- 1Department of Agroecology, Aarhus University, Tjele, Denmark
- 2Geological Survey of Denmark and Greenland (GEUS), Copenhagen, Denmark
- 3USDA– Agricultural Research Service, Grassland, Soil & Water Research Laboratory, Temple, TX, United States
- 4Department of Science and Environment, Roskilde University, Roskilde, Denmark
- 5Department of Geoscience and Natural Resource Management, University of Copenhagen, Copenhagen, Denmark
- 6Colorado School of Public Health, Center for Global Health, Aurora, CO, United States
Soil provides essential ecosystem services sustaining and improving human life, but mapping soil functions is an ongoing challenge. Denmark has a long history of carrying out soil assessments − originally in order to determine tax revenues for the king, and, more recently, for aiding policymakers and farmers. This knowledge has supported the development of intensive agricultural systems while maintaining the provision of ecosystem services (e.g., clean water). Getting an overview of historical soil surveys and pedological mapping approaches can generate useful information for mapping soil, identifying gaps and proposing directions for future research. In this review, we explore the evolution of soil and environmental inventories, the historical development of soil mapping methods, and how these factors contributed to a better spatial understanding of soil functions. Specifically, we discuss soil functions related to water regulation (e.g., drainage, groundwater and water surface interactions, water table), water filtering (e.g., nitrogen leaching), carbon sequestration (e.g., peatlands), agricultural production (e.g., land suitability, wheat yields), and threats related to soil degradation (e.g., soil erosion). Denmark has benefitted from a government-coordinated approach, promoting detailed and systematic national soil surveys and environmental monitoring programmes. The large databases produced in the surveys formed the basis for mapping several soil properties and functions at increasingly high resolutions over the last many years based on developments in machine learning. In contrast to methodological advances in soil mapping and relevant contributions to pedometric research, we identified a lack of spatial information on soil biodiversity. Detailed spatial information about soil functions is essential to address global issues, such as climate change, food security and water security, and the experience of mapping soil functions in Denmark can be a source of inspiration to other parts of the world.
1 Introduction
Soil provides ecosystem services for human well-being (1–3) and constitutes a fulcrum for global issues such as food security and climate change mitigation (4–6). Nevertheless, soils remain neglected as a resource in global discussions on these issues, and do not even receive a mention in the United Nations (UN) Sustainable Development Goals (SDGs) (7). It is therefore crucial for soil researchers to promote the importance of soil in supporting human life and well-being to policymakers and society. Soil mapping can be an easy way to communicate soil science to a wider audience (8), and technological advances have enabled the development of reproducible digital soil maps based on globally available data (9). However, most studies in digital soil mapping (DSM) have focused on soil physico-chemical properties (e.g., soil texture) (10). Although these maps improve our knowledge about the spatial distribution of soil properties, they are unfamiliar to the public, which may limit a wider interest and recognition of the importance of soil functions to human well-being. Soil functions are characterized by the complex interactions between physical, chemical and biological processes in soil (11), resulting in the provision of ecosystem services such as clean water. Carré et al. (12) argued that digital soil assessments (DSA) aiming to map soil functions (e.g., water filtration, carbon sequestration) would provide more suitable information for stakeholders than maps of soil properties, as in DSM.
Moving from DSM to DSA advances our understanding of soil functions and their influence on human well-being. However, the use of DSA for evaluating soil functions and soil threats on a spatial scale is still limited, which is a challenge for soil science and the future of pedometrics (12, 13). The well-established knowledge on methods for mapping soil properties can be useful for mapping soil functions. However, to understand and map soil functions, researchers need to move beyond the “stable” soil solid phase and venture into the highly dynamic and complex interactions between soil constituents, soil water, chemical processes, gases, soil organisms, and life at the surface. The challenge here is not so much the development of new methods as the additional environmental data acquisition, which depends on funding, mainly from the public sector. The focus on the soil solid phase has led to an information gap for soil water dynamics, gas transport, and soil biodiversity, making it difficult or impossible to map soil functions worldwide. Some recent studies have mapped specific soil functions at local or regional scales (14, 15), but information and studies at national scales are scarce (16).
Coordinated national soil monitoring and assessment are essential for achieving global objectives (i.e., SGDs). Global initiatives such as the GlobalSoilMap project aim to provide high-resolution soil information at the global level (17). However, data availability in developing countries and restricted access to databases in developed countries are still a hindrance to mapping soil functions at national levels and hence also for a global overview. Denmark has a long history of collecting environmental information to support government decisions. Early soil assessments in Denmark were mostly subjective, based on human observations and judgments, as a result of shared knowledge among farmers and research institutions (18).
This changed in the 1970s where objective sampling strategies and laboratory analyses, databases and a soil information system replaced the subjective approaches in new national soil surveys aiming to optimize agricultural yields and protect the best soils (19). In addition, since the 1980s, Danish governments have allocated resources to continually monitor soil and environmental conditions through detailed national field surveys. These efforts have provided large amounts of information related to water dynamics, nutrient cycling and land management. These datasets and the subsequent development of DSM techniques made it possible to map several soil functions related to water regulation, filtration of contaminants, carbon (C) sequestration, and biomass production.
In this review, we explore the linkages between government initiatives to finance soil inventories and environmental monitoring programmes, and the development of soil mapping in Denmark. Specifically, we discuss the evolution of soil and environmental inventories and the historical development of soil mapping approaches from conventional to digital. We also investigate how these factors contributed to a better understanding of soil functions such as C sequestration, water dynamics, agricultural production, risks of pollution from fertilizers and pesticides, and the soil threats related to land degradation. We discuss research gaps and future directions to improve our knowledge of the spatial distributions of soil functions, and how this knowledge may aid decision-makers and farmers. We believe that experiences from mapping functions in Denmark can be a source of information and inspiration to other parts of the world.
2 Soil observations and environmental data
Environmental investigations and monitoring programmes in Denmark have generally aimed to support government decisions, but the motivation and purposes have changed over time. The historical developments of Danish soil assessment and sampling have been summarized by Madsen et al. (18). During the last four centuries, we can identify three soil mapping periods, each with a different purpose. From 1688 to 1844 the main objective for soil surveys was to form a basis for taxation, and from 1844 to 1975 the purpose was to value land, increase productivity and expand agricultural areas. From 1975 to the present, the purposes of soil mapping efforts have expanded in scope. Mainly, they aim to provide information for policy-makers and farmers, but they also give input to data-driven models to elucidate soil processes, information for land use planning, regulations regarding the application of fertilizers and pesticides, and ecosystem restoration efforts. Furthermore, the technologies used in the soil surveys and monitoring programmes have developed over time. In the 1970s, objective reproducible methods including standardized field methods as well as chemical and physical laboratory measurements replaced the use of subjective soil assessments. Since the beginning of the 21st century, proximal sensors have complemented these methods.
2.1 Soil information
The history of soil mapping in Denmark shows how the evolution of soil classification systems and the gradual incorporation of additional soil and environmental data helped to improve the knowledge on Danish soils. The work on the first soil inventory in Denmark started in 1688 when the King called for the Land Register to provide the basis for taxation. The surveyors, therefore, evaluated the land based on potential crop yields rather than specific soil characteristics. Four experienced farmers were responsible for evaluating the land in each parish. The surveys did not use standardized methods, so the classification varied between administrative regions. In Jutland, which comprises the largest part of Denmark, the soils were classified ranging from “most fertile” to “poorest”. According to this classification, a certain number of area units (1 tønde land [tdl] = 0.53 ha) was associated with the tax value of one barrel of “hard grain” [th] (i.e., barley or rye). For instance, the poorest soils had a value of 1 th per 16 tdl, while the most fertile soils had a value of 1 th per 2 tdl. The register did not map the classes but instead listed the values for the fields in each parish.
In the 18th century, the Land Register produced the first soil maps at 1:4,000 scale for two regions in Denmark, and the land evaluation now incorporated soil characteristics, such as “good mull and sand for rye and barley”. These new surveys laid the basis for the Great Danish Land Register of 1844, which resulted in a national land value map for taxation (20). The Great Danish Land Register was a milestone in soil inventories, in which the land evaluation explicitly considered soil characteristics in addition to yield potentials. The new surveys adopted a characterization system of grades from 0 to 24. The “optimal soil” had a grade of 24 and represented a reference of suitability for farming in wet and dry conditions. This reference soil, located in Karlslunde just southeast of Copenhagen, had a thick topsoil (> 31 cm) with a mixture of clay and mull, a clayey subsoil, and a gentle south-facing slope. Later, four soils with grades 20, 16, 10, and 3 were added to the reference list of “normal soils”. The soil evaluation relied on a combination of factors described by the surveyors in the field. The land register formed the basis for taxation until the end of the 19th century. However, due to agricultural advances and management (e.g., tile drainage), the productivity of the land started to diverge from the assigned values. As a consequence, from 1903 onwards, new tax legislation used the commercial value of the land as a replacement (18).
A new National Land Assessment was conducted in 1949 to provide a comprehensive overview of the agricultural lands in Denmark. The soils were rated on a 0–100 point scale for their natural characteristics and crop yields, and mineral soils were classified into seven classes ranging from heavy clay (50–90 points) to gravelly sand (0–30 points) (21). The rating was partly based on the presence of mull, water retention, drainage conditions, soil colour, and the presence of plant roots. Despite advances in describing soil characteristics, soil sampling and classification still relied on human judgment and support from experienced farmers. The surveys comprised assessments from more than 25,000 locations and enabled the production of top- and subsoil textural maps. In 1970, the Land Assessment Commission recommended a new national survey, but the government considered the plan too expensive.
However, in 1975, the Danish government realized that the information on soil in agricultural land was insufficient for effective land use planning. This motivated the introduction of the Danish Soil Classification (DSC), and between 1975 and 1980, a new campaign collected about 36,000 soil samples (the DSC database) and produced maps of textural classes at a scale of 1:50,000 (18, 22). For the first time, the survey used laboratory measurements of texture, soil organic carbon (SOC) and CaCO3. The data was stored in databases and a GIS-based soil information system was developed (23, 24). The classification system comprised 12 soil types, later simplified to eight colour codes based on soil texture and organic matter and calcium carbonate content. The soil classes were manually delineated based on knowledge of soil variation from local agricultural advisors and supported by detailed geological surface maps. The soil survey also published 320 paper map sheets at 1:50,000 scale. These maps also provided information on the surface geology, slope and drainage.
Additional inventories assembled in the 1980s further contributed to the knowledge on soil variability in Denmark (25, 26). During the construction of a national gas pipeline between 1981 and 1984, about 8,500 soil profile investigations were carried out along two transects [NSGP database (19)]. In the same period, a survey of potentially acid sulfate soils collected samples from more than 8,000 augering sites [Ochre Classification database (27)]. Lastly, in 1987−1989, a nationwide programme to study nitrogen (N) dynamics described and sampled about 850 soil profiles located in a 7-km grid [Danish Soil Monitoring Grid or DSMG database (18, 22)]. As the DSMG forms the basis for a monitoring programme for SOC, subsequent campaigns have collected augering samples in 1997, 2009 and 2019 (28).
Until the 1990s, most soil investigations focused on agricultural areas. However, in the beginning of the 1990s, a Danish Forest Site Classification system was developed for mapping soil prior to afforestation to ensure the long-term health of the forest. The surveys included one auger boring per hectare and a soil profile description for each soil type present in the area (29). Later soil surveys for afforestation projects have also employed the classification system developed for forest soils [e.g (30)]. As a consequence of the Kyoto protocol, a national soil survey of Danish peatlands (SINKS) was initiated, and about 10,000 auger samples were originally collected between 2009 and 2010 in order to map the distribution of organic wetland soils (31). Under the European Union (EU) structure, soil samples were also collected across Denmark to improve the LUCAS database over time (32). Also, from Denmark’s many soil profile- related experiences, the nation took a leading role in the design of a European Soil Profile Analytical Database (SPADE 18) and a harmonized soil map for the EU at a scale of 1:1,000,000 (33). As a result of several soil survey programmes, Denmark has one of the most representative (~ 2 soil sampling locations per km2) and detailed national soil databases in the world. The Danish soil information is presently under a process of harmonization following the existing regulations and directives for Open Databases (https://inspire.ec.europa.eu/data-specifications/2892).
The use of proximal sensors opened up for the opportunity to obtain more information about soil characteristics [e.g (34)], and visible near-infrared (Vis-NIR) spectroscopy has been successfully applied, showing great potential in supplementing wet chemistry for estimating soil properties in Denmark (35). The Danish Vis-NIR spectral database consists of measurements from about 7,000 soil samples from the DSMG investigations (1986 and 2009), and from surface and profile samples from several field surveys, thus covering the different soil types representative for the country and different spatial scales. Data was gathered using different sensor types in the laboratory and in the field, including bench-top, portable and on-the-go systems.
2.2 Environmental data
Denmark started to monitor the environment to understand and manage problems related to agricultural areas and water pollution, and later to protect natural habitats. This resulted in spatial and temporal representative environmental datasets related to land use and management, water dynamics quality, and land surface characteristics at high resolution.
Agricultural lands cover about two thirds of Denmark, and information about land use and crops has been available at the regional level since 1848. Denmark joined EF (European Community) in 1972 and the Common Agricultural Policy in 1996. Field blocks were introduced in Denmark − a dataset containing information on the maximum eligible agricultural land use as defined by the EU. Field blocks are digitized GIS polygons, and a block encompasses one to approximately 15 fields delimited by stable landscape structures such as roads and fences (36, 37). This dataset was further detailed in 2011, when farmers started to register the field boundaries and crop types in each field every year (within a block). This resulted in a detailed land use database, which has the potential to be used in several research areas. For instance, this dataset was one of the main components in the creation of the present land cover map for Denmark (38). Furthermore, national surveys with the Light Detection and Ranging (LiDAR) sensors in 2006/07 (1.6-m), 2014/15 (0.4-m), 2018/22 (0.4-m) provide information on topography and vegetation at high resolutions (39). The LiDAR-based DTM products (DHM Terræn) are freely available (https://dataforsyningen.dk/). Recently, drones with high-resolution spectral cameras and proximal sensors have started to be employed to evaluate land, vegetation dynamics, and sub-surface soil properties at high resolution.
The Danish government funded several programmes to monitor water quality and dynamics to inform protection efforts for the extensive coastline as well as groundwater, which is the main source of drinking water. A national groundwater monitoring programme (GRUMO) was established in 1988, covering 74 well catchment areas and six small agricultural catchments with more than 1,500 screens (i.e., portions of the well that serve as water intake at different depths) (40). The water is analysed in these locations, mostly annually, for main components, inorganic trace elements, organic micro-pollutants, and pesticides. Additional water samples from about 10,000 wells across Denmark are analysed every three to five years. Relevant parameters on geology, groundwater quality and water levels at wells are collected in the national open-access well database, JUPITER [https://data.geus.dk/JupiterWWW (41)].
The lack of knowledge about forests motivated the creation of the Forest Tree Species Trial Plots in the 1960s, with each containing even-aged single-species trees distributed in clusters across 13 sites with different characteristics. The 13 sites were used to compare growth and health of 12 tree species in typical site types across Denmark: oceanic climate and sandy outwash plains in the west, continental climate and clayey soils in the east, and intermediate climate and gravelly tills in the central regions (42). The studies on tree species increased and a total of 2,130 forested plots were measured during the Danish National Forest Inventory (NFI) in 2006/2007 (43). The Danish NFI is based on a 2 × 2 km grid across the Danish land surface. In each grid cell, a cluster of four circular plots for measuring forest factors (e.g., growing stock, biomass, and total forest C stock) is placed in the corners of a 200 × 200 m square.
3 Methodological development and covariates for soil mapping
The availability of soil and environmental data has supported several soil mapping studies over the last decades, and it has also allowed Danish researchers to develop methodological approaches to improve global knowledge on soil mapping. Soil is the product of multiple environmental factors (44), and the evolution of soil mapping is linked to the development of approaches that use local environmental characteristics to predict the occurrence of soil types and properties. Until the 21st century, the environmental data for mapping soils in Denmark only comprised topographic, landscape and geological maps. However, advances in data processing and digital tools provided the opportunity to develop and use multiple variables to explain soil variability. McBratney et al. (9) introduced the SCORPAN framework for soil mapping, which treats soil characteristics at a certain point as a function of soil (s), climate (c), organisms (o), relief (r), parent materials (p), age (a) and spatial location (n). The history of soil mapping in Denmark is intrinsically linked to the development and use of explanatory variables, and we can identify three generations of soil maps since 1688 (Figure 1).
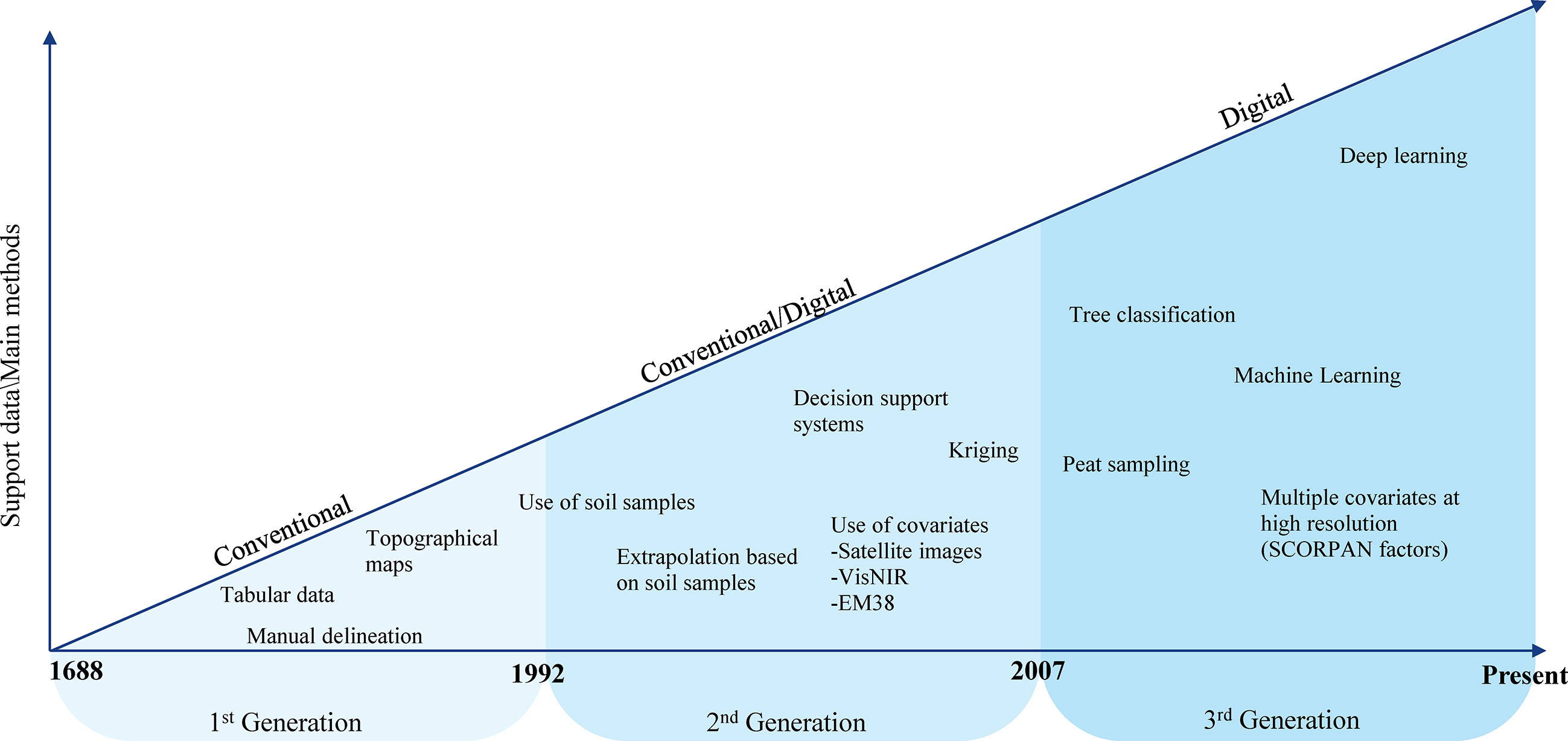
Figure 1 Evolution of soil mapping in Denmark highlighting the increase in the availability of support data and the primary use of methods across the three generations of soil maps generated at national level.
The first Danish land and soil maps were created by farmers, agronomists, and governmental staff using conventional mapping approaches. This method is based on the soil surveyor’s knowledge of the local environment and subjectivity is part of the process. The land value map of 1844 (values 1-24) is based on soil texture, and despite the coarse scale we can identify good soils in the east and sandy soils in the west (Figure 2A). Later surveys also mapped soil textural classes with conventional methods until the initiation of the Danish Soil Classification project in 1975 (section 2.1.). This project produced a national soil map showing eight textural classes for the topsoil (0–20 cm) [Figure 2B (18)]. The use of pedologic soil types in Denmark started with the development of the world soil map at 1:5,000,000 scale (43), and the later 1984 European edition at 1:1,000,000 scale only presented very general information on Danish soils (46). At the same time, Jacobsen (45) developed an alternative map of Denmark at 1:2,000,000 scale showing 14 soil types according to the FAO-UNESCO legend. These studies used maps of geology, landscape morphology and climate to map soil types. With the publication of the revised FAO legend (48), Madsen (26) produced a new map of Danish soil classes at 1:1,000,000 scale. The new map included information from the major soil profile investigations conducted since the previous efforts (Section 2.1.) and used maps of landscape types, wetlands, textural classes from the Danish Soil Classification and potentially acid sulfate soils. While the surveys prior to the 20th century relied on field judgment, later surveys incorporated topographic and geological maps. The two sources of information arguably provide information on the relief [r] and parent materials (p). However, the surveyors did not seek to quantify the relationships between these factors and the soil.
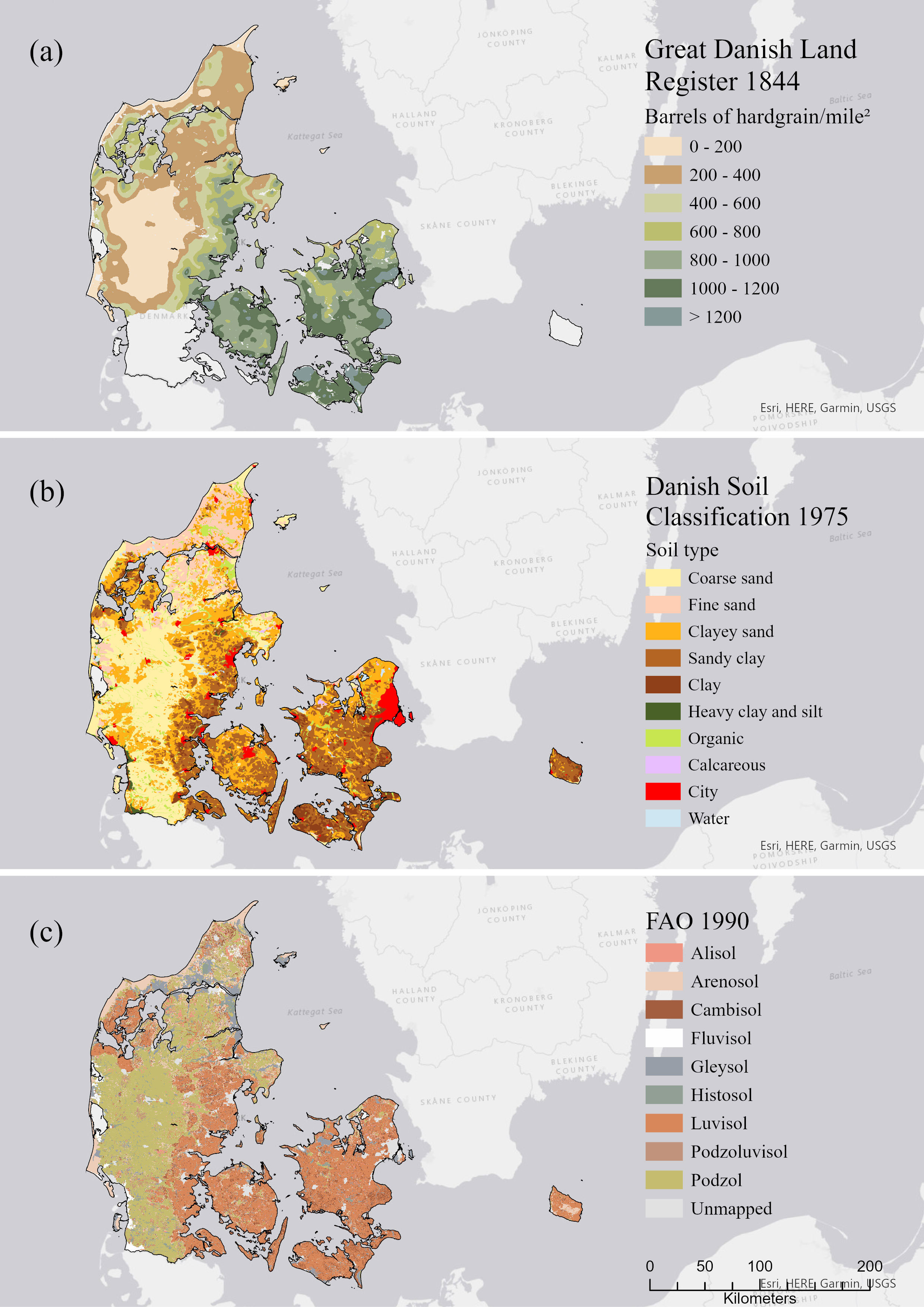
Figure 2 The maps represent: (A) the land value used to tax farmers in 1844, (B) soil textural classes developed by the Danish Soil Classification in 1975, and (C) the soil types according to the FAO legend from 1990.
The second generation of maps marked a transition from conventional mapping approaches to the use of DSM techniques. This process enabled the quantitative use of explanatory variables that can give additional insights on the soil-forming factors. The development of data-processing techniques to handle large numbers of spatial covariates and the application of mathematical models helped to move from the subjectivity of conventional mapping approaches (e.g., delineation by hand) to more deterministic and reproducible methods. The use of soil samples for mapping soil classes (18) and the application of geostatistical approaches clearly illustrate this transition and emphasize the importance of soil inventories. The first map of soil organic C at the national level resulted from a simple statistical upscaling of soil measurements to polygons from existing maps of land use and texture classes (49). However, the map provided no information on the spatial variation within the polygons. Greve et al. (50) made the first effort to change from polygon to raster format when they mapped soil texture and organic matter at 250-m resolution using kriging. They first stratified the landscape using maps of landscape types, land use and wetlands, and fitted individual variograms for each stratum. This study marks a transition to the third generation of soil maps.
The third generation of soil maps is characterized by the development and application of DSM techniques to predict soil classes and properties using multiple covariates. This period witnessed the worldwide development of the DSM framework, and Denmark contributed to the development of methods and covariates. The first studies in Denmark used geostatistical methods, and the introduction of machine learning algorithms in the form of decision trees followed soon after. The increase in computer performance and advances in modeling approaches allowed researchers to train models that combined multiple explanatory variables with higher resolutions, which improved the capacity to predict soil characteristics based on the SCORPAN framework. For instance, the use of covariates derived from remote sensing data (e.g., vegetation indices, bare soil composite rasters) has helped improve the prediction of soil properties. Adhikari et al. (51) mapped the distribution of soil types at 30-m resolution according to the FAO legend using a machine learning model and data from 1,171 soil profiles (Figure 2C). In addition to the previously available maps of parent materials, land use and wetlands, this new study also used ten covariates derived from a digital elevation model and clay contents mapped with DSM techniques. Several soil properties maps were produced in this period: clay, silt, and fine and coarse sand [Figures 3A–D (52)], SOC content [Figure 3E (53)], pH [Figure 3F (54)] and bulk density [Figure 3I (54)]. Another attempt to map the nationwide spatial variability of soil parameters is found in Balstrøm et al. (55), describing variations in pH influenced by geology and land using spatial autocorrelation methods (Local Moran’s I and Gi* statistics). A recent study also explored the spatial distributions of oxalate-extractable aluminium (Figure 3G) and oxalate-extractable iron (Figure 3H) (56, 57). The use of Vis-NIR spectroscopy, electromagnetic induction and aerial imagery allowed the development of proxies and spatial covariates to predict SOC, soil classes and soil texture at a high resolution (up to 1.6-m) at field scale (35, 58, 59). The use of machine learning algorithms makes it possible to quantify prediction uncertainties, indicating the locations where the model cannot reliably predict soil properties. This is important to improve soil maps in the future and orient new soil surveys, and it is one of the major advantages of machine learning compared to conventional soil mapping.
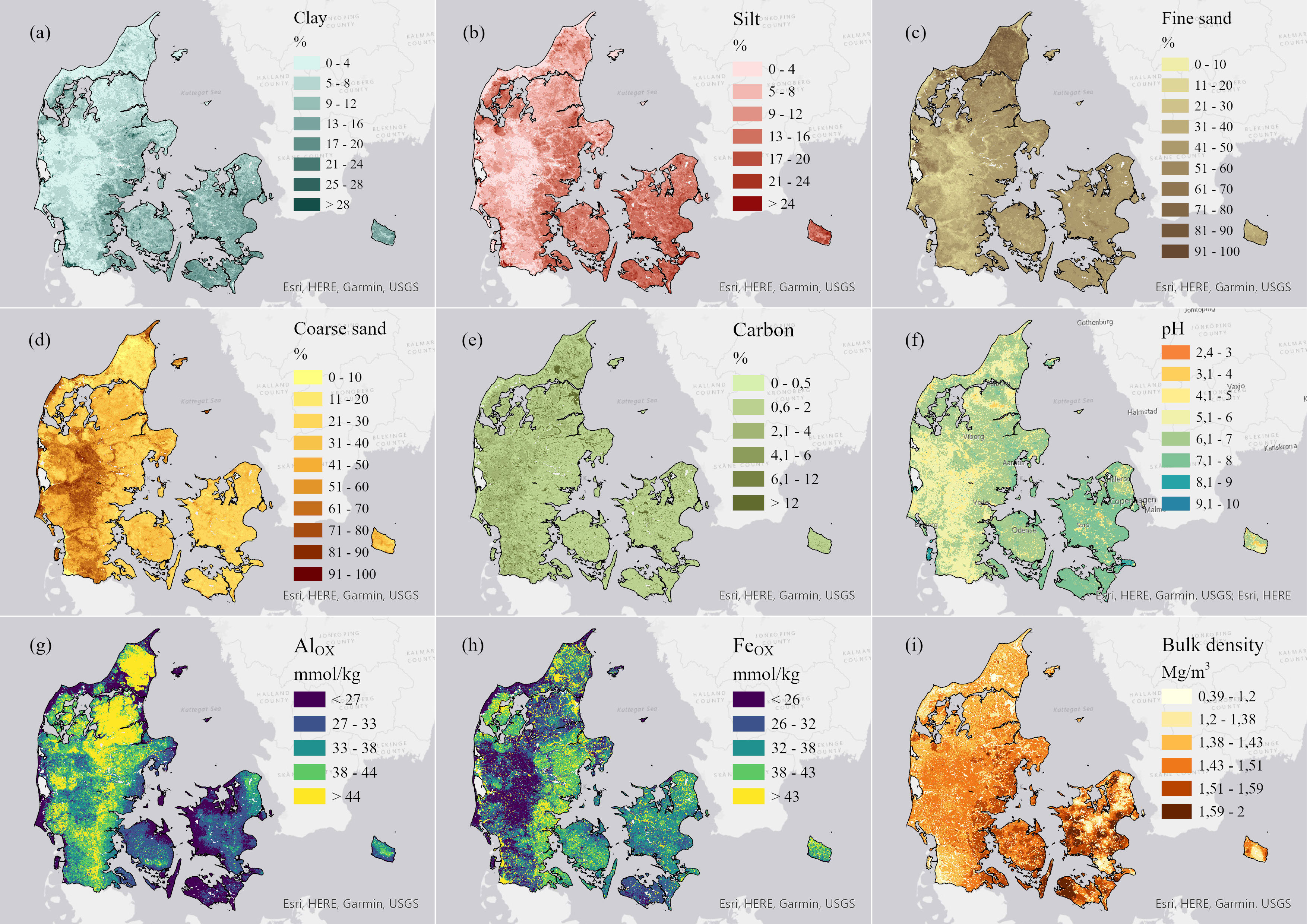
Figure 3 Maps of soil properties for Denmark for the topsoil layer: (A) clay, (B) silt, (C) fine sand, (D) coarse sand, (E) soil organic carbon content, (F) soil pH, (G) oxalate-extractable aluminium, (H) oxalate-extractable iron, (I) soil bulk density.
The soil maps produced in the last two decades have helped to improve our knowledge on the spatial distribution of soil properties in Denmark. However, they also contributed to mapping efforts for soil functions such as water regulation, filtering of nutrients and contaminants, food and biomass production and C sequestration. In the next sections, we will explore the multiple activities towards mapping soil functions and threats using soil property and soil class maps described in the previous sections, and the importance of these maps to inform the government, farmers and society.
4 Mapping soil functions
Denmark was one of the first countries to have a national map of soil properties at high resolution, and several subsequent studies used these maps as a basis for mapping soil functions. This represented the transition from DSM to DSA in Denmark. In the following subsections, we will demonstrate the evolution and the main factors that contributed to the process of mapping soil functions in Denmark. In addition, we will discuss how stakeholders have used the soil function maps, the potential for additional uses and their significance for the achievement of SDGs.
4.1 Water regulation
Understanding the water dynamics in the soil is crucial for the economy and human well-being, especially in Denmark, where artificial drainage is a prerequisite for agricultural production in many places. At the same time, the groundwater provides clean water for consumption and is a source of irrigation in areas with sandy soils. Artificial drainage played a crucial role during the agricultural expansion and intensification in the 19th century in Denmark and the cultivation of wetland soils in the 20th century (60). Today, about 50% of the arable land in Denmark is artificially drained (61).
Agricultural drainage, combined with the increasing use of fertilizers and pesticides in the 20th century, increased the risk of groundwater contamination and eutrophication in surface waters. In the 1980s, these risks created a need to monitor water quantity and quality (62). New monitoring programmes therefore aimed to collect information on water dynamics in streams and groundwater to assess the risks of nutrient and pesticide leaching. The efforts included a comprehensive network of wells and gauging stations to collect information on water quality and flow. However, these monitoring efforts did not provide sufficient information for an understanding of the nutrient transport dynamics in the soil. This need motivated the establishment of the national DSMG programme (Section 2), and additional programmes focused on water permeability in representative soils across Denmark (63), although his data was available only for specific sites. With the development of DSM, it became possible to map water dynamics in more detail. New studies therefore aimed to map natural soil drainage (64, 65), artificially drained areas (66), macropore flow (67), interactions between the groundwater and surface waters (68), and variations in the groundwater table (69).
Møller et al. (64) mapped the five soil drainage classes for Denmark using information from 1,702 soil profiles from the gas pipeline investigation and DSMG database in a decision tree model. Later, Beucher et al. (65) used artificial neural networks to map soil drainage classes and found that their predictive accuracy was comparable to decision tree classification. Moderately drained soils are the dominant drainage class in the loamy till areas in eastern Denmark, while well-drained and very well-drained soils mainly occur in the sandy upland soils in the west.
Although soil drainage classes give a good indication of the expected water saturation in the soil, artificial drainage often overshadows the patterns of natural soil drainage. Olesen (61) compiled 745 field observations of the presence or absence of artificial drainage across Denmark. Møller (66) used this dataset and an ensemble of 77 machine learning models to map artificially drained areas for Denmark and the associated uncertainties. The models predicted a high presence of artificial drainage in the loamy tills of eastern Denmark and in the large wetland areas of northern Denmark. However, even with this information, the location of the individual drainage pipes is still often poorly documented or unknown. The information on drainage pipe locations can help farmers repair damaged pipes and install new systems, but it can also inform the establishment of mitigation measures to remove nutrients, pesticides and pathogens from the drainage water. Conventional methods for mapping drainage pipes include probing or the use of trenching equipment, which are time-consuming and invasive. Several studies assessing the use of proximal soil sensors were conducted in Fensholt, Denmark (Figure 4D). In particular, Koganti et al. (70) showed that ground-penetrating radar (GPR) was potentially useful to localize drainage pipes, while a magnetic gradiometer proved ineffective. Furthermore, proximal sensors have a limited capacity to cover large areas, and efforts are therefore underway to test the suitability of unmanned aerial vehicle (UAV) imagery for this purpose. Koganti et al. (71) found that the best approach was to use both GPR and UAV techniques when possible, as they can provide complementary information for mapping drainage pipes. They also provided recommendations for the timing of sensor surveys, while they argued that future research should focus on developing a formal framework with specific guidelines for different soil types.
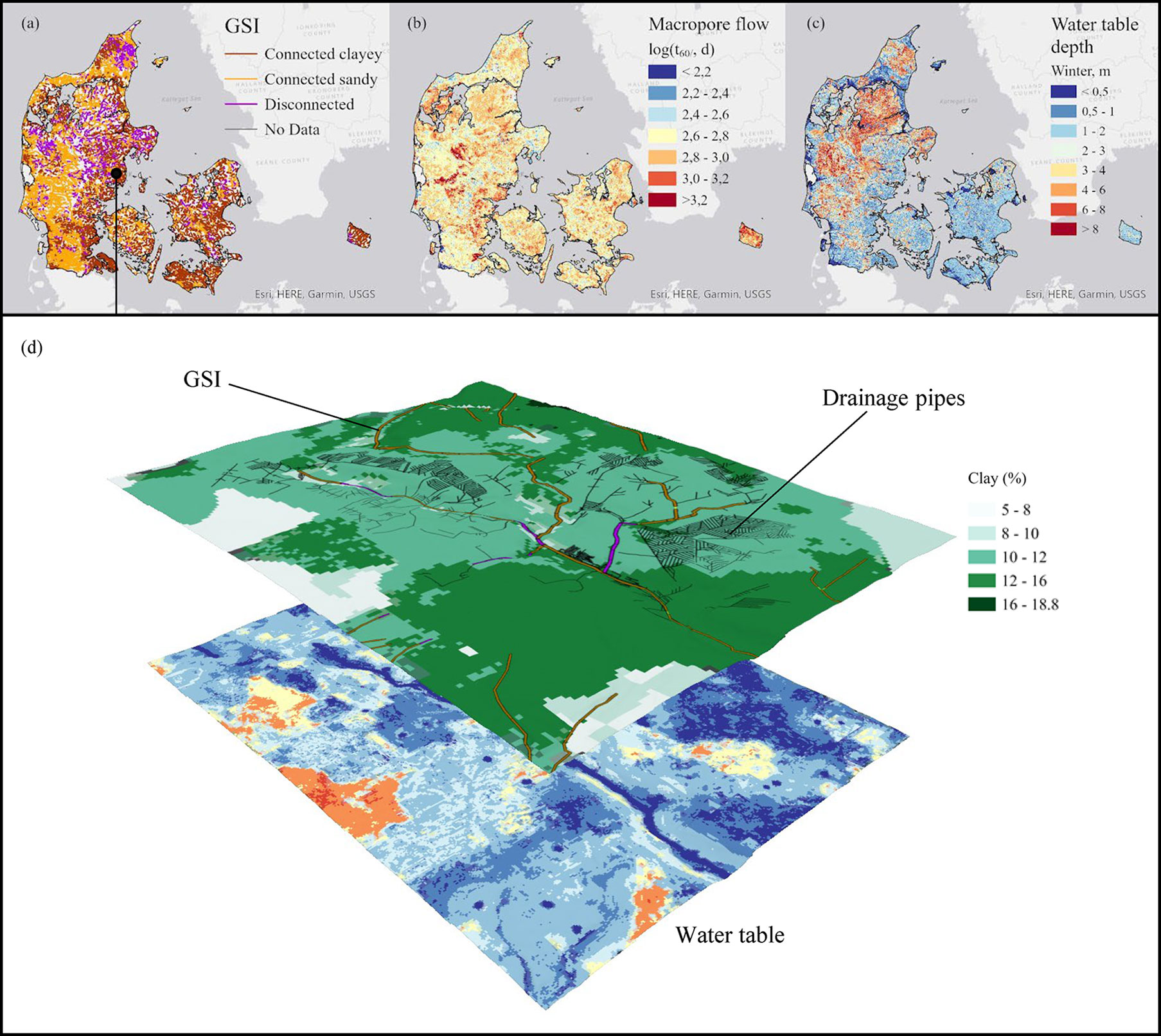
Figure 4 Water dynamics in Denmark represented by the maps of (A) groundwater surface interaction-GSI, (B) macropore flow, and (C) water table for the winter season. The selected area is the Fensholt region (D) and highlights the GSI and pre-existing drainage pipes over the clay map (%) and the water table for winter (m).
The drainage pipes increase the volume of water transported to surface water bodies, and predicting the drainage discharge could be an important source of information to analyse the risks of water contamination (72). Using machine learning, hydrological data from 53 drainage stations across Denmark and several explanatory variables, Motarjemi et al. (73) predicted the national-scale tile drainage discharge. They found that precipitation, topographic elevation, and clay contents below tile drains were the most important explanatory variables. However, this study did not take into consideration the variation of the predictor variables at the catchment scale.
An important function for the soil is its capacity to filter nutrients and pesticides from the water that moves through it. This filtering process depends very strongly on the flow paths of the water. For instance, preferential flow through macropores can cause P and pesticides to appear in the groundwater (74). Using pedotransfer functions (PTFs), soil texture maps and data from several locations in Denmark, Iversen et al. (67) mapped the risk of macropore flow in Denmark. The availability of soil texture maps in raster format (50) therefore directly enabled the spatial prediction of macropore flow. The higher resolution of the soil textural maps from 2013–2014 and robust PTFs enabled the development of maps of saturated and unsaturated hydraulic conductivities as well as water contents at specific pressure heads [Figure 4B (75)]. These results indicated that soils in eastern Denmark, which contain higher amounts of clay, have a higher probability for macropore flow.
Soil texture also affects groundwater-surface water interaction (GSI), which occurs when groundwater leaks into surface water or surface water percolates into the groundwater. GSI helps manage water resources and protect land and aquatic environments, essential for sound policymaking (76). Danish researchers reviewed GSI studies to create an eco-hydrological typology (77). Landscape, riparian hydrogeology, and flow path types were classified on arbitrary catchment scales of >5 km, 1–5 km, and 10–1000 m stream lengths. This set the stage for spatial classification mapping by first delineating Denmark’s river valley bottom (78). Sechu et al. (68) then developed a mapping routine to classify the Danish stream network using the typology. Three GSI contact types were mapped (Figure 4A): connected clayey, connected sandy, disconnected; and riparian flow paths: diffuse/overland, direct, and artificial drainage. The resulting maps revealed that 85% of Danish streams are connected to groundwater in sandy and clayey subsoils, and 87% receive riparian water through direct flow paths. For the stream sections, 41% and 19% receive riparian water through artificial drainage or diffuse/overland flow. These numbers match Denmark’s land use with 61% under agriculture (79), limiting runoff, and 50% artificially drained (61). The maps improve our understanding of flow of water and nutrients from uplands to streams and can help decision-makers manage agricultural land to protect receiving waters.
The combined water movement in the soil and drainage systems will eventually affect the water table, and its seasonal variations can influence the environment and society, especially in the context of future climate changes. In Denmark, knowledge of the water table is crucial for mapping flood risk, which is of special interest during wintertime when a shallow water table can induce flooding by constraining water storage. In the agricultural context, knowledge of high and low water tables is crucial for managing the cultivation of the land. The water table in Denmark has been monitored since 1970 and data has been recorded in the national well database, JUPITER (41). The first maps of the water table in Denmark were made using the National water resources model of Denmark (DK-model). The DK-model is a dynamic hydrological model that integrates groundwater and surface water processes. The model was originally set up at a spatial resolution of 500 m, which has been updated to 100-m resolution (80–82). Recently, Koch et al. (69) mapped the water table at 10-m resolution by applying a knowledge-guided machine learning framework that was built upon simulation results from the DK-model, the Danish well database JUPITER with 13,000 wells and 19,000 groundwater proxy observations for lakes, streams and the coastline. Koch et al. (69) mapped the water table for typical winter- (Figure 4C) and summertime conditions. Future work should include the development of hybrid models that enhance physically based models, like the DK-model, with machine learning to model water table dynamics at high spatial resolutions while also resolving temporal dynamics more accurately (83).
Mapping the spatial variables related to water movement through soil at high resolution will aid land management. This information could reveal the potential synergies and tradeoffs between provisioning (e.g., food production) and regulating ecosystem services (e.g., climate regulation) and orient future strategies for sustainable landscapes. For instance, the drainage systems increase food production and influence the Zero Hunger goal (SDG 2), but they can also speed up the movement of nutrients to water bodies and compromise Life Below Water (SDG 14). The future challenge is to find the balance between soil functions.
4.2 Spatial dynamics of nitrogen, phosphorus, and pesticides in soils
Storing, filtering and transforming nutrients are some of the main soil functions and are linked to the provision of Clear Water (SDG 6). Understanding the spatial dynamics of nutrient cycling in the soil can be an important tool to improve land management, especially in Denmark where agriculture and livestock production add a large volume of nutrients to soils through synthetic fertilizers and manure. In addition, the use of pesticides in agriculture and the risk of leaching pose a challenge to groundwater quality.
In the 1980s, environmental problems, such as the eutrophication of coastal waters prompted the Danish government to accelerate research into the losses of nitrogen and phosphorus from agricultural land and to regulate the application of chemical fertilizers and animal manures on soils. The government devised national Actions Plans for mitigating N losses to the aquatic environment and implemented the National Monitoring and Assessment Programme for the Aquatic and Terrestrial Environment (62). In general, Denmark started to monitor water quality in the 1980s, and the N concentrations were measured at monitoring stations (84). The Danish Environmental Agency mapped N leaching from the root zone (Figure 5A). However, de-nitrification can occur and there are available maps at the national level that show this potential (Figure 5B). Nevertheless, the N-reduction maps are still at catchment level, and higher resolution maps would be better suited to take local characteristics that affect N-reduction into account (41). Future studies exploring the transport and reduction of nitrate in Danish landscapes at various scales, could contribute to the detailed spatial target regulation of N use. The existing DSM approaches and the availability of data at the field level could be used to create N-reduction maps at high resolution.
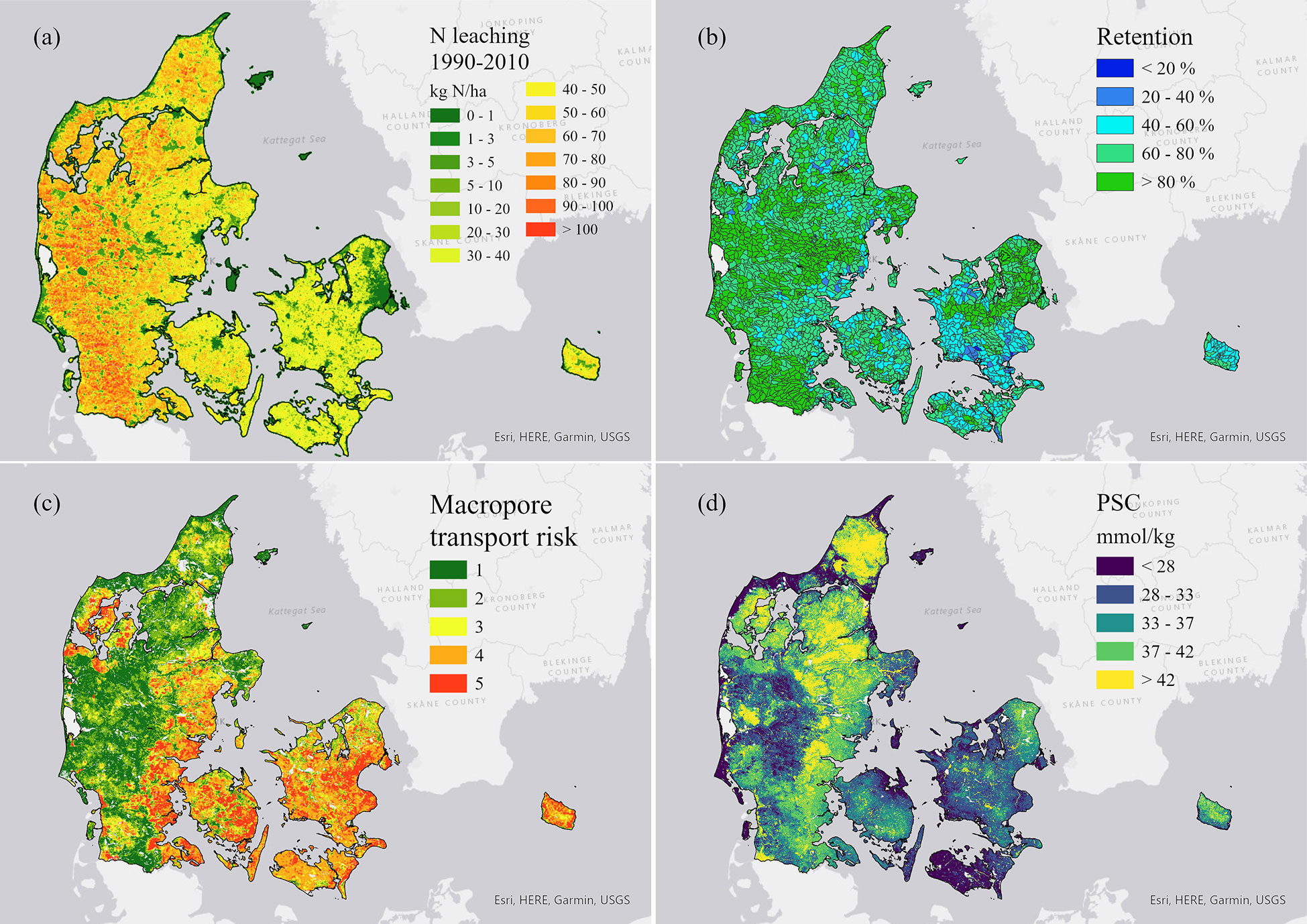
Figure 5 Maps of (A) nitrogen leaching, (B) nitrogen reduction, (C) risk of colloid transport in macropores, (D) phosphorus sorption capacity in Denmark.
Unlike N, which can be leached in the soil matrix/macropores through water movement, the dynamic of phosphorus (P) is more complex and is intrinsically related to the soil mineral properties and erosion processes. One of the first attempts to identify the risk of soil P in Denmark was the development of the Danish P index on a local (85) and national scale (86). However, the Danish P index was based on a qualitative framework and the absence of absolute P losses made the application of local regulations impractical. P can be lost from the soil system by water erosion as particulate P, bound P in the macropore flow transport, and as leaching of dissolved P. Onnen et al. (87) estimated the soil loss at 10-m resolution using the soil texture maps [Figures 3A–D (52)] and the erosion model WaTEM. Considering the average P concentration in the soil, P losses by erosion are estimated to be 56 t per ha per year (56). The potential of soil P loss through macropore transport was estimated using the map of soil P mobilization potential from 475 sites in Denmark (56) combined with the risk map of macropore transport [Figure 5C (75)]. The P sorption capacity of Danish soils was assessed [Figure 5D (57)] as well as the annual P leaching through the soil matrix (56), thus associating the knowledge of phosphorus binding kinetics in soil with annual drainage runoff (73) and drainage water transport in macropores and the soil matrix.
Pesticide transport is another potential environmental problem in Denmark, which various efforts have aimed to identify (88, 160). The pesticide maps mostly use data from monitoring wells, groundwater and gauging stations. A new Danish pesticide load risk indicator was developed in 2019 (159) and showed higher values for several pesticides in areas with loamy soils. This could be linked to the intense crop cultivation on the loamy Danish soils. In addition, Rosenbom et al. (90) identified a higher transport of pesticides in loamy soils compared with sandy soils, and this was associated with the transport through the macropore system, which is higher in loamy clay soils. Therefore, the association of soil macropore transport with pesticide application at a specific scale represents an important subject for future studies.
The studies on N, P and pesticide dynamics support the importance of the soil as a filter, which brings many benefits such as clean water for human needs and protection of the aquatic environments (Life Below Water - SDG 13). Historic mapping approaches proved to be efficient in orienting policy regulations, but future detailed mapping efforts can inform targeted actions by taking into consideration the local characteristics. The farmers could benefit from these targeted regulations, especially related to the use of N on croplands. In this case, assessing N dynamics at the field scale could give a better indication of the optimal N application for the local conditions. Future studies might go in this direction.
4.3 Mapping acid sulfate soils
Acid sulfate (AS) soils mostly occur in wetlands located in Jutland (c. 6,500 km2). The main characteristic of wetland soils is the presence of saturated hydromorphic conditions, and farming in such areas therefore typically requires artificial drainage, which can lead to the formation of AS soils. Aeration oxidizes iron sulfides (mainly pyrite), which produces sulfuric acid that causes metals to leach and the soil pH to drop below 3. The resulting toxic combination of acidity and metals (e.g., iron and aluminium) can cause severe ecological damages to the recipient watercourses (i.e., killing fish and other aquatic organisms) and degrade concrete and steel structures located underground to the point of failure. Since small hotspots of AS soils may impact large water bodies, accurate maps are crucial for effective mitigation. The Danish legislation prohibits drainage of areas registered as potential AS soils without prior permission from environmental authorities.
A first survey of Danish AS soils was carried out between 1981 and 1984 (19). The survey comprised soil samples from approximately 8,000 locations, with pH measurements at the time of sampling and after incubation, and an estimation of pyrite content and acid-neutralizing capacity (27). All the data was then collated within the Ochre Classification database, ochre referring to characteristic orange/brown-coloured iron oxides leaching into the drainage pipes and recipient watercourses. Madsen et al. (18) presented the resulting conventional map of potential AS soils. The map classified wetland areas depending on the frequency of potential AS soils (Figure 6A).
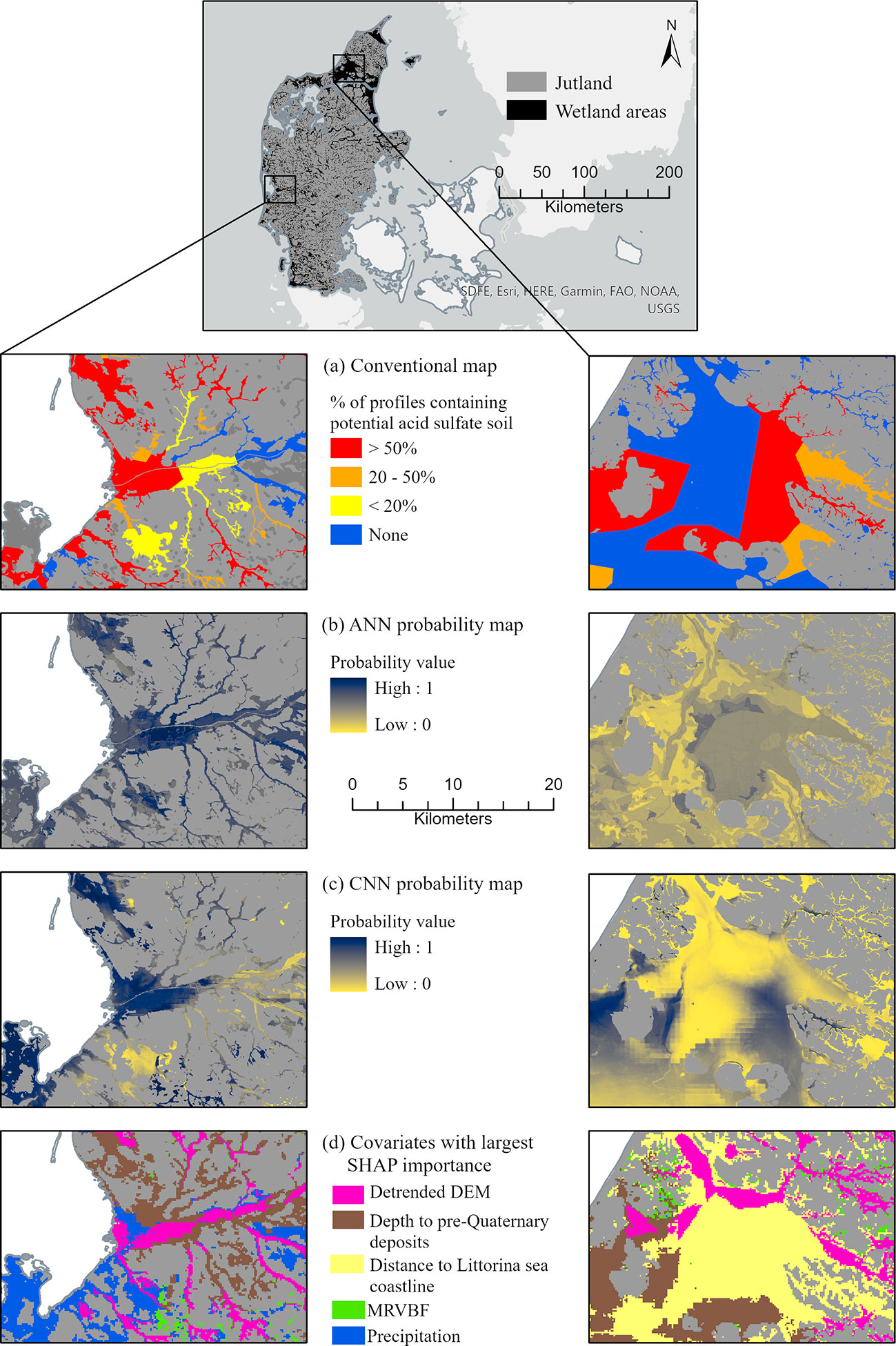
Figure 6 Mapping occurrence of potential acid sulfate soils for the Skjern River catchment area (left) and the Store Vildmose raised bog area (right): (A) conventional map, probability maps created with (B) artificial neural networks (ANN) and (C) convolutional neural networks (CNN), and (D) covariates with the largest SHAP importance within the CNN model.
More recently, Beucher et al. (91, 92) used the DSM approach for mapping the occurrence of potential AS soils. The study used the Ochre classification database and an array of environmental variables as input data. The environmental variables included a digital elevation model and derived terrain attributes, as well as legacy data on soil, landscape, land use and climate. While the first study employed Artificial Neural Networks (ANN) for prediction, the second evaluated Convolutional Neural Networks (CNN). While the ANN model already provided promising results in terms of accuracy, the CNN model was more accurate and yielded a more realistic pattern for the occurrence of potential AS soils. Figure 6 focuses on two areas of interest, the Store Vildmose raised bog area and the Skjern River catchment area located in northern and western Jutland, respectively. Particularly in the Store Vildmose area, the map generated by the ANN model showed many small sub-areas, often without natural transitions in the probability values (Figure 6B). In contrast, the predictive map created with the CNN model shows more logical transitions and patterns (Figure 6C). The CNN-based map also corresponds more closely to the conventional map (Figure 6A) than the ANN-based map, which can also be noted in the Skjern River catchment area. This consistency can be explained by the fact that CNN enables the inclusion of spatial contextual information extracted from environmental covariates around each soil observation used in the model as input data. Therefore, the resulting predictive map renders a more accurate representation of the occurrence of potential AS soils than the ANN-based model. Despite its lack of detail, the conventional map was developed using solid expert knowledge, which corroborates the CNN-based map. The predictions of the best-performing CNN model could also be elucidated using the model-agnostic interpretation method SHapley Additive exPlanations (SHAP) which outlined the most important covariates in terms of contribution to the model, not only quantitatively, but also geographically [Figure 6D (92)].
4.4 Agriculture and forest biomass production
One of the main challenges of the 21st century is to increase food production to meet the projected population growth (93). However, the expansion of agriculture over natural areas is not a sustainable solution, and the focus should therefore be to manage the land in a way that optimizes the usage and conservation of resources. Denmark has a long history of collecting information about land management and crop yields, and since 2011 the land use information has been available at the field level (94). The specific land use and management are typically the results of multiple factors, varying from environmental, economic, social and political factors. Understanding the factors that drive land use change, agricultural land suitability and crop yields is an important step to improve the use of natural resources (e.g., soil). Advances in remote sensing, mapping and modelling approaches have generated new possibilities for combining these factors and providing detailed spatial information for stakeholders.
Denmark has historical data about crops from the years 1644 and 1844 at parish level and high-resolution land use maps from 1990 to the present (38). The methodology used to create the recent land use maps is based mainly on the combination of a topographical database, field parcel maps from 2014 to 2020 and information about wetlands. In general, the land use change between 1990 and 2020 was characterized by a relative decrease of 6.5% (195,000 ha) in cropland areas, and increases in grasslands (26,000 ha; 18.6%), forests (96,800 ha; 17.8%), wetlands (partly water-covered; 19,000 ha; 37.9%) and surface water bodies (6,000 ha; 11.6%) (38). The study presented the maps in a raster format at a 25-m resolution. Furthermore, every year Danish farmers register the field crops used in order to receive support from the Common Agricultural Policy of the EU, which has provided near-complete field level information on cropping patterns in Denmark since 2011 (https://eng.lbst.dk/). However, even more detailed information within the land use polygons could be made available. Remote sensing images and advances in machine learning could potentially help to produce maps that present not only the vegetation class but also the status of the vegetation. For instance, supervised land use classification could potentially map different levels of degradation in grasslands. This additional information could be useful for farmers to monitor the crops, but also for policy-makers for targeted regulations and subsidies.
The observed land use generally depends on soil characteristics, climate, relief, the water table, as well as economic, social and political factors. Placing the most suitable crop in the right environment would not only favour productivity; in many cases it would also be the most sustainable option. Laville (95) coined the term “Natural Terroir Unit” (NTU) to define locations that share the same topography, soil and climate, providing a unique high-quality characteristic for an agricultural product. Several studies have applied DSM methods to identify NTUs using environmental characteristics in Denmark (96–98) and around the world (99, 100). Importantly, Peng (98) noted a missing link between the existing terron maps and agricultural data. The authors addressed this issue by comparing their terron maps (Figure 7B) with historical wheat yield (Figure 7C). This allowed them to assess the validity of the terrons and their relative productivity for a specific crop.
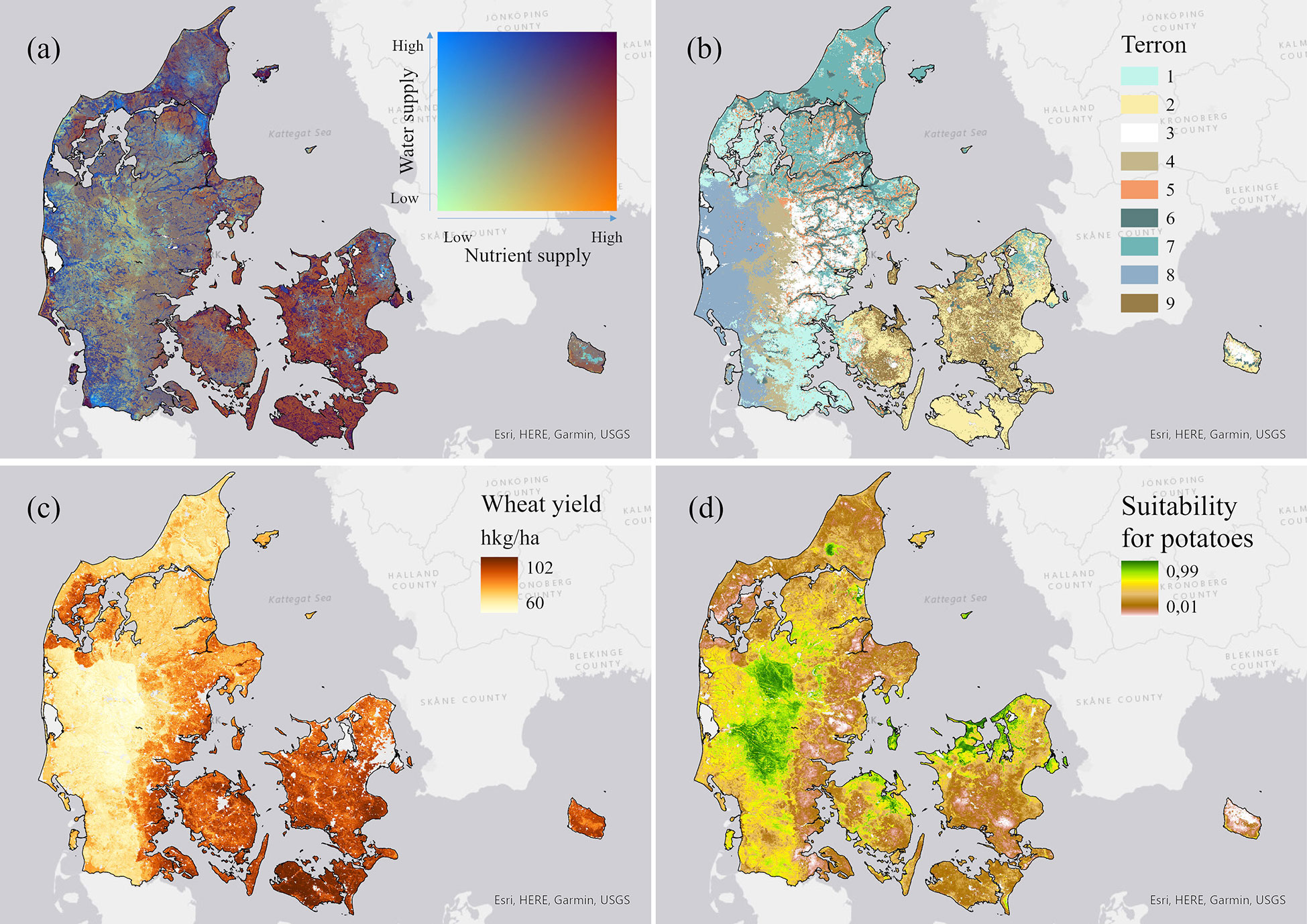
Figure 7 Examples of maps related to agricultural production in Denmark: (A) spatial distribution of water and nutrient supply in soils, (B) terron classes, (C) wheat yield, and (D) land suitability for potatoes.
It is also possible to predict the agricultural land suitability with crop growth models, such as EcoCrop (101), and species distribution models (94, 102) mapped the land suitability for specialty crops in Denmark (Figure 7D) using EcoCrop and machine learning models based on MaxEnt. They found that the advantage of machine learning models was their ability to include explanatory variables related to economic and social factors, which are intrinsic to the land use. Selecting the appropriate crop for a certain location would minimize risks from adverse weather, pests, diseases, and changes in market conditions and policies. However, the authors also stressed that maps need to have a clear and unambiguous interpretation in order to be useful for end users.
The need for selecting healthy sites is crucial when planting trees, especially when wood production is the goal. Denmark intends to double the 1989 baseline forest cover within 80-100 years, and due to the longevity of forest stands, not only does the present site quality need to be considered to ensure healthy trees as climate shifts, but so does also the future site quality. One way to evaluate forest site quality for various species is to use ecograms. Ecograms are used to determine site quality for various tree species by determining the nutrient and water availability at a site, and comparing them to the requirements for the specific species. Figure 7A shows the spatial distribution of nutrient and water supply in Danish soils (103). To build this map, the landscape was classified into six nutrient classes and nine water classes based on four variables: pH at 1 to 2 m depth, average precipitation between April and October, groundwater depth, and plant-available water. Since the 1990s, Denmark has used ecograms for small-scale implementation when assessing sites for various tree species (29), and for the implementation of close-to-nature forest management. Recently, national ecogram maps were generated for five species to help foresters quickly determine which regions are suitable or unsuitable for the different species for future afforestation efforts (104).
Machine learning is also a potential means to map crop yields. The availability of historical and current wheat yields in Denmark made it possible to model the potential and actual yield through time (105). The authors found that advances in agricultural management, including fertilization, pest management, irrigation and drainage allowed farmers to exceed the historical yield predictions. Larsen et al. (106) also modelled the potential yield of the energy crops willow (Salix) and silver grass (Miscanthus) across Denmark. Their results showed that willow yields were likely to be higher than silver grass yields on sandy soils, whereas silver grass yields were highest on clay-rich soils. This information could be important for selecting feedstocks for bioenergy.
Agricultural production is one of the most generally recognized soil functions, and studies related to land suitability and crop yields highlight the undeniable importance of the soil. The growing conditions for most crops depend very strongly on the soil. Therefore, outlining the optimal environments for specific crops has the potential to improve the use of local resources and reduce the severity of the risks that farmers face. It also helps to provide a clear link between food production and soil functions. These efforts therefore help to improve and maintain agricultural production in Denmark. This is highly relevant in Denmark, which produces three times more food than the local population consumes (79), which contributes to the progress toward Zero Hunger (SDG 2).
4.5 Peatlands
Peatlands are unique, organic, wetland ecosystems and very important for climate change mitigation and the environment. Global peatland coverage is estimated to be about 3% of the total land area only, but they contain about 5-20% of the global soil C stock (107, 108). Peatlands provide several ecosystem services that contribute to several SDGs, such as Climate Action - SDG13 (C sequestration), Life on Land - SGD15 (biodiversity conservation), Clean Water - SDG 6 (hydrological regulation). However, human-mediated degradation due to poor management impairs the delivery of many peatland ecosystem services (109–111). Under refined management systems, however, peatlands may offer opportunities to achieve key global sustainability objectives (112).
In Denmark, peatlands cover approx. 2,400 km2 (Figure 8). Various peatland inventories have been established through time. The first real peatland mapping attempt on a national scale commenced in 1919 (focusing specifically on bogs). This was to ascertain the quality and potential use of bog resources for fuel due to failed supply of foreign fuel during World War I and II (113). From the early 1920s, the Danish Land Development Service classified meadows and bogs larger than five hectares into four groups depending on the quality of the peat for fuel (113, 114). Between 1975 and 2011, several national soil databases including peatland soils were established: the DSC, the Ochre Classification, and the SINKS databases. The original SINKS database resulted from a national field survey carried out in 2010 and 2011. About 9,800 soil samples were collected following a systematic sampling scheme (using three regular grids of 250-, 275- and 500-m spacing: Figure 8A). At each sampling location, four subsamples were taken at 30-cm depth increments from the soil surface down to 120 cm depth. Furthermore, other detailed inventories have been established at field scale for peatlands of interest.
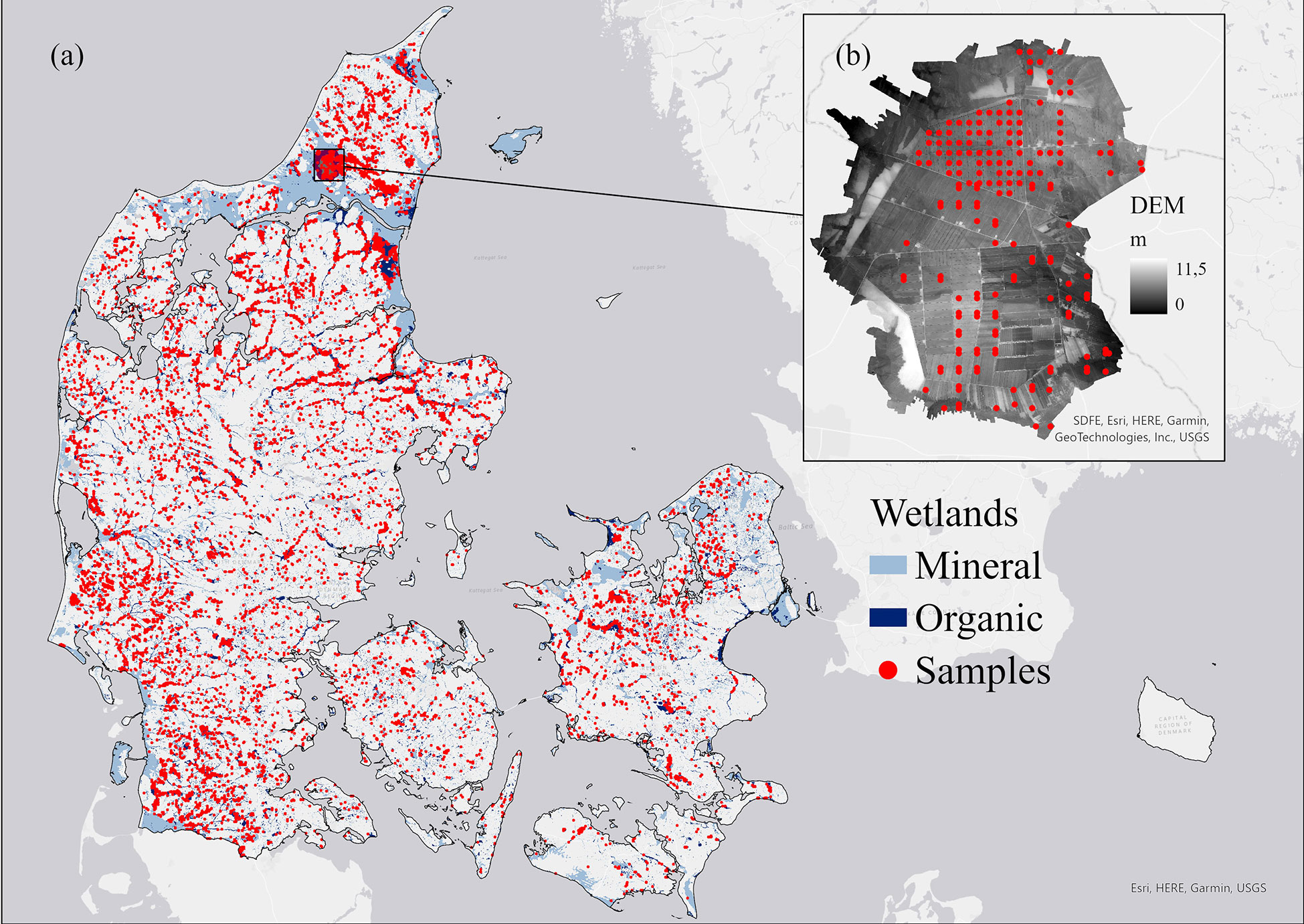
Figure 8 Maps showing (A) the extent of wetland areas and location of samples from the original SINKS database, and (B) the Store Vildmose raised bog area (with the digital elevation model as a background layer).
To realize national commitments to international climate agreements (i.e., Kyoto Protocol, Paris Agreement), accurate mapping of peatlands is required. Thus, the detailed mapping of peat properties is needed; conversely, this is also difficult to achieve using solely conventional surveys due to the challenges of time, labour input, and inaccuracies in measurements (115, 116). To this end, DSM techniques have been employed in Danish peatland studies. Generally, DSM techniques enable the mapping of peatland properties and processes such as the extent, diminishing C stocks, response to climate change and the impacts of rapid land use changes more accurately (107). At the national scale, both the distribution of SOC and changes in peatland extent were mapped by Greve (31) and Kheir et al. (117) using geostatistical and machine learning techniques, respectively. Moreover, at field and farm scales, proximal geophysical sensors, which are known to provide opportunities to rapidly acquire more accurate ancillary data due to the unique electrical properties of peat (e.g., water content, electrical conductivity, relative dielectric permittivity), have been used (115, 118). Specific applications of these techniques include studies by Knadel (119) and Beucher (120), who employed not only spectroscopic techniques for SOC mapping but also electromagnetic induction techniques for peat thickness mapping, both at field scale. Recently, a policy support report by Møller et al. (121) described potential methods for an improved mapping of SOC in peatland areas. The study also outlined the possible improvements in accuracy that would be gained from the use of geophysical soil sensors.
The various soil mapping activities have provided valuable insights on the functioning and status of Danish peatlands. For example, Beucher et al. (120) could accurately predict peat thickness at field scale (from apparent electrical conductivity and other relevant predictors), which is vital for estimating C stocks and meeting binding climate policies such as the EU 2030 Climate and Energy Framework. Additionally, the effect of drainage and historical peat extraction for fuel by humans was quantified by Greve et al. (31) as a 35% reduction in the national extent of peatland areas between 1975 to 2010. To halt the further decline in the peat coverage, this finding was significant for stakeholder action. Similarly, the human-induced subsidence of the Store Vildmose raised bog (Figure 8B) over a 130-year period revealed near complete loss of peat in extracted areas and subsidence of at least 2 m along the greater part of an established transect. In contrast, areas with peat growth (2-6 mm per year) were also observed and this was due to laws that protected such areas and thereby prevented the drainage and degradation of the peat (122). Currently, several peat mapping activities are underway to restore the Store Vildmose peatland to its natural state. This includes plans to stop agricultural activities and to rewet the area. The purpose of the lowlying project is to reduce CO2 emissions to promote the natural qualities of the area, to improve coherence and robustness of natural areas and to restore more natural hydrology (123).
5 Mapping soil threats
Anthropogenic actions (i.e., agriculture intensification) can threaten the natural soil functioning processes and their potential to provide ecosystem services. Soil erosion, soil compaction, SOC decline, and soil sealing have been identified as the main soil threats in Denmark (86, 124), but spatial and temporal information about these issues is still scarce. The spatial assessment of these soil threats can be a tool to inform policymakers and farmers on land planning and management. In this review, we will focus on the discussion of soil erosion, SOC loss and soil compaction due to intensive agriculture in Denmark.
SOC performs a crucial role in ecosystem functioning and the global C cycle, and its decline can affect important soil processes, such as regulating water dynamics, stabilizing the soil structure, and releasing and holding nutrients for plants (125). Although the spatial assessment of SOC has been predicted by several methods (49, 50, 53, 117) and supports the Danish Kyoto protocol, the spatial distribution of the SOC gain and losses across time is still lacking at the national scale. Taghizadeh‐Toosi et al. (126) identified a decline of SOC in Denmark based on sampling data from SOC in 1986, 1997, 2009 at depth intervals of 25 cm down to 1 m from the grid soil monitoring inventory. This study associated the SOC decline with heavy ploughing and widespread grain cultivation on a large portion of Danish agricultural land, but the spatial links with soil characteristics and climate could give important insights on SOC loss susceptibility. Another source of SOC loss is the land use change from peatlands to agricultural areas or extraction for commercial uses. Peatlands contain more than 12% of SOC and Greve et al. (31) showed that Denmark lost about 37,000 ha of peatland areas from 1975 to 2010. Although SOC losses due to peatland degradation has not been estimated yet, this had a large impact on the SOC sequestration on Danish soils.
Monitoring SOC changes at national scale is a challenge since soil inventories should be carried out at greater frequency and it has several limitations. SOC measuring and monitoring methods have been based on intensive sampling and are thus highly time- and resource-consuming, and there is a need for a more robust, accurate, and inexpensive method to estimate SOC content for the SOC inventory and to show temporal changes. The first spatial assessment of SOC changes used Vis-NIR spectroscopy, monitoring and mapping spatial and temporal changes in topsoil SOC between 1986 and 2009 in a region of Denmark (127). At a finer scale, the temporal changes in the spatial distribution of erosional and depositional processes have been examined and related to the temporal SOC changes during a 16-year period (1998-2014) to elucidate controlling factors on SOC patterns in Danish cultivated soils (128). These attempts to understand the SOC content at various scales have revealed a knowledge gap related to the linking of SOC changes over time with designing environmental and agronomical strategies to foster C storage in soils (129, 130).
Globally, the soil potential to sequester C and provide C-related function over space and time has been examined through soil monitoring networks using feasible indices based on easily quantifiable soil parameters (130, 131). SOC sequestration could be better elucidated based on ‘complexed’ and ‘non-complexed’ OC since the bonding of OC to fine mineral particles is deemed one of the most significant SOC stabilization mechanisms (129, 132). Dexter et al. (133) suggested that complexed OC would be more stable than non-complexed OC, thus C sequestration in the soil would be closely related to complexed C by clay particles, a theory also supported by de Jonge et al. (134) and Schjønning et al. (135) for Danish arable soils. Greve et al. (124) measured the spatial distribution of the topsoil clay/OC ratio (Dexter index represented in Figure 9A) and found that 10% of the agricultural soils have an index higher than 10, which indicates a critically low OC content. More studies are needed, nevertheless, to explore the long-term dynamic of this ratio in a spatio-temporal context in Denmark.
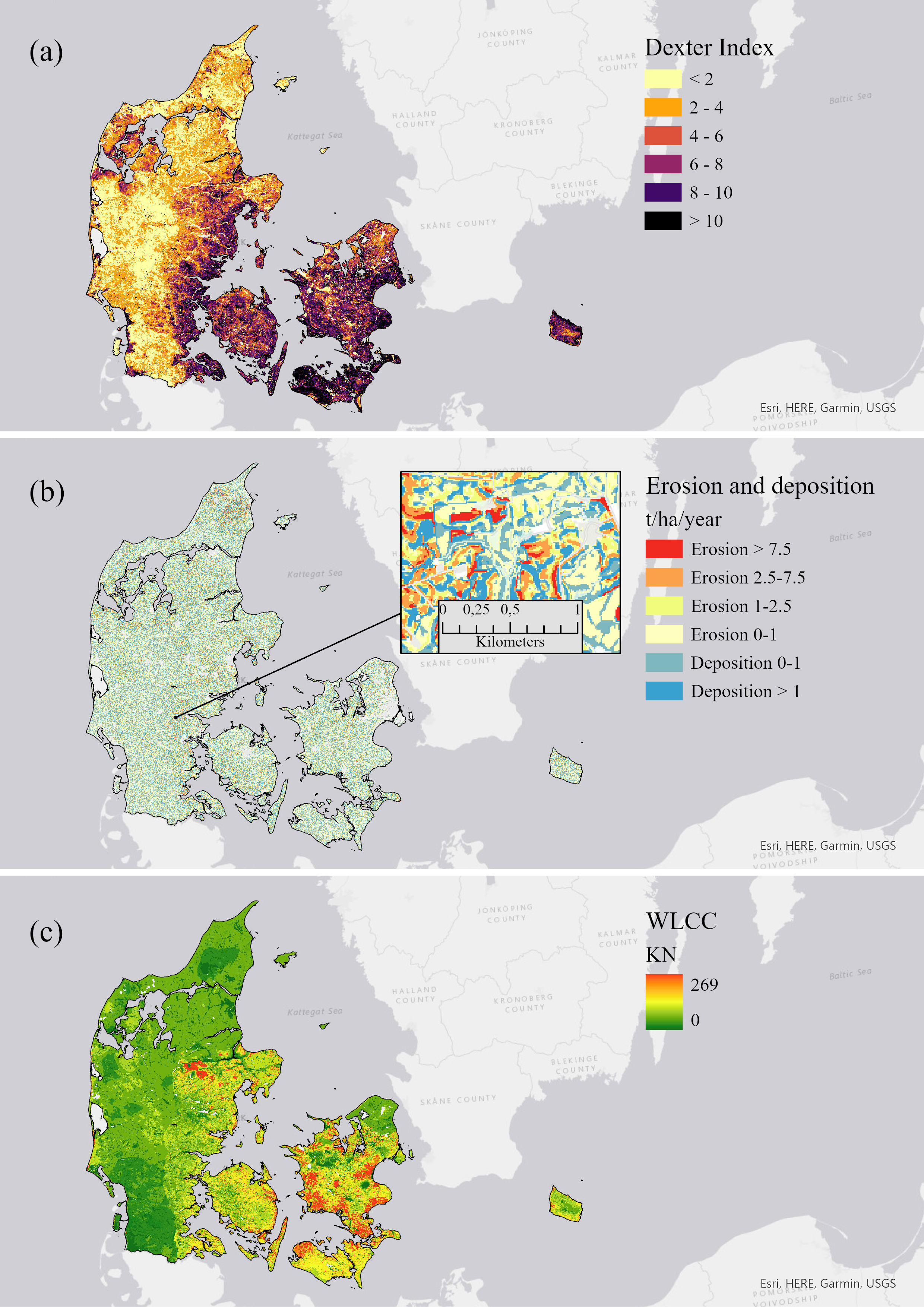
Figure 9 Main soil threats in Denmark represented by (A) Dexter index, (B) water erosion risk, and (C) risk of soil compaction as the maximum load that can be carried by a given tyre without compaction at 0.35 m depth (i.e., wheel load carrying capacity or WLCC, in kN).
Wind, water, and tillage erosion are important processes of soil degradation on cropland in Denmark. Historically, wind erosion has been a severe problem in the western part of the country for centuries (18). However, widespread planting of shelterbelts since the late 19th century and an increasing shift from spring crops to winter crops in recent decades with a consequent better soil cover in the critical spring period have limited the threat. Arable land in Denmark is vulnerable to water erosion (136) with soil redistribution rates comparable to those of other countries in Northern Europe (87). Tillage erosion describes net soil redistribution caused solely by tillage operations (137). Tillage erosion removes soil at convex landforms and deposits it again at concave landforms within fields. In undulating landscapes, intensive tillage, such as mouldboard ploughing, thus causes a massive redistribution of soil rich in organic matter and nutrients, severely impairing soil quality maintenance and soil productivity in the long term (138–140). Preliminary modelling of tillage-induced soil loss on all cropland in Denmark indicates a substantially greater impact on soil quality compared to water erosion (unpublished data).
The redistribution of soil resulting from water erosion has been predicted at 10-m resolution for all of Denmark [Figure 9B (87)]. This study applied the Water and Tillage Erosion Model (WaTEM) (141), which links a topography-driven, spatially distributed sediment transport model and the empirical Revised Universal Soil Loss Equation (RUSLE) to predict patterns of soil loss and gain in landscapes as well as sediment delivery to surface waters. The national maps of soil erosion risk and sediment delivery support targeted mitigation planning on agricultural land and the work of national regulators in implementing the EU’s Common Agricultural Policy (142).
Soil compaction is characterized by an increase in soil bulk density, mostly due to a decrease in air-filled porosity (143). It compromises the soil functions and the provision of a range of ecosystem services (144, 145). Although SOC losses and soil erosion can occur due to climatic factors and be amplified by human activities, soil compaction is mainly caused by anthropogenic interventions. Many Danish soils are already compacted due to historical land uses. Madsen and Jensen (19) concluded that about 39% of soil samples from the Danish Soil Profile database had a critically high relative normalized density.
While mitigation of most of the effects of compaction in the topsoil can be achieved using mechanical operations, mechanically loosened subsoils are extremely susceptible to severe recompaction (146). Consequences of compaction in the subsoil (beneath the tilled layer) might last for decades (147), even for resilient soils subjected to shrink/swell and freeze/thaw (148). Because of the persistence of subsoil compaction and its negative impacts on soil functions, this urgently needs to be prevented. Soil compaction will only occur if the mechanical stress applied to the soil exceeds its mechanical strength. Therefore, risk assessment of soil compaction should be based on a comparison of the stress applied to the soil and the soil strength (149). Figure 9C presents the maximum wheel load (i.e., wheel load carrying capacity or WLCC, in kN) for a specific tyre and inflation pressure that does not exceed the soil strength at 0.35 m depth and at a matric potential of -50 hPa. The method used to derive this map is described in detail in Lamandé et al. (150).
6 Discussion
In this review, we explored the underlying evolutionary factors of soil assessment in Denmark since the 17th century and how they contributed to the development of soil function maps in the last decade. We found that the constant improvement in both soil inventories and environmental monitoring programmes across the country were essential to the building of solid knowledge about the soil and the environment. The increasing number of soil surveys at national level supported the development of soil assessment methodologies. The methods evolved from purely subjective approaches to the present use of digital technologies and multiple sensors to map soil characteristics and patterns. The DSM approaches enabled the development of soil property maps at high resolution, which laid the basis for mapping soil functions.
Detailed spatial environmental data is essential for government decisions, but information about vegetation dynamics and especially soil information is still neglected in many countries around the world. In Denmark, the generation of soil and environmental data is characterized by a government-coordinated approach. Despite the different aims for soil assessments over time, ranging from taxation assessments in the 17th century to environmental monitoring in the present day, the soil inventories have always been the outcome of government initiatives conducted by central commissions. This resulted in nationally coordinated activities with standardized methods for soil sampling, laboratory measurements and classification. They were also generally finished within relatively short time spans. For instance, the Danish Soil Classification project collected and measured more than 36,000 soil samples in five years (1975–1980), and the original SINKS project sampled almost 10,000 wetland locations over the course of two summers (2009–2010). Soil surveys of this size require large financial resources, also explaining the global geographical bias for soil sampling, with low soil sampling densities in developing countries (151). Despite the improvement in transport, logistics and technologies for soil analysis, new national soil survey programmes are rare, even in developed countries. Governments may regard soil surveys as prohibitively expensive and prioritize other needs instead. However, the history of soil mapping in Denmark shows that the knowledge generated from soil data can bring about significant long-term benefits for society. The fact that data collected more than 40 years ago in Denmark still provides relevant knowledge for groundwater protection serves as a clear example in this context. The investment in soil inventories has therefore enabled the government to avoid severe costs from cleaning water and managing the potential environmental and human health problems. However, this is not straightforward, and the governments must be open to applying the data-driven knowledge generated in science. In Denmark, there is a strong collaboration and communication between the scientific community and the government, and a frequent production of scientific reports helps to update the present knowledge on environmental issues and support the development of new policies. Nevertheless, there is only limited government funding for the collection of new soil data and monitoring programmes. The present review shows the importance of continued soil and environmental assessments and their benefits for management and planning, enabling a better understanding of soil functions and preserving the provision of ecosystem services for future generations.
The soil is an open system, and updated soil information, especially from underrepresented areas, constitutes the first step for insights on the spatial distribution of soil functions. Soil functions are mainly related to the links between chemical and biological soil characteristics. In this sense, soil structure is a key factor in regulating soil functions (152). Moreover, it is a very sensitive indicator of soil quality, as it reflects influences from physical, chemical and biological processes as well as management practices. However, soil structure is difficult to quantify, and physical soil descriptions often only include soil texture. In 2007, Denmark was one of the first countries in the world to produce a national soil texture map based on soil samples using digital technologies (50). This map helped to provide the first geographic insights on water transport in soil macropores and N dynamics. The next generation of national soil texture maps from 2014 also supported efforts to map soil functions at a higher resolution (30-m). Some studies have used proximal sensors to map soil properties at field level in Denmark (59, 120, 153). Detailed field maps would be useful to farmers who wish to adopt precision agriculture techniques. Although many countries do not have their own soil properties maps, global studies such as SoilGrids provide global soil maps at 250-m resolution and could be used to map soil functions (151). Such understanding of soil functions requires interdisciplinary research and new knowledge must be associated with DSM approaches to improve their spatio-temporal analysis. For this, global environmental monitoring efforts should focus on generating temporal information about soil function such as water dynamics, vegetation dynamics and water pollution. The absence of or decreasing the frequency of environmental monitoring around the world could become a greater limitation to the expansion of knowledge on soil functions than methodological challenges, and it may limit our capacity to mitigate global issues such as climate change, water scarcity and contamination, and food security.
The UN SDGs are useful for setting the main objectives to be achieved to mitigate the main global issues that threaten nature and human well-being. However, to achieve many of these objectives, we must protect the world’s ecosystems. Soil functions provide some of the most basic ecosystem services needed to achieve many SGDs (4). The mapping of soil functions in Denmark shows a clear link between soil functions, provision of ecosystem services and the related SDGs. The world is presently facing severe issues that are likely to worsen in the future, and detailed knowledge of the environment is necessary to mitigate or adapt to these future challenges.
Although the SDG indicators represent the final results from several ecosystem services, they do not in themselves provide an understanding of the basic environmental processes and how they influence the progress towards the SDGs. The actual SDG indicators related to environmental preservation are too general, and attention should be given to improving our understanding of basic soil processes and how soil functions provide ecosystem services. This would allow us to change agricultural management systems and then achieve future goals. For instance, the indicator of water regulation in the SGD framework is the water retention capacity, but water regulation is more complex than this soil characteristic, and in order to achieve Clean Water (SGD2), several socio-environmental factors should be involved. Instead of focusing on the application of industrial systems to clean water, we aim to prevent water pollution. Another example concerns the Zero Hunger goal (SDG2). Soils provide the medium for most of the world’s crops, and the pressure of intensive cultivation causes a decline in SOC and general soil degradation, threatening the future capacity for food production. It is therefore impossible to achieve and maintain zero hunger if we do not achieve a sustainable use of the soil. The same idea can be applied to SDG 14 (Life Below Water) with the filtering function of the soil, to SDG 13 (Climate Action) with the vital role of soils in the C cycle and to SDG 15 (Life On Land) with the soil acting as a habitat for a vast multitude of microorganisms, animals and plants. The UN should call for future global soil monitoring programmes with the specific aim of understanding soil functions, which would provide us with the necessary information to manage ecosystems and ensure their capacity to help us achieve the SDGs.
The experience in mapping soil properties and functions in Denmark highlights the fact that understanding the spatial dimensions of soil functionality and their benefits for human well-being is a long-term effort that evolves over time. It also shows the persistence of many knowledge gaps related to soil information and methodologies, soil processes at pore scale, and threats. Soil organisms are the active part of the soil system and responsible for improving soil structure, which is essential for many ecosystem services. However, we still lack spatial information on soil biodiversity. This information could help us design our management practices to protect the gene pool in soils, which can serve multiple purposes, such as the discovery of new medicines, control of pathogens, and remediation of polluted soils. Although farmers in Denmark are required to report on the use of pesticides and fertilizers, spatial information at field level is still lacking. Such information could help us monitor soil functions and consequences for the environment, helping society to achieve a sustainable compromise between agricultural production and environmental protection. With the increase in information on soil systems, environmental variables and methodological approaches, there is an opportunity to improve the existing soil property and function maps and their uncertainty assessment, but also to link the machine learning results with existing pedological knowledge (154). For instance, the SHAP method enables us to spatially interpret the importance of covariates (92, 155–158), which can further improve our understanding of soil functionality. Until now, most of the maps of soil functions cover only individual subjects, such as water dynamics, C sequestration and movement of pollutants to water. However, all these soil functions occur simultaneously and are interconnected, and the use of multivariate mapping is a promising way to connect this multitude of factors. For instance, agricultural management can influence SOC contents that affect soil structure and porosity, which in turn affect soil water retention and the movement of pollutants. Although there are mechanistic models that connect many of these factors, the future challenge is to identify the synergies and trade-offs of soil functions according to different land uses and management practices. This will allow us to optimize the use of soils and their ecosystem services with minimum trade-offs. This can highlight the importance of soils for human well-being and increase public awareness of the urgent need to protect our soils.
Author contributions
LCG and MHG contributed to the conception and design of the study. All authors contributed to writing and reviewing the manuscript. All authors contributed to the article and approved the submitted version.
Funding
The study was supported by the SustainScapes project - Center for Sustainable Landscapes under Global Change (grant number: NNF20OC0059595), and the ReDoCO2 project with funding from the Innovation Fund Denmark (grant number: 0177-00086A).
Conflict of interest
The authors declare that the research was conducted in the absence of any commercial or financial relationships that could be construed as a potential conflict of interest.
Publisher’s note
All claims expressed in this article are solely those of the authors and do not necessarily represent those of their affiliated organizations, or those of the publisher, the editors and the reviewers. Any product that may be evaluated in this article, or claim that may be made by its manufacturer, is not guaranteed or endorsed by the publisher.
References
1. Adhikari K, Hartemink AE. Linking soils to ecosystem services — a global review. Geoderma (2016) 262:101–11. doi: 10.1016/J.GEODERMA.2015.08.009
2. Bünemann EK, Bongiorno G, Bai Z, Creamer RE, De Deyn G, de Goede R, et al. Soil quality–a critical review. Soil Biol Biochem (2018) 120:105–25. doi: 10.1016/j.soilbio.2018.01.030
3. Daily GC, Matson PA, Vitousek PM. Ecosystem services supplied by soil. Nature’s services: societal Depend Natural Ecosyst (1997) Island Press, 113–32.
4. Keesstra SD, Bouma J, Wallinga J, Tittonell P, Smith P, Cerdà A, et al. The significance of soils and soil science towards realization of the united nations sustainable development goals. Soil (2016) 2(2):111–28. doi: 10.5194/soil-2-111-2016
5. Evans DL, Janes-Bassett V, Borrelli P, Chenu C, Ferreira CSS, Griffiths RI, et al. Sustainable futures over the next decade are rooted in soil science. Eur J Soil Sci (2022) 73(1):e13145. doi: 10.1111/ejss.13145
6. Lal R. Soil carbon sequestration impacts on global climate change and food security. Science (2004) 304(5677):1623–7. doi: 10.1126/science.1097396
7. Bouma J, Montanarella L, Evanylo G. The challenge for the soil science community to contribute to the implementation of the un sustainable development goals. Soil Use Manage (2019) 35(4):538–46. doi: 10.1111/sum.12518
8. Rossiter DG, Liu J, Carlisle S, Zhu AX. Can citizen science assist digital soil mapping? Geoderma (2015) 259-260:71–80. doi: 10.1016/j.geoderma.2015.05.006
9. McBratney AB, Mendonça Santos ML, Minasny B. On digital soil mapping. Geoderma (2003) 117(1):3–52. doi: 10.1016/S0016-7061(03)00223-4
10. Chen S, Arrouays D, Leatitia Mulder V, Poggio L, Minasny B, Roudier P, et al. Digital mapping of globalsoilmap soil properties at a broad scale: A review. Geoderma (2022) 409:115567. doi: 10.1016/j.geoderma.2021.115567
11. Vogel HJ, Bartke S, Daedlow K, Helming K, Kögel-Knabner I, Lang B, et al. A systemic approach for modeling soil functions. SOIL (2018) 4(1):83–92. doi: 10.5194/soil-4-83-2018
12. Carré F, McBratney AB, Mayr T, Montanarella L. Digital soil assessments: Beyond dsm. Geoderma (2007) 142(1-2):69–79. doi: 10.1016/J.GEODERMA.2007.08.015
13. Alexandre MJCW, Gerard BMH, Lark RM, Philippe L, Johan B, Vera LM, et al. Ten challenges for the future of pedometrics. Geoderma (2021) 401:115155. doi: 10.1016/j.geoderma.2021.115155
14. Greiner L, Nussbaum M, Papritz A, Zimmermann S, Gubler A, Grêt-Regamey A, et al. Uncertainty indication in soil function maps – transparent and easy-to-Use information to support sustainable use of soil resources. SOIL (2018) 4(2):123–39. doi: 10.5194/soil-4-123-2018
15. Styc Q, Lagacherie P. What is the best inference trajectory for mapping soil functions: An example of mapping soil available water capacity over languedoc roussillon (France). Soil Syst (2019) 3(2):34. doi: 10.3390/soilsystems3020034
16. Aitkenhead MJ, Coull MC. Digital mapping of soil ecosystem services in Scotland using neural networks and relationship modelling. part 2: Mapping of soil ecosystem services. Soil Use Manage (2019) 35(2):217–31. doi: 10.1111/sum.12491
17. Arrouays D, Grundy MG, Hartemink AE, Hempel JW, Heuvelink GBM, Hong SY, et al. Chapter three - globalsoilmap: Toward a fine-resolution global grid of soil properties. In: Sparks DL, editor. Advances in agronomy, vol. 125. Academic Press (2014). p. 93–134. doi: 10.1016/B978-0-12-800137-0.00003-0
18. Madsen HB, Nørr AH, Holst KA. The Danish soil classification: Atlas over Denmark I. (1992) 3:56.
19. Madsen HB, Jensen NH. The establishment of pedological soil data bases in Denmark. Geografisk Tidsskrift-Danish J Geogr (1985) 85(1):1–8. doi: 10.1080/00167223.1985.10649211
20. Rothe C. Beretning om den I aaret 1844, for kongeriget indførte Nye skyldsætnings væsen og historie. Kjøbenhavn (1844).
21. Jordboniteringskommissionen. 1. Dei: Om Udarbejdelsen Af Vejledende Regler for Udførelsen Af Jordboniteringer. 2. Del: Om Kommissionens Arbejde Med Ansættelse Af Prøvetakster I De Enkelte Kommuner. (1970)
23. Nørr AHSWP. Edb-baseret kortproduktion ved landbrugsministeriets arealdatakontor. Landinspektøren (1984).
24. Platou SW. (1984). The adk data base systems (Pds and lads) used in Denmark: In soil information system technology, in: Proceedings of the sixth meeting of the ISSS Working Group on Soil Information System, Bolkesjø, Norway. Pudoc Wageningen.
25. Breuning Madsen H, Platou S. Land use planning in Denmark: The use of soil physical data in irrigation planning. Hydrology Res (1983) 14(5):267–76. doi: 10.2166/nh.1983.0021
26. Madsen HB. Computerized soil data used in agricultural water planning, Denmark. Soil Use Manage (1986) 2(4):134–9. doi: 10.1111/j.1475-2743.1986.tb00697.x
27. Madsen HB, Jensen NH. Potentially acid sulfate soils in relation to landforms and geology. Catena (1988) 15(2):137–45. doi: 10.1016/0341-8162(88)90025-2
28. Harbo LS, Olesen JE, Liang Z, Christensen BT, Elsgaard L. Estimating organic carbon stocks of mineral soils in Denmark: Impact of bulk density and content of rock fragments. Geoderma Regional (2022) 30:e00560. doi: 10.1016/j.geodrs.2022.e00560
29. Sørensen PD K. Vejledning I forstlig lokalitetskortlægning. Geologisk Institut Aarhus Universitet (1993).
31. Greve MH, Christensen OF, Greve MB, Kheir RB. Change in peat coverage in Danish cultivated soils during the past 35 years. Soil Sci (2014) 179(5):250–7. doi: 10.1097/SS.0000000000000066
32. Orgiazzi A, Ballabio C, Panagos P, Jones A, Fernández-Ugalde O. Lucas Soil, the largest expandable soil dataset for Europe: A review. Eur J Soil Sci (2018) 69(1):140–53. doi: 10.1111/ejss.12499
33. Kristensen JA, Balstrøm T, Jones RJA, Jones A, Montanarella L, Panagos P, et al. Development of a harmonised soil profile analytical database for Europe: A resource for supporting regional soil management. SOIL (2019) 5(2):289–301. doi: 10.5194/soil-5-289-2019
34. Greve MH, Greve MB. Determining and representing width of soil boundaries using electrical conductivity and multigrid. Comput Geosciences (2004) 30(6):569–78. doi: 10.1016/j.cageo.2004.01.005
35. Knadel M, Stenberg B, Deng F, Thomsen A, Greve MH. Comparing predictive abilities of three visible-near infrared spectrophotometers for soil organic carbon and clay determination. J Near Infrared Spectrosc (2013) 21(1):67–80. doi: 10.1255/jnirs.1035
36. (2006). Larsen. Muligheder Og Begrænsninger for Anvendelse Af Gis Og Geodata. Danmarks Jordbrugsforskning.
37. Odgaard MV, Bøcher PK, Dalgaard T, Svenning J-C. Climatic and non-climatic drivers of spatiotemporal maize-area dynamics across the northern limit for maize production–a case study from Denmark. Agriculture Ecosyst Environ (2011) 142(3-4):291–302. doi: 10.1016/j.agee.2011.05.026
38. Levin G, Gyldenkærne S. Estimating land Use/Land cover and changes in Denmark. Technical documentation for the assessment of annual land use/land cover and changes since 2011. Aarhus University, DCE – Danish Centre for Environment and Energy (2022) pp. 39. Technical Report No. 227. Available at: http://dce2.au.dk/pub/TR227.pdf.
39. Assmann JJ, Moeslund JE, Treier UA, Normand S. Ecodes-Dk15: High-resolution ecological descriptors of vegetation and terrain derived from denmark's national airborne laser scanning data set. Earth Syst Sci Data (2022) 14(2):823–44. doi: 10.5194/essd-14-823-2022
40. Jørgensen LF, Stockmarr J. Groundwater monitoring in Denmark: Characteristics, perspectives and comparison with other countries. Hydrogeology J (2009) 17(4):827–42. doi: 10.1007/s10040-008-0398-7
41. Hansen M, Pjetursson B. Free, online Danish shallow geological data. Geus Bull (2011) 23:53–6. doi: 10.34194/geusb.v23.4842
42. Nord-Larsen T, Pretzsch H. Biomass production dynamics for common forest tree species in Denmark–evaluation of a common garden experiment after 50 yrs of measurements. For Ecol Manage (2017) 400:645–54. doi: 10.1016/j.foreco.2017.06.035
43. Nord-Larsen T, Johannsen VK, Jørgensen BB, Bastrup-Birk A. Skove og plantager 2006. Denmark: Skov & Landskab Hørsholm (2008).
44. Jenny H. Factors of soil formation: A system of quantitative pedology. Courier Corporation (1994). doi: 10.2134/agronj1941.00021962003300090016x
46. (CEC) COTEC. Soil map of the European communities, scale 1:1,000,000. Luxembourg: Directorate-General for Agriculture. Office for Official Publications of the European Communities (1985). doi: 10.2134/agronj1941.00021962003300090016x
47. Jacobsen NK. Soil map of Denmark according to the fao-unesco legend. Geografisk Tidsskrift-Danish J Geogr (1984) 84(1):93–8.
48. FAO. Fao-unesco soil map of the world, revised legend, withcorrections and updates. Rome: FAO (1988).
49. Krogh L, Noergaard A, Hermansen M, Greve MH, Balstroem T, Breuning-Madsen H. Preliminary estimates of contemporary soil organic carbon stocks in Denmark using multiple datasets and four scaling-up methods. Agriculture Ecosyst Environ (2003) 96(1-3):19–28. doi: 10.1016/S0167-8809(03)00016-1
50. Greve MH, Greve MB, Bøcher PK, Balstrøm T, Breuning-Madsen H, Krogh L. Generating a Danish raster-based topsoil property map combining choropleth maps and point information. Geografisk Tidsskrift (2007) 107(2):1–12. doi: 10.1080/00167223.2007.10649565
51. Adhikari K, Minasny B, Greve MB, Greve MH. Constructing a soil class map of Denmark based on the fao legend using digital techniques. Geoderma (2014) 214-215:101–13. doi: 10.1016/J.GEODERMA.2013.09.023
52. Adhikari K, Kheir RB, Greve MB, Bøcher PK, Malone BP, Minasny B, et al. High-resolution 3-d mapping of soil texture in Denmark. Soil Sci Soc America J (2013) 77(3):860–76. doi: 10.2136/sssaj2012.0275
53. Adhikari K, Hartemink AE, Minasny B, Bou R, Greve MB, Greve MH. Digital mapping of soil organic carbon contents and stocks in Denmark. PloS One (2014) 9(8):e105519. doi: 10.1371/journal.pone.0105519
54. Adhikari K, Kheir RB, Greve M, Greve M, Malone M, Minasny B, et al. Mapping soil ph and bulk density at multiple soil depths in Denmark. In: GlobalSoilMap: basis of the global spatial soil information system. London: Taylor & Francis (2014). p. 155–60.
55. Balstrøm T, Breuning-Madsen H, Krüger J, Jensen NH, Greve MH. A statistically based mapping of the influence of geology and land use on soil ph: A case study from Denmark. Geoderma (2013) 192:453–62. doi: 10.1016/j.geoderma.2012.08.024
56. Andersen HE, Heckrath GJ. Fosforkortlægning af dyrkningsjord og vandområder I Danmark. DCE-Nationalt Center Miljø og Energi: Aarhus Universitet (2020). pp. 340. Contract No.: 397. Available at: https://dce2.au.dk/pub/SR397.pdf.
57. Møller AB, Heckrath G, Hermansen C, Nørgaard T, de Jonge LW, Greve MH., et al. Mapping the phosphorus sorption capacity of Danish soils in four depths with quantile regression forests and uncertainty propagation. Geoderma (2023) 430:116316. doi: 10.1016/j.geoderma.2022.116316
58. Peng Y, Xiong X, Adhikari K, Knadel M, Grunwald S, Greve MH. Modeling soil organic carbon at regional scale by combining multi-spectral images with laboratory spectra. PloS One (2015) 10(11):e0142295. doi: 10.1371/journal.pone.0142295
59. Bjørn Møller A, Koganti T, Beucher A, Iversen BV, Greve MH. Downscaling digital soil maps using electromagnetic induction and aerial imagery. Geoderma (2021) 385:114852–. doi: 10.1016/J.GEODERMA.2020.114852
60. Kronvang B, Audet J, Baattrup-Pedersen A, Jensen HS, Larsen SE. Phosphorus load to surface water from bank erosion in a Danish lowland river basin. J Environ Qual (2012) 41(2):304–13. doi: 10.2134/jeq2010.0434
61. Olesen SE. Kortlægning af potentielt dræningsbehov på landbrugsarealer opdelt efter landskabselement, geologi, jordklasse, geologisk region samt Høj/Lavbund: Det jordbrugsvidenskabelige fakultet. Institut for Jordbrugsproduktion og Miljø (2009). Available at: https://pure.au.dk/portal/files/45277971/intrma21.pdf.
62. Dalgaard T, Hansen B, Hasler B, Hertel O, Hutchings NJ, Jacobsen BH, et al. Policies for agricultural nitrogen management–trends, challenges and prospects for improved efficiency in Denmark. Environ Res Lett (2014) 9(11):115002. doi: 10.1088/1748-9326/9/11/115002
63. Iversen B, Moldrup P, Schjønning P, Loll P. Air and water permeability in differently textured soils at two measurement scales. Soil Sci (2001) 166(10):643–59. doi: 10.1097/00010694-200110000-00001
64. Møller AB, Iversen BV, Beucher A, Greve MH. Prediction of soil drainage classes in Denmark by means of decision tree classification. Geoderma (2019) 352:314–29. doi: 10.1016/j.geoderma.2017.10.015
65. Beucher A, Møller A, Greve M. Artificial neural networks and decision tree classification for predicting soil drainage classes in Denmark. Geoderma (2019) 352:351–9. doi: 10.1016/j.geoderma.2017.11.004
66. Møller AB, Beucher A, Iversen BV, Greve MH. Predicting artificially drained areas by means of a selective model ensemble. Geoderma (2018) 320:30–42. doi: 10.1016/J.GEODERMA.2018.01.018
67. Iversen BV, Børgesen CD, Lægdsmand M, Greve MH, Heckrath G, Kjærgaard C. Risk predicting of macropore flow using pedotransfer functions, textural maps, and modeling. Vadose Zone J (2011) 10(4):1185–95. doi: 10.2136/vzj2010.0140
68. Sechu GL, Nilsson B, Iversen BV, Møller AB, Greve MB, Troldborg L, et al. Mapping groundwater-surface water interactions on a national scale for the stream network in Denmark. J Hydrology: Regional Stud (2022) 40:101015–. doi: 10.1016/J.EJRH.2022.101015
69. Koch J, Gotfredsen J, Schneider R, Troldborg L, Stisen S, Henriksen HJ. High resolution water table modeling of the shallow groundwater using a knowledge-guided gradient boosting decision tree model. Front Water (2021) 81. doi: 10.3389/frwa.2021.701726
70. Koganti T, Van De Vijver E, Allred BJ, Greve MH, Ringgaard J, Iversen BV. Mapping of agricultural subsurface drainage systems using a frequency-domain ground penetrating radar and evaluating its performance using a single-frequency multi-receiver electromagnetic induction instrument. Sensors (2020) 20(14):3922. doi: 10.3390/s20143922
71. Koganti T, Ghane E, Martinez LR, Iversen BV, Allred BJ. Mapping of agricultural subsurface drainage systems using unmanned aerial vehicle imagery and ground penetrating radar. Sensors (2021) 21(8):2800. doi: 10.3390/s21082800
72. Schneider R, Stisen S, Højberg AL. Hunting for information in streamflow signatures to improve modelled drainage. Water (2022) 14(1):110. doi: 10.3390/w14010110
73. Motarjemi SK, Møller AB, Plauborg F, Iversen BV. Predicting national-scale tile drainage discharge in Denmark using machine learning algorithms. J Hydrology: Regional Stud (2021) 36:100839–. doi: 10.1016/J.EJRH.2021.100839
74. Jarvis N, Koestel J, Larsbo M. Understanding preferential flow in the vadose zone: Recent advances and future prospects. Vadose Zone J (2016) 15(12):vzj2016.09.0075. doi: 10.2136/vzj2016.09.0075
75. Kotlar AM, QdJ vL, HE A, Nørgaard T, Iversen BV. Quantification of macropore flow in Danish soils using near-saturated hydraulic properties. Geoderma (2020) 375:114479. doi: 10.1016/j.geoderma.2020.114479
76. Haris Khan HH, Khan A. “Chapter 14 - Groundwater and Surface Water Interaction” In: Venkatramanan S, Prasanna MV, Chung SY Eds. GIS and Geostatistical Techniques for Groundwater Science. Elsevier (2019). pp. 197–207. doi: 10.1016/B978-0-12-815413-7.00014-6
77. Dahl M, Nilsson B, Langhoff J, Refsgaard J. Review of classification systems and new multi-scale typology of groundwater–surface water interaction. J Hydrology (2007) 344(1-2):1–16. doi: 10.1016/j.jhydrol.2007.06.027
78. Sechu GL, Nilsson B, Iversen BV, Greve MB, Børgesen CD, Greve MH. A stepwise gis approach for the delineation of river valley bottom within drainage basins using a cost distance accumulation analysis. Water (2021) 13(6):827. doi: 10.3390/w13060827
79. Council DAF. Facts & figures. In: Denmark – a food and farming country, vol. 3. . Copenhagen: Danish Agriculture & Food Council (2019). p. 1609. Axelborg, AxeltorvV.
80. Højberg AL, Troldborg L, Stisen S, Christensen BB, Henriksen HJ. Stakeholder driven update and improvement of a national water resources model. Environ Model software (2013) 40:202–13. doi: 10.1016/j.envsoft.2012.09.010
81. Stisen S, Ondracek M, Troldborg L, Schneider RJM, van Thil MJ. National Vandressource Model (in Danish). Modelopstilling Og Kalibrering af DK-model 2019 GEUS Rapport, 2019/31. (2019). Available at: https://vandmodel.dk/media/8096/geusrapport2019_31_dkmodel2019_web-1.pdf.
82. Henriksen H, Kragh S, Gotfredsen J, Ondracek M, van Til M, Jakobsen A, et al. Sammenfatningsrapport vedr. Modelleverancer Til Hydrologisk Informations-Og Prognosesystem. (2020). Available at: https://sdfe.dk/Media/637558092689251964/5majHIP4Plus_sammenfatningsrapport_v23Feb.pdf.
83. Refsgaard JC, Stisen S, Koch J. Hydrological process knowledge in catchment modelling – lessons and perspectives from 60 years development. Hydrological Processes (2022) 36(1):e14463. doi: 10.1002/hyp.14463
84. Windolf J, Thodsen H, Troldborg L, Larsen SE, Bøgestrand J, Ovesen NB, et al. A distributed modelling system for simulation of monthly runoff and nitrogen sources, loads and sinks for ungauged catchments in Denmark. J Environ Monit (2011) 13(9):2645–58. doi: 10.1039/c1em10139k
85. Andersen HE, Kronvang B. Modifying and evaluating a p index for Denmark. Water air Soil pollut (2006) 174(1):341–53. doi: 10.1007/s11270-006-9123-0
86. Schjønning P, Heckrath G, Christensen BT. Threats to soil quality in Denmark-a review of existing knowledge in the context of the EU soil thematic strategy. Aarhus University, Faculty of Agricultural Sciences (2009). Available at: https://dcapub.au.dk/djfpublikation/djfpdf/djfma143.pdf.pdf.
87. Onnen N, Heckrath G, Stevens A, Olsen P, Greve MB, Pullens JW, et al. Distributed water erosion modelling at fine spatial resolution across Denmark. Geomorphology (2019) 342:150–62. doi: 10.1016/j.geomorph.2019.06.011
88. Malaguerra F, Albrechtsen H-J, Thorling L, Binning PJ. Pesticides in water supply wells in Zealand, Denmark: A statistical analysis. Sci Total Environ (2012) 414:433–44. doi: 10.1016/j.scitotenv.2011.09.071
89. Kudsk P, Jørgensen LN, Ørum JE. Pesticide load–a new Danish pesticide risk indicator with multiple applications. Land Use Policy (2018) 70:384–93. doi: 10.1016/j.landusepol.2017.11.010
90. Rosenbom AE, Olsen P, Plauborg F, Grant R, Juhler RK, Brusch W, et al. Pesticide leaching through sandy and loamy fields - long-term lessons learnt from the Danish pesticide leaching assessment programme. Environ pollut (2015) 201:75–90. doi: 10.1016/j.envpol.2015.03.002
91. Beucher A, Adhikari K, Breuning-Madsen H, Greve M, Österholm P, Fröjdö S, et al. Mapping potential acid sulfate soils in Denmark using legacy data and lidar-based derivatives. Geoderma (2017) 308:363–72. doi: 10.1016/j.geoderma.2016.06.001
92. Beucher A, Rasmussen CB, Moeslund TB, Greve MH. Interpretation of convolutional neural networks for acid sulfate soil classification. Front Environ Sci (2022) 679. doi: 10.3389/fenvs.2021.809995
94. Møller AB, Mulder VL, Heuvelink GBM, Jacobsen NM, Greve MH. Can we use machine learning for agricultural land suitability assessment? Agronomy (2021) 11(4):703. doi: 10.3390/AGRONOMY11040703
95. Laville P. Natural terroir units and terroir. a necessary distinction to give more coherence to the system of appellation of origin [Delimitation, geographic information System][1993]. Bull l'OIV (France) (2014) 66:227–51.
96. Peng Y, Roell YE, Møller AB, Adhikari K, Beucher A, Greve MB, et al. Identifying and mapping terrons in Denmark. Geoderma (2020) 363:114174. doi: 10.1016/j.geoderma.2020.114174
97. Roell YE, Peng Y, Beucher A, Greve MB, Greve MH. Development of hierarchical terron workflow based on gridded data–a case study in Denmark. Comput Geosciences (2020) 138:104454. doi: 10.1016/j.cageo.2020.104454
98. Peng Y, Roell YE, Odgers NP, Møller AB, Beucher A, Greve MB, et al. Mapping and describing natural terroir units in Denmark. Geoderma (2021) 394:115014–. doi: 10.1016/J.GEODERMA.2021.115014
99. Priori S, Barbetti R, L'Abate G, Bucelli P, Storchi P, Costantini EA. Natural terroir units, Siena province, Tuscany. J Maps (2014) 10(3):466–77. doi: 10.1080/17445647.2014.885853
100. Vaudour E, Costantini E, Jones G, Mocali S. An overview of the recent approaches to terroir functional modelling, footprinting and zoning. Soil (2015) 1(1):287–312. doi: 10.5194/soil-1-287-2015
101. Ramirez-Villegas J, Jarvis A, Läderach P. Empirical approaches for assessing impacts of climate change on agriculture: The ecocrop model and a case study with grain sorghum. Agric For Meteorology (2013) 170:67–78. doi: 10.1016/j.agrformet.2011.09.005
102. Heumann BW, Walsh SJ, McDaniel PM. Assessing the application of a geographic presence-only model for land suitability mapping. Ecol Inf (2011) 6(5):257–69. doi: 10.1016/j.ecoinf.2011.04.004
103. ESRI. Esri map book. (2022) pp. 196. Available at: https://www.esri.com/en-us/esri-press/browse/the-esri-map-book-volume-3
104. Roell YE, Nord-Larsen T, Beucher A, Greve MB, Greve MH. Mapping tree species ecograms based on soil ph and soil water availability across Denmark. ForestryAnI nternational J For Res Forestry (2022) 95:287–99. doi: 10.1093/foresj/cpab037
105. Roell YE, Beucher A, Møller PG, Greve MB, Greve MH. Comparing a random forest based prediction of winter wheat yield to historical yield potential. Agronomy (2020) 10(3):395. doi: 10.3390/agronomy10030395
106. Larsen S, Jaiswal D, Bentsen NS, Wang D, Long SP. Comparing predicted yield and yield stability of willow and miscanthus across Denmark. GCB Bioenergy (2016) 8(6):1061–70. doi: 10.1111/gcbb.12318
107. Minasny B, Berglund Ö, Connolly J, Hedley C, de Vries F, Gimona A, et al. Digital mapping of peatlands–a critical review. Earth-Science Rev (2019) 196:102870. doi: 10.1016/j.earscirev.2019.05.014
108. Schumann M, Joosten H. Global peatland restoration: Manual. (2008). Available at: http://biblioteca.cehum.org/bitstream/CEHUM2018/1358/1/Schumann%2C%20Joosten.%20Global%20Peatland%20Restoration%20Manual.pdf.
109. Bonn A, Reed MS, Evans CD, Joosten H, Bain C, Farmer J, et al. Investing in nature: Developing ecosystem service markets for peatland restoration. Ecosystem Serv (2014) 9:54–65. doi: 10.1016/j.ecoser.2014.06.011
110. Evans CD, Bonn A, Holden J, Reed MS, Evans MG, Worrall F, et al. Relationships between anthropogenic pressures and ecosystem functions in UK blanket bogs: Linking process understanding to ecosystem service valuation. Ecosystem Serv (2014) 9:5–19. doi: 10.1016/j.ecoser.2014.06.013
111. Mishra S, Page SE, Cobb AR, Lee JSH, Jovani-Sancho AJ, Sjögersten S, et al. Degradation of southeast Asian tropical peatlands and integrated strategies for their better management and restoration. J Appl Ecol (2021) 58(7):1370–87. doi: 10.1111/1365-2664.13905
112. Tanneberger F, Appulo L, Ewert S, Lakner S, Ó Brolcháin N, Peters J, et al. The power of nature-based solutions: How peatlands can help us to achieve key EU sustainability objectives. Advanced Sustain Syst (2021) 5(1):2000146. doi: 10.1002/adsu.202000146
113. Thøgersen F. Danmarks moser. beretning om hedeselskabets systematiske eng-og moseundersøgelser Vol. 1942. . Viborg: Talrige Kort (1942). p. 170.
114. Greve MH, Breuning-Madsen H. Soil mapping in Denmark. In: Soil Resources of Europe, second edition Jones RJA, Houšková B, Bullock P, Montanarella L (eds) Luxembourg: Office for Official Publications of the European Communities (2005). pp. 420. European Soil Bureau Research Report No.9, EUR 20559 EN. (1999) 6:49–56.
115. Parry L, West L, Holden J, Chapman P. Evaluating approaches for estimating peat depth. J Geophysical Research: Biogeosciences (2014) 119(4):567–76. doi: 10.1002/2013JG002411
116. Siemon B, Ibs-von Seht M, Frank S. Airborne electromagnetic and radiometric peat thickness mapping of a bog in Northwest Germany (Ahlen-falkenberger moor). Remote Sens (2020) 12(2):203. doi: 10.3390/rs12020203
117. Kheir RB, Greve MH, Bøcher PK, Greve MB, Larsen R, McCloy K. Predictive mapping of soil organic carbon in wet cultivated lands using classification-tree based models: The case study of Denmark. J Environ Manage (2010) 91(5):1150–60. doi: 10.1016/j.jenvman.2010.01.001
118. Comas X, Slater L. Low-frequency electrical properties of peat. Water Resour Res (2004) 40(12):W12414. doi: 10.1029/2004WR003534
119. Knadel M, Thomsen A, Greve MH. Multisensor on-the-Go mapping of soil organic carbon content. Soil Sci Soc America J (2011) 75(5):1799–806. doi: 10.2136/sssaj2010.0452
120. Beucher A, Koganti T, Iversen BV, Greve MH. Mapping of peat thickness using a multi-receiver electromagnetic induction instrument. Remote Sens (2020) 12(15):2458. doi: 10.3390/rs12152458
121. Møller AB, Greve MH, Beucher A. (2022). Opfølgende bestilling om muligheder for forbedring af kortgrundlag for udpegning af kulstofrige lavbundsjorder. Aarhus Universitet - DCA - Nationalt Center for Fødevarer og Jordbrug. Rådgivningsrapport fra DCA - Nationalt Center for Fødevarer og Jordbrug. Opfølgende Bestilling Om Muligheder for Forbedring Af Kortgrundlag for Udpegning Af Kulstofrige Lavbundsjorder. Aarhus Universitet - DCA - Nationalt Center for Fødevarer og Jordbrug: Rådgivningsrapport fra DCA - Nationalt Center for Fødevarer og Jordbrug. 2022
122. Regina K, Budiman A, Greve MH, Grønlund A, Kasimir Å, Lehtonen H, et al. Ghg mitigation of agricultural peatlands requires coherent policies. Climate Policy (2016) 16(4):522–41. doi: 10.1080/14693062.2015.1022854
123. Naturstylrelsen M. Ringfenner low-lying project in st. vildmose – realisation (2021). Available at: https://naturstyrelsen.dk/media/294399/ringfennertfu_med-bilag.pdf (Accessed September 12, 2022).
124. Greve M, Adhikari K, Beucher A, Heckrath G, Iversen BV, Knadel M, et al. Soil mapping and priorities in Denmark. Geoderma Regional (2022) 29:e00527. doi: 10.1016/j.geodrs.2022.e00527
125. Johnston AE, Poulton PR, Coleman K. Soil organic matter: Its importance in sustainable agriculture and carbon dioxide fluxes. Adv Agron (2009) 101:1–57. doi: 10.1016/S0065-2113(08)00801-8
126. Taghizadeh-Toosi A, Olesen J, Kristensen K, Elsgaard L, Østergaard H, Lægdsmand M, et al. Changes in carbon stocks of d anish agricultural mineral soils between 1986 and 2009. Eur J Soil Sci (2014) 65(5):730–40. doi: 10.1111/ejss.12169
127. Deng F, Minasny B, Knadel M, McBratney A, Heckrath G, Greve MH. Using vis-nir spectroscopy for monitoring temporal changes in soil organic carbon. Soil Sci (2013) 178(8):389–99. doi: 10.1097/SS.0000000000000002
128. Quijano L, Aldana-Jague E, Heckrath G, Van Oost K. Estimating temporal and spatial changes in soil organic carbon stocks and its controlling factors in moraine landscapes in Denmark. CATENA (2021) 206:105502. doi: 10.1016/j.catena.2021.105502
129. Chen S, Arrouays D, Angers DA, Martin MP, Walter C. Soil carbon stocks under different land uses and the applicability of the soil carbon saturation concept. Soil Tillage Res (2019) 188:53–8. doi: 10.1016/j.still.2018.11.001
130. Deluz C, Nussbaum M, Sauzet O, Gondret K, Boivin P. Evaluation of the potential for soil organic carbon content monitoring with farmers. Front Environ Sci (2020) 8:113. doi: 10.3389/fenvs.2020.00113
131. Adhikari K, Owens PR, Libohova Z, Miller DM, Wills SA, Nemecek J. Assessing soil organic carbon stock of Wisconsin, USA and its fate under future land use and climate change. Sci Total Environ (2019) 667:833–45. doi: 10.1016/j.scitotenv.2019.02.420
132. Prout JM, Shepherd KD, McGrath SP, Kirk GJ, Hassall KL, Haefele SM. Changes in organic carbon to clay ratios in different soils and land uses in England and Wales over time. Sci Rep (2022) 12(1):1–13. doi: 10.1038/s41598-022-09101-3
133. Dexter A, Richard G, Arrouays D, Czyż E, Jolivet C, Duval O. Complexed organic matter controls soil physical properties. Geoderma (2008) 144(3-4):620–7. doi: 10.1016/j.geoderma.2008.01.022
134. de Jonge LW, Moldrup P, Schjønning P. Soil infrastructure, interfaces & translocation processes in inner space (" soil-It-Is"): Towards a road map for the constraints and crossroads of soil architecture and biophysical processes. Hydrology Earth System Sci (2009) 13(8):1485–502. doi: 10.5194/hess-13-1485-2009
135. Schjønning P, de Jonge LW, Munkholm LJ, Moldrup P, Christensen B, Olesen J. Clay dispersibility and soil friability–testing the soil clay-to-Carbon saturation concept. Vadose Zone J (2012) 11(1):vzj2011.0067. doi: 10.2136/vzj2011.0067
136. Veihe A, Hasholt B, Schiøtz IG. Soil erosion in Denmark: Processes and politics. Environ Sci Policy (2003) 6(1):37–50. doi: 10.1016/S1462-9011(02)00123-5
137. Govers G, Lobb DA, Quine TA. Tillage erosion and translocation: Emergence of a new paradigm in soil erosion research. Soil Tillage Res (1999) 51:167–74.
138. Heckrath G, Djurhuus J, Quine T, Van Oost K, Govers G, Zhang Y. Tillage erosion and its effect on soil properties and crop yield in Denmark. J Environ Qual (2005) 34(1):312–24. doi: 10.2134/jeq2005.0312a
139. Van Oost K, Van Muysen W, Govers G, Heckrath G, Quine T, Poesen J. Simulation of the redistribution of soil by tillage on complex topographies. Eur J Soil Sci (2003) 54(1):63–76. doi: 10.1046/j.1365-2389.2003.00470.x
140. Jandl G, Baum C, Heckrath G, Greve MH, Kanal A, Mander Ü, et al. Erosion induced heterogeneity of soil organic matter in catenae from the Baltic Sea catchment. Soil Syst (2019) 3(2):42. doi: 10.3390/soilsystems3020042
141. Van Oost K, Govers G, Desmet P. Evaluating the effects of changes in landscape structure on soil erosion by water and tillage. Landscape Ecol (2000) 15(6):577–89. doi: 10.1023/A:1008198215674
142. Thorsøe MHG, Kjeldsen C, Odgaard MV, Dalgaard T. Strategisk miljøvurdering af cap-plan 2023-2027. Report Department of Agroecology, Aarhus University (2021). Available at: https://lbst.dk/fileadmin/user_upload/NaturErhverv/Filer/Tvaergaaende/CAP2020/Temaside/Annex_I_Strategisk_miljoevurdering.pdf.
143. Jensen L, McQueen D, Shepherd T. Effects of soil compaction on n-mineralization and microbial-c and-Ni field measurements. Soil Tillage Res (1996) 38(3-4):175–88. doi: 10.1016/S0167-1987(96)01033-1
144. Schjønning P, van den Akker JJ, Keller T, Greve MH, Lamandé M, Simojoki A, et al. Driver-Pressure-State-Impact-Response (DPSIR) analysis and risk assessment for soil compaction–a European perspective. Adv Agron (2015) 133:183–237. doi: 10.1016/bs.agron.2015.06.001
145. Schjønning P, Lamandé M, Thorsøe M. Soil compaction-drivers, pressures, state, impacts and responses. DCA Rep (2019) 155:34.
146. Schäfer-Landefeld L, Brandhuber R, Fenner S, Koch H-J, Stockfisch N. Effects of agricultural machinery with high axle load on soil properties of normally managed fields. Soil Tillage Res (2004) 75(1):75–86. doi: 10.1016/S0167-1987(03)00154-5
147. Berisso F, Schjønning P, Keller T, Lamandé M, Etana A, De Jonge L, et al. Persistent effects of subsoil compaction on pore size distribution and gas transport in a loamy soil. Soil Tillage Res (2012) 122:42–51. doi: 10.1016/j.still.2012.02.005
148. Schjønning P, Thomsen I. Shallow tillage effects on soil properties for temperate-region hard-setting soils. Soil Tillage Res (2013) 132:12–20. doi: 10.1016/j.still.2013.04.006
149. Van den Akker JJ, Hoogland T. Comparison of risk assessment methods to determine the subsoil compaction risk of agricultural soils in the Netherlands. Soil Tillage Res (2011) 114(2):146–54. doi: 10.1016/j.still.2011.04.002
150. Lamandé M, Greve M, Schjønning P. Risk assessment of soil compaction in Europe–rubber tracks or wheels on machinery. Catena (2018) 167:353–62. doi: 10.1016/j.catena.2018.05.015
151. Poggio L, De Sousa LM, Batjes NH, Heuvelink G, Kempen B, Ribeiro E, et al. Soilgrids 2.0: Producing soil information for the globe with quantified spatial uncertainty. Soil (2021) 7(1):217–40. doi: 10.5194/soil-7-217-2021
152. Vogel HJ, Balseiro-Romero M, Kravchenko A, Otten W, Pot V, Schlüter S, et al. A holistic perspective on soil architecture is needed as a key to soil functions. Eur J Soil Sci (2022) 73(1):e13152. doi: 10.1111/ejss.13152
153. Knadel M, Thomsen A, Schelde K, Greve MH. Soil organic carbon and particle sizes mapping using vis–nir, ec and temperature mobile sensor platform. Comput Electron Agric (2015) 114:134–44. doi: 10.1016/J.COMPAG.2015.03.013
154. Wadoux AMC, Samuel-Rosa A, Poggio L, Mulder VL. A note on knowledge discovery and machine learning in digital soil mapping. Eur J Soil Sci (2020) 71(2):133–6. doi: 10.1111/ejss.12909
155. Wadoux AM-C, Molnar C. Beyond prediction: Methods for interpreting complex models of soil variation. Geoderma (2022) 422:115953. doi: 10.1016/j.geoderma.2022.115953
156. Jones EJ, Bishop TF, Malone BP, Hulme PJ, Whelan BM, Filippi P. Identifying causes of crop yield variability with interpretive machine learning. Comput Electron Agric (2022) 192:106632. doi: 10.1016/j.compag.2021.106632
157. Padarian J, McBratney AB, Minasny B. Game theory interpretation of digital soil mapping convolutional neural networks. Soil (2020) 6(2):389–97. doi: 10.5194/soil-6-389-2020
158. Padarian J, Minasny B, McBratney A, Smith P. Soil carbon sequestration potential in global croplands. PeerJ (2022) 10:e13740. doi: 10.7717/peerj.13740
159. Levin G, Blemmer M, Gyldenkærne S, Johannsen VK, Caspersen OH, Petersen HS, et al. Estimating land use/land cover changes in Denmark from 1990 – 2012. Technical documentation for the assessment of land use/land cover changes for estimation of carbon dioxide fixation in soil. Aarhus University, DCE – Danish Centre for Environment and Energy (2014), 34 pp. Technical Report from DCE – Danish Centre for Environment and Energy No. 38. Availabe at: http://www.dce.au.dk/pub/TR38.pdf
Keywords: digital soil mapping, ecosystem services, sustainable development goals, machine learning, soil security, soil threats, soil functions
Citation: Gomes LC, Beucher AM, Møller AB, Iversen BV, Børgesen CD, Adetsu DV, Sechu GL, Heckrath GJ, Koch J, Adhikari K, Knadel M, Lamandé M, Greve MB, Jensen NH, Gutierrez S, Balstrøm T, Koganti T, Roell Y, Peng Y and Greve MH (2023) Soil assessment in Denmark: Towards soil functional mapping and beyond. Front. Soil Sci. 3:1090145. doi: 10.3389/fsoil.2023.1090145
Received: 05 November 2022; Accepted: 05 January 2023;
Published: 31 January 2023.
Edited by:
Songchao Chen, ZJU-Hangzhou Global Scientific and Technological Innovation Center, ChinaReviewed by:
Brendan Malone, Commonwealth Scientific and Industrial Research Organisation (CSIRO), AustraliaYuxin Ma, Manaaki Whenua Landcare Research, New Zealand
Copyright © 2023 Gomes, Beucher, Møller, Iversen, Børgesen, Adetsu, Sechu, Heckrath, Koch, Adhikari, Knadel, Lamandé, Greve, Jensen, Gutierrez, Balstrøm, Koganti, Roell, Peng and Greve. This is an open-access article distributed under the terms of the Creative Commons Attribution License (CC BY). The use, distribution or reproduction in other forums is permitted, provided the original author(s) and the copyright owner(s) are credited and that the original publication in this journal is cited, in accordance with accepted academic practice. No use, distribution or reproduction is permitted which does not comply with these terms.
*Correspondence: Lucas Carvalho Gomes, bHVjYXMuZ29tZXNAYWdyby5hdS5kaw==