- 1Integrative Omics Group, Biological Sciences Division, Pacific Northwest National Laboratory, Richland, WA, United States
- 2Department of Energy, Agile BioFoundry, Emeryville, CA, United States
- 3Biological Systems and Engineering Division, Lawrence Berkeley National Laboratory, Berkeley, CA, United States
- 4Department of Biology and Biological Engineering, Chalmers University of Technology, Gothenburg, Sweden
- 5Functional and Systems Biology Group, Environmental Molecular Sciences Division, Pacific Northwest National Laboratory, Richland, WA, United States
Editorial on the Research Topic
Multi-Omics Technologies for Optimizing Synthetic Biomanufacturing
Industrial manufacturing endures as an essential human activity yielding a variety of useful products; it plays a significant role in the global economy with huge impacts in everyday life. However, the manufacturing process requires consumption of various raw materials (especially petroleum derivatives), generates a variety of harmful waste products, causes pollution, and is energetically inefficient. Biological manufacturing from sustainable, affordable, and scalable feedstocks potentially enables the displacement of the entire portfolio of currently available products produced by industrial processes, enabling the manufacturing of renewable and eco-friendly products (Clomburg et al., 2017). Thus, successful development of a robust biomanufacturing strategy and technology platform, based on the latest advances in synthetic biology and chemical catalysis, will decrease both the cost and production time compared with previous manufacturing processes. Development of biomanufacturing processes using a synthetic biology platform requires the multidisciplinary efforts of science and engineering fields including molecular biology, microbiology, genetic engineering, informatics, metabolic modeling and chemical or process engineering (El Karoui et al., 2019).
In this research topic, Amer and Baidoo discussed the importance of using multi-omic analytical approaches to monitor and improve the biomanufacturing process. These approaches include genomics, transcriptomics, proteomics, metabolomics and fluxomics (Figure 1). The multi-omics data acquired from the biomanufacturing process not only provides potential solutions to low production efficiency by identifying underlying metabolic bottlenecks or pathway sinks, but also guides the understanding of how these modified biological systems function. Furthermore, such multi-omics technologies are constantly innovated and improved to expand molecular detection coverage, obtain data with increased accuracy by using new or novel analytical instruments, achieve better computational algorithms, and create wider and deeper databases to support a growing variety of biological host systems. Roy et al. described a combined computational tool to optimize the DBTL (Design-Build-Test-Learn) cycle in biomanufacturing process by collecting, visualizing, and utilizing large multi-omics datasets from various biological systems and emphasized their importance in the following metabolic engineering processes with machine learning approaches.
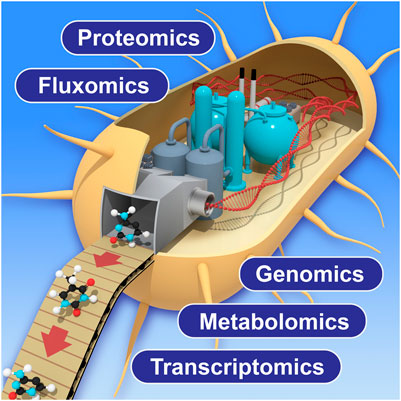
FIGURE 1. Schematic view of multi-omics application to biomanufacturing process. Improvement of each technology will enhance the measurement coverage and accuracy in future applications.
Gao et al. compared microflow and nanoflow liquid chromatography-selected reaction monitoring (LC-SRM) methods for analysis of hundreds of targeted peptides associated with 132 proteins in major pathways of Pseudomonas putida, a versatile bacterial host for production of bioproducts and biofuels via metabolic engineering. The increased throughput and accuracy of protein measurement will not only reduce the DBTL cycle time in future applications, but is, in addition, easily applied to other biomanufacturing host organisms.
Fletcher and Baetz reviewed the toxicity of phenolic compounds which are produced from pretreatment or hydrolysis of natural lignocellulosic biomass based on functional genomics and transcriptomics approaches, especially to the important model organism and industrial bioproduction host strain, Saccharomyces cerevisiae. Information regarding physiological tolerances of toxic phenolic compounds may be applied and evaluated in other host strains for future improvement. In that regard, Garcia et al. developed the genome-scale metabolic model of Clostridium thermocellum for efficient conversion of lignocellulosic biomass which has unique preference for its anaerobic and thermophilic growth attributes. This model will provide a useful tool to understand physiological and metabolic parameters associated with potential future biomanufacturing process.
Pinheiro et al. studied a xylose metabolism by Rhodosporidium toruloides, an oleaginous yeast with significant emerging potential in industrial applications, using a detailed physiological characterization interpreted with absolute proteomics and genome scale metabolic models. Kim et al. performed a multi-omics analysis on R. toruloides and the transcriptomics, proteomics, metabolomics and RB-TDNA sequencing data improved the current genome-scale model to make it a more exhaustive and accurate metabolic network model.
Pomraning et al. integrated high-throughput proteomics and metabolomics data as part of a DBTL cycle focused on improving production efficiency of 3-hydroxypropionic acid (3HP) in engineered Aspergillus pseudoterreus strains. This was the first report of 3HP production in a filamentous fungus amenable to industry-level biomanufacturing of organic acids at high titer and low pH. Chroumpi et al. studied another filamentous fungus Aspergillus niger for better understanding of pentose catabolic pathways by deletion of the key genes. The high-throughput multi-omics data (i.e., transcriptome, metabolome and proteome) generated on the mutant strains revealed that these genes are critical for metabolic pathways but not as critical for growth of A. niger on more complex biomass substrates, which raises fundamental questions on nutrient acquisition during growth on various carbon sources.
Wu et al. investigated the metabolic potential of Zymomonas mobilis for conversion of glucose and xylose to 2,3-butanediol. This study used calculated thermodynamic and kinetic parameters to generate insights of Z. mobilis metabolism. They also performed pathway and dynamic flux balance analysis to understand metabolic potential and production efficiency for future industrial applications. Nitta et al. acquired metabolomics and transcriptomics data on antibiotic producing strain, Streptomyces coelicolor to understand the functional connections between the production of antibiotic, actinorhodin and the level of cAMP. They found that higher levels of cAMP improved cell growth and production of actinorhodin, which was confirmed by the metabolomic and transcriptomic data.
We conclude by emphasizing that high-throughput multi-omics data play a critical role to unravel the complexities of metabolic engineering to improve production efficiency and product titer produced by a variety of industrial microbes. In addition, generation of multi-omics datasets accelerates the adoption and subsequent application of artificial intelligence approaches such as machine learning to design of improved microbial bioproduction host systems (Lawson et al., 2021). In terms of technological perspectives, enhanced high-throughput measurement and improved coverage of multi-omics analyses with higher accuracy will not only benefit in shortened DBTL cycle times for the metabolic engineering process, but also will lead to improved fundamental understanding of engineered biosystems. Refining tools and analytical platforms will benefit manipulating, modifying, and reshaping potential host systems. The long-term outcomes of these efforts will impact the world and our future by decarbonizing the current manufacturing processes via an environmental-friendly manner.
Author Contributions
Y-MK, CP, EK, and SB served as co-editors for the Research Topic: Multi-omics technologies for optimizing synthetic biomanufacturing. Y-MK conceived of the idea for the research topic, and all the authors contributed to writing the editorial.
Funding
The work was supported by Agile BioFoundry (http://agilebiofoundry.org), funded by the United States Department of Energy, Office of Energy Efficiency and Renewable Energy, Bioenergy Technologies Office, under Award No. DE-NL0030038. Pacific Northwest National Laboratory (PNNL) is operated for the U.S. Department of Energy by Battelle Memorial Institute under contract DE-AC05-76RL01830.
Conflict of Interest
The authors declare that the research was conducted in the absence of any commercial or financial relationships that could be construed as a potential conflict of interest.
Publisher’s Note
All claims expressed in this article are solely those of the authors and do not necessarily represent those of their affiliated organizations, or those of the publisher, the editors and the reviewers. Any product that may be evaluated in this article, or claim that may be made by its manufacturer, is not guaranteed or endorsed by the publisher.
References
Clomburg, J. M., Crumbley, A. M., and Gonzalez, R. (2017). Industrial Biomanufacturing: The Future of Chemical Production. Science 355, aag0804. doi:10.1126/science.aag0804
El Karoui, M., Hoyos-Flight, M., and Fletcher, L. (2019). Future Trends in Synthetic Biology-A Report. Front. Bioeng. Biotechnol. 7, 175. doi:10.3389/fbioe.2019.00175
Keywords: biomanufacturing, multi-omics analysis, synthetic biology, DBTL cycle, metabolic engineering
Citation: Kim Y-M, Petzold CJ, Kerkhoven EJ and Baker SE (2021) Editorial: Multi-Omics Technologies for Optimizing Synthetic Biomanufacturing. Front. Bioeng. Biotechnol. 9:818010. doi: 10.3389/fbioe.2021.818010
Received: 18 November 2021; Accepted: 29 November 2021;
Published: 15 December 2021.
Edited and reviewed by:
Jean Marie François, Institut Biotechnologique de Toulouse (INSA), FranceCopyright © 2021 Kim, Petzold, Kerkhoven and Baker. This is an open-access article distributed under the terms of the Creative Commons Attribution License (CC BY). The use, distribution or reproduction in other forums is permitted, provided the original author(s) and the copyright owner(s) are credited and that the original publication in this journal is cited, in accordance with accepted academic practice. No use, distribution or reproduction is permitted which does not comply with these terms.
*Correspondence: Young-Mo Kim, eW91bmctbW8ua2ltQHBubmwuZ292