- 1Department of Mechanical and Production Engineering, Guru Nanak Dev Engineering College, Ludhiana, India
- 2Jadara Research Center, Jadara University, Irbid, Jordan
- 3Department of Computer Science and Engineering, Guru Nanak Dev Engineering College, Ludhiana, India
- 4Department of Information Technology, Guru Nanak Dev Engineering College, Ludhiana, India
- 5Management and Science University, Shah Alam, Malaysia
- 6Department of Mechanical Engineering, Graphic Era (Deemed to be University), Dehradun, India
- 7Department of Chemistry and Biochemistry, School of Sciences, JAIN (Deemed to be University), Bangalore, India
- 8Department of Electrical Engineering, Chandigarh University, Mohali, India
- 9Chitkara University Institute of Engineering and Technology, Centre for Research Impact & Outcome, Chitkara University, Rajpura, India
- 10Department of Pharmacy, Mazaya University College, Dhiqar, Iraq
- 11Laboratories Techniques Department, College of Health and Medical Techniques, Al-Mustaqbal University, Babylon, Iraq
- 12Department of Engineering, FH Campus Wien - University of Applied Sciences, Vienna, Austria
This review provides a thorough and organized overview of machine learning (ML) applications in predicting heart disease, covering technological advancements, challenges, and future prospects. As cardiovascular diseases (CVDs) are the leading cause of global mortality, there is an urgent demand for early and precise diagnostic tools. ML models hold considerable potential by utilizing large-scale healthcare data to enhance predictive diagnostics. To systematically investigate this field, the literature is organized into five thematic categories such as “Heart Disease Detection and Diagnostics,” “Machine Learning Models and Algorithms for Healthcare,” “Feature Engineering and Optimization Techniques,” “Emerging Technologies in Healthcare,” and “Applications of AI Across Diseases and Conditions.” The review incorporates performance benchmarking of various ML models, highlighting that hybrid deep learning (DL) frameworks, e.g., convolutional neural network-long short-term memory (CNN-LSTM) consistently outperform traditional models in terms of sensitivity, specificity, and area under the curve (AUC). Several real-world case studies are presented to demonstrate the successful deployment of ML models in clinical and wearable settings. This review showcases the progression of ML approaches from traditional classifiers to hybrid DL structures and federated learning (FL) frameworks. It also discusses ethical issues, dataset limitations, and model transparency. The conclusions provide important insights for the development of artificial intelligence (AI) powered, clinically applicable heart disease prediction systems.
1 Introduction
1.1 Background of the study
Cardiovascular diseases (CVDs) cause around 17.9 million deaths each year, accounting for 32% of deaths worldwide. Heart disease continues to be one of the most significant health problems globally and nationally, as it is the leading cause of death around the globe and in the US. In 2021 alone, coronary heart disease was accountable for approximately 9 million deaths. In the US, coronary heart disease caused 1 out of 5 deaths in 2022, affecting all genders and races. The magnitude of this issue is enormous; in the United States alone, heart disease caused approximately two hundred and 52.2 billion dollars in direct and indirect costs from 2019 to 2020 (Khan Minhas et al., 2024). The prevalence of CVDs in the US is anticipated to increase sharply, as 61% of adults are expected to be hypertensive by 2050. The worldwide burden of CVDs is expected to rise by 90% from 2025 to 2050, increasing the number of deaths from 20.5 million in 2025 to 35.6 million by 2050. Therefore, immediate attention needs to be put towards effective heart disease preventive measures, greater detection capabilities, and fairness in healthcare access (Roth et al., 2020; Al-Ajlouni et al., 2024).
Many patients can be kept alive through effective healthcare interventions. This, however, requires early detection (Ferdous Azam and Sarwar, 2023). By taking proactive measures, one can help alleviate the bad consequences of the disease, improve the possible prognosis, and save money to be spent on treating the problem. Unfortunately, most diagnostic methods, such as Electrocardiogram (ECG), echocardiograms, and stress testing, need considerable time and skill to administer, and even then, accurate diagnosis may still not be achieved (Dala Ali et al., 2023; Faraji et al., 2023). Such limitations are even more pronounced in underdeveloped areas where such facilities are hard to come by. Machine Learning (ML), a subfield of artificial intelligence (AI), provides solutions to such problems (Pathirana et al., 2018; Pathirana et al., 2019). Complex ML algorithms can recognize intricate structures and correlations existing within a vast data set that are not readily available using traditional techniques. Such an attribute enables chronic diseases of the heart to be diagnosed at intervals much earlier than is possible when patients start showing symptoms. Thus, ML can facilitate the adoption of preventive measures and strides towards a patient-centered approach. Further, ML gives global health a powerful tool for applying affordable and efficient diagnostic technology to populations that need it most (Naruka et al., 2022). Figure 1 depicts the worldwide prevalence (in millions) of major cardiovascular conditions as of 2021. Coronary heart disease remains the most prevalent, impacting roughly 250 million people, followed by peripheral arterial disease (110 million), stroke (94 million), and atrial fibrillation (53 million) (Jagannathan et al., 2019). These figures highlight the significant global challenge posed by CVDs and emphasize the urgent need for effective predictive models driven by ML and AI to facilitate early diagnosis and prompt intervention. Incorporating these technologies into healthcare systems can significantly reduce mortality and enhance patient outcomes.
1.2 Role of machine learning in healthcare
The adoption of electronic health records (EHRs) and wearable devices, and sophisticated imaging technologies is aiding the healthcare industry in data management (Bai and Mardini, 2024). The ability of ML to use such data to improve clinical processes and patient interaction is astounding. In predicting heart disease, diverse data sources are harnessed by ML models such as (Pathirana et al., 2019; Dissanayake and Johar, 2023):
• Clinical Data: Data about the patient that includes demographics, medical history, lab results, and medications.
• Imaging Data: Echocardiograms, angiograms, and computed tomography (CT) scans.
• Biometric Signals include ECG, heart rate variability, and blood pressure.
• Data from wearable devices includes daily physical activities, sleep patterns, and vital signs.
Devices that classify people as high risk and low risk of heart disease are based on supervised learning techniques like random forest (RF), support vector machine (SVM), and neural network (NN). Slicers of the ML set are DL, which enhances a machine’s learning capabilities. For instance, convolutional neural network (CNN) has been used to identify arrhythmias from ECG signals with great precision. Furthermore, clustering techniques consider the unsupervised learning approach in which algorithms detect groups within a patient population that can be linked to specific risk levels or responses to treatment, thus paving the way for targeted medicine (Dissanayake and Johar, 2021; Dissanayake et al., 2023). Another innovative area is the application of reinforcement learning (RL) to improve treatment plans and the allocation of resources in healthcare settings. Figure 2 depicts ML in heart disease prediction, from data collection to model deployment (Nadeem et al., 2021; Kwon and Dong, 2022). Figure 3 presents an integrated heart disease prediction (Siramshetty et al., 2018; Benhar et al., 2020).
1.3 Objectives and scope of the review
The review aims to incorporate findings from previous research studies on heart diseases while creating, developing, and applying ML technologies that predict heart diseases. Grouping these studies into thematic clusters may help understand the advancements in the field, highlight strengths and challenges, and lay out the following objectives.
• Categorization of Research: Formulating a primary information scheme by grouping the studies into five main clusters.
• Analysis of ML Models: Performed in-depth analysis of the models in terms of algorithms, techniques, components of the systems, and their merits and demerits alongside real-world applications.
• Feature Engineering and Optimization: Striving to improve model performance through feature selection, dimensionality reduction, and hyperparameter tuning.
• Emerging Technologies: Exploiting the effects of innovations such as FL, quantum computing (QC), and Internet of Things (IoT) devices on the diagnostics of heart diseases.
• Other Applications of AI: AI’s involvement in treating diseases like cancer, diabetes, and other neurological disorders should be emphasized to better understand how heart disease prediction can be approached.
This review proposes to find patterns, gaps, and trends by incorporating these clusters in literature patterns to provide better insights for evolving studies and their implementations.
1.4 Comparison with existing literature and novel contributions
There is a substantial body of literature on ML applications in the healthcare sector, with review articles examining ML usage in healthcare diagnoses, predictions, and treatments. The literature is extensive. While many studies have been conducted, a gap remains in the focus on heart disease prediction through ML systems. We directly compare what this review achieves to existing works to address this gap.
1.4.1 Comparative analysis of related reviews
To illustrate how this review differentiates from prior works, Table 1 compares key literature, focusing on scope, methodology, datasets, and technological advancements.
1.4.2 Novel contributions of this review
Though other reviews provide essential insights into ML-based heart disease prediction, they tend to suffer from some drawbacks. Unlike other works, our work is unique in the following aspects.
1.4.2.1 Newer dataset analysis
○ This review covers newer datasets such as IoT-based healthcare data, wearable sensor datasets, and FL-enabled datasets, unlike other reviews focusing on classical datasets like UCI heart disease data and Framingham data.
○ Covers real regulatory challenges such as imbalance and noise.
1.4.2.2 Emerging technology integration
This review deeply analyzes new emerging AI tools like:
○ FL—privacy-preserving ML.
○ QC—high-speed disease modeling.
○ Explainable AI (XAI)—model understanding and trust.
○ Other reviews hardly address the confluence of AI with regulatory frameworks like General Data Protection Regulation (GDPR), Food and Drug Administration (FDA), and Health Insurance Portability and Accountability Act (HIPAA).
1.4.2.3 Ethical and regulatory considerations
○ Covers biases and patient privacy ML in healthcare ethics issues and explainability.
○ Provides an in-depth discussion of the legal aspects of AI implementation in healthcare.
1.4.2.4 Structured thematic classification
○ Unlike other systematic reviews, this piece of work classifies and indexes research into 5 different thematic clusters.
○ Methods and Algorithms of Heart Disease Detection and Diagnostics.
○ ML Models and Algorithms.
○ Emerging Feature Molding and Engineering.
○ Advanced Emerging Technology.
○ Multi-Disease AI Technology Applications.
1.4.2.5 Bridging the gap between research and clinical application
○ Prior works focus primarily on ML model accuracy, while this review focuses on actual clinical application.
○ Explains how different hospitals, medical practitioners, and policymakers can use ML-based systems for diagnosis in real-life settings.
1.4.3 Heatmap provides feature correlations
A heatmap is used in a dataset with heart disease features to represent the relationships of different attributes. Redundancy may exist for the features with high correlations, such as cholesterol and blood pressure, but weakly correlated features suggest that they would be independent in their ability to predict. Figure 4 presents a feature correlation heatmap that depicts the relationships between essential clinical variables like cholesterol, blood pressure, and age, which are vital for enhancing feature selection in heart disease prediction models.
• Proposition: ‘Blood Pressure and Cholesterol,’ a high correlation suggests potential multicollinearity and multivariate relationships.
• Counter Proposition: ‘Age and Resting ECG,’ the low correlation suggests a weak direct association.
• Supporting Proposition: ‘Smoking and Heart disease’ have a moderate correlation, which supports known medical findings.
2 Methodology
2.1 Literature search and inclusion criteria
The review methodology entailed a comprehensive literature search. The search involved a comprehensive query in Scopus using the search string:
((TITLE-ABS-KEY (‘heart disease prediction’) AND (‘ML’) OR (‘machine learning’)) AND (LIMIT-TO (DOCTYPE, “ar”)) AND (LIMIT-TO (LANGUAGE, “English”))).
It filtered relevant articles on heart disease prediction using ML, limited to English-language articles and journal articles. The curated results form the foundation for the insights and analyses presented in this review. Studies were excluded if they lacked adequate methodological detail, were not peer-reviewed, or were focused on unrelated topics. Quantitative and qualitative insights were extracted to identify trends, assess proposed methods’ effectiveness, and uncover existing research gaps.
2.2 Classification of clusters
The identified studies have been classified into five major clusters based on their primary focus using keywords. Table 2 depicts keywords considered and cluster names based on keywords and the occurrence of keywords. The first cluster, ‘Heart Disease Detection and Diagnostics’, includes advanced research on diagnostics and predictions concerning heart-related conditions. The second cluster, ‘Machine Learning Models and Algorithms for Healthcare,’ studies the different types of ML model frameworks, including supervised and DL models. The third cluster, ‘Feature Engineering and Optimization Techniques,’ discusses papers on feature selection, dimensionality reduction, and other optimization methods in model performance improvement. The fourth cluster, ‘Emerging Technologies in Healthcare’, reviews articles on modern innovations like QC, FL, and IoT in heart disease prediction and other applications. The last or fifth cluster, ‘Applications of AI Across Diseases and Conditions,’ gives more context by including AI’s applications in managing and predicting other diseases such as cancer, diabetes, and neurological disorders. This categorization is shown in Figure 5, facilitating a more profound literature review and allowing a better understanding of each cluster’s contributions and limitations.
3 Cluster wise insights: literature review
3.1 Cluster 1: heart disease detection and diagnostics
Technological advancements and methodologies provide innovative approaches and tools for the early detection and prediction of cardiovascular ailments. This group focuses on new imaging and ML model development, which improves diagnostic precision and appropriate timing of interventions to improve the prognosis for patients. Heart disease displays itself as more than just coronary artery disease (CAD); it includes arrhythmias and even heart failure. Therefore, it is essential to stress the multi-dimensional approach to conditions related to heart diseases. As outlined in Figure 6, critical steps are monitoring ECG for electrical activity, echocardiograms for the anatomical view, stress tests for function, cardiac catheterization for the coronary details, and blood tests for the troponin marker, along with the cholesterol marker, which are the most important for attention.
Table 3 shows key insights and performance metrics in heart disease detection and diagnostics. The classification of heart disease proved accurate with DL algorithms and Sand Cat Swarm Optimization (SCSO) for feature selection. Important features were identified using patient pathology data, and models, including CNN, PCA, Restricted Boltzmann Machine (RBM), and deep convolutional generative adversarial networks (DCGAN), analyzed intricate correlations that improved the accuracy of predictions. The method enhanced the reliability of heart disease prognosis through metrics such as accuracy and F1-score (Baviskar et al., 2023). The detection of CVD was improved using both RF and eXtreme Gradient Boosting (XGB) on ECG datasets, particularly Physionet 2016, PASCAL, and MIT-BIH. Pre-processing feature extraction with empirical wavelet transform (EWT), discrete wavelet transform (DWT), and SHapley Additive exPlanations (SHAP) will improve a prediction model’s accuracy, and a significant peak can reach up to 98.25 AUC for XGB-based proposed models (Majhi and Kashyap, 2024). A novel Wolf-based Generative Adversarial System (WbGAS), was developed to classify heart diseases, using ECG data to identify normal sinus rhythm, arrhythmia, and congestive heart failure (Goud et al., 2024). A hyperparameter-tuned CNN-based Inception Network model was created to diagnose heart disorders with heart sound data from standard repositories. The model achieves 99.65% accuracy, 98.8% sensitivity, and 98.2% specificity, surpassing the other classifiers (Roy et al., 2024). An electronic stethoscope has been developed, integrated with Raspberry Pi 4B and a CNN-based EfficientNet-B3 model for diagnosing valvular heart diseases. The system reported an accuracy of 99.35%, sensitivity of 98.84%, and specificity of 98.23%, with real-time Phonocardiogram (PCG) signal analysis and cloud-based data storage (Roy et al., 2023). Fatima and Siddiqi (2024) evaluated ML techniques for predicting Myocardial Infarction (MI) and analyzing risk factors using a dataset of 350 individuals, including MI and non-MI patients of both genders.
Maran (2023) developed advanced CNN-based models to analyze multimodal data, including BMI, ECG, and PTB, achieving 98% accuracy in predicting heart diseases. The models were trained on 80% of the data, validated on 20%. Saleh Alghamdi et al. (2024) proposed a method by integrating autoencoder and DenseNet architectures to predict heart disease based on the UCI Cleveland dataset, obtaining a mean accuracy of 99.67% and test accuracy of 99.99%.
3.2 Cluster 2: machine learning models and algorithms for healthcare
Table 4 shows Advances in ML Models and Algorithms for Healthcare. A three-stage wireless body area network (WBAN)-based heart disease prediction model was developed. The three stages include data aggregation, channel selection, and prediction. Channel selection was optimized using the Tunicate Swarm-Sail Fish Optimization algorithm, and statistical features were extracted from the data using a weighted entropy-based method. An enhanced RNN tuned with Tunicate Swarm-Sail Fish Optimization achieved high prediction accuracy (Muthu Ganesh and Nithiyanantham, 2022). This research developed ML models to predict cancer, diabetes, diabetic retinopathy, and heart-related outcomes using EHRs. SVMs achieved 97.08 and 79.75% accuracy for cancer and Pima diabetes datasets, respectively, while Decision Tree (DT) reached 86.42% for heart-related predictions. The study showed that ML could enhance disease prediction and patient outcomes (Sheik Abdullah et al., 2024).
The real-time data will be utilized to predict heart disease with the support of the ML algorithm, as well as attributes like BP, sugar, and heartbeat. The attributes are applied in the dataset on 300 instances with 14. It is used to train and test an R model. Accuracy Evaluation: Accuracy is measured as the basis of several classifiers (Jayakiran et al., 2019). Napa et al. (2024) evaluated recursive feature elimination (RFE) for classifying chronic heart disease based on the Cleveland Hungarian CHD dataset. Different methods of supervised learning have been tested. The KNN model and the DT attained 89.91%. Seeli and Thanammal (2024) explored ML algorithms’ performance, specifically artificial neural network (ANN) and logistic regression (LR) for disease prediction. Without scaling, ANN obtained 86.13% accuracy in the heart disease prediction, and when ensemble normalization and standardization were applied, the improvement in ANN accuracy was 98.81%. Patil et al. (2023) presented a prediction model for heart disease incorporating IoT and ML techniques using data from several sensors: BP monitors, blood oxygen sensors, and EEGs. Hybrid feature extraction methods were combined with ML algorithms, where RNN yields higher accuracy than the traditional approach using methods like SVM, Naive Bayes (NB), and RF.
3.3 Cluster 3: feature engineering and optimization techniques
Table 5 shows Feature Engineering and Optimization Techniques in Heart Disease Prediction. The study proposed a method that combines PCA and feature selection to reduce the dimensionality of data and improve the prediction of coronary heart disease. The model using classifiers such as PCA, RF, DT, and AdaBoost achieved 96% accuracy and outperformed traditional precision, recall, and AUC models. The approach effectively enhanced CHD prediction and patient outcomes (Cheekati et al., 2024). Gupta et al. (2022) proposed a computational intelligence system, C-CADZ, for diagnosing CAD using the Z-Alizadeh Sani CAD dataset. The applied feature extraction, Synthetic Minority Over-sampling Technique (SMOTE) for dealing with class imbalance, and ML classifiers such as RF and Extra Trees resulted in an accuracy of 97.37%. C-CADZ outperformed prior methods by 5.17% and exhibited robust performance, thus making it applicable for heart disease predictions.
Shimpi et al. (2024) proposed a model optimized for diabetes detection via SVM, KNN, and RF classifiers with decision-level fusion. The classifiers were optimized utilizing a PSO algorithm, considering clinical data such as age, BMI, blood pressure, and glucose. The model performed at a diabetes detection rate of 94.27%. It outperformed single classifiers and previous methods on the Indian Pima diabetes dataset. Barfungpa et al. (2024) anticipated a new SMOTE-based hybrid DL network for predicting patient survival in CVD. The SMOTE-HDL network, tested on the Heat-failure-clinical-records dataset, obtained a predictive accuracy of 95.52% that outperformed any existing classifiers. Anand et al. (2022) utilized deep NNs on the PTB-XL ECG dataset for cardiac disorder detection, presenting the ST-CNN-GAP-5 model that resulted in an AUC of 93.41%. The model was tested on an arrhythmia dataset and yielded 95.8% accuracy with an AUC of 99.46%, better than other existing approaches. The SHAP analysis showed that the model is interpretable and reveals critical ECG wave changes that can help make diagnoses in resource-constrained environments. Vetrithangam et al. (2024) proposed the Hybrid CCRF model of heart disease prediction, which applied Canonical Correlation Analysis and RF together. The model represented the non-linear relationships and maximized the feature correlations because it generated polynomial features and synthesized canonical variables. Mandula and Vijaya Kumar (2024) proposed the ALAN method, which combines ANOVA and Lasso regression to identify the most essential features for heart disease prediction. The Extra Trees Adaptive Boosted Decision Forest model reached 88.0% accuracy, 89.81% precision, and 96.21% AUC, which is superior to other algorithms. Lenin and Venkatasalam (2024) utilized DL and SCSO for accurate heart disease classification. SCSO selected key features from patient pathology data, enabling CNNs combined with advanced models like PCA and GANs to predict disease severity.
3.4 Cluster 4: emerging technologies in healthcare
Table 6 shows Emerging Technologies in Healthcare and evaluated Quantum Support Vector Classifier (QSVC) and variational quantum classifier (VQC) for chronic heart disease prediction in healthcare 4.0. QSVC outperformed VQC with an accuracy of 82%, showing the potential of quantum ML in healthcare. Several metrics, such as precision, recall, and F1 score, supported the findings (Munshi et al., 2024a). Bhatt et al. (2024) proposed an AI-enabled stroke prediction architecture using FL based on an ANN model, which uses real stroke cases. The architecture, implemented on healthcare wearable devices, aggregates optimizer weights via a 5G communication channel to enhance performance. It outperformed traditional approaches, achieving 5 to 10% higher accuracy.
The study developed a smart healthcare system for heart disease prediction using Bi-LSTM, which integrated data from IoT devices and electronic clinical records. The system achieved an accuracy of 98.86%, along with high precision, sensitivity, specificity, and F-measure, outperforming existing prediction models (Nancy et al., 2022). Vellore Pichandi et al. (2024) introduced a safe e-healthcare system with accurate HD prediction and enhanced cloud storage security. This classifier achieves a high degree of accuracy as 99.36% through HybBPF-ELM, and Intelligent Encryption Framework enhances cloud data security. The system minimizes encryption and decryption time to process the file by 2.2 GB, at 127.55 s and 452.01 s, respectively. Natarajan et al. (2024) proposed a secure e-healthcare system combining accurate heart disease (HD) prediction and enhanced cloud security. A Hybrid Binary Particle Firefly Optimized Extreme Learning Machine classifier achieved 99.36% accuracy, while an intelligent encryption framework improved data security. The system reduced the processing time for encryption and decryption of a 2.2 GB file to 127.55 s and 452.01 s, respectively. FL has allowed it to develop ML models on distributed datasets, like those in hospitals and mobile devices while maintaining data privacy. This survey reviews prior research on its healthcare applications, including key challenges, methods, and use cases. It outlines existing studies and explores its potential for the healthcare industry (Joshi et al., 2022). CVDs were analyzed using hybrid classical-quantum (CQ) transfer learning models to detect cardiomegaly in chest X-rays. The pre-trained DenseNet-121 integrated with quantum circuits through Qiskit and PennyLane obtained Receiver Operating Characteristic (ROC) and AUC scores of up to 0.93 and accuracies of up to 0.87 on a balanced dataset. Grad-CAM++ heatmaps with QC models showed more trustworthiness, thus supporting possible clinical adoption (Decoodt et al., 2023). AI was used in Healthcare 4.0 for early and accurate disease prediction supported by IoT sensors capturing patient data for ML analysis. The seven-classifier ML model predicted nine fatal diseases, where RF obtained the highest accuracy of 97.62% and AUC of 99.32%. This model is intended to help doctors with early diagnosis and better patient outcomes (Kishor and Chakraborty, 2022).
3.5 Cluster 5: applications of AI across diseases and conditions
Table 7 shows the Application of AI Across Diseases and Conditions. This work presented an intelligent heart disease diagnosis method using an integrated filter-evolutionary search-based feature selection (iFES-FS) and an optimized ensemble classifier. The feature selection combined adaptive threshold information gain (aTIG-FS) and evolutionary gravity-search, and a firefly-driven Firefly-Driven Multi-Objective Multi-Verse Optimizer algorithm optimized the classifier’s hyperparameters. This model outperformed the existing methods regarding accuracy, precision, sensitivity, specificity, and ROC curve evaluation (Venkata MahaLakshmi and Rout, 2024). The work presented an optimized dual-directional temporal convolution and attention-based density clustering for predicting and classifying diabetic risk levels. The proposed approach also outperformed the previous methods and obtained 98.21% accuracy, 94.46% recall, and 99.01% F1-score on the five datasets considered (Jenefer et al., 2024).
The wearable sensor-based prototype proposed for the early detection and monitoring of Parkinson’s disease (PD) using brain wave data and other human records. The FKNN algorithm allowed for accurate classification and tracking of patient progress (Sakthisudhan et al., 2024). Jayasree and Usha (2022) proposed a reliable approach to analyzing CVD risk factors based on ML. The efficacy of the methods was assessed using various statistical and visualization indicators. Kumar and Belinda (2024) designed a Multi-Layered Acoustic Neural (MLAN) Network to identify the symptoms of Rheumatic Heart Disease (RHD) by heart sound and ECG measurements. Compared to other models, the proposed approach achieved 10–17% higher accuracy in RHD detection.
Assegie et al. (2024) conducted to analyze the performance and scalability of DT in predicting CVD. The model of a DT attained 88.8% accuracy in heart disease by metrics such as the confusion matrix, cross-validation score, and model complexity. Jothi Prakash et al. (2024) introduced an Attention-Based Cross-Modal transfer learning (ABCM) framework to enhance CVD prediction by integrating clinical records, medical imagery, and genetic data. The model achieved 93.5% accuracy, 94.5% recall, and a 97.2% AUC, outperforming traditional approaches. Hassan et al. (2024) attempted to predict heart failure and associated mortality by identifying key attributes and using machine-learning methods. After pre-processing the heart failure dataset, the models achieved high accuracy: RF reached 85.23% on the whole dataset, and Flexible Discriminant Analysis reached 86.36% on the XGBoost dataset. A DL-based multi-disease prediction model using big data was developed for the diseases of diabetes, hepatitis, and Alzheimer’s. Datasets from the UCI repository were normalized and passed through the optimization of Jaya Algorithm-Multi-Verse Optimizer and hybrid algorithms, such as deep belief network (DBN) and RNN (Ampavathi and Saradhi, 2021). An advanced ML system was designed to predict heart attack risks and patient survival using age, blood pressure, and BMI features. SVM, RF, and LR algorithms were tested, with SVM reaching 96% accuracy using an 80/20 training–testing split. This model was aimed at improving early cardiac condition detection (Mishra and Mohapatra, 2023). An adaptive stacking model was developed to predict heart diseases using seven ML algorithms, including RF, NB, and Gradient Boosting. The model, evaluated with an 80:20 training–testing split, used metrics like precision and accuracy. Gradient Boosting achieved the highest accuracy of 94.67%, outperforming other methods (Mohapatra et al., 2023).
3.6 Comparison of clusters in heart disease prediction using machine learning
Five clusters use ML and AI to emphasize a distinct aspect of heart disease prediction. Table 8 depicts a comparative analysis.
3.6.1 Key observations across clusters in heart disease prediction using machine learning
The cluster analysis reveals distinct trends, advancements, and challenges in ML applications for heart disease prediction. Below is a detailed breakdown of key observations across the clusters:
3.6.1.1 Accuracy and performance trends
• Most clusters report high prediction accuracy, with models often exceeding 95% accuracy in heart disease classification.
• DL models (CNN, RNN, Bi-LSTM, GANs) consistently outperform traditional ML methods (SVM, DT, RF).
• Optimization techniques (Feature Selection, Hyperparameter Tuning) further boost model performance, often improving F1 scores and AUC values.
• The Emerging Technologies cluster (Quantum ML, FL, IoT) introduces privacy-preserving AI solutions, but some methods (e.g., QSVC) show lower accuracy (~82%), requiring further advancements.
3.6.1.2 Role of feature selection and engineering
• Feature selection significantly impacts performance, with methods like:
○ PCA for dimensionality reduction.
○ Lasso Regression, GA, PSO enhancing feature selection efficiency.
○ Hybrid Feature Engineering (SMOTE-HDL, Hybrid CCRF) achieving accuracy >95% by addressing data imbalance and feature redundancy.
• Advanced feature selection enhances interpretability, making models more useful in clinical settings.
3.6.1.3 ML model trends and emerging frameworks
• Supervised Learning Models dominate, particularly:
○ CNNs for image-based diagnostics (ECG, PCG)
○ RNN for time-series analysis
○ Ensemble models (XGBoost, RF) for structured datasets
• Hybrid AI frameworks are gaining popularity:
○ Autoencoder-DenseNet hybrid networks achieve 99.67% accuracy.
○ Attention-Based Models (ABCM, Bi-LSTM with Attention Mechanisms) optimize long-term dependency learning in cardiac data.
• Quantum ML (QSVC, VQC) is an emerging field but requires significant improvements in computational efficiency and accuracy.
3.6.1.4 Impact of emerging technologies
• FL is transforming healthcare AI, offering:
○ Privacy-preserving AI by training models on decentralized hospital datasets.
○ 5%–10% higher accuracy compared to traditional ML models.
• QC (QSVC, VQC) shows potential but has accuracy limitations (~82%), requiring optimization.
• IoT-based ML models (Wearables, Remote Monitoring)
○ Improve real-time disease prediction.
○ Ensure data security via cloud encryption models (e.g., HybBPF-ELM framework with 99.36% accuracy).
• AI-driven Remote Healthcare is gaining traction, particularly in rural areas with limited hospital access.
3.6.1.5 Multi-disease prediction capabilities
• AI models are no longer limited to heart disease. Several ML approaches now extend to:
○ Diabetes prediction (SVM achieves 97.33% accuracy).
○ Cancer detection (RF reaches 97.08% accuracy).
○ Neurological disorders (Parkinson’s, Alzheimer’s, Stroke prediction).
• Cross-modal AI techniques (ABCM, Transfer Learning) integrate clinical, imaging, and genetic data, improving CVD risk prediction to 93.5% accuracy.
• RL and DBN are beginning to be explored for multi-disease diagnosis.
3.6.1.6 Real-world challenges and limitations
• Dataset Limitations
○ Many studies rely on public datasets (Cleveland, Framingham, Physionet 2016), limiting real-world generalizability.
○ FL can help but requires cross-institutional collaborations.
• Model Interpretability and Explainability
○ Many DL models function as black boxes.
○ SHAP analysis and feature importance mapping improve explainability.
• Computational Efficiency
○ Quantum ML and DL models require high computational resources, limiting real-world deployment.
• Scalability for Global Healthcare
○ Most ML models lack scalability across patient demographics, ethnicities, and geographic regions.
3.6.1.7 Future directions and recommendations
• Hybrid AI models (combining CNN, RNN, Transformers) will likely dominate future research.
• Ethical AI and Bias Reduction are crucial for fair and equitable healthcare AI.
• Integration with Blockchain for secure patient data management.
• Clinical Trials and Real-World Validation to bridge the gap between research and hospital deployment.
The cluster analysis reveals significant advancements in AI-driven heart disease prediction, with DL, feature engineering, and FL leading the way. However, key barriers remain to dataset quality, model interpretability, and computational efficiency. Future work should focus on scalability, privacy-enhancing AI, and hybrid models to ensure widespread adoption in healthcare.
4 Methodology flowchart for ML implementation in heart disease prediction
ML models for heart disease prediction follow a structured methodology, from data collection to model evaluation and deployment. Figure 7 shows a visual flowchart that clarifies the workflow involved in ML-based heart disease diagnosis.
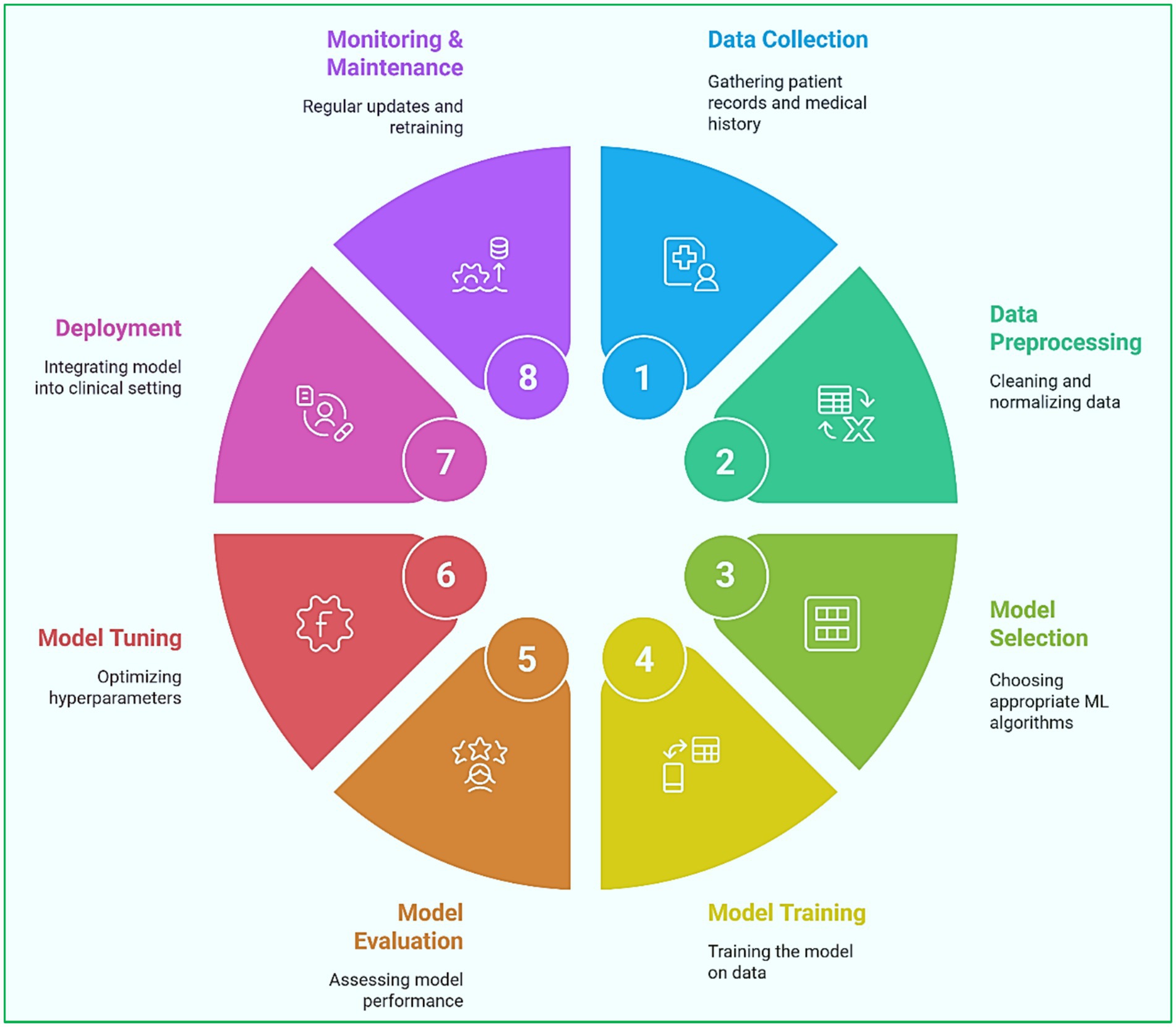
Figure 7. Machine learning pipeline for heart disease prediction: from data acquisition to clinical deployment.
4.1 ML implementation workflow
The methodology consists of the following steps (Helmi et al., 2021; Elghanuni et al., 2022; Abdullah et al., 2023):
a. Collection of Information:
○ Clinical information (EHRs, demographic information, and patient’s medical history).
○ Biometric signals (such as an ECG, blood pressure, and heart rate variability).
○ Imaging data (echocardiograph, CT scans).
○ Data from wearable devices (such as smartwatches and fitness trackers).
b. Data Organization:
○ Management of the missing values and outliers.
○ Selection of significant features and reduction in the number of features.
○ Standardization and normalization of data.
○ Solving class imbalance problems through specified data augmentation techniques.
c. Enhancement of Features:
○ Selection of relevant attributes (cholesterol levels, blood pressure, heart rate).
○ Transformation of features (PCA, LDA).
○ Time series feature extraction from ECG signals.
d. Selected Models Learning:
○ Choice of ML models (LR, SVM, RF, CNN, LSTM).
○ Model training with labeled datasets.
○ Fine-tuning hyperparameters and applying cross-validation.
e. Performance of Models:
○ The model’s performance is evaluated on the data sets with Accuracy, Sensitivity, Specificity, AUC-ROC, and F1 measure.
○ Against baseline models.
○ Models that yield explainable results (SHAP, Local Interpretable Model-agnostic Explanations).
f. Training New Models for Models:
○ Placement of ML models in hospitals and on wearable devices.
○ Live inference for monitoring a patient.
○ Regular retraining for models to increase accuracy.
This structured approach ensures transparency in developing and deploying ML models for heart disease prediction.
5 Data augmentation strategies for addressing class imbalance in heart disease prediction
A significant complication with using ML in heart disease diagnosis is class imbalance. Positive cases (heart disease) are much less common than negative ones, which can cause skewed bias in the model’s predictions. Models are inaccurate because they tend to prefer the majority class. Data augmentation methods help resolve this problem through overlap-based synthetic minority instance generation (Fattepur et al., 2018; Ghazi et al., 2018; Dissanayake and Johar, 2021; Faizah et al., 2024).
5.1 Comprehending class imbalance in heart disease databases
Many heart disease databases (like the UCI Heart Disease, Framingham) have a non-homogeneous class distribution where the non-disease population dramatically exceeds the diseased population. Imbalanced datasets cause:
○ Weak model performance.
○ Excessive false-negative proportions.
○ Reduced sensitivity in detecting disease.
5.2 Data augmentation techniques
Several techniques can be used to generate synthetic samples and balance dataset distribution:
(i) Oversampling Techniques
• Synthetic Minority Over-Sampling Technique (SMOTE):
○ Generates synthetic instances of the minority class by interpolating existing samples.
○ Example: If the dataset has only 20% positive cases, SMOTE can synthetically create new minority class samples.
○ Advantage: It helps balance the dataset without losing original data.
• Adaptive Synthetic Sampling (ADASYN):
○ Similar to SMOTE but focuses on harder-to-learn examples by generating synthetic data where misclassification is higher.
○ Advantage: Enhances model performance for complex datasets.
(ii) Under sampling Techniques
• Random Under sampling (RUS):
○ Reduces the majority class by randomly removing samples, ensuring a balanced class distribution.
○ Advantage: Reduces training time, but may lead to loss of valuable data.
• Cluster Centroid Under sampling:
○ Replaces samples in the majority class with their centroid representations, ensuring minimal data loss.
○ Advantage: Preserves the majority class distribution while balancing data.
(iii) Hybrid Sampling Techniques
• SMOTE + Tomek Links:
○ A combination of oversampling and undersampling techniques.
○ Tomek links help remove noisy data points close to the decision boundary.
○ Advantage: Reduces overfitting and improves model generalization.
• SMOTE + Edited Nearest Neighbor (ENN):
○ SMOTE generates new samples, and ENN removes misclassified samples from the majority class.
○ Advantage: Improves dataset quality for ML training.
(iv) Data Augmentation for DL.
For DL models (e.g., CNN, LSTM), image and signal-based augmentation can be applied:
• ECG Data Augmentation:
○ Techniques: Time warping, jittering, flipping, permutation.
○ Application: Helps DL models generalize better on ECG signals.
• Medical Imaging Augmentation:
○ Rotation, scaling, flipping, and noise addition.
○ Application: Improves CNN-based heart disease classifiers.
5.3 Experimental validation: the impact of data augmentation
Several studies have demonstrated the effectiveness of augmentation techniques in improving heart disease prediction. Table 9 presents the effect of data augmentation on ML model performance.
Key findings indicate that augmentation techniques significantly improve model accuracy. DL architectures such as CNN and LSTM gain considerable advantages from data augmentation methods. Combining hybrid sampling techniques (SMOTE + ENN) notably enhances ML models.
5.3.1 Future considerations for data augmentation
Although augmentation techniques enhance performance, specific challenges need to be tackled (Wei et al., 2023; Jiao and Abdullah, 2024):
• Overfitting Risk: An abundance of synthetic data generation may result in overfitting if adequate validation measures are not implemented.
• Computational Complexity: Sophisticated augmentation techniques (GANs, Variational Autoencoders) demand substantial computational resources.
• Model Interpretability: It is essential to validate augmented data for its applicability and significance in real-world clinical settings.
6 Performance benchmarking of ML models for heart disease prediction
ML models for predicting heart disease have advanced considerably, showcasing a range of algorithms that exhibit varying degrees of accuracy, sensitivity, specificity, and computational efficiency. This section presents a comparative analysis of ML models, focusing on essential performance metrics.
6.1 Comparative benchmarking of ML models
To assess the performance of various ML algorithms, we examine significant research that has documented metrics, including accuracy, sensitivity, specificity, area under the curve (AUC), and computational complexity. Table 10 provides a comparative analysis of ML models for predicting heart disease. The critical insights include conventional ML models, such as LR and SVMs, which demonstrate satisfactory performance but exhibit reduced accuracy when juxtaposed with DL models. Ensemble methods such as RF and XGBoost demonstrate enhanced performance owing to their capacity to identify intricate data patterns. DL methodologies (CNN, Hybrid CNN-LSTM) yield superior accuracy but demand significant computational resources. FL methodologies ensure optimal performance while tackling privacy issues in practical implementations.
Table 11 offers a detailed comparative overview of ML models frequently used for predicting heart disease. Each ML model is evaluated across five critical dimensions: predictive performance, interpretability, computational efficiency, potential for clinical adoption, and privacy preservation.
7 Visualization of ML model performance trends
We employ visual analytics, including box plots, violin plots, and trend graphs, to provide deeper insights into the evolution and effectiveness of ML models for heart disease prediction.
7.1 Box plot analysis of model performance
A box plot illustrates the accuracy distribution across different ML models, showing the median, interquartile range, and outliers. The box plot description is shown in Figure 8. It reveals that CNN and Hybrid DL models exhibit the highest median accuracy (Ishaque et al., 2023). Traditional ML models, such as LR and SVM, show wider variability in performance. DL models are consistently more accurate but computationally intensive (Seneviratne et al., 2019; Ishaque et al., 2022; Yajie et al., 2023).
7.2 Sensitivity and specificity of ML models
Figure 9 illustrates the sensitivity and specificity values of various ML models used for heart disease prediction (Pouretemad et al., 2009; Gros et al., 2019; Jimmy and Bakar, 2023). Each model’s performance is shown with two bars, blue for sensitivity and orange for specificity, enabling direct comparisons among models. Hybrid DL models and CNN-based architectures stand out with superior and consistent performance, achieving sensitivity and specificity values above 97%, indicating their effectiveness in accurately identifying true positives and negatives. In contrast, traditional ML models like LR and SVM demonstrate moderate performance with more significant variability in their metric distributions. This visual representation emphasizes the significance of selecting models based on performance metrics crucial for clinical diagnostic accuracy. It also highlights the growing reliability of DL frameworks in essential healthcare applications, such as predicting cardiovascular risk.
7.3 Trend graph: ML model accuracy over time
A trend graph illustrates the improvement in ML model accuracy over time, reflecting advancements in algorithm development. The trend graph description in Figure 10 shows that the accuracy of ML models has steadily increased over the past decade. Introducing DL (CNN, LSTM, attention-based models) has significantly improved predictive capabilities. FL models show increasing adoption due to their privacy-preserving benefits.
8 Performance benchmarking and case studies of ML models for heart disease prediction
The utilization of ML in predicting heart disease has advanced considerably, showcasing a range of models that exhibit varying degrees of accuracy, sensitivity, specificity, and computational efficiency. This section offers a detailed analysis of ML models through key performance metrics and includes case studies highlighting successful applications in real-world scenarios.
8.1 Case studies of successful ML applications in heart disease prediction
Beyond benchmarking, actual studies within the scope capture ML practices’ value in clinical settings. Case studies from real-world applications are vital because they demonstrate the actual effectiveness of ML models, extending beyond just theoretical performance. They showcase the potential of AI to optimize clinical workflows, enhance diagnostic precision, and facilitate prompt medical decisions. Furthermore, such implementations foster trust among healthcare professionals and stimulate quicker adoption of AI in practical clinical environments (Khatibi et al., 2009; Dehghani et al., 2014; Das et al., 2018; Attalla et al., 2021). The following case studies show how various ML models have achieved successful outcomes.
8.1.1 Case study 1: AI-enhanced ECG interpretation for arrhythmias identification
• Approach: Using the MIT-BIH Arrhythmia Database, a CNN-based DL model was used to classify various forms of heart arrhythmia.
• Result: 98.6% accuracy, which dwarfed traditional methods of ECG analysis.
• Significance: The model was incorporated into portable ECG monitoring instruments to allow real-time detection of arrhythmias (Muzammil et al., 2024).
8.1.2 Case study 2: heart disease risk assessment without data interchange using FL
• Approach: An FL model was implemented in five hospitals without the need for patient data exchange.
• Result: The model obtained over 92.6% accuracy, comparable to other models trained on central databases.
• Significance: It enabled hospital collaboration in building predictive models while ensuring data privacy compliance (HIPAA, GDPR) (Otoum et al., 2024).
8.1.3 Case study 3: heart disease monitoring using PPG and ECG signals by wearing a smartwatch
• Instruments utilized: A hybrid LSTM-CNN architecture is used for classification, and the ECG and PPG smartwatch and medical-grade wearable data were trained on it.
• Result: 97.8% accuracy was achieved in predicting abnormal heart rhythms.
• Consequence: Incorporation into Apple Watch and Fitbit for heart disease real-time risk analysis (Prieto-Avalos et al., 2022).
8.1.4 Case study 4: cardiac risk evaluation based on the abilities of AI
• Instruments utilized: CatBoost Gradient Boosting Models trained with the Framingham Biobank data were used to predict 5-year cardiovascular risk.
• Result: The model received an AUC of 93.5%, surpassing the standard Framingham RSB models.
• Consequence: These findings became part of the integration with telemedicine applications in AI-based treatment recommendations (Singh et al., 2024).
8.1.5 Case study 5: AI-enhanced ECG for identifying sex-specific cardiovascular risk
In a retrospective cohort study, researchers developed an AI-enhanced electrocardiography (AI-ECG) model to investigate sex-specific cardiovascular risk. Utilizing a CNN trained on 1,163,401 ECGs from the Beth Israel Deaconess Medical Center (BIDMC) dataset, the model was designed to classify sex based on 12-lead ECG data. The model’s performance was externally validated using 42,386 ECGs from the UK Biobank cohort. A novel metric, termed the ‘sex discordance score,’ was introduced, representing the difference between AI-predicted sex and biological sex. Findings indicated that females with higher sex discordance scores exhibited an increased risk of cardiovascular death, heart failure, and myocardial infarction, despite having normal ECGs. This association was not observed in males. The study suggested that the sex discordance score could serve as a valuable biomarker for identifying females at elevated cardiovascular risk, potentially guiding enhanced risk factor modification and surveillance strategies (Sau et al., 2025).
8.1.6 Case study 6: AI-ECG reveals elevated cardiovascular risk in women
A recent study employed a CNN to evaluate more than a million ECGs from the Beth Israel Deaconess Medical Center and the UK Biobank. The AI-driven ECG model displayed significant accuracy in determining sex and introduced a new metric called the ‘sex discordance score’—the gap between the sex predicted by the AI and the biological sex. The results showed that women with higher sex discordance scores were at a greater risk for cardiovascular death and heart-related issues, a pattern not found in men. This innovative method highlights the potential of AI-ECG models to enhance the early detection and monitoring of cardiovascular risks, especially for women, leading to customized interventions (Market Access, 2025).
8.1.7 Case study 7: AI-enhanced ECG for predicting future heart failure risk
Researchers at the Yale School of Medicine’s Cardiovascular Data Science (CarDS) Lab have created an AI tool that predicts individuals at high risk for developing heart failure by analyzing ECG images. This model was trained and validated with data from varied populations across the United States, the United Kingdom, and Brazil, showcasing its wide-ranging applicability. Using standard 12-lead ECG images, the AI tool facilitates the early detection of heart failure risk, which may lead to reduced hospital visits and early mortality. This breakthrough marks a significant advancement in scalable, non-invasive cardiac risk assessment, especially useful in settings with limited resources (Dhingra et al., 2025).
8.1.8 Case study 8: XAI in clinical practice
The adoption of ML models in healthcare is often hindered by the “black-box” nature of complex algorithms. XAI techniques address this challenge by providing insights into model decision-making processes.
• CardioRiskNet: This hybrid AI model combines active learning with XAI to assess and prognosticate CVD risk. By offering transparent predictions, CardioRiskNet enhances clinician trust and supports informed decision-making.
• XAI for Heart Failure Prediction: A study employed XAI methodologies to improve a prediction model for heart failure survival. By identifying key features influencing predictions, such as follow-up time and serum creatinine levels, the model achieved a balanced accuracy of 85.1% with cross-validation, facilitating better clinical understanding and application (Talaat et al., 2024).
8.2 Future directions in ML-based heart disease prediction
While current models perform well, several challenges remain:
1. Explainability and Interpretability: Ensuring AI-driven models provide transparent decision-making explanations.
2. Real-World Deployment: Bridging the gap between research prototypes and clinical implementation.
3. Adaptive Learning: Developing self-updating AI models that improve over time as new data becomes available.
4. Multimodal Data Fusion: Integrating genetic, imaging, and wearable sensor data for holistic cardiovascular risk assessment.
9 Ethical considerations and bias mitigation in ML-based medical diagnostics
ML has made considerable progress in predicting heart disease; however, ethical concerns continue to pose significant challenges to its implementation in clinical settings (Matthews et al., 2021). Ethical considerations encompass data privacy, bias mitigation, transparency, accountability, and fairness, all essential for maintaining patient trust and achieving equitable healthcare outcomes (Ibrahim et al., 2018; Draman et al., 2019).
9.1 Data privacy and security in ML for healthcare
ML models intended to predict heart disease focus on EHRs, wearable sensors, and imaging data. This gives rise to serious privacy issues. Patients can suffer significantly from unauthorized access, data breaches, and even misuse of sensitive health information (Marzo et al., 2022). Suggestions for Ensuring Data Protection:
• Federated Learning: FL allows ML model training across different hospitals without sharing raw patient data, complying with data privacy regulations.
• Differential Privacy: This technique involves adding noise to a dataset, making it impossible to re-identify the patient but still allowing for valuable ML insights.
• Blockchain in Healthcare: Patient data storage is decentralized and immutable, so unauthorized changes cannot be made, and the safe exchange of patient data is guaranteed.
9.2 Algorithmic bias and fairness in heart disease prediction
ML models predict data sets that often do not reflect every patient group, resulting in biased outcomes. This is especially troublesome for the diagnosis of heart disease because gender, race, and social class differences may lower the model’s accuracy (Mohammadi et al., 2012; Khatibi et al., 2015; Todd et al., 2016).
9.2.1 Common bias issues
• Gender Bias: The majority of datasets used to study heart disease have an overflow of male participants, hence leading to high deficiencies in prediction for women.
• Ethnic and Racial Bias: A model established using data from one ethnicity only will almost always underperform in a multicultural environment.
• Healthcare Disparities: Economically disadvantaged people may suffer greatly when these models are relied on to interpret their medical records, as the files may not be comprehensive.
9.2.2 Steps to reduce bias
• Dataset Diversity: Ensure training datasets are comprehensive and accurate representations across different demographics.
• Bias Detection: Use Demographic Parity, Equalized Odds, and Disparate Impact metrics to evaluate fairness.
• Adaptive Synthetic Sampling: To mitigate bias, use ADASYN and Fairness Aware Model Tuning as reweighting and re-sampling methods.
9.3 Ethical AI regulations and compliance in healthcare
Ways to Soften the Effect of Bias:
• Improved Dataset Collection: Training sets should be diverse and represent different population segments.
• Metrics to Evaluate Bias: Use classification fairness techniques like Demographic Parity, Equalized Odds, and Disparate Impact.
• Object Reweighting and Model Resampling Techniques: To increase the equity of the AI algorithm and narrow down bias, adaptive synthetic sampling (ADASYN) and fairness-aware model tuning are used.
Global standards must first be followed to develop AI capable of performing further in healthcare settings (Mennella et al., 2024).
9.4 Primary regulations related to ethical AI
• General Data Protection Regulation (GDPR): The standard for patient data privacy in European healthcare institutions.
• Health Insurance Portability and Accountability Act (HIPAA): U.S. law that protects patient health information and sets privacy rules.
• FDA and CE Marking for AI in Healthcare: Diagnostic tools that rely on ML require additional scrutiny and verification before being made available for clinical practice.
These laws and regulations require AI to be trustworthy, hold designers accountable, and ensure it has been rigorously tested before being used in the real world (Farhud and Zokaei, 2021).
10 Explainable AI (XAI) for enhanced clinician trust and adoption
A significant obstacle to the clinical implementation of ML models for heart disease prediction is their opaque nature, which makes it challenging for clinicians to comprehend the rationale behind the model’s predictions. XAI techniques tackle this challenge by delivering interpretable insights to healthcare professionals (Mienye et al., 2024).
10.1 The need for explainability in ML-based medical diagnostics
Unlike traditional diagnostic technologies, ML models utilize opaque DL structures. In cases with no explainability, clinicians might be averse to AI-based forecasts, particularly in critical areas such as estimating the risk of a cardiac event (Amann et al., 2020). Obstacles Concerning Black Box AI:
• Lack of Clinical Justification: In all cases where AI models make predictions, there should be a rational basis accompanying the models for the predictions to be accepted by medical professionals.
• Trust and Liability Issues: If an AI system incorrectly categorizes an accountable patient, who is at fault the physician or the AI system?
• Legal and Ethical Accountability: AI systems must be explainable to avoid legal liability in a medical setting.
10.2 XAI techniques for ML-based heart disease prediction
Different approaches can improve the understanding of AI-centered heart disease diagnosis, enabling practitioners to comprehend the reasoning behind a model’s prediction (Guleria et al., 2022). Model Agnostic Explainability Approaches:
• SHAP: Determines which aspects like blood pressure and ECG most impacted the prediction.
• Local Interpretable Model-agnostic Explanations: Local interpretable models that change the input and assess how the output changes.
• Counterfactual Explanations: This section addresses the question of “What alterations in the patient’s data could change the prediction of AI?”
DL-Specific Explainability Methods:
• Grad-CAM (Gradient-weighted Class Activation Mapping): Identifies significant areas in medical images utilized by CNN models for disease classification.
• Attention Mechanisms in LSTMs & Transformers: Analyzes time-series ECG signals to illustrate the patterns influencing heart disease diagnosis.
10.3 Benefits of XAI for clinical adoption
• Increased Clinician Engagement with AI Systems: AI models that reason about their actions have a higher chance of being utilized by clinicians during patient interactions (Stafie et al., 2023).
• Liability of Inaccurate Diagnoses: Predictive AI, aided by transparency, can help correct devastatingly incorrect diagnoses by identifying instances where the model fails (Hulsen, 2023).
• Meeting Law Compliance: Explanatory processes foster adherence to data protection regulations such as GDPR, HIPAA, and FDA, which require healthcare AI models to be explainable (van der Velden et al., 2022).
10.4 Future directions in XAI for medical AI
Despite (XAI) Achieving Administrative Objectives in Healthcare AI Sectors, Obstacles Persist (Saw et al., 2024):
• Explainability vs. Accuracy: Well-interpreted models like DT may be mathematically less accurate than DL-based approaches.
• Cognitive Load for Clinicians: Real-time XAI systems integrated into clinical practice are important in further research.
• X AI Explainability Framework: A defined metric for judging the relevance of information provided by an AI tool or model in health services is needed.
11 Challenges and limitations
Figure 11 highlights the key challenges in healthcare data management that obstruct the successful integration of AI and ML in clinical situations. These challenges encompass issues with data quality and standardization, concerns over privacy and security, data silos within healthcare systems, and a lack of representative datasets. To overcome these obstacles, it also proposes strategic solutions, including implementing data governance frameworks, enhancing data security, promoting system interoperability, and using synthetic data. Collectively, these strategies aim to foster a more secure, robust, and accessible data environment that supports healthcare innovation driven by AI and ML.
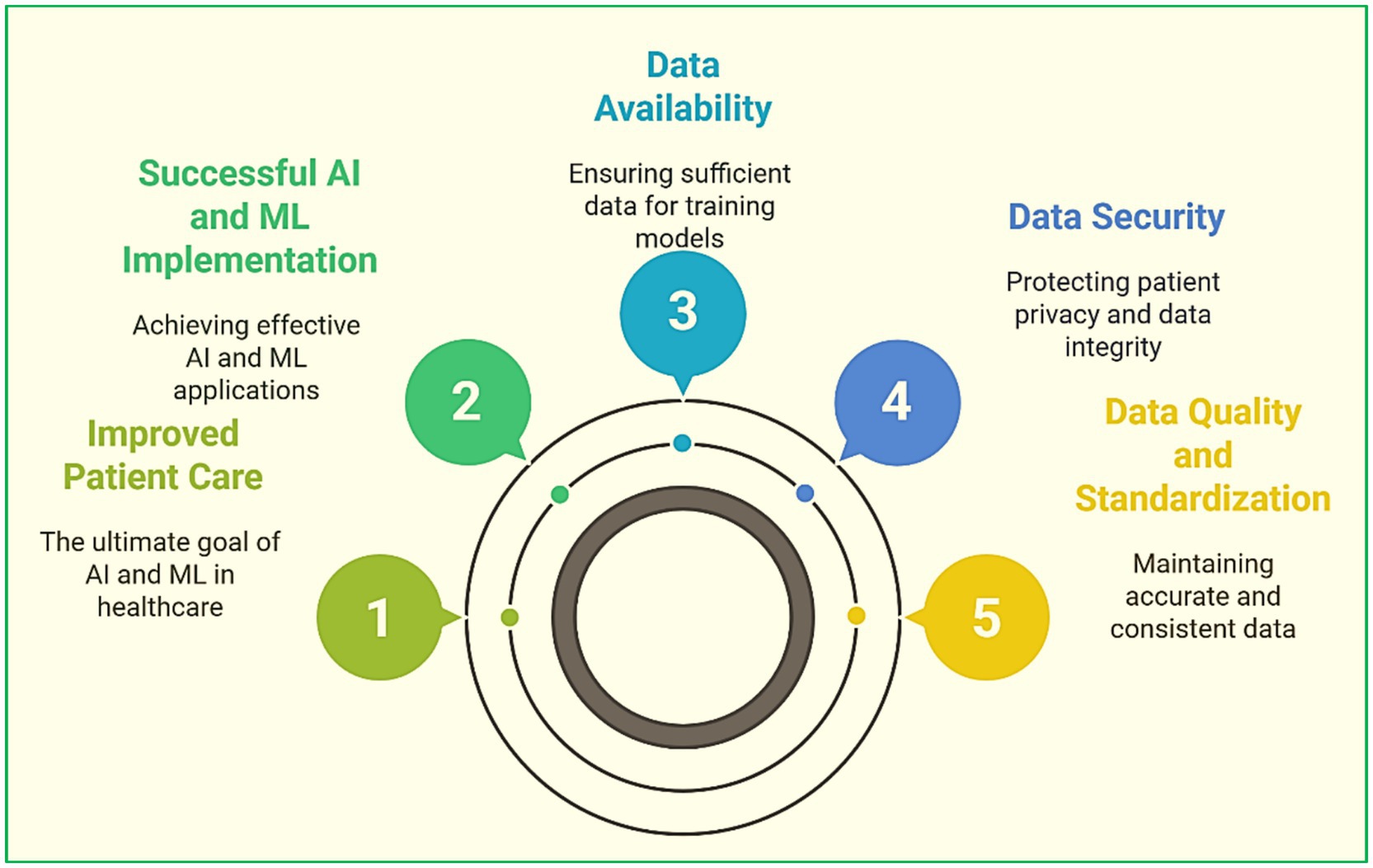
Figure 11. Key challenges and strategic solutions for AI and ML integration in healthcare data management.
11.1 Dataset availability and quality issues
The availability and quality of datasets represent a significant challenge when applying ML and AI in healthcare. Adequate training of ML models is only possible with high-quality, well-annotated data. However, health data often suffers from the following issues (Mohsin et al., 2025).
11.1.1 Data scarcity
The scope and range of datasets relevant to particular patient populations or rare diseases are limited or non-existent and, hence, are insufficient to train generalizable models (Priyadarshi et al., 2024). The research examined Meta-Learning (MtL) application in the diagnosis of rare diseases, especially in the context of disorders of the central nervous system (CNSD), ophthalmic disorders (OD), and cardiac disorders (CD). It covered literature published between 2015 and 2022, which captured the shift of DL towards MtL in these diagnostic modalities. The paper also provided the literature review, including the comparative analysis and research gaps, and constructed an MRI-based Meta-Health framework targeting rare disorders (Singh and Malhotra, 2023).
11.1.2 Data imbalance
Imbalanced data is a common problem in healthcare datasets like the BRFSS heart disease dataset, as patients from certain conditions or groups are underrepresented as opposed to others. The imbalance, as mentioned earlier, makes model predictions biased due to their inability to capture intricate patterns in the classes as mentioned earlier (Benhar et al., 2020; Abdellatif et al., 2024). The study specifically focused on class imbalance in the BRFSS heart disease dataset from 2021 using the resampling approach SMOTE-ENN and with the help of CatBoost and XGBoost classifiers. The findings indicate that CatBoost, Optuna-controlled SMOTE-ENN, achieved the best performance recall, 88%, and AUC, 82% in CVD risk prediction. These results suggest an improvement in developing CVD prevention strategies with the incorporation of ML systems (Tompra et al., 2024).
11.1.3 Data quality and consistency
The inaccuracy stems from multiple means of collection and reporting that generate noise, incompleteness, or inconsistency, finally affecting model precision and dependability (Chen et al., 2021). To achieve forefront quality in the training data used to develop the ML model implemented in the healthcare sector, a three-dimensional Data Quality Framework (DQF) was developed concerning data accuracy, completeness, and otherwise. It was designed to augment performance, predictability, and interpretability and eliminate biases to the extent possible. Moreover, the analysis gaps of this particular set of ML healthcare system data, as well as the groundwork offered, are ideal for supporting future work in the area (Al-Hgaish et al., 2025).
11.1.4 Data standardization
Much healthcare data is kept in varied formats or uses different coding systems within institutions, complicating information integration from multiple sources. Data standardization will enable reliable models and ensure that the system is interoperable. The framework combined smartphone sensing data from ET patients and healthy subjects, employing feature extraction and expert ratings to improve model performance. The interpretability methods, including SHAP and Local Interpretable Model-agnostic Explanations, enabled the identification of key features, offering valuable decision-making insights for early diagnosis and healthcare (Zhang et al., 2024).
11.2 Ethical and privacy concerns
Significant ethical and privacy concerns exist with using AI and ML in healthcare. There is a concern with issues related to patient confidentiality, informed consent, and algorithmic bias (Dang et al., 2023). These include:
11.2.1 Patient privacy and data security
Healthcare information is delicate and could result in severe privacy breaches if misused. Safeguarding this information is of utmost importance, and measures like encryption, secure storage, and FL can help train models without having to share the raw data. The patient’s privacy can also be somewhat protected (Chato and Regentova, 2023). The paper presented a method for real-time anomaly detection using FPGA (Field Programmable Gate Array) based IoT edge devices that employ LR classifiers. This system could monitor ingress traffic and predict anomalies at very low latency. It also used hardware-software partitions and introduced a different approach to the twin-based retraining of the LR classifier. The feasibility and timing analysis proved that the system is better than the implementation for software-only systems (Chaitanya et al., 2024).
11.2.2 Informed consent
As regards the development of AI instruments that utilize patient data, informed consent must be provided clearly and comprehensively. Patients must be made aware of how their data will be used, who will be able to access it, and the risks of using it (Linga et al., 2024). The project merges ancient Ayurvedic treatments with a new ML non-invasive Nadi Pariksha system that attempts to diagnose diseases through pulse reading. The system emphasized the analysis of pulses located at the wrist vata, pitta, and kapha regions employing signal processing and advanced ML with RF, which achieved 86.4 percent classification accuracy. The attempt was towards improving efficiency concerning the early detection of diseases, reduction in mortality, clinical validation, and a balance between patient privacy and ethical implementation (Priya et al., 2023).
11.2.3 Bias and fairness
If an AI model is trained with data that includes social constructs of race, gender, and class, ML models are prone to bias. In the healthcare sector, such biases can result in discrimination. Mitigating these biases is vital to guarantee fairness and that AI systems are unbiased towards different groups of patients. The paper illustrated the ethical, legal, and social aspects of integrating AI and healthcare. Focused issues included privacy, bias, transparency, and accountability of using AI in healthcare, all posing significant risks. Even as AI improved diagnostics and treatment processes with excellent economy, efficiency, and effectiveness, questions wanting answers persist concerning equity, safety, and social balance. This to be dealt with over time needed collaboration to mitigate possible risks while trying to achieve balance (Upreti et al., 2023).
11.2.4 Transparency and accountability
Considering all the ML models, especially DL systems, it has been said that they are ‘black boxes’ since very little is known about their inner workings. In the context of health care, this is an issue because all patients’ care choices are supposed to be actionable and dependable on the part of the clinicians. To provide such accountability while at the same time reducing errors that can potentially harm a patient, attention to these issues is needed. The tutorial focused on the topic of responsible AI, which is concerned with proactively positive AI solutions that need explaining, justifying, and being fair and secure. The tutorial illustrated how processes of reproducibility, data provenance, and model management/monitoring of ML models are crucial to ensure responsible development. The purpose of the tutorial was to arm the attendees with the needed knowledge and skills to practice Responsibility AI (Paliwal et al., 2022).
11.3 Integration into clinical practices
AI and ML integration in clinical practice comes with numerous challenges (Gautam et al., 2023; Bai and Mardini, 2024).
11.3.1 Disruption of clinical workflow
Healthcare professionals may be unwilling to adopt AI-based tools because they fear disruption of established workflows. Clinicians are accustomed to traditional methods and may be wary of relying on new technologies, especially if perceived as complex or unreliable. AI tools should complement existing practices without overwhelming healthcare providers (Rush et al., 2019; Sufian et al., 2024).
11.3.2 Regulatory and legal barriers
It is a highly regulated field with the introduction of new technology. It is a field where regulatory standards and approval must be met for implementation. It may refer to the FDA or EMA. The process of acquiring approval for AI-based diagnostic tools is lengthy and pricey, which can delay adoption. There are legal issues concerning liability in case of errors (Handoko et al., 2024).
11.3.3 Lack of training and education
Healthcare professionals will be less likely to embrace these technologies without the appropriate education on the understanding or practical application of AI and ML. Providers will require educational tools and resources explaining the technologies and their potential benefits and limitations to support adoption. Investment in training programs is crucial to ensure clinicians’ comfort with incorporating AI into practice. The paper proposed a novel FL framework for early COVID-19 detection using PSO. The framework achieved faster convergence and improved performance, with a 94.36% accuracy on a COVID-19 image dataset from multiple healthcare institutions. FL ensured data privacy by decentralizing patient information and sharing only aggregated model updates (Dasaradharami Reddy et al., 2024).
11.3.4 Interoperability
Healthcare systems have tended to operate independently with minimal integration across technologies and platforms. For effective adoption, AI systems must seamlessly integrate with EHRs, medical devices, and other systems in use within healthcare settings. The main barrier to interoperability is, perhaps, the standardization of data structures and rules negotiated by stakeholders in healthcare settings. Prabha et al. (2024) IoTs will likely change the healthcare domain, especially concerning providing personalized care, telemedicine, and remote care monitoring. Five classification schemes for IoT target energy consumption, privacy, and scalability issues while showing the importance of these problems for managing healthcare data with ML. Solving these problems positioned IoT as a viable means to increase the accessibility of healthcare services and improve the quality of life (Balasingam et al., 2017; Gevers-Montoro et al., 2022).
11.3.5 Trust and adoption
Even when the precision is verified, AI instruments encounter issues because of reliability problems and limited human participation. Clinicians and patients must rely on the trustworthiness of AI-based tools before they allow them to affect clinical or patient decision-making. This trust can be obtained by having a development process that is transparent and genuine validation combined with AI’s ongoing performance monitoring. This tutorial concentrated on the concept of Responsible AI, which encompasses the need for transparency, accountability, fairness, and security that AI solution development requires. It highlighted AI’s repetitiveness and the data provenance and control of ML models in responsible development. The tutorial concentrated on ensuring the audience is equipped with the knowledge and skills needed to practice Responsible AI (Paliwal et al., 2022).
AI, ML, and emerging technologies hold significant promise to redefine healthcare. However, they must first overcome challenges related to data quality, ethical issues, and clinical integration. These challenges will be prerequisites in determining how best to utilize these technologies for the optimal benefit of both patients and providers. Figure 12 highlights the primary challenges healthcare organizations face in adopting technology and the associated strategic solutions. It identifies key obstacles such as resistance to change, data security challenges, interoperability problems, and significant implementation costs, matched with proactive strategies that encourage an innovative culture, emphasize cybersecurity, support standardized interoperability, and consider alternative funding options. This organized overview underscores that effective integration of digital technologies in healthcare necessitates a comprehensive approach that tackles both technical and human aspects.
12 Future directions and emerging trends in ML-based heart disease prediction
12.1 Emerging trends in healthcare technology
The healthcare sector is experiencing rapid developments in several areas. It is expected to revolutionize how healthcare is delivered (Junaid et al., 2022). Some of these emerging trends include:
12.1.1 Personalized medicine
Faster healthcare delivery is now achievable due to technological progress in genomics, biomarker discovery, and AI analytics. With the implementation of IoT and ML in healthcare, there is a drastic improvement in diagnosis and care delivery, such as early kidney disease detection and proactive precision care (Loncaric et al., 2020). With the adoption of IoT, subtle risk factors were captured in real-time to assist an ML algorithm that supported personal risk models to provide timely interventions. Therefore, the model ensures continuous patient-provider communication is achieved, thus moving towards personalized healthcare while ensuring strong security measures (Ravi et al., 2025).
12.1.2 AI-driven diagnostics
AI and ML focus on improving the accuracy and timing of diagnosing diseases. From analyzing medical imaging like tumor scanning and cardiovascular monitoring to forecasting patient health through EHR logs, the precision of medicine is improved by automation. In the long term, AI promises to become a ready aid for quick and efficient diagnosis (Sufian et al., 2024; Yenurkar et al., 2024). This research utilizes SVM algorithms to distinguish between types of diabetes, Type 1 and 2, using linear, polynomial, and sigmoid functions. It enhanced the degree of classification incidence of diabetes and enabled the formulation of narrower treatment strategies. It showcased the promise that more developed computational methods can have on medicine with AI-driven healthcare management tasks (Suvartha et al., 2024).
12.1.3 Telemedicine and remote monitoring
Incorporating telemedicine services and remote patient monitoring technologies transforms healthcare delivery, especially in low-resource regions or during public health crises. IoT devices, wearables, and telehealth platforms enable patients to be continuously monitored for their vital signs, medical conditions, and treatment responses (Ardeti et al., 2023; Wang et al., 2024). This development seems poised to continue offering more efficient and inexpensive healthcare services. This work investigated the application of IoT and ML to personalized telemedicine systems with the objective of patient health monitoring and prediction for better care delivery. The effectiveness of the ML model was assessed through recall, sensitivity, error rate, F-score, and other measures, which gave insight into prediction accuracy and complexity. The goal was to shift the paradigm of healthcare to support routine health monitoring and timely proactive interventions (Ramalingam et al., 2024).
12.1.4 Quantum computing (QC)
The emergence of QC promises to revolutionize fields like the modeling of complex diseases and genomics, even improving the standards of drug discovery. Quantum computers could simulate complex biological processes and novel therapeutic targets at speed rivaling thousands of classical computers (Ghazi Enad and Abed Mohammed, 2023). Predicting an enormous acceleration of medical research and precision treatment development is reasonable. In this research, two Quantum ML models, VQC and Pegasos QSVC, were implemented to predict lung cancer in smart healthcare systems. They were benchmarked against baseline models by measuring training accuracy and performing statistical analyses. The Pegasos QSVC model achieved the highest classification accuracy of 85% (Munshi et al., 2024b).
12.1.5 Federated learning (FL)
By not sharing sensitive patient information, FL allows training AI models collaboratively without compromising privacy, which helps gain insights. This innovation facilitates collaboration between healthcare institutions for research and model building while adhering to stringent data privacy regulations. As the fear around the security and privacy of healthcare information further increases, FL is a promising solution for creating AI models that require diverse information while ensuring privacy (Otoum et al., 2024). An ensemble FL approach for developing a DL model to classify data streams from distributed Internet of Medical Things (IoMT) environments was presented. Local federated models were deployed on IoMT devices, with ensemble learning performed on the cloud servers. The comparison results indicated that the ensemble federated model outperformed the primary federated models (Arya and Hanumat Sastry, 2022).
12.1.6 Interdisciplinary approaches for enhanced diagnostics
The merging of ML, AI, and contemporary technologies into healthcare has proven exceedingly useful and requires interdisciplinary teamwork. These technologies’ potential is best capitalized with a multidisciplinary approach toward improving diagnostics. This includes:
• Cooperation Between Health Workers and Data Science Specialists: The adoption of AI in healthcare has proven successful through the collaboration of health workers and data science experts. The data science expert optimizes the ML models, and the healthcare expert adds value with their pathophysiology, clinical diagnosis, and therapeutics knowledge. Their collaboration empowers the development of AI-driven tools that are clinically relevant and accurate, hence ensuring enhanced patient and public healthcare.
• Hybridization of Medicine with Engineering: Biomedical engineers and other engineers are responsible for improving and developing new healthcare technology. Wearable devices, medical imaging systems, or diagnostic aids are some examples of other engineering branches that can be integrated. Engineering and medicine can be united to develop better devices or solutions that match the growing demands of patients and healthcare providers.
• Multidisciplinary Data Integration: Healthcare systems that integrate various data sources like EHRs, Genomics, Imaging, Wearables, and even Environmental Data can enhance diagnosis and treatment direction. Such fusion can be accomplished by interdisciplinary teams using integrated platforms that combine the various datasets to create a more wholesome understanding of the patient’s health and enhance the efficacy of AI models.
• Ethics, Policy, and Technology Integration: To solve AI-related problems in healthcare, collaboration with ethics and policy experts should also take place. Data use’s legal, regulatory, and ethical concerns, such as data privacy, bias, and accountability, must be addressed while technology is developed. Multidisciplinary teams will guarantee the realization of AI tools that are technologically accurate, ethically correct, and legally compliant.
• Patient and Community Involvement: Patients should participate in developing and adopting technology. When patients and communities are brought together through interdisciplinary teams, their issues, preferences, and needs will be addressed so that AI and ML technologies are patient-centric and culturally acceptable. This approach affirms that trust in AI is significantly improved within the frameworks in which diverse populations are formed.
To summarize, the advancement of healthcare technology rests on traditional sectors, which lie in ever-increasing exciting practices and cross-cutting collaborations that will improve diagnostics. New technology like AI, QC, and FL is expected to make healthcare more personalized, accessible, and efficient. Additionally, harnessing multidisciplinary learning will ensure that these results are scientifically valid and ethically responsible, resulting in significant health advantages for patients in all parts of the globe.
13 Conclusion
This review encompasses a wide range of ML applications for predicting heart disease, organized into five main themes: detection and diagnostics, ML models and algorithms, feature engineering and optimization, new technologies in healthcare, and cross-disease AI applications. The findings indicate that while DL models, especially hybrid CNN-LSTM architectures, tend to surpass traditional methods, the success of any model heavily relies on high-quality data, effective feature engineering, and clinical interpretability.
A rising trend in FL, monitoring systems based on wearables, and XAI practices that are gradually narrowing the gap between research and practical application. Nevertheless, significant challenges remain, such as ensuring model generalizability across different populations, maintaining data privacy, achieving interpretability, and integrating with EHRs.
Future studies should prioritize the practical implementation of AI systems via clinically validated trials, focusing on ethical and regulatory standards, and investigating interoperable, patient-centered AI platforms. Moreover, creating transparent models that healthcare providers can trust is essential for broad acceptance. By tackling these issues, ML-driven tools can transition from experimental concepts to game-changing solutions in cardiovascular healthcare.
13.1 Summary of key insights
■ Diagnostic Accuracy: ML and AI have significantly increased accuracy towards diagnosing heart diseases, cancers, and even neurological disorders. The AI systems utilize EHRs, medical imaging, and large datasets alongside sensors to diagnose and improve patient outcomes.
■ Use of Feature Engineering and Its Optimization: Advanced algorithms, GA, PSO, and GWO, have played a crucial role in improving prediction models, which enhances the accuracy with which disease detection can be made. The AI system was optimized for better interpretability and used to determine feature selection, reduce dimensionality, and use other AI-enabled techniques. Moreover, the AI system can select and interpret relevant features, reduce noise, and improve interoperability through Dimensionality Reduction.
■ Emerging Technologies: Healthcare innovation is changing with QC, FL, and the IoT. QC can speed up complex drug discovery and disease modeling. FL alleviates privacy concerns because sensitive information is not expeditiously sent during collaborative model training. Moreover, the application of IoT devices enables proactive fitness healthcare management by providing real-time monitoring.
■ Issues Relating to Data Quality and Ethical Considerations: The availability, quality, and privacy of healthcare data affect AI implementation in healthcare. The success of AI tools hinges on the availability of accurate and secure healthcare data. Other ethical issues, such as consent, privacy, and the algorithms’ impartiality raisers, must also be addressed for AI to properly deploy in healthcare.
■ Interdisciplinary Collaboration: Collaboration between healthcare personnel, such as doctors and nurses, data analysts and scientists, engineers, and ethics specialists, is essential for creating and implementing AI systems in healthcare. Interdisciplinary approaches ensure that AI technologies are technically sound and respond ethically and socially to the complex conditions surrounding patients and legal affairs.
13.2 Implications for research and practice
• Research:
Significant work is currently being done to optimize AI model performance and data assimilation and address ethical concerns. Improving the quality and representativeness of available healthcare datasets will enhance the generalizability of various AI models across different healthcare populations. Further research into other technologies, such as QC and FL, could contribute to advancing AI systems in healthcare. The lack of transparency and interpretability of AI models should also be a focus of future research to foster trust and facilitate smoother integration into clinical decision-making.
• For Practice:
Older healthcare professionals and service providers need to adapt AI and ML-based technologies into their clinical routines to ensure improved diagnostic and treatment results. Sufficient instruction on working with AI systems must be provided alongside the implementation of AI tools to clinicians. Patients should also actively participate in incorporating the technologies so that the solutions work for them. Organizations providing healthcare must also coordinate in fostering interdisciplinary cooperation for the safe, ethical, and beneficial application of AI systems.
AI and ML can transform the health sector, but significant challenges remain in data management, ethics, and deployment. Responsible interdisciplinary collaboration facilitated through innovation has the power to surpass these challenges, which could result in improved health care globally through better utilization of these technologies.
Author contributions
RaK: Conceptualization, Formal analysis, Data curation, Investigation, Methodology, Validation, Visualization, Writing – original draft. SG: Investigation, Software, Writing – review & editing. RuK: Writing – review & editing. MJ: Writing – review & editing. SS: Writing – review & editing. SM: Writing – review & editing. PK: Writing – review & editing. AH: Writing – review & editing. SH: Writing – review & editing. JL: Conceptualization, Formal analysis, Funding acquisition, Supervision, Validation, Writing – review & editing.
Funding
The author(s) declare that no financial support was received for the research and/or publication of this article.
Conflict of interest
The authors declare that the research was conducted in the absence of any commercial or financial relationships that could be construed as a potential conflict of interest.
Generative AI statement
The authors declare that no Gen AI was used in the creation of this manuscript.
Publisher’s note
All claims expressed in this article are solely those of the authors and do not necessarily represent those of their affiliated organizations, or those of the publisher, the editors and the reviewers. Any product that may be evaluated in this article, or claim that may be made by its manufacturer, is not guaranteed or endorsed by the publisher.
Supplementary material
The Supplementary material for this article can be found online at: https://www.frontiersin.org/articles/10.3389/frai.2025.1583459/full#supplementary-material
References
Abdellatif, A., Mubarak, H., Abdellatef, H., Kanesan, J., Abdelltif, Y., Chow, C. O., et al. (2024). Computational detection and interpretation of heart disease based on conditional variational auto-encoder and stacked ensemble-learning framework. Biomed. Signal Process. Control 88:105644. doi: 10.1016/j.bspc.2023.105644
Abdullah, M. I., Hao, S. K., Abdullah, I., and Faizah, S. (2023). “Parkinson’s disease symptom detection using hybrid feature extraction and classification model”, in: 2023 IEEE 14th Control and System Graduate Research Colloquium, ICSGRC 2023 – Conference Proceeding), 93–98.
Ahsan, M. M., and Siddique, Z. (2022). Machine learning-based heart disease diagnosis: a systematic literature review. Artif. Intell. Med. 128:102289. doi: 10.1016/j.artmed.2022.102289
Al-Ajlouni, Y. A., Al Taani, O., Shamaileh, G., Nagi, Y., Tanashat, M., Al-Bitar, F., et al. (2024). The burden of cardiovascular diseases in Jordan: a longitudinal analysis from the global burden of disease study, 1990–2019. BMC Public Health 24:879. doi: 10.1186/s12889-024-18316-0
Al-Hgaish, A., Atan, R., Yaakob, R., and Osman, M. H. (2025). “Enhancing performance of machine learning models in healthcare: an analytical framework for assessing and improving data quality”, in: Lecture notes in networks and systems, eds. V. Goar, M. Kuri, and R. Kumar & T. Senjyu: Springer Science and Business Media Deutschland GmbH), 137–153.
Amann, J., Blasimme, A., Vayena, E., Frey, D., and Madai, V. I. Precise4Q consortium (2020). Explainability for artificial intelligence in healthcare: a multidisciplinary perspective. BMC Med. Inform. Decis. Mak. 20:310. doi: 10.1186/s12911-020-01332-6
Ampavathi, A., and Saradhi, T. V. (2021). Multi disease-prediction framework using hybrid deep learning: an optimal prediction model. Comput. Methods Biomech. Biomed. Engin. 24, 1146–1168. doi: 10.1080/10255842.2020.1869726
Anand, A., Kadian, T., Shetty, M. K., and Gupta, A. (2022). Explainable AI decision model for ECG data of cardiac disorders. Biomed. Signal Process. Control 75:103584. doi: 10.1016/j.bspc.2022.103584
Ardeti, V. A., Kolluru, V. R., Varghese, G. T., and Patjoshi, R. K. (2023). An overview on state-of-the-art electrocardiogram signal processing methods: traditional to AI-based approaches. Expert Syst. Appl. 217:119561. doi: 10.1016/j.eswa.2023.119561
Arya, M., and Hanumat Sastry, G. (2022). “Ensemble federated learning for classifying IoMT data streams”, in: 2022 IEEE 7th international conference for convergence in technology, I2CT 2022: Institute of Electrical and Electronics Engineers Inc.).
Assegie, T. A., Napa, K. K., Thulasi, T., Kumar, A. K., Priya, M. J. T. V., and Dhamodaran, V. (2024). Scalability and performance of decision tree for cardiovascular disease prediction. IAES Int. J. Artif. Intell. 13, 2540–2545. doi: 10.11591/ijai.v13.i3.pp2540-2545
Attalla, S. M., Ibrahim, J., Ads, H., Bakar, H. A., Hamid, N. A. A., and Johar, M. G. M. (2021). Medical students’ perception for online learning during COVID-19 pandemic: a CASE study in a Malaysian private medical SCHOOL. Int. J. Med. Toxicol. Legal Med. 24, 75–80. doi: 10.5958/0974-4614.2021.00011.5
Bai, C., and Mardini, M. T. (2024). Advances of artificial intelligence in predicting frailty using real-world data: a scoping review. Ageing Res. Rev. 101:102529. doi: 10.1016/j.arr.2024.102529
Balasingam, M., Ebrahim, J., and Ariffin, I. A. (2017). Tele-echocardiography – made for astronauts, now in hospitals. Indian Heart J. 69, 252–254. doi: 10.1016/j.ihj.2017.01.010
Barfungpa, S. P., Samantaray, L., and Sarma, H. K. D. (2024). SMOTE-based adaptive coati Kepler optimized hybrid deep network for predicting the survival of heart failure patients. Multimed. Tools Appl. 83, 65497–65524. doi: 10.1007/s11042-023-18061-3
Baviskar, V., Verma, M., Chatterjee, P., Singal, G., and Gadekallu, T. R. (2023). Optimization using internet of agent based stacked sparse autoencoder model for heart disease prediction. Expert. Syst. 41:e13359. doi: 10.1111/exsy.13359
Benhar, H., Idri, A., and Fernández-Alemán, J. L. (2020). Data preprocessing for heart disease classification: a systematic literature review. Comput. Methods Prog. Biomed. 195:105635. doi: 10.1016/j.cmpb.2020.105635
Bhatt, H., Jadav, N. K., Kumari, A., Gupta, R., Tanwar, S., Polkowski, Z., et al. (2024). Artificial neural network-driven federated learning for heart stroke prediction in healthcare 4.0 underlying 5G. Concurr. Comput. Pract. Exp. 36. doi: 10.1002/cpe.7911
Chaitanya, C., Reddy, Y. A., Pasupuleti, H., Gvk, S., and Bapat, J. (2024). “Real-time anomaly detection at IoT-edge ingress port using FPGA based ML classifiers” in ACM international conference proceeding series. eds. S. Dua and V. Saxena (New York City: Association for Computing Machinery), 309–315.
Chato, L., and Regentova, E. (2023). Survey of transfer learning approaches in the machine learning of digital health sensing data. J. Pers. Med. 13:1703. doi: 10.3390/jpm13121703
Cheekati, V. R., Kaja, K. M., Pbv, R. R., Subbarao, G., and Chakravarthi, A. M. (2024). Amalgamate approaches can aid in the early detection of coronary heart disease. J. Theor. Appl. Inf. Technol. 102, 6669–6678.
Chen, X., Owen, C. A., Huang, E. C., Maggard, B. D., Latif, R. K., Clifford, S. P., et al. (2021). Artificial intelligence in echocardiography for anesthesiologists. J. Cardiothorac. Vasc. Anesth. 35, 251–261. doi: 10.1053/j.jvca.2020.08.048
Dala Ali, A. H. H., Harun, S. N., Othman, N., Ibrahim, B., Abdulbagi, O. E., Abdullah, I., et al. (2023). Determinants of inadequate empiric antimicrobial therapy in ICU Sepsis patients in Al-Madinah Al-Munawwarah, Saudi Arabia: a comparison of artificial neural network and regression analysis. Antibiotics 12:1305. doi: 10.3390/antibiotics12081305
Dang, T., Spathis, D., Ghosh, A., and Mascolo, C. (2023). Human-centred artificial intelligence for mobile health sensing: challenges and opportunities. Royal Soc. Open Science 10:230806. doi: 10.1098/rsos.230806
Das, S., Selvaraji, K., Dutta, M., Isa, M. Z. A., and Nataraja Moorthy, T. (2018). A pilot study on glaucoma and depth variation, a factor that affect the accuracy of eyewitness identification. Int. J. Med. Toxicol. Legal Med. 21, 239–242. doi: 10.5958/0974-4614.2018.00077.3
Dasaradharami Reddy, K., Srivastava, G., Zhu, Y., Supriya, Y., Yenduri, G., Victor, N., et al. (2024). “Federated learning using the particle swarm optimization model for the early detection of COVID-19” in Communications in Computer and Information Science. eds. B. Luo, L. Cheng, Z. G. Wu, H. Li, and C. Li (Berlin: Springer Science and Business Media Deutschland GmbH), 425–436.
Decoodt, P., Liang, T. J., Bopardikar, S., Santhanam, H., Eyembe, A., Garcia-Zapirain, B., et al. (2023). Hybrid classical-quantum transfer learning for cardiomegaly detection in chest X-rays. J. Imaging 9:128. doi: 10.3390/jimaging9070128
Dehghani, M., Sharpe, L., and Khatibi, A. (2014). Catastrophizing mediates the relationship between fear of pain and preference for elective caesarean section. Eur. J. Pain (United Kingdom) 18, 582–589. doi: 10.1002/j.1532-2149.2013.00404.x
Dhingra, L. S., Aminorroaya, A., Sangha, V., Pedroso, A. F., Asselbergs, F. W., Brant, L. C. C., et al. (2025). Heart failure risk stratification using artificial intelligence applied to electrocardiogram images: a multinational study. Eur. Heart J. 46, 1044–1053. doi: 10.1093/eurheartj/ehae914
Dissanayake, K., and Johar, M. G. M. (2021). Comparative study on heart disease prediction using feature selection techniques on classification algorithms. Appl. Comput. Intell. Soft Comput. 2021, 1–17. doi: 10.1155/2021/5581806
Dissanayake, K., and Johar, M. G. M. (2023). Two-level boosting classifiers ensemble based on feature selection for heart disease prediction. Indones. J. Electr. Eng. Comput. Sci. 32, 381–391. doi: 10.11591/ijeecs.v32.i1.pp381-391
Dissanayake, K., Johar, M. G. M., and Ubeysekara, N. H. (2023). Data mining techniques in disease classification: descriptive bibliometric analysis and visualization of global publications. Int. J. Comput. Digit. Syst. 13, 289–301. doi: 10.12785/ijcds/130124
Draman, W. M. A. A. W., Chun-Phuoc, J., and Yajid, M. S. A. (2019). Halal certification of medical device in Malaysia: a Shariah analysis into the compliance process and legal requirements. Int. J. Med. Toxicol. Legal Med. 22, 94–99. doi: 10.5958/0974-4614.2019.00066.4
Elghanuni, R. H., Helmi, R. A. A., and Abdullah, M. I. (2022). “Improved detection of Facebook anomalies and abnormalities using graph-based and machine learning techniques”, in: AIP Conference Proceedings).
Faizah, S., Nugraha, D., Abdulrazaq, M. N., Dionova, B. W., Abdullah, M. I., and Novianti, L. (2024). A rest tremor detection system based on internet of thing technology. Indones. J. Electr. Eng. Comput. Sci. 33, 476–484. doi: 10.11591/ijeecs.v33.i1.pp476-484
Faraji, A., Dehghani, M., and Khatibi, A. (2023). Familial aspects of fear of cancer recurrence: current insights and knowledge gaps. Front. Psychol. 14:1279098. doi: 10.3389/fpsyg.2023.1279098
Farhud, D. D., and Zokaei, S. (2021). Ethical issues of artificial intelligence in medicine and healthcare. Iran. J. Public Health 50, i–v. doi: 10.18502/ijph.v50i11.7600
Fatima, N., and Siddiqi, S. (2024). Acute myocardial infarction: prediction and patient assessment through different ML techniques. Int. J. Intell. Syst. Appl. Eng. 12, 106–121.
Fattepur, S., Nilugal, K. C., Rajendran, R., Asmani, F., and Yusuf, E. (2018). Anti-hyperlipidemic activity of methanolic extract of Boesenbergia pandurata (finger root) in experimental induced hypercholestrolemic Sprague dawley rats. Asian J. Pharm. Clin. Res. 11, 8–12. doi: 10.22159/ajpcr.2018.v11s3.29962
Ferdous Azam, S. M., and Sarwar, A. (2023). Consumer purchase intention towards traditional Chinese medicine. Int. J. Bus. Glob. 34, 391–404. doi: 10.1504/IJBG.2023.133273
Gautam, N., Mueller, J., Alqaisi, O., Gandhi, T., Malkawi, A., Tarun, T., et al. (2023). Machine learning in cardiovascular risk prediction and precision preventive approaches. Curr. Atheroscler. Rep. 25, 1069–1081. doi: 10.1007/s11883-023-01174-3
Gevers-Montoro, C., Deldar, Z., Conesa-Buendía, F. M., Lazar, E. A., Mahillo-Fernandez, I., Khatibi, A., et al. (2022). Pain catastrophizing mediates rapid benefits of accessing in-person chiropractic care during the COVID-19 lockdown. Eur. J. Pain (United Kingdom) 26, 463–479. doi: 10.1002/ejp.1872
Ghazi, H. F., Elnajeh, M., Abdalqader, M. A., Baobaid, M. F., and Ariffin, I. A. (2018). Non-communicable diseases and its association with body composition and nutrition among general population in Subnag Jaya, Selangor: community-based study. Pak. J. Nutr. 17, 255–259. doi: 10.3923/pjn.2018.255.259
Ghazi Enad, H., and Abed Mohammed, M. (2023). A review on artificial intelligence and quantum machine learning for heart disease diagnosis: current techniques, challenges and issues, recent developments, and future directions. Fusion Pract. Appl. 11, 08–25. doi: 10.54216/FPA.110101
Goud, P. S., Sastry, P. N., and Sekhar, P. C. (2024). A novel intelligent deep optimized framework for heart disease prediction and classification using ECG signals. Multimed. Tools Appl. 83, 34715–34731. doi: 10.1007/s11042-023-16850-4
Gros, C., De Leener, B., Badji, A., Maranzano, J., Eden, D., Dupont, S. M., et al. (2019). Automatic segmentation of the spinal cord and intramedullary multiple sclerosis lesions with convolutional neural networks. NeuroImage 184, 901–915. doi: 10.1016/j.neuroimage.2018.09.081
Guleria, P., Naga Srinivasu, P., Ahmed, S., Almusallam, N., and Alarfaj, F. K. (2022). XAI framework for cardiovascular disease prediction using classification techniques. Electronics [Online] 11:4086. doi: 10.3390/electronics11244086
Gupta, A., Kumar, R., Arora, H. S., and Raman, B. (2022). C-CADZ: computational intelligence system for coronary artery disease detection using Z-Alizadeh Sani dataset. Appl. Intell. 52, 2436–2464. doi: 10.1007/s10489-021-02467-3
Hajiarbabi, M. (2024). Heart disease detection using machine learning methods: a comprehensive narrative review. Journal of medical. Artif. Intell. 7:21. doi: 10.21037/jmai-23-152
Handoko, M. L., de Man, F. S., Brugts, J. J., van der Meer, P., Rhodius-Meester, H. F. M., Schaap, J., et al. (2024). Embedding routine health care data in clinical trials: with great power comes great responsibility. Neth. Hear. J. 32, 106–115. doi: 10.1007/s12471-023-01837-5
Hassan, M. M., Zaman, S., Rahman, M. M., Bairagi, A. K., El-Shafai, W., Rathore, R. S., et al. (2024). Efficient prediction of coronary artery disease using machine learning algorithms with feature selection techniques. Comput. Electr. Eng. 115:109130. doi: 10.1016/j.compeleceng.2024.109130
Helmi, R. A. A., Elghanuni, R. H., and Abdullah, M. I. (2021). “Effect the graph metric to detect anomalies and non-anomalies on Facebook using machine learning models”, in: 2021 IEEE 12th Control and System Graduate Research Colloquium, ICSGRC 2021 – Proceedings), 7–12.
Hulsen, T. (2023). Explainable artificial intelligence (XAI): concepts and challenges in healthcare. AI [Online] 4, 652–666. doi: 10.3390/ai4030034
Ibrahim, J., Attalla, S. M., Jayaram, J., and Ariffin, I. A. (2018). Adherence of medical students to medical ethics during clinical training – pilot study. Int. J. Med. Toxicol. Legal Med. 21, 208–211. doi: 10.5958/0974-4614.2018.00088.8
Ishaque, M., Johar, M. G. M., Khatibi, A., and Yamin, M. (2022). “Intrusion detection system using binary and multiclass deep neural network classification”, in: Proceedings of the 2022 9th International Conference on Computing for Sustainable Global Development, INDIACom 2022), 749–753.
Ishaque, M., Johar, M. G. M., Khatibi, A., and Yamin, M. (2023). Hybrid deep learning-based intrusion detection system using modified chicken swarm optimization algorithm. ARPN J. Eng. Appl. Sci. 18, 1707–1718. doi: 10.59018/0723212
Jafari, M., Shoeibi, A., Khodatars, M., Ghassemi, N., Moridian, P., Alizadehsani, R., et al. (2023). Automated diagnosis of cardiovascular diseases from cardiac magnetic resonance imaging using deep learning models: a review. Comput. Biol. Med. 160:106998. doi: 10.1016/j.compbiomed.2023.106998
Jagannathan, R., Patel, S. A., Ali, M. K., and Narayan, K. V. (2019). Global updates on cardiovascular disease mortality trends and attribution of traditional risk factors. Curr. Diab. Rep. 19, 1–12. doi: 10.1007/s11892-019-1161-2
Jayakiran, K., Pranavi, M., Novika, M., Tejaswini, V., and Rajesh, N. (2019). Prediction of heart diseases in comparison with different machine learning algorithms. Int. J. Innov. Technol. Explor. Eng. 8, 328–332.
Jayasree, L., and Usha, D. (2022). Statistical analysis with machine and neural learning-based model on cardiovascular diseases and stroke prediction. Int. J. Recent Innov. Trends Comput. Commun. 10, 127–138. doi: 10.17762/ijritcc.v10i2s.5919
Jenefer, G. G., Deepa, A. J., and Linda, M. M. (2024). Diabetic prediction and classification of risk level using ODDTADC method in big data analytics. J. Comb. Optim. 47:80. doi: 10.1007/s10878-024-01179-x
Jiao, L., and Abdullah, M. I. (2024). Neutrosophic enhancement of YOLO-MD algorithm for automated metal surface Micro defect detection. Int. J. Neutrosophic Sci. 23, 308–316. doi: 10.54216/IJNS.230225
Jimmy, D. D., and Bakar, H. A. (2023). “Evaluation of urinary tract infection among neonates and children using urine FEME and urine culture”, in: AIP Conference Proceedings).
Joshi, M., Pal, A., and Sankarasubbu, M. (2022). Federated learning for healthcare domain – pipeline, applications and challenges. ACM Trans. Comput. Healthcare 3, 1–36. doi: 10.1145/3533708
Jothi Prakash, V., Arul Antran Vijay, S., Ganesh Kumar, P., and Karthikeyan, N. K. (2024). A novel attention-based cross-modal transfer learning framework for predicting cardiovascular disease. Comput. Biol. Med. 170:107977. doi: 10.1016/j.compbiomed.2024.107977
Junaid, S. B., Imam, A. A., Balogun, A. O., De Silva, L. C., Surakat, Y. A., Kumar, G., et al. (2022). Recent advancements in emerging Technologies for Healthcare Management Systems: a survey. Healthcare (Basel) 10:1940. doi: 10.3390/healthcare10101940
Kayalvizhi, K., Kanchana, S., Silvia Priscila, S., Padmavathy, C., Ramana Murthy, B. S. N. V., and Thangavel, V. (2024). Optimized CNN-BiLSTM with attention: a high performance model for predicting heart disease using Cleveland and Framingham datasets. J. Mach. Comput. 4, 1195–1205. doi: 10.53759/7669/jmc202404110
Khan Minhas, A. M., Sedhom, R., Jean, E. D., Shapiro, M. D., Panza, J. A., Alam, M., et al. (2024). Global burden of cardiovascular disease attributable to smoking, 1990–2019: an analysis of the 2019 global burden of disease study. Eur. J. Prev. Cardiol. 31, 1123–1131. doi: 10.1093/eurjpc/zwae040
Khatibi, A., Dehghani, M., Sharpe, L., Asmundson, G. J. G., and Pouretemad, H. (2009). Selective attention towards painful faces among chronic pain patients: evidence from a modified version of the dot-probe. Pain 142, 42–47. doi: 10.1016/j.pain.2008.11.020
Khatibi, A., Sharpe, L., Jafari, H., Gholami, S., and Dehghani, M. (2015). Interpretation biases in chronic pain patients: an incidental learning task. Eur. J. Pain (United Kingdom) 19, 1139–1147. doi: 10.1002/ejp.637
Kishor, A., and Chakraborty, C. (2022). Artificial intelligence and internet of things based healthcare 4.0 monitoring system. Wirel. Pers. Commun. 127, 1615–1631. doi: 10.1007/s11277-021-08708-5
Kumar, K. A., and Belinda, M. J. C. M. (2024). A multi-layer acoustic neural network-based intelligent early diagnosis system for rheumatic heart disease. Int. J. Image Graph. 24:2450012. doi: 10.1142/S0219467824500128
Kwon, S. H., and Dong, L. (2022). Flexible sensors and machine learning for heart monitoring. Nano Energy 102:107632. doi: 10.1016/j.nanoen.2022.107632
Lenin, S. T., and Venkatasalam, K. (2024). Effective classification of heart disease using convolutional neural networks. Circuits Syst. Signal Process. 44, 911–935. doi: 10.1007/s00034-024-02851-7
Linga, B. G., Mohammed, S. G. A. A., Farrell, T., Rifai, H. A., Al-Dewik, N., and Qoronfleh, M. W. (2024). Genomic newborn screening for pediatric Cancer predisposition syndromes: a holistic approach. Cancers 16. doi: 10.3390/cancers16112017
Loncaric, F., Cikes, M., Sitges, M., and Bijnens, B. (2020). Comprehensive data integration—toward a more personalized assessment of diastolic function. Echocardiography 37, 1926–1935. doi: 10.1111/echo.14749
Majhi, B., and Kashyap, A. (2024). Explainable AI-driven machine learning for heart disease detection using ECG signal. Appl. Soft Comput. 167:112225. doi: 10.1016/j.asoc.2024.112225
Mandula, A., and Vijaya Kumar, B. S. (2024). Integrated feature selection and ensemble learning for heart disease detection: a 2-tier approach with ALAN and ET-ABDF machine learning model. Int. J. Inf. Technol. (Singapore) 16, 4489–4503. doi: 10.1007/s41870-024-02016-4
Maran, P. S. (2023). Multi-layered architecture convolution neural networks for diagnosing and predicting heart diseases on multi-modal data. Malaysian J. Comput. Sci. sp2023, 29–43. doi: 10.22452/mjcs.sp2023no1.3
Marzo, R. R., Chen, H. W. J., Abid, K., Chauhan, S., Kaggwa, M. M., Essar, M. Y., et al. (2022). Adapted digital health literacy and health information seeking behavior among lower income groups in Malaysia during the COVID-19 pandemic. Front. Public Health 10:998272. doi: 10.3389/fpubh.2022.998272
Matthews, D., Elgueta Cancino, E., Falla, D., and Khatibi, A. (2021). Exploring pain interference with motor skill learning in humans: a protocol for a systematic review. BMJ Open 11:e045841. doi: 10.1136/bmjopen-2020-045841
Mennella, C., Maniscalco, U., De Pietro, G., and Esposito, M. (2024). Ethical and regulatory challenges of AI technologies in healthcare: a narrative review. Heliyon 10:e26297. doi: 10.1016/j.heliyon.2024.e26297
Mienye, I. D., Obaido, G., Jere, N., Mienye, E., Aruleba, K., Emmanuel, I. D., et al. (2024). A survey of explainable artificial intelligence in healthcare: concepts, applications, and challenges. Inform. Med. Unlocked 51:101587. doi: 10.1016/j.imu.2024.101587
Mishra, I., and Mohapatra, S. (2023). An enhanced approach for analyzing the performance of heart stroke prediction with machine learning techniques. Int. J. Inf. Technol. (Singapore) 15, 3257–3270. doi: 10.1007/s41870-023-01321-8
Mohammadi, S., Dehghani, M., Sharpe, L., Heidari, M., Sedaghat, M., and Khatibi, A. (2012). Do main caregivers selectively attend to pain-related stimuli in the same way that patients do? Pain 153, 62–67. doi: 10.1016/j.pain.2011.08.021
Mohapatra, S., Mishra, I., and Mohanty, S. (2023). Stacking model for heart stroke prediction using machine learning techniques. EAI Endorsed Trans. Pervasive Health Technol. 9, 1–5. doi: 10.4108/eetpht.9.4057
Mohsin, S. S., Salman, O. H., Jasim, A. A., Al-Nouman, M. A., and Kairaldeen, A. R. (2025). A systematic review on the roles of remote diagnosis in telemedicine system: coherent taxonomy, insights, recommendations, and open research directions for intelligent healthcare solutions. Artif. Intell. Med. 160:103057. doi: 10.1016/j.artmed.2024.103057
Munshi, M., Gupta, R., Jadav, N. K., Polkowski, Z., Tanwar, S., Alqahtani, F., et al. (2024a). Quantum machine learning-based framework to detect heart failures in healthcare 4.0. Softw. Pract. Experience 54, 168–185. doi: 10.1002/spe.3264
Munshi, M., Gupta, R., Jadav, N. K., Tanwar, S., Nair, A., and Garg, D. (2024b). “Quantum machine learning-based lung Cancer prediction framework for healthcare 4.0” in: 2024 Asia Pacific Conference on Innovation in Technology, APCIT 2024: Institute of Electrical and Electronics Engineers Inc.).
Muthu Ganesh, V., and Nithiyanantham, J. (2022). Heuristic-based channel selection with enhanced deep learning for heart disease prediction under WBAN. Comput. Methods Biomech. Biomed. Engin. 25, 1429–1448. doi: 10.1080/10255842.2021.2013828
Muzammil, M. A., Javid, S., Afridi, A. K., Siddineni, R., Shahabi, M., Haseeb, M., et al. (2024). Artificial intelligence-enhanced electrocardiography for accurate diagnosis and management of cardiovascular diseases. J. Electrocardiol. 83, 30–40. doi: 10.1016/j.jelectrocard.2024.01.006
Nadeem, M. W., Goh, H. G., Khan, M. A., Hussain, M., Mushtaq, M. F., and Ponnusamy, V. A. P. (2021). Fusion-based machine learning architecture for heart disease prediction. Comput. Mater. Continua 67, 2481–2496. doi: 10.32604/cmc.2021.014649
Nancy, A. A., Ravindran, D., Raj Vincent, P. M. D., Srinivasan, K., and Gutierrez Reina, D. (2022). IoT-cloud-based smart healthcare monitoring system for heart disease prediction via deep learning. Electronics (Switzerland) 11:2292. doi: 10.3390/electronics11152292
Napa, K. K., Kumar, A. K., Murugan, S., Mahammad, K., Assegie, T. A., and Okokpujie, K. (2024). Early prediction of chronic heart disease with recursive feature elimination and supervised learning techniques. IAES Int. J. Artif. Intell. 13, 730–736. doi: 10.11591/ijai.v13.i1.pp730-736
Naruka, V., Arjomandi Rad, A., Subbiah Ponniah, H., Francis, J., Vardanyan, R., Tasoudis, P., et al. (2022). Machine learning and artificial intelligence in cardiac transplantation: a systematic review. Artif. Organs 46, 1741–1753. doi: 10.1111/aor.14334
Natarajan, K., Vinoth Kumar, V., Mahesh, T. R., Abbas, M., Kathamuthu, N., Mohan, E., et al. (2024). Efficient heart disease classification through stacked ensemble with optimized firefly feature selection. Int. J. Comput. Intell. Syst. 17:174. doi: 10.1007/s44196-024-00538-0
Otoum, Y., Hu, C., Said, E. H., and Nayak, A. (2024). Enhancing heart disease prediction with federated learning and Blockchain integration. Future Internet 16:372. doi: 10.3390/fi16100372
Paliwal, M., Rao, D., and Tarcar, A. (2022). “Responsible AI Tutorial”, in: ACM International Conference Proceeding Series: Association for Computing Machinery), 339–341.
Pathirana, S., Asirvatham, D., and Johar, M. G. M. (2018). “A multi-agent system for improving electroencephalographic data classification accuracy”, in: IEEE Region 10 Humanitarian Technology Conference, R10-HTC.
Pathirana, S., Asirvatham, D., and M’D Johar, M. G. (2019). Applicability of multi-agent systems for electroencephalographic data classification. Proc. Comput. Sci. 152, 36–43. doi: 10.1016/j.procs.2019.05.024
Patil, R. S., Arjariya, T., and Gangwar, M. (2023). Detection of cardiac abnormalities and heart disease using machine learning techniques. Int. J. Intell. Syst. Appl. Eng. 11, 598–605.
Pouretemad, H. R., Khatibi, A., Ganjavi, A., Shams, J., and Zarei, M. (2009). Validation of addenbrooke’s cognitive examination (ACE) in a persian-speaking population. Dement. Geriatr. Cogn. Disord. 28, 343–347. doi: 10.1159/000252772
Prabha, C., Garg, G., and Ahuja, B. (2024) “IoT in healthcare: applications, challenges and future concerns”, in: 2024 OPJU international technology conference on smart computing for innovation and advancement in industry 4.0, OTCON 2024: Institute of Electrical and Electronics Engineers Inc.
Prieto-Avalos, G., Cruz-Ramos, N. A., Alor-Hernandez, G., Sánchez-Cervantes, J. L., Rodriguez-Mazahua, L., and Guarneros-Nolasco, L. R. (2022). Wearable devices for physical monitoring of heart: a review. Biosensors 12:292. doi: 10.3390/bios12050292
Priya, E., Pradeep, S., Krishna, K. S., and Saran, S. (2023). “Characterization of wrist pulse using machine learning techniques”, in: 2023 Intelligent Computing and Control for Engineering and Business Systems, ICCEBS 2023: Institute of Electrical and Electronics Engineers Inc.).
Priyadarshi, R., Ranjan, R., Vishwakarma, A. K., Yang, T., and Rathore, R. S. (2024). Exploring the Frontiers of unsupervised learning techniques for diagnosis of cardiovascular disorder: a systematic review. IEEE Access 12, 139253–139272. doi: 10.1109/ACCESS.2024.3468163
Ramalingam, S., Dharani, S. P., Priya, R., Devi, E. P., and Pavithra, S. (2024). “Machine learning based remote health care data monitoring and prediction system using IoMT”, in: 10th International Conference on Advanced Computing and Communication Systems, ICACCS 2024: Institute of Electrical and Electronics Engineers Inc.), 2113–2118.
Ravi, M., Krishnaiah, P., Dileep, C., Annapoorna, B., Reddy, M. J., and Satyanarayana, B. (2025). “Early detection of kidney disease risk factors through IoT-enabled machine learning systems” in Lecture notes in electrical engineering. eds. A. Kumar, V. K. Gunjan, S. Senatore, and Y. C. Hu (Berlin: Springer Science and Business Media Deutschland GmbH), 1320–1333.
Roth, G. A., Mensah, G. A., Johnson, C. O., Addolorato, G., Ammirati, E., Baddour, L. M., et al. (2020). Global burden of cardiovascular diseases and risk factors, 1990–2019: update from the GBD 2019 study. J. Am. Coll. Cardiol. 76, 2982–3021. doi: 10.1016/j.jacc.2020.11.010
Roy, T. S., Roy, J. K., and Mandal, N. (2023). Design and development of electronic stethoscope for early screening of valvular heart disease prediction. Biomed. Signal Process. Control 86:105086. doi: 10.1016/j.bspc.2023.105086
Roy, T. S., Roy, J. K., and Mandal, N. (2024). Improvement in the performance of deep learning based on CNN to classify the heart sound by evaluating hyper-parameters. Soft. Comput. 28, 11587–11600. doi: 10.1007/s00500-024-09909-3
Rush, B., Celi, L. A., and Stone, D. J. (2019). Applying machine learning to continuously monitored physiological data. J. Clin. Monit. Comput. 33, 887–893. doi: 10.1007/s10877-018-0219-z
Sakthisudhan, K., Pamungkas, W., Isnawati, A. F., Ahmad, U. A., Mohan Raj, S., and Septiawan, R. R. (2024). The development of an IoT-based cyber-physical system for Parkinson’s disease patients using ML prediction algorithm. El-Cezeri J. Sci. Eng. 11, 256–266. doi: 10.31202/ecjse.1419630
Saleh Alghamdi, N., Zakariah, M., Shankar, A., and Viriyasitavat, W. (2024). Heart disease prediction using autoencoder and DenseNet architecture. Egypt. Inform. J. 28:100559. doi: 10.1016/j.eij.2024.100559
Sau, A., Sieliwonczyk, E., Patlatzoglou, K., Pastika, L., McGurk, K. A., Ribeiro, A. H., et al. (2025). Artificial intelligence-enhanced electrocardiography for the identification of a sex-related cardiovascular risk continuum: a retrospective cohort study. Lancet Digit. Health 7, e184–e194. doi: 10.1016/j.landig.2024.12.003
Saw, S. N., Yan, Y. Y., and Ng, K. H. (2024). Current status and future directions of explainable artificial intelligence in medical imaging. Eur. J. Radiol. 183:111884. doi: 10.1016/j.ejrad.2024.111884
Seeli, D. J. J., and Thanammal, K. K. (2024). Quantitative analysis of gradient descent algorithm using scaling methods for improving the prediction process based on artificial neural network. Multimed. Tools Appl. 83, 15677–15691. doi: 10.1007/s11042-023-16136-9
Seneviratne, K., Hamid, J. A., Khatibi, A., Azam, F., and Sudasinghe, S. (2019). Multi-faceted professional development designs for science teachers’ self-efficacy for inquiry-based teaching: a critical review. Univ. J. Educ. Res. 7, 1595–1611. doi: 10.13189/ujer.2019.070714
Sheik Abdullah, A., Naga Pranava Shashank, V., and Altrin Lloyd Hudson, D. (2024). Disseminating the risk factors with enhancement in precision medicine using comparative machine learning models for healthcare data. IEEE Access 12, 72794–72812. doi: 10.1109/ACCESS.2024.3400023
Shimpi, J. K., Shanmugam, P., and Stonier, A. A. (2024). Analytical model to predict diabetic patients using an optimized hybrid classifier. Soft. Comput. 28, 1883–1892. doi: 10.1007/s00500-023-09487-w
Singh, M., Kumar, A., Khanna, N. N., Laird, J. R., Nicolaides, A., Faa, G., et al. (2024). Artificial intelligence for cardiovascular disease risk assessment in personalised framework: a scoping review. EClinicalMedicine 73:102660. doi: 10.1016/j.eclinm.2024.102660
Singh, K., and Malhotra, D. (2023). Meta-health: learning-to-learn (Meta-learning) as a next generation of deep learning exploring healthcare challenges and solutions for rare disorders: a systematic analysis. Arch. Comput. Methods Eng. 30, 4081–4112. doi: 10.1007/s11831-023-09927-8
Siramshetty, V. B., Chen, Q., Devarakonda, P., and Preissner, R. (2018). The Catch-22 of predicting hERG blockade using publicly accessible bioactivity data. J. Chem. Inf. Model. 58, 1224–1233. doi: 10.1021/acs.jcim.8b00150
Srinivasulu, A., and Pushpa, A. (2020). Disease prediction in big data healthcare using extended convolutional neural network techniques. Int. J. Adv. Appl. Sci. 9, 85–92. doi: 10.11591/ijaas.v9.i2.pp85-92
Stafie, C. S., Sufaru, I. G., Ghiciuc, C. M., Stafie, I. I., Sufaru, E. C., Solomon, S. M., et al. (2023). Exploring the intersection of artificial intelligence and clinical healthcare: a multidisciplinary review. Diagnostics (Basel) 13:1995. doi: 10.3390/diagnostics13121995
Sufian, M. A., Hamzi, W., Zaman, S., Alsadder, L., Hamzi, B., Varadarajan, J., et al. (2024). Enhancing clinical validation for early cardiovascular disease prediction through simulation, AI, and web technology. Diagnostics 14:1308. doi: 10.3390/diagnostics14121308
Suvartha, M., Bhoomika, S., Kalyan, R., Nandan, T. M., Pavithra, G., and Manjunath, T. C. (2024) “Multi-type diabetes using machine learning – a new method of Prediction & Determination”, in: 15th international conference on advances in computing, control, and telecommunication technologies, ACT 2024, eds. J. Stephen, P. Sharma, Y. Chaba, K. U. Abraham, P. K. Anooj, and N. Mohammad, et al.: Grenze Scientific Society International Journal of Engineering & Technology (GIJET) 10, 6805–6810.
Talaat, F. M., Elnaggar, A. R., Shaban, W. M., Shehata, M., and Elhosseini, M. (2024). CardioRiskNet: a hybrid AI-based model for explainable risk prediction and prognosis in cardiovascular disease. Bioengineering 11:822. doi: 10.3390/bioengineering11080822
Todd, J., Sharpe, L., Colagiuri, B., and Khatibi, A. (2016). The effect of threat on cognitive biases and pain outcomes: an eye-tracking study. Eur. J. Pain (United Kingdom) 20, 1357–1368. doi: 10.1002/ejp.887
Tompra, K. V., Papageorgiou, G., and Tjortjis, C. (2024). Strategic machine learning optimization for cardiovascular disease prediction and high-risk patient identification. Algorithms 17:178. doi: 10.3390/a17050178
Upreti, K., Vats, P., Nasir, M. S., Alam, M. S., Shahi, F. I., and Kamal, M. S. (2023). “Managing the ethical and sociologically aspects of AI incorporation in medical healthcare in India: unveiling the conundrum”, in: Proceedings – 2023 IEEE world conference on applied intelligence and computing, AIC 2023: Institute of Electrical and Electronics Engineers Inc., eds. G. S. Tomar and J. Bansal, 293–299.
van der Velden, B. H. M., Kuijf, H. J., Gilhuijs, K. G. A., and Viergever, M. A. (2022). Explainable artificial intelligence (XAI) in deep learning-based medical image analysis. Med. Image Anal. 79:102470. doi: 10.1016/j.media.2022.102470
Vellore Pichandi, K., Janarthanan, V., Annamalai, T., and Arumugam, M. (2024). Enhancing healthcare in the digital era: a secure e-health system for heart disease prediction and cloud security. Expert Syst. Appl. 255:124479. doi: 10.1016/j.eswa.2024.124479
Venkata MahaLakshmi, N., and Rout, R. K. (2024). An intelligence method for heart disease prediction using integrated filter-evolutionary search based feature selection and optimized ensemble classifier. Multimed. Tools Appl. 83, 39841–39865. doi: 10.1007/s11042-023-16924-3
Vetrithangam, D., Krishnan, S. B., Shankar, S. S., and Chakrabarti, P. (2024). Integrating canonical correlation analysis with random Forest for heart disease prediction. J. Mach. Comput. 4, 1180–1194. doi: 10.53759/7669/jmc202404109
Vizhi, K., and Dash, A. (2020). Diabetes prediction using machine learning. Int. J. Adv. Sci. Technol. 29, 2842–2852.
Wang, R., Veera, S. C. M., Asan, O., and Liao, T. (2024). A systematic review on the use of consumer-based ECG wearables on cardiac health monitoring. IEEE J. Biomed. Health Inform. 28, 6525–6537. doi: 10.1109/JBHI.2024.3456028
Wei, Q., Binti Ariffin, I. A., and Tham, J. (2023). Comparison of effects of teacher training programs on TPACK and self-efficacy of primary school teachers. Eurasian J. Educ. Res. 2023, 31–47. doi: 10.14689/ejer.2023.107.003
Yajie, L., Johar, M. G. M., and Hajamydeen, A. I. (2023). Poultry disease early detection methods using deep learning technology. Indones. J. Electr. Eng. Comput. Sci. 32, 1712–1723. doi: 10.11591/IJEECS.V32.I3.PP1712-1723
Yenurkar, G., Mal, S., Nyangaresi, V. O., Kamble, S., Damahe, L., and Bankar, N. (2024). Revolutionizing chronic heart disease management: the role of IoT-based ambulatory blood pressure monitoring system. Diagnostics 14:1297. doi: 10.3390/diagnostics14121297
Zhang, L., Zhu, Y., Ni, Q., Zheng, X., Gao, Z., and Zhao, Q. (2024). Local/global explainability empowered expert-involved frameworks for essential tremor action recognition. Biomed. Signal Process. Control 95:106457. doi: 10.1016/j.bspc.2024.106457
Glossary
ASVM - Acoustic Support Vector Machine
ADASYN - Adaptive Synthetic Sampling
aTIG-FS - Adaptive Threshold Information Gain Feature Selection
AUC - Area Under the Curve
AI - Artificial Intelligence
ANN - Artificial Neural Network
ABCM - Attention-Based Cross-Modal Transfer Learning
Bi-LSTM - Bidirectional Long Short-Term Memory
CVD - Cardiovascular Disease
CNN - Convolutional Neural Network
DT - Decision Tree
DCGAN - Deep Convolutional Generative Adversarial Network
DL - Deep Learning
ENN - Edited Nearest Neighbor
ECG - Electrocardiogram
EHR - Electronic Health Record
XAI - Explainable Artificial Intelligence
XGB - Extreme Gradient Boosting
FL - Federated Learning
FDA - Food and Drug Administration
GDPR - General Data Protection Regulation
GANs - Generative Adversarial Networks
HIPAA - Health Insurance Portability and Accountability Act
KNN - K-Nearest Neighbor
LSTM - Long Short-Term Memory
ML - Machine Learning
NB - Naive Bayes
PCG - Phonocardiogram
PTB-XL - Physikalisch-Technische Bundesanstalt Extended ECG Dataset
PCA - Principal Component Analysis
QC - Quantum Computing
QSVC - Quantum Support Vector Classifier
RF - Random Forest
ROC - Receiver Operating Characteristic
RNN - Recurrent Neural Network
RFE - Recursive Feature Elimination
RBM - Restricted Boltzmann Machine
SCSO - Sand Cat Swarm Optimization
SHAP - SHapley Additive exPlanations
SVM - Support Vector Machine
SMOTE - Synthetic Minority Over-sampling Technique
VQC - Variational Quantum Classifier
WBAN - Wireless Body Area Network
Keywords: heart disease prediction, machine learning (ML), deep learning models, federated learning, explainable artificial intelligence (XAI)
Citation: Kumar R, Garg S, Kaur R, Johar MGM, Singh S, Menon SV, Kumar P, Hadi AM, Hasson SA and Lozanović J (2025) A comprehensive review of machine learning for heart disease prediction: challenges, trends, ethical considerations, and future directions. Front. Artif. Intell. 8:1583459. doi: 10.3389/frai.2025.1583459
Edited by:
Tim Hulsen, Rotterdam University of Applied Sciences, NetherlandsReviewed by:
Minjae Yoon, Seoul National University Bundang Hospital, Republic of KoreaAnnalisa Santucci, University of Siena, Italy
Copyright © 2025 Kumar, Garg, Kaur, Johar, Singh, Menon, Kumar, Hadi, Hasson and Lozanović. This is an open-access article distributed under the terms of the Creative Commons Attribution License (CC BY). The use, distribution or reproduction in other forums is permitted, provided the original author(s) and the copyright owner(s) are credited and that the original publication in this journal is cited, in accordance with accepted academic practice. No use, distribution or reproduction is permitted which does not comply with these terms.
*Correspondence: Jasmina Lozanović, amFzbWluYS5sb3phbm92aWNAZ21haWwuY29t; Rupinder Kaur, cnVwaW5kZXJrYXVyMTU4OEBnbWFpbC5jb20=
†ORCID: Raman Kumar, https://orcid.org/0000-0003-2934-7609
Sarvesh Garg, https://orcid.org/0009-0006-2511-7303
Rupinder Kaur, https://orcid.org/0000-0002-1346-191X
M. G. M. Johar, https://orcid.org/0000-0001-7986-3834
Sehijpal Singh, https://orcid.org/0000-0003-2562-0524
Pulkit Kumar, https://orcid.org/0009-0004-1737-3660