- 1Division of Spine, Department of Orthopedics, Tongji Hospital affiliated to Tongji University School of Medicine, Shanghai, China
- 2Key Laboratory of Spine and Spinal cord Injury Repair and Regeneration, Tongji University, Ministry of Education, Shanghai, China
- 3Department of Orthopedics, School of Medicine, Shanghai General Hospital, Shanghai Jiaotong University, Shanghai, China
- 4Department of Orthopedics, The First Affiliated Hospital of Zhengzhou University, Zhengzhou, China
- 5Department of Pathology, Shanghai Tenth People's Hospital, Tongji University School of Medicine, Shanghai, China
- 6Department of Prevention, Tongji University School of Medicine, Tongji University, Shanghai, China
Background: Kidney renal clear cell carcinoma (KIRC) is the malignancy originated from the renal epithelium, with a high rate of distant metastasis. Aberrant alternative splicing (AS) of pre-mRNA are widely reported to be involved in the tumorigenesis and metastasis of multiple cancers. The aim of this study is to explore the mechanism of alternative splicing events (ASEs) underlying tumorigenesis and metastasis of KIRC.
Methods: RNA-seq of 537 KIRC samples downloaded from the TCGA database and ASEs data from the TCGASpliceSeq database were used to identify ASEs in patients with KIRC. The univariate and Lasso regression analysis were used to screen the most significant overall survival-related ASEs (OS-SEs). Based on those, the OS-SEs model was proposed. The interaction network of OS-SEs and splicing factors (SFs) with absolute value of correlation coefficient value >0.750 was constructed by Pearson correlation analysis. The OS-SEs significantly related to distant metastasis and clinical stage were identified by non-parametric test, and those were also integrated into co-expression analysis with prognosis-related Kyoto Encyclopedia of Genes and Genomes (KEGG) pathways identified by Gene Set Variation Analysis (GSVA). ASEs with significance were selected for multiple online database validation.
Results: A total of prognostic 6,081 overall survival-related ASEs (OS-SEs) were identified by univariate Cox regression analysis and a prediction model was constructed based on 5 OS-SEs screened by Lasso regression with the Area Under Curve of 0.788. Its risk score was also illustrated to be an independent predictor, which the good reliability of the model. Among 390 identified candidate SFs, DExD-Box Helicase 39B (DDX39B) was significantly correlated with OS and metastasis. After external database validation, Retained Intron of Ras Homolog Family Member T2 (RHOT2) and T-Cell Immune Regulator 1 (TCIRG1) were identified. In the co-expression analysis, overlapped co-expression signal pathways for RHOT2 and TCIRG1 were sphingolipid metabolism and N-glycan biosynthesis.
Conclusions: Based on the results of comprehensive bioinformatic analysis, we proposed that aberrant DDX39B regulated RHOT2-32938-RI and TCIRG1-17288-RI might be associated with the tumorigenesis, metastasis, and poor prognosis of KIRC via sphingolipid metabolism or N-glycan biosynthesis pathway.
Introduction
Kidney renal clear cell carcinoma (KIRC) is a malignant cancer originated from renal epitheliums, accounting for about 75% of kidney tumors (Hsieh et al., 2017). Therapeutically, although radical nephrectomy is performed for localized renal masses, distant metastasis may be observed in a large proportion of patients at diagnosis, especially metastasis in lung, bone and brain (Gupta et al., 2008). With regard to these advanced KIRCs, the treatment option was limited with only sunitinib widely approved (Porta et al., 2019). Even systematic therapy were applied, including immunotherapeutic agents, antiangiogenic agents and mTOR inhibitors, the prognosis was still poor (Jonasch et al., 2014; Jonasch, 2018). In order to prolong the overall survival of patients with KIRC, there is a pressing need to explore its pathogenic mechanism and identify the potential therapeutic targets related to tumorigenesis, metastasis and prognosis.
Nowadays, most studies of KIRC focused on alteration of transcriptome level and the posttranscriptional process was largely underestimated. Alternative splicing (AS), plays an important role in the maturation of mRNAs from its precursors, leading to diverse mRNA isoforms spliced and protein variants translated (Montes et al., 2019). In this process, splicing factors (SFs) work as regulatory catalyst of alternative splicing events (ASEs) and both build up an intricate regulatory network (Frankiw et al., 2019; Wu et al., 2019). Functionally, AS has been reported to take part in cell differentiation, lineage determination and tissue-specificity acquisition (Wang et al., 2008). The aberrant AS of some genes and somatic mutations of SFs, which make network dysregulated, have been shown to modulate malignant transformation of cells and epithelial-mesenchymal transition (EMT) (Sveen et al., 2016; Kouyama et al., 2019; Wu et al., 2019; Xing et al., 2019). Thus, identifying the dysregulated network may shed light upon the molecular biomarkers for prognosis, metastasis, and potential therapeutic targets (Lee and Abdel-Wahab, 2016; Zhou et al., 2016; Wang et al., 2019).
Nowadays, although a systematic analysis of ASEs was unveiled in KIRC, the regulatory network of ASEs and SFs was not explored (Song et al., 2019). Additionally, metastasis-related ASEs, and potential therapeutic targets were also underestimated. In this study, we performed a comprehensive analysis of AS profiling to identify the overall survival-related ASEs (OS-SEs) in patients with KIRC and construct a prognostic model. Additionally, metastasis-related ASEs along with corresponding SFs and pathways were also identified by Pearson correlation analysis to illuminate the underlying mechanism of metastasis in KIRC. The prediction model might assist oncologists in clinical decision-making. Moreover, we also identified a new candidate molecular mechanism and two potential therapeutic targets for KIRC metastasis treatment, especially to the bone metastasis.
Materials and Methods
Data Collection
Clinical information, RNA sequencing profiles, and SFs of KIRC samples were collected from the Cancer Genome Atlas (TCGA) database (https://portal.gdc.cancer.gov). Meanwhile, ASEs data were retrieved from the TCGASpliceSeq database (https://bioinformatics.mdanderson.org/TCGASpliceSeq/) (Ryan et al., 2016) including seven types (alternate acceptor site, AA; exon skip, ES; alternate terminator, AT; mutually exclusive exons, ME; retained intron, RI; alternate donor site, AD; alternate promoter, AP) (Chen et al., 2019). Samples with more than 25 percent of missing percent splicing (PSI) values were excluded. The ASE was presented with gene name, ID number from the TCGASpliceSeq database (AS ID) and splicing pattern.
The Identification of OS-SEs
The K-Nearest Neighbor algorithm was performed to impute ASEs with missing expression data. ASEs, whose means and standard deviations of PSI < 0.05 and 0.01, were excluded, neither were samples without follow-up records. Then, the combined ASEs along with clinical data were put into the univariate Cox regression analysis to evaluate the prognostic value of each filtered ASE. The UpSet plot was developed to illustrate OS-SEs and volcano plot was used to display the prognosis-related and -unrelated ASEs integrally. The bubble plots were generated to present the top 20 OS-SEs for seven types of ASEs, in which the color and size of bubbles symbolize the value of ASEs for overall survival.
The Construction of the Prognostic Model Based on the OS-SEs
The Lasso regression was firstly performed to screen the top 20 significant prognostic OS-SEs and then the significant prognostic OS-SEs were evaluated by the multivariate Cox regression model with β value, which represented the regression coefficient of each integrated OS-SE in the model. Risk score was thus acquired by the following formula:
According to the median risk score, samples were divided into two risk groups medially. The area under receiver operating characteristic ROC curve was used to evaluate the accuracy of the model. In addition, Kaplan-Meier survival analysis was also conducted to compare the difference between high- and low-risk group. Samples were reordered according to risk score and then the risk curve, scatterplot and expression heatmap were generated.
The univariate and multivariate Cox regression analysis, modified by baseline information, were applied to evaluate the prognostic role of risk score, along with age, gender, grade, clinical stage, and TNM stage.
The Construction of the Interaction and Correlation Network
In the SpliceAid2 database, 390 splicing factors were retrieved (Piva et al., 2009). Pearson correlation analysis was performed to explore the interaction and correlation between SFs and OS-SEs. The regulation network of SFs and OS-SEs was plotted by Cytoscape (3.7.1) (Shannon et al., 2003), in which the regulation pairs with P > 0.001 and the absolute value of correlation coefficient < 0.750 were excluded. In the network, we defined SF and OS-SEs as arrows and ellipses, high and low risk of OS-SEs as red and purple, positive and negative regulations as red and green lines, respectively.
The Identification of Metastasis- and/or Stage-Related OS-SEs
To identify the OS-SEs related to metastasis and/or TNM stage, we performed Kruskal-Wallis test and Mann-Whitney-Wilcoxon test, which were displayed by beeswarm plots. Besides, the regulation network of these metastasis-, and/or stage- related OS-SEs were also explored.
The Co-expression Analysis Between ASEs and Signaling Pathways
The univariate Cox analysis was performed to screen the prognosis-related signaling pathways identified by Gene Set Variation Analysis (GSVA) (Hänzelmann et al., 2013). Then, metastasis and stage-related OS-SEs and prognosis-related KEGG pathways were put into the co-expression analysis to identify the possible downstream mechanism of OS-SEs.
Online Database Validation
In order to ensure the roles of selected metastasis and stage-related OS-SEs, multiple databases including the UALCAN (Chandrashekar et al., 2017), UCSC Treehouse Childhood Cancer Initiative, Kaplan Meier plotter (Nagy et al., 2018), LinkedOmics (Vasaikar et al., 2018), SurvExpress (Aguirre-Gamboa et al., 2013) and Firebrowse (Deng et al., 2017) were used to detect their gene and protein expression levels in KIRC and normal kidney tissues.
Immunohistochemistry (IHC)
The IHC slides and information were obtained from the Human Protein Atlas. Immunostaining on each slide was assessed by experienced pathologists to examine the percentage of RHOT2 and TCIRG1 positive tumor cells and presented as histochemistry score (H-score). H-score = Σpi(i+1) where i is the intensity score and pi is the percent of the cells with that intensity.
Statistical Analysis
All statistical analysis was applied by R version 3.5.1 (Institute for Statistics and Mathematics, Vienna, Austria; https://www.r-project.org) (Package: impute, UpSetR, ggplot2, rms, glmnet, preprocessCore, forestplot, survminer, survivalROC, beeswarm). For descriptive statistics, mean ± standard deviation was used for the continuous variables in normal distribution while the median (range) was used for continuous variables in abnormal distribution. Categorical variables were described by counts and percentages. Two-tailed P < 0.05 was regarded statistically significant.
Results
Overview of ASEs and OS-SEs in KIRC
The analysis process was presented in the flow chart (Figure 1). The sequencing data of 537 cases KIRC were downloaded from the TCGA database, with the median overall survival of 1,091 (range, 0–3,668) days. Throughout the follow-up period, 165 patients died and 496 experienced tumor metastases. A total of 46,415 ASEs in 10,600 parent genes were detected in patients with KIRC, including 3,821 AAs (2,683 genes), 3,270 ADs (2,300 genes), 9,509 APs (3,805 genes), 8,632 ATs (3,770 genes), 18,117 ESs (6,915 genes), 235 MEs (227 genes), and 2,831 RIs (1,902 genes). Thus, one gene could undergo more than 4 splicing patterns (Figure 2A). Among the seven types of ASEs, ES was the most prevalent one, followed by AT. A total of 6,081 OS-ASEs from 3,444 parent genes were identified and the UpSet plot revealed that AP was the most common splicing patterns associated with KIRC prognosis (Figure 2B). The volcano plot suggested that most of ASEs were OS-SEs in KIRC (Figure 2C). The top 20 OS-ASEs in seven types of splicing patterns were illustrated in bubble plots (Supplementary Figures 1A–G).
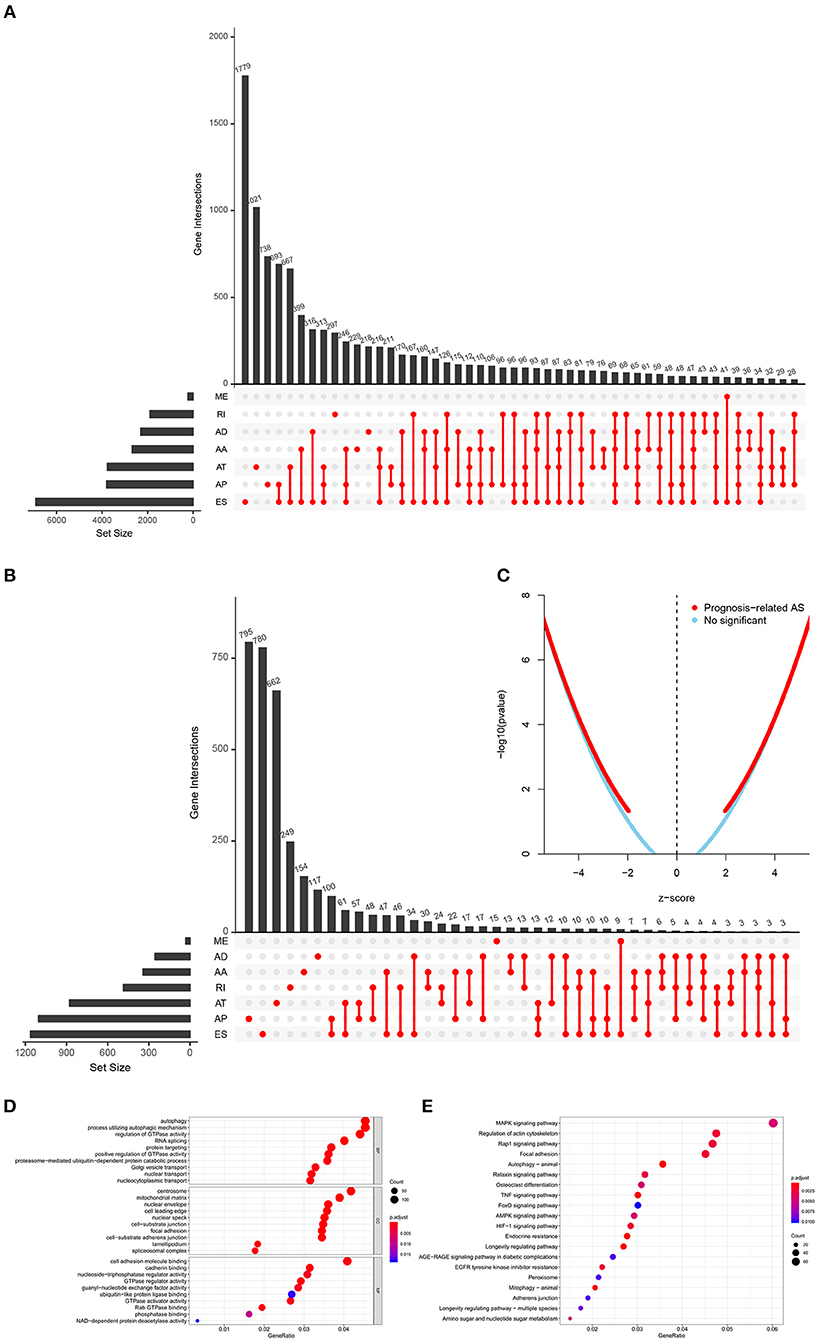
Figure 2. The identification of OS-SEs in KIRC patients. The Upset plots of ASEs and OS-SEs: (A) The number of ASEs in different types of splicing patterns; (B) The number of OS-SEs in different types of splicing patterns. (C) The volcano plot of the prognosis-related and no significant ASEs, respectively; The GO analysis (D) and the KEGG pathways enrichment analysis (E) of the parent genes of OS-ASEs. ASEs, Alternative splicing events; OS-SEs, overall survival-related ASEs; KIRC, kidney renal clear cell carcinoma; AA, alternate acceptor; AD, alternate donor; AP, alternate promoter; AT, alternate terminator; ES, exon skip; ME, mutually exclusive exons; RI, retained intron; GO, Gene Ontology; KEGG, Kyoto Encyclopedia of Genes and Genomes.
Functional Enrichment Analysis of Prognostic AS Events
In order to illuminate the potential mechanism underlying the OS-ASEs, 2,077 parent genes of the 6,081 OS-ASEs in KIRC were sent for Gene Ontology (GO) and Kyoto Encyclopedia of Genes and Genomes (KEGG) enrichment analysis (Figures 2D,E). The biological process of GO analysis revealed the enrichment of some well-known pathways, in relation to “autophagy”, “process utilizing autophagic mechanism” and “regulation of GTPase activity” (Figure 2D). Besides, the RNA splicing was also enriched significantly, which meant the active aberrant splicing patterns of KIRC. Additionally, “centrosome” “mitochondrial matrix” and “cell adhesion molecule binding” were also significantly enriched as cellular component or molecular function. The KEGG enrichment analysis suggested some key pathways were associated with the OS of patients with KIRC, such as “MAPK signaling pathway”, “Regulation of actin cytoskeleton”, “Rap1 signaling pathway” and “Focal adhesion” (Figure 2E).
Establishment of the Prediction Model
In order to avoid over-fitting of the predict model, the Lasso regression was performed to screen the top 20 OS-SEs. The result showed that C4orf19-69001-AT, C16orf13-32924-ES, KIAA0930-62645-AP, FAM120C-89237-AT, UACA-31439-AP were included in the multivariate Cox regression analysis (Figures 3A,B), with Area Under Curve (AUC) of 0.788 in ROC curve (Figure 3C). Accordingly, risk score of each sample was calculated, with a median value of 0.853. Then, Kaplan-Meier curve revealed that prediction model of risk score had a good effectiveness (P < 0.001) (Figure 3D).
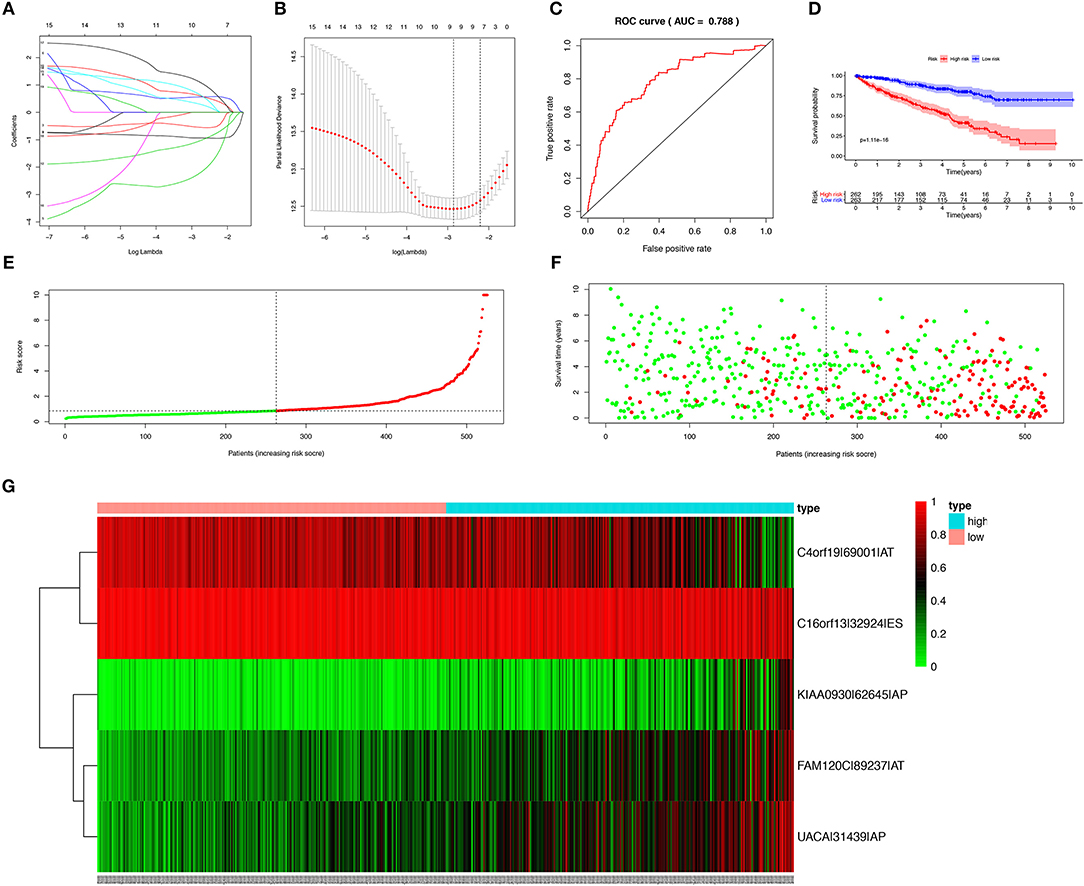
Figure 3. Establishment and assessment of the predict model. (A) The coefficients in the Lasso regression for OS-SEs screening; (B) Cross-validation for tuning parameter selection in the proportional hazards model; (C) The ROC curve for assessing the reliability of the predict model; (D) The Kaplan-Meier curve of the predict model; (E) The risk curve of each sample reordered by risk score; (F) The scatter plot of the samples. The green and red dots representing survival and death, respectively; (G) The heatmap of expression level of 5 OS-SEs filtered by Lasso regression. OS-SEs, overall survival-related ASEs; ROC, receiver operating characteristic.
Risk curve and scatterplot were generated to show the risk score and vital status of each patient with KIRC. Patient in high-risk group had a higher mortality than patient in low-risk group (Figures 3E,F). The heatmap showed the expressions of OS-SEs screened by Lasso regression, indicating that C40rf19-69001-AT and C16orf13-32924-ES were lower and KIAA0930-62645-AP, FAM120C-89237-AT, UACA-31439-AP were higher in high-risk group (Figure 3G).
The Risk Score Predicted Prognosis
The risk score along with age, gender, grade and TNM stage were evaluated in the univariate and multivariate Cox regression analysis. The risk score was confirmed as an independent predictor in both univariate (HR = 1.089, 95%CI (1.067–1.111), P < 0.001), and multivariate Cox regression analysis (HR = 1.064, 95%CI (1.037–1.091), P < 0.001), Figures 4A,B).
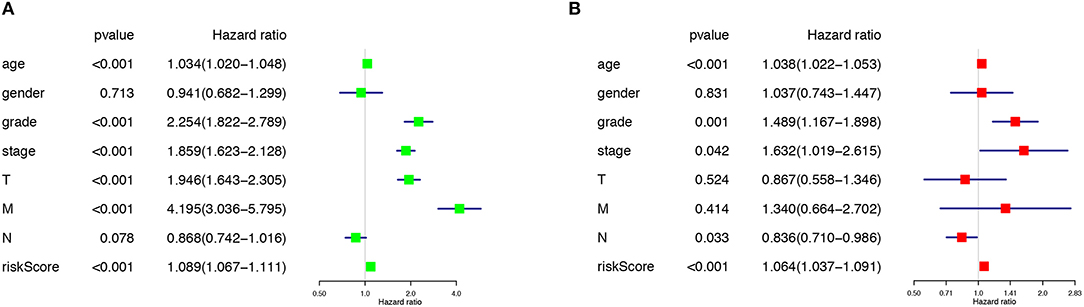
Figure 4. The Cox regression analysis for evaluating the independent prognostic value of the risk score. The univariate (A) and multivariate (B) Cox regression analysis of risk score, age, gender, grade, and TNM stage.
The potential splicing regulatory network of SFs and OS-SEs, and their metastasis or clinical stage correlation.
With access to RNA-seq data and corresponding clinical information of patients with KIRC, we identified 390 candidate SFs whose expression levels were significantly associated with OS of KIRC patients. Among them, DExD-Box Helicase 39B (DDX39B) was the only SF, who was correlated with prognosis (Figure 5A), TNM staging system (Figures 5B–D), clinical stage (Figure 5E), and tumor purity (Figure 5F). Then, a network was established to demonstrate the interaction and correlation between SFs and OS-SEs. DDX39B was correlated with 34 favorable OS-SEs (purple ellipses) negatively (green lines) and 166 adverse OS-SEs (red ellipses) positively (red lines) (Figure 6A). Among them, 7 OS-SEs (CALCOCO1-22108-RI, CIRBP-46432-RI, P4HTM-64788-ES, RHOT2-32938-RI, TBC1D17-51116-ES, TCIRG1-17288-RI, THOP1-46623-AP) were significantly related to both metastasis and stage in the Venn plot (Figures 6B–F, Supplementary Figures 2A–J).
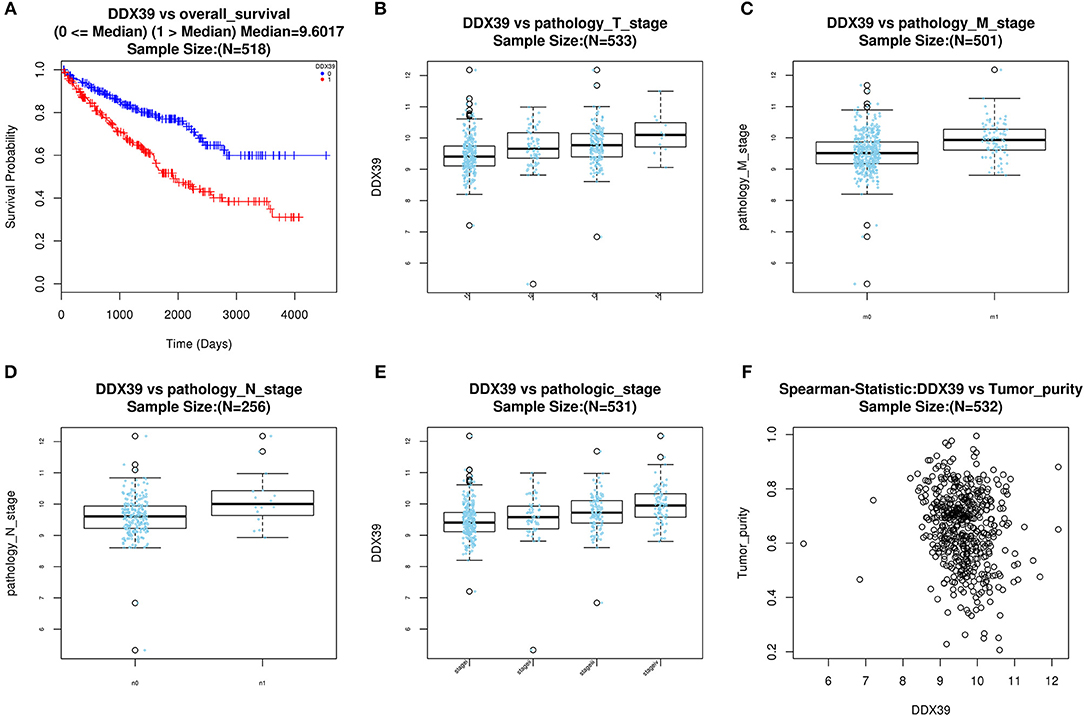
Figure 5. Evaluate prognostic value and clinical correlation of splicing factor DDX39B (Gene symbol: DDX39). (A) The Kaplan-Meier curve of DDX39; The expressions of DDX39 according to T (B), N (C), M (D) staging system, and clinical stage (E); (F) The spearman correlation analysis of DDX39 and tumor purity. DDX39B, DExD-Box Helicase 39B.
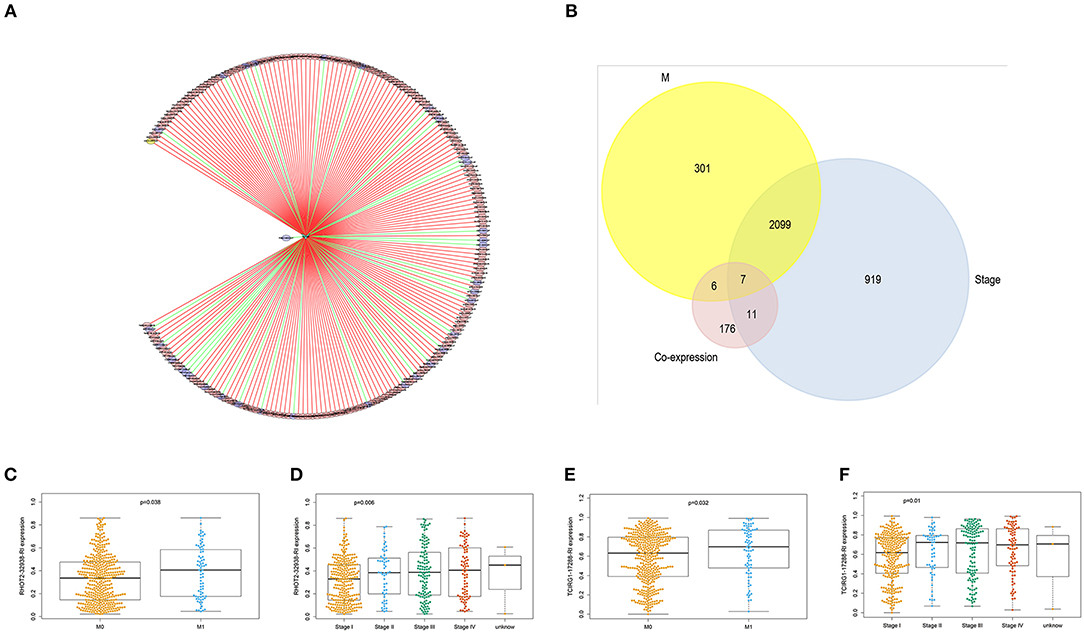
Figure 6. The identification of metastasis- and/or stage-related OS-SEs. (A) The network of OS-SEs and prognosis-related SFs; (B) The Venn plot to identify the overlapped OS-SEs related to clinical status and metastasis; The beeswarm plots of RHOT2-32938-RI (C) and TCIRG1-17288-RI (D) according to metastasis or not; The beeswarm plots of RHOT2-32938-RI (E), and TCIRG1-17288-RI (F) according to clinical status. OS-SEs, overall survival-related ASEs; SFs, splicing factors; RHOT2, Retained Intron of Ras Homolog Family Member T2; TCIRG1, T-Cell Immune Regulator 1.
External Validation
The parent genes of the 7 OS-SEs were validated in external databases. RHOT2 and TCIRG1 were confirmed in all the external databases (Table 1). In the database of UALCAN and LinkedOmics, RHOT2, and TCIRG1 were up-regulated in tumor than normal tissue (Figure 7A, Supplementary Figure 3A) and associated with tumor stage (Figure 7B, Supplementary Figure 3B) and OS (Figure 7C, Supplementary Figure 3C) significantly. In the Kaplan Meier plotter and SurvExpress, RHOT2, and TCIRG1 were also associated with OS significantly (Figure 7D, Supplementary Figure 3D). The survicalROC was also described in Figure 7E. Validation in the Human Protein Atlas revealed that the protein levels of RHOT2 and TCIRG1 in KIRC were significantly higher than those in normal kidney tissue (Figures 7F,F′, Table 2).
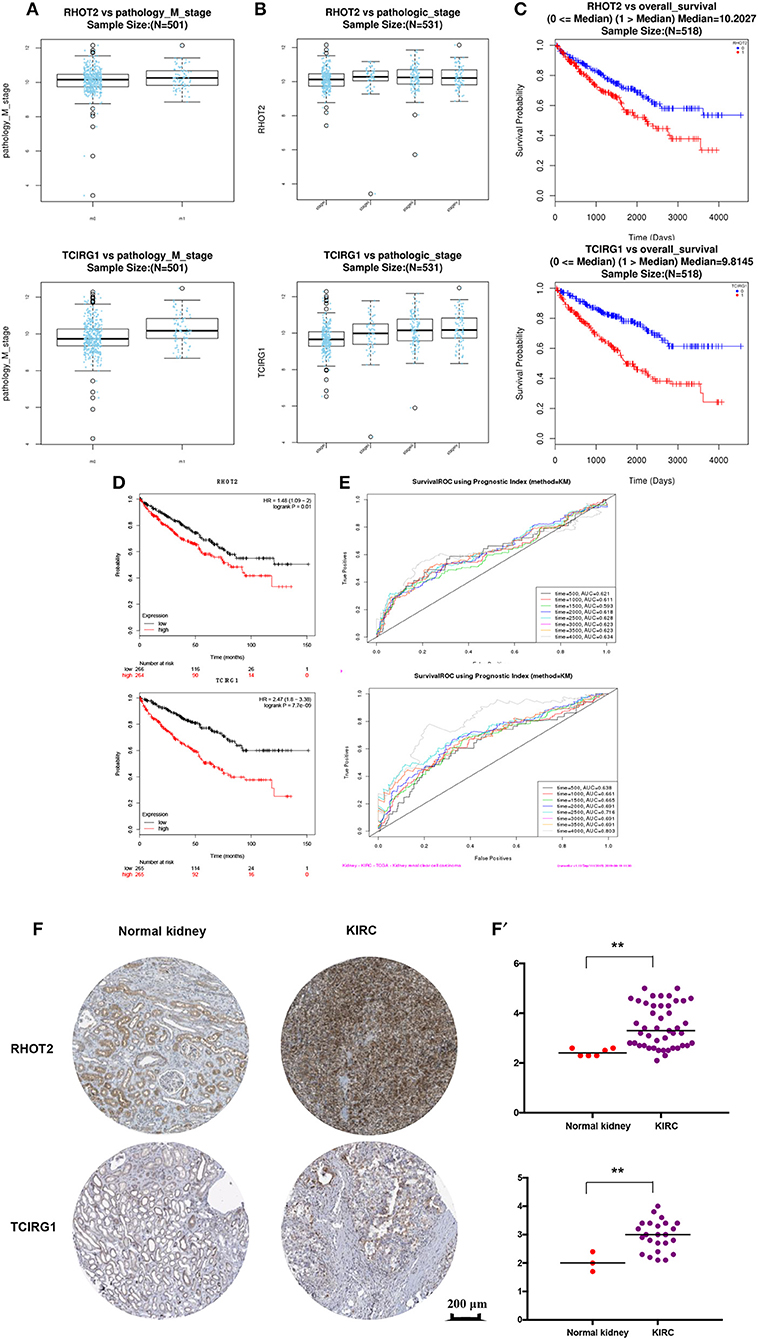
Figure 7. The external validation of RHOT2 and TCIRG1: (A) The expressions of RHOT2 and TCIRG1 between normal kidney and KIRC in UALCAN; (B) The expressions of RHOT2 and TCIRG1 according to clinical stage in UALCAN; The Kaplan-Meier curve of RHOT2 and TCIRG1 in LinkedOmics (C) and Kaplan Meier plotter (D); (E) The survicalROC of RHOT2 and TCIRG1; The IHC (F) and H-score (F′) of RHOT2 and TCIRG1 between normal kidney and KIRC in the Human Protein Atlas. RHOT2, Retained Intron of Ras Homolog Family Member T2; TCIRG1, T-Cell Immune Regulator 1; KIRC, kidney renal clear cell carcinoma; IHC, Immunohistochemistry; H-score, histochemistry score.
Comprehensive Analysis of ASEs and Signaling Pathways
A total of 90 KEGG pathways were identified as the OS-related KEGG pathways in GSVA and the univariate Cox regression analysis (Figure 8A). As shown, RHOT2–32938–RI was associated with sphingolipid metabolism, N-glycan biosynthesis and glycosphingolipid biosynthesis lacto and neolacto series. TCIRG1-17288-RI was associated with sphingolipid metabolism, purine metabolism and N-glycan biosynthesis (Figure 8B).
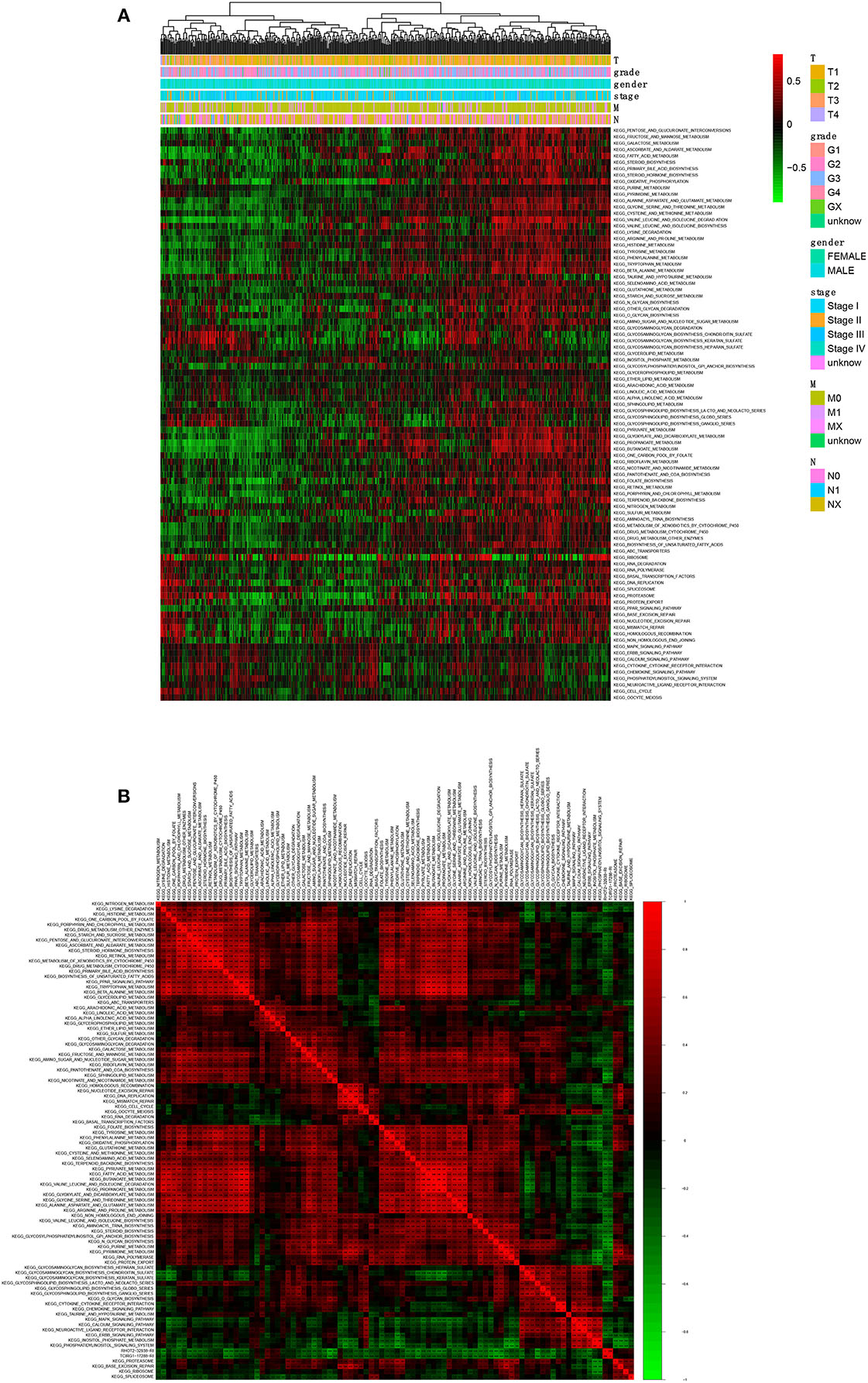
Figure 8. The co-expression analysis between ASEs and signaling pathways. (A): The co-expression heatmap of OS-related KEGG pathways; (B) The co-expression heatmap of OS-related KEGG pathways, RHOT2-32938-RI and TCIRG1-17288-RI. KEGG, Kyoto Encyclopedia of Genes and Genomes; RHOT2, Retained Intron of Ras Homolog Family Member T2; TCIRG1, T-Cell Immune Regulator 1.
Discussion
KIRC, one of the most prevalent genitourinary malignancies, is often associated with malignant disease progression and poor therapeutic outcomes. Approximately 30 % KIRC patients were found to be metastastic at initial diagnosis (Gupta et al., 2008). Metastatic KIRC evolves from primary KIRC and harbors multiple subpopulations with transcriptomic features, however, the molecular mechanisms of KIRC tumorigenesis and metastasis still remain unclear. In the meantime, effective diagnostic and prognostic biomarkers were still lacking (Song et al., 2018). Recently, aberrant ASs along with corresponding SFs were shown to have great potential value in exploring cancer biology, as both ASEs and SFs have been proved to produce different isoforms of onco-proteins which are associated with cell proliferation, anti-apoptosis and metastasis (Zhang et al., 2019). However, the roles of OS-SEs, SFs and signaling pathways in the tumorigenesis, metastasis, and prognosis of patients with KIRC were not quite clear.
In our study, a total of 6,081 OS-SEs were identified by univariate Cox regression analysis and we constructed a prediction model based on 5 OS-SEs (C4orf19-69001-AT, C16orf13-32924-ES, KIAA0930-62645-AP, FAM120C-89237-AT, UACA-31439-AP) screened by Lasso regression. Compared with previous prediction model of KIRC, the present on had a higher reliability (AUC: 0.788), with less predicters (Song et al., 2019). Additionally, the risk score was proved to be an independent prognostic factor, suggesting the good applicability in clinic for patients with KIRC.
DDX39B, as a DExD RNA helicase, was known to be involved in transportation of mRNA from nuclear to cytoplasm and pre-mRNA splicing (Shen, 2009). Particularly, the ATPase activity of DDX39B played an essential role in unwinding U4/U6 snRNA duplex and connecting U2 snRNP to the pre-mRNA in the process of ASE (Shen et al., 2008). In the present study, DDX39B was the only SF, whose associated OS-SEs were correlated with OS and metastasis by developing the network of OS-SEs and prognosis-related SFs. Similar to our study, mounting evidences regarded DDX39B as an important SF in triggering the progression and metastasis of various cancers. In BioXpress database, the expression level DDX39B was elevated in 75% (12 in 16) types of cancers (Awasthi et al., 2018a,b). Awastthi S et al. found that DDX39B could regulate the transcription and stability of pre-ribosomal RNA, the global translation, cell growth and proliferation. Furthermore, a study regarding to androgen receptor splice variant AR-V7 indicated that DDX39B could serve as the accelerator of AR-V7 mRNA expression and escalated DDX39B could result in resistance to androgen deprivation therapy and poor prognosis in patients with prostate cancer (Nakata et al., 2017).
Among these identified metastasis-associated OS-SEs, the parent genes of RHOT2-32938-RI and TCIRG1-17288-RI were verified by comprehensive databases. RHOT2 gene encodes a member of Rho family of GTPase, which are localized to the outer membrane of mitochondria (Wang et al., 2011). It plays an active role in mitochondrial fusion-fission dynamics, trafficking mitophagy function (Zheng et al., 2015). Mitochondrial dynamics was shown to be reprogrammed in tumor cells via gathering mitochondria at the cortical cytoskeleton (Caino et al., 2016). The mechanism could power the membrane machinery of cell movements, maintained phosphorylation of cell motility kinases, and heightened tumor invasion, chemotaxis, and metastasis (Caino et al., 2016; Agarwal et al., 2019). Besides, remodeling of mitochondrial functions is considered the commonest modified downstream of MYC gene, due to the MYC-dependent transcriptional control of GTPase RHOT1/RHOT2 and posttranslational modifications, such as RHOT phosphorylation by PINK kinase (Wang et al., 2011; Bailey et al., 2018). However, the exact function of RHOT2 has not been explored yet in KIRC. In our study, we found that abnormal expression of ASE of RHOT2 regulated by aberrant DDX39B could result in poor prognosis and tumor metastasis in patients with KIRC. Additionally, we also found out RHOT2-32938-RI was associated with sphingolipid metabolism, N-glycan biosynthesis and glycosphingolipid biosynthesis lacto and neolacto series by co-expression analysis. This might be a novel posttranslational regulation and new function for RHOT2 in KIRC tumorigenesis, progression and metastasis.
TCIRG1 (T-Cell Immune Regulator 1), also known as TIRC7, is essential in T cell activation (Heinemann et al., 1999). Previous studies revealed that TCIRG1 was widely up-regulated in numerous tumors, such as hepatocellular carcinoma, esophageal adenocarcinoma and breast cancer, which might be associated with autophagic sequestration and degradation (Blair and Athanasou, 2004; Hinton et al., 2009; Botelho et al., 2010). With regard to tumor metastasis, TCIRG1 was reported to modulate the EMT regulatory proteins, such as E-cadherin, N-cadherin, Fibronectin, Vimentin, Snail and Slug, and regulate tumor invasion and metastasis in MDA-MB-231, B16-F10, and SNU475 cells (Hinton et al., 2009; Yotsumoto et al., 2013; Yang et al., 2018; Zhou et al., 2018). In our study, we found that aberrant ASE of TCIRG1 was associated with poor prognosis and tumor metastasis in patients with KIRC. In addition, the parent gene TCIRG1 was verified to be associated with OS and metastasis by external database. With regard to KIRC metastasis, bone is one of the most common sites. In this case, patients often experience local pain and even pathological fracture due to osteolytic destruction. As TCIRG1 encodes the osteoclast-specific 116-kD subunit of the vacuolar proton pump and its defect is responsible for a subset of human autosomal recessive osteopetrosis (Frattini et al., 2000), TCIRG1 could be regarded as the potential targets for KIRC metastasis, especially to skeletal metastasis. Nowadays, many anti-TCIRG1 specific monoclonal antibody (mAb) have been developed for different diseases (Kumamoto et al., 2004; Utku et al., 2006). Thus, further cell and animal experiments should be performed to detect the therapeutic effects of anti-TCIRG1 specific mAb in KIRC skeletal metastasis.
To further investigate the deep mechanism of DDX39B regulating RHOT2-32938-RI and TCIRG1-17288-RI, sphingolipid metabolism and N-glycan biosynthesis were identified as the overlapped co-expression signal pathways. Sphingolipids are the major molecules presenting on the cell membranes, which are composed of sphingosine-1-phosphate (S1P) and ceramide (Ogretmen, 2018). Sphingolipids metabolism is pivotal for normal cellular homeostasis with various events, including endocytosis, nutrient transport and protein synthesis. Bioactive sphingolipid could induce cell motility, migration and phenotypic plasticity, which result in tumor invasion and metastasis (Kumamoto et al., 2004; Bonora et al., 2015; Ogretmen, 2018).
N-glycans biosynthesis play important roles in the immune system, pathogen recognition and tumor metastasis via regulating cell adhesion and ligand recognition (Kadam, 2016). The modification of N-glycans also alter cell-cell or cell-matrix contacts and contribute to EMT, invasion and metastasis (Kadam, 2016). E-cadherin, an adhesion molecule, harbors mainly bisecting N-glycans by MGAT3 enzyme in normal epithelial cells. In the tumorigenesis, MGAT3 is down-regulated by promoter methylation and its counterpart MGAT5 is up-regulated. This change results in the formation of tri- and tetraantennary complex glycans on cadherins and E-cadherin internalization to the cytoplasm (Pinho et al., 2013).
There were still some limitations in our study. First of all, this study was a pure bioinformatics study, and the scientific hypothesis was not proved by biological experiments. Second, although the results were verified by external database, the sequencing data were obtained from one single cohort and the sample size was limited. Third, only the primary samples were found in TCGA database and the lack of samples of metastatic sites, such as lung, bone and brain, made this study less complete. At last, the limitation of single omics analysis was also an inherent defect of this study.
In the future, in order to verify the important roles of RHOT2 and TCIRG1 in KIRC metastasis, the functional experiment, such as wound healing and transwell, will be performed in KIRC in which the RHOT2 or TCIRG1 gene has been knocked out (Calabretta et al., 2016; Qi et al., 2016; Chen et al., 2017; Couture et al., 2017; Zhou et al., 2017). Next, these cells will also be used in the nude mouse tumor metastasis model. In addition, a direct mechanism experiment proving the direct mechanism of the splicing factor DDX39B producing splicing isoforms of RHOT2 and TCIRG1 will also be performed. Furthermore, clinical sampes of lung, bone and brain metastasis from KIRC will also be used to detect the expressions of RHOT2 and TCIRG1.
Conclusions
In conclusion, we established the prediction model with good with good performance in external validation. Based on the comprehensive bioinformatics analysis, we proposed that aberrant DDX39B regulated RHOT2-32938-RI and TCIRG1-17288-RI might be related to the tumorigenesis, metastasis and poor prognosis of KIRC via sphingolipid metabolism or N-glycan biosynthesis pathway.
Data Availability Statement
All datasets for this study are included in the TCGA-KIRC program.
Ethics Statement
This study was approved by the Ethics Committee of Tongji Hospital, Tongji University School of Medicine.
Author Contributions
TM, RH, ZZ, ZH, HY, CJ, PY, PH, XZ, ZL, DS, JZ, and LC: conception, design, manuscript writing, and final approval of manuscript. DS, JZ, and LC: provision of study material. TM, RH, and ZZ: collection and assembly of data. TM, RH, ZZ, ZH, HY, CJ, PY, PH, XZ, and ZL: Data analysis and interpretation.
Funding
This study was supported in part by the National Natural Science Foundation of China (Nos. 81702659, 81772856), Youth Fund of Shanghai Municipal Health Planning Commission (Nos. 2017YQ054, 2017Y0117); Henan medical science and technology research project (Grant No. 201602031).
Conflict of Interest
The authors declare that the research was conducted in the absence of any commercial or financial relationships that could be construed as a potential conflict of interest.
Acknowledgments
We thank the TCGA team of the National Cancer Institute for using their data.
Supplementary Material
The Supplementary Material for this article can be found online at: https://www.frontiersin.org/articles/10.3389/fbioe.2019.00270/full#supplementary-material
Supplementary Figure 1. Bubble plots of the top 20 most significant OS-SEs among seven types of splicing patterns: (A) AA; (B) AD; (C) AP; (D) AT; (E) ES; (F) ME; (G) RI. OS-SEs, overall survival-related ASEs; AA, alternate acceptor; AD, alternate donor; AP, alternate promoter; AT, alternate terminator; ES, exon skip; ME, mutually exclusive exons; RI, retained intron.
Supplementary Figure 2. The beeswarm plots of CALCOCO1-22108-RI (A), CIRBP-46432-RI (B), P4HTM-64788-ES (C), TBC1D17-51116-ES (D), THOP1-46623-AP (E) according to metastasis or not; The beeswarm plots of CALCOCO1-22108-RI (F), CIRBP-46432-RI (G), P4HTM-64788-ES (H), TBC1D17-51116-ES (I), THOP1-46623-AP (J) according to clinical status. CALCOCO1, calcium binding and coiled-coil domain 1; CIRBP, cold inducible RNA binding protein; P4HTM, prolyl 4-hydroxylase, transmembrane; TBC1D17, TBC1 domain family member 17; THOP1, thimet oligopeptidase 1.
Supplementary Figure 3. The expressions of RHOT2 and TCIRG1 according to M (A) and clinical stage (B) in LinkedOmics; The Kaplan-Meier curve of RHOT2 and TCIRG1 in UALCAN (C) and SurvExpress (D). RHOT2, Retained Intron of Ras Homolog Family Member T2; TCIRG1, T-Cell Immune Regulator.
Abbreviations
KIRC, Kidney renal clear cell carcinoma; AS, Alternative splicing; ASEs, alternative splicing events; OS-SEs, overall survival-related ASEs; DDX39B, DExD-Box Helicase 39B; RHOT2, Retained Intron of Ras Homolog Family Member T2; TCIRG1, T-Cell Immune Regulator 1; SFs, splicing factors; EMT, epithelial-mesenchymal transition; GO, Gene Ontology; KEGG, Kyoto Encyclopedia of Genes and Genomes; mAb, monoclonal antibody; S1P, sphingosine-1-phosphate; TCGA, the Cancer Genome Atlas; PSI, percent splicing; GSVA, Gene Set Variation Analysis; IHC, Immunohistochemistry; AUC, Area under curve.
References
Agarwal, E., Altman, B. J., Ho Seo, J., Bertolini, I., Ghosh, J. C., Kaur, A., et al. (2019). Myc regulation of a mitochondrial trafficking network mediates tumor cell invasion and metastasis. Mol. Cell. Biol. 39, e00109–19. doi: 10.1128/MCB.00109-19
Aguirre-Gamboa, R., Gomez-Rueda, H., Martínez-Ledesma, E., Martínez-Torteya, A., Chacolla-Huaringa, R., Rodriguez-Barrientos, A., et al. (2013). SurvExpress: an online biomarker validation tool and database for cancer gene expression data using survival analysis. PLoS ONE 8:e74250. doi: 10.1371/journal.pone.0074250
Awasthi, S., Chakrapani, B., Mahesh, A., Chavali, P. L., Chavali, S., and Dhayalan, A. (2018a). DDX39B promotes translation through regulation of pre-ribosomal RNA levels. RNA Biol. 15, 1157–1166. doi: 10.1080/15476286.2018.1517011
Awasthi, S., Verma, M., Mahesh, A., K Khan, M. I., Govindaraju, G., Rajavelu, A., et al. (2018b). DDX49 is an RNA helicase that affects translation by regulating mRNA export and the levels of pre-ribosomal RNA. Nucleic Acids Res. 46, 6304–6317. doi: 10.1093/nar/gky231
Bailey, M. H., Tokheim, C., Porta-Pardo, E., Sengupta, S., Bertrand, D., Weerasinghe, A., et al. (2018). Comprehensive characterization of cancer driver genes and mutations. Cell 174, 1034–1035. doi: 10.1016/j.cell.2018.07.034
Blair, H. C., and Athanasou, N. A. (2004). Recent advances in osteoclast biology and pathological bone resorption. Histol. Histopathol. 19, 189–199. doi: 10.14670/HH-19.189.
Bonora, M., Wieckowsk, M. R., Chinopoulos, C., Kepp, O., Kroemer, G., Galluzzi, L., et al. (2015). Molecular mechanisms of cell death: central implication of ATP synthase in mitochondrial permeability transition. Oncogene 34:1608. doi: 10.1038/onc.2014.462
Botelho, N. K., Schneiders, F. I., Lord, S. J., Freeman, A. K., Tyagi, S., Nancarrow, D. J., et al. (2010). Gene expression alterations in formalin-fixed, paraffin-embedded Barrett esophagus and esophageal adenocarcinoma tissues. Cancer Biol. Ther. 10, 172–179. doi: 10.4161/cbt.10.2.12166
Caino, M. C., Seo, J. H., Aguinaldo, A., Wait, E., Bryant, K. G., Kossenkov, A. V., et al. (2016). A neuronal network of mitochondrial dynamics regulates metastasis. Nat. Commun. 7:13730. doi: 10.1038/ncomms13730
Calabretta, S., Bielli, P., Passacantilli, I., Pilozzi, E., Fendrich, V., Capurso, G., et al. (2016). Modulation of PKM alternative splicing by PTBP1 promotes gemcitabine resistance in pancreatic cancer cells. Oncogene 35, 2031–2039. doi: 10.1038/onc.2015.270
Chandrashekar, D. S., Bashel, B., Balasubramanya, S. A. H., Creighton, C. J., Ponce-Rodriguez, I., et al. (2017). UALCAN: a portal for facilitating tumor subgroup gene expression and survival analyses. Neoplasia 19, 649–658. doi: 10.1016/j.neo.2017.05.002
Chen, K., Xiao, H., Zeng, J., Yu, G., Zhou, H., Huang, C., et al. (2017). Alternative splicing of EZH2 pre-mRNA by SF3B3 contributes to the tumorigenic potential of renal cancer. Clin. Cancer Res. 23, 3428–3441. doi: 10.1158/1078-0432.CCR-16-2020
Chen, Q. F., Li, W., Wu, P., Shen, L., and Huang, Z. L. (2019). Alternative splicing events are prognostic in hepatocellular carcinoma. Aging (Albany. NY) 11, 4720–4735. doi: 10.18632/aging.102085
Couture, F., Sabbagh, R., Kwiatkowska, A., Desjardins, R., Guay, S. P., Bouchard, L., et al. (2017). PACE4 undergoes an oncogenic alternative splicing switch in cancer. Cancer Res. 77, 6863–6879. doi: 10.1158/0008-5472.CAN-17-1397
Deng, M., Brägelmann, J., Kryukov, I., Saraiva-Agostinho, N., and Perner, S. (2017). FirebrowseR: an R client to the broad institute's firehose pipeline. Database (Oxford) 2017:baw160. doi: 10.1093/database/baw160
Frankiw, L., Baltimore, D., and Li, G. (2019). Alternative mRNA splicing in cancer immunotherapy. Nat. Rev. Immunol. doi: 10.1038/s41577-019-0195-7. [Epub ahead of print].
Frattini, A., Orchard, P. J., Sobacchi, C., Giliani, S., Abinun, M., Mattsson, J. P., et al. (2000). Defects in TCIRG1 subunit of the vacuolar proton pump are responsible for a subset of human autosomal recessive osteopetrosis. Nat. Genet. 25, 343–346. doi: 10.1038/77131
Gupta, K., Miller, J. D., Li, J. Z., Russell, M. W., and Charbonneau, C. (2008). Epidemiologic and socioeconomic burden of metastatic renal cell carcinoma (mRCC): a literature review. Cancer Treat. Rev. 34, 193–205. doi: 10.1016/j.ctrv.2007.12.001
Hänzelmann, S., Castelo, R., and Guinney, J. (2013). GSVA: gene set variation analysis for microarray and RNA-seq data. BMC Bioinformatics 14:7. doi: 10.1186/1471-2105-14-7
Heinemann, T., Bulwin, G. C., Randall, J., Schnieders, B., Sandhoff, K., Volk, H. D., et al. (1999). Genomic organization of the gene coding for TIRC7, a novel membrane protein essential for T cell activation. Genomics 57, 398–406. doi: 10.1006/geno.1999.5751
Hinton, A., Sennoune, S. R., Bond, S., Fang, M., Reuveni, M., Sahagian, G. G., et al. (2009). Function of a subunit isoforms of the V-ATPase in pH homeostasis and in vitro invasion of MDA-MB231 human breast cancer cells. J. Biol. Chem. 284, 16400–16408. doi: 10.1074/jbc.M901201200
Hsieh, J. J., Purdue, M. P., Signoretti, S., Swanton, C., Albiges, L., Schmidinger, M., et al. (2017). Renal cell carcinoma. Nat. Rev. Dis. Primers 3:17009. doi: 10.1038/nrdp.2017.9
Jonasch, E. (2018). Updates to the management of kidney cancer. J. Natl. Compr. Canc. Netw. 16, 639–641. doi: 10.6004/jnccn.2018.0039
Jonasch, E., Gao, J., and Rathmell, W. K. (2014). Renal cell carcinoma. BMJ 349:g4797. doi: 10.1136/bmj.g4797
Kadam, P. D. (2016). Rectocutaneous fistula with transmigration of the suture: a rare delayed complication of vault fixation with the sacrospinous ligament. Int. Urogynecol. J. 27, 155–157. doi: 10.1007/s00192-015-2823-5
Kouyama, Y., Masuda, T., Fujii, A., Ogawa, Y., Sato, K., Tobo, T., et al. (2019). Oncogenic splicing abnormalities induced by DEAD-Box Helicase 56 (DDX56) amplification in colorectal cancer. Cancer Sci. 110, 3132–3144. doi: 10.1111/cas.14163
Kumamoto, Y., Tomschegg, A., Bennai-Sanfourche, F., Boerner, A., Kaser, A., Schmidt-Knosalla, I., et al. (2004). Monoclonal antibody specific for TIRC7 induces donor-specific anergy and prevents rejection of cardiac allografts in mice. Am. J. Transplant 4, 505–514. doi: 10.1111/j.1600-6143.2004.00367.x
Lee, S. C., and Abdel-Wahab, O. (2016). Therapeutic targeting of splicing in cancer. Nat. Med. 22, 976–986. doi: 10.1038/nm.4165
Montes, M., Sanford, B. L., Comiskey, D. F., and Chandler, D. S. (2019). RNA splicing and disease: animal models to therapies. Trends Genet. 35, 68–87. doi: 10.1016/j.tig.2018.10.002
Nagy, A., Lanczky, A., Menyhart, O., and Gyorffy, B. (2018). Validation of miRNA prognostic power in hepatocellular carcinoma using expression data of independent datasets. Sci. Rep. 8:9227. doi: 10.1038/s41598-018-29514-3
Nakata, D., Nakao, S., Nakayama, K., Araki, S., Nakayama, Y., Aparicio, S., et al. (2017). The RNA helicase DDX39B and its paralog DDX39A regulate androgen receptor splice variant AR-V7 generation. Biochem. Biophys. Res. Commun. 483, 271–276. doi: 10.1016/j.bbrc.2016.12.153
Ogretmen, B. (2018). Sphingolipid metabolism in cancer signalling and therapy. Nat. Rev. Cancer 18, 33–50. doi: 10.1038/nrc.2017.96
Pinho, S. S., Figueiredo, J., Cabral, J., Carvalho, S., Dourado, J., Magalhães, A., et al. (2013). E-cadherin and adherens-junctions stability in gastric carcinoma: functional implications of glycosyltransferases involving N-glycan branching biosynthesis, N-acetylglucosaminyltransferases III and V. Biochim. Biophys. Acta 1830, 2690–2700. doi: 10.1016/j.bbagen.2012.10.021
Piva, F., Giulietti, M., Nocchi, L., and Principato, G. (2009). SpliceAid: a database of experimental RNA target motifs bound by splicing proteins in humans. Bioinformatics 25, 1211–1213. doi: 10.1093/bioinformatics/btp124
Porta, C., Cosmai, L., Leibovich, B. C., Powles, T., Gallieni, M., and Bex, A. (2019). The adjuvant treatment of kidney cancer: a multidisciplinary outlook. Nat. Rev. Nephrol. 15, 423–433. doi: 10.1038/s41581-019-0131-x
Qi, Y., Yu, J., Han, W., Fan, X., Qian, H., Wei, H., et al. (2016). A splicing isoform of TEAD4 attenuates the Hippo-YAP signalling to inhibit tumour proliferation. Nat. Commun. 7:ncomms11840. doi: 10.1038/ncomms11840
Ryan, M., Wong, W. C., Brown, R., Akbani, R., Su, X., Broom, B., et al. (2016). TCGASpliceSeq a compendium of alternative mRNA splicing in cancer. Nucleic Acids Res. 44, D1018–1022. doi: 10.1093/nar/gkv1288
Shannon, P., Markiel, A., Ozier, O., Baliga, N. S., Wang, J. T., Ramage, D., et al. (2003). Cytoscape: a software environment for integrated models of biomolecular interaction networks. Genome Res. 13, 2498–2504. doi: 10.1101/gr.1239303
Shen, H. (2009). UAP56- a key player with surprisingly diverse roles in pre-mRNA splicing and nuclear export. BMB Rep. 42, 185–188. doi: 10.5483/BMBRep.2009.42.4.185
Shen, H., Zheng, X., Shen, J., Zhang, L., Zhao, R., and Green, M. R. (2008). Distinct activities of the DExD/H-box splicing factor hUAP56 facilitate stepwise assembly of the spliceosome. Genes Dev. 22, 1796–1803. doi: 10.1101/gad.1657308
Song, E., Song, W., Ren, M., Xing, L., Ni, W., Li, Y., et al. (2018). Identification of potential crucial genes associated with carcinogenesis of clear cell renal cell carcinoma. J. Cell. Biochem. 119, 5163–5174. doi: 10.1002/jcb.26543
Song, J., Liu, Y. D., Su, J., Yuan, D., Sun, F., and Zhu, J. (2019). Systematic analysis of alternative splicing signature unveils prognostic predictor for kidney renal clear cell carcinoma. J. Cell. Physiol. 234, 22753–22764 doi: 10.1002/jcp.28840
Sveen, A., Kilpinen, S., Ruusulehto, A., Lothe, R. A., and Skotheim, R. I. (2016). Aberrant RNA splicing in cancer; expression changes and driver mutations of splicing factor genes. Oncogene 35, 2413–2427. doi: 10.1038/onc.2015.318
Utku, N., Heinemann, T., Winter, M., Bulwin, C. G., Schlawinsky, M., Fraser, P., et al. (2006). Antibody targeting of TIRC7 results in significant therapeutic effects on collagen-induced arthritis in mice. Clin. Exp. Immunol. 144, 142–151. doi: 10.1111/j.1365-2249.2006.03044.x
Vasaikar, S. V., Straub, P., Wang, J., and Zhang, B. (2018). LinkedOmics: analyzing multi-omics data within and across 32 cancer types. Nucleic Acids Res. 46, D956–D963. doi: 10.1093/nar/gkx1090
Wang, C., Zheng, M., Wang, S., Nie, X., Guo, Q., Gao, L., et al. (2019). Whole genome analysis and prognostic model construction based on alternative splicing events in endometrial cancer. Biomed Res. Int. 2019:2686875. doi: 10.1155/2019/2686875
Wang, E. T., Sandberg, R., Luo, S., Khrebtukova, I., Zhang, L., Mayr, C., et al. (2008). Alternative isoform regulation in human tissue transcriptomes. Nature 456, 470–476. doi: 10.1038/nature07509
Wang, X., Winter, D., Ashrafi, G., Schlehe, J., Wong, Y. L., Selkoe, D., et al. (2011). PINK1 and Parkin target Miro for phosphorylation and degradation to arrest mitochondrial motility. Cell 147, 893–906. doi: 10.1016/j.cell.2011.10.018
Wu, H. Y., Peng, Z. G., He, R. Q., Luo, B., Ma, J., Hu, X. H., et al. (2019). Prognostic index of aberrant mRNA splicing profiling acts as a predictive indicator for hepatocellular carcinoma based on TCGA SpliceSeq data. Int. J. Oncol. 55, 425–438. doi: 10.3892/ijo.2019.4834
Xing, S., Li, Z., Ma, W., He, X., Shen, S., Wei, H., et al. (2019). DIS3L2 promotes progression of hepatocellular carcinoma via hnRNP U-mediated alternative splicing. Cancer Res. 79, 4923–4936. doi: 10.1158/0008-5472.CAN.-19-0376
Yang, H. D., Eun, J. W., Lee, K. B., Shen, Q., Kim, H. S., Kim, S. Y., et al. (2018). T-cell immune regulator 1 enhances metastasis in hepatocellular carcinoma. Exp. Mol. Med. 50:e420. doi: 10.1038/emm.2017.166
Yotsumoto, F., Tokunaga, E., Oki, E., Maehara, Y., Yamada, H., Nakajima, K., et al. (2013). Molecular hierarchy of heparin-binding EGF-like growth factor-regulated angiogenesis in triple-negative breast cancer. Mol. Cancer Res. 11, 506–517. doi: 10.1158/1541-7786.MCR-12-0428
Zhang, Y., Yan, L., Zeng, J., Zhou, H., Liu, H., Yu, G., et al. (2019). Pan-cancer analysis of clinical relevance of alternative splicing events in 31 human cancers. Oncogene 8, 6678–6695. doi: 10.1038/s41388-019-0910-7
Zheng, L., Li, X., Gu, Y., Lv, X., and Xi, T. (2015). The 3'UTR of the pseudogene CYP4Z2P promotes tumor angiogenesis in breast cancer by acting as a ceRNA for CYP4Z1. Breast Cancer Res. Treat. 150, 105–118. doi: 10.1007/s10549-015-3298-2
Zhou, M., Diao, Z., Yue, X., Chen, Y., Zhao, H., Cheng, L., et al. (2016). Construction and analysis of dysregulated lncRNA-associated ceRNA network identified novel lncRNA biomarkers for early diagnosis of human pancreatic cancer. Oncotarget 7:56383. doi: 10.18632/oncotarget.10891
Zhou, M., Zhang, Z., Zhao, H., Bao, S., Cheng, L., and Sun, J. (2018). An immune-related six-lncRNA signature to improve prognosis prediction of glioblastoma multiforme. Mol. Neurobiol. 55, 3684–3697. doi: 10.1007/s12035-017-0572-9
Keywords: alternative splicing, kidney renal clear cell carcinoma, prognosis, tumor metastasis, RHOT2, TCIRG1
Citation: Meng T, Huang R, Zeng Z, Huang Z, Yin H, Jiao C, Yan P, Hu P, Zhu X, Li Z, Song D, Zhang J and Cheng L (2019) Identification of Prognostic and Metastatic Alternative Splicing Signatures in Kidney Renal Clear Cell Carcinoma. Front. Bioeng. Biotechnol. 7:270. doi: 10.3389/fbioe.2019.00270
Received: 02 September 2019; Accepted: 30 September 2019;
Published: 15 October 2019.
Edited by:
Meng Zhou, Wenzhou Medical University, ChinaReviewed by:
Fang Zhong, Icahn School of Medicine at Mount Sinai, United StatesCong Jiang, Harvard University, United States
Copyright © 2019 Meng, Huang, Zeng, Huang, Yin, Jiao, Yan, Hu, Zhu, Li, Song, Zhang and Cheng. This is an open-access article distributed under the terms of the Creative Commons Attribution License (CC BY). The use, distribution or reproduction in other forums is permitted, provided the original author(s) and the copyright owner(s) are credited and that the original publication in this journal is cited, in accordance with accepted academic practice. No use, distribution or reproduction is permitted which does not comply with these terms.
*Correspondence: Dianwen Song, b3NvbmdkaWFud2VuQDEyNi5jb20=; Jie Zhang, amllemhhbmdAdG9uZ2ppLmVkdS5jbg==; Liming Cheng, bGltaW5nY2hlbmdAdG9uZ2ppLmVkdS5jbg==
†These authors have contributed equally to this work as co-first authors