Application possibilities of artificial intelligence in facial vascularized composite allotransplantation—a narrative review
- 1Department of Plastic, Hand- and Reconstructive Surgery, University Hospital Regensburg, Regensburg, Germany
- 2Division of Plastic Surgery, Department of Surgery, Yale New Haven Hospital, Yale School of Medicine, New Haven, CT, United States
- 3Department of Oral and Maxillofacial Surgery, University Hospital Regensburg, Regensburg, Germany
- 4Craniologicum, Center for Cranio-Maxillo-Facial Surgery, Bern, Switzerland
- 5Faculty of Medicine, University of Bern, Bern, Switzerland
- 6Division of Hand, Plastic and Aesthetic Surgery, Ludwig-Maximilians University Munich, Munich, Germany
Facial vascularized composite allotransplantation (FVCA) is an emerging field of reconstructive surgery that represents a dogmatic shift in the surgical treatment of patients with severe facial disfigurements. While conventional reconstructive strategies were previously considered the goldstandard for patients with devastating facial trauma, FVCA has demonstrated promising short- and long-term outcomes. Yet, there remain several obstacles that complicate the integration of FVCA procedures into the standard workflow for facial trauma patients. Artificial intelligence (AI) has been shown to provide targeted and resource-effective solutions for persisting clinical challenges in various specialties. However, there is a paucity of studies elucidating the combination of FVCA and AI to overcome such hurdles. Here, we delineate the application possibilities of AI in the field of FVCA and discuss the use of AI technology for FVCA outcome simulation, diagnosis and prediction of rejection episodes, and malignancy screening. This line of research may serve as a fundament for future studies linking these two revolutionary biotechnologies.
Introduction
Facial vascularized composite allotransplantation (FVCA) is a surgical procedure that involves transplanting composite tissue to patients with devastating and irreversible (mid-)facial defects (1–4). FVCA transplants are comprised of different tissue types such as skin, muscle, bone, nerves, and blood vessels (5). This approach represents a novel surgical therapy for complex clinical conditions (e.g., extensive burn or gunshot wounds) that cannot be effectively addressed through conventional reconstructive techniques (6). As of April 2023, there have been at least 47 FVCA worldwide with promising short- and longer-term outcomes (4, 7). Yet, FVCA research still needs to overcome different hurdles to further enhance postoperative results and increase the feasibility and accessibility of FVCA procedures. Such hurdles range from individualized preoperative planning to life-long immunosuppressive drug regimens that can increase the risk of malignancies (1, 8).
While various basic science and translational projects are underway to solve these issues, there is a scarcity of studies elucidating the integration of artificial intelligence (AI) into the field of FVCA. AI refers to computer systems that can learn, infer, synthesize, and perceive information similar to human cognition. AI is commonly established on large data sets that serve as training models to produce mathematical and computational programs; these computational designs are often based on machine learning (ML) and its subsets including deep learning (DL), and neural networks, among other computational methods (Figure 1). More importantly, AI has recently seen a rapid expansion in capabilities as computational power has exponentially increased in the past decade; however, these advancements have yet to translate into the field of FVCA.
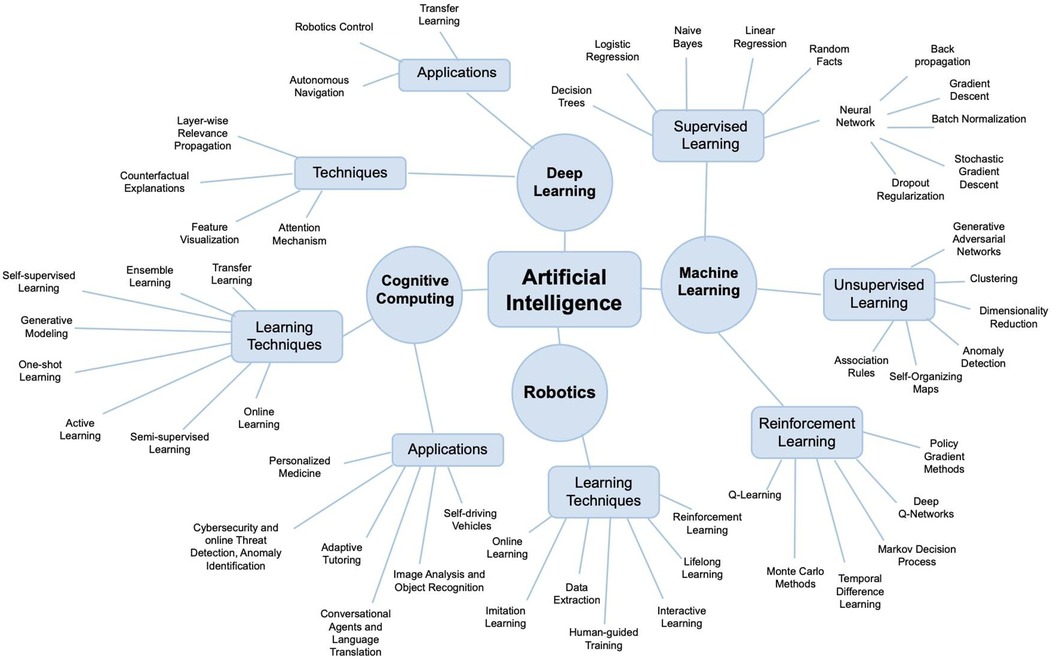
Figure 1. Artificial intelligence (AI) is an umbrella term for various computational strategies including machine learning, robotics, or cognitive computing. Note that, there is no scientific consensus on how to subcategorize AI and classifications may vary between different authors.
Herein, we aim to shed light on the application possibilities of AI systems in FVCA and discuss future research directions. This scoping review may provide a fundament to leverage the innovation of FVCA and the limitless learning capacities of AI.
AI-Aided outcome simulation
Over the past decades, preoperative surgical outcome simulation has become a vital component of comprehensive perioperative patient management (9). Preoperative outcome simulation encompasses visual and statistical prognoses of surgical procedures. Currently, the combination of patient photo series, mathematical calculation models, in-person consultation, and the surgeon's clinical experience still represent the goldstandard to provide patients with realistic outcome predictions (10, 11). Yet, AI-aided simulations have been demonstrated to automatically produce precise prognoses for various procedures in plastic and reconstructive surgery (9, 12–14).
A 2020 study by Bashiri-Bawil et al. trained an AI-based computer model to visualize postoperative rhinoplasty results using 400 patient images (frontal and side view) and 87 facial landmarks. In a testing set of 20 patients, this approach resulted in an accuracy level of >80% (15). Similarly, Bottino et al. utilized 125 face profile images to train an AI algorithm on rhinoplasties. Besides possible outcome visualization, this computer model also suggested a best-matching face profile based on the patient's individual facial characteristics. This automated, yet personalized approach yielded positive qualitative results with a quantitative assessment of postoperative attractiveness improvement still pending (16). A recent study by Mussi et al. introduced an AI-aided strategy for the preoperative simulation of autologous ear reconstruction procedures. The authors proposed a semi-automated workflowvthat integrated AI-based strategies to provide advanced design and customization techniques for this type of surgery. The authors further underscored that such easy-to-use tools may allow for streamlining the production process and reducing overall treatment costs (17). Persing et al. used the 3D VECTRA [Canfield, Fairfield, NJ; a 3D photosystem based on visual assessment management software (VAM)] to compare AI-generated to actual rhinoplasty outcomes. To this end, the authors enrolled 40 patients and concluded that this simulation technique is a powerful planning tool for rhinoplasty candidates (18).
However, such concepts cannot be directly transferred to FVCA, since preoperative outcome simulation needs to integrate the donor's and patient's facial features at the same time. Subsequently, reliable predictions can only be made once the donor is identified. Automated facial landmark recognition systems such as Emotrics (a ML algorithm that automatically localizes 68 facial landmarks) or 3D VECTRA could be used for quick and precise facial measurements (19, 20). Following facial feature recognition (e.g., nasal dorsal humps, prominent cheekbones) and exact skin color assessment, DL models [e.g., convolutional neural networks (CCN)] or VAM-based systems could generate postoperative FVCA outcome simulation and support optimal skin color matching. Of note, novel AI-based models can predict surgical outcomes based on limited datasets (i.e., less than ten observations per predictor variable) reducing the time from donor identification to outcome simulation (21). AI-aided outcome prediction may play a vital role in managing patients' expectations given that the number of FVCA performed worldwide is still limited with large interpatient variabilities. For example, there has been only one case of a Black FVCA patient (4). Previous outcome images, therefore, provide little decision support for the recipient's preoperative informed consent. Yet, future studies are warranted to establish an efficient workflow starting with donor identification over facial feature recognition to outcome simulation.
AI-Aided intraoperative image-guidance
The potential advantages of AI are substantial, particularly for intricate procedures such as FVCA. The degree to which preoperative planning can be detailed and meticulously executed largely predicts the potential success of the outcome.
Precision and real-time feedback are essential pillars of contemporary surgical practice. These elements are considerably amplified with the integration of AI into intraoperative image-guidance systems (22). These advanced systems have the capability to discern minute details that may escape the human eye or rare anatomical variations (23). For instance, den Boer et al. proposed a DL algorithm to identify anatomical structures (e.g., aorta, right lung) based on 83 video sequences. They calculated accuracy levels of up to 90% underscoring the potential clinical applicability (24). Hashimoto et al. programmed an artificial neural network to automatically detect the cystic duct and artery yielding an accuracy of 95% (25). Such DL algorithms for landmark identification may help preserve critical anatomical structures such as the facial nerve, ultimately improving transplantation outcomes.
During FVCA, the precise fitting of the donor tissue onto the recipient's facial structure is paramount for the success of the procedure (26, 27). AI software can analyze intraoperative images in real-time, providing surgeons with detailed instructions and assisting in for example the precise positioning (22, 23). Moreover, the precision offered by AI extends beyond simple visual analysis. AI systems are capable of predicting potential risks and outcomes with increased accuracy based on patient history and health records. For instance, Formeister et al. enrolled 364 patients undergoing head and neck free tissue transfer to predict surgical complications. To this end, they trained a supervised ML algorithm to prognosticate surgical complications using 14 clinicopathologic patient characteristics (e.g., age, smoking pack-years). The ML algorithm yielded a prediction accuracy of 75% (28). Further, a meta-analysis including 13 studies concluded that CNN are sensitive and specific tools to capture intraoperative adverse events (e.g., bleeding, perfusion deficiencies) (29). These features aid surgeons in making precise and informed decisions during critical phases of the operation.
Real-time feedback plays a significant role during surgery, a role that might be efficiently fulfilled by AI-integrated intraoperative imaging systems. These systems provide surgeons with real-time data, enabling immediate adjustments to their surgical approach, which can prove invaluable during complex procedures like FVCA (30). More precisely, Nespolo et al. enrolled ten phacoemulsification cataract surgery patients and trained a deep neural network (DNN) based on the intraoperative video sequences. The DNN showed an area under the curve of up to 0.99 for identifying different surgical procedure steps such as capsulorhexis and phacoemulsification with a processing speed of 97 images per second (31). Studier-Fischer et al. trained a DNN on 9,059 hyperspectral images from 46 pigs to test its intraoperative tissue detection accuracy. The authors calculated accuracy levels of 95% when automatically differentiating 20 organ and tissue types (32). In another study of nine thyroidectomy patients, Maktabi et al. programmed a supervised ML classification algorithm to automatically discriminate the parathyroid, the thyroid, and the recurrent laryngeal nerve from surrounding tissue and surgical instruments. The mean accuracy was 68% ± 23% with an image processing time of 1.4 s (33). However, such intraoperative approaches should still be considered add-ons to the surgeon's real-time feedback rather than making hands-on experience obsolete.
AI-Aided diagnosis and prediction of rejection episodes
Despite distinct advancements in the perioperative patient management including more patient-specific risk profiles, novel biomarkers, and targeted immunosuppressants, allograft rejection persists as a major burden in transplant surgery still affecting more than 15% of solid organ transplant (SOT) patients (34, 35). More precisely, acute rejection reactions occur in approximately 30% of liver, 40% of heart, and 33% of renal transplant recipients resulting in increased mortality rates, delayed graft function, and poor long-term outcomes (36–38). While tissue histopathology assessment represents the goldstandard for graft rejection diagnosis, it poses the downsides of low reproducibility and high inter-observer variability and has been shown to be inefficient for assessing graft steatosis, (i.e., a clinical parameter for predicting post-transplant graft function) (39, 40).
In SOT, ML and DNN (especially CNN) have been successfully investigated to identify risk patients for transplant rejection. Thongprayoon et al. performed ML consensus cluster analysis for 22,687 Black kidney transplant recipients identifying four distinct clusters. Interestingly, the authors found that the risk for transplant rejection was significantly increased in highly sensitized recipients of deceased donor kidney retransplants and young recipients with hypertension (41). In liver transplantation, Zare et al. programmed a feed-forward neural network (i.e., a type of artificial neural network where information flows in one direction from input to output) based on laboratory values and clinical data from 148 recipients. The network outperformed conventional logistic regression models in predicting the risk of acute rejection seven days after transplant, with 87% sensitivity, 90% specificity, and 90% accuracy and correctly determined eight out of ten acute rejection patients and 34 out of 36 non-rejection ones in the testing set (42). The combination of different AI methods (i.e., ML and DNN) and microarray analysis also have been deployed to detect molecular signatures correlating with rejection patterns in lung transplant transbronchial biopsies and heart transplant recipients. Given the patterns' complexity, such prediction pathways only have become available since the advent of AI and the ability to make sense of enormous data set (43–45). Further, CNN have been demonstrated to facilitate the diagnostic workflow for transplant rejection. Abdeltawab et al. merged data from 56 patients (e.g., diffusion-weighted MRI images and clinical biomarkers such as creatinine clearance and serum plasma creatinine) to predict kidney graft rejection with an accuracy of 93%, sensitivity of 93%, and specificity of 92% (46).
Across the different vascularized composite allograft (VCA) surgery subtypes, 85% of VCA patients experience at least one rejection episode one year post transplantation with more than 50% of cases reporting multiple rejection episodes (47). Such episodes can lead to irreversible loss of graft function and represent a major hurdle for integrating FVCA surgery into routine clinical care (48). Current diagnosis protocols recommend a prompt biopsy and histologic examination in case of suspicious cutaneous changes. For histologically confirmed rejection reactions, immediate steroid bolus administration and/or other immunosuppressants are commonly administered (49). Besides a more standardized method for prediction and diagnosis of FVCA rejection, AI models could allow for non-invasive graft examination based on distinct macroscopic changes typical of transplant rejection (e.g., a maculopapular erythematous rash of varying color intensities) (50–52). For instance, a CNN classifier could be trained based on FVCA patient images to detect rejection episodes. Skin photographs of patients with transplant rejection compared to healthy individuals may serve as input data (48, 53, 54). Alternatively, patient mucosa photographs could be utilized for training purposes since the mucosal tissue was recently identified as one of the primary target sites for rejection episodes by Kauke-Navarro et al. (2, 55). Given the limited number of FVCA procedures, researchers may integrate data augmentation techniques such as flipping (i.e., horizontally or vertically mirroring an image or data point), shearing (i.e., distorting the image by tilting it in a specific direction), or color channel shifting (i.e., altering the intensity of individual color channels in the image) (56, 57). Further, computer vision (CV) could be used to extract visual data from patient images or videos. Following image classification, detection, and recognition, CV may autonomously analyze and interpret signs of VCA rejection (58, 59). Moreover, AI technology (e.g., CNN) may support large data storage and maintenance through automatized deduplication and error detection, ultimately leading to a more holistic approach to rejection prediction and diagnosis. Such a database could integrate genetic, metabolomic, immunoproteomic, histological variables, and laboratory values to leverage their diagnostic and predictive value (60, 61).
AI-Aided malignancy screening
Cancer represents a widely recognized complication of both SOT and VCA, since there is a two to four-fold elevated risk of malignancies following organ transplant. Remarkably, transplant recipients are at a 100-fold higher risk for developing skin cancer (62). While the exact mechanisms are still under investigation, immunosuppressants have been linked to an increased incidence of cancer in transplant recipients when compared to age-matched control groups (63). Of note, different regimes of immunosuppressants resulted in comparable cancer rates (64). Therefore, follow-up guidelines commonly include routine check-ups such as skin examinations (65). Yet, there are various barriers for access to such dermatologic care (e.g., long wait times, lack of specialized transplant dermatologists, long travel distances) and aftercare in general that reduce patient compliance and the effectiveness of follow-up care (66).
AI technology (especially CNN and DL) has been shown to provide a versatile and precise screening platform for diverse cancer entities and detect mammographic abnormalities with comparable accuracy to radiologists and reduce radiologist workloads (67–70). Yi et al. programmed DeepCAT (a DL platform) including two key elements for mammogram suspicion scoring: (1) discrete masses and (2) other image features indicative of cancer, such as architectural distortion. The authors used a training set of 1,878 2D mammographic scans. The model classified 315 out of 595 images (53%) as “low priority”. Notably, none of these low-priority images contained any tumorous malignancies (71). Moreover, Kulkarni et al. used digital pathology images of 108 breast cancer patients to predict disease-specific survival. The authors reported an area under the curve of 0.90 in their testing set which consisted of 104 patients. Based on this cut-off, the CNN model could reliably assess the disease-specific survival (72). Lotter et al. developed a DL-aided diagnostic tool to effectively screen mammographies. Strikingly, the algorithm outperformed five radiologists, each fellowship-trained in breast imaging, on a cancer-enriched dataset. The algorithm resulted in an absolute increase in sensitivity of 14% and specificity of 24%, respectively. Further, the AI-based approach showed promising potential to detect interval cancers (increase in sensitivity of 18%; increase in specificity of 16%) (73). Tschandl et al. further used ML to categorize skin neoplasia. When comparing the screening accuracy of 511 human evaluators (dermatologists, general practitioners) to the AI model, the authors found that the algorithm yielded a significantly higher accuracy. Interestingly, the AI platform even outperformed dermatologists with more than ten years of clinical experience (74). For skin cancer screening, AI yielded an average accuracy value of 87% (with a maximum of 99% and a minimum of 67%) (75–79). Further, Pham et al. programmed a ML platform, based on 17,302 images of melanoma and nevus. The model performance was compared to that of 157 dermatologists from twelve university hospitals in Germany. The authors reported that the AI-based screening approach yielded a sensitivity of 85%, and specificity of 95%, ultimately outperforming all human evaluators (80). DL has also been demonstrated to reliably detect soft tissue sarcomas using 506 histopathological slides from 291 patients. The screening program showed an accuracy of 80% in diagnosing the five most common soft tissue sarcoma subtypes, as well as significantly improving the accuracy of the pathologists from 46% to 87% (81).
Following FVCA, one transplant recipient patient was diagnosed with basal cell carcinoma of the native facial skin six years post-transplant, while another FVCA patient presented with a B cell lymphoma which was treated with rituximab, cyclophosphamide, vincristine, doxorubicin, and prednisolone (82). Despite the successful treatment of the lymphoma, the patient was diagnosed with posttransplant-related liver tumors (83). Vice versa, there were also two cases of patients who underwent FVCA because of their cancer disease. The first was a Chinese patient with advanced melanoma who received a graft including the scalp and both ears. Of note, the long-term outcome of this case remains unreported (84). The other case involved a 42-year-old, HIV-positive male from Spain. While pseudosarcomatous spindle cell proliferation was diagnosed eleven months postoperatively and successfully treated, the patient ultimately died of a transplant-related lymphoma (85). AI technology carries promising potential to address this medical complication by facilitating posttransplant screening examinations and allowing for more frequent follow-up checks. Given that FVCA still remains a novel procedure with a specific indication, AI-aided cancer screening could also accelerate the examiner's diagnosis-making, thus leveraging AI and the limited human clinical experience. This semi-automated, human-controlled pathway may represent a valuable alternative to fully automatized strategies when discussing possible liability issues (Figure 2) (86).
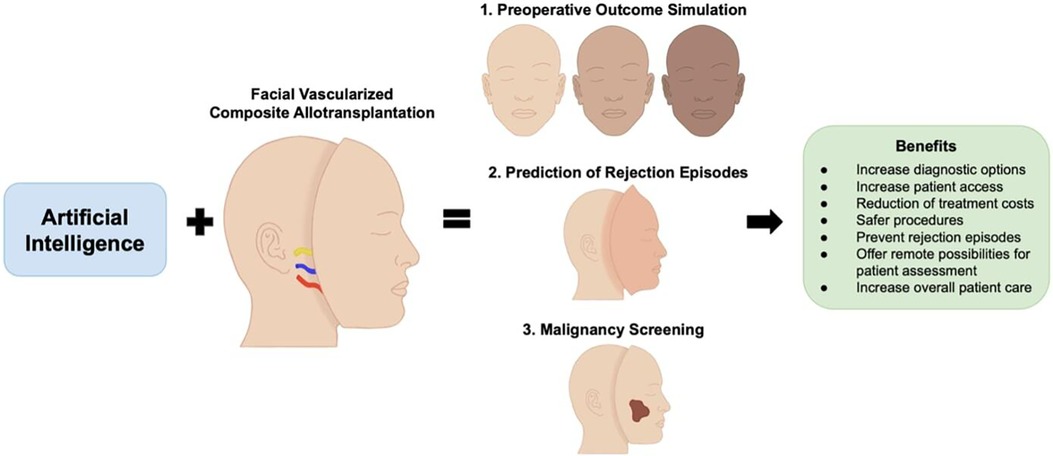
Figure 2. The combination of artificial intelligence and facial vascularized composite allotransplantation carries promising potential in advancing preoperative outcome simulation, prediction and diagnosis of rejection episodes, and cancer screening.
Discussion
FVCA has emerged as a novel and versatile reconstructive technique to provide adequate therapy for patients with devastating facial wounds and trauma (61, 87). While the surgical outcomes have proven its effectiveness, further steps are needed to streamline the perioperative workflow in FVCA surgery, thus broadening access to this type of surgery and increasing the work volume (7). Further research is needed to overcome persisting hurdles in the field. For instance, AI performance is based on access to patient datasets used for training. However, recent research has underpinned that AI algorithms could be susceptible to data security breaches (88). Given that FVCA involves highly sensitive patient information that allows to identify FVCA patients, any data breach could have severe ethical and legal implications. Therefore, the integration of AI into the field of FVCA calls for comprehensive data security strategies at the same time. Moreover, Obermeyer et al. revealed racial disparities in a state-of-the-art U.S. healthcare algorithm. The authors found that Black patients were in significantly poorer condition compared to White patients assigned the same level of risk by the algorithm, ultimately reducing the number of Black patients receiving extra care by ∼50% (89). Our group has recently highlighted the risk of racial disparities in FVCA patients (90). Therefore, FVCA providers should be sensitized on AI technology potentially catalyzing racial inequities. To this date, FVCA surgery still represents a highly individualized and resource-intensive therapy concept. Therefore, AI could help reduce work costs and time, as well as address persisting challenges in this uprising field such as outcome simulation, rejection detection, and postoperative cancer screening. This line of research may serve as the fundament for large-scale studies investigating possible points of leverage between FVCA and different AI concepts. Future research areas may include the use of AI in personalized drug therapy following FVCA. AI algorithms such as neural networks are being used to tailor drug regimens (i.e., drug combinations, drug dosages) in liver transplantation patients (NCT03527238). This approach could reduce the therapy-induced side effects after FVCA and expand the FVCA recipient pool onto patients with severe preexisting comorbidities resulting in complex drug interaction (91). In addition, AI-driven natural language processing systems could facilitate remote monitoring of FVCA patients. There is evidence that AI-supported telemedicine helps improve patient-reported outcomes in chronic disease care (e.g., diabetes mellitus type II, malignancies) (92, 93). Currently, at least 23 U.S. institutions have established FVCA programs with some patients traveling long-distance for pre- and postoperative clinic visits (94). Long distances between healthcare providers and patients have been demonstrated to reduce compliance leading to poorer postoperative outcomes (95). AI-powered telemedicine may fill in this healthcare gap facilitating closer perioperative FVCA patient management. Overall, the symbiosis of FVCA and AI carries untapped potential for improving patient healthcare and advancing this revolutionary surgical approach.
Author contributions
LK: Writing – original draft. SK: Writing – review & editing. OA: Writing – review & editing. KR: Visualization, Writing – review & editing. MM: Writing – review & editing. AS: Writing – review & editing. MA: Conceptualization, Writing – review & editing. BP: Writing – review & editing. MK: Writing – original draft, Writing – review & editing.
Funding
The author(s) declare that no financial support was received for the research, authorship, and/or publication of this article.
Conflict of interest
The authors declare that the research was conducted in the absence of any commercial or financial relationships that could be construed as a potential conflict of interest.
Publisher's note
All claims expressed in this article are solely those of the authors and do not necessarily represent those of their affiliated organizations, or those of the publisher, the editors and the reviewers. Any product that may be evaluated in this article, or claim that may be made by its manufacturer, is not guaranteed or endorsed by the publisher.
References
1. Kauke M, Safi AF, Panayi AC, Palmer WJ, Haug V, Kollar B, et al. A systematic review of immunomodulatory strategies used in skin-containing preclinical vascularized composite allotransplant models. J Plast Reconstr Aesthet Surg. (2022) 75(2):586–604. doi: 10.1016/j.bjps.2021.11.003
2. Kauke-Navarro M, Tchiloemba B, Haug V, Kollar B, Diehm Y, Safi AF, et al. Pathologies of oral and sinonasal mucosa following facial vascularized composite allotransplantation. J Plast Reconstr Aesthet Surg. (2021) 74(7):1562–71. doi: 10.1016/j.bjps.2020.11.028
3. Safi AF, Kauke M, Nelms L, Palmer WJ, Tchiloemba B, Kollar B, et al. Local immunosuppression in vascularized composite allotransplantation (VCA): a systematic review. J Plast Reconstr Aesthet Surg. (2021) 74(2):327–35. doi: 10.1016/j.bjps.2020.10.003
4. Kauke M, Panayi AC, Tchiloemba B, Diehm YF, Haug V, Kollar B, et al. Face transplantation in a black patient — racial considerations and early outcomes. N Engl J Med. (2021) 384(11):1075–6. doi: 10.1056/NEJMc2033961
5. Kauke-Navarro M, Knoedler S, Panayi AC, Knoedler L, Noel OF, Pomahac B. Regulatory T cells: liquid and living precision medicine for the future of VCA. Transplantation. (2023) 107(1):86–97. doi: 10.1097/TP.0000000000004342
6. Haug V, Panayi AC, Knoedler S, Foroutanjazi S, Kauke-Navarro M, Fischer S, et al. Implications of vascularized composite allotransplantation in plastic surgery on legal medicine. J Clin Med. (2023) 12(6):2308–13. doi: 10.3390/jcm12062308
7. Tchiloemba B, Kauke M, Haug V, Abdulrazzak O, Safi AF, Kollar B, et al. Long-term outcomes after facial allotransplantation: systematic review of the literature. Transplantation. (2021) 105(8):1869–80. doi: 10.1097/TP.0000000000003513
8. Knoedler L, Knoedler S, Panayi AC, Lee CAA, Sadigh S, Huelsboemer L, et al. Cellular activation pathways and interaction networks in vascularized composite allotransplantation. Front Immunol. (2023) 14:14–24. doi: 10.3389/fimmu.2023.1179355
9. Chartier C, Watt A, Lin O, Chandawarkar A, Lee J, Hall-Findlay E. BreastGAN: artificial intelligence-enabled breast augmentation simulation. Aesthet Surg J Open Forum. (2022) 4:ojab052. doi: 10.1093/asjof/ojab052
10. Martínez-Alario J, Tuesta ID, Plasencia E, Santana M, Mora ML. Mortality prediction in cardiac surgery patients. Circulation. (1999) 99(18):2378–82. doi: 10.1161/01.CIR.99.18.2378
11. Houts PS, Doak CC, Doak LG, Loscalzo MJ. The role of pictures in improving health communication: a review of research on attention, comprehension, recall, and adherence. Patient Educ Couns. (2006) 61(2):173–90. doi: 10.1016/j.pec.2005.05.004
12. Chartier C, Gfrerer L, Knoedler L, Austen WG Jr. Artificial intelligence-enabled evaluation of pain sketches to predict outcomes in headache surgery. Plast Reconstr Surg. (2023) 151(2):405–11. doi: 10.1097/PRS.0000000000009855
13. Knoedler L, Odenthal J, Prantl L, Oezdemir B, Kehrer A, Kauke-Navarro M, et al. Artificial intelligence-enabled simulation of gluteal augmentation: a helpful tool in preoperative outcome simulation? J Plast Reconstr Aesthet Surg. (2023) 80:94–101. doi: 10.1016/j.bjps.2023.01.039
14. Rokhshad R, Keyhan SO, Yousefi P. Artificial intelligence applications and ethical challenges in oral and maxillo-facial cosmetic surgery: a narrative review. Maxillofac Plast Reconstr Surg. (2023) 45(1):14. doi: 10.1186/s40902-023-00382-w
15. Preoperative computer simulation in rhinoplasty using previous postoperative images. Facial Plast Surg Aesthet Med. (2020) 22(6):406–11. doi: 10.1089/fpsam.2019.0016
16. Bottino A, Laurentini A, Rosano L. International conference in central Europe on computer graphics and visualization. A new computer-aided technique for planning the aesthetic outcome of plastic surgery (2008).
17. Mussi E, Servi M, Facchini F, Carfagni M, Volpe Y. A computer-aided strategy for preoperative simulation of autologous ear reconstruction procedure. Int J Interact Des Manuf (IJIDeM). (2021) 15(1):77–80. doi: 10.1007/s12008-020-00723-3
18. Persing S, Timberlake A, Madari S, Steinbacher D. Three-Dimensional imaging in rhinoplasty: a comparison of the simulated versus actual result. Aesthetic Plast Surg. (2018) 42(5):1331–5. doi: 10.1007/s00266-018-1151-9
19. Guarin DL, Yunusova Y, Taati B, Dusseldorp JR, Mohan S, Tavares J, et al. Toward an automatic system for computer-aided assessment in facial palsy. Facial Plast Surg Aesthet Med. (2020) 22(1):42–9. doi: 10.1089/fpsam.2019.29000.gua
20. Metzler P, Sun Y, Zemann W, Bartella A, Lehner M, Obwegeser J, et al. Validity of the 3D VECTRA photogrammetric surface imaging system for cranio-maxillofacial anthropometric measurements. Oral Maxillofac Surg. (2013) 18(3):297–304. doi: 10.1007/s10006-013-0404-7
21. Allen C, Aryal S, Do T, Gautum R, Hasan MM, Jasthi BK, et al. Deep learning strategies for addressing issues with small datasets in 2D materials research: microbial corrosion. Front Microbiol. (2022) 13:1059123. doi: 10.3389/fmicb.2022.1059123
22. Staub BN, Sadrameli SS. The use of robotics in minimally invasive spine surgery. J Spine Surg. (2019) 5(Suppl 1):S31–s40. doi: 10.21037/jss.2019.04.16
23. Park JJ, Tiefenbach J, Demetriades AK. The role of artificial intelligence in surgical simulation. Front Med Technol. (2022) 4:1076755. doi: 10.3389/fmedt.2022.1076755
24. den Boer RB, Jaspers TJM, de Jongh C, Pluim JPW, van der Sommen F, Boers T, et al. Deep learning-based recognition of key anatomical structures during robot-assisted minimally invasive esophagectomy. Surg Endosc. (2023) 37(7):5164–75.36947221
25. Hashimoto DA, Rosman G, Witkowski ER, Stafford C, Navarette-Welton AJ, Rattner DW, et al. Computer vision analysis of intraoperative video: automated recognition of operative steps in laparoscopic sleeve gastrectomy. Ann Surg. (2019) 270(3):414–21. doi: 10.1097/SLA.0000000000003460
26. Fullerton ZH, Tsangaris E, De Vries CEE, Klassen AF, Aycart MA, Sidey-Gibbons CJ, et al. Patient-reported outcomes measures used in facial vascularized composite allotransplantation: a systematic literature review. J Plast Reconstr Aesthet Surg. (2022) 75(1):33–44. doi: 10.1016/j.bjps.2021.09.002
27. Chandawarkar AA, Diaz-Siso JR, Bueno EM, Jania CK, Hevelone ND, Lipsitz SR, et al. Facial appearance transfer and persistence after three-dimensional virtual face transplantation. Plast Reconstr Surg. (2013) 132(4):957–66. doi: 10.1097/PRS.0b013e3182a0143b
28. Formeister EJ, Baum R, Knott PD, Seth R, Ha P, Ryan W, et al. Machine learning for predicting complications in head and neck microvascular free tissue transfer. Laryngoscope. (2020) 130(12):E843–e849. doi: 10.1002/lary.28508
29. Eppler MB, Sayegh AS, Maas M, Venkat A, Hemal S, Desai MM, et al. Automated capture of intraoperative adverse events using artificial intelligence: a systematic review and meta-analysis. J Clin Med. (2023) 12(4):1687–92. doi: 10.3390/jcm12041687
30. Cofano F, Di Perna G, Bozzaro M, Longo A, Marengo N, Zenga F, et al. Augmented reality in medical practice: from spine surgery to remote assistance. Front Surg. (2021) 8:657901. doi: 10.3389/fsurg.2021.657901
31. Garcia Nespolo R, Yi D, Cole E, Valikodath N, Luciano C, Leiderman YI. Evaluation of artificial intelligence-based intraoperative guidance tools for phacoemulsification cataract surgery. JAMA Ophthalmol. (2022) 140(2):170–7. doi: 10.1001/jamaophthalmol.2021.5742
32. Studier-Fischer A, Seidlitz S, Sellner J, Özdemir B, Wiesenfarth M, Ayala L, et al. Spectral organ fingerprints for machine learning-based intraoperative tissue classification with hyperspectral imaging in a porcine model. Sci Rep. (2022) 12(1):11028. doi: 10.1038/s41598-022-15040-w
33. Maktabi M, Köhler H, Ivanova M, Neumuth T, Rayes N, Seidemann L, et al. Classification of hyperspectral endocrine tissue images using support vector machines. Int J Med Robot. (2020) 16(5):1–10. doi: 10.1002/rcs.2121
34. Black CK, Termanini KM, Aguirre O, Hawksworth JS, Sosin M. Solid organ transplantation in the 21(st) century. Ann Transl Med. (2018) 6(20):409. doi: 10.21037/atm.2018.09.68
35. Pilch NA, Bowman LJ, Taber DJ. Immunosuppression trends in solid organ transplantation: the future of individualization, monitoring, and management. Pharmacotherapy. (2021) 41(1):119–31. doi: 10.1002/phar.2481
36. Linden PK. History of solid organ transplantation and organ donation. Crit Care Clin. (2009) 25(1):165–84., ix. doi: 10.1016/j.ccc.2008.12.001
37. Demetris AJ, Murase N, Lee RG, Randhawa P, Zeevi A, Pham S, et al. Chronic rejection. A general overview of histopathology and pathophysiology with emphasis on liver, heart and intestinal allografts. Ann Transplant. (1997) 2(2):27–44.9869851
38. Choudhary NS, Saigal S, Bansal RK, Saraf N, Gautam D, Soin AS. Acute and chronic rejection after liver transplantation: what a clinician needs to know. J Clin Exp Hepatol. (2017) 7(4):358–66. doi: 10.1016/j.jceh.2017.10.003
39. Gotlieb N, Azhie A, Sharma D, Spann A, Suo NJ, Tran J, et al. The promise of machine learning applications in solid organ transplantation. npj Digital Medicine. (2022) 5(1):89. doi: 10.1038/s41746-022-00637-2
40. Moccia S, Mattos LS, Patrini I, Ruperti M, Poté N, Dondero F, et al. Computer-assisted liver graft steatosis assessment via learning-based texture analysis. Int J Comput Assist Radiol Surg. (2018) 13:1357–67. doi: 10.1007/s11548-018-1787-6
41. Thongprayoon C, Vaitla P, Jadlowiec CC, Leeaphorn N, Mao SA, Mao MA, et al. Use of machine learning consensus clustering to identify distinct subtypes of black kidney transplant recipients and associated outcomes. JAMA Surg. (2022) 157(7):e221286. doi: 10.1001/jamasurg.2022.1286
42. Zare A, Zare MA, Zarei N, Yaghoobi R, Zare MA, Salehi S, et al. A neural network approach to predict acute allograft rejection in liver transplant recipients using routine laboratory data. Hepat Mon. (2017) 17(12):e55092. doi: 10.5812/hepatmon.55092
43. Parkes MD, Aliabadi AZ, Cadeiras M, Crespo-Leiro MG, Deng M, Depasquale EC, et al. An integrated molecular diagnostic report for heart transplant biopsies using an ensemble of diagnostic algorithms. J Heart Lung Transplant. (2019) 38(6):636–46. doi: 10.1016/j.healun.2019.01.1318
44. Halloran KM, Parkes MD, Chang J, Timofte IL, Snell GI, Westall GP, et al. Molecular assessment of rejection and injury in lung transplant biopsies. J Heart Lung Transplant. (2019) 38(5):504–13. doi: 10.1016/j.healun.2019.01.1317
45. Halloran K, Parkes MD, Timofte IL, Snell GI, Westall GP, Hachem R, et al. Molecular phenotyping of rejection-related changes in mucosal biopsies from lung transplants. Am J Transplant. (2020) 20(4):954–66. doi: 10.1111/ajt.15685
46. Abdeltawab H, Shehata M, Shalaby A, Khalifa F, Mahmoud A, El-Ghar MA, et al. A novel CNN-based CAD system for early assessment of transplanted kidney dysfunction. Sci Rep. (2019) 9(1):5948. doi: 10.1038/s41598-019-42431-3
47. Sarhane KA, Tuffaha SH, Broyles JM, Ibrahim AE, Khalifian S, Baltodano P, et al. A critical analysis of rejection in vascularized composite allotransplantation: clinical, cellular and molecular aspects, current challenges, and novel concepts. Front Immunol. (2013) 4:406. doi: 10.3389/fimmu.2013.00406
48. Leonard DA, Amin KR, Giele H, Fildes JE, Wong JKF. Skin immunology and rejection in VCA and organ transplantation. Curr Transplant Rep. (2020) 7(4):251–9. doi: 10.1007/s40472-020-00310-1
49. Alhefzi M, Aycart MA, Bueno EM, Kiwanuka H, Krezdorn N, Pomahac B, et al. Treatment of rejection in vascularized composite allotransplantation. Curr Transplant Rep. (2016) 3(4):404–9. doi: 10.1007/s40472-016-0128-3
50. Schneeberger S, Kreczy A, Brandacher G, Steurer W, Margreiter R. Steroid-and ATG-resistant rejection after double forearm transplantation responds to campath-1H. Am J Transplant. (2004) 4(8):1372–4. doi: 10.1111/j.1600-6143.2004.00518.x
51. Schneeberger S, Zelger B, Ninkovic M, Margreiter R. Transplantation of the hand. Transplant Rev. (2005) 19(2):100–7. doi: 10.1016/j.trre.2005.07.001
52. Schuind F, Abramowicz D, Schneeberger S. Hand transplantation: the state-of-the-art. J Hand Surg British Eur Vol. (2007) 32(1):2–17. doi: 10.1016/j.jhsb.2006.09.008
53. Kaufman CL, Cascalho M, Ozyurekoglu T, Jones CM, Ramirez A, Roberts T, et al. The role of B cell immunity in VCA graft rejection and acceptance. Hum Immunol. (2019) 80(6):385–92. doi: 10.1016/j.humimm.2019.03.002
54. Iske J, Nian Y, Maenosono R, Maurer M, Sauer IM, Tullius SG. Composite tissue allotransplantation: opportunities and challenges. Cell Mol Immunol. (2019) 16(4):343–9. doi: 10.1038/s41423-019-0215-3
55. Kauke M, Safi AF, Zhegibe A, Haug V, Kollar B, Nelms L, et al. Mucosa and rejection in facial vascularized composite allotransplantation: a systematic review. Transplantation. (2020) 104(12):2616–24. doi: 10.1097/TP.0000000000003171
56. Chlap P, Min H, Vandenberg N, Dowling J, Holloway L, Haworth A. A review of medical image data augmentation techniques for deep learning applications. J Med Imaging Radiat Oncol. (2021) 65(5):545–63. doi: 10.1111/1754-9485.13261
57. Goceri E. Medical image data augmentation: techniques, comparisons and interpretations. Artif Intell Rev. (2023) 14:1–45. doi: 10.1007/s10462-023-10453-z
58. Mascagni P, Alapatt D, Sestini L, Altieri MS, Madani A, Watanabe Y, et al. Computer vision in surgery: from potential to clinical value. NPJ Digit Med. (2022) 5(1):163. doi: 10.1038/s41746-022-00707-5
59. Marwaha JS, Raza MM, Kvedar JC. The digital transformation of surgery. NPJ Digit Med. (2023) 6(1):103. doi: 10.1038/s41746-023-00846-3
60. Lee CAA, Wang D, Kauke-Navarro M, Russell-Goldman E, Xu S, Mucciarone KN, et al. Insights from immunoproteomic profiling of a rejected full face transplant. Am J Transplant. (2023) 23(7):1058–61. doi: 10.1016/j.ajt.2023.04.008
61. Kauke-Navarro M, Knoedler S, Panayi AC, Knoedler L, Haller B, Parikh N, et al. Correlation between facial vascularized composite allotransplantation rejection and laboratory markers: insights from a retrospective study of eight patients. J Plast Reconstr Aesthet Surg. (2023) 83:155–64. doi: 10.1016/j.bjps.2023.04.050
62. Wheless L, Anand N, Hanlon A, Chren MM. Differences in skin cancer rates by transplanted organ type and patient age after organ transplant in white patients. JAMA Dermatol. (2022) 158(11):1287–92. doi: 10.1001/jamadermatol.2022.3878
63. Dantal J, Soulillou J-P. Immunosuppressive drugs and the risk of cancer after organ transplantation. N Engl J Med. (2005) 352(13):1371–3. doi: 10.1056/NEJMe058018
64. Gallagher MP, Kelly PJ, Jardine M, Perkovic V, Cass A, Craig JC, et al. Long-term cancer risk of immunosuppressive regimens after kidney transplantation. J Am Soc Nephrol. (2010) 21(5):852–8. doi: 10.1681/ASN.2009101043
65. Lam K, Coomes EA, Nantel-Battista M, Kitchen J, Chan AW. Skin cancer screening after solid organ transplantation: survey of practices in Canada. Am J Transplant. (2019) 19(6):1792–7. doi: 10.1111/ajt.15224
66. Najmi M, Brown AE, Harrington SR, Farris D, Sepulveda S, Nelson KC. A systematic review and synthesis of qualitative and quantitative studies evaluating provider, patient, and health care system-related barriers to diagnostic skin cancer examinations. Arch Dermatol Res. (2022) 314(4):329–40. doi: 10.1007/s00403-021-02224-z
67. Sasieni P. Evaluation of the UK breast screening programmes. Ann Oncol. (2003) 14(8):1206–8. doi: 10.1093/annonc/mdg325
68. Maroni R, Massat NJ, Parmar D, Dibden A, Cuzick J, Sasieni PD, et al. A case-control study to evaluate the impact of the breast screening programme on mortality in England. Br J Cancer. (2021) 124(4):736–43. doi: 10.1038/s41416-020-01163-2
69. Schaffter T, Buist DS, Lee CI, Nikulin Y, Ribli D, Guan Y, et al. Evaluation of combined artificial intelligence and radiologist assessment to interpret screening mammograms. JAMA network Open. (2020) 3(3):e200265–e200265. doi: 10.1001/jamanetworkopen.2020.0265
70. Rodriguez-Ruiz A, Lång K, Gubern-Merida A, Broeders M, Gennaro G, Clauser P, et al. Stand-alone artificial intelligence for breast cancer detection in mammography: comparison with 101 radiologists. JNCI: J Natl Cancer Inst. (2019) 111(9):916–22. doi: 10.1093/jnci/djy222
71. Yi PH, Singh D, Harvey SC, Hager GD, Mullen LA. DeepCAT: deep computer-aided triage of screening mammography. J Digit Imaging. (2021) 34:27–35. doi: 10.1007/s10278-020-00407-0
72. Kulkarni PM, Robinson EJ, Sarin Pradhan J, Gartrell-Corrado RD, Rohr BR, Trager MH, et al. Deep learning based on standard H&E images of primary melanoma tumors identifies patients at risk for visceral recurrence and death. Clin Cancer Res. (2020) 26(5):1126–34. doi: 10.1158/1078-0432.CCR-19-1495
73. Lotter W, Diab AR, Haslam B, Kim JG, Grisot G, Wu E, et al. Robust breast cancer detection in mammography and digital breast tomosynthesis using an annotation-efficient deep learning approach. Nat Med. (2021) 27(2):244–9. doi: 10.1038/s41591-020-01174-9
74. Tschandl P, Codella N, Akay BN, Argenziano G, Braun RP, Cabo H, et al. Comparison of the accuracy of human readers versus machine-learning algorithms for pigmented skin lesion classification: an open, web-based, international, diagnostic study. Lancet Oncol. (2019) 20(7):938–47. doi: 10.1016/S1470-2045(19)30333-X
75. S P, Tr GB. An efficient skin cancer diagnostic system using bendlet transform and support vector machine. An Acad Bras Cienc. (2020) 92(1):e20190554. doi: 10.1590/0001-3765202020190554
76. Ramlakhan K, Shang Y. 2011 IEEE 23rd international conference on tools with artificial intelligence. A mobile automated skin lesion classification system (2011). IEEE.
77. Zhang J, Xie Y, Xia Y, Shen C. Attention residual learning for skin lesion classification. IEEE transactions on Medical Imaging. (2019) 38(9):2092–103. doi: 10.1109/TMI.2019.2893944
78. Marchetti MA, Codella NC, Dusza SW, Gutman DA, Helba B, Kalloo A, et al. Results of the 2016 international skin imaging collaboration international symposium on biomedical imaging challenge: comparison of the accuracy of computer algorithms to dermatologists for the diagnosis of melanoma from dermoscopic images. J Am Acad Dermatol. (2018) 78(2):270–7. doi: 10.1016/j.jaad.2017.08.016
79. Takiddin A, Schneider J, Yang Y, Abd-Alrazaq A, Househ M. Artificial intelligence for skin cancer detection: scoping review. J Med Internet Res. (2021) 23(11):e22934. doi: 10.2196/22934
80. Pham TC, Luong CM, Hoang VD, Doucet A. AI Outperformed every dermatologist in dermoscopic melanoma diagnosis, using an optimized deep-CNN architecture with custom mini-batch logic and loss function. Sci Rep. (2021) 11(1):17485. doi: 10.1038/s41598-021-96707-8
81. Foersch S, Eckstein M, Wagner DC, Gach F, Woerl AC, Geiger J, et al. Deep learning for diagnosis and survival prediction in soft tissue sarcoma. Ann Oncol. (2021) 32(9):1178–87. doi: 10.1016/j.annonc.2021.06.007
82. Kanitakis J, Petruzzo P, Gazarian A, Testelin S, Devauchelle B, Badet L, et al. Premalignant and malignant skin lesions in two recipients of vascularized composite tissue allografts (face, hands). Case Rep Transplant. (2015) 2015:246–51. doi: 10.1155/2015/356459
83. Petruzzo P, Kanitakis J, Testelin S, Pialat JB, Buron F, Badet L, et al. Clinicopathological findings of chronic rejection in a face grafted patient. Transplantation. (2015) 99(12):2644–50. doi: 10.1097/TP.0000000000000765
84. Jiang HQ, Wang Y, Hu XB, Li YS, Li JS. Composite tissue allograft transplantation of cephalocervical skin flap and two ears. Plast Reconstr Surg. (2005) 115(3):31e–5e. doi: 10.1097/01.PRS.0000153038.31865.02
85. Uluer MC, Brazio PS, Woodall JD, Nam AJ, Bartlett ST, Barth RN. Vascularized composite allotransplantation: medical complications. Curr Transplant Rep. (2016) 3(4):395–403. doi: 10.1007/s40472-016-0113-x
86. Knoedler L, Knoedler S, Kauke-Navarro M, Knoedler C, Hoefer S, Baecher H, et al. Three-dimensional medical printing and associated legal issues in plastic surgery: a scoping review. Plast Reconstr Surg—Glob Open. (2023) 11(4):e4965. doi: 10.1097/GOX.0000000000004965
87. Kauke M, Panayi AC, Tchiloemba B, Diehm YF, Haug V, Kollar B, et al. Face transplantation in a black patient—racial considerations and early outcomes. N Engl J Med. (2021) 384(11):1075–6. doi: 10.1056/NEJMc2033961
88. Khan B, Fatima H, Qureshi A, Kumar S, Hanan A, Hussain J, et al. Drawbacks of artificial intelligence and their potential solutions in the healthcare sector. Biomed Mater Devices. (2023) 8:1–8.
89. Obermeyer Z, Powers B, Vogeli C, Mullainathan S. Dissecting racial bias in an algorithm used to manage the health of populations. Science. (2019) 366(6464):447–53. doi: 10.1126/science.aax2342
90. Kauke-Navarro M, Knoedler L, Knoedler S, Diatta F, Huelsboemer L, Stoegner VA, et al. Ensuring racial and ethnic inclusivity in facial vascularized composite allotransplantation. Plast Reconstr Surg Glob Open. (2023) 11(8):e5178. doi: 10.1097/GOX.0000000000005178
91. Shokri T, Saadi R, Wang W, Reddy L, Ducic Y. Facial transplantation: complications, outcomes, and long-term management strategies. Semin Plast Surg. (2020) 34(4):245–53. doi: 10.1055/s-0040-1721760
92. El-Sherif DM, Abouzid M, Elzarif MT, Ahmed AA, Albakri A, Alshehri MM. Telehealth and artificial intelligence insights into healthcare during the COVID-19 pandemic. Healthcare (Basel). (2022) 10(2):385–95. doi: 10.3390/healthcare10020385
93. Cingolani M, Scendoni R, Fedeli P, Cembrani F. Artificial intelligence and digital medicine for integrated home care services in Italy: opportunities and limits. Front Public Health. (2022) 10:1095001. doi: 10.3389/fpubh.2022.1095001
94. Diep GK, Berman ZP, Alfonso AR, Ramly EP, Boczar D, Trilles J, et al. The 2020 facial transplantation update: a 15-year compendium. Plast Reconstr Surg Glob Open. (2021) 9(5):e3586. doi: 10.1097/GOX.0000000000003586
Keywords: vascularized composite allotransplantation, VCA, facial VCA, face transplant, artificial intelligence, AI, machine learning, deep learning
Citation: Knoedler L, Knoedler S, Allam O, Remy K, Miragall M, Safi A-F, Alfertshofer M, Pomahac B and Kauke-Navarro M (2023) Application possibilities of artificial intelligence in facial vascularized composite allotransplantation—a narrative review. Front. Surg. 10:1266399. doi: 10.3389/fsurg.2023.1266399
Received: 24 July 2023; Accepted: 26 September 2023;
Published: 30 October 2023.
Edited by:
Sergio Olate, University of La Frontera, ChileReviewed by:
Hayson Chenyu Wang, Shanghai Jiao Tong University, ChinaOtacílio Luiz Chagas-Júnior, Federal University of Pelotas, Brazil
© 2023 Knoedler, Knoedler, Allam, Remy, Miragall, Safi, Alfertshofer, Pomahac and Kauke-Navarro. This is an open-access article distributed under the terms of the Creative Commons Attribution License (CC BY). The use, distribution or reproduction in other forums is permitted, provided the original author(s) and the copyright owner(s) are credited and that the original publication in this journal is cited, in accordance with accepted academic practice. No use, distribution or reproduction is permitted which does not comply with these terms.
*Correspondence: Leonard Knoedler Leonard.Knoedler@ukr.de Martin Kauke-Navarro kauke-navarro.martin@yale.edu