- 1Department of Land, Air and Water Resources, University of California, Davis, Davis, CA, United States
- 2Department of Plant Sciences, University of California, Davis, Davis, CA, United States
- 3Bioinformatics Core Facility, Genome Center, University of California, Davis, Davis, CA, United States
Introduction: Irrigation management dramatically alters soil water availability and distribution and could impact soil microbial communities and carbon (C) and nitrogen (N) cycling to an even greater degree than observed in rainfed systems. Adoption of subsurface drip irrigation (SDI) in California’s Mediterranean agroecosystems provides agronomic benefits but wets only a portion of the soil volume near the root zone, leaving the rest dry throughout the growing season. In contrast, traditional furrow irrigation (FI) has periodic wetting events with more homogenous moisture distribution. With conversion to precision irrigation methods, how will the microbiome respond to changes moisture availability, and how is their response influenced by soil C and N resource levels?
Methods: In a field experiment in California, we compared SDI and FI’s effects on microbial communities and evaluated how long-term organic and conventional management systems impact outcomes. Throughout the growing season, soil samples were collected at two depths (0-15, 15-30 cm) and three distances from bed center (10, 25, 45 cm) where the drip tape is located.
Results: At harvest, soils irrigated using SDI had lower microbial biomass C (MBC) than under FI at the surface and showed a build-up of soluble C and N relative to MBC at the bed edge, indicating reduced microbial uptake. Community composition at the bed edge also diverged between SDI and FI, favoring Actinobacteria in the former and Acidobacteria and Gemmatimonadetes in the latter. Regardless of irrigation type, dry areas of the bed had the highest alpha diversity indices. Response to SDI was similar in organic and conventional systems, though organic had higher MBC, DOC, and relative abundance of Proteobacteria and fungal lipids, regardless of irrigation.
Discussion: Prolonged dry conditions in SDI appeared to limit microbial access to resources and changed community composition. As seen in non-agricultural systems, the severity and frequency of moisture changes, adaptation of the communities, and resource availability affect microbial response. Decoupling of C and N pools in dry surface soils under SDI may increase the potential for losses of DOC and nitrate with the first winter rains in this Mediterranean climate.
1 Introduction
Agricultural soils rely on networks of soil microbes to decompose organic matter, cycle nutrients to plant-available forms, and create compounds that build soil aggregates to promote soil tilth (1–6); the resiliency of these important functions through changing land uses and environmental conditions, whether from natural or managed causes, is key to long-term health and productivity of cropping systems (7). Systems with high carbon-based inputs, such as organic systems, are particularly reliant on microbial activity.
Microbial communities and their processes are highly sensitive to changes in soil moisture, be it desiccation, rapid changes in water potential, anoxic conditions, or interactions with soil temperature (8, 9). As periods of soil drying are projected to increase in severity and duration in many terrestrial ecosystems, particularly in arid and semi-arid climates (10), soil microbial processes will be influenced directly by their physiological response to lack of water and indirectly by impacts on substrate dissolution, diffusion, and transport (11–13). Rewetting events after prolonged dry periods create their own perturbation to the system, often causing cell death with a sudden change in water potential while also creating a resource flush that can drive a burst of microbial activity (12). Therefore, impacts of wet-dry cycling on microbial communities and processes can have implications for key soil functions and ecosystem carbon (C) and nitrogen (N) balances. The effects will depend on the duration of the dry period, the magnitude of change in soil moisture, the degree to which the microbial communities are adapted to wet-dry cycles, and the resources (e.g., soil organic C) available to the biota (12, 13).
Microbial cells respond to moisture stress by modifying allocation of resources from building biomass to tactics that will improve chances of survival, such as uptake or production of osmolytes, energetically expensive compounds that regulate cellular water potential and maintain hydration (8, 13). Therefore, direct (e.g., organic matter inputs) and indirect (e.g., moisture-limited substrate diffusivity) impacts on soil resource availability will have implications for microbial biomass response to dry-down and rewetting cycles. Two major hypotheses have been proposed describing the potential impact of wet-dry cycles on microbial biomass and resource availability. The first states that microbial biomass decreases as the number of wet-dry cycles increases (14) and resources needed to produce osmolytes are used up, hampering a cell’s capacity to withstand moisture stress and capitalize on wetter conditions for growth and functions (8, 15). The second suggests that microbial biomass increases with wet-dry cycling in response to C and N-rich substrates released during rewetting events as aggregates disperse and previously occluded compounds come into solution, creating the “Birch Effect,” or a pulse of CO2 release due to a peak in mineralization activity (16, 17). In reality, both mechanisms occur simultaneously (15, 18), and dead microbial cells (i.e., necromass) and osmolytes released from stressed microbial cells provide substrates for others (13). The balance of these processes, and the resulting fate of soil C and N, will again be moderated by the severity and frequency of the wet-dry cycles, life strategy adaptations of the microbial community to those cycles, and soil organic C concentrations (12, 13, 19).
Foundational studies conducted in non-agricultural systems have shown that soil communities in arid and semi-arid climates often become adapted to prolonged dry periods and rapid rewetting (12) and that community compositions shift as moisture changes (e.g., 20–22). Gram-negative bacteria tend to be more susceptible to drought and rewetting shock, while many Gram-positive bacteria and fungi have thicker cell walls, ability to sporulate, and are more resistant to water stress, causing proliferation for these groups in systems with long dry periods (23). Microbial diversity may be affected if selection for adapted organisms occurs, though impacts of drought on diversity have been variable and seemingly dependent on pre-exposure of the communities (23). Changes in community composition may have implications for biogeochemical cycling, as organisms within these groups have different nutrient cycling capabilities, C source preferences, and ability to maintain function in dry conditions (8, 23). The response to soil moisture co-occurs with response to shifts in substrates, and therefore it is still unclear the degree to which drying and rewetting shapes soil community metabolic processes and thus soil organic matter composition and stability (13).
Agricultural soils are subject to these moisture perturbations just like non-agricultural soils, but in irrigated systems, the magnitude and frequency of wet-dry perturbations will be controlled by the water application method. Precision irrigation management technologies such as subsurface drip irrigation (SDI) can dramatically alter water availability and distribution within the soil profile compared to traditional methods that wet more of the soil from the surface. Even in dry climates, microbial communities in traditionally irrigated soils may not be adapted to prolonged drying and rewetting. How will these communities respond to changes moisture availability, and how is their response influenced by soil C and N resource levels? For many years, processing tomatoes (Solanum lycopersicum L.) in California’s Central Valley were traditionally irrigated using furrow irrigation (FI), where the spaces between beds (furrows) are filled with water that infiltrates and seeps laterally throughout the bed. Now, SDI is used on the majority of the state’s tomato acreage (24, 25). Precision placement of water and nutrients (particularly using soluble mineral fertilizer) through drip lines has improved yields, water use efficiency, crop quality, and weed control compared to FI and other surface irrigation methods (24, 26–28). However, a major consequence of this shift in irrigation is that now a substantially smaller volume of soil (roughly 25% in the upper 30 cm) receives water and is continuously wet, with a major portion of the soil left dry until winter rains occur. Thus, SDI creates more severe and extended wet-dry cycles than the moderate fluctuations in FI. Schmidt et al. (2018) and Li et al. (2020) explored potential agroecosystem trade-offs of SDI in organic systems, including soil salinity, aggregate stability, root distribution, and C and N cycling enzymes and functional genes. However, much remains to be known about the response of microbial communities, including microbial biomass, community composition, and their relationships with available C, N, and moisture resources.
We investigated how SDI and FI affect soil microbial biomass, composition, diversity, as well as extractable C and N pools within specific bed zones in organic and conventional agricultural management systems that differ in C and N inputs. We hypothesized that 1) extended dry periods at the surface and edge of the beds in the SDI system negatively affect microbial communities, resulting in lower microbial biomass compared to moderate wet-dry cycles in the FI system; 2) SDI shifts microbial community composition toward more drought stress-tolerant taxa in dry areas of the beds, reducing microbial diversity; 3) effects of SDI on microbes are more pronounced in organic than conventional management systems due to greater resource availability (extractable C and N) in organic systems and reliance on microbial activity for nutrient and organic matter cycling. The potential impacts of shifting irrigation practices on soil microbial communities may have implications for the resiliency of the important functions they provide.
2 Materials and methods
2.1 Field experimental design
This study was located at the Russell Ranch Sustainable Agricultural Facility, part of the University of California Davis’s Agricultural Sustainability Institute in Davis, CA (asi.ucdavis.edu/programs/rr). The experimental plots are part of Russell Ranch’s Century Experiment, which began in 1993 (29, 30). We compared two management systems: “conventional” (Conv), which receives mineral fertilizer, and “organic” (Org), which is amended with poultry manure compost annually and has a winter cover crop mix of bell bean (Vicia faba L.), vetch (Vicia villosa), and oats (Avena sativa L.). Though Org and Conv differ based on more than just fertility inputs, for the purposes of this paper we will refer to these as “fertility systems.” Both systems are in an annual rotation of maize (Zea mays L.) and processing tomatoes (Solanum lycopersicum L., variety Heinz 8504). All Century Experiment plots were under FI until 2015, when most irrigated plots were converted to SDI with the drip line buried at 30 cm in the center of the 100-cm beds. An exception was three organic plots in which only one-quarter of the plot was converted to SDI (as described in 28). In Spring 2017, immediately prior to the beginning of this project, the irrigation in these plots was re-established, and half of each plot was switched to SDI while half remained under FI.
Management treatments were 1) organic, furrow-irrigated (Org-FI), 2) organic, subsurface drip-irrigated (Org-SDI), and 3) conventional, subsurface drip-irrigated (Conv-SDI). Each treatment was replicated three times in 0.2-ha (Org-FI and Org-SDI) or 0.4-ha (Conv-SDI) plots, and treatment plots were blocked with one replicate of each treatment per block. A Conv-FI system was not in place, but these three treatments allowed comparisons of FI and SDI in the organic system and of Conv and Org within SDI plots.
The soils are a fine, smectitic, thermic Mollic Haploxeralf (Blocks 1 and 2; Rincon silty clay loam) and a fine-silty, mixed, superactive, nonacid, thermic Mollic Xerofluvent (Block 3; Yolo silt loam). At the time of this study, Org soils had an average total C content of 1.34% C in the surface (0-15 cm) and 1.08% C in the subsurface (15-30 cm), while Conv soils had 0.97% C from 0-15 cm and 0.88% C from 15-30 cm. Soil pH in Org plots averaged 7.36 and 7.83 at each depth, respectively, and in Conv plots averaged 7.45 and 7.96. Soil characterization data in all treatments at all measured depths can be found in Supplementary Table S1. During the 2017 growing season, maximum air temperature averaged ~32 °C and minimum air temperature averaged ~14 °C. Total precipitation was 19.6 mm, which came almost entirely in early June (31).
Prior to tomato transplanting on May 1, 2017, both Org treatments had cover crop biomass incorporated (March 2017) and received 9 T ha-1 of poultry manure compost (April 2017) either trenched above the drip line (Org-SDI) or spread on the surface and disked to 15 cm (Org-FI), in keeping with typical practices of local growers. The Conv-SDI plot had 28 kg ha-1 of liquid starter fertilizer applied through shanking (April 2017) with 8% ammonia N, 25% phosphate (P2O5), 6% soluble potash (K2O). During the growing season, Conv-SDI plots also received urea ammonium nitrate (UAN-32) through the drip line four times and potassium fertilizer once during the growing season (Supplementary Figure S1). Irrigation water use was measured on one replicate plot per treatment using flow meters, and replicates of each treatment were irrigated similarly. Org-FI plots were irrigated by flooding alternate furrows every 3-5 days for a total of 21 irrigation events delivering ~11,222 m3 ha-1 of water over a 12-week period (Supplementary Figure S1). SDI treatments were irrigated 5-6 days per week for 3-8 hours in a single event, delivering a total of ~4,859 m3 ha-1 of water over 64 irrigation events.
2.2 Soil sampling
The triplicate plots of each treatment were sampled four times between transplant and harvest: early May (1 week after transplanting), mid-June (early green fruit stage), mid-July (pink fruit stage), and mid-August (1 week before harvest). At each time point, two sets of three soil cores were collected from a randomly selected location in each plot. In each set, 45-cm deep cores were taken at 10 cm, 25 cm, and 45 cm from the center of the bed and then divided into 15-cm depth segments (Supplementary Figure S2). Samples were taken using a 5-cm diameter hand corer with removable plastic liner tubes (Giddings Machine Company, USA). Core sets in each plot were collected 89 cm apart or 2.5 times the lateral distance between buried drip emitters (35.5 cm) to account for the unknown location of the emitters in relation to the cores. Cores from each set were analyzed separately for gravimetric water content (GWC), ammonium-N (NH4+-N), nitrate-N (NO3–-N), dissolved organic carbon (DOC), and microbial biomass carbon (MBC), then results of paired samples from each set were averaged. For all other analyses, corresponding samples from sets within a plot were composited and analyzed.
All samples were immediately subsampled (~45 g) and analyzed for GWC (drying at 105 °C for 72 h). Another subsample was immediately frozen for phospholipid fatty acid (PLFA) analysis and 16S rRNA gene sequencing while the remaining portion of each sample was stored at 4 °C until further analysis.
2.3 Soil chemical analyses
For NH4+-N, NO3–-N, and DOC measurements, 20 g of field-moist soil, sieved to 8 mm, was extracted with 100 mL of 0.5 M potassium sulfate (K2SO4). Extracts were analyzed colorimetrically using the salicylate-hypochlorite method for NH4+-N (32) and vanadium (III) chloride reduction method for NO3–-N (33). Scaled-down versions of these methods were used in 96-well microplates. Absorbance values were measured at 540 nm (NO3–N) and 650 nm (NH4+-N) on a Tecan GENios microplate reader. NH4+-N concentrations were low (< 3 mg kg-1) throughout most of the season, and therefore we report total inorganic N as the sum of extractable NH4+-N and NO3–-N. Extracts were also analyzed for DOC on a Shimadzu TOC-V Analyzer (Shimadzu, Duisburg, Germany). MBC was analyzed using the chloroform fumigation extraction method (34, 35), with 20-g samples extracted with 0.5 M K2SO4 as above after a 72-h fumigation (extended from the typical 24-h fumigation due to the high clay content of these soils). MBC is reported as the difference between fumigated and unfumigated (DOC) samples; no correction factors were applied. Soil pH was measured on a 2:1 ratio of nanopure water to air-dried soil. Total C and N were determined by combustion elemental analysis (Costech Analytical Technologies, Inc., Valencia, CA).
2.4 Phospholipid fatty acid analysis
PLFA profiles were measured on samples from May (1 week post-transplant) and August (1 week pre-harvest). Freeze-dried soils were sent to Microbial ID, Inc (Newark, DE) for extraction, analysis, and identification of lipids following the procedures described in Buyer and Sasser (2012: 36). Lipids were analyzed with gas chromatography (Agilent Technologies, Santa Clara, CA) and identified using MIDI, Inc.’s Sherlock PLFA Analysis Software. Individual lipids were assigned to microbial groups according to Buyer and Sasser (2012).
2.5 DNA extraction and 16S rRNA amplicon sequencing
Amplicon sequencing of the 16S rRNA gene was performed on samples collected in May and August. DNA was extracted from 0.25g of frozen soil from each sample using MoBio PowerSoil DNA isolation kits (MoBio Laboratories, Carlsbad, CA, USA) and quantified using a Qubit dsDNA HS Assay kit and fluorometer (Life Technologies, Grand Island, NY, USA). The V4 hypervariable region of the 16S rRNA gene was amplified in duplicate PCR reactions for each DNA extract using 515F and 806R primers (37). For library preparation, each reaction had 10 µL Phusion High-Fidelity PCR Master Mix (Thermo Scientific, Waltham, MA), 1 µL of each primer (10 µM), 1 µL bovine serum albumin (BSA), and 14 ng DNA. Reactions were completed on a C1000 Touch Thermocycler (BioRad Laboratories, Inc., Hercules, CA), and negative controls were included in each run using sterile nanopure water instead of DNA. PCR products, including negative controls, were verified on 1.5% agarose gels and quantified using a Qubit 3 fluorometer (Thermo Fisher Scientific Inc., Waltham, MA. Next, 50 ng of product of each duplicate reaction (100 ng per sample) was pooled and purified with the Qiagen QIAquick PCR Purification kit (Qiagen, Inc., Germantown, MD). The library was sequenced on an Illumina MiSeq PE250 platform in the Genome Center DNA Technologies Core at UC Davis. This yielded 15.95 million reads (8.7% PhiX) with an overall Q30 > 83%.
DNA sequence reads were demultiplexed and classified using the custom Python application dbcAmplicons, version 0.6.7 (https://github.com/msettles/dbcAmplicons), to identify and assign reads by both expected barcode and primer sequences. During pre-processing, barcodes were allowed to have ≤1 mismatch (hamming distance), and primers were allowed to have ≤4 mismatches (Levenshtein distance) as long as the final 4 bases of the primer perfectly matched the target sequence. Reads were then trimmed of their primer sequence and merged into a single amplicon sequence using a modified version of FLASH2, version 2.2.00 (https://github.com/dstreett/FLASH2) (38). The Ribosomal Database Project Bayesian classifier (39), version 2.0.2, was used to assign sequences to phylotypes. Reads were assigned to the first Ribosomal Database Project taxonomic level with a bootstrap score ≥50.
2.6 Statistical analyses
2.6.1 Analysis of variance
Analyses were conducted using the R statistical software environment, version 3.4.4 (40). Treatment effects and spatial patterns for soil moisture, soil chemical parameters (DOC, MBC, and inorganic N), and relative abundance of microbial groups (PLFA) and phyla (16S) were analyzed with analysis of variance (ANOVA) and mixed models using the lmer function in the lme4 R package (41). Treatment, distance from bed center, depth, and their interactions were fixed effects while block was a random effect. When analyzing repeated measures, timepoint was included as a fixed effect and plot (experimental unit) was included as a random effect. Model assumptions of normality and homogeneity of variance were tested with diagnostic plots and the Shapiro-Wilk test, and data were transformed using natural log or square root transformations if assumptions were not met. If interactions with depth were significant (p < 0.05), treatment and distance factors were analyzed within each depth. If treatment*distance interactions were significant, simple effects were analyzed using ANOVA and Tukey’s Honest Significant Difference (HSD.test with the agricolae package (42). To assess the trends of each treatment as a function of distance from bed center, linear models (lmer) were compared to quadratic models and chosen based on lower Bayesian Information Criterion (BIC). Significance of the linear model slopes was assessed to test the relationship with distance from bed center. Otherwise, the main effects of each factor were analyzed. Plots were generated using the ggplot2 package in R (43).
2.6.2 PLFA analysis
Canonical correspondence analysis (CCA) was performed on lipid profiles using the cca function in the vegan R package (44). Exploratory models with combinations of all soil variables were tested, and ultimately a model with GWC, DOC, and inorganic N was chosen since it minimized multicollinearity as indicated by the variance inflation factor (VIF < 2). Significance of model factors and axes was analyzed by a permutational significance test using the anova function in the vegan package (1000 permutations).
2.6.3 16S rRNA gene sequencing analysis
Differential abundance analyses were conducted using the limma-voom Bioconductor pipeline (Ritchie et al., 2015), which used packages limma version 3.32.10 and edgeR version 3.18.1. In brief, this pipeline calculates normalized counts using TMM normalization, log transforms them, calculates variance weights using the voom algorithm (45), fits a linear model using weighted least squares to each taxon, and applies empirical Bayes shrinkage to the standard errors of the model coefficients (46). The model fitted included effects for timepoint, treatment, distance from the bed center and depth, their interactions, and block as a random effect. Standard errors for estimates were adjusted for within-plot correlations. Associations between taxon abundance and continuous environmental variables such as GWC were analyzed in the limma-voom pipeline using models with effects for the environmental variable and block. Standard errors of estimates were adjusted for within-plot correlation. Additionally, to evaluate how genera within the broader taxonomic groups were affected by these variables, we created regressions of each variable against the log2-fold change in absolute abundance of a genus normalized for sample-wide differences in total abundance. We tested the significance of these regressions by determining the Benjamini-Hochberg false discovery rate adjusted p-value (p < 0.05).
Shannon and Chao1 alpha diversity indices were calculated at the genus level in R using the estimate_richness function in the phyloseq package, version 1.20.0 (47), and were analyzed using a linear mixed effects model in nlme (48) with fixed effects for timepoint, treatment, distance from bed center and depth (categorical) and their interactions and with random effects for block and plot (experimental unit). Associations between alpha diversity and continuous environmental variables were analyzed using linear mixed effects models with fixed effects for the environmental variable and random effects for block and plot.
3 Results
3.1 Soil moisture patterns
Irrigation treatments altered spatial patterns of soil moisture throughout the growing season. At the soil surface (0-15 cm), average seasonal GWC (June-August) was similar between treatments at the bed center (10-cm distance; 0.143-0.148 g g-1) but was lower in Org-SDI and Conv-SDI than Org-FI at the 25- and 45-cm bed distances (Figure 1; Supplementary Table S2). Generally, SDI treatments showed stronger gradients in GWC at both 0-15 cm and 15-30 cm depths, getting drier with distance from the bed center. All treatments were wetter at 15-30 cm than in the surface layer (p < 0.0001; Supplementary Table S2). Though the 30-45 cm depth showed strong moisture gradients in SDI, where the center at this depth was the wettest portion of the bed, we saw no treatment effects for other parameters. Therefore, soil chemical and microbial results from the 30-45 cm depth are not presented.
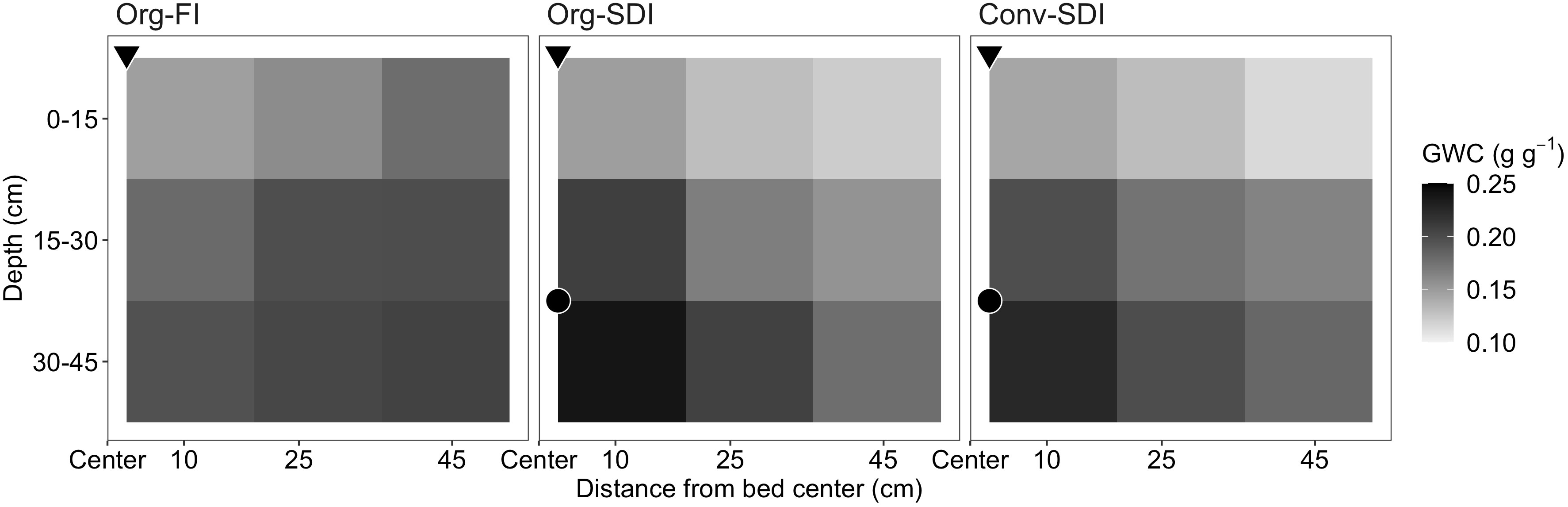
Figure 1 Mean gravimetric water content (GWC) at different depths and distances from bed center in Org-FI, Org-SDI, and Conv-SDI treatments. Values shown are an average of measurements from June, July, and August. The position of the plant and drip line are represented by a black triangle and black circle, respectively.
3.2 Microbial biomass and extractable C and N
MBC was most affected by irrigation treatments at the soil surface (0-15 cm) despite larger moisture differences between SDI and FI in the subsurface (15-30 cm). At the bed center, Org-SDI and Org-FI had similar MBC throughout the season, but at the bed edge, the mean MBC in Org-SDI was 54% lower than Org-FI in July (p = 0.0643) and 32% lower than Org-FI in August (Figure 2A; p = 0.0796). Both Org treatments had similar DOC concentrations throughout most of the season and across the bed, except in August at the bed edge, where Org-SDI had 24% higher DOC than Org-FI plots (Figures 2B, 3B; p = 0.0005).
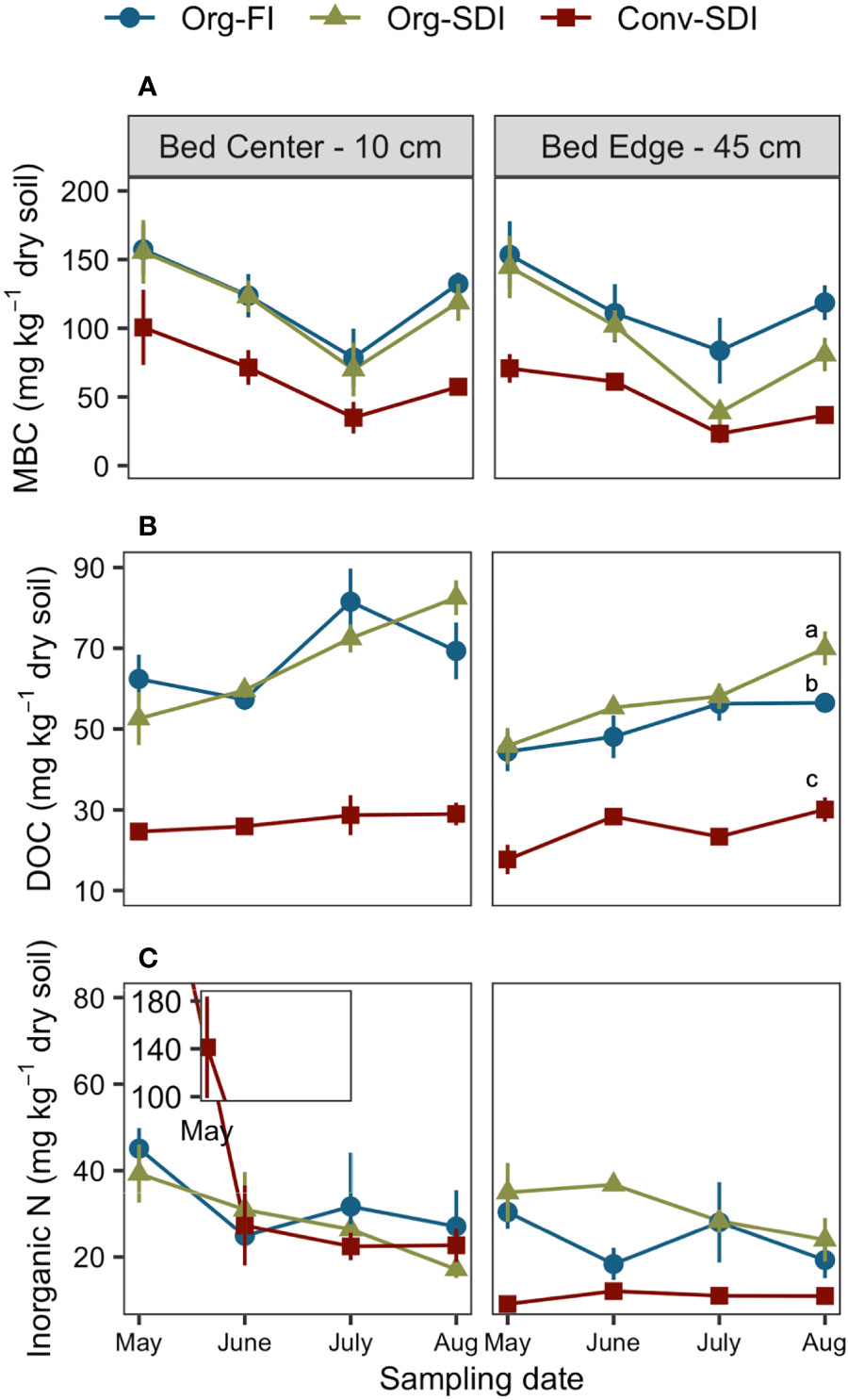
Figure 2 Mean ± SE of (A) microbial biomass carbon (MBC), (B) dissolved organic carbon (DOC), and (C) inorganic N (sum of extractable NH4+-N and NNO3–-N) over the growing season in each treatment at the center (10 cm) and edge (45 cm) of the beds. Data shown are from the 0-15 cm depth. Lower case letters indicate significant differences between treatments (Tukey HSD, α = 0.05) at a particular time point when Treatment*Distance interactions were significant.
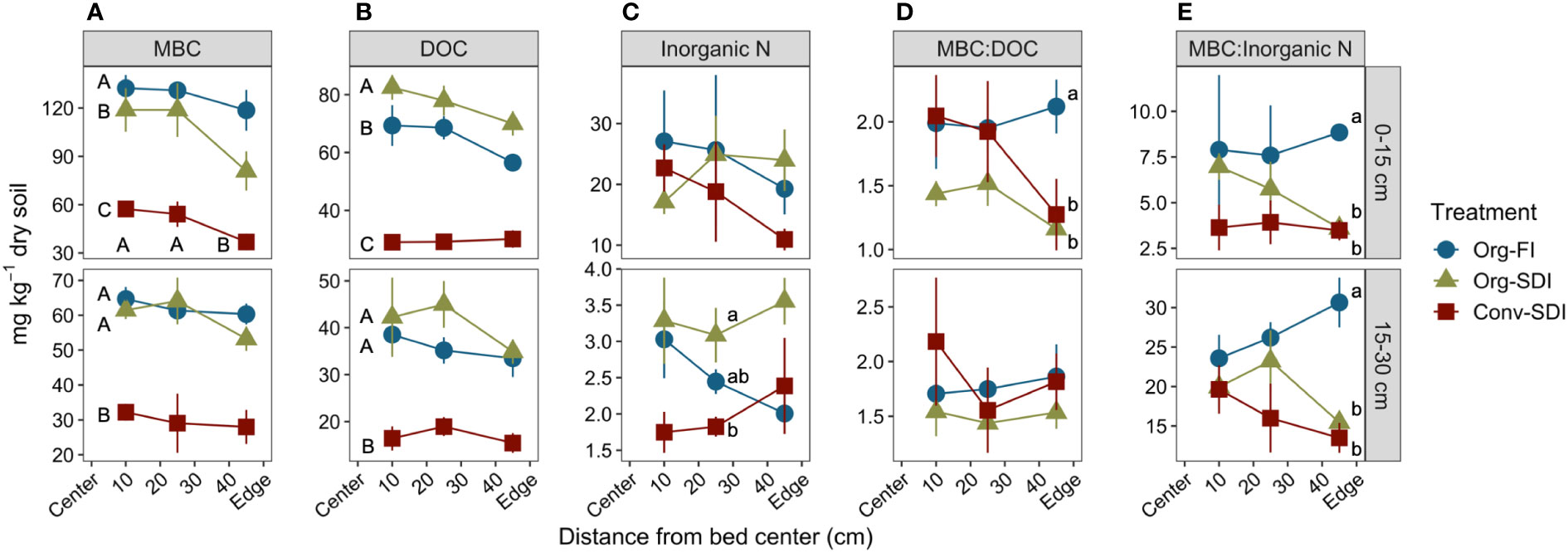
Figure 3 Mean ± SE of (A) microbial biomass carbon (MBC), (B) dissolved organic carbon (DOC), (C) the ratio of concentrations of MBC-to-DOC, (D) inorganic N (sum of extractable NH4+-N and NO3–-N), and (E) the ratio of concentrations of inorganic N -to-MBC for each treatment as a function of distance from the bed center. Data shown are from samples collected in August from 0-15 cm (top row) and 15-30 cm (bottom row) depths. Lower case letters indicate significant differences between treatments (Tukey HSD, α = 0.05) at a particular distance when Treatment*Distance interactions were significant, and capital letters indicate significant differences between treatments (Tukey HSD α = 0.05) across distances when the interaction was non-significant.
The fertility system had even greater effects on these pools. Throughout the growing season, the average MBC across the bed was 40-64% lower in Conv-SDI than Org-SDI and Org-FI at the 0-15 cm depth (p < 0.0001; Figure 2A) and was 31-53% lower at the 15-30 cm depth (seasonal data not shown, p = 0.0012). The Conv treatment had average DOC concentrations across the bed 46-63% lower than Org treatments at 0-15 cm (Figure 2B, p < 0.0001) and 49-69% lower at 15-30 cm (seasonal data not shown, p = 0.0020). Inorganic N at the bed center had similar seasonal dynamics across all treatments except for the Conv-SDI treatment in May, which had approximately 3.5 times more inorganic N than the Org treatments (Figure 2C). At the bed edge, both Org treatments had higher inorganic N concentrations than Conv throughout the season (p = 0.0001).
Differences between SDI and FI and significant time-by-treatment interactions were most apparent at the end of the growing season. Therefore, spatial patterns (depth and distance) of MBC and extractable C and N pools were explored further for the August timepoint, also coinciding with microbial community analyses. In August, all treatments had similar trends in MBC and DOC across the bed (non-significant distance factor and treatment*distance interaction, α = 0.05), but differed in concentrations (Figures 3A, B). At the 0-15 cm depth, Org-FI had greater MBC than Org-SDI across the bed (p = 0.0359), while Org-SDI had higher DOC concentrations. At 15-30 cm, Org-FI and Org-SDI had similar MBC and DOC and Conv-SDI had lower concentrations than the Org treatments at both depths. Inorganic N concentrations did not differ by treatment at the surface (p = 0.4267), but in the subsurface, SDI and FI had contrasting trends in extractable inorganic N (pTrt*Dist = 0.0274), with FI showing decreasing concentrations with distance from bed center and SDI treatments increasing (Figure 3C).
We evaluated microbial metabolic quotients by quantifying microbial biomass relative to available C and N resources using the ratios of MBC:DOC and MBC:inorganic N. The ratios of MBC:DOC in Org-SDI and Org-FI were similar at the 10- and 25-cm distances (0-15 cm depth), but at the 45-cm distance, the MBC:DOC ratio in Org-SDI was lower (p = 0.0488), close to the same level as Conv-SDI (Figure 3D). A similar pattern was found for the MBC:inorganic N ratio at the bed edge at both depths, with Org-FI having higher MBC per unit of extractable N than both SDI treatments (Figure 3E; p = 0.0023).
3.3 Phospholipid fatty acid analysis
In May, PLFA profiles of the microbial communities in surface soils did not differ by treatment at the bed center (Figure 4A), and at the bed edge were affected by only fertility system, with Org and Conv systems separating along the primary CCA axis (51.3% of variation explained, p = 0.010; Figure 4B). In August, PLFA profiles separated along the first axis by fertility system at bed center (31.4%, p = 0.071; Figure 4C) and by both fertility system and irrigation management at the bed edge (Figure 4D), with samples from all three treatments clustered separately and fertility system diverging along the first axis (34.6%, p = 0.002) and irrigation system separating along the secondary axis (21.7%, p = 0.032). These results show that irrigation treatments caused a rapid shift in microbial community composition during the 3.5-month growing season. DOC concentration was the primary explanatory variable for separation of the fertility system treatments and was significant in both CCAs in August (10 cm: pDOC = 0.011; 45 cm: pDOC = 0.002). At the bed edge, GWC was associated the separate clustering of Org-FI and Org-SDI (pGWC = 0.010). Shift in PLFA composition associated with irrigation management was only seen at the surface soil. At the 15-30 cm depth in August, lipid profiles separated along the primary axis by fertility system (Supplementary Figure S3); DOC explained this separation and was positively associated with the Org systems (10 cm: pDOC = 0.014; 45 cm: pDOC = 0.029).
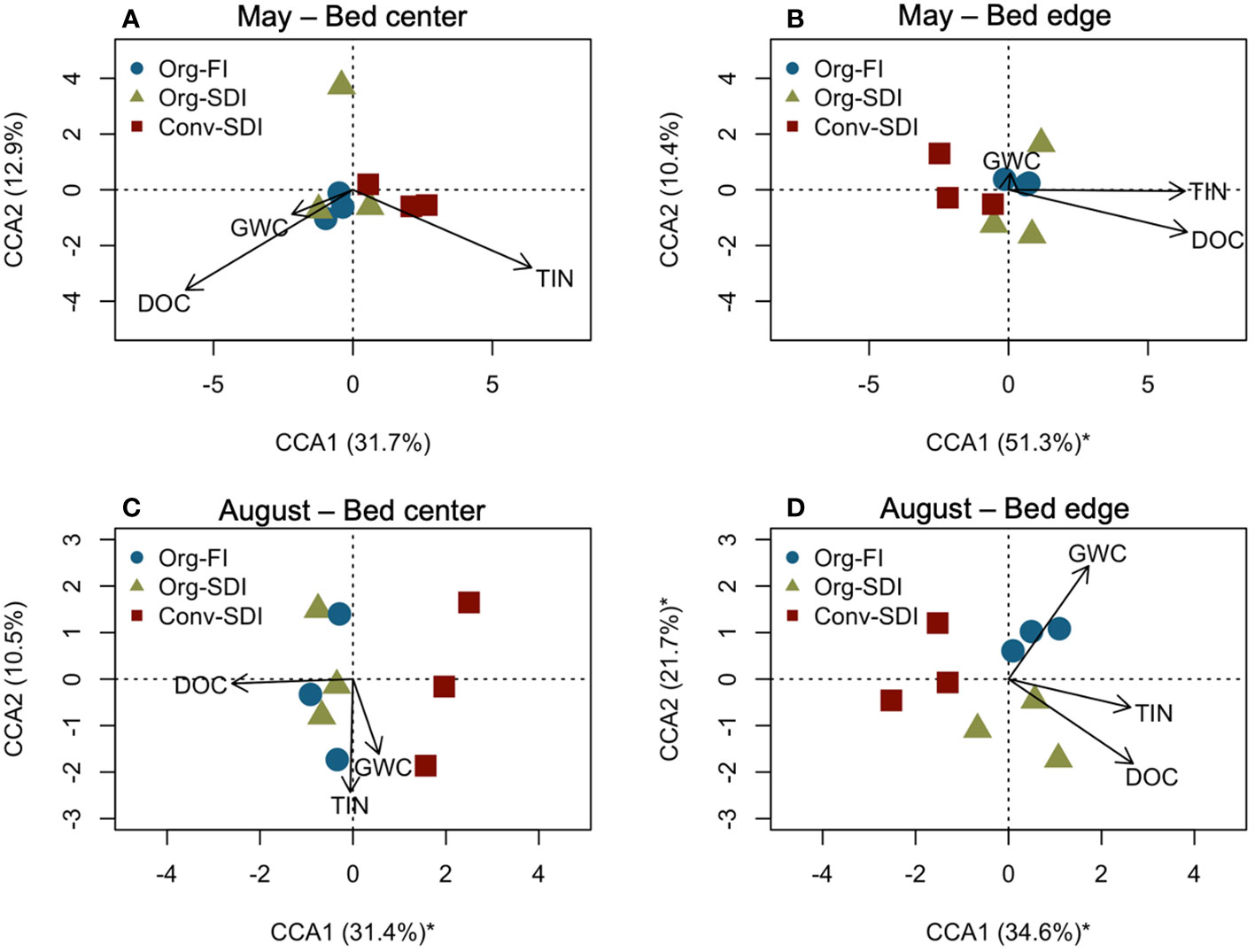
Figure 4 Canonical correspondence analysis (CCA) ordination of PLFA lipids from 0-15 cm in May at (A) the bed center (10-cm distance) and at (B) the bed edge and in August at (C) the bed center and (D) bed edge. Vectors represent soil physiochemical properties constraining the analysis and were selected to explain as much variation as possible while minimizing redundancy of constraining factors. The proportion of the total variation explained by each axis is presented, and an asterisk indicates statistical significance of that axis (p < 0.05) based on permutation tests. DOC, Dissolved organic carbon; GWC, Gravimetric water content; TIN, Total inorganic nitrogen, summation of extractable NO3–-N and NH4+-N.
Though CCA of lipid profiles in August showed limited effects of irrigation from 15-30 cm, there were several cases at this depth where SDI treatments caused relative abundance of specific PLFA marker groups to trend positively or negatively as a function of distance from bed center, while the FI treatment was more homogeneous across the bed (Figure 5). In Org-SDI and Conv-SDI, relative abundance of actinomycetes and the Gram+:Gram– ratio increased moving towards the bed edge, while lipids associated with eukaryotes and the fungi:bacteria ratio decreased (see slopes and p-values in Supplementary Table S3). These relationships were not found in soils collected in May (data not shown), further evidence that microbiome shifts occurred during the growing season in response to irrigation management. Total PLFA concentrations, another measurement of microbial biomass, were homogenous across the bed in Org-FI, but declined moving towards the bed edge in both SDI treatments (Supplementary Tables S3, S4). Across all systems, total PLFA concentrations had a significant positive relationship with MBC (R2 = 0.62; p < 0.0001).
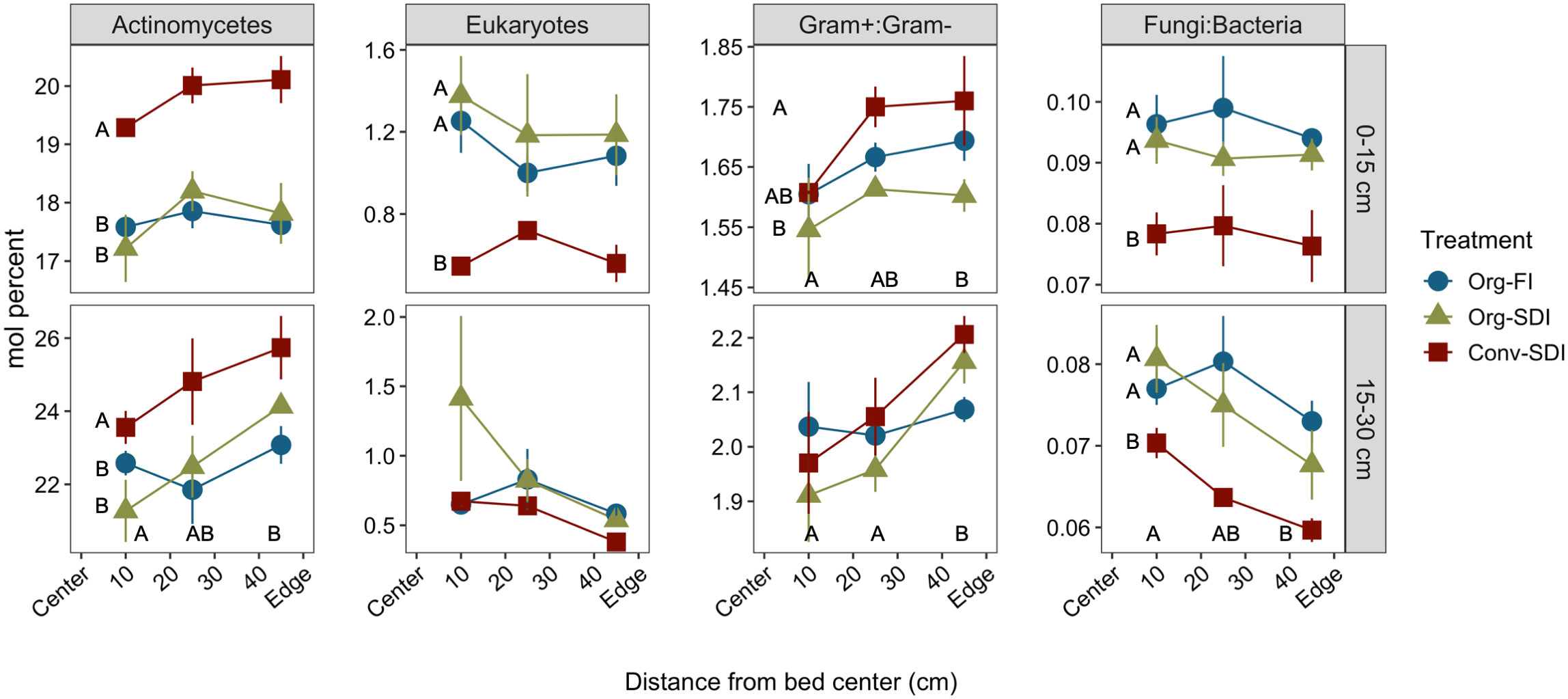
Figure 5 Mean ± SE of relative abundance and ratios of indicator PLFA lipids in soil from 0-15 cm (top row) and 15-30 cm (bottom row) collected in August, immediately before tomato harvest. Lower case letters indicate significant differences between treatments (Tukey HSD, p < 0.05) at a particular distance when treatment*distance interactions were significant, and capital letters indicate significant differences between main effects of treatment or distance (Tukey HSD, p < 0.05) when the interaction was non-significant.
3.4 16S rRNA gene sequencing
3.4.1 Microbial community composition
16S rRNA gene sequencing showed that community composition shifted over the growing season due to irrigation treatments. In May, microbial community composition at the center (Supplementary Figure S4) and edge of the bed (Figure 6) were similar between all treatments at the soil surface. By August, however, microbial community composition at the bed edge had diverged between the two treatments (Figure 6) with lower relative abundance of Actinobacteria in soil at the surface and edge of Org-FI plots and higher relative abundance of Acidobacteria and Gemmatimonadetes with respect to both SDI treatments (Figures 6, 7).
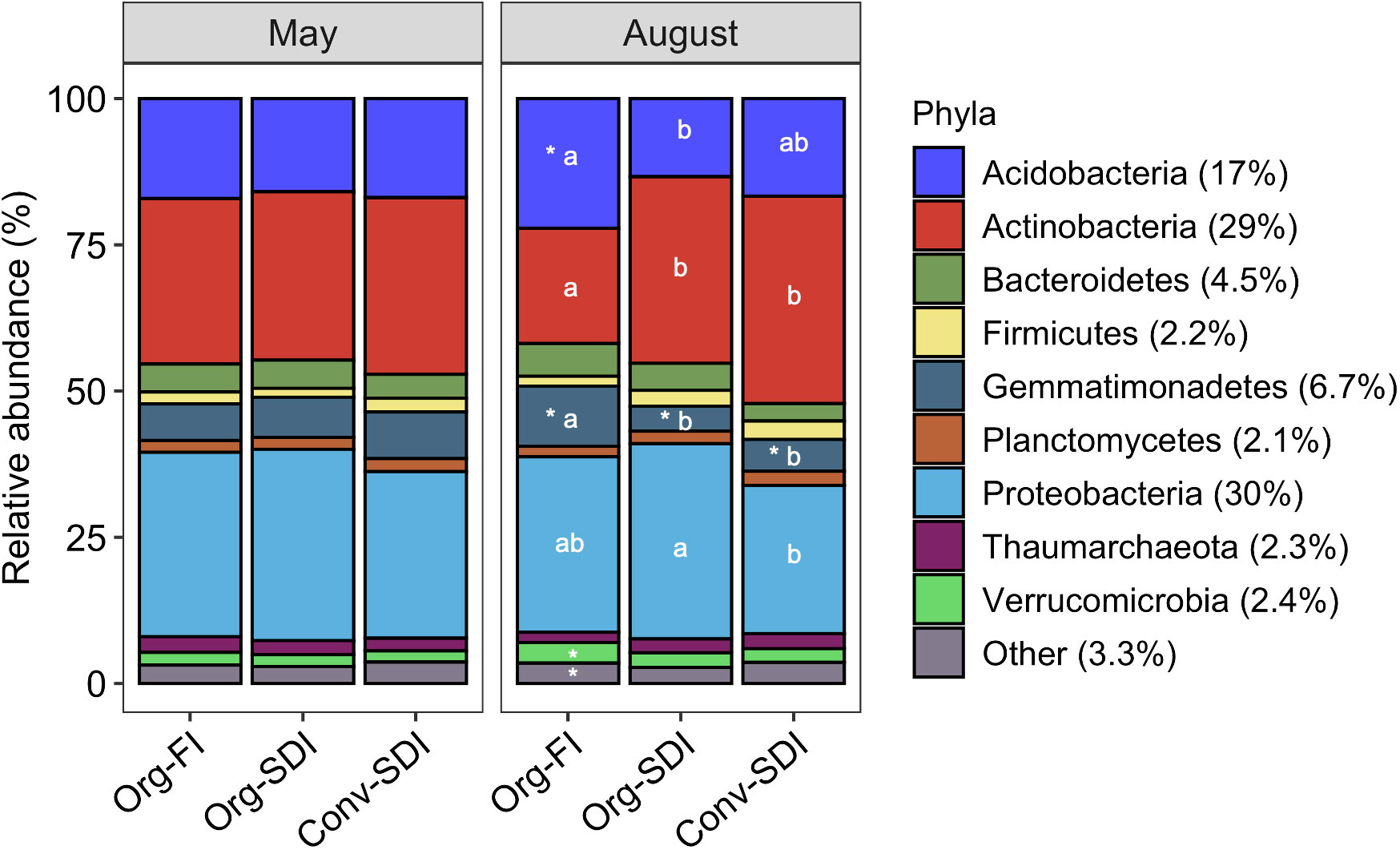
Figure 6 Relative abundance of major phyla at the surface (0-15 cm) and bed edge (45-cm distance) in May and August. “Other” represents the sum of all phyla that represented less than 1% relative abundance. Asterix represent in differences in phylum relative abundance between timepoints within a treatment (p < 0.1), and different lowercase letters indicate differences in phylum relative abundance between treatments within a timepoint. The overall percentages of each phylum are shown in the legend.
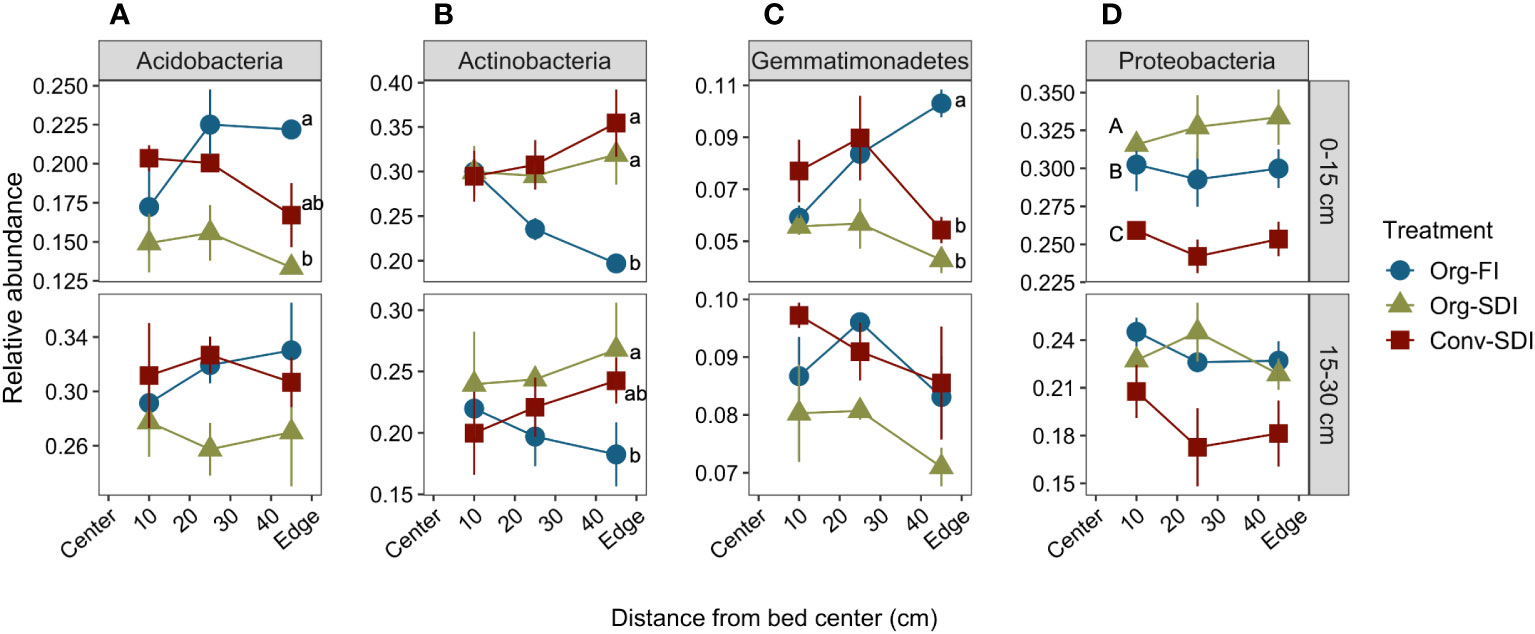
Figure 7 Mean ± SE of relative abundance of major phyla (A) Acidobacteria, (B) Actinobacteria, (C) Gemmatimonadetes, (D) Proteobacteria in soils from 0-15 cm (top row) and 15-30 cm (bottom row) collected in August, immediately before tomato harvest. Lower case letters indicate significant differences between treatments (Tukey HSD, p < 0.05) at a particular distance when treatment*distance interactions were significant, and capital letters indicate significant differences between treatments (Tukey HSD, p < 0.05) across distances when the interaction was non-significant.
In all treatments, larger shifts in relative abundance of phyla at the bed edge led to more spatially heterogeneous microbial community composition throughout the bed by the end of the growing season. While the relative abundance of Actinobacteria was similar among all treatments at the center and surface of the bed, in Org-FI it decreased as a function of distance from center (slope = -0.003, p = 0.0047; Figure 7B). Abundance of Gemmatimonadetes linearly increased with distance from center at the surface in Org-FI (slope = 0.012, p < 0.0001; Figure 7C). Acidobacteria did not show a linear trend, but its relative abundance was higher at the edge than the center for Org-FI surface soils (Figure 7A).
Relative abundance of Proteobacteria showed little spatial variation in any treatment, but across the surface, relative abundance was lower in Org-FI than in Org-SDI (Figure 7D). This phylum also showed the most differentiation between fertility management treatments, with lower relative abundance in Conv than both Org treatments at both depths (p = 0.0180). Despite strong linear moisture gradients (Figure 1), both SDI treatments showed fewer linear relationships of relative abundance with distance compared to FI. In general, relative abundance among treatment replicates was more variable at 15-30 cm than 0-15 cm, resulting in fewer significant trends in subsurface soil.
3.4.2 Spatial changes in alpha diversity
A spatial representation of Shannon diversity shows that in all treatments, drier areas of the bed had higher diversity (Figure 1). Shannon and Chao1 were also higher in the surface than the subsurface. When averaging across depth and distance to account for alpha diversity throughout the bed, Conv-SDI had lower Chao1 richness than Org treatments in both May (pOD = 0.0211, pOF = 0.0251; data not shown) and August (pOD = 0.0155, pOF = 0.0261; Supplementary Figure S5). Org-FI had slightly lower overall Shannon diversity than Org-SDI, but it was not significant at an alpha level of 0.05 (p = 0.0821). However, the difference in Shannon diversity between Org-FI and Org-SDI at the surface and edge of the bed became greater over the growing season (p = 0.0431).
3.4.3 Effects of environmental variables
We assessed effects of soil environmental variables on community composition at the end of the growing season (August), using GWC, DOC, and inorganic N as determined by covariance analysis (VIF) in the CCAs. Regressions of each of these variables against the log2-fold change in absolute abundance of a genus show how microbial diversity and different genera responded to changes in soil moisture (GWC) and labile C (DOC) and N (Inorganic N) in the upper 15 cm. Genera with significant positive or negative relationship with GWC, DOC, or inorganic N are plotted in Figure 8. Both Shannon diversity and Chao1 richness indices were negatively correlated with increasing GWC (p = 0.0003 and p = 0.0062, respectively), but positively correlated with DOC (Shannon: p < 0.0001, Chao: p < 0.0001) and total inorganic N (Shannon: p = 0.0005, Chao: p = 0.0034).
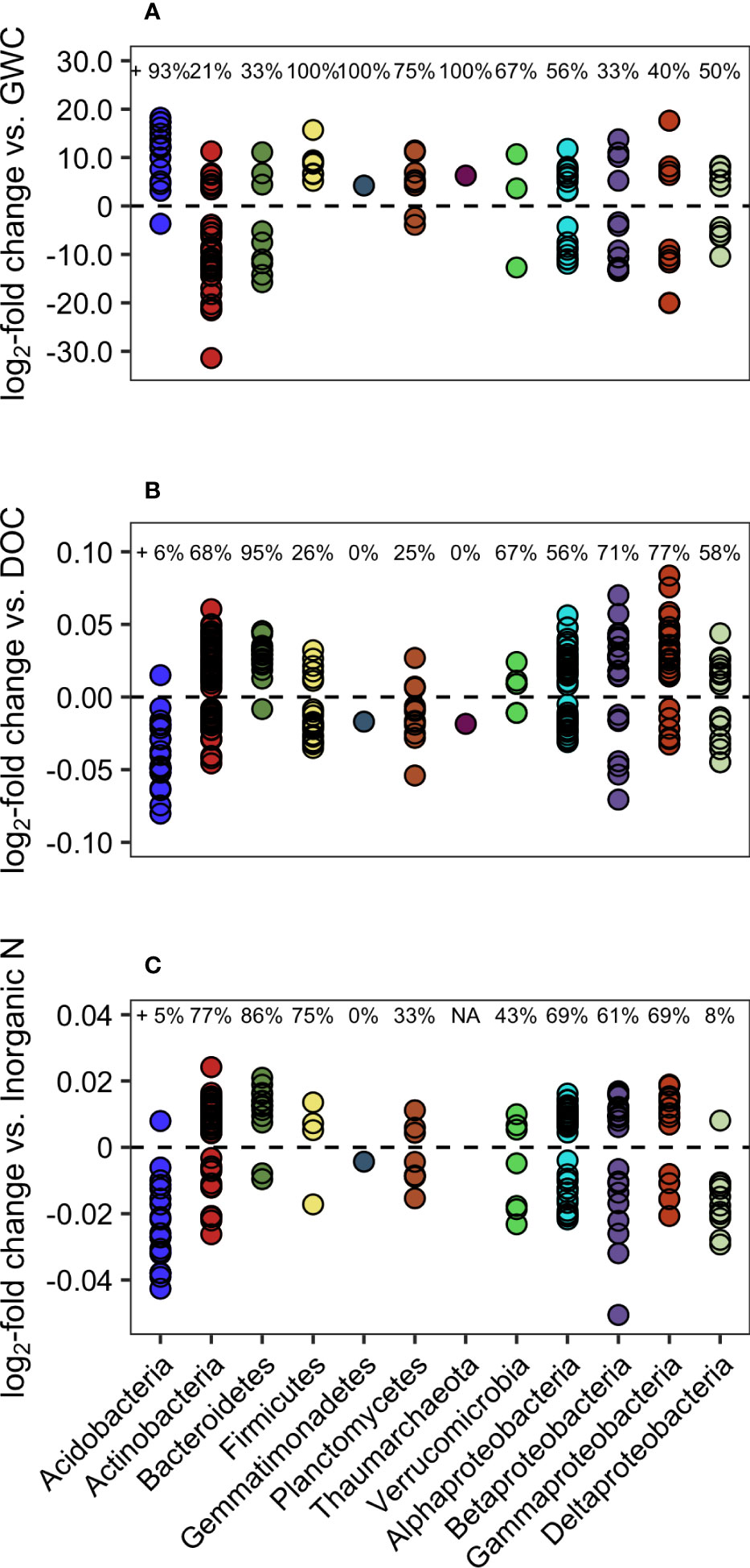
Figure 8 Log2-fold change in normalized abundance of genera vs. (A) GWC, (B) DOC, (C) Inorganic N. Genera have been grouped by phylum or class (for Proteobacteria) and only genera with significant positive or negative regressions are shown (Benjamini-Hochberg false discovery rate adjusted p-value < 0.05). Percentages at the top of each graph represent the percentage within each grouping that had a positive relationship with the environmental variable.
Overall, GWC significantly explained variation in abundance of 146 of the 503 identified genera. Actinobacteria accounted for 29% of those affected, and the majority (79%) of that phyla had a negative relationship with GWC (Figure 8A). Alternatively, of the 15 genera of Acidobacteria affected by soil moisture, 14 increased in abundance with increasing GWC (the exception was the genus Gp10). Proteobacteria (50 genera affected) were split evenly between positive (23) and negative effects (27), and a closer look at specific classes showed no patterns among Alpha-, Beta-, Gamma-, and Deltaproteobacteria. Genera of phyla Firmicutes (7), Gemmatimonadetes (1), Parcubacteria (1), and Thaumarchaeota (1) all increased with higher GWC.
Changes in DOC concentrations significantly impacted abundance of 328 genera (Figure 8B), while inorganic N concentration affected 222 (Figure 8C). In both cases, Acidobacteria tended to be negatively affected by increasing concentrations (DOC: 20 out of 21 genera; N: 18 out of 19 genera), but in both cases the exception was the genus Aridibacter. Other phyla also had similar responses to both DOC and inorganic N concentrations. Several phyla tended to be enriched with greater C and N resources, including Actinobacteria (DOC: 77%, N: 68%), Proteobacteria (DOC: 60%, N: 64%), and Bacteroidetes (DOC: 86%, N: 95%), while Gemmatimonadetes genus Gemmatimonas decreased with increasing C and N concentrations. Breakdown of Proteobacteria by class did not elucidate patterns, except in the case of Deltaproteobacteria’s response to inorganic N, in which 11 of 12 affected genera decreased in abundance, while other classes tended to increase.
4 Discussion
With conversion to SDI from traditional FI in this Mediterranean agroecosystem, the magnitude and frequency of wet-dry cycles is intensified. Soil microbial communities in our study showed similar responses as previously observed in unmanaged systems in terms of reduced microbial biomass, accumulation of labile resources, and shifting community composition towards more drought-resistant phyla.
4.1 Response of microbial biomass to changes in moisture and resource availability
As we hypothesized, extended dry periods in areas of the SDI beds led to reduced microbial biomass compared to moderate wet-dry cycles occurring in FI beds, even in an organic system where C and N resources were sufficient. Lower MBC:DOC and MBC:inorganic N ratios in Org-SDI at the bed edge suggest that resources are available but there is reduced uptake by microbes in this system, potentially due to desiccation stress response (13, 49) and less access to resources from diffusion limitation as water films connecting soil pores dry out (21). Schimel (2018) emphasized the importance of soil water not only as a resource for microbes but also as a solvent and transport medium and concluded that substrate limitation from reduced diffusivity often has a larger impact than direct physiological stress on microbial community composition and biogeochemical cycling.
Accumulation of DOC and inorganic N in continuously dry soils has been reported previously in other systems and climatic zones (e.g., 12, 15, 50, 51) and attributed to be a consequence of the “decoupling” of C and N cycles (52). Uptake of C and N in microbial biomass links the two elements and promotes N retention in soils. Disruption of microbial activity can cause labile C and N to accumulate in forms more vulnerable to loss (13, 53). Additionally, concentration of roots in areas with higher soil moisture in the SDI system (54) also likely reduced plant uptake. Extended dry conditions in SDI beds accumulated DOC and inorganic N at a place and time asynchronous with plant N uptake (i.e., near harvest), which could lead to a large flush of C mineralization activity and N loss through leaching or denitrification with the first winter rain.
With higher labile C and N concentrations, the magnitude of potential C and N losses may be greater in Org-SDI than Conv-SDI, which in August had 50% lower DOC than either Org system and lower concentrations of inorganic N than Org-SDI, particularly in surface edge soils and in the subsurface. Therefore, in accordance with our hypothesis, adoption of SDI is likely to have a greater effect on C and N cycling dynamics in organic systems than in lower C-input conventional systems, such as this one, where these cycles have already been largely decoupled.
4.2 Changes in microbial community composition
4.2.1 Effects of irrigation management
Impacts of irrigation management on microbial community composition appeared only a few months after conversion. By the end of the growing season, communities in FI and SDI had diverged, particularly at the bed edge surface where MBC was also affected. Higher soil moisture in this area of the FI beds favored Gram-negative phyla Acidobacteria and Gemmatimonadetes, whereas relative abundance of Gram-positive Actinobacteria decreased in Org-FI bed edges, with evidence that higher water content negatively affected their proliferation.The contrasting response of Acidobacteria and Actinobacteria to available moisture, C, and N was the primary driver of shifts in community composition between SDI and FI in surface soils at the bed edge. The inverse linkage of these two phyla found in this and other studies may support their use as indicators of biologically relevant changes to the soil environment. Acidobacteria have been reported to be opportunistic in response to short-term changes in soil moisture in Mediterranean grasslands (21, 55), but their abundance can decline with increased aridity (21, 56). In a grassland system, Acidobacteria reduced ribosomal synthesis during desiccation to conserve energy but quickly resumed transcription when conditions became wet (21). Alternatively, Actinobacteria increase in relative abundance during dry-down, ramping up transcription and ribosomal synthesis to survive periods of drought, but they can be outcompeted upon rewetting. Our work supports the hypothesis of Barnard et al. (2014) that Actinobacteria and Acidobacteria exhibit contrasting life strategies of preparedness and responsiveness in response to drying and rewetting, and we show that this response is conserved in highly disturbed agricultural soils with varying concentrations and ratios of C and N pools.
We found the majority of Actinobacterial genera in our agroecosystem were correlated with both DOC (68%) and inorganic N (77%) whereas almost all Acidobacterial genera showed the opposite trend. Studies in unmanaged systems on the response of these phyla to available C (57) and nutrients (58) have found Actinobacteria to be copiotrophic (quick to respond to available resources) and Acidobacteria oligotrophic (slower growing but more competitive with low resource availability). These responses to nutrients contrast with their responses to soil moisture, but the accumulation of DOC and inorganic N observed under low soil moisture could explain this discrepancy. The fact that changes in phyla relative abundance were similar between Org-SDI and Conv-SDI highlights that soil moisture may be the primary control on these phyla, with indirect effects of moisture on C and nutrient uptake playing a secondary role, particularly in agroecosystems where nutrients and (in some cases) C are less limiting.
Relative abundance of actinomycetes and the ratio of Gram-positive (such as Actinobacteria) to Gram-negative bacteria (such as Acidobacteria) from PLFA data followed similar trends to 16S results in the subsurface, but not the surface layer. From 15-30 cm, actinomycete lipid relative abundance and Gram+: Gram- increased moving toward the bed edge in SDI treatments but remained constant across Org-FI beds. In the surface, however, Gram+:Gram- lipids increased toward the bed edge in all treatments. Because DNA persists longer than phospholipids after the death of cells (59, 60), PLFA is likely more representative of organisms who were actually active at the sampling time; this may explain the discrepancy between these methods. Samples were collected near harvest when irrigation had not occurred in any treatment for at least 7 days, so lipid signatures of organisms that respond quickly to moisture shifts may show less treatment differentiation at this timepoint, whereas the DNA-based community analysis reflects a record of changes in community composition occurring throughout the growing season. Use of multiple methodological approaches can more fully detect microbial community responses, particularly to drying and rewetting (61).
Though only one genus from Gemmatimonadetes was detected in our study, this taxon (Gemmatimonas) was particularly sensitive to irrigation treatment, increasing in FI at the bed edge. In a biogeographical analysis, Gemmatimonadetes were prevalent in semiarid, arid, and desert environments, and relative abundance was negatively correlated with moisture content but not affected by soil nutrient content or pH (62). Additional studies found relative abundance of Gemmatimonadetes was lowest in soils exposed to wet-dry cycles and highest in air-dry controls (63) and that organisms from the family Gemmatimonadaceae increased in relative abundance with rewetting after drying, but the response was variable at the phylum level (64). Thus, certain taxa in this phylum may be able to endure the air-dry conditions occurring between irrigation events and be readily proliferate when water is reintroduced. In another study across three climatic zones, members of Gemmatimonadetes were found primarily within soil microaggregates, which was attributed to stable moisture status and slower turnover rates of organic C and organisms (65). While we did not measure aggregate stability on edge soils, measurements at the 25 cm distance showed slightly better aggregation of soils in Org-FI (Supplementary Figure S6). Therefore, it may be that this system provides more habitat for this phylum.
4.2.2 Effects of fertility management systems
Though irrigation management was the main driver of spatial trends in soil properties and microbial communities, the magnitude of measured parameters was driven by the long-term Conv and Org management histories. Conv and Org differed primarily in their relative abundance of Proteobacteria and PLFAs associated with actinomycetes. These differences between Org and Conv treatments were driven in large part by the soil DOC concentrations, which were ~2 times higher in Org plots, and by inorganic N at bed center at the beginning of the season. Proteobacterial abundance correlated with increasing DOC and inorganic N (based on log2-fold change), and genera within Beta- and Gammaproteobacteria in particular had largely positive relationships with these properties. While our findings are correlative, similar relationships were observed in nutrient manipulation studies across grasslands globally (58) and in humid grassland and forest soils where direct addition of low molecular weight C substrates in microcosms increased Beta- and Gammaproteobacteria, as well as Actinobacteria (66). In our study, PLFA biomarkers associated with actinomycetes were lower in abundance in Org than Conv plots. This discrepancy may be due to one of the challenges of interpreting relative abundance values, as the relative abundance of actinomycetes lipids in Conv may be inflated from relatively low abundances of other groups.
4.3 Bacterial diversity across tomato beds
Higher taxonomic diversity was associated with lower water content, which could suggest greater functional resilience despite changes in composition due to functional redundancy of diverse communities (7); however, it may also indicate that organisms that dominate in higher moisture conditions are poorly adapted to desiccation stress. Dry conditions are often selective for organisms that can withstand desiccation, which can reduce microbial diversity (21), and global biogeographical community analyses have found low bacterial diversity and abundance in arid soils (56), in contrast to our results. Field scale short-term effects of soil water content on microbial communities in high nutrient and disturbed environments are likely different from longer term effects of climatic aridity or mean annual soil moisture deficit at a global scale (e.g., 59, 67–69). The uniqueness of agricultural soils along with selection of a few microbial groups that outcompete less opportunistic taxa when moisture, and perhaps dissolved C and N, are more accessible could drive some of these differences.
Similarly, it is important to consider prior history, and potential adaptation, of the soil biota to the frequency and intensity of moisture perturbations. At the edge of the Org-FI beds, where moderate, frequent wet-dry perturbations are occurring, diversity remained stable. Fierer et al. (2003) similarly found that wet-dry cycling had little effect on microbial diversity and evenness in incubated grassland soils that typically experienced large moisture changes, but that wet-dry cycling increased microbial diversity in soil from under oak canopies, where moisture fluctuations are typically less extreme. Mediterranean climates have drastic seasonal variation in precipitation, and organisms in unmanaged soils may be adapted to prolonged dry periods (summer) followed by sudden influx of rain (winter). However, in traditionally flood-irrigated California agroecosystems, biota may be adapted to more frequent, less extreme, year-round moisture fluctuations. SDI introduces another manipulation of water availability that over time will likely cause new adaptations in microbial communities. The increase in alpha diversity seen in the driest areas of the beds in our experiment may be a response of less-adapted communities, as Fierer et al. (2003) saw in oak soils, where drought-tolerant organisms that might be selected against in moist soils are more abundant. Our study took place the season after SDI was established in these beds, and it will be important to evaluate whether soil biota in SDI beds for multiple years have adapted to dry conditions, or whether winter rains “reset” the communities.
4.4 Implications for agroecosystem functions and productivity
Impacts of irrigation management on microbial communities can drive significant shifts in soil ecological processes underlying sustainability and resilience such as N losses, water cycling, and productivity. The decoupling of C and N pools in continuously dry surface soils of the Org-SDI system, likely from diffusion limitations that limit microbial uptake, leaves labile forms more vulnerable to loss during the first winter rain events in this Mediterranean climate. This accumulation of resources, particularly inorganic N, is the reason more extreme precipitation cycles driven by climate change are projected to exacerbate N losses through leaching and denitrification in unmanaged and rainfed agricultural systems across climatic zones (53). Rewetting of SDI surface soils with winter rain after a dry summer may show similar microbial effects, and thus may have similar N loss consequences as extreme wet-dry cycling in non-irrigated systems (12).
Moisture regulation of microbial activity could also impact soil physical structure regulating water cycling. While the organic systems had greater microbial biomass and diversity than the conventional, stresses such as extended soil desiccation can reduce functioning of even diverse microbial communities. Gram-positive and spore-forming bacteria may become more abundant (23) but may still shut down metabolism with desiccation or be limited by substrate diffusion and accessibility (11). In a field trial at the same location as ours, Schmidt et al. (2018) found lower microbial activity (fluorescein diacetate [FDA] hydrolysis) at the edge of SDI plots and reduced water-stable aggregates in SDI surface soils compared to FI, results that are supported by aggregate measurements taken in our study (Supplementary Figure S6). Therefore, future studies should explore whether reduced microbial biomass and activity from extended dry periods may reduce the resilience of soils under SDI to infiltrate water from winter rain events.
Finally, tomato fruit yields in Org-SDI were 14% and 16% lower on average than in Org-FI and Conv-SDI, respectively (Supplementary Figure S7; 54), suggesting there were negative effects on important soil functions that feed back into supporting crop yields. Vine biomass was highest in the Org-SDI plots indicating that, though we did not capture treatment differences in soil inorganic N values, the timing of microbial N cycling is altered in Org-SDI such that nutrients are not plant-available at the optimal time for fruit production. This reduction in yield could have substantial economic implications for organic growers investing in SDI infrastructure and organic amendments.
5 Conclusion
Our investigation into the spatial and temporal effects of irrigation management in row crops provides an agricultural context in which to test mechanisms described in an extensive literature on soil wet-dry cycling and drought focused primarily on less managed ecosystems. Irrigation type—i.e., furrow versus subsurface drip—had major effects on soil microbial biomass and community composition, with SDI causing responses similar to those in extended dry-down events in non-irrigated systems, particularly in the organic system. As seen in non-agricultural systems, the severity and frequency of moisture changes, adaptation of the communities, and resource availability affect microbial response. Decoupling of C and N pools in dry surface soils under SDI may increase the potential for losses of DOC and nitrate with the first winter rains in this Mediterranean climate, an important aspect to quantify in future studies. Given this was the first year that drip irrigation was implemented in this field, microbial communities are likely to adapt to these conditions over the years. Future studies should assess whether divergence of microbial communities between irrigation systems is long-term or reset by winter rains.
We know many of the attributes of healthy soils—resilient aggregate structure, organic matter storage, and tightly coupled nutrient cycles—are mediated by soil microbial communities. To effectively manage cropping systems for both high productivity and resilience, we must consider trade-offs of specific practices, like drip irrigation, that may be detrimental to soil microbial community functions.
Data availability statement
The raw data supporting the conclusions of this article will be made available by the authors, without undue reservation.
Author contributions
DGL: Conceptualization, Formal analysis, Investigation, Methodology, Visualization, Writing – original draft. DW: Conceptualization, Investigation, Writing – review & editing. AG: Conceptualization, Writing – review & editing. BDJ: Formal Analysis, Writing – review & editing. MS: Formal analysis, Writing – review & editing. KS: Conceptualization, Funding acquisition, Methodology, Resources, Supervision, Writing – review & editing.
Funding
The author(s) declare financial support was received for the research, authorship, and/or publication of this article. This work was funded by the California Tomato Research Institute. Additionally, DGL was supported by a National Science Foundation (NSF) Graduate Research Fellowship (NSF DGE-1148897). Additionally, funding was provided by the USDA/NIFA through Hatch project 1014527 and by the College of Agricultural and Environmental Sciences Dean’s Office and the Agricultural Sustainability Institute at UC Davis.
Acknowledgments
We are grateful to Israel Herrera, Luis Loza, and the staff at the Russell Ranch Sustainable Agriculture Facility for their oversight of the Century Experiment and assistance for this trial. We also thank Dinh Giang, Evelyn Peña, Evan Dumas, and Thanh Tu Le for assistance with field sampling and lab analyses, and Tad Doane, Daniel Geisseler, Jorge Rodrigues, Nikhil Joshi, and Jordan Sayre for guidance on analysis protocols. Finally, we thank our funding sources for supporting this research.
Conflict of interest
The authors declare that the research was conducted in the absence of any commercial or financial relationships that could be construed as a potential conflict of interest.
The author(s) AG and DW declared that they were editorial board members of Frontiers, at the time of submission. This had no impact on the peer review process and the final decision.
Publisher’s note
All claims expressed in this article are solely those of the authors and do not necessarily represent those of their affiliated organizations, or those of the publisher, the editors and the reviewers. Any product that may be evaluated in this article, or claim that may be made by its manufacturer, is not guaranteed or endorsed by the publisher.
Supplementary material
The Supplementary Material for this article can be found online at: https://www.frontiersin.org/articles/10.3389/fsoil.2023.1267685/full#supplementary-material
References
1. Schmidt MWI, Torn MS, Abiven S, Dittmar T, Guggenberger G, Janssens IA, et al. Persistence of soil organic matter as an ecosystem property. Nature (2011) 478(7367):49–56. doi: 10.1038/nature10386
2. Lehmann J, Kleber M. The contentious nature of soil organic matter. Nature (2015) 528:60–8. doi: 10.1038/nature16069
3. Trivedi P, Wallenstein MD, Delgado-Baquerizo M. Chapter 3 – Microbial modulators and mechanisms of soil carbon storage. Soil Carbon Storage (2018) 73–115. doi: 10.1016/B978-0-12-812766-7.00003-2
4. Kästner M, Miltner A. Chapter 5 – SOM and microbes-what is left from microbial life. In: The future of soil carbon: its conservation and formation (2018). p. 125–63.
5. Kallenbach CM, Frey SD, Grandy AS. Direct evidence for microbial-derived soil organic matter formation and its ecophysiological controls. Nat Commun (2016) 7:1–10. doi: 10.1038/ncomms13630
6. Blankinship JC, Fonte SJ, Six J, Schimel JP. Plant versus microbial controls on soil aggregate stability in a seasonally dry ecosystem. Geoderma [Internet] (2016) 272:39–50. doi: 10.1016/j.geoderma.2016.03.008
7. Kibblewhite MG, Ritz K, Swift MJ. Soil health in agricultural systems. Philos Trans R Soc Lond B Biol Sci (2008) 363:685–701. doi: 10.1098/rstb.2007.2178
8. Schimel JP, Balser TC, Wallenstein MD. Microbial stress-response physiology and its implications for ecosystem function. Ecology (2007) 88(6):1386–94. doi: 10.1890/06-0219
9. Evans SE, Wallenstein MD, Burke IC. Is bacterial moisture niche a good predictor of shifts in community composition under long-term drought. Ecology (2014) 95(1):110–22. doi: 10.1890/13-0500.1
10. Schumacher DL, Keune J, Dirmeyer P, Miralles DG. Drought self-propagation in drylands due to land–atmosphere feedbacks. Nat Geosci (2022) 15(4):262–8. doi: 10.1038/s41561-022-00912-7
11. Schimel JP. Life in dry soils: effects of drought on soil microbial communities and processes. Annu Rev Ecol Evol Syst [Internet] (2018) 49:409–32. doi: 10.1146/annurev-ecolsys-110617-%0Ahttps://doi.org/10.1146/annurev-ecolsys-110617-062527
12. Barnard RL, Blazewicz SJ, Firestone MK. Rewetting of soil: Revisiting the origin of soil CO2 emissions. Soil Biol Biochem (2020) 147:107819. doi: 10.1016/j.soilbio.2020.107819
13. Malik AA, Bouskill NJ. Drought impacts on microbial trait distribution and feedback to soil carbon cycling. Funct Ecol (2022), 36:1442–56. doi: 10.1111/1365-2435.14010
14. Van Gestel M, Merckx R, Vlassak K. Microbial biomass responses to soil drying and rewetting: The fate of fast- and slow-growing microorganisms in soils from different climates. Soil Biol Biochem (1993) 25(1):109–23. doi: 10.1016/0038-0717(93)90249-B
15. Xiang SR, Doyle A, Holden PA, Schimel JP. Drying and rewetting effects on C and N mineralization and microbial activity in surface and subsurface California grassland soils. Soil Biol Biochem (2008) 40(9):2281–9. doi: 10.1016/j.soilbio.2008.05.004
16. Denef K, Six J, Bossuyt H, Frey SD, Elliott ET, Merckx R, et al. Influence of dry-wet cycles on the interrelationship between aggregate, particulate organic matter, and microbial community dynamics. Soil Biol Biochem (2001) 33:1599–611. doi: 10.1016/S0038-0717(01)00076-1
17. Fierer N, Schimel JP. Effects of drying-rewetting frequency on soil carbon and nitrogen transformations. Soil Biol Biochem (2002) 34(6):777–87. doi: 10.1016/S0038-0717(02)00007-X
18. Sawada K, Funakawa S, Kosaki T. Effect of repeated drying–rewetting cycles on microbial biomass carbon in soils with different climatic histories. Appl Soil Ecol (2017) 120:1–7. doi: 10.1016/j.apsoil.2017.07.023
19. Gordon H, Haygarth PM, Bardgett RD. Drying and rewetting effects on soil microbial community composition and nutrient leaching. Soil Biol Biochem (2008) 40(2):302–11. doi: 10.1016/j.soilbio.2007.08.008
20. Placella SA, Brodie EL, Firestone MK. Rainfall-induced carbon dioxide pulses result from sequential resuscitation of phylogenetically clustered microbial groups. Proc Natl Acad Sci USA (2012) 109(27):10931–6. doi: 10.1073/pnas.1204306109
21. Barnard RL, Osborne CA, Firestone MK. Responses of soil bacterial and fungal communities to extreme desiccation and rewetting. Isme J [Internet] (2013) 7(11):2229–41. doi: 10.1038/ismej.2013.104
22. Evans SE, Wallenstein MD. Climate change alters ecological strategies of soil bacteria. Ecol Lett (2014) 17(2):155–64. doi: 10.1111/ele.12206
23. Naylor D, Coleman-Derr D. Drought stress and root-associated bacterial communities. Front Plant Sci (2018) 8:2223/full. doi: 10.3389/fpls.2017.02223/full
24. Ayars JE, Fulton A, Taylor B. Subsurface drip irrigation in California-Here to stay? Agric Water Manag [Internet] (2015) 157:39–47. doi: 10.1016/j.agwat.2015.01.001
25. Taylor R, Zilberman D. Diffusion of drip irrigation: The case of California. Appl Econ Perspect Policy (2017) 39(1):16–40. doi: 10.1093/aepp/ppw026
26. Hanson B, May D. Effect of subsurface drip irrigation on processing tomato yield, water table depth, soil salinity, and profitability. Agric Water Manage (2004) 68(1):1–17. doi: 10.1016/j.agwat.2004.03.003
27. Hanson BR, May DE, Hopmans JW, Robert B. Drip irrigation provides the salinity control needed for profitable irrigation of tomatoes in the San Joaquin Valley. Calif Agric (2009), 131–6. doi: 10.3733/ca.v063n03p131
28. Schmidt JE, Peterson C, Wang D, Scow KM, Gaudin ACM. Agroecosystem tradeoffs associated with conversion to subsurface drip irrigation in organic systems. Agric Water Manag (2018) 202:1–8. doi: 10.1016/j.agwat.2018.02.005
29. Wolf KM, Herrera I, Tomich TP, Scow KM. Long-term agricultural experiments inform the development of climate-smart agricultural practices. Calif Agric (2017) 71(3):120–4. doi: 10.3733/ca.2017a0022
30. Wolf KM, Torbert EE, Bryant D, Burger M, Denison RF, Herrera I, et al. The century experiment: the first twenty years of UC Davis’ Mediterranean agroecological experiment. Ecology (2018) 99(2):503. doi: 10.1002/ecy.2105
31. CIMIS. California irrigation management information system (2018). Available at: http://www.cimis.water.ca.gov (Accessed 2018 Nov 14).
32. Verdouw H, Van Echteld CJA, Dekkers EMJ. Ammonia determination based on indophenol formation with sodium salicylate. Water Res (1978) 12(6):399–402. doi: 10.1016/0043-1354(78)90107-0
33. Doane TA, Horwath WR. Spectrophotometric determination of nitrate with a single reagent. Anal Lett (2003) 36(12):2713–22. doi: 10.1081/AL-120024647
34. Vance E, Brookes P, Jenkinson D. An extraction method for measuring soil microbial biomass C. Soil Biol Biochem (1987) 19:703–7. doi: 10.1016/0038-0717(87)90052-6
35. Wu J, Joergensen RG, Pommerening B, Chaussod R, Brookes PC. Measurement of soil microbial biomass by fumigation-extraction - An automated procedure. Soil Biol Biochem (1990) 22(8):1167–9. doi: 10.1016/0038-0717(90)90046-3
36. Buyer JS, Sasser M. High throughput phospholipid fatty acid analysis of soils(2012) (Accessed 2015 Jan 9).
37. Caporaso JG, Lauber CL, Walters WA, Berg-Lyons D, Huntley J, Fierer N, et al. Ultra-high-throughput microbial community analysis on the Illumina HiSeq and MiSeq platforms. ISME J [Internet] (2012) 6(8):1621–4. doi: 10.1038/ismej.2012.8
38. Magoč T, Salzberg S. FLASH: fast length adjustment of short reads to improve genome assemblies. Bioinformatics (2011) 27:2957–63. doi: 10.1093/bioinformatics/btr507
39. Wang Q, Garrity G, Tjedie J, Cole J. Naïve Bayesian classifier for rapid assignment of rRNA sequences into the new bacterial taxonomy. Appl Environ Microbiol (2007) 73:5261–7. doi: 10.1128/AEM.00062-07
40. R Core Team. R: A language and environment for statistical computing. R foundation for statistical computing. R foundation for statistical computing. In: R foundation for statistical computing. Vienna, Austria (2018). Available at: http://www.r-project.org/.
41. Bates D, Maechler M, Bolker B, Walker S. Fitting linear mixed-effects models using lme4. J Stat Software (2015) 67(1):1–48. doi: 10.18637/jss.v067.i01
42. de Mendiburu F. agricolae: Statistical Procedures for Agricultural Research. (2023). R package version 1. 3–6. Available at: https://CRAN.R-project.org/package=agricolae.
44. Oksanen J, Simpson G, Blanchet F, Kindt R, Legendre P, Minchin P, et al. vegan: Community Ecology Package. (2022). R package version 2. 6–4. Available at: https://CRAN.R-project.org/package=vegan.
45. Law CW, Chen Y, Shi W, Smyth GK. voom: Precision weights unlock linear model analysis tools for RNA-seq read counts. Genome Biol (2014) 15(2):R29. doi: 10.1186/gb-2014-15-2-r29
46. Smyth GK. Linear models and empirical bayes methods for assessing differential expression in microarray experiments. Stat Appl Genet Mol Biol (2004) 3(1):1–25. doi: 10.2202/1544-6115.1027
47. McMurdie PJ, Holmes S. phyloseq: an R package for reproducible interactive analysis and graphics of microbiome census data. PloS One (2013) 8:e61217. doi: 10.1371/journal.pone.0061217
48. Pinheiro J, Bates D, DebRoy S, Sarkar D. nlme: Linear and nonlinear mixed effects models. R package version 3. Available at: https://cran.r-project.org/package=nlme.
49. Manzoni S, Schimel JP, Porporato A. Responses of soil microbial communities to water stress: Results from a meta-analysis. Ecology (2012) 93(4):930–8. doi: 10.1890/11-0026.1
50. Whitford WG, Martinez-Turanzas G, Martinez- Meza E. Persistence of desertified ecosystems: Explanations and implications. Environ Monit Assess (1995) 37:319–32. doi: 10.1007/BF00546898
51. Lundquist EJ, Jackson LE, Scow KM. Wet-dry cycles affect dissolved organic carbon in two California agricultural soils. Soil Biol Biochem (1999) 31:1031–8. doi: 10.1016/S0038-0717(99)00017-6
52. Evans SE, Burke IC. Carbon and nitrogen decoupling under an 11-year drought in the shortgrass steppe. Ecosystems (2012) 16(1):20–33. doi: 10.1007/s10021-012-9593-4
53. Bowles TM, Atallah SS, Campbell EE, Gaudin ACM, Wieder WR, Grandy AS. Addressing agricultural nitrogen losses in a changing climate. Nat Sustain (2018) 1:399–408. doi: 10.1038/s41893-018-0106-0
54. Li M, Schmidt JE, LaHue DG, Lazicki P, Kent A, Machmuller MB, et al. Impact of irrigation strategies on tomato root distribution and rhizosphere processes in an organic system. Front Plant Sci (2020), 11:1–12. doi: 10.3389/fpls.2020.00360
55. Barnard RL, Osborne CA, Firestone MK. Changing precipitation pattern alters soil microbial community response to wet-up under a Mediterranean-type climate, The ISME Journal (2014) 9(4):946–57. doi: 10.1038/ismej.2014.192
56. Maestre FT, Delgado-Baquerizo M, Jeffries TC, Eldridge DJ, Ochoa V, Gozalo B, et al. Increasing aridity reduces soil microbial diversity and abundance in global drylands. Proc Natl Acad Sci [Internet] (2015) 112(51):201516684. doi: 10.1073/pnas.1516684112
57. Fierer N, Bradford MA, Jackson RB. Toward an ecological classification of soil bacteria. Ecology (2007) 88(6):1354–64. doi: 10.1890/05-1839
58. Leff JW, Jones SE, Prober SM, Barberán A, Borer ET, Firn JL, et al. Consistent responses of soil microbial communities to elevated nutrient inputs in grasslands across the globe. Proc Natl Acad Sci [Internet] (2015) 112(35):10967–72. doi: 10.1073/pnas.1508382112
59. Watzinger A. Microbial phospholipid biomarkers and stable isotope methods help reveal soil functions. Soil Biol Biochem (2015) 86:98–107. doi: 10.1016/j.soilbio.2015.03.019
60. Zhang Y, Zheng N, Wang J, Yao H, Qiu Q, Chapman SJ. High turnover rate of free phospholipids in soil confirms the classic hypothesis of PLFA methodology. Soil Biol Biochem (2019) 135:323–330. doi: 10.1016/j.soilbio.2019.05.023
61. Osburn ED, McBride SG, Kupper JV, Nelson JA, McNear DH, McCulley RL, et al. Accurate detection of soil microbial community responses to environmental change requires the use of multiple methods. Soil Biol Biochem (2022) 169:108685. doi: 10.1016/j.soilbio.2022.108685
62. DeBruyn JM, Nixon LT, Fawaz MN, Johnson AM, Radosevich M. Global biogeography and quantitative seasonal dynamics of Gemmatimonadetes in soil. Appl Environ Microbiol (2011) 77(17):6295–300. doi: 10.1128/AEM.05005-11
63. Fawaz M. Revealing the ecological role of gemmatimonadetes through cultivation and molecular analysis of agricultural soils. In: Masters thesis, University of Tennessee (2013). Available at: http://trace.tennessee.edu/utk_gradthes/1652.
64. Aanderud ZT, Lennon JT. Validation of heavy-water stable isotope probing for the characterization of rapidly responding soil bacteria. Appl Environ Microbiol (2011) 77(13):4589–96. doi: 10.1128/AEM.02735-10
65. Mummey D, Holben W, Six J, Stahl P. Spatial stratification of soil bacterial populations in aggregates of diverse soils. Microb Ecol (2006) 51(3):404–11. doi: 10.1007/s00248-006-9020-5
66. Eilers KG, Lauber CL, Knight R, Fierer N. Shifts in bacterial community structure associated with inputs of low molecular weight carbon compounds to soil. Soil Biol Biochem [Internet] (2010) 42(6):896–903. doi: 10.1016/j.soilbio.2010.02.003
67. Jones RT, Robeson MS, Lauber CL, Hamady M, Knight R, Fierer N. A comprehensive survey of soil acidobacterial diversity using pyrosequencing and clone library analyses. ISME J (2009) 3(4):442–53. doi: 10.1038/ismej.2008.127
68. Lauber CL, Hamady M, Knight R, Fierer N. Pyrosequencing-based assessment of soil pH as a predictor of soil bacterial community structure at the continental scale. Appl Environ Microbiol (2009) 75(15):5111–20. doi: 10.1128/AEM.00335-09
Keywords: soil moisture, wet-dry cycles, subsurface drip irrigation, organic and conventional management, C and N cycling, microbial biomass, 16S rRNA gene sequencing, PLFA
Citation: Griffin-LaHue D, Wang D, Gaudin ACM, Durbin-Johnson B, Settles ML and Scow KM (2023) Extended soil surface drying triggered by subsurface drip irrigation decouples carbon and nitrogen cycles and alters microbiome composition. Front. Soil Sci. 3:1267685. doi: 10.3389/fsoil.2023.1267685
Received: 26 July 2023; Accepted: 19 October 2023;
Published: 17 November 2023.
Edited by:
Lucas William Mendes, University of São Paulo, BrazilReviewed by:
Thierry Pellegrinetti, University of São Paulo, BrazilDennis Goss-Souza, Science and Technology of Paraná, Brazil
Diogo Paes da Costa, Federal University of Agreste of Pernambuco (UFAPE), Brazil
Copyright © 2023 Griffin-LaHue, Wang, Gaudin, Durbin-Johnson, Settles and Scow. This is an open-access article distributed under the terms of the Creative Commons Attribution License (CC BY). The use, distribution or reproduction in other forums is permitted, provided the original author(s) and the copyright owner(s) are credited and that the original publication in this journal is cited, in accordance with accepted academic practice. No use, distribution or reproduction is permitted which does not comply with these terms.
*Correspondence: Deirdre Griffin-LaHue, ZC5ncmlmZmluQHdzdS5lZHU=
†Present addresses: Deirdre Griffin-LaHue, Department of Crop and Soil Sciences, Northwestern Washington Research and Extension Center, Washington State University, Mount Vernon, WA, United States
Daoyuan Wang, Department of Environmental Science and Engineering, Shanghai University, Shanghai, China