fitting linear mixed-effects models using lme4. J Stat Softw (2015) 67:1–48. doi: 10.48550/arXiv.1406.5823
45. R-Core-Team. R: A language and environment for statistical computing. Vienna, Austria: R Foundation for Statistical Computing (2019). Available at: https://www.R-project.org/.
46. Kuhn M. Caret: Classification and regression training. R package version 6.0-93 (2022). Available at: https://CRAN.R-project.org/package=caret (Accessed 2022).
47. Oksanen J, Simpson GL, Blanchet FG, Kindt R, Legendre P, Minchin PR, et al. Vegan: Community ecology package. R package version 2.6-2 (2022). Available at: https://CRAN.R-project.org/package=vegan (Accessed 2022).
49. Friedman J, Hastie T, Tibshirani R. Regularization paths for generalized linear models via coordinate descent. J Stat Softw (2010) 33:1–22.
50. Butterbach-Bahl K, Gasche R, Huber C, Kreutzer K, Papen H. Impact of N-input by wet deposition on N-trace gas fluxes and CH4-oxidation in spruce forest ecosystems of the temperate zone in Europe. Atmos Environ (1998) 32(3):559–64. doi: 10.1016/S1352-2310(97)00234-3
51. Rosenkranz P, Brüggemann N, Papen H, Xu Z, Seufert G, Butterbach-Bahl K. N2O, NO and CH4 exchange, and microbial N turnover over a Mediterranean pine forest soil. Biogeosciences (2006) 3(2):121–33. doi: 10.5194/bgd-2-673-2005
Keywords: nitrous oxide, pore-space gases, microbial abundance, semi-arid soils, no-tillage, cover crop
Citation: McDonald MD, Lewis KL, DeLaune PB, Hux BA, Boutton TW and Gentry TJ (2023) Nitrogen fertilizer driven nitrous and nitric oxide production is decoupled from microbial genetic potential in low carbon, semi-arid soil. Front. Soil Sci. 2:1050779. doi: 10.3389/fsoil.2022.1050779
Received: 22 September 2022; Accepted: 12 December 2022;
Published: 10 January 2023.
Edited by:
Jacobo Arango, International Center for Tropical Agriculture (CIAT), ColombiaReviewed by:
Eduardo Vázquez, Polytechnic University of Madrid, SpainMaría B. Villamil, University of Illinois at Urbana-Champaign, United States
Copyright © 2023 McDonald, Lewis, DeLaune, Hux, Boutton and Gentry. This is an open-access article distributed under the terms of the Creative Commons Attribution License (CC BY). The use, distribution or reproduction in other forums is permitted, provided the original author(s) and the copyright owner(s) are credited and that the original publication in this journal is cited, in accordance with accepted academic practice. No use, distribution or reproduction is permitted which does not comply with these terms.
*Correspondence: Mark D. McDonald, mcdonaldm@anl.gov