- 1Laboratory of Systems and Synthetic Biology, Wageningen University and Research, Wageningen, Netherlands
- 2MSD Animal Health, Boxmeer, Netherlands
- 3Bioprocess Engineering, Wageningen University and Research, Wageningen, Netherlands
- 4LifeGlimmer GmbH, Berlin, Germany
Systems and Synthetic Biology are complementary fields emerging side-by-side into mainstream scientific research. Whilst systems biologists focus on understanding natural systems, synthetic biologists wish to modify, adapt and re-purpose biological systems towards certain desired goals, for example enhancing efficiency and robustness of desired biological traits. In both fields, data analysis, predictive mathematical modelling, experimental design, and controlled experimentation are crucial to obtain reproducible results and understand how applications can be scaled to larger systems and processes. As such, students from Life Sciences, Engineering, and Mathematics backgrounds must be taught fundamentals in biological systems, experimental techniques, mathematics, and data analysis/statistics. In addition, students must be trained for future multidisciplinary careers, where the interaction and communication between experimental and modelling researchers is fundamental. With the acceleration of technological developments (both computational and experimental) continuing unabated, educators need to bridge the increasing gap between fundamentally-required knowledge and skills that students need to pursue future academic or industrial research projects. In this paper, we will discuss how we have re-designed an introductory course in Systems and Synthetic Biology at Wageningen University and Research (Netherlands) that is targeted simultaneously to mathematical/computational students with an interest in biology and experimental methods, and to Life Science students interested in learning how biological systems can be mathematically analysed and modelled. The course highlights the links between fundamental methodologies and recently developed technologies within the Systems and Synthetic Biology fields. The course was re-designed for the 2021/22 academic year, we report that students from biology and biotechnology programmes graded their satisfaction of the course as 4.4 out of 5. We discuss how the course can act as a gateway to advanced courses in Systems Biology-oriented curricula (comprising: data infrastructure, modelling, and experimental synthetic biology), and towards future research projects.
1 Introduction
We are living in the century of biology (Venter and Cohen, 2004). The life sciences domain is becoming increasingly multi-disciplinary (Kitano, 2002), and recent major breakthroughs have required collaborative systems biology approaches, such as the recently-improved human genome sequence (Nurk et al., 2022) or the protein structure prediction tool AlphaFold (Jumper et al., 2021). In parallel to the increase in biological information, the development of fast and affordable DNA sequencing and synthesis is fueling the rise of synthetic biology to solve problems in medicine, agriculture, and manufacturing in sustainable and efficient ways (BBSRC, 2011). These novel technologies also have the capability to “create” organisms (Hutchison et al., 2016). As a consequence of these technological developments, green economies are becoming a reality (Delebecque and Philp, 2015), and synthetic biology is leading to the creation of enterprises and jobs (Vilanova and Porcar, 2019; Kampers et al., 2021). Given the speed at which life sciences research and careers are evolving into multi-disciplinary ventures, educators require refreshed and up-to-date course designs to train the future innovators in the biological revolution.
Previous studies of multi-disciplinary research groups and educational programmes have highlighted the presence of “boundaries” (Akkerman, 2011; Akkerman and Bakker, 2011): for students and researchers, these boundaries present not only a challenge to overcome but also an opportunity to broaden their knowledge, consider new methodologies or ideologies, and obtain novel insights. Within the Systems and Synthetic Biology field, one such boundary is communication and understanding between experimental and theoretical researchers. For the course outlined in this paper, we will refer often to experimental researchers as those who build genetic circuits and theoretical modellers as those who wish to predict novel experiments with ordinary differential equation (ODE) models that fit the available data. In this instance, it is desired that experimental laboratory researchers understand the value of using (predictive) mathematical models for experimental design and planning, whereas theoretical modellers need to understand experimental constraints that limit the scope of model development (equations or data fitting) and the refinement of any further predictions.
To cater to this educational need, pan-European educational consortia (Cvijovic, et al., 2016) and national programmes (like those promoted by the Dutch Research Council, NWO, in the Netherlands and by the Biotechnology and Biological Sciences Research Council, BBSRC, and the Engineering and Physical Sciences Research Council, EPSRC, in the UK, see https://www.nwo.nl/en/projects/022004006-0 and https://gow.epsrc.ukri.org/NGBOViewGrant.aspx?GrantRef=EP/L016494/1) have supported the creation of training centers for early career researchers, where students can follow courses covering fundamental topics in experimental and theoretical spheres of Systems and Synthetic Biology. At the academic level, educators have also described how problem-based learning and flipped classrooms can be used effectively to simulate computational biology research assuming that experimental data has already been obtained (Robeva and Laubenbacher, 2009; Eager, Peirce and Barlow, 2014; Sauter et al., 2022).
In this paper, and as an illustration of this integrative mindset for biology education, we discuss the development of a problem-based course aiming to train students in synthetic biology research that brings together predictive models and experimental methods for the design of, building of, testing of, and learning from biological circuits. To the best of our knowledge, this has not been discussed elsewhere in the literature.
2 Course structure
2.1 Course requirements
The first question that arises when designing an academic course on Systems and Synthetic Biology should be what makes the course different from those of other related fields like genetics, molecular biology, genomics, or microbiology. The leading theme of synthetic biology is the application of engineering principles in biology such as the standardization of parts, hierarchically combining modules into more complex systems, and the separation of system design from system construction (Endy, 2005). Following the engineering analogy, mathematical models bring together multiple sources of biological information to design and predict quantitatively the desired outcomes of the engineered biological systems (Chandran et al., 2008; Zheng and Sriram, 2010). However, the complexity and stochasticity of (at times understudied) living systems ensures that engineering of biology is a huge challenge requiring genetics, systems biology, chemistry, mathematics, and (bio)informatics (Farny, 2018; Honegger and de Bivort, 2018).
To guide system development, and building upon concepts from more traditional engineering disciplines, many synthetic biologists have adopted the DBTL (Design-Build-Test-Learn) framework (for examples see Miskovic et al., 2017; Carbonell et al., 2018). The researcher designs a series of constructs, builds them in the lab, tests their outcomes against model predictions and learns from the synthetic systems to redesign new and improved constructs, starting the cycle again. This cycle can be used both for top-down or bottom-up research. In the former, biological principles are uncovered by reducing large datasets or gaining insights using large systems level (e.g., whole cell) or statistical models [for example, see Ahn-Horst et al. (2022)]. In the latter, smaller, more detailed mechanistic models are iteratively expanded or edited as new data emerges that challenges or falsifies the underlying model [for a recent example of such modelling see Santos-Moreno et al. (2022)]. The recent review by Aydin et al. (2022) provides a thorough overview of how far the synthetic biology field has come following the DBTL cycle, and where current research is focusing for the future. On top of the purely scientific content, synthetic biologists also promote ethical values and considerations (Venter and Cohen, 2004; Farny, 2018). In particular, open science and education is actively pursued, by sharing knowledge, genetic parts, data and data analysis scripts or tools within the science community and with society (for example, through the iGEM Foundation; www.igem.org). As such, any synthetic biology course should go beyond scientific investigations to consider their impact on the world.
Given the multidisciplinarity of Systems and Synthetic Biology highlighted above, the student intake for any course will contain students that are stronger in one or two of the following areas: biology, mathematics, (bio)engineering, or ethics (Delebecque and Philp, 2015). This heterogeneity of skills and interests inherently creates the disciplinary boundaries discussed above which students need to be trained to overcome.
In light of this, the DBTL concept provides a useful framework for a synthetic biology course and is well suited for teacher-student collaborative projects with an active, project-based learning focus (Kuldell, 2007). By performing a complete DBTL cycle to solve a given problem, the students can reach the higher cognitive levels in synthetic biology, learn the skills necessary to use the different disciplines that are integrated in the field, and reach the “apply” level according to Bloom’s taxonomy (Bloom, 1956). The DBTL cycle also ensures a diversity of learning activities, which helps students with different learning styles, including: designing DNA devices and circuits, predicting the outcome of genetic circuits with models, analyzing papers in search of a solution to the problem or considering ethical issues. Some students will reach many of the learning goals whilst computationally designing their experiments and biological systems, others will reach them by experimenting in the laboratory.
In fact, there is, in our opinion, a high similarity between the DBTL cycle for engineering and Kolbs’ Learning Cycle for learning through experience (Figure 1; Kolbs, 1984). Following a DBTL cycle in a synthetic biology course ensures activities for the completion of Kolb’s learning cycle. During the Design phase activities, students abstractly conceptualize the biological problem based on their previous knowledge and assumptions. This is in the form of mathematical formulas, genetic designs and experiment proposals. The students proceed into active experimentation activities (both wet and dry) during the Build and Test phases, in which they perform or simulate their designed experiments and acquire new experimental data. Analyzing their newly acquired data and any observations during the active experimentation constitutes new concrete experiences for the learners. Finally, with this concrete experience completed, students make reflective observations as to how their previous knowledge and assumptions during the Learn phase have been confirmed or challenged. That might lead to a new Design and abstract conceptualization phases, hence starting both cycles again.
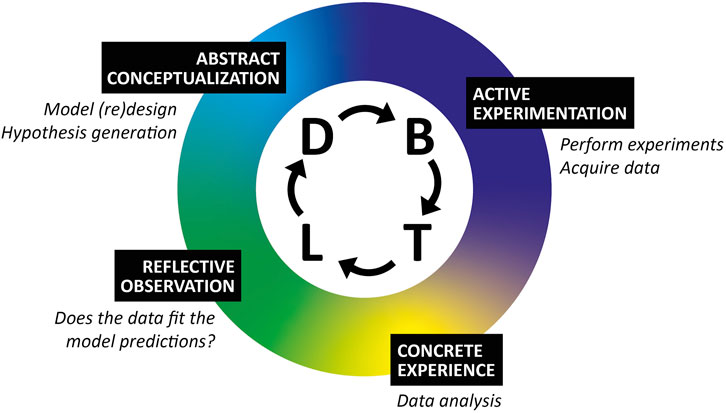
FIGURE 1. Comparison of DBTL and Kolbs’ Learning Cycle. Kolb’s learning cycle (Kolbs, 1984) can be superimposed on the DBTL cycle to show how students complete both processes in tandem. In this schematic, the design phase of the DBTL cycle is linked with abstract conceptualisation of the biological problem and hypothesis generation, active experimentation and concrete experiences are obtained during the build and test phases where data is acquired and analysed, whilst learning from new data requires reflective observations and whether the data matches the predicted expectations.
In this paper, we will discuss how we have re-designed an introductory course in Systems and Synthetic Biology following the DBTL cycle. The course was first offered in 2012 and has evolved towards this DBTL structure alongside changes in available technologies in the synthetic biology field. After providing an overview of the course, we will highlight the positive satisfaction observed by students in the re-designed course.
2.2 Course overview
By modularizing the weeks to discuss modelling software and methods, and experimental techniques with laboratory practice, educators can rotate the course to start at other parts of the DBTL cycle (see Figure 2). Following the first 5 weeks of teaching fundamental topics, students are then able to complete the DBTL cycle themselves in collaborative project work revolving around a published CRISPR (clustered regularly interspaced short palindromic repeats) interference toggle switch (Santos Moreno et al., 2020). After introducing the course and discussing its content, we will highlight the feedback received from students in course evaluations, how students can progress to move advanced topics, and discuss how the course has contributed to ongoing research efforts, providing a neat link between education and research. The course took place over 8 weeks from the end of August to October 2021 (the first teaching period in the academic year of Wageningen University) and was aimed at final year B.Sc., or early M.Sc., students. The course afforded the students six European Credit Transfer and Accumulation System (ECTS) credits towards their respective education programmes. We hope our illustration of a multi-disciplinary course provides inspiration to other teachers, lecturers, and researchers in the community.
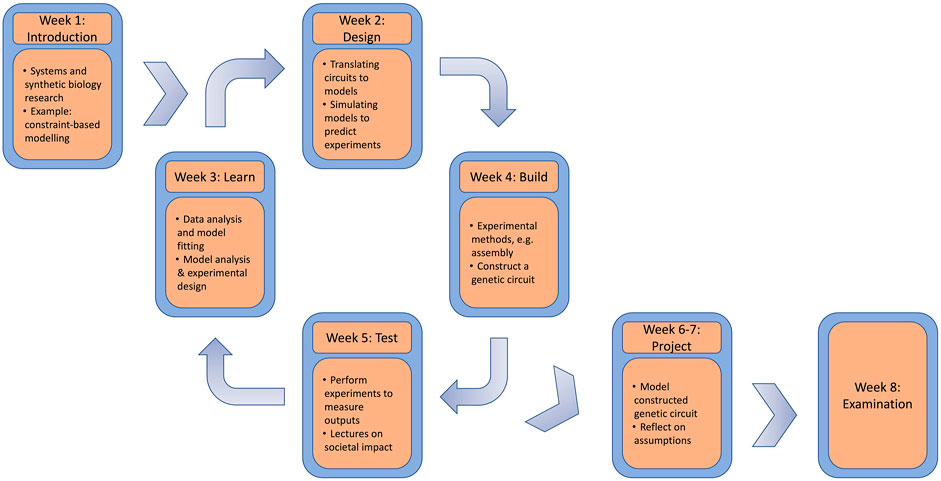
FIGURE 2. Course overview and relation to DBTL cycle. Whilst we do not explicitly follow the DBTL cycle in chronological order, we divide our teaching weeks into the four categories. After an introduction week, the DBTL cycle begins to show students how modelling and experimental synthetic biology can be combined. Later weeks then require students to write a report critically reflecting on their research, before a final exam tests the students on the knowledge they have obtained.
The learning outcomes for the course are:
• To recognize the basic theoretical and applied concepts of Systems and Synthetic Biology,
• To assess a biological system in terms of mathematical models,
• To implement in Python a (dynamic) model describing a biological system,
• To perform an iterative computational-laboratory (dry-wet) experiment based on a given genetic circuit,
• To employ standardized DNA assembly methods of synthetic biology to construct a genetic circuit,
• To discuss systems and synthetic biology experiments found in the literature,
• To understand the impact of synthetic biology in the near future, including its wider societal implications.
These learning goals address different cognitive levels. If we follow Bloom’s taxonomy (Bloom, 1956), this introductory course covers the first three goals of six: “to remember,” “to understand,” and “to apply” throughout. Through the second learning goal and the project work undertaken in the course (see below), we also touch on the fourth level: “to analyze.” However, we anticipate that only the best students will successfully achieve this fourth level within the course.
In the first 5 weeks, students are taught how to construct and analyze mathematical models, build synthetic systems experimentally, and understand societal impact issues related to synthetic biology. Each week is divided into lectures and practical classes or tutorials to allow students to practise the theory they have learnt. The focus of our DBTL cycle—towards which the entire course is directed—will be on constructing a previously published CRISPR interference toggle switch (Santos-Moreno et al., 2020). On the accompanying GitLab page we share the course calendar, material and protocols. This circuit covers many topics of interest to budding synthetic biologists: genetic engineering with CRISPR, constructing inducible genetic circuits, (modelling) non-linear system dynamics and behaviours, and the potential to control downstream cellular processes (e.g., kill-switches). However, our course structure could be easily implemented and redesigned for the purpose of constructing other synthetic circuits. For example, optogenetic (light controlled) transcriptional circuits have been constructed using transcription activator-like effector nucleases (TALENs) that, depending on the application, may be a better choice than CRISPR systems (Konermann et al., 2013; Jusiak et al., 2016; Bhardwaj and Nain, 2021).
In weeks six and seven, the students must write a report on their research throughout the course and explain differences observed between their modelling efforts and laboratory data, and discussing how the toggle switch could be utilised for future synthetic biology applications. The elected programming language for our course is Python, but other teachers may wish to adopt other languages like R, MATLAB, Octave, COPASI, or Julia.
In week eight, which at Wageningen University is the so-called exam-week, students then need to take a short written exam on the material covered in the course (usually closed-book exam consisting of open questions).
To highlight the topics covered in each part of the cycle, we will now provide a brief overview of the course content.
2.2.1 Week one: An introduction to systems and synthetic biology
In this first week, the course overview is presented and the key concepts of Systems and Synthetic Biology are defined and introduced to the students. A key element of the teaching is to convey the message that any biological system is made up of the (dynamic) interactions among its components (genes, proteins, metabolites, cells, microbes, organisms) and that Systems Biology deals essentially with understanding and quantifying these components and their interaction. If required, it is at this moment that the biological background required for the course can also be introduced. For example, the central dogma of biology can be presented whereby DNA is transcribed to RNA, that is further translated to protein. Importantly, the effect of CRISPR interference on blocking transcription, and that protein production will influence cellular metabolism and growth rates which in turn feeds back on to reaction rates in the system, can be introduced. With this knowledge to hand, we hope that even non-biologists will have the conceptual overview of the system details and genetic engineering the course will be focusing on.
To relate genetic engineering to synthetic biology, particular attention is given to the presentation of the DBTL cycle as this provides a useful conceptual overview of the course. As a motivating illustration (for the use) of the DBTL cycle, we provide lectures and computer practicals on genome-scale, constraint-based modelling of metabolism (of which the most common approach is flux balance analysis; Orth et al., 2010). We believe this to be beneficial for students in three ways. First, modelling metabolism at steady-state reduces the mathematical complexity of the system to a set of algebraic equations that students should be able to recognise from earlier mathematics education. Second, by using genome-scale, constraint-based modelling students learn how to compare models with data, e.g., simulated and experimental growth rates for well-studied bacteria, and using simulation methods to predict how to re-engineer a cell’s metabolism towards a desired goal or which pathways are important for metabolic function (as an example of the top-down Systems Biology approach). Finally, synthetic circuits are constructed within a growing cellular environment that is regulated by metabolism. By understanding how a cell can grow, we can later introduce to students how dilution due to cell growth impacts the function of genetic circuits (e.g., see Qian et al., 2017). These ideas will be required for the analysis and the understanding of more complex, non-linear, and time-dependent systems which will be presented in weeks two and three.
The computer practical introduces students to the Python computer programming language (and Jupyter notebooks) used in the course, and how to install packages such as COBRApy that are used in their project to help analyse their experimental methods. See the Jupyter notebooks Week1_Pr2_Thursday.ipynb and Week1_selfStudy_Friday.ipynb in the GitLab repository for example COBRApy tutorials that are based on those provided by the package developers (Ebrahim et al., 2013). We will discuss later how constraint-based modelling methods are discussed further in advanced courses but it is important to note here that the tutorial could also be provided in MATLAB using the COBRA toolbox if that is the teacher’s preference.
2.2.2 Week two: Designing a synthetic circuit and constructing an ODE model (Design)
With the mathematical concepts and tools provided in Week one, the course can now move on to (mathematically) designing a synthetic circuit. For this purpose, we have utilised the iBioSim platform (Watanabe et al., 2018). This package is a good example of ongoing developments in the synthetic biology field to create computer-aided design (CAD) tools for experimental practitioners and possesses a number of key features that we look to take advantage of, and can be used alongside design tools such as CELLO (Fontanarrosa et al., 2020).
1. In iBioSim, genetic circuits can be designed in a modular way using the notation for parts (promoters, ribosome binding sites, operons, stop codons, etc.) that Life Science students are taught in earlier courses.
2. iBioSim is connected to an online wikidata-style FAIR (findable, accessible, interoperable, reusable) database, SynBioHub, where information for relevant genetic parts are stored (McLaughlin et al., 2018). These databases contain descriptions of the part’s function, its DNA sequence, and some related reaction rates. This means that when parts are put together in iBioSim, the researcher can output a novel genetic sequence that can be engineered on plasmids and used experimentally.
3. iBioSim automatically constructs mathematical models of the designed system using the law of mass action and implementing time-scale separation arguments such as Hill functions to describe transcriptional regulation. These can then be simulated using ODE and stochastic model solvers to visualise model predictions. Finally, all designs are saved in systems biology-oriented markup languages (SBML and SBOL) that can be used with other software.
Using iBioSim software, we show students how they can design simple systems/modules (e.g., transcriptional regulation of a fluorescent reporter) that they can build further into their genetic toggle switch. We can then teach students further about the modelling process from the obtained mathematical equations. As with the use of COBRA tools in Python or MATLAB, iBioSim will likely develop further in the future, or other preferred CAD tools may be available for the course provider to take advantage of.
2.2.3 Week three: Simulating and fitting models to data (Learn)
In this last week dedicated to modelling, students learn how the designed ODEs obtained from iBioSim can be implemented into Python Jupyter notebooks. The purpose for this is to show students how models can be coded and fit to data in a hands-on manner (as this is very similar across multiple languages: Python, MATLAB, Octave, COPASI, Julia, and R). iBioSim and other similar software tools also include the potential to learn model structures and reaction rates from data (i.e., data fitting), but we do not utilise this functionality here. These skills are then advanced in further courses and thesis research (discussed below).
The week starts with a discussion of the use of Hill functions and how they arise, mathematically, through simplifying assumptions of the biological system. The resulting ODE models can then be coded into a Jupyter notebook and compared. If required, students can be provided with equations to account for cell population expansion and dilution rates—as obtained by flux balance analysis—that are required to explain the data correctly (the theory behind these equations can be described in advanced courses). Next, we discuss how model simulations can be compared to data using scoring functions. These scoring functions are then applied to find the most likely reaction rates (from, e.g., a Latin hypercube sample) underpinning our data. An important aspect to consider here are the bounds of feasible values that reaction rates can take. The synthetic biology community has already developed online calculator tools that can link genetic sequences with Gibbs free energy values and reaction rates (e.g., the RBS calculator for translation rates; Cetnar and Salis, 2021). Students can be taught about these tools, the underlying models that allow reaction rates to be calculated, and their use in designing systems—either for parameter fitting or initial simulations. Then, based on the comparisons between the simulations and data, we discuss how students can draw conclusions from their model: whether the model is an accurate representation of reality, how to design new experiments, and make model-based predictions. If time allows, or in further courses, students can be shown how to analyse their models using simulated sensitivity analysis, or taught further mathematical analysis to observe system behaviour across a viable range of reaction rate values, such as simulating bifurcation diagrams for toggle switches. These steps are then practically implemented in their Python scripts and can be reused and applied when they have performed experiments for their project report.
2.2.4 Week four: Experimentally building synthetic circuits (Build)
Week four is the start of the wet-lab activities in the build phase. Students learn about the main assembly methods used in synthetic biology, such as restriction/ligation-based, Gibson, Golden Gate and in vivo assembly methods; and genome editing tools including homologous recombination, recombineering, and CRISPR. Focus is given to the pros and cons of each technology and how their characteristics fulfil the engineering pillars of synthetic biology: standardisation, modularization, and abstraction (Endy, 2005). We found that providing students with schemes and self-study material for the different methods means that the lecture time is used for the discussion of these topics. The self-study material we use includes YouTube videos (Dy et al., 2019) and the use of computer software to simulate and visualise system assembly. Use of tools, such as Benchling (benchling.com), allows the students to visualise how the assembly occurs base-by-base in the real experiment. Although multiple software packages are available to simulate DNA assemblies, we chose Benchling because it is free, easy-to-use and facilitates the sharing of projects, folders and DNA sequences. The repository sequences for the toggle switch nodes and final vector can be found in the accompanying GitLab repository, exported from Benchling as Genbank files.
In our course, we decided to continue with the Golden Gate assembly method for practical teaching due to its wide use in synthetic biology, its straightforward protocol, and its high success rate. The students are provided with pSB1C3 plasmids each already containing one cloned toggle switch node from the published toggle switch system (pJ 2016.2_TS; Santos-Moreno et al., 2020). The pSB1C3 plasmid was chosen as it is the main repository vector used by, e.g., the iGEM Foundation for synthetic biology applications (http://parts.igem.org/Part:pSB1C3). From here, using the SEVA3.1 standard oligonucleotides (Damalas et al., 2020), students can rebuild the toggle switch in any SEVAb or converged plasmid using Golden Gate. Alternative plasmid systems could be used by other course providers (such as BioBrick/3A assembly, Rokke et al., 2014) depending on the teacher’s preference.
The comparison of the toggle switch performance in different standardised vectors may also make an interesting study project for students at the end of the course. After the students have performed the Golden Gate ligation and obtained successful colonies, the students perform PCR and gel electrophoresis to check the presence of their construct in cells. Students with a biological background are likely to have encountered these methods in other molecular courses and can aid the computational students. To circumvent problems with nucleic acid purification (Campbell et al., 2014), we recommend the use of commercial kits during these steps of the construction process. Although in our courses, students have a highly dispersed amount and qualities of the extracted DNA, this has not hindered the building of the final plasmid by the golden gate assembly, which in turn demonstrates the robustness of this assembly method. Detailed protocols can be found on the accompanying GitLab repository in week4_protocol.docx.
2.2.5 Week five: Characterising circuits (Test) and societal impacts of synthetic biology
In week five, students perform laboratory practicals corresponding to the test phase of the DBTL cycle. Our aim here is to show the students that the toggle switch can be changed from one state to another depending on the inputs used. Hence, they grow their selected positive clones for E. coli strains in LB media overnight containing the inducers required to trigger the toggle switch (in our case acyl homoserine lactone, AHL, turns the system ON and arabinose turns the system OFF). After washing and dilution (such that the optical density, OD, is 0.1), the cells are then added to fresh minimal media with the second inducer present to either switch the system OFF or ON, respectively (see the supplied protocols). Different concentrations of each inducer may be tested: in doing this, more data is available for later optimisation of the mathematical model and to provide validation datasets if students wish to use their model to make predictions. The students then distribute their samples across well plates suitable for fluorescence measurement. The plates are then placed in a plate reader programmed to measure the OD and fluorescence produced by green fluorescent protein (GFP). Once the results are obtained, they are distributed to the students for analysis. These datasets could be used in the final project that students work on in later weeks.
Furthermore in week five, students follow two lectures on the broader impacts of synthetic biology, including considering safety-by-design across the development process and responsible research and innovation. The students are presented with, and critically discuss unforeseen consequences of the novel synthetic biology technology. These range from the potential economic and environmental impacts as synthetic biology enables a shift from petrochemical to biobased economies, to far-reaching effects on, e.g., job displacements elsewhere in the world, ownership of (modified) biological resources, public perception of synthetic biology, co-creation and stakeholder engagement.
Finally, the students are given an exercise to read and present a scientific article on synthetic biology. Each student presents one article and is the first questioner to the presentation of another, as a way to ensure a structured debate and full participation of all students. This activity allows the students to find similarities with their conducted project, and see how the different parts of synthetic biology are combined in academic research. On the accompanying GitLab repository you can find instructions we provide to students. As an example, we have used the work of Carbonell et al. (2018) to highlight an example of the use of DBTL cycles within synthetic biology that students can discuss.
2.2.6 Modular structure of DBTL course
We remark that in our course organisation we do not strictly follow the DBTL cycle within the calendar overview of the course, but other educational practitioners could consider such a course redesign: we highlight in Figure 2 how that could be achieved. The key change would consist of moving discussion related to comparing mathematical and statistical models (simulations) with experimental data (the learn phase) from week three in our current design to week five. In this construction, a model of the toggle switch would be designed, the students can then enter the lab to build and test the system, before they return to their mathematical model to see what they learn about the system. Such choices, though, are often constrained by the availability of educational laboratories scheduled by the academic institution.
Other teaching teams may also consider gamifying the course structure (Dicheva et al., 2015). In this scenario, the design stage could become personalised or cooperative amongst team members (with a team constituting a subset of the class). Individuals or teams could then compete throughout the course to earn experience badges or complete the DBTL trajectory. The winners would be those that complete the DBTL cycle and are able to explain (dis)agreements between their model and experimental data (the start of a new DBTL cycle). As found in other technical subjects, such a course structure can help enthuse students about the topic under study (Iosup and Epema, 2014).
2.3 The research project
After being taught the fundamental methods and tools of Systems and Synthetic Biology during the first 5 weeks of the course, the students have learned how to design, construct and test a mathematical model of a genetic circuit. In weeks six and seven of our course, the students now need to put these skills together and apply them to model and test the CRISPR interference toggle switch (Santos-Moreno et al., 2020) experimentally constructed during the course. The two-weeks project consists of the following key steps:
1. Design of a mathematical model of the toggle switch that has been built experimentally during practicals in weeks four and five.
2. Estimation of reaction rates using online resources for, e.g., transcription, translation and degradation rates, and COBRApy for dilution rates if the cell population size is not controlled for in the experimental design. Note that we discuss dilution models in advanced courses, so the required equations are provided to students here.
3. Write a Python script to find optimal reaction rates for their model in relation to their data. If the experiments with the toggle switch have been successful then students can use their own data. If for some reason the experiment fails, the students will be provided data to work with from experiments performed by teachers.
4. Analyse the results of their mathematical model in relation to experimental data. To understand (possible) discrepancies between model results and experiment, students can reflect on: Hypothetical missing mechanisms that could be added to the model to improve the fit to data, predicting new experiments or matching their model to validation datasets, performing parameter sensitivity analysis relative to their data, or obtaining bifurcation plots to understand in which parameter regime the toggle switch functions as desired.
5. Discussion/suggestion of possible uses for the toggle switch to control biological systems (e.g., metabolism) or of new system designs to construct a more robust toggle switch system. A possible extension to this discussion is to consider ethical and/or biosafety aspects of such synthetic constructs in natural systems.
The results of the research need to be written as a short report. By developing a model to analyse their experimental results, the students will take into account all aspects of the DBTL cycle, mimicking higher-level scientific research. The results and the hypotheses developed by the students could, potentially, go on to inform future research efforts within the coordinating group - either by altering experimental protocols, or utilising well-designed tools for applications. The link to (thesis) research projects will be discussed below.
3 Examination and evaluation
3.1 Student examination
In the 2021/22 academic year at Wageningen University, 10 students (five B Sc., and five M.Sc.,) from Biotechnology (five students) and Biology (three students, the remaining two students were external students from other institutions) curricula followed the course Systems and Synthetic Biology that we have presented and discussed here. One of the BSc students dropped out of the course before the graded project and the short, written exam; the remaining nine students obtained grades between 7 and 8.5 out of 10 (average grade = 7.72 with standard deviation = 0.62; note that in the Dutch education system a rounded score of lower than six is a fail). Given the high average grade and small standard deviation, one could suggest that all the students in the course were able to recall and implement the key methodologies taught in the course. However, given that no students were able to score the highest grades (or, even, fail), future considerations for course design includes trying to ensure that students can reach high (or low) grades; one possibility could be to allow for more creativity within the project, e.g., by incorporating discussions of technology ethics and impact or model-driven experimental design. By allowing the high-achieving students to “spread their wings,” students will be able to obtain a wider range of grades within the course.
3.2 Course evaluation
Following the course (but before they had received their grades), the students were asked to complete a survey providing us with their thoughts about the course over three categories: course quality, student effort, and examination quality (Figure 3). These are ranked on a scale of 1–5, with one representing strong disagreement with a statement related to course quality, too low effort required to pass the course, or too easy an exam, and five representing strong agreement with, too high, and too hard, respectively. Based on the results of the survey, the students rated their course satisfaction very highly (average score = 4.4 out of 5, with standard deviation = 0.9) with all the students acquiring new knowledge (average score = 4.7, standard deviation = 0.5). The difficulty level of the course was given 3.3 out of 5 (standard deviation = 0.7), suggesting that students did not find the course too difficult or too easy, and this was also reflected in the difficulty of the exam that scored three out of 5 (standard deviation = 0). As with the course satisfaction, the quality of the examination was rated 4.6 out of 5 (standard deviation = 0.5).
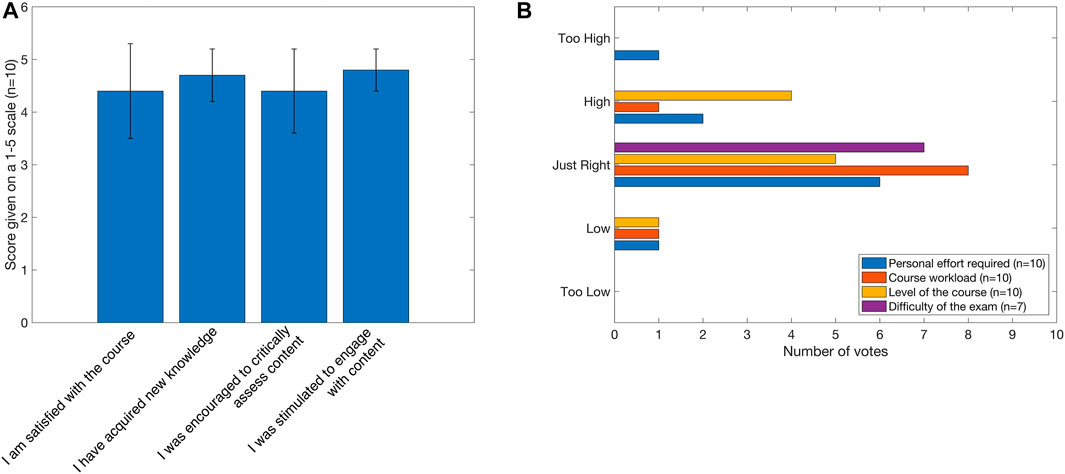
FIGURE 3. Summary statistics of course survey. (A) Students evaluated the course satisfaction level by rating each statement from one (low) to five (high) scores. Error bars represent standard deviation. (B) Students provided opinions on the course and exam by providing a rank (from “too low/easy” to “too high/hard”) for a set of statements. As some students did not sit the exam, n is less for this statement than others. The full survey and statements can be found in the GitLab repository.
In summary, from the 2021/22 class, all the students 1) learnt new concepts and ideas from Systems and Synthetic Biology that are not present in other parts of their curricula, 2) passed the course with average to above average grades, and 3) ranked the course highly in satisfaction surveys. Such positive results are encouraging for the future development of multidisciplinary educational courses at the B.Sc., and M.Sc., level.
4 Transitioning to advanced courses
The course we have presented here is an introductory gate-way course for students into the Systems and Synthetic Biology fields. In the 8 weeks, students will have been exposed to concepts related to data infrastructure and computer-aided design, modelling methods from Systems Biology, and experimental synthetic biology. Each of these topics requires its own in-depth course to deepen and expand the knowledge gained during the course presented here. Here we will discuss the content of hypothesised/desired courses in a Systems and Synthetic Biology curriculum (Figure 4; also see Cvijovic, et al., 2016).
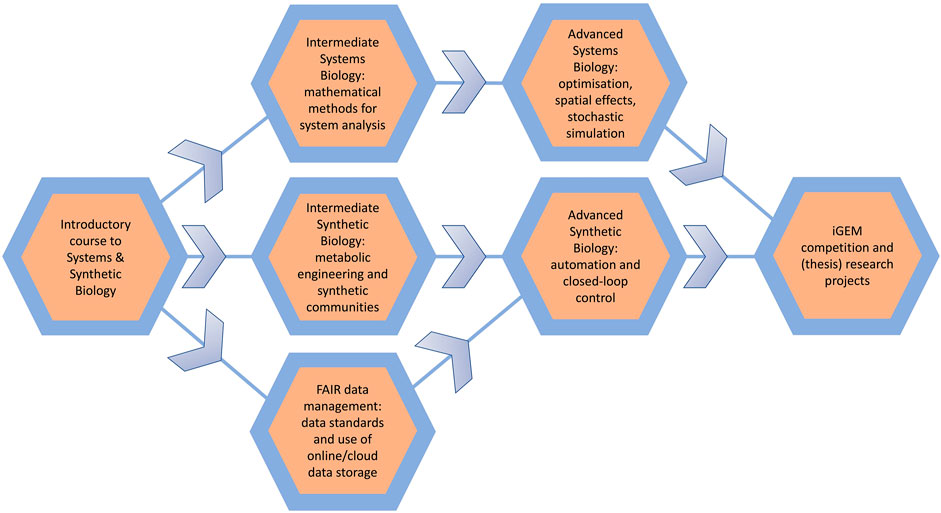
FIGURE 4. An overview of an idealised systems and synthetic biology course curriculum. Starting with the introductory course we have discussed in the main text, students could follow a range of further courses at an intermediate and then advanced level. Through one channel, students can learn more advanced systems biology topics and modelling methods; through a second channel, students could extend their laboratory experience towards automated closed-loop control systems; and in a third channel, students could deepen their knowledge further on open data and open science. Ultimately, the final goal should be for students to use the gained knowledge and skills within thesis research of the iGEM competition.
4.1 Multi-disciplinary synthetic biology
In the course outlined above, we have focused on the construction of a CRISPRi genetic circuit. Consequently, students have learnt fundamental experimental techniques such as plasmid design, Golden Gate assembly, agarose gel electrophoresis and polymerase chain reaction (PCR) purification, as well as measuring optical density (OD) using plate readers and circuit function from fluorescent protein readouts and plate readers. An envisioned advanced course should look to refresh and use these skills to tackle cutting-edge research questions (Aydin et al., 2022). This could be to construct circuitry to ensure condition-dependent survival of bacteria (Preston et al., 2016), to switch bacterial metabolism from growth-promoting to compound production phases (Izard et al., 2015), to compare circuit function and robustness across different organisms (see, e.g., Chatelle et al., 2018), or to generate synthetic microbial communities (Shahab et al., 2020).
Alongside the experimental implementation of these extended examples which highlight the uses of synthetic biology, new modelling approaches to aid system optimisation are required. Within an advanced course, students can learn how to create or use larger models of metabolic systems in extended ODE systems (Millard et al., 2017; Ahn-Horst et al., 2022), and take into account metabolic-controlled dilution effects in growing cell populations (de Jong et al., 2017). Advanced topics could then consider metabolic burden imposed on cells by synthetic circuits (Nikolados et al., 2019), resource competition of parts within a synthetic circuit (Qian et al., 2017), or modelling open- and closed-loop control systems (Milias-Argeitis et al., 2016). This would push students towards the current edge of synthetic biology research where increasingly automated experimental and modelling approaches are being combined to increase the speed and reproducibility of DBTL cycles (Carbonell et al., 2018).
4.2 Mathematical modelling and analysis
Based on the output of iBioSim, we teach the students in our course how to implement an ODE model in Python and simulate the system. However, mathematical analysis and model development goes much further than this in the Systems Biology field. Further courses on mathematical modelling should look to include topics such as: model building and understanding how to pick functions and terms within equations, fitting the model to desired quantitative or qualitative behaviours, how to reduce model complexity to allow for analysis (e.g., phase portraits, bifurcation diagrams, identifiability), and adapting the model to incorporate spatial or stochastic effects. These topics can be taught using analytical (hand-written) or numerical (computational) tools in multiple languages (Python, MATLAB, R, Octave, COPASI, or Julia). Through combining these methods, students will learn general skills that are applicable regardless of the future research problem or environment they face.
Importantly though, we have found that students are enthused to learn about modelling problems through research-driven approaches. In these instances, students are provided with an “open” research problem and can apply skills to understand how a biological system works (relative to available data) and to design new experiments that could be performed by a hypothetical laboratory scientist. As many different aspects of modelling need to be dealt with in order to reach this objective, modelling skills could be split over multiple follow-on courses. By focusing on producing research-style outputs, students see the benefit of Systems Biology approaches for both natural and synthetic systems.
4.3 FAIR infrastructure for data and models
One aspect that is touched on in our introductory course is the FAIR online storage of (biological) data and models. The tools we discuss within the course make use of computer-readable XML/SBML languages to improve the sharing and interoperability of data and models. In the first instance, iBioSim makes use of and can query the SynBioHub repository to obtain information related to the DNA parts and synthetic constructs used in a system design (e.g., DNA sequences, system function, and related measurements or publications). In the second instance, the final model design from iBioSim can be exported into SBML format such that models can then be simulated in other platforms (e.g., using the Tellurium package for Python; Medley et al., 2018). To modify a model, iBioSim can also make use of pre-existing SBML models found in the BioModels database (Watanabe et al., 2018; Malik-Sheriff et al., 2020).
Given the increasing importance of data and model reusability, one suggested follow-up course would be to go more in-depth with such wiki databases: their development, their usage, and how they can be queried by users. By using examples from the Systems and Synthetic Biology field, the envisioned course can highlight theoretical and practical skills in tandem, students will be enthused by ongoing developments in this research area. This is crucial in three respects as 1) future researchers need to understand the requirements for making their data FAIR, 2) they will be able to make use of already existing FAIR databases to automate and speed up their research or model development, and 3) the open research ethos of the Systems and Synthetic Biology fields will be encouraged from the earliest possibility in a research career.
4.4 Future research and the iGEM competition
The ultimate aim of any Synthetic Biology curriculum is to attract students to the field for future research-based careers, either in academia or in the private and public sectors. In this sense, providing students with multi-disciplinary skills from the outset and throughout the curriculum is imperative as cellular biology aims to replicate engineering disciplines in the future. To simulate and teach students about research careers, many university programmes include a thesis component that takes place towards the end of a student’s study. The thesis projects allow the students to bring together all they have learnt to develop their skills to understand a novel research problem. The courses outlined above should provide the training required for students to undertake, almost independently, thesis research and collaborate with other researchers to uncover novel biological insights. Notably, students may wish to continue their research into synthetic toggle switches for further modelling analysis, experimental applications, or as a sub-module within a larger system.
Within the Synthetic Biology field, though, the iGEM (international genetically engineered machine, see www.igem.org) competition provides student teams with a unique opportunity to design their own research project. As part of this, the teams need to consider philosophical, ethical, and societal concerns related to synthetic biology research, going beyond the practical execution of projects. We have discussed our views of iGEM as an educational tool elsewhere (Garcia-Morales et al., 2022), and the ability to incorporate iGEM within educational curricula. Importantly, the iGEM competition is a key driver of synthetic biology research both in academia and industry (Kelwick et al., 2015). As such, adding iGEM to the final stages of a synthetic biology curriculum adds a novel extra dimension that is not seen in many other educational programmes, generating strong researchers for the future.
5 Concluding remarks
In this paper we have discussed the organisation of an introductory synthetic biology course. The course is aimed at final year B.Sc., and early M.Sc., students as a means of expanding on concepts that students have been exposed to earlier in their education. By combining experimental and theoretical approaches, students are introduced to multi-disciplinary concepts for, potentially, the first time. To design the course we use a research-driven approach, focusing on methodologies at the forefront of the Systems and Synthetic Biology fields. In addition, we include how synthetic biology technologies have a wider impact on society. We believe this applied approach to education 1) increases student satisfaction as they are able to visualise how research will be conducted in their future lives, and 2) develops more general cognitive problem-solving skills that can be translated to other fields. This does, of course, imply that some topics or concepts cannot be covered in detail or are not included in the course—for example, theoretical assumptions underlying statistical tools often used for data analysis, technical implementation of numerical methods, or the biochemistry of fluorescent reporters and CRISPR systems often used in synthetic biology research. Our hope is that this knowledge is developed elsewhere in a curriculum or that strong students are able to research such details independently given the cognitive skills developed during this course. Ultimately, we believe our modular course design is flexible enough to be translated to other institutions interested in alternative synthetic biology research problems, providing the next generation of synthetic biologists with the skills to push the field beyond its current frontiers.
6 Related course materials
Experimental protocols, Jupyter notebooks, detailed course schedule, and other material is available in the course GitLab repository https://gitlab.com/wurssb/systemsand-synthetic-biology.
Author contributions
RS and LG wrote the initial manuscript. RS and LG created figures. RS, LG, VM, and ES edited the manuscript.
Funding
LG and VM thankfully acknowledge financial support from the EU-H2020 project IBISBA.eu, contracts no. 730976 and 871118.
Acknowledgments
We would like to acknowledge the students that followed the course during the previous academic years and helped us shape the course into its current form. We are grateful to Cherrie Creations (aW5mb0BjaGVycmllY3JlYXRpb25zLm5s) for designing Figure 1. Additionally, thanks to Pedro Fontanarrosa, Lukas Buecherl, and Chris Myers for iBioSim support and advice when developing our models. Furthermore, we are grateful to Yolanda Schaerli for providing the plasmids pJ2018 (Addgene plasmid #140665), pJ2077.2 (Addgene plasmid #140666), and pJ2076.2_TS (Addgene plasmid #140667). Finally, we would like to kindly thank our colleagues and lecturers at the Laboratory of Systems and Synthetic Biology (Wageningen University and Research, Netherlands) and collaborators for discussion on course development, not just for this course but for others in our educational portfolio.
Conflict of interest
Author LG was employed by the company MSD Animal Health and author VM was employed by the company LifeGlimmer GmbH.
The remaining authors declare that the research was conducted in the absence of any commercial or financial relationships that could be construed as a potential conflict of interest.
Publisher’s note
All claims expressed in this article are solely those of the authors and do not necessarily represent those of their affiliated organizations, or those of the publisher, the editors and the reviewers. Any product that may be evaluated in this article, or claim that may be made by its manufacturer, is not guaranteed or endorsed by the publisher.
References
Ahn-Horst, T. A., Mille, L., Sun, G., Morrison, J. H., and Covert, M. W. (2022). An expanded whole-cell model of E. coli links cellular physiology with mechanisms of growth rate control. NPJ Syst. Biol. Appl. 8, 30. doi:10.1038/s41540-022-00242-9
Akkerman, S. F., and Bakker, A. (2011). Boundary crossing and boundary objects. Rev. Educ. Res. 81, 132–169. doi:10.3102/0034654311404435
Akkerman, S. F. (2011). Learning at boundaries. Int. J. Educ. Res. 50, 21–25. doi:10.1016/j.ijer.2011.04.005
Aydin, O., Passaro, A. P., Raman, R., Spellicy, S. E., Weinberg, R. P., Kamm, R. D., et al. (2022). Principles for the design of multicellular engineered living systems. Apl. Bioeng. 6, 010903. doi:10.1063/5.0076635
Bhardwaj, A., and Nain, V. (2021). TALENs-an indispensable tool in the era of CRISPR: A mini review. J. Genet. Eng. Biotechnol. 19, 125. doi:10.1186/s43141-021-00225-z
Bloom, B. S. (1956). Taxonomy of educational objectives: The classification of educational goals. New York: Longmans Green & Co.
Campbell, A. M., Eckdahl, T., Cronk, B., Andresen, C., Frederick, P., Huckuntod, S., et al. (2014). pClone: Synthetic biology tool makes promoter research accessible to beginning biology students. Lse 13, 285–296. doi:10.1187/cbe.13-09-0189
Carbonell, P., Jervis, A. J., Robinson, C. J., Yan, C., Dunstan, M., Swainston, N., et al. (2018). An automated design-build-test-learn pipeline for enhanced microbial production of fine chemicals. Commun. Biol. 1, 66. doi:10.1038/s42003-018-0076-9
Cetnar, P. D., and Salis, H. M. (2021). Systematic quantification of sequence and structural determinants controlling mRNA stability in bacterial operons. ACS Synth. Biol. 10, 318–332. doi:10.1021/acssynbio.0c00471
Chandran, D., Copeland, W. B., Sleight, S. C., and Sauro, H. M. (2008). Mathematical modeling and synthetic biology. Drug Discov. Today Dis. Models 5, 299–309. doi:10.1016/j.ddmod.2009.07.002
Chatelle, C., Ochoa-Fernandez, R., Engesser, R., Schneider, N., Beyer, H. M., Jones, A. R., et al. (2018). A green-light-responsive system for the control of transgene expression in mammalian and plant cells. ACS Synth. Biol. 7, 1349–1358. doi:10.1021/acssynbio.7b00450
Cvijovic, M., Höfer, T., Aćimović, J., Alberghina, L., Almaas, E., Besozzi, D., et al. (2016). Strategies for structuring interdisciplinary education in systems biology: An European perspective. NPJ Syst. Biol. Appl. 2, 16011. doi:10.1038/npjsba.2016.11
Damalas, S. G., Batianis, C., Martin-Pascual, M., Lorenzo, V., and Martins dos Santos, V. A. P. (2020). Seva 3.1: Enabling interoperability of DNA assembly among the SEVA, BioBricks and type IIS restriction enzyme standards. Microb. Biotechnol. 13, 1793–1806. doi:10.1111/1751-7915.13609
de Jong, H., Casagranda, S., Giordano, N., Cinquemani, E., Ropers, D., Geiselmann, J., et al. (2017). Mathematical modelling of microbes: Metabolism, gene expression and growth. J. R. Soc. Interface. 14, 20170502. doi:10.1098/rsif.2017.0502
Delebecque, C., and Philp, J. (2015). Training for synthetic biology jobs in the new bioeconomy. Science. doi:10.1126/science.caredit.a1500143
Dicheva, D., Dichev, C., Agre, G., and Angelova, G. (2015). Gamification in education: A systematic mapping study. J. Educ. Techno. Soc. 18, 75–88.
Dy, J., Aurand, E. R., and Friedman, D. C. (2019). YouTube resources for synthetic biology education. Synth. Biol. (Oxf) 4, ysz022. doi:10.1093/synbio/ysz022
Eager, E. A., Peirce, J., and Barlow, P. (2014). Math bio or biomath? Flipping the mathematical biology classroom. Lett. Biomath I, 139–155.
Ebrahim, A., Lerman, J. A., Palsson, B. O., and Hyduke, D. R. (2013). COBRApy: Constraints-based reconstruction and analysis for Python. BMC Syst. Biol. 7, 74. doi:10.1186/1752-0509-7-74
Farny, N. G. (2018). A vision for teaching the values of synthetic biology. Trends Biotechnol. 36, 1097–1100. doi:10.1016/j.tibtech.2018.07.019
Fontanarrosa, P., Doosthosseini, H., Borujeni, A. E., Dorfan, Y., Voigt, C. A., and Myers, C. (2020). Genetic circuit dynamics: Hazard and glitch analysis. ACS Synth. Biol. 9, 2324–2338. doi:10.1021/acssynbio.0c00055
Garcia-Morales, L., Savelkoul, N. H. A., Robaey, Z., Claassens, N. J., Staals, R. H. J., and Smith, R. W. (2022). Ten simple rules for building an enthusiastic iGEM team. PLOS Comp. Biol. 18, e1009916.
Honegger, K., and de Bivort, B. (2018). Stochasticity, individuality and behavior. Curr. Biol. 28, R8–R12. doi:10.1016/j.cub.2017.11.058
Hutchison, C. A., Chuang, R. Y., Noskov, V. N., Assad-Garcia, N., Deerinck, T. J., Ellisman, M. H., et al. (2016). Design and synthesis of a minimal bacterial genome. Science 351, aad6253. doi:10.1126/science.aad6253
Iosup, A., and Epema, D. (2014). “An experience report on using gamification in technical higher education,” in Proceedings of the 45th ACM technical symposium on computer science education (New York: Association for Computing Machinery). doi:10.1145/2538862.2538899
Izard, J., Gomez Balderas, C. D. C., Ropers, D., Lacour, S., Song, X., Yang, Y., et al. (2015). A synthetic growth switch based on controlled expression of RNA polymerase. Mol. Syst. Biol. 11, 840. doi:10.15252/msb.20156382
Jumper, J., Evans, R., Pritzel, A., Green, T., Figurnov, M., Ronneberger, O., et al. (2021). Highly accurate protein structure prediction with AlphaFold. Nature 596, 583–589. doi:10.1038/s41586-021-03819-2
Jusiak, B., Cleto, S., Perez-Piñera, P., and Lu, T. K. (2016). Engineering synthetic gene circuits in living cells with CRISPR technology. Trends Biotechnol. 34, 535–547. doi:10.1016/j.tibtech.2015.12.014
Kampers, L. F. C., Asin-Garcia, E., Schaap, P. J., Wagemakers, A., and Martins dos Santos, V. A. P. (2021). From innovation to application: Bridging the valley of death in industrial biotechnology. Trends Biotechnol. 39, 1240–1242. doi:10.1016/j.tibtech.2021.04.010
Kelwick, R., Bowater, L., Yeoman, K. H., and Bowater, R. P. (2015). Promoting microbiology education through the iGEM synthetic biology competition. FEMS Microbiol. Lett. 362, fnv129. doi:10.1093/femsle/fnv129
Kitano, H. (2002). Systems biology: A brief overview. Science 295, 1662–1664. doi:10.1126/science.1069492
Kolbs, D. A. (1984). Experiential learning: Experience as the source of learning and development. Englewood Cliffs, NJ: Prentice-Hall.
Konermann, S., Brigham, M. D., Trevino, A. E., Hsu, P. D., Heidenreich, M., Le Cong, L., et al. (2013). Optical control of mammalian endogenous transcription and epigenetic states. Nature 500, 472–476. doi:10.1038/nature12466
Kuldell, N. (2007). Authentic teaching and learning through synthetic biology. J. Biol. Eng. 1, 8. doi:10.1186/1754-1611-1-8
Malik-Sheriff, R. S., Glont, M., Nguyen, T. V. N., Tiwari, K., Roberts, M. G., Xavier, A., et al. (2020). BioModels-15 years of sharing computational models in life science. Nucleic Acids Res. 48, D407–D415. doi:10.1093/nar/gkz1055
McLaughlin, J. A., Myers, C. J., Zundel, Z., Mısırlı, G., Zhang, M., Ofiteru, I. D., et al. (2018). SynBioHub: A standards-enabled design repository for synthetic biology. ACS Synth. Biol. 7, 682–688. doi:10.1021/acssynbio.7b00403
Medley, J. K., Choi, K., König, M., Smith, L., Gu, S., Hellerstein, J., et al. (2018). Tellurium notebooks-An environment for reproducible dynamical modeling in systems biology. PLoS Comput. Biol. 14, e1006220. doi:10.1371/journal.pcbi.1006220
Millard, P., Smallbone, K., and Mendes, P. (2017). Metabolic regulation is sufficient for global and robust coordination of glucose uptake, catabolism, energy production and growth in Escherichia coli. PLoS Comput. Biol. 13, e1005396. doi:10.1371/journal.pcbi.1005396
Milias-Argeitis, A., Rullan, M., Aoki, S. K., Buchmann, P., and Khammash, M. (2016). Automated optogenetic feedback control for precise and robust regulation of gene expression and cell growth. Nat. Commun. 7, 12546.
Miskovic, L., Alff-Tuomala, S., Soh, K. C., Barth, D., Salusjärvi, L., Pitkänen, J. P., et al. (2017). A design-build-test cycle using modeling and experiments reveals interdependencies between upper glycolysis and xylose uptake in recombinant S. cerevisiae and improves predictive capabilities of large-scale kinetic models. Biotechnol. Biofuels 10, 166. doi:10.1186/s13068-017-0838-5
Nikolados, E. M., Weiße, A. Y., Ceroni, F., and Oyarzún, D. A. (2019). Growth defects and loss-of-function in synthetic gene circuits. ACS Synth. Biol. 8, 1231–1240. doi:10.1021/acssynbio.8b00531
Nurk, S., Koren, S., Rhie, A., Rautiainen, M., Bzikadze, A. V., Mikheenko, A., et al. (2022). The complete sequence of a human genome. Science 376, 44–53. doi:10.1126/science.abj6987
Orth, J. D., Thiele, I., and Palsson, B. O. (2010). What is flux balance analysis? Nat. Biotechnol. 28, 245–248. doi:10.1038/nbt.1614
Preston, M. A., Pimentel, B., Bermejo-Rodríguez, C., Dionne, I., Turnbull, A., and de la Cueva-Méndez, G. (2016). Repurposing a prokaryotic toxin-antitoxin system for the selective killing of oncogenically stressed human cells. ACS Synth. Biol. 5, 540–546. doi:10.1021/acssynbio.5b00096
Qian, Y., Huang, H. H., Jiménez, J. I., and Del Vecchio, D. (2017). Resource competition shapes the response of genetic circuits. ACS Synth. Biol. 6, 1263–1272. doi:10.1021/acssynbio.6b00361
Robeva, R., and Laubenbacher, R. (2009). Mathematical biology education: Beyond calculus. Science 325, 542–543. doi:10.1126/science.1176016
Røkke, G., Korvald, E., Pahr, J., Oyås, O., and Lale, R. (2014). BioBrick assembly standards and techniques and associated software tools. Methods Mol. Biol. 1116, 1–24.
Santos-Moreno, J., Tasiudi, E., Kusumawardhani, H., Stelling, J., and Schaerli, Y. (2022). Synthetic genotype networks. bioRxiv. doi:10.1101/2022.09.01.506159
Santos-Moreno, J., Tasiudi, E., Stelling, J., and Schaerli, Y. (2020). Multistable and dynamic CRISPRi-based synthetic circuits. Nat. Commun. 11, 2746. doi:10.1038/s41467-020-16574-1
Sauter, T., Bintener, T., Kishk, A., Presta, L., Prohaska, T., Guignard, D., et al. (2022). Project-based learning course on metabolic network modelling in computational systems biology. PLoS Comput. Biol. 18, e1009711. doi:10.1371/journal.pcbi.1009711
Shahab, R. L., Brethauer, S., Davey, M. P., Smith, A. G., Vignolini, S., Luterbacher, J. S., et al. (2020). A heterogeneous microbial consortium producing short-chain fatty acids from lignocellulose. Science 369, eabb1214. doi:10.1126/science.abb1214
Venter, C., and Cohen, D. (2004). The century of biology. New Perspect. Q. 21, 73–77. doi:10.1111/j.1540-5842.2004.00701.x
Vilanova, C., and Porcar, M. (2019). Synthetic microbiology as a source of new enterprises and job creation: A mediterranean perspective. Microb. Biotechnol. 12, 8–10. doi:10.1111/1751-7915.13326
Watanabe, L., Nguyen, T., Zhang, M., Zundel, Z., Zhang, Z., Madsen, C., et al. (2018). iBioSim 3: a tool for model-based genetic circuit design. ACS Synth. Biol. 8, 1560–1563. doi:10.1021/acssynbio.8b00078
Keywords: systems biology, synthetic biology, education, DBTL, genetic circuit
Citation: Smith RW, Garcia-Morales L, Martins dos Santos VAP and Saccenti E (2022) Research-driven education: An introductory course to systems and synthetic biology. Front. Syst. Biol. 2:981800. doi: 10.3389/fsysb.2022.981800
Received: 29 June 2022; Accepted: 07 September 2022;
Published: 23 September 2022.
Edited by:
Gurkan Bebek, Case Western Reserve University, United StatesReviewed by:
Prashanth N. Suravajhala, Amrita Vishwa Vidyapeetham University, IndiaTara Deans, The University of Utah, United States
Copyright © 2022 Smith, Garcia-Morales, Martins dos Santos and Saccenti. This is an open-access article distributed under the terms of the Creative Commons Attribution License (CC BY). The use, distribution or reproduction in other forums is permitted, provided the original author(s) and the copyright owner(s) are credited and that the original publication in this journal is cited, in accordance with accepted academic practice. No use, distribution or reproduction is permitted which does not comply with these terms.
*Correspondence: Robert W. Smith, cm9iZXJ0MS5zbWl0aEB3dXIubmw=
†These authors have contributed equally to this work and share first authorship