- 1Integrated Research Literacy Group, Draper, UT, United States
- 2Department of Psychiatry, University of Cambridge, Cambridge, England
- 3Department of Clinical Neurosciences, University of Cambridge, Cambridge, England
- 4Department of Applied Mathematics and Theoretical Physics, University of Cambridge, Cambridge, England
- 5Department of Bioengineering, University of Pennsylvania, Philadelphia, PA, United States
- 6Department of Physics and Astronomy, The University of Utah, Salt Lake City, UT, United States
- 7Department of Molecular and Cellular Biology, Harvard University, Cambridge, MA, United States
- 8Department of Biology, Stanford University, Stanford, CA, United States
- 9Instituto de Biomedicina de Sevilla (IBiS) HUVR/CSIC/Universidad de Sevilla/CIBERSAM, ISCIII, Dpto. de Fisiología Médica y Biofísica
Background: Augmented reality (AR) is increasingly being explored in neurosurgical practice. By visualizing patient-specific, three-dimensional (3D) models in real time, surgeons can improve their spatial understanding of complex anatomy and pathology, thereby optimizing intra-operative navigation, localization, and resection. Here, we aimed to capture applications of AR in glioma surgery, their current status and future potential.
Methods: A systematic review of the literature was conducted. This adhered to the Preferred Reporting Items for Systematic Reviews and Meta-Analyses (PRISMA) guideline. PubMed, Embase, and Scopus electronic databases were queried from inception to October 10, 2022. Leveraging the Population, Intervention, Comparison, Outcomes, and Study design (PICOS) framework, study eligibility was evaluated in the qualitative synthesis. Data regarding AR workflow, surgical application, and associated outcomes were then extracted. The quality of evidence was additionally examined, using hierarchical classes of evidence in neurosurgery.
Results: The search returned 77 articles. Forty were subject to title and abstract screening, while 25 proceeded to full text screening. Of these, 22 articles met eligibility criteria and were included in the final review. During abstraction, studies were classified as “development” or “intervention” based on primary aims. Overall, AR was qualitatively advantageous, due to enhanced visualization of gliomas and critical structures, frequently aiding in maximal safe resection. Non-rigid applications were also useful in disclosing and compensating for intra-operative brain shift. Irrespective, there was high variance in registration methods and measurements, which considerably impacted projection accuracy. Most studies were of low-level evidence, yielding heterogeneous results.
Conclusions: AR has increasing potential for glioma surgery, with capacity to positively influence the onco-functional balance. However, technical and design limitations are readily apparent. The field must consider the importance of consistency and replicability, as well as the level of evidence, to effectively converge on standard approaches that maximize patient benefit.
Introduction
Gliomas account for 78% of primary malignant brain tumors (1). They originate from glial progenitor cells, namely astrocytes or oligodendrocytes, that constitute a significant portion of the mammalian brain. As such, gliomas are highly heterogeneous, known for their diverse histopathology, molecular genetics, and clinical behavior. In the United States, the incidence of gliomas varies from 4.7 to 5.7 per 100,000 persons (2), representing more than 18,500 new cases and 13,000 deaths annually (3). Glioblastoma multiforme (GBM), the most common and aggressive form of glioma, has a median survival of 16 months (4), carrying a five-year post-diagnosis survival rate of 6.8% (5). While the pathogenesis differs considerably, low-grade glioma has a more favorable timespan, ranging from 5.6 to 13.3 years, depending on several prognostic factors (6). Nonetheless, 70% of these tumors transform to GBM within 10 years (7). This eventually causes disability and premature death (8, 9).
Currently, the primary care pathway for gliomas is surgical resection followed by chemoradiotherapy, with concomitant temozolomide or other alkylating drugs (10, 11). Maximizing the extent of resection (EOR), until functional borders are encountered, is central to prolonging survival, improving the efficacy of adjuvant therapies, and delaying anaplastic transformation in both low- and high-grade glioma (12–15). The rationale for performing this type of “supratotal” resection is based on evidence that gliomas infiltrate the parenchyma well beyond magnetic resonance imaging (MRI)-defined abnormalities (16). Tumor recurrence may thus arise from undetected glioma cells growing beyond signal abnormalities, typically found 1–2 cm outside of contrast enhancement, as detected on volumetric fluid-attenuated inversion recovery (FLAIR) images. However, supratotal resection is not always practical or feasible to achieve.
Diffusely infiltrating gliomas often limit radical resection strategies, which preferentially invade along myelinated fibers in white matter tracts (17); cluster in eloquent brain regions with dense functional connections, like the basal ganglia and internal capsule (18); and develop functional multi-cellular network structures (19). Therefore, surgically-acquired lesions in functionally critical areas may cause significant neurologic morbidity and mortality (20, 21). Neural plasticity is another barrier to radical resection, due to functional reorganization (22, 23). Injury to white matter tracts, dynamically interacting with gliomas, is linked to post-operative deficits, accordingly (24, 25). Hence, the true benefit of resection depends on the “onco-functional balance”: (26) maximizing the extent of tumor removal while preserving patients’ functional integrity and quality of life.
Augmented reality (AR) is a technology that superimposes computer-generated, three-dimensional (3D) holograms, as well as auditory and sensory feedback, on reality in real time and space. This composite view of virtual objects with the real world creates a semi-immersive environment. Dating back to 1986 (27), AR has been applied in neurosurgery for nearly 30 years, carrying several advantages over conventional approaches (28).
First, AR maps patient-specific neuroanatomy directly onto the operating field, rendering display of surface and sub-surface targets. This has proven useful in visualizing anatomical structures, vasculature and hemodynamics, and deep-seated lesions in stereotactic, neurovascular, and tumor surgery, respectively (29). It also allows surgeons to access and contextualize radiological images and pre-operative planning. Second, AR eliminates attentional shifts between patients on operating tables and screens displaying relevant clinical information. This can reduce fatigue, cognitive load, and inattention blindness among surgeons, leading to more focused and efficient procedures (30). Third and finally, AR may disclose and compensate for intra-operative brain shift (31, 32): a highly prevalent and complex phenomenon of brain deformation due to changes in gravity and hydrostatic pressure, loss of cerebrospinal fluid, tissue manipulation or removal, and other factors (33). For image- and function-guided neurosurgery, this can invalidate patient-to-image registration and reduce the accuracy of localizing and resecting intra-cranial targets, as well as positioning surgical tools (34, 35). Thus, AR can be used to update virtual scenes, when combined with multimodal imaging and functional testing, to precisely identify pathologies, probe subcortical pathways, and tailor resection plans (28, 36, 37).
To date, applications of AR in neurosurgery have been limited to early clinical research. Given this stage, a variety of AR devices have been used for image projection, including head-up displays (HUDs), head-mounted displays (HMDs), microscopes, endoscopes, smartphones, and tablets (29, 37–40). Commercial display devices have also emerged, such as Google Glass (Google LLC, Mountain View, California, USA), HoloLens (Microsoft Corp., Redmond, Washington, USA), and Magic Leap (Magic Leap Inc., Plantation, Florida, USA) (29). Such innovation underscores the growing clinical and commercial interest in AR for neurosurgical practice, with proposed roles in skin incision, craniotomy, and resection (37, 38, 40). This is of particular import for eloquent brain tumors, as serious threats to human life and health, whereby AR may positively influence maximal safe resection and functional outcomes. In this review, we summarize current applications of AR in glioma surgery, as described in the scientific literature, with the aim of characterizing emerging trends and providing avenues for future research.
Methods
We performed an in-depth systematic review, adhering to the Preferred Reporting Items for Systematic Reviews and Meta-Analyses (PRISMA) guideline (41). The review protocol was registered a priori with the Open Science Framework (OSF) (42), developed and maintained by the Center for Open Science (COS), which can be accessed via the digital object identifier (DOI): 10.17605/OSF.IO/DJ72P. The PRISMA 2020 Checklist (41), review strategy, and review protocol are additionally available for consultation upon reasonable request.
PubMed (National Library of Medicine), Embase (Elsevier), and Scopus (Elsevier) electronic databases were queried from inception to October 10, 2022, for relevant articles. The following search strategy was employed: PubMed: ((“augmented reality”[All Fields] OR “mixed reality”[All Fields]) AND (“glioma”[MeSH Terms] OR “glioma”[All Fields] OR “gliomas”[All Fields] OR “glioma s”[All Fields])); Embase: ((“augmented reality”/exp OR “augmented reality” OR “mixed reality”/exp OR “mixed reality”) AND (“glioma”/exp OR “glioma”) AND [article]/lim AND [humans]/lim); and Scopus: TITLE-ABS-KEY (((“augmented reality” OR “mixed reality”) AND (glioma))). No publication date or study type restrictions were applied.
Inclusion criteria consisted of (1) phantoms or patients of any age and biological sex diagnosed with glioma; (2) AR developed for or applied in glioma surgery, specifically to aid intra-operative navigation, localization, and/or resection; (3) protocol or technical note papers; case-control studies, case series, or case reports; retrospective, prospective, or concurrent cohort studies; or non-randomized, randomized, or post-hoc analyses of clinical trials; and (4) peer-reviewed studies published in the English language. In contrast, exclusion criteria comprised (1) phantoms or patients without glioma; (2) studies not developing or applying AR for/in glioma surgery, such as for patient education and surgical planning purposes; (3) reviews, editorials, expert opinion pieces, commentaries, letters to the editor, and articles with inaccessible full texts; and (4) studies not peer-reviewed and published in the English language. Duplicates were excluded prior to screening and studies that failed to meet full inclusion criteria were excluded from the overall analysis. The “Population, Intervention, Comparison, Outcomes, and Study design” (PICOS) (43) framework was applied for evaluating eligibility criteria in the qualitative synthesis.
Two independent reviewers, one with and one without prior content knowledge (N.B., M.H.Z.), screened articles against PICOS criteria, initially evaluating their titles and abstracts. Relevant studies were then selected for full text screening and assessed for eligibility. Inter-rater agreement was reported (Cohen's k = 0.74), with disagreements reconciled through discussion and/or by involvement of a third independent reviewer (A.R.) until a consensus was reached.
Another set of independent reviewers (W.H., A.K.U.) subsequently extracted data from eligible studies into a Microsoft Excel Spreadsheet (Microsoft Corp., Redmond, Washington, USA). Table cells were labeled as “Not applicable” (N/A) if data were missing. To ensure global data integrity, an independent reviewer (Z.O.U.) performed quality assurance checks at random. Data extracted from eligible studies included: study year, study location, study design, study type, number of total patients, number of glioma patients, number of phantom patients, glioma pathology, other pathologies, image acquisition phase, image data source, image segmentation technique, geometric modeling software, registration method, registration accuracy, display device, display brand, clinical application, primary outcomes, and any other pertinent findings. Following extraction, data were qualitatively described, using frequency (count, percentage), central tendency (mean, median, mode), and variability (range, standard deviation), as applicable, via R version 4.1.3 (44). Pooled statistical analyses, such as meta-regressions, were not performed due to heterogeneity in study designs and measured outcomes.
To assess the quality of evidence, a risk of bias assessment was conducted, using hierarchical classes of evidence in neurosurgery (45). This involved ranking the methodological rigor of each study, whereby “Level V” indicates the lowest or weakest level of evidence, such as case reports, and “Level I” indicates the highest or strongest level of evidence, such as randomized trials. As the study design becomes more rigorous, the quality of evidence increases, and the probability of bias decreases. Two independent reviewers (V.N.C., L.A.) conducted the risk of bias assessment, with inter-rater agreement reported (Cohen's k = 0.82).
Results
The initial search query returned 77 articles for potential inclusion in the review [PubMed (n = 21), Embase (n = 25), and Scopus (n = 31)]. Of these, 40 (52%) unique articles remained following removal of duplicates (35, 46%) and inaccessible texts (2, 3%). After title and abstract screening, 15 (38%) articles were excluded for being literature reviews (7, 18%), investigating non-glioma tumors (1, 3%), not involving AR (4, 10%), or falling out of scope with PICOS criteria (3, 8%). Accordingly, 25 (63%) full text articles were assessed for eligibility, with 3 (12%) deemed non-eligible for inclusion.
The final review comprised 22 articles, with the first study published in 2003 (n = 1), the largest number published in 2021 (n = 6), and the most recent published in 2022 (n = 4). See studies by publication year in Figure 1. Regarding location, the majority of studies were conducted in Asia (13, 59%) followed by Europe (7, 32%) and North America (2, 9%). The PRISMA 2020 flow diagram, describing the search strategy and selection schema, is displayed in Figure 2. Characteristics of the studies included in the review are summarized in Tables 1–3.
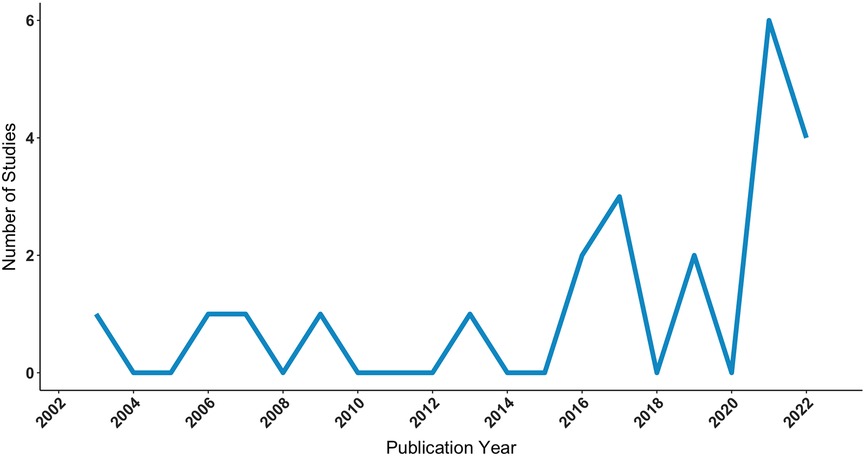
Figure 1. Number of studies applying AR in glioma surgery by publication year, as identified via PubMed, Embase, and Scopus electronic database searches, executed on October 22, 2022. AR, augmented reality.
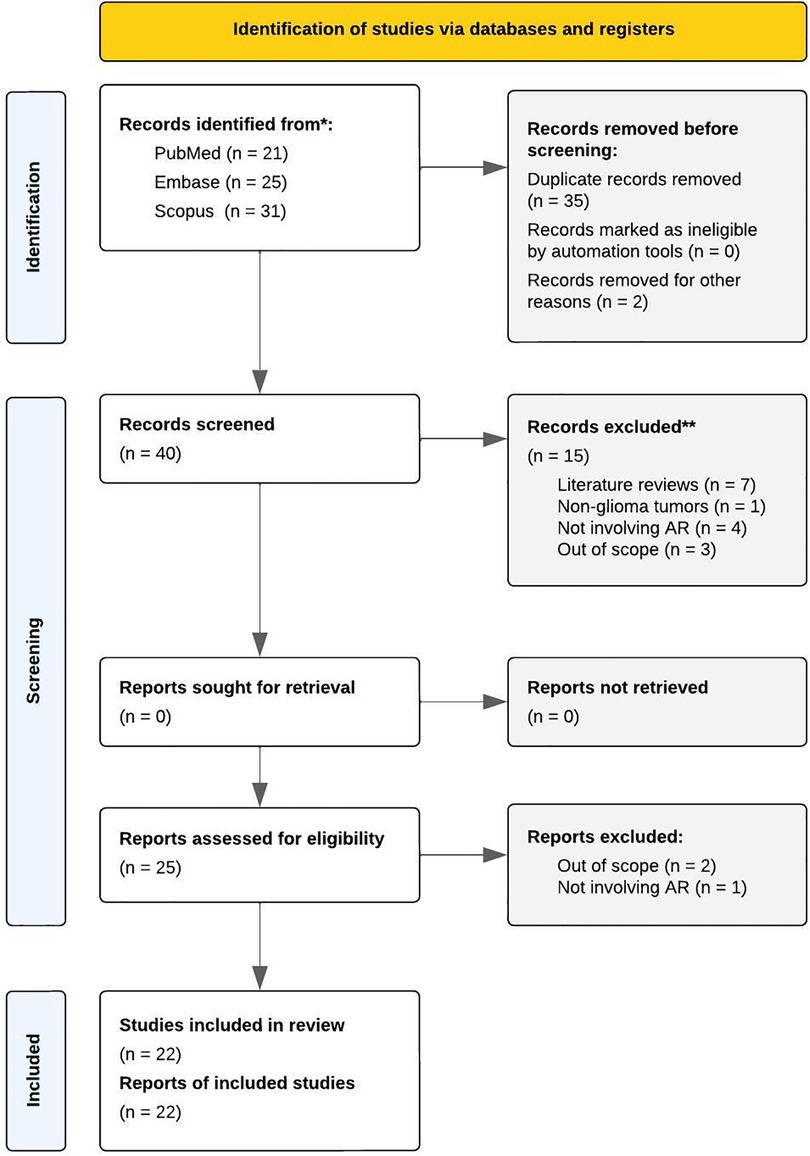
Figure 2. PRISMA 2020 flow diagram, describing the search strategy and selection schema of the review process.
At the time of data abstraction, based on primary aims, studies were sub-grouped into the following categories: “development” and “intervention”. Ten (45%) studies (46, 48–50, 53, 54, 57–59, 67) evaluated the technical design and suitability of AR for glioma surgery, principally assessing feasibility, accuracy, and/or reliability benchmarks (i.e., development). The remaining 12 (55%) studies (47, 51, 52, 55, 56, 60–66) investigated the clinical utility of AR for glioma surgery, with a focus on feasibility, safety, and/or efficacy profiles (i.e., intervention). Across studies, there was a total of 909 patients (41.3 ± 54.1), of which 488 were diagnosed with gliomas (22.2 ± 35.7). Other pathologies included meningioma, lymphoma, angioma, papilloma, craniopharyngioma, hemangioblastoma, and arachnoid cysts, among others. Figure 3A–B illustrates the number of glioma patients and imaging acquisition protocol across study types. Three studies (51, 59, 67) additionally leveraged phantoms (n = 22, 1.0 ± 4.3). Steps for applying AR models in glioma surgery comprised: (1) image acquisition, (2) image segmentation, (3) geometric model generation, (4) registration and tracking, and (5) intra-operative navigation via fused image overlay.
1. Image acquisition
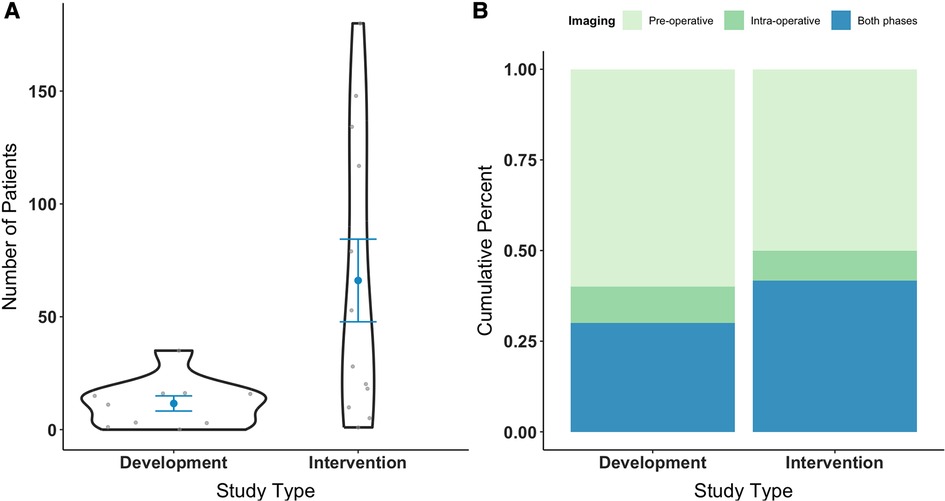
Figure 3. Number of patients diagnosed with glioma recruited in AR studies by study type, excluding phantom patients (A). Medical image acquisition phase by study type, spanning pre-operative and/or intra-operative stages (B). Study types are grouped by primary aim into “development” or “intervention”, with respect to AR application. AR, augmented reality.
Data for AR were obtained from a variety of medical imaging sources that occasionally integrated with network analysis and brain mapping. All studies performed anatomical or volumetric magnetic resonance imaging (MRI: 22, 100%), followed by computerized tomography (CT: 16, 73%), diffusion weighted or tensor imaging (DWI/DTI: 13, 59%), functional magnetic resonance imaging (fMRI: 4, 20%), computerized tomography angiography (CTA: 3, 14%), 3D rotational angiography (3DRA: 3, 14%), and magnetic resonance spectroscopy (MR spectroscopy: 2, 9%). Across image acquisitions, pertinent factors included spatial resolution, slice thickness, signal- and contrast-to-noise ratios, and image artifact.
DTI-based tractography, ultrasound, and navigated transcranial magnetic stimulation (nTMS) were additionally carried out as complimentary techniques in 8 (36%), 1 (5%), and 1 (5%) study, respectively. Of those involving DTI-based tractography, two studies (61, 62) performed high-definition fiber tractography with sodium fluorescein (HDFT-F), motivated by the prospect of increasing tumor resectability as well as survival rates. In another study (52), ultrasound images were obtained prior to corticectomy and following resection, with the aim of delineating lesion borders and post-resection cavity. See Figure 4 for the distribution of imaging sources used for AR. Most images were obtained pre-operatively (12, 55%), with 2 (9%) studies obtaining images intra-operatively and 8 (36%) from both phases (Figure 3B).
2. Image segmentation
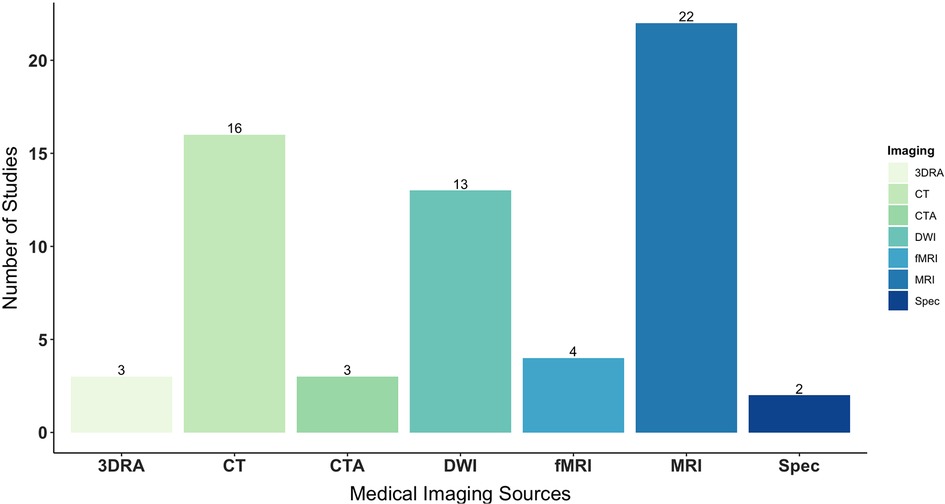
Figure 4. Types of medical imaging used as data sources for AR, specifically segmenting, modeling, formatting, and projecting virtual objects onto phantoms or patients’ real anatomy. AR, augmented reality.
Imaging data was partitioned, or “segmented”, into anatomical regions of interest, removing unnecessary and irrelevant information, commonly exported as Digital Imaging and Communications in Medicine (DICOM) images. This included target and adjacent structures, namely tumors and surrounding blood vessels, nerves, and other tissues; in addition to cortical and sub-cortical areas, such as the postcentral gyrus and corticospinal tract. In general, segmentation techniques involved thresholding, edge pixel detection, and region growing. Liao et al. (59) specifically developed a rapid, autostereoscopic segmentation method, using fuzzy connectedness for open MRI-guided glioma surgery.
3. Model generation
Three-dimensional modeling was used to convert segmentations to virtual objects, most frequently with 3D Slicer (7, 32%) and BrainLab (5, 23%) visualization software, based on DICOM images. To achieve this, tumor and cortical surface meshes, for instance, were exported as files suitable for 3D printing and computer-aided design (CAD), generally in stereolithrography (STL) file format. Unique to studies, Ghimire et al. (52) transformed positive motor responses, acquired from pre-operative nTMS, in 3D objects projected onto a tractography model of the corticospinal tract.
4. Registration and tracking
Registration was performed to format, align, and superimpose virtual objects—including drilling axis and cutting planes—onto patients' real anatomy. For studies using neuronavigation, this typically involved registering the patient to the system and co-registering the display device (e.g., surgical microscope) to determine the necessary transformation. In manual registration, virtual objects were scaled, translated, and/or rotated in relation to patients' head or brain, by the user, based on anatomical landmarks and fiducial markers. While this was the simplest approach, it was also the most time intensive and susceptible to human error, requiring continuous interaction between surgeons and technicians to update the AR scene. In automatic registration, landmarks and fiducials were often used as starting points with further processing and no user interaction. This approach was more expeditious, though relied on the quality of machine learning methods (i.e., training datasets). Registration also involved rigid, non-rigid, or hybrid surface interactions. Here, an assumption was made whether virtual and real objects—to be aligned—had the same shape, relating them by a single or multiple rigid transformations.
Given the variety and inconsistency in registration techniques, fused image overlay greatly varied across studies. Multiple terms were also used to express positional accuracy, or the estimate of error, as an indication of the system's ability to guide surgical targets. These terms included the target registration error (TRE), fiducial registration error (FRE), root-mean-squared error (RMSE), and target deviation (D). The TRE and FRE were the most widely calculated. Other factors contributing to variation comprised: geometric and optical distortions, such as incorrect tracking and display abnormalities; “swimming” effects, like bed movement and brain shift; and glioma presentation. Mascitelli et al. (63), for example, reported greater accuracy for superficial lesions compared to deep-seated ones (88.0% vs. 64.4%, p = .029). They also disabled HUDs in 59.6% of cases due to lack of use, distraction, and inaccuracy. Considering the aforementioned issues, several studies developed novel registration methods.
Fick et al. (50) designed a custom reference array, as an adjunct to HMDs, to correct initial registration for bed movements in GBM. While their technical workflow functioned as desired, and improved spatial understanding for surgeons, their registration accuracy was sub-optimal for clinical use (FRE = 8.55 mm). Relatedly, Archip et al. (46) evaluated a volumetric, non-rigid registration scheme to compensate for intra-operative brain shift. In 11 patients with eloquent supratentorial glioma, they revealed significant improvement in alignment accuracy compared to rigid-based, state-of-the-art technology (p < 0.001), with a mean residual displacement of D = 1.82 mm. Another study (67) leveraged a markerless spatial drift registration method to precisely align real and virtual objects. Pre-operatively, this aided surgeons in diagnosis and surgical planning; whereas intra-operatively, it helped them distinguish lesion boundaries and localize nerves, thereby increasing accurate resection of gliomas (RMSE = 1.86 mm). Liao et al. (59) similarly developed a spatial image registration method for integral videography image overlay, demonstrating satisfactory accuracy (TRE = 0.90 ± 0.21 mm). Nonetheless, there was high heterogeneity in registration methods and measurements, with no standard criteria for defining nor evaluating accuracy. Others failed to measure and/or report accuracy altogether (49, 52, 61–63, 65, 66).
5. Fused image overlay
Devices used to display virtual objects on the skull or dura of phantoms and patients included surgical microscopes (7, 32%), cameras (5, 23%), HMDs (3, 14%), tablets (2, 9%), smartphones (2, 9%), videos (1, 5%), and endoscopes (1, 5%). Of the studies utilizing surgical microscopes, two integrated AR via HUD (47, 63). Display device was unspecified for one study (46), which broadly referenced surgical instruments. See display devices by study design in Figure 5.
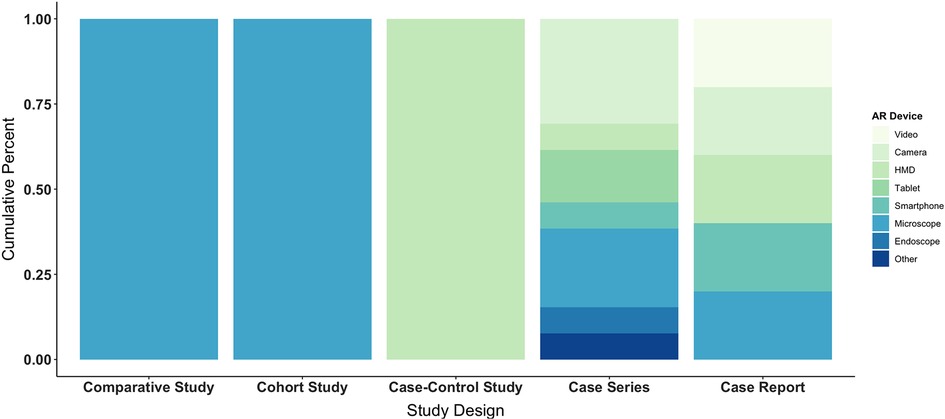
Figure 5. Types of augmented reality display devices used across study designs, including comparative, cohort, and case-control studies as well as case series and case reports. AR, augmented reality; HMD, head-mounted display.
Augmented reality applications
Iseki et al. (55) were among the first to evaluate AR in tumor surgery. An analysis of 42 patients with malignant gliomas, located in or adjacent to functional regions, showed markedly increased EOR (≥90%) when open MRI was simultaneously applied with real-time update navigation, which continuously refreshed intra-operative images. Using a similar concomitant method, Sun et al. (66) achieved complete resection in 69.6% of glioma patients (n = 79) compared to 36.4% of control patients (n = 55), with an average EOR of 95.2% ± 8.5% and 84.9% ± 15.7%, respectively (p < 0.01). The rates of post-operative recovery in motor, visual, and language function were also higher in the study group at two weeks and three months (p < 0.05). In both studies, intra-operative MRI disclosed and corrected for brain shift, providing surgeons with accurate and objective information as well as quality control during procedures.
A retrospective study (63) also showed potential for AR in intra-cranial surgery, detailing early experience with HUDs. For superficial and intra-axial lesions, HUD provided greater utility for skin incision, craniotomy, dural opening, and corticectomy, whereas the device was most useful for patient positioning and bone removal in those with skull base lesions. Although their sample with low-grade gliomas was small (n = 4), the authors postulated that HUD would be practical for guiding localization and resection, though more robust data was needed.
Recently, Luzzie et al. (62) tested the safety and efficacy of a new multimodal AR technique. They compared patients with supratentorial high-grade glioma undergoing AR HDFT-F-based cytoreductive surgery (n = 54) to a cohort of patients undergoing conventional white-light surgery assisted by infrared neuronavigation (n = 63). See Figure 6 for an illustrative case. Results showed higher EOR (p = 0.019), lower post-operative neurological deficits (p = 0.011), and longer progression-free survival (p = 0.006) in the study vs. control group. The EOR was specifically ≥98% in 85% of study cases. However, the types, grades, and percentages of complications in both groups were analogous (9.2% vs. 9.5%).
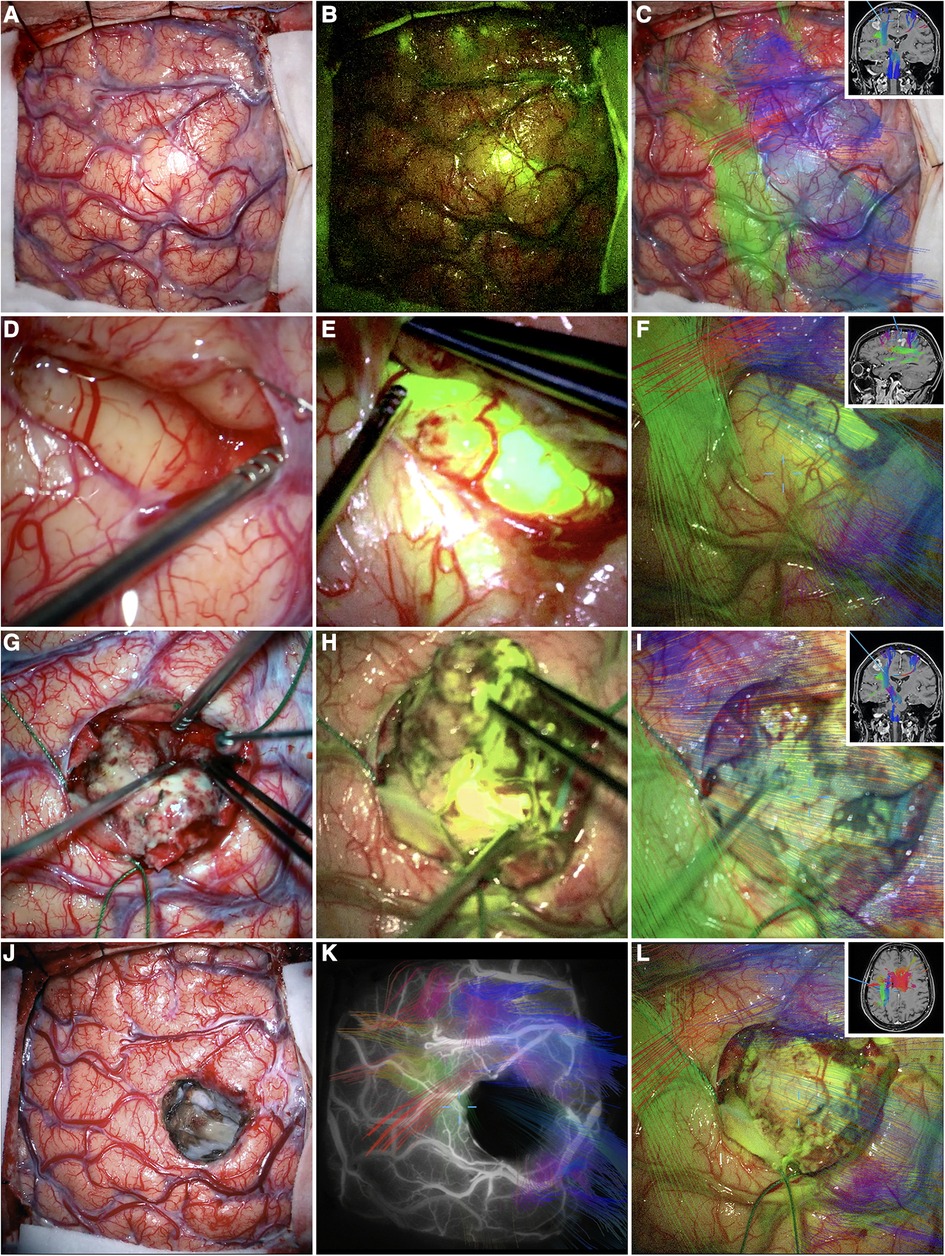
Figure 6. Illustrative case. Primary motor cortex glioblastoma of the dominant hemisphere. Intraoperative photographs obtained under white light (A), YELLOW 560 filter (B), and AR HDFT (C) before tumor resection. (D–I) The main steps of the surgery that were performed in large part along with the AR HDFT-F technique. (J–L) The surgical field at the end of the tumor resection obtained under white light (J), combined INFRARED 800 and AR HDFT during indocyanine green videoangiography (K), and AR HDFT-F (L). Insets in panels (C,F,I,L) are the screenshots obtained during the microscope focus-based neuronavigation. “Supratentorial High-Grade Gliomas: Maximal Safe Anatomical Resection Guided by Augmented Reality High-Definition Fiber Tractography and Fluorescein.” This figure is protected by Copyright, is owned by The Journal of Neurosurgery Publishing Group (JNSPG), and is used with permission only within this document. Permission to use it otherwise must be secured from JNSPG. Full text of the article containing the original figure is available at thejns.org.
This AR HDFT-F technique was further validated in the maximal safe resection of a postcentral gyrus GBM (61). Post-operatively, the patient reported significant improvement in upper extremity motor function and regained their ability to walk, with no recurrence at nine months follow-up. In a similar case, Inoue et al. (54) applied a newly developed AR neuronavigation system that superimposed tumors and vasculature plus motor tractography. This proved useful in visualizing the patient's lesion border and corticospinal tract, yet it had limitations in depth perception and accuracy. Their tumor resided at the corpus callosum inside the resection cavity as a result. However, no new neurological deficits were observed.
Two studies leveraged a tablet-based AR navigation system, called the “trans-visible navigator” (TVN). Satoh et al. (65) first applied this apparatus to stereotactic biopsy in three cases of deep-seated lesions, allowing surgeons to confirm target point accuracy and trajectory suitability, as well as precisely advance biopsy probes. This evaded the drawbacks of frame-based stereotactic navigation, resulting in satisfactory histopathology without complication. In a separate study (64), the TVN was applied in seven surgeries of low- and high-grade glioma, to which surgeons rated its utility across the neurosurgical workflow. Based on their findings, the apparatus was most practical for resecting superficial tumors, but less so for deep-seated ones, except when using transcortical and interhemispheric approaches. Results emphasized the importance of pre-operative discussions with surgeons in maximizing the effectiveness of AR.
Given the expense of modern navigation systems, two studies examined mobile AR (mAR) for localizing low- and high-grade glioma, as alternative low-cost solutions. Hou et al. (53) compared an iPhone-based method to a frameless neuronavigation system (n = 6), demonstrating technical feasibility with comparable accuracy (D ≤ 5 mm). Further, their device simplified image pre-processing, co-registration, and projection, all of which were completed under 10 min. Chen et al. (48) also examined mAR in the localization of a supratentorial glioma, leveraging the Sina Intraoperative Neurosurgical Assist app. Despite registration meriting improvement, their system was practical and reliable over standard neuronavigation (D = 4.4 ± 1.1 mm). Notably, both studies used manual registration in their AR workflows, likely increasing time and attenuating projection alignment, which the authors noted.
Other applications included intra-ventricular neuroendoscopy and intra-dural spinal surgery. Finger et al. (51) described their first experience with AR-guided neuroendoscopy among six patients with glioma in addition to one phantom model. By integrating pre-operative information into the endoscope's field of view, they were able to optimally place burr holes, estimate tumor location and surrounding structures, and apply trajectories for surgical intervention. Carl et al. (47), on the other hand, applied microscope-based AR via HUD in two patients with intradural spinal gliomas. In both study cases, AR provided intuitive visualization of tumor extent and neighboring structures, with high registration accuracy (TRE = 0.72 ± 0.24). This was particularly useful for visualizing the cranio-caudal extent of an intra-medullary ependymoma.
Mixed reality applications
Six studies applied mixed reality (MR), a blend of augmented reality (AR) and virtual reality (VR), wherein physical and virtual objects co-exist and interact in real time. Motivated by computer-aided surgery (CAS), De Mauro et al. (49) developed a prototypical MR system for pre-operative training (VR) and intra-operative use (AR) embedded in a surgical microscope. Their VR feature simulated visual and tactile sensations of brain palpation, with force feedback interaction of soft and hard tissues. Using real patient data, this allowed surgeons to distinguish between normal and pathological tissue affected by low-grade glioma. In contrast, their AR feature enabled stereoscopic visualization of relevant 3D data for real-time brain navigation. This was specifically designed to aid image-guided neurosurgery.
Zhou et al. (67) similarly evaluated a novel MR navigation system. Using a markerless spatial registration method, they tested its ability to diagnose and perform surgical planning pre-operatively, as well as identify lesion boundaries intra-operatively. Compared to standard applications, and under ideal conditions, their system met accuracy, efficacy, and reliability benchmarks along with time requirements. This was validated in both phantom experiments (n = 20; RMSE = 1.18 mm; Mtime = 6.02 min) and clinical trials (n = 16; RMSE = 1.86 mm; Mtime = 7.95 min) among patients with high-grade glioma, indicating suitability for clinical use.
Moreover, using MR projection mapping (MRPM), Koike et al. (58) developed an image-guided surgery system that projects intra-operative brain surface photographs (BSPs; real space) onto high-resolution 3D computer graphics (3DCGs; virtual space). See Figure 7. This was accomplished without the need for large-scale equipment, such as neuronavigation and other computer-assisted technologies. In 14 glioma patients, their system displayed accurate alignment of patient anatomy (BSPs) and medical images (3DCGs), presented in the same coordinate system, even after brain shift due to craniotomy (TRE = 1.19 ± 0.14 mm). Alignment accuracy was evaluated by two neurosurgeons, together under consultation, who measured the difference between BSPs and 3DCGs. This was performed after dividing craniotomy areas into 16 fields. Further, MRPM made it possible for surgeons to plan trajectories for intervention based on cortical stimulation mapping. No post-operative complications were observed.
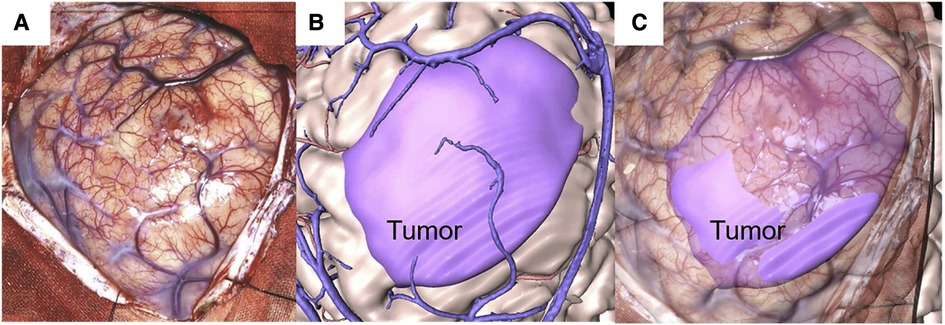
Figure 7. Illustrative case. A 31-year-old man with oligodendroglioma. (A) Intraoperative brain surface photograph in JPEG (Joint Photographic Experts Group) format. (B) Fusion 3-dimensional computer graphics (3DCG) created from preoperative imaging studies. The purple highlight indicates the tumor area. (C) Mixed-reality computer graphics created by aligning the intraoperative brain surface photograph and fusion 3DCG. The purple highlight indicates the tumor area. “Development of Innovative Neurosurgical Operation Support Method Using Mixed-Reality Computer Graphics.” © 2021 The Author(s). Published by Elsevier Inc. Licensed under CC-BY-NC-ND.
In a follow-up study (N = 15) (51, 52), recruiting patients with left hemispheric glioma, MRPM allowed surgeons to visualize the spatial correlation between medical images and the surgical field, specifically language-function hubs in the frontal lobe (TRE = 0.70 mm). This was likewise achieved despite brain shift at the time of the intra-operative BSP. Both studies leveraged a registration method previously developed and validated by Koike et al. (57), which demonstrated high spatial accuracy (TRE = 0.72 ± 0.04 mm).
In a retrospective, case-control study, Liu et al. (60) compared MR holographic imaging technology (n = 30) to ultrasound (n = 23) in neurosurgery for spinal cord glioma. Findings showed a significantly higher total tumor resection rate in the experimental group than in the control group (96.7%, vs. 82.6%; p < 0.05). This extended to more accurate complete resections (93.3%, vs. 73.5%; p < 0.05), far lower post-operative complications (3.3%, vs. 21.7%; p < 0.05), and improved recovery rates at 12 months follow-up (56.7%, vs. 41.1%; p < 0.05). Additionally, the tumor recurrence rate was lower in the experimental group compared to the control group at 12 months follow-up (0.0%, vs. 4.3%); however, this was not significant (p > 0.05). Enhanced MRI results were used to master the accuracy of intra-operative complete resections, informed by prior literature on AR and anatomic pathology (69). Post-operative MRI was then used to evaluate complete resections and their rate, as well the incidence of complications after surgery. Functional recovery was assessed via Modified McCormick Scale (MMS) grading.
Discussion
In this review, we summarized applications of AR in glioma surgery. Qualitatively, AR is a valuable tool that precisely overlays multiple imaging datasets, plus other relevant clinical information, onto the surgical field in real time. This obviates the need for surgeons to shift focus away from patients to nearby monitors for guidance, and mentally relate 2D information into 3D anatomy. Inattention blindness and interpretation error can therefore be mitigated. Further, AR enhances visualization of tumor complexity and its relationship to critical structures. This facilitates spatial understanding of neuroanatomy; aids surgeons in navigating, localizing, and resecting lesions; and may subsequently lead to improved patient outcomes.
However, at present, there is limited data that AR effectively extends the EOR and PFS as well as preserves motor, visual, and language function post-operatively. This is evidenced by the number, breadth, and quality of published studies. Of the 22 included in this review, 10 studies developed and tested AR systems, including segmentation and registration techniques, while the remaining 12 applied AR interventionally. Notably, 86% of studies were of low-level or weak evidence, based on hierarchical classes of evidence in neurosurgery (45), largely comprising case series and case reports. Accordingly, 77% of studies included 18 glioma patients or less and 73% lacked standard control groups. No studies involved random sampling nor random assignment.
Another critical limitation observed here, relevant to future research, was the inability to “double blind” patients and surgeons a key method for reducing detection bias. This problem underlies any technical advancement in surgery, namely trials of non-pharmacological treatments with physical components (70–73). As a result, surgeons who trusted in the efficacy of AR may have—unconsciously or deliberately—influenced the EOR. This might have led to an overestimation of treatment effects and perhaps more significant outcomes. Blinding of outcome assessors was additionally absent and/or unreported; yet it serves an important role in the case of soft endpoints, such as psychosocial function and quality of life. Still, this is not readily achieved (70, 72, 74). A possible solution is to apply the IDEAL Framework (75, 76), a paradigm for incorporating evidence-based advances in neurosurgery. Here, specific study designs and reporting standards are recommended across five stages of surgical innovation: Idea, Development, Exploration, Assessment, and Long-term study. A controlled, interrupted-time series design is one acceptable alternative, suggested by the IDEAL framework, for minimizing known bias (77).
From a technical standpoint, AR workflows considerably varied across studies. Diverse approaches were employed to acquire and segment images, model virtual objects, register and track systems, and display fused data intra-operatively. This variation extended to measurements, with several terms used to express registration error, or the accuracy of overlaid virtual objects on patients’ head and/or brain, each with its own shortcomings. The TRE, for instance, measures the anatomical region of interest for surgeons in 3D space, yet has poor depth perception. Other indices fail to correlate with (TRE) or underestimate (TE) true accuracy. This lack of standardization in measurement and reporting is well-documented in the field (78), and likely affects the validity of AR and its ability to guide localization and resection of intra-cranial targets, as well as the positioning of surgical instruments. Registration thus presents a significant and pressing challenge to precision neurosurgery. Along the same accord, most studies relied on software and hardware not formally vetted for pre- and/or intra-operative use. This chiefly applied to novel AR systems and techniques, which may lead to publication bias. As such, reported outcomes, at this stage, must be interpreted with caution.
One strategy towards standardization is founding a consortium of AR workflows in neurosurgery, including image acquisitions and open-source software, that could be utilized across research institutions. Compiling larger, more homogeneous, and longitudinal datasets may help identify universal methods; and allow for the development, validation, and use of machine learning algorithms (79)—and complimentary techniques (e.g., ultrasound 80, 81)—to maximize accuracy throughout procedures. This space also stands to benefit from a “readiness framework” to evaluate AR suitability for surgical implementation, drawing parallels to Tang et al.'s (82) analytic model. Their team specifically developed an evidence-based schema for assessing AR in medical education, underscoring four criteria: quality, application content, outcome, and feasibility. Adapting this model to AR in glioma surgery may address inconsistency in assessment tools and reliably gauge clinical utility. Mastering the use of AR in educational, training, and pre-operative settings will likewise increase its intra-operative value.
Limitations
This systematic review carries inherent limitations. First, no automated tools were employed during the selection and data collection process, increasing susceptibility to human error. In the event this occurred, studies returned by databases may have been overlooked, incorrectly excluded, and/or misreported in this review. Second, ascertaining the quality of evidence for each study was subjective, despite following hierarchical classes for neurosurgery. Studies may have been misclassified accordingly, especially those meeting criteria for more than one level. However, additional quality assurance measures, outlined in our methodology, were taken to ensure global data integrity. Finally, our qualitative analysis is limited by its data, which is heterogeneous at best. Standards for measurements and reporting will help improve the therapeutic value of AR in glioma surgery moving forward, and will enable meta-analytic approaches to precisely estimate both technical performance and treatment effects.
Conclusion
AR has increasing potential in the surgical management of glioma. It enables improved understanding of complex relationships between anatomy and pathology, aiding in real-time intra-operative navigation, localization, and resection. Further, there are signals of improvement in neurofunctional preservation associated with AR use, pointing to real, discernable benefit to patient care. This is evermore salient given the poor prognosis of gliomas, especially those with malignant and invasive presentations. However, technical and design limitations are readily apparent. A universal approach for developing, applying, and measuring AR systems, for instance, is critically absent. The field must consider the importance of consistency and replicability to effectively converge on standard uses of AR and its therapeutic value.
Data availability statement
The original contributions presented in the study are included in the article/Supplementary Material, further inquiries can be directed to the corresponding author.
Author contributions
A.R.: Conceptualization, Methodology, Investigation, Software, Formal Analysis, Visualization, Writing—Original Draft, Project Administration. C.L.: Writing—Review & Editing, Supervision. M.Z.: Investigation, Data Curation, Validation, Writing—Review & Editing. M.M.: Writing—Review & Editing, Supervision. V.C.: Data Curation, Validation, Writing—Original Draft. W.H.: Investigation, Data Curation, Writing—Review & Editing. N.B.: Investigation, Data Curation, Writing—Review & Editing. A.K.U.: Investigation, Data Curation, Software, Formal Analysis, Visualization, Writing—Review & Editing. L.A.: Data Curation, Validation, Writing—Review & Editing. Z.O.U.: Validation, Writing—Review & Editing. R.R.G.: Writing—Review & Editing, Supervision. J.S.: Writing—Review & Editing, Supervision.
Funding
All research at the Department of Psychiatry in the University of Cambridge was supported by the NIHR Cambridge Biomedical Research Centre (NIHR203312) and the NIHR Applied Research Collaboration East of England. The views expressed are those of the author(s) and not necessarily those of the NIHR or the Department of Health and Social Care.
Acknowledgments
We express our gratitude to the patients, clinicians, and researchers—described in this report—for their valuable contributions to science understanding the potential of augmented reality for glioma surgery.
Conflict of interest
The authors declare that the research was conducted in the absence of any commercial or financial relationships that could be construed as a potential conflict of interest.
Publisher's note
All claims expressed in this article are solely those of the authors and do not necessarily represent those of their affiliated organizations, or those of the publisher, the editors and the reviewers. Any product that may be evaluated in this article, or claim that may be made by its manufacturer, is not guaranteed or endorsed by the publisher.
References
1. American Association of Neurological Surgeon. Brain Tumors. Available at: https://www.aans.org/en/Patients/Neurosurgical-Conditions-and-Treatments/Brain-Tumors (Accessed August 19, 2021).
2. Ostrom QT, Bauchet L, Davis FG, Deltour I, Fisher JL, Langer CE, et al. The epidemiology of glioma in adults: a “state of the science” review. Neuro-Oncology. (2014) 16(7):896–913. doi: 10.1093/neuonc/nou087
3. Lin D, Wang M, Chen Y, Gong J, Chen L, Shi X, et al. Trends in intracranial glioma incidence and mortality in the United States, 1975–2018. Front Oncol. (2021) 11:748061. doi: 10.3389/fonc.2021.748061
4. Gerritsen JKW, Broekman MLD, De Vleeschouwer S, Schucht P, Nahed BV, Berger MS, et al. Safe surgery for glioblastoma: recent advances and modern challenges. Neurooncol Pract. (2022) 9(5):364–79. doi: 10.1093/nop/npac019
5. Rabab’h O, Al-Ramadan A, Shah J, Lopez-Negrete H, Gharaibeh A. Twenty years after glioblastoma multiforme diagnosis: a case of long-term survival. Cureus. (2021) 13(6). doi: 10.7759/cureus.16061
6. Lombardi G, Barresi V, Castellano A, Tabouret E, Pasqualetti F, Salvalaggio A, et al. Clinical management of diffuse low-grade gliomas. Cancers (Basel). (2020) 12(10):3008. doi: 10.3390/cancers12103008
7. Kiran M, Chatrath A, Tang X, Keenan DM, Dutta A. A prognostic signature for lower grade gliomas based on expression of long non-coding RNAs. Mol Neurobiol. (2019) 56(7):4786–98. doi: 10.1007/s12035-018-1416-y
8. Jakola AS, Skjulsvik AJ, Myrmel KS, Sjåvik K, Unsgård G, Torp SH, et al. Surgical resection versus watchful waiting in low-grade gliomas. Ann Oncol. (2017) 28(8):1942–8. doi: 10.1093/annonc/mdx230
9. Sanai N, Chang S, Berger MS. Low-grade gliomas in adults: a review. JNS. (2011) 115(5):948–65. doi: 10.3171/2011.7.JNS101238
10. Stupp R, Brada M, van den Bent MJ, Tonn JC, Pentheroudakis G. High-grade glioma: ESMO clinical practice guidelines for diagnosis, treatment and follow-up. Ann Oncol. (2014) 25:iii93–iii101. doi: 10.1093/annonc/mdu050
11. Witteler J, Kjaer TW, Tvilsted S, Schild SE, Rades D. Re-Evaluation of prognostic factors for survival after radiotherapy of cerebral gliomas: a supplementary analysis to a previous study. Anticancer Res. (2020) 40(11):6513–5. doi: 10.21873/anticanres.14674
12. Duffau H. Long-term outcomes after supratotal resection of diffuse low-grade gliomas: a consecutive series with 11-year follow-up. Acta Neurochir. (2016) 158(1):51–8. doi: 10.1007/s00701-015-2621-3
13. Hervey-Jumper SL, Berger MS. Maximizing safe resection of low- and high-grade glioma. J Neurooncol. (2016) 130(2):269–82. doi: 10.1007/s11060-016-2110-4
14. Rossi M, Ambrogi F, Gay L, Gallucci M, Conti Nibali M, Leonetti A, et al. Is supratotal resection achievable in low-grade gliomas? Feasibility, putative factors, safety, and functional outcome. J Neurosurg. (2020) 132(6):1692–705. doi: 10.3171/2019.2.JNS183408
15. Stummer W, van den Bent MJ, Westphal M. Cytoreductive surgery of glioblastoma as the key to successful adjuvant therapies: new arguments in an old discussion. Acta Neurochir. (2011) 153(6):1211–8. doi: 10.1007/s00701-011-1001-x
16. Pallud J, Varlet P, Devaux B, Geha S, Badoual M, Deroulers C, et al. Diffuse low-grade oligodendrogliomas extend beyond MRI-defined abnormalities. Neurology. (2010) 74(21):1724–31. doi: 10.1212/WNL.0b013e3181e04264
17. Giammalva GR, Brunasso L, Costanzo R, Paolini F, Umana GE, Scalia G, et al. Brain mapping-aided SupraTotal resection (SpTR) of brain tumors: the role of brain connectivity. Front Oncol. (2021) 11:645854. doi: 10.3389/fonc.2021.645854
18. Mandal AS, Romero-Garcia R, Hart MG, Suckling J. Genetic, cellular, and connectomic characterization of the brain regions commonly plagued by glioma. Brain. (2020) 143(11):3294–307. doi: 10.1093/brain/awaa277
19. Osswald M, Jung E, Sahm F, Solecki G, Venkataramani V, Blaes J, et al. Brain tumour cells interconnect to a functional and resistant network. Nature. (2015) 528(7580):93–8. doi: 10.1038/nature16071
20. Liang J, Lv X, Lu C, Ye X, Chen X, Fu J, et al. Prognostic factors of patients with gliomas—an analysis on 335 patients with glioblastoma and other forms of gliomas. BMC Cancer. (2020) 20(1):35. doi: 10.1186/s12885-019-6511-6
21. Gulati S, Jakola AS, Nerland US, Weber C, Solheim O. The risk of getting worse: surgically acquired deficits, perioperative complications, and functional outcomes after primary resection of glioblastoma. World Neurosurg. (2011) 76(6):572–9. doi: 10.1016/j.wneu.2011.06.014
22. Ius T, Angelini E, Thiebaut de Schotten M, Mandonnet E, Duffau H. Evidence for potentials and limitations of brain plasticity using an atlas of functional resectability of WHO grade II gliomas: towards a “minimal common brain.”. NeuroImage. (2011) 56(3):992–1000. doi: 10.1016/j.neuroimage.2011.03.022
23. De Benedictis A, Duffau H, Paradiso B, Grandi E, Balbi S, Granieri E, et al. Anatomo-functional study of the temporo-parieto-occipital region: dissection, tractographic and brain mapping evidence from a neurosurgical perspective. J Anat. (2014) 225(2):132–51. doi: 10.1111/joa.12204
24. Sarubbo S, De Benedictis A, Merler S, Mandonnet E, Balbi S, Granieri E, et al. Towards a functional atlas of human white matter: functional atlas of white matter. Hum Brain Mapp. (2015) 36(8):3117–36. doi: 10.1002/hbm.22832
25. Fults DW. Commentary: occurrence, risk factors, and consequences of postoperative ischemia after glioma resection: a retrospective study. Neurosurgery. (2023) 92(1):e5–6. doi: 10.1227/neu.0000000000002173
26. Mandonnet E, Duffau H. An attempt to conceptualize the individual onco-functional balance: why a standardized treatment is an illusion for diffuse low-grade glioma patients. Crit Rev Oncol Hematol. (2018) 122:83–91. doi: 10.1016/j.critrevonc.2017.12.008
27. Roberts DW, Strohbehn JW, Hatch JF, Murray W, Kettenberger H. A frameless stereotaxic integration of computerized tomographic imaging and the operating microscope. J Neurosurg. (1986) 65(4):545–9. doi: 10.3171/jns.1986.65.4.0545
28. Chidambaram S, Stifano V, Demetres M, Teyssandier M, Palumbo MC, Redaelli A, et al. Applications of augmented reality in the neurosurgical operating room: a systematic review of the literature. J Clin Neurosci. (2021) 91:43–61. doi: 10.1016/j.jocn.2021.06.032
29. Cho J, Rahimpour S, Cutler A, Goodwin CR, Lad SP, Codd P. Enhancing reality: a systematic review of augmented reality in neuronavigation and education. World Neurosurg. (2020) 139:186–95. doi: 10.1016/j.wneu.2020.04.043
30. Léger É, Drouin S, Collins DL, Popa T, Kersten-Oertel M. Quantifying attention shifts in augmented reality image-guided neurosurgery. Healthc Technol Lett. (2017) 4(5):188–92. doi: 10.1049/htl.2017.0062
31. Haouchine N, Juvekar P, Wells WM III, Cotin S, Golby A, Frisken S, et al. Deformation aware augmented reality for craniotomy using 3D/2D non-rigid registration of cortical vessels. In: Martel AL, Abolmaesumi P, Stoyanov D, editors. Medical image computing and computer assisted intervention—mICCAI 2020. Vol. 12264. Lecture Notes in Computer Science. Lima: Springer International Publishing (2020). p. 735–44. doi: 10.1007/978-3-030-59719-1_71
32. Haouchine N, Juvekar P, Nercessian M, Wells W, Golby A, Frisken S. Pose estimation and non-rigid registration for augmented reality during neurosurgery. IEEE Trans Biomed Eng. (2022) 69(4):1310–7. doi: 10.1109/TBME.2021.3113841
33. Bayer S, Maier A, Ostermeier M, Fahrig R. Intraoperative imaging modalities and compensation for brain shift in tumor resection surgery. Int J Biomed Imaging. (2017) 2017:1–18. doi: 10.1155/2017/6028645
34. Gerard IJ, Kersten-Oertel M, Petrecca K, Sirhan D, Hall JA, Collins DL. Brain shift in neuronavigation of brain tumors: a review. Med Image Anal. (2017) 35:403–20. doi: 10.1016/j.media.2016.08.007
35. Orringer DA, Golby A, Jolesz F. Neuronavigation in the surgical management of brain tumors: current and future trends. Expert Rev Med Devices. (2012) 9(5):491–500. doi: 10.1586/erd.12.42
36. Furht B, editor. Handbook of augmented reality. New York: Springer (2011). doi: 10.1007/978-1-4614-0064-6
37. Contreras López WO, Navarro PA, Crispin S. Intraoperative clinical application of augmented reality in neurosurgery: a systematic review. Clin Neurol Neurosurg. (2019) 177:6–11. doi: 10.1016/j.clineuro.2018.11.018
38. Guha D, Alotaibi NM, Nguyen N, Gupta S, McFaul C, Yang VXD. Augmented reality in neurosurgery: a review of current concepts and emerging applications. Can J Neurol Sci. (2017) 44(3):235–45. doi: 10.1017/cjn.2016.443
39. Lee C, Wong GKC. Virtual reality and augmented reality in the management of intracranial tumors: a review. J Clin Neurosci. (2019) 62:14–20. doi: 10.1016/j.jocn.2018.12.036
40. Meola A, Cutolo F, Carbone M, Cagnazzo F, Ferrari M, Ferrari V. Augmented reality in neurosurgery: a systematic review. Neurosurg Rev. (2017) 40(4):537–48. doi: 10.1007/s10143-016-0732-9
41. Page MJ, McKenzie JE, Bossuyt PM, Boutron I, Hoffmann TC, Mulrow CD, et al. The PRISMA 2020 statement: an updated guideline for reporting systematic reviews. Syst Rev. (2021) 10(1):89. doi: 10.1186/s13643-021-01626-4
42. Foster ED, Deardorff A. Open science framework (OSF). JMLA. (2017) 105(2). doi: 10.5195/jmla.2017.88
43. Amir-Behghadami M, Janati A. Population, intervention, comparison, outcomes and study (PICOS) design as a framework to formulate eligibility criteria in systematic reviews. Emerg Med J. (2020) 37(6):387. doi: 10.1136/emermed-2020-209567
44. R: A Language and Environment for Statistical Computing. (2018). Available at: https://www.R-project.org/
45. Rutka JT. Editorial. Classes of evidence in neurosurgery. JNS. (2016) 126(6):1747–8. doi: 10.3171/2016.4.JNS161067
46. Archip N, Clatz O, Whalen S, Kacher D, Fedorov A, Kot A, et al. Non-rigid alignment of pre-operative MRI, fMRI, and DT-MRI with intra-operative MRI for enhanced visualization and navigation in image-guided neurosurgery. NeuroImage. (2007) 35(2):609–24. doi: 10.1016/j.neuroimage.2006.11.060
47. Carl B, Bopp M, Saß B, Pojskic M, Nimsky C. Augmented reality in intradural spinal tumor surgery. Acta Neurochir. (2019) 161(10):2181–93. doi: 10.1007/s00701-019-04005-0
48. Chen JG, Han KW, Zhang DF, Li ZX, Li YM, Hou LJ. Presurgical planning for supratentorial lesions with free slicer software and sina app. World Neurosurg. (2017) 106:193–7. doi: 10.1016/j.wneu.2017.06.146
49. De Mauro A, Raczkowsky J, Halatsch ME, Wörn H. Mixed reality neurosurgical microscope for training and intra-operative purposes. In: Shumaker R, editor. Virtual and mixed reality. Vol. 5622. Lecture Notes in Computer Science. San Diego, CA: Springer Berlin Heidelberg (2009). p. 542–9. doi: 10.1007/978-3-642-02771-0_60
50. Fick T, van Doormaal JAM, Hoving EW, Regli L, van Doormaal TPC. Holographic patient tracking after bed movement for augmented reality neuronavigation using a head-mounted display. Acta Neurochir. (2021) 163(4):879–84. doi: 10.1007/s00701-021-04707-4
51. Finger T, Schaumann A, Schulz M, Thomale UW. Augmented reality in intraventricular neuroendoscopy. Acta Neurochir. (2017) 159(6):1033–41. doi: 10.1007/s00701-017-3152-x
52. Ghimire P, Lavrador JP, Baig Mirza A, Pereira N, Keeble H, Borri M, et al. Intraoperative mapping of pre-central motor cortex and subcortex: a proposal for supplemental cortical and novel subcortical maps to Penfield’s motor homunculus. Brain Struct Funct. (2021) 226(5):1601–11. doi: 10.1007/s00429-021-02274-z
53. Hou Y, Ma L, Zhu R, Chen X, Zhang J. A low-cost iPhone-assisted augmented reality solution for the localization of intracranial lesions. PLoS ONE. (2016) 11(7):e0159185. doi: 10.1371/journal.pone.0159185
54. Inoue D, Cho B, Mori M, Kikkawa Y, Amano T, Nakamizo A, et al. Preliminary study on the clinical application of augmented reality neuronavigation. J Neurol Surg A Cent Eur Neurosurg. (2013) 74(2):071–6. doi: 10.1055/s-0032-1333415
55. Iseki H, Muragaki Y, Naemura K, Hayashi M, Hori T, Takakura K. Clinical application of augmented reality in neurosurgical field. Proceedings Computer Graphics International 2003. IEEE Comput. Soc (2003). p. 44–9. doi: 10.1109/CGI.2003.1214446
56. Koike T, Tanaka S, Kin T, Suzuki Y, Takayanagi S, Takami H, et al. Accurate preoperative identification of motor speech area as termination of arcuate Fasciculus depicted by Q-ball imaging tractography. World Neurosurg. (2022) 164:e764–71. doi: 10.1016/j.wneu.2022.05.041
57. Koike T, Kin T, Tanaka S, Takeda Y, Uchikawa H, Shiode T, et al. Development of innovative neurosurgical operation support method using mixed-reality computer graphics. World Neurosurgery: X. (2021) 11:100102. doi: 10.1016/j.wnsx.2021.100102
58. Koike T, Kin T, Tanaka S, Sato K, Uchida T, Takeda Y, et al. Development of a new image-guided neuronavigation system: mixed-reality projection mapping is accurate and feasible. Oper Neurosurg. (2021) 21(6):549–57. doi: 10.1093/ons/opab353
59. Liao H, Inomata T, Sakuma I, Dohi T. Surgical navigation of integral videography image overlay for open MRI-guided glioma surgery. In: Yang GZ, Jiang T, Shen D, Gu L, Yang J, editors. Medical imaging and augmented reality. Vol. 4091. Lecture Notes in Computer Science. Shanghai: Springer Berlin Heidelberg (2006). p. 187–94. doi: 10.1007/11812715_24
60. Liu Z, Li X, Lu J. Application evaluation of mixed-reality holographic imaging technology in the surgical treatment of spinal cord glioma. Pak J Med Sci. (2022) 38(7):1958. doi: 10.12669/pjms.38.7.4777
61. Luzzi S, Giotta Lucifero A, Baldoncini M, Del Maestro M, Galzio R. Postcentral gyrus high-grade glioma: maximal safe anatomic resection guided by augmented reality with fiber tractography and fluorescein. World Neurosurg. (2022) 159:108. doi: 10.1016/j.wneu.2021.12.072
62. Luzzi S, Giotta Lucifero A, Martinelli A, Maestro MD, Savioli G, Simoncelli A, et al. Supratentorial high-grade gliomas: maximal safe anatomical resection guided by augmented reality high-definition fiber tractography and fluorescein. Neurosurg Focus. (2021) 51(2):E5. doi: 10.3171/2021.5.FOCUS21185
63. Mascitelli JR, Schlachter L, Chartrain AG, Oemke H, Gilligan J, Costa AB, et al. Navigation-linked heads-up display in intracranial surgery: early experience. Operative Surg. (2018) 15(2):184–93. doi: 10.1093/ons/opx205
64. Satoh M, Nakajima T, Yamaguchi T, Watanabe E, Kawai K. Evaluation of augmented-reality based navigation for brain tumor surgery. J Clin Neurosci. (2021) 94:305–14. doi: 10.1016/j.jocn.2021.10.033
65. Satoh M, Nakajima T, Yamaguchi T, Watanabe E, Kawai K. Application of augmented reality to stereotactic biopsy. Neurol Med Chir(Tokyo). (2019) 59(11):444–7. doi: 10.2176/nmc.tn.2019-0128
66. Chen JG, Han KW, Zhang DF, Li ZX, Li YM, Hou LJ. Impact of virtual and augmented reality based on intraoperative magnetic resonance imaging and functional neuronavigation in glioma surgery involving eloquent areas. World Neurosurg. (2016) 96:375–82. doi: 10.1016/j.wneu.2016.07.107
67. Zhou Z, Yang Z, Jiang S, Zhuo J, Zhu T, Ma S. Augmented reality surgical navigation system based on the spatial drift compensation method for glioma resection surgery. Med Phys. (2022) 49(6):mp.15650. doi: 10.1002/mp.15650
68. Aschke M, Wirtz CR, Raczkowsky J, Worn H, Kunze S. Augmented reality in operating microscopes for neurosurgical interventions. First international IEEE EMBS conference on neural engineering, 2003. Conference proceedings. IEEE (2003). p. 652–5. doi: 10.1109/CNE.2003.1196913
69. Hanna MG, Ahmed I, Nine J, Prajapati S, Pantanowitz L. Augmented reality technology using microsoft HoloLens in anatomic pathology. Arch Pathol Lab Med. (2018) 142(5):638–44. doi: 10.5858/arpa.2017-0189-OA
70. Boutron I, Tubach F, Giraudeau B, Ravaud P. Blinding was judged more difficult to achieve and maintain in nonpharmacologic than pharmacologic trials. J Clin Epidemiol. (2004) 57(6):543–50. doi: 10.1016/j.jclinepi.2003.12.010
71. Probst P, Zaschke S, Heger P, Harnoss JC, Hüttner FJ, Mihaljevic AL, et al. Evidence-based recommendations for blinding in surgical trials. Langenbecks Arch Surg. (2019) 404(3):273–84. doi: 10.1007/s00423-019-01761-6
72. Solomon MJ, McLeod RS. Should we be performing more randomized controlled trials evaluating surgical operations? Surgery. (1995) 118(3):459–67. doi: 10.1016/S0039-6060(05)80359-9
73. Takroni R, Sharma S, Reddy K, Zagzoog N, Aljoghaiman M, Alotaibi M, et al. Randomized controlled trials in neurosurgery. Surg Neurol Int. (2022) 13:379. doi: 10.25259/SNI_1032_2021
74. Probst P, Grummich K, Harnoss JC, Hüttner FJ, Jensen K, Braun S, et al. Placebo-controlled trials in surgery: a systematic review and meta-analysis. Medicine (Baltimore). (2016) 95(17):e3516. doi: 10.1097/MD.0000000000003516
75. Barkun JS, Aronson JK, Feldman LS, Maddern GJ, Strasberg SM. Evaluation and stages of surgical innovations. Lancet. (2009) 374(9695):1089–96. doi: 10.1016/S0140-6736(09)61083-7
76. McCulloch P, Altman DG, Campbell WB, Flum DR, Glasziou P, Marshall JC, et al. No surgical innovation without evaluation: the IDEAL recommendations. Lancet. (2009) 374(9695):1105–12. doi: 10.1016/S0140-6736(09)61116-8
77. Hirst A, Philippou Y, Blazeby J, Campbell B, Campbell M, Feinberg J, et al. No surgical innovation without evaluation: evolution and further development of the IDEAL framework and recommendations. Ann Surg. (2019) 269(2):211–20. doi: 10.1097/SLA.0000000000002794
78. Fick T, van Doormaal JAM, Hoving EW, Willems PWA, van Doormaal TPC. Current accuracy of augmented reality neuronavigation systems: systematic review and meta-analysis. World Neurosurg. (2021) 146:179–88. doi: 10.1016/j.wneu.2020.11.029
79. Tonutti M, Gras G, Yang GZ. A machine learning approach for real-time modelling of tissue deformation in image-guided neurosurgery. Artif Intell Med. (2017) 80:39–47. doi: 10.1016/j.artmed.2017.07.004
80. Gerard IJ, Kersten-Oertel M, Drouin S, Hall JA, Petrecca K, De Nigris D, et al. Combining intraoperative ultrasound brain shift correction and augmented reality visualizations: a pilot study of eight cases. J Med Imag. (2018) 5(2):1. doi: 10.1117/1.JMI.5.2.021210
81. Gerard IJ, Kersten-Oertel M, Hall JA, Sirhan D, Collins DL. Brain shift in neuronavigation of brain tumors: an updated review of intra-operative ultrasound applications. Front Oncol. (2021) 10:618837. doi: 10.3389/fonc.2020.618837
Keywords: augmented reality, brain tumor, glioma, mixed reality, neuronavigation, neurosurgery, systematic review, virtual reality
Citation: Ragnhildstveit A, Li C, Zimmerman MH, Mamalakis M, Curry VN, Holle W, Baig N, Uğuralp AK, Alkhani L, Oğuz-Uğuralp Z, Romero-Garcia R and Suckling J (2023) Intra-operative applications of augmented reality in glioma surgery: a systematic review. Front. Surg. 10:1245851. doi: 10.3389/fsurg.2023.1245851
Received: 23 June 2023; Accepted: 4 August 2023;
Published: 21 August 2023.
Edited by:
Mohammad Mofatteh, Queen’s University Belfast, United KingdomReviewed by:
Mark Ter Laan, Radboud University Medical Centre, NetherlandsSabino Luzzi, University of Pavia, Italy
© 2023 Ragnhildstveit, Li, Zimmerman, Mamalakis, Curry, Holle, Baig, Uğuralp, Alkhani, Oğuz-Uğuralp, Romero-Garcia and Suckling. This is an open-access article distributed under the terms of the Creative Commons Attribution License (CC BY). The use, distribution or reproduction in other forums is permitted, provided the original author(s) and the copyright owner(s) are credited and that the original publication in this journal is cited, in accordance with accepted academic practice. No use, distribution or reproduction is permitted which does not comply with these terms.
*Correspondence: Anya Ragnhildstveit YW1yMjEwQGNhbS5hYy51aw==
†These authors have contributed equally to this work and share senior authorship