Dynamic risk prediction models for different subtypes of hypertensive disorders in pregnancy
- 1Faculty of Environment and Life, Beijing University of Technology, Beijing, China
- 2Department of Obstetrics, Peking University People’s Hospital, Beijing, China
Background: Hypertensive disorders in pregnancy (HDP) are diseases that coexist with pregnancy and hypertension. The pathogenesis of this disease is complex, and different physiological and pathological states can develop different subtypes of HDP.
Objective: To investigate the predictive effects of different variable selection and modeling methods on four HDP subtypes: gestational hypertension, early-onset preeclampsia, late-onset preeclampsia, and chronic hypertension complicated with preeclampsia.
Methods: This research was a retrospective study of pregnant women who attended antenatal care and labored at Beijing Maternity Hospital, Beijing Haidian District Maternal and Child Health Hospital, and Peking University People's Hospital. We extracted maternal demographic data and clinical characteristics for risk factor analysis and included gestational week as a parameter in this study. Finally, we developed a dynamic prediction model for HDP subtypes by nonlinear regression, support vector machine, stepwise regression, and Lasso regression methods.
Results: The AUCs of the Lasso regression dynamic prediction model for each subtype were 0.910, 0.962, 0.859, and 0.955, respectively. The AUC of the Lasso regression dynamic prediction model was higher than those of the other three prediction models. The accuracy of the Lasso regression dynamic prediction model was above 85%, and the highest was close to 92%. For the four subgroups, the Lasso regression dynamic prediction model had the best comprehensive performance in clinical application. The placental growth factor was tested significant (P < 0.05) only in the stepwise regression dynamic prediction model for early-onset preeclampsia.
Conclusion: The Lasso regression dynamic prediction model could accurately predict the risk of four HDP subtypes, which provided the appropriate guidance and basis for targeted prevention of adverse outcomes and improved clinical care.
Introduction
Hypertensive disorders in pregnancy (HDP) are diseases that coexist with pregnancy and hypertension, which are major causes of increased maternal morbidity and mortality (1–3). HDP includes gestational hypertension, preeclampsia, eclampsia, chronic hypertension complicated with preeclampsia, and gestational combined chronic hypertension (4, 5). PE can be divided into two subtypes according to the time of onset: early-onset preeclampsia and late-onset preeclampsia (6, 7). HDP can be predicted by relevant risk factors, leading to early treatment (8–11).
The pathogenesis of HDP is complex. Risk factors for HDP are related to clinical epidemiological factors (12, 13), hemodynamic factors (14, 15), basic biochemical factors (16), and biomarkers (17, 18). For vascular biomarkers, numerous studies confirmed that placental growth factor (PlGF) had the function of regulating placental trophoblast and endothelial cells, and had a good predictive value for preeclampsia (19–21). HDP has multiple risk factors, which cannot be accurately predicted by a single factor and requires a combined assessment of multiple risk factors (22, 23). To improve the accuracy of prediction, researchers carried out a variety of combinations of different risk factors. Stepan et al. (24) found that a combination of ultrasound, mean arterial pressure, clinical features, and PlGF improved the prediction of preeclampsia in the first trimester of pregnancy. Chen et al. (25) found that the combination of mean arterial pressure, PlGF, and pregnancy-associated plasma protein A was far superior to a single factor. Current studies on the prediction of HDP focused on static studies at specific gestational weeks (26, 27), while pregnancy is a dynamic process and various physiological factors are constantly changing during pregnancy (28). Therefore, it is necessary to conduct a continuous dynamic study of HDP.
Different HDP subtypes are based on different physiological and pathological conditions of pregnant women, and a single modeling approach is not effective in predicting HDP subtypes. Poon et al. (29) found that the early-onset preeclampsia prediction model had a high detection rate of 93.1% for early-onset preeclampsia, but only 35.7% and 18.3% for late-onset preeclampsia and gestational hypertension. Sun et al. (30) compared the prediction effects of different methods on HDP and found that the Lasso regression method had the best prediction effect.
In this paper, we integrated multiple risk factors and multiple modeling approaches to develop dynamic prediction models for HDP subtypes. The prediction effects of various models were compared to select the optimal prediction model for effective prediction of each subtype.
Materials and methods
Research object
We performed a retrospective study on pregnant women who attended antenatal checkups at Beijing Maternity Hospital from 2006 to 2008, at Beijing Haidian District Maternal and Child Health Hospital from 2015 to 2016, and at Peking University People's Hospital from July 2015 to 2017. Our control group was healthy pregnant women without hypertensive disorders during pregnancy, not taking long-term medication, and without fetal malformations. A total of 1,267 women were included in this study, and they were divided into four HDP subgroups and a normal pregnancy group (Table 1).
Factors included in the analysis
The following data were collected from the maternal electronic medical records of the hospital: (1) the demographic data of pregnant women; (2) the clinical examination index. We classified the collected factors according to whether they changed with pregnancy: (a) static factors; (b) dynamic factors.
Static factors
Static factors were divided into two categories (Table 2): (i) quantitative factors, included age, height, and pre-pregnancy body mass index; (ii) qualitative factors, included first birth, multiple pregnancy, maternal history of disease, maternal family history of disease and maternal complications.
Dynamic factors
Dynamic factors were divided into four categories (Table 3): (i) clinical epidemiologic factors; (ii) hemodynamic factors; (iii) basic biochemical factors; (iiii) biomarkers.
Dynamic prediction model
In this paper, the data were characterized by a large variety of parameters and the data volume was a small sample (in thousands), so we chose nonlinear regression, support vector machine (SVM), stepwise regression and Lasso regression to develop the prediction models. The advantages of these methods were that they were suitable for small samples and had good generalization ability. Among these methods, stepwise regression and Lasso regression had the function of automatic filtering variables.
Dynamic factors changed continuously during pregnancy, so we included the gestational week as a parameter in this research from both the formula and algorithm perspectives: we constructed a custom regression dynamic gestational week fitting formula by using nonlinear regression; we developed dynamic prediction models by using SVM, stepwise regression and Lasso regression. In model training for each subgroup, we selected 15 pregnant women in the subgroup and control group to form the validation set, and the rest pregnant women were divided into training set and test set at a ratio of 7:3.
Statistical analysis
Quantitative factors are presented as X (mean) ± SD (standard deviation). Qualitative factors are presented as percentages (%). Risk factors were screened for each HDP subgroup. For static factors, we conducted independent sample T test for quantitative factors and selected factors with P < 0.05; we performed chi-square test for qualitative factors, and selected factors with OR > 1 and P < 0.05. For dynamic factors, the clinical epidemiological factors and biomarkers for this research were body mass index and PlGF. A large number of researches had confirmed that body mass index and PlGF were risk factors for HDP (31, 32), so we analyzed the hemodynamic and basic biochemical factors. We divided the pregnant woman's gestational weeks into five stages: 0–13, 14–20, 21–28, 29–34, 35–40 weeks. We performed independent sample t-tests for hemodynamic and basic biochemical factors at each stage, and selected factors with P < 0.05 and abnormal value (mean value outside the normal range).
We used IBM SPSS Statistics 26.0 to develop a custom dynamic gestational week fitting formula. Matlab 2019b was used for SVM model research. R software (v4.0.1) was used for stepwise regression and Lasso regression model researches. We compared model prediction effects by area under the ROC curve (AUC), accuracy, and the model was externally validated by the validation set.
Results
Analysis of static risk factors
For gestational hypertension, we compared qualitative factors between the gestational hypertension group and the control group, and found there were statistically significant differences in multiple pregnancy, gestational diabetes and pregestational diabetes mellitus between the two groups (OR > 1 and P < 0.05). The pre-pregnancy body mass index of gestational hypertension group was significantly higher than that of control group (P < 0.05) (Table 4). For early-onset preeclampsia, the qualitative factors that met OR > 1 and P < 0.05 were multiple pregnancy, history of spontaneous abortion and history of HDP. The quantitative factors that met P < 0.05 was pre-pregnancy body mass index (Table 5). For late-onset preeclampsia, we found significant differences between the late-onset preeclampsia group and control group in multiple pregnancy, history of spontaneous abortion, history of HDP, family history of hypertension and family history of diabetes (OR > 1 and P < 0.05). The quantitative factors that were significantly different between the two groups was pre-pregnancy body mass index (P < 0.05) (Table 6). For chronic hypertension complicated with preeclampsia, qualitative factors of multiple pregnancy, family history of hypertension, gestational diabetes, pregestational diabetes mellitus, pregnancy combined with immune system disorders and pregnancy combined with thyroid disorders were risk factors of chronic hypertension complicated with preeclampsia (OR > 1 and P < 0.05). Pre-pregnancy body mass index among the quantitative factors was a risk factor for chronic hypertension combined with preeclampsia (P < 0.05) (Table 7).
Analysis of dynamic risk factors
We analyzed all dynamic factors within the five gestational stages, and found dynamic factors were significantly different between the gestational hypertension group and the control group (Table 8). The difference in platelet count (PLT) between the early-onset preeclampsia group and the control group was not statistically significant, and the mean value did not exceed the normal range (Table 9). In this paper, we did not consider PLT as a risk factor for early-onset preeclampsia. We found there was no statistically significant differences in total peripheral resistance (TPR) between the late-onset preeclampsia group and the control group, but the TPR was outside the normal range at 10–13 and 35–40 weeks (Table 10). Therefore, we considered TPR as a risk factor for late-onset preeclampsia. The difference in pulse pressure (PP) between the chronic hypertension combined with preeclampsia group and the control group was not statistically significant, and the mean value did not exceed the normal range (Table 11). Therefore, we did not consider PP as a risk factor for chronic hypertension combined with preeclampsia.
Model construction results
We used nonlinear regression, SVM, step regression and Lasso regression for each HDP subgroup to develop prediction models. The P-values of the models were all less than 0.001, which indicated that the models were stable. We compared the prediction results of the four models, the Lasso regression prediction model of the gestational hypertension was optimal: accuracy = 90.32%, AUC = 0.910, sensitivity = 75.86%, specificity = 93.32%; the Lasso regression prediction model of the early-onset preeclampsia was optimal: accuracy = 91.78%, AUC = 0.962, sensitivity = 86.21%, specificity = 92.18%; Lasso regression prediction model for late-onset preeclampsia was optimal: accuracy = 85.58%, AUC = 0.859, sensitivity = 72.73%, specificity = 89.47%; Lasso regression prediction model for chronic hypertension complicated with preeclampsia was optimal: accuracy = 91.72%, AUC = 0.955, sensitivity = 93.10%, specificity = 91.63% (Figure 1 and Table 12). PlGF was tested significant (P < 0.05) only in the stepwise regression dynamic prediction model for early-onset preeclampsia (Table 13), the predictive effect of PlGF in gestational hypertension, late-onset preeclampsia, and chronic hypertension complicated with preeclampsia was not significant, with parameter term coefficients of −3.26E−03, −1.39E−04, and −6.11E−03, respectively.
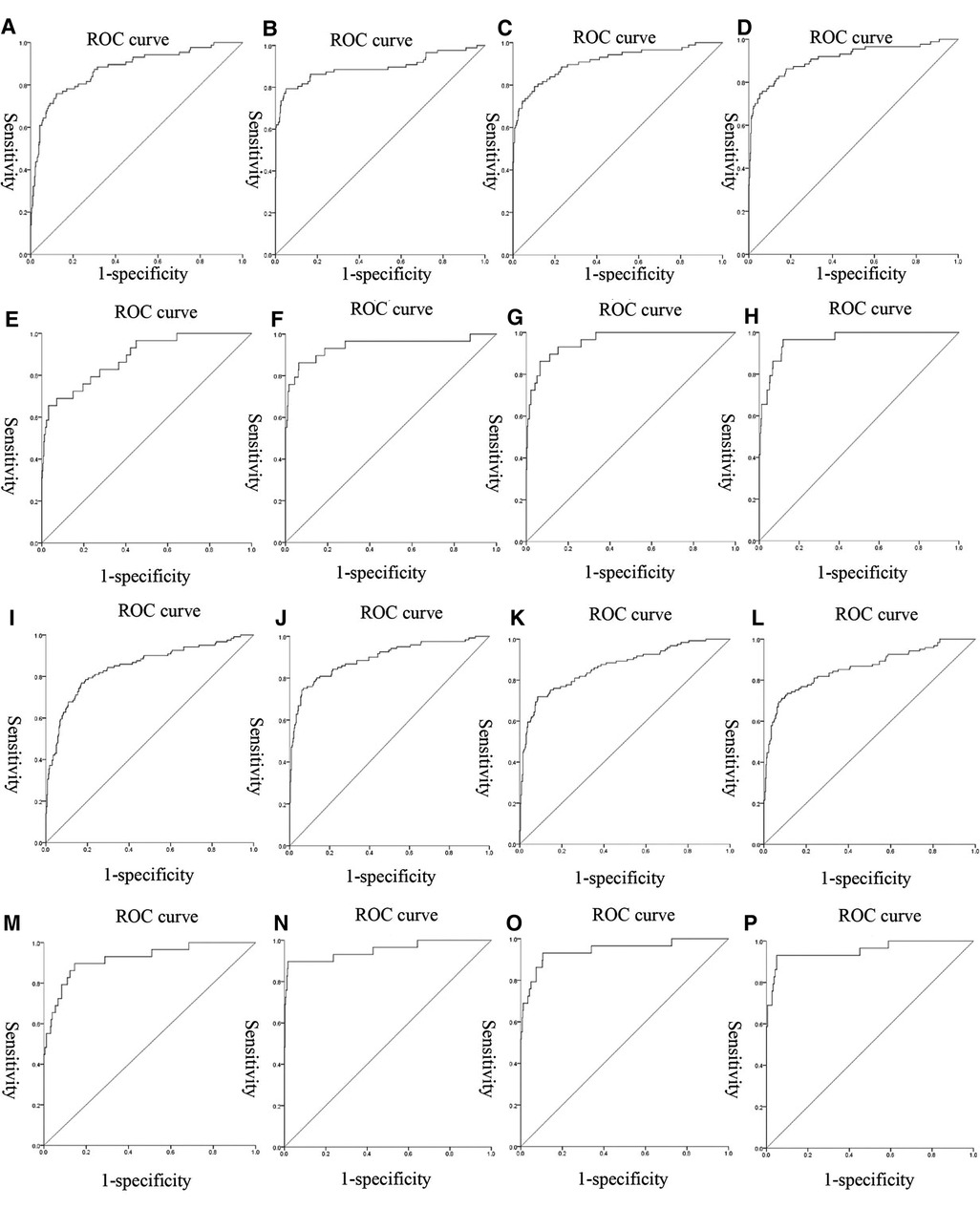
Figure 1. ROC curves of the models: (A–D) were the ROC curves of the four models of gestational hypertension; (E–H) were the ROC curves of the four models of early-onset preeclampsia; (I–L) were the ROC curves of the four models of early-onset preeclampsia; (M–P) were the ROC curves of the four models of chronic hypertension complicated with preeclampsia.
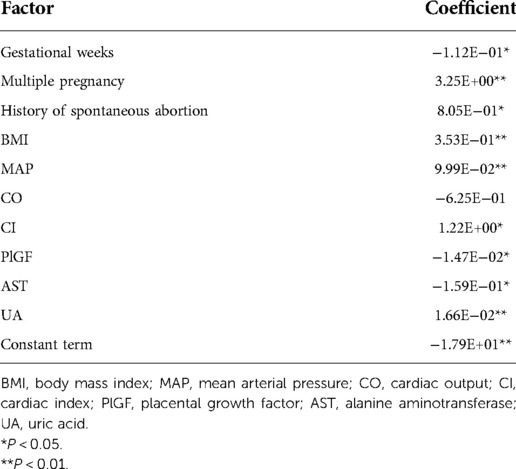
Table 13. Parameters of the stepwise regression dynamic prediction model for the early-onset preeclampsia.
The validation results showed that Lasso regression prediction model had the highest accuracy among the four prediction models in the chronic hypertension complicated with preeclampsia (Table 14).
Discussion
Hypertensive pregnancy in disorders are pregnancy-specific systematic disorders that globally affect 5%–10% of all pregnancies (33, 34). We performed a comprehensive screening of high-risk factors for gestational hypertension, early-onset preeclampsia, late-onset preeclampsia and chronic hypertension combined with preeclampsia, which through the acquisition of clinical medical records of patients. For each HDP subtype, we constructed dynamic prediction models using nonlinear regression, support vector machines, stepwise regression, and Lasso regression. The results showed that the Lasso regression dynamic prediction model had the best prediction effect for the four HDP subtypes, which could help clinicians accurately assess the risk of HDP.
We compared the AUC of the four prediction models for each HDP subgroup, and we found that the AUC of the Lasso regression prediction model was higher than the other three prediction models. The accuracy of Lasso regression prediction model was over 85% for each HDP subgroup, and 91.78% for EOPE subgroup was the highest (Table 12). External validation of the model through the validation set, we found that Lasso regression prediction model had a good identification effect, with the accuracy of 86.67%, 83.33%, 76.67% and 100.00% for each HDP subtype, respectively (Table 14). Lasso regression allows automatic filtering of model parameters, and the Lasso regression model simplifies the input parameters of the model and makes the model structure simpler (Table 13).
PlGF is a member of the vascular endothelial growth factor family and has important functions in regulating placental trophoblast and endothelial cell function (35). Numerous studies have shown that PlGF is a risk factor for HDP and has a predictive value for preeclampsia in particular (36, 37). PlGF was tested significant only in the stepwise regression model for the early-onset preeclampsia, which indicated a significant predictive effect of PlGF on the early-onset preeclampsia (Table 13).
Finally, there were some limitations in this research. First, this research was carried out in China, and the medical records used for model construction were all from pregnant Chinese women. Due to differences among regions and races, the applicability of the model to other countries needs to be further verified. Second, we developed prediction models for the four HDP subtypes in this study and found that the lasso regression prediction model had the best prediction effect, so it was impossible to explore the predictive ability of other HDP subtype.
Conclusion
We investigated the predictive effect of different variable selection and modeling approaches on HDP subtypes, and found the Lasso regression prediction model performed well and accurately predicted the risk of HDP subtypes. The Lasso regression prediction model provided corresponding guidance and served as a basis for preventing adverse outcomes and improving clinical treatment.
Data availability statement
The original contributions presented in the study are included in the article/Supplementary Material, further inquiries can be directed to the corresponding author/s.
Ethics statement
The studies involving human participants were reviewed and approved by Ethics Committee of Science and Technology of Beijing University of Technology. The patients/participants provided their written informed consent to participate in this study.
Author contributions
XZ, LY, and GL: conception and design of the research. QX, GL, and GS: acquisition of data. XZ, GS, and QX: analysis and interpretation of the data and statistical analysis. LY, DH, YY and XL: funding acquisition. XZ: writing of the manuscript. LY, CL, ZL, and XZ: critical revision of the manuscript for intellectual content. All authors contributed to the article and approved the submitted version.
Funding
This research was funded by the National Key R/D Program of China (2019YFC0119700), National Natural Science Foundation of China (U20A201163).
Acknowledgments
We would like to thank all participants in this research and the obstetrics staff of Beijing Maternity Hospital, Beijing Haidian District Maternal and Child Health Hospital and Peking University People's Hospital for their work in patient registration.
Conflict of interest
The authors declare that the research was conducted in the absence of any commercial or financial relationships that could be construed as a potential conflict of interest.
Publisher's note
All claims expressed in this article are solely those of the authors and do not necessarily represent those of their affiliated organizations, or those of the publisher, the editors and the reviewers. Any product that may be evaluated in this article, or claim that may be made by its manufacturer, is not guaranteed or endorsed by the publisher.
References
1. Smith AL, Owen H, Reynolds KJ. Heart rate variability indices for very short-term (30 beat) analysis. Part 2: validation. J Clin Monit Comput. (2013) 27(5):577–85. doi: 10.1007/s10877-013-9473-2
2. Boulet SL, Platner M, Joseph NT, Campbell A, Williams R, Stanhope KK, et al. Hypertensive disorders of pregnancy, cesarean delivery, and severe maternal morbidity in an urban safety-net population. Am J Epidemiol. (2020) 189(12):1502–11. doi: 10.1093/aje/kwaa135
3. Wu P, Chew-Graham CA, Maas AH, Chappell LC, Potts JE, Gulati M, et al. Temporal changes in hypertensive disorders of pregnancy and impact on cardiovascular and obstetric outcomes. Am J Cardiol. (2020) 125(10):1508–16. doi: 10.1016/j.amjcard.2020.02.029
4. Li F, Qin J, Zhang S, Chen L. Prevalence of hypertensive disorders in pregnancy in China: a systematic review and meta-analysis. Pregnancy Hypertens. (2021) 24:13–21. doi: 10.1016/j.preghy.2021.02.001
5. ACOG practice bulletin no. 202: gestational hypertension and preeclampsia. Obstet Gynecol. (2019) 133(1):1. doi: 10.1097/aog.0000000000003018
6. Burton GJ, Redman CW, Roberts JM, Moffett A. Pre-eclampsia: pathophysiology and clinical implications. Br Med J. (2019) 366:l2381. doi: 10.1136/bmj.l2381
7. von Dadelszen P, Magee LA, Roberts JM. Subclassification of preeclampsia. Hypertens Pregnancy. (2003) 22(2):143–8. doi: 10.1081/prg-120021060
8. Verma J, Thomas DC, Jhingan G, Puri RD, Verma IC. Mom cutoffs for variables, an important tool for multivariate analysis and accurate interpretation of preeclampsia risk in high-risk pregnancy at 11-13(+6) weeks gestation. Hypertens Pregnancy. (2016) 35(4):548–58. doi: 10.1080/10641955.2016.1211675
9. Shahid R, Bari MF, Hussain M. Serum biomarkers for the prediction and diagnosis of preeclampsia: a meta-analysis. J Taibah Univ Med Sci. (2022) 17(1):14–27. doi: 10.1016/j.jtumed.2021.07.003
10. DeVries CR, Starbird WT, Arab A, Bailey B. Investigation of factors that predict risk for hospital readmission following delivery of pregnancies complicated by hypertensive disorders of pregnancy. J Matern Fetal Neonatal Med. (2022) 1:1–5. doi: 10.1080/14767058.2022.2030306
11. Barquiel B, Herranz L, Grande C, Castro-Dufourny I, Llaro M, Parra P, et al. Body weight, weight gain and hyperglycaemia are associated with hypertensive disorders of pregnancy in women with gestational diabetes. Diabetes Metab. (2014) 40(3):204–10. doi: 10.1016/j.diabet.2013.12.011
12. Abraham T, Romani AMP. The relationship between obesity and pre-eclampsia: incidental risks and identification of potential biomarkers for pre-eclampsia. Cells. (2022) 11(9):1548. doi: 10.3390/cells11091548
13. Visser S, Hermes W, Ket JC, Otten RH, van Pampus MG, Bloemenkamp KW, et al. Systematic review and metaanalysis on nonclassic cardiovascular biomarkers after hypertensive pregnancy disorders. Am J Obstet Gynecol. (2014) 211(4):373.e1–9. doi: 10.1016/j.ajog.2014.03.032
14. Ferrazzi E, Stampalija T, Monasta L, Di Martino D, Vonck S, Gyselaers W. Maternal hemodynamics: a method to classify hypertensive disorders of pregnancy. Am J Obstet Gynecol. (2018) 218(1):124.e1–124.e11. doi: 10.1016/j.ajog.2017.10.226
15. van Balen VA L, Spaan JJ, Ghossein C, van Kuijk SM, Spaanderman ME, Peeters LL. Early pregnancy circulatory adaptation and recurrent hypertensive disease: an explorative study. Reprod Sci. (2013) 20(9):1069–74. doi: 10.1177/1933719112473658
16. Lin J, Hong XY, Tu RZ. The value of serum uric acid in predicting adverse pregnancy outcomes of women with hypertensive disorders of pregnancy. Ginekol Pol. (2018) 89(7):375–80. doi: 10.5603/GP.a2018.0064
17. Kehler S, Rayens MK, Ashford K. Determining whether hypertensive status and stress level are associated with inflammatory markers. Biol Res Nurs. (2019) 21(3):245–52. doi: 10.1177/1099800419828104
18. Jim B, Sharma S, Kebede T, Acharya A. Hypertension in pregnancy: a comprehensive update. Cardiol Rev. (2010) 18(4):178–89. doi: 10.1097/CRD.0b013e3181c60ca6
19. Sun XW, Li XH, Zhang C, Meng FQ, Xing YG, Ding Y. Correlation analysis of serum placental growth factor, pregnancy-related plasma protein-A and disease severity in patients with hypertensive disorder in pregnancy. Eur Rev Med Pharmacol Sci. (2021) 25(4):1788–95. doi: 10.26355/eurrev_202102_25072
20. Nguyen TH, Bui TC, Vo TM, Tran QM, Luu LT, Nguyen TD. Predictive value of the sFlt-1 and PlGF in women at risk for preeclampsia in the south of Vietnam. Pregnancy Hypertens. (2018) 14:37–42. doi: 10.1016/j.preghy.2018.07.008
21. Bian X, Biswas A, Huang X, Lee KJ, Li TK, Masuyama H, et al. Short-term prediction of adverse outcomes using the sFlt-1 (soluble fms-like tyrosine kinase 1)/PlGF (placental growth factor) ratio in Asian women with suspected preeclampsia. Hypertension. (2019) 74(1):164–72. doi: 10.1161/hypertensionaha.119.12760
22. Cnossen JS, Morris RK, ter Riet G, Mol BWJ, van der Post JAM, Coomarasamy A, et al. Use of uterine artery Doppler ultrasonography to predict pre-eclampsia and intrauterine growth restriction: a systematic review and bivariable meta-analysis. Can Med Assoc J. (2008) 178(6):701–11. doi: 10.1503/cmaj.070430
23. Laskin S, Payne B, Hutcheon JA, Qu Z, Douglas MJ, Ford J, et al. The role of platelet counts in the assessment of inpatient women with preeclampsia. J Obstet Gynaecol Can. (2011) 33(9):900–8. doi: 10.1016/s1701-2163(16)35015-0
24. Stepan H, Hund M, Andraczek T. Combining biomarkers to predict pregnancy complications and redefine preeclampsia: the angiogenic-placental syndrome. Hypertension. (2020) 75(4):918–26. doi: 10.1161/hypertensionaha.119.13763
25. Chen Y, Wang X, Hu W, Chen Y, Ning W, Lu S, et al. A risk model that combines MAP, PlGF, and PAPP-A in the first trimester of pregnancy to predict hypertensive disorders of pregnancy. J Hum Hypertens. (2022) 36(2):184–91. doi: 10.1038/s41371-021-00488-6
26. Vainio M, Kujansuu E, Koivisto AM, Mäenpää J. Bilateral notching of uterine arteries at 12–14 weeks of gestation for prediction of hypertensive disorders of pregnancy. Acta Obstet Gynecol Scand. (2005) 84(11):1062–7. doi: 10.1111/j.0001-6349.2005.00889.x
27. Hasija A, Balyan K, Debnath E VR, Kumar M. Prediction of hypertension in pregnancy in high risk women using maternal factors and serial placental profile in second and third trimester. Placenta. (2021) 104:236–42. doi: 10.1016/j.placenta.2021.01.005
28. Meah VL, Cockcroft JR, Backx K, Shave R, Stöhr EJ. Cardiac output and related haemodynamics during pregnancy: a series of meta-analyses. Heart. (2016) 102(7):518–26. doi: 10.1136/heartjnl-2015-308476
29. Poon LC, Kametas NA, Maiz N, Akolekar R, Nicolaides KH. First-trimester prediction of hypertensive disorders in pregnancy. Hypertension. (2009) 53(5):812–8. doi: 10.1161/hypertensionaha.108.127977
30. Sun G, Xu Q, Zhang S, Yang L, Liu G, Meng Y, et al. Predicting hypertensive disorders in pregnancy using multiple methods: models with the placental growth factor parameter. Technol Health Care. (2021) 29(S1):427–32. doi: 10.3233/thc-218040
31. Gootjes DV, Posthumus AG, Jaddoe VWV, van Rijn BB, Steegers EAP. Maternal hypertensive disorders in pregnancy and early childhood cardiometabolic risk factors: the generation R study. PLoS One. (2021) 16(12):e0261351. doi: 10.1371/journal.pone.0261351
32. Verlohren S, Herraiz I, Lapaire O, Schlembach D, Moertl M, Zeisler H, et al. The sFlt-1/PlGF ratio in different types of hypertensive pregnancy disorders and its prognostic potential in preeclamptic patients. Am J Obstet Gynecol. (2012) 206(1):58.e1–8. doi: 10.1016/j.ajog.2011.07.037
33. Umesawa M, Kobashi G. Epidemiology of hypertensive disorders in pregnancy: prevalence, risk factors, predictors and prognosis. Hypertens Res. (2017) 40(3):213–20. doi: 10.1038/hr.2016.126
34. Hutcheon JA, Lisonkova S, Joseph KS. Epidemiology of pre-eclampsia and the other hypertensive disorders of pregnancy. Best Pract Res Clin Obstet Gynaecol. (2011) 25(4):391–403. doi: 10.1016/j.bpobgyn.2011.01.006
35. Duhig KE, Webster LM, Sharp A, Gill C, Seed PT, Shennan AH, et al. Diagnostic accuracy of repeat placental growth factor measurements in women with suspected preeclampsia: a case series study. Acta Obstet Gynecol Scand. (2020) 99(8):994–1002. doi: 10.1111/aogs.13818
36. Armaly Z, Jadaon JE, Jabbour A, Abassi ZA. Preeclampsia: novel mechanisms and potential therapeutic approaches. Front Physiol. (2018) 9:973. doi: 10.3389/fphys.2018.00973
Keywords: hypertensive disorders in pregnancy, subtype, risk factor analysis, modeling method, dynamic prediction model, lasso regression
Citation: Zhang X, Xu Q, Yang L, Sun G, Liu G, Lian C, Li Z, Hao D, Yang Y and Li X (2022) Dynamic risk prediction models for different subtypes of hypertensive disorders in pregnancy. Front. Surg. 9:1005974. doi: 10.3389/fsurg.2022.1005974
Received: 28 July 2022; Accepted: 4 October 2022;
Published: 26 October 2022.
Edited by:
David Alan Ellwood, Griffith University, AustraliaReviewed by:
Nazan Yurtcu, Sivas Cumhuriyet University Faculty of Medicine, TurkeyShan Chang, Jiangsu University of Technology, China
© 2022 Zhang, Xu, Yang, Sun, Liu, Lian, Li, Hao, Yang and Li. This is an open-access article distributed under the terms of the Creative Commons Attribution License (CC BY). The use, distribution or reproduction in other forums is permitted, provided the original author(s) and the copyright owner(s) are credited and that the original publication in this journal is cited, in accordance with accepted academic practice. No use, distribution or reproduction is permitted which does not comply with these terms.
*Correspondence: Lin Yang yanglin@bjut.edu.cn Guoli Liu liuguoli@pkuph.edu.cn
Specialty Section: This article was submitted to Obstetrics and Gynecological Surgery, a section of the journal Frontiers in Surgery