- 1College of Mechanical and Electronic Engineering, Fujian Agriculture and Forestry University, Fuzhou, China
- 2College of Computer and Information Sciences, Fujian Agriculture and Forestry University, Fuzhou, China
Phenotypic analysis has significant potential for aiding breeding efforts. However, there is a notable lack of studies utilizing phenotypic analysis in the field of edible fungi. Pleurotus geesteranus is a lucrative edible fungus with significant market demand and substantial industrial output, and early-stage phenotypic analysis of Pleurotus geesteranus is imperative during its breeding process. This study utilizes image recognition technology to investigate the phenotypic features of the mycelium of P. geesteranus. We aim to establish the relations between these phenotypic characteristics and mycelial quality. Four groups of mycelia, namely, the non-degraded and degraded mycelium and the 5th and 14th subcultures, are used as image sources. Two categories of phenotypic metrics, outline and texture, are quantitatively calculated and analyzed. In the outline features of the mycelium, five indexes, namely, mycelial perimeter, radius, area, growth rate, and change speed, are proposed to demonstrate mycelial growth. In the texture features of the mycelium, five indexes, namely, mycelial coverage, roundness, groove depth, density, and density change, are studied to analyze the phenotypic characteristics of the mycelium. Moreover, we also compared the cellulase and laccase activities of the mycelium and found that cellulase level was consistent with the phenotypic indices of the mycelium, which further verified the accuracy of digital image processing technology in analyzing the phenotypic characteristics of the mycelium. The results indicate that there are significant differences in these 10 phenotypic characteristic indices (
1 Introduction
Pleurotus geesteranus, a globally cultivated edible fungus, plays a significant role in economies worldwide (Singh et al., 2021; Wei et al., 2023). Analysis of P. geesteranus market prospects in 2024 showed that the global P. geesteranus market size is approximately 10.9 billion US dollars and is expected to grow at an annual rate of about 5%. In 2023, P. geesteranus accounted for 17.45% of the output of edible fungi in China. The seeds of edible fungi are referred to as edible fungus strains. According to the source, generation of propagation, and production purposes, they can be divided into three levels: mother strain, original strain, and cultivation strain. The mycelial quality is intricately tied to its yield, making it a crucial factor influencing economic benefits (Zhang et al., 2019). Mycelial degeneration refers to the phenomenon where the traits of edible fungus populations deteriorate, leading to deviations in yield, quality, and resistance that do not meet human needs. Mycelial degeneration is a serious issue commonly encountered in the cultivation of various edible fungi. In practical production, mycelial degradation generally takes place during its preservation and subculture. Using degenerated strains for cultivation often results in delayed fruiting, low yield, and poor quality, which cause significant economic losses to producers of edible fungus (Pérez et al., 2021). The mycelial phenotype is the collective expression of its individual traits within a certain environment and provides fundamental information for breeding purposes (Helgason and Fitter, 2009). Mycelial degeneration of P. geesteranus is both complex and varied. To optimize the high production cultivation of the mycelium of P. geesteranus, breeders need to evaluate the mycelial phenotype by gathering phenotypic information through sampling, measurement, observation, and calculation techniques (Lehmann et al., 2019; James et al., 2022). Phenotypic parameters including mycelium growth rate, density, color, and content of specific substances such as polysaccharides, active proteins, and other bioactive components of the strain serve as the primary criteria for assessing the mycelial quality of P. geesteranus (Yang et al., 2022). In the initial stages of cultivation, accurately distinguishing the degenerated mycelium based on phenotypic characteristics is crucial (Danner et al., 2023). This process aids in effective selection and cultivation of the mycelium, while also preventing the degenerated mycelium from flowing into the production process, ultimately enhancing overall economic efficiency.
To date, the phenotypic characteristics of edible fungi have been assessed through observation, resulting in drawbacks like low precision, inadequate standardization, time-intensive procedures, and high-intensity labor (Furbank and Tester, 2011; Yin et al., 2022; Liu et al., 2023). Digital image processing is capable of precise computation for qualitative and quantitative analyses, instead of manual measurement, which often relies on subjective judgment based on experience. Recently, digital image processing has emerged as a vital analytical method for evaluating the phenotypic attributes of crops, encompassing factors like crop color, growth, density, and uniformity (Mahajan et al., 2015; Zhao et al., 2019; Omari et al., 2020). This approach involves analyzing pixel attributes and inter-pixel attributes and analyzing the dynamic characteristics of their trait expression over growth time (Chen et al., 2014). Guo et al. (2018) extracted a total of 51 image-based traits from 507 rice samples for studying the plant drought tolerance traits and assisting in drought-resistant breeding. Li B. et al. (2020) conducted a systematic analysis of 119 image-based digital traits of 200 cotton seedlings under drought stress. Li H. et al. (2020) quantified the correlation among 43 phenotypic characteristics of rapeseed with their growth conditions and predicted the final rapeseed yield. Currently, researchers have attained high-throughput, high-precise, and non-destructive acquisition of crop phenotypes for staple food crops like rice, wheat, and soybean, utilizing digital image processing technologies. This motivates the focus toward digital and efficient breeding research (Shakoor et al., 2017).
The phenotypes of edible fungi associated with mycelial quality are varied and intricate, encompassing factors such as growth rate, density, color, and content of specific substances. These phenotypic characteristics have a strong correlation with mycelial quality (Yoo et al., 2019). Specifically, it is generally manifested in the following aspects: 1) mycelial morphology: the relation between mycelial morphology and quality lies in the fact that the more regular and faster-growing species often have higher quality; 2) mycelial density: the higher the mycelial density, the more vigorous the mycelial growth and the higher the yield; 3) mycelial growth rate: the mycelium with faster growth rates can quickly occupy growth space and reduce competitive pressure, thereby improving yield; 4) mycelial color: the color of the mycelium can reflect the metabolic state and growth environment. The mycelium with bright and uniform coloration usually grows vigorously.
At present, the current research and applications of digital image processing for phenotypic analysis of P. geesteranus remain open. The objective of this paper is to investigate the inherent relations between the phenotypic characteristics of P. geesteranus and its mycelial quality. We acquired imaging data depicting four groups of the mycelium of P. geesteranus, encompassing both non-degraded and degraded states. We extracted two pivotal phenotypic characteristics of the mycelium, outline and texture, including a total of 10 indices and carried out quantitative calculation and analysis through digital image processing technology (Williams et al., 2013; Minervini et al., 2014; Yang et al., 2019; Basak et al., 2021).
Additionally, in order to study the correlation between phenotypic traits and mycelial quality, we also compared cellulase and laccase activities for verification. Cellulase, known as carboxymethyl cellulase (CL), is present ubiquitously in bacteria, fungi, and animals (Ghose, 1987). CL facilitates the degradation of carboxymethyl cellulose and finds extensive applications in medicine, food, cotton spinning, environmental protection, and renewable resource utilization (Khan et al., 2023). When cellulase breaks down cellulose, it is converted into glucose units and other reducing sugars. These reducing sugars can react with an anthrone reagent to form a blue–green complex, the intensity of which is proportional to the concentration of the reducing sugar. By measuring the color intensity after the reaction, the activity of cellulase can be indirectly calculated, that is, the amount of reducing sugar produced by cellulose breakdown per unit time. Therefore, we used the anthrone colorimetric method to analyze the cellulase activity on the mycelium. Laccase is a copper-containing polyphenol oxidase, belonging to the cupric blue oxidase family, widely distributed in fungi and higher plants, with strong redox ability, and has a wide range of applications in pulp biobleaching, degradation of environmental pollutants, lignocellulosic degradation, and biological detection.
Finally, the paper concluded that the phenotypic indices of cellulase and the mycelium were consistent and analyzed the internal relationship between mycelial quality and these phenotypic characteristics concerning the mycelial outline and texture.
2 Materials and methods
2.1 Strain image materials
2.1.1 Mycelium of P. geesteranus
This study selected the mycelium from P. geesteranus (Xiu 2), along with subcultures up to the 5th and 14th generations, as well as degraded strains. All these materials were sourced from the Fungi Research Center of Fujian Agriculture and Forestry University. Among them, the non-degenerated strain was labeled as X2-N, the 5th generation subculture strain was labeled as X2-5, the 14th generation subculture strain was labeled as X2-14, and the degenerated strain was labeled as X2-T.
During the experiment, the solid medium, Potato Dextrose Agar (PDA), comprised 200
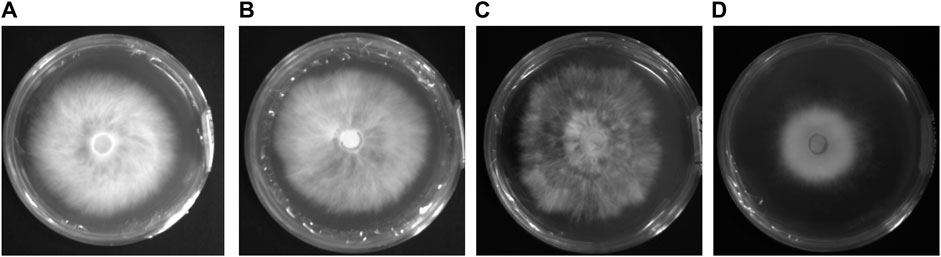
Figure 1. Image of four activated mycelium samples of Pleurotus geesteranus. (A) non-degenerated strain labeled X2-N; (B) 5th generation subculture strain labeled X2-5; (C) 14th generation subculture strain labeled X2-14; (D) degenerated strain labeled X2-T.
2.1.2 Image acquisition
This experiment captured the images of the mushroom by using an industrial camera (Basler acA 1300-30 gm; Germany), which was equipped with a Sony ICX445 CCD chip, 20 frames per second, resolution of 1.3 million pixels through Pylon Viewer 64-Bit software. The camera was positioned at a fixed distance of 25
2.1.3 Image processing software
In order to realize the analysis and quantitative calculation of mycelial images on outline and texture indices, this experiment first used MATLAB 2020b (9.9.0.1467703) 64-bit (win64) software to perform the outline and texture processing, allowing computation in the formulations of 10 indices, namely, radius, perimeter, area, growth rate, change speed, mycelium coverage, integrity, groove depth, density, and density change. Furthermore, IBM SPSS Statistics R26.0.0.0 64-bit was utilized to analyze and optimize the normality and correlation of the acquired image data. In addition, OriginPro 2022 software outputted the statistical maps of the data. The computer environment was Windows 10 with the graphics card driver being version 27.21.14.5167 of NVIDIA GeForce GT 730.
2.2 Strain image processing methods
The comprehensive technology is presented in Figure 3. We obtained 10 phenotypic indicator datasets for each strain sample by employing both outline and texture processing methods. Initially, in the outline formulation, binarization and threshold segmentation were employed to delineate the mycelial boundary, minimum circumscribed rectangle, and horizontal and vertical axes (Chen et al., 2008; Underwood et al., 2017). Following this, five indices, namely, mycelial perimeter, radius, area, growth rate, and change speed, were calculated to scrutinize the morphological and phenotypic traits of the mycelial outline (Bae et al., 2011; Le et al., 2012). Subsequently, the gray-level co-occurrence matrix was used to extract pixel count, contrast, and entropy (Xuan et al., 2022; Wu et al., 2023). An additional set of five indices, namely, mycelium coverage, roundness, groove depth, density, and density change, were calculated to analyze the phenotypic characteristics of the mycelium texture (Hu et al., 2022). Moreover, the cellulase and laccase activity indexes of the mycelium were compared, providing additional validation for the analysis results on the phenotypic characteristics of the mycelium (Samy et al., 2022). Finally, the study analyzed the internal correlation between the outline and texture concerning the phenotypic characteristics of the mycelium for P. geesteranus.
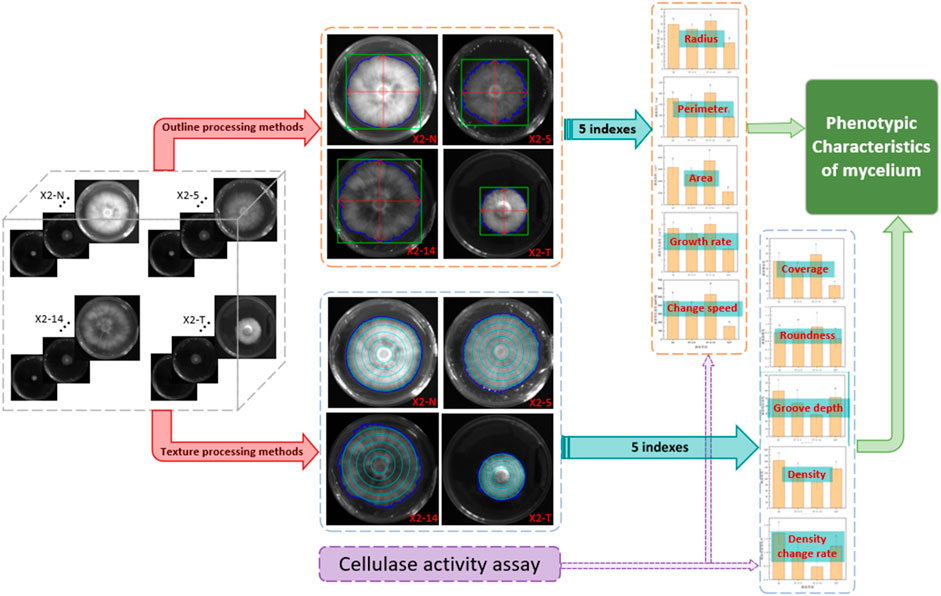
Figure 3. Comprehensive technical framework for phenotypic characteristics of the mycelium. 1) Four categories of mycelium samples, non-degraded (X2-N) and degraded (X2-T) mycelium and the 5th (X2-5) and 14th (X2-14) subcultures, were processed using two methods: contour and texture; 2) the calculations generated 10 corresponding indices of mycelial phenotypic characteristics; 3) statistical analysis of these data revealed the relationship between mycelial phenotypic characteristics and its quality; 4) the cellulase activity assessments were used to verify the accuracy of the above two methods.
2.2.1 Outline processing methods
We made observations at two time points—on day 2, following mycelium activation, and on day 8 of its growth—calculating the growth rate and change speed accordingly. The calculation steps and methods are shown in Figure 4.
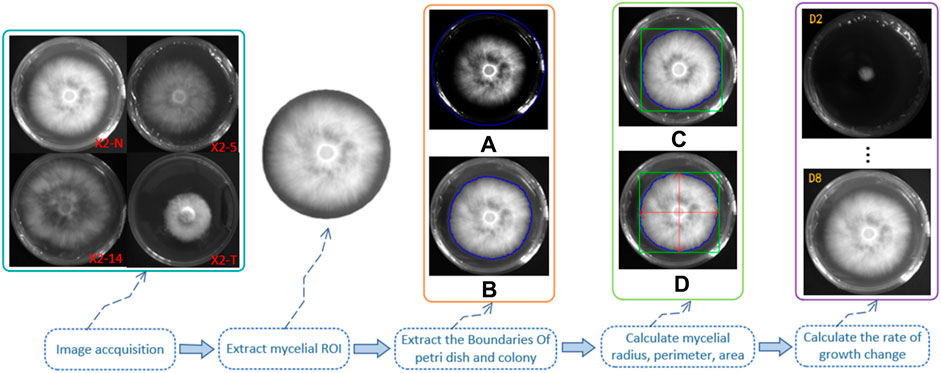
Figure 4. Calculation steps and methods of the mycelial outline. 1) Grayscale of the digital image of the mycelium was obtained using the camera, and the region of interest (ROI) was extracted; 2) outline of the boundary of the Petri dish (A) and the mycelium (B), as shown by the blue line; 3) the minimum external matrix of the mycelial boundary is denoted by the green line (C); 4) the radius, perimeter, and area were calculated through the horizontal and vertical axes by the red double-headed arrow (D); 5) days 2 and 8 of mycelium growth were set as two unit time observation points to calculate their growth rate and change speed.
The detailed procedure for analyzing the phenotypic characteristics of the mycelium concerning outline morphology is summarized as follows:
Algorithm 1.The outline processing methods.
Input: The gray value of the mycelial image
1) Grayscale processing on the mycelial image:
2) Use the number of all 2D pixels contained within the boundary:
3) Calculate the minimum circumscribed circle matrix:
4) Day 2 of strain growth was regarded as the first observation time point
Output: Use Eqs 1–5 to obtain five indexes of the mycelium outline (
The five indices of the mycelial outline, namely, the mycelial radius, perimeter, area, growth rate, and change speed, were calculated to analyze the morphological and phenotypic characteristics (Hu et al., 2022), and the obtained results are shown in Table 1; Figure 5.
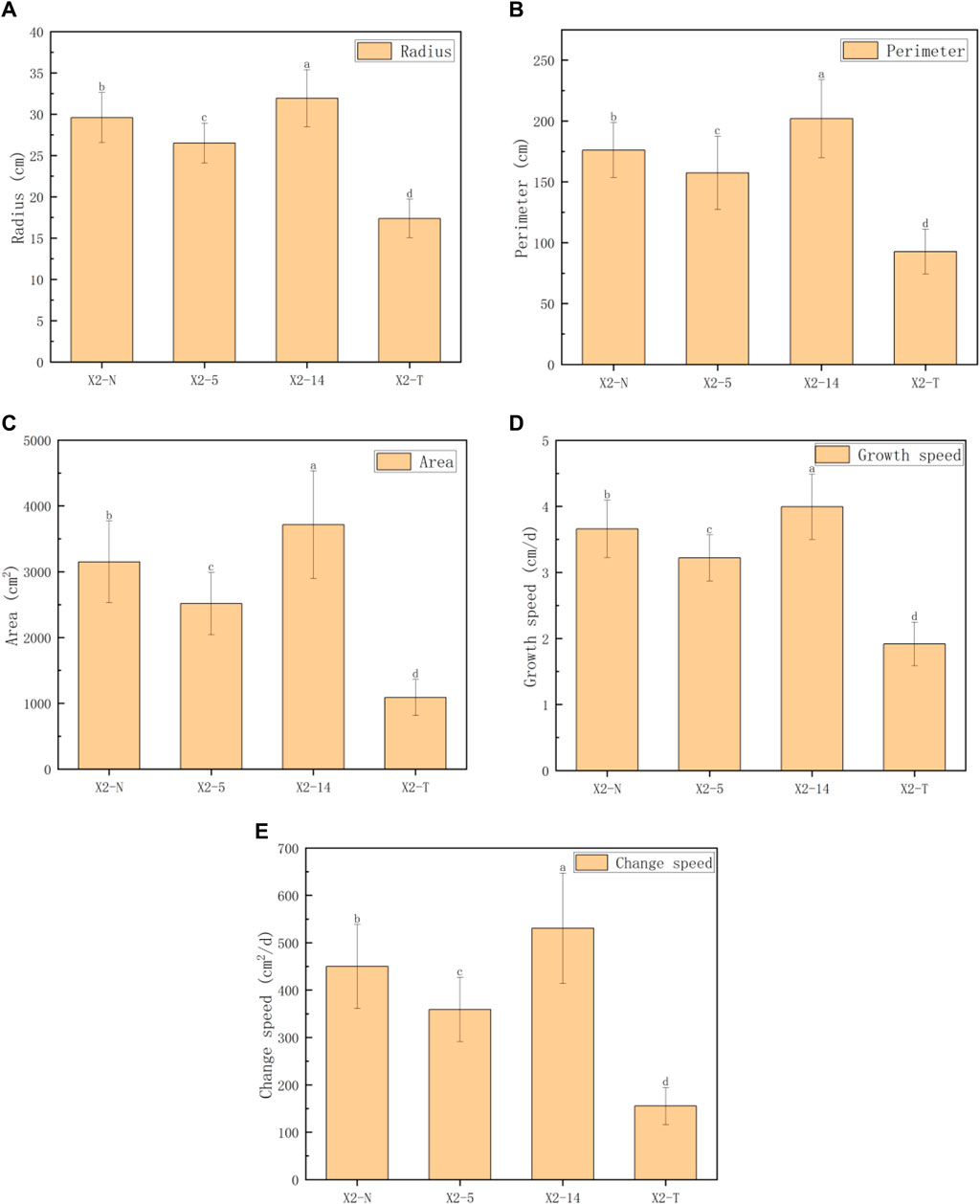
Figure 5. Comparison of five phenotypic characteristic indexes on the mycelial outline. (A) Radius (cm), (B) perimeter (cm), (C) area (cm2), (D) growth speed (cm/d), and (E) change speed (cm2/d). 1) The horizontal axis represented the four groups of mycelia, and the vertical axis represented each phenotypic data index; 2) all characteristic data were recorded on day 8 after subcultures and subject to the mean
2.2.1.1 Radius
where
2.2.1.2 Perimeter
where
2.2.1.3 Area
where
2.2.1.4 Growth rate
where
2.2.1.5 Change speed
where
2.2.2 Texture processing methods
The steps of processing the phenotypic characteristics of the mycelium concerning texture morphology are summarized as follows:
Algorithm 2.The texture processing methods.
Input: The gray value of the original image of the mycelium is 0–255
1)
2)
3)
4)
5)
Output: Five indices of mycelial texture morphology,
The five indices of the mycelial texture, namely, coverage, roundness, groove depth, density, and density change, were computed to analyze the morphological and phenotypic characteristics (Hu et al., 2022), and the obtained results are shown in Table 2; Figure 6.
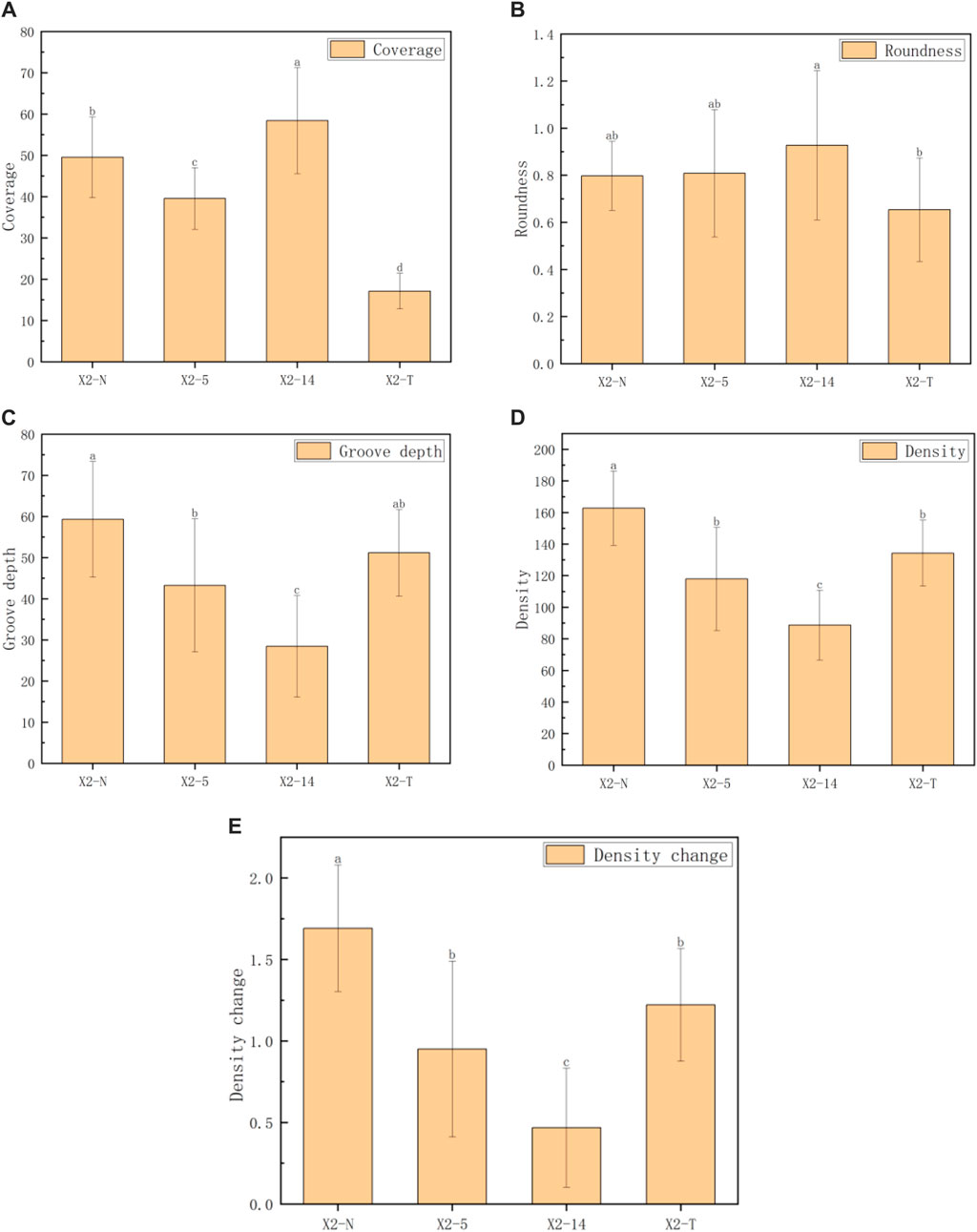
Figure 6. Comparison of five indexes of the mycelial texture. (A) Coverage, (B) roundness, (C) groove depth, (D) density, and (E) density change. 1) The horizontal axis represented the four groups of mycelia, and the vertical axis represented each phenotypic data index; 2) all characteristic data were recorded on day 8 after subcultures and subject to mean
2.2.2.1 Coverage
where
2.2.2.2 Roundness
where
2.2.2.3 Groove depth
where
2.2.2.4 Density
where
2.2.2.5 Density change
where
2.3 Methods for extracting enzyme activity from the mycelium
2.3.1 Extraction method for cellulase activity
The activated mycelium was placed in a centrifuge tube using a hole puncher with a diameter of 5 mm and was then stored in a refrigerator at 4
We adapted the anthrone colorimetric method to quantify the reducing sugar content generated through the degradation of sodium carboxymethyl cellulose, which was catalyzed by CL (Wood and Bhat, 1988). The procedure involved shaking the sample at 37
The regression equation for determining standard conditions was given by
where
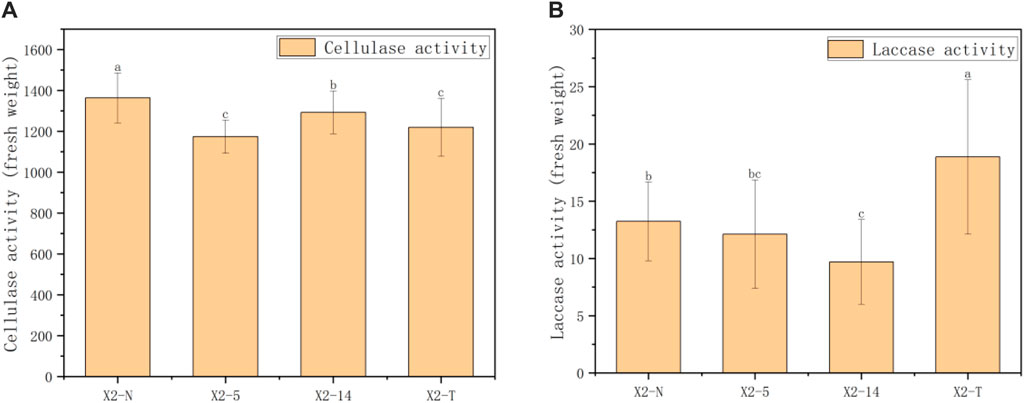
Figure 7. Results of enzyme activity in four groups of mycelia. (A) Results of cellulase activity in four groups of mycelia and (B) results of laccase activity in four groups of mycelia. 1) The horizontal axis represented the four groups of mycelia, and the vertical axis represented the enzyme activity in units of fresh weight; 2) all characteristic data were recorded on day 8 after subcultures and subject to the mean
2.3.2 Extraction method for laccase activity
The activated mycelium was placed in a centrifuge tube using a hole puncher with a diameter of 5
Laccase decomposes the substrate ABTS to produce ABTS free radicals, which have a significantly higher absorbance coefficient at 420
where
3 Results
3.1 Results of the mycelial outline
For convenience, four groups of mycelium, namely, the non-degraded and degraded mycelium and the 5th and 14th subcultures, were denoted as X2-N, X2-T, X2-5, and X2-14, respectively. We took 100 samples selected from each group. A total of 400 samples were photographed and recorded on day 8 after inoculation. We precisely computed the outline morphology of the mycelium by using Algorithm 1, resulting in a total of five phenotypic characteristic indices: radius, perimeter, area, growth speed, and change speed. We adopted the one-way ANOVA method, and the mean and standard deviation (SD) of the five indices for the four groups of mycelium are shown in Table 1. The results showed that there were significant statistical differences in the five indices among the four groups (all
Figure 5 shows the performance trends of the five indices on the phenotypic characteristics of the mycelial outline (radius, perimeter, area, growth rate, and change speed), which were basically consistent. The growth rate of the mycelium weakened from the activation stage to X2-5, but it accelerated from X2-5 to X2-14. However, for X2-T, there was a significant downward trend in all aspects.
3.2 Results of the mycelial texture
For the 400 existing samples, we accurately calculated the texture morphology of the mycelium by using Algorithm 2, resulting in five indices of phenotypic characteristics: coverage, roundness, groove depth, density, and density change. We took the one-way ANOVA method, and the mean and SD of the five indices of the four groups of mycelium are given in Table 2. The results showed that there were statistical differences in the five indices among the four groups (all
The coverage indices given in Figure 6 were generally consistent. The roundness of the four groups of mycelia showed little difference. However, as the subculture times increase, the groove depth, mycelial density, and their rate of changes significantly decreased, even falling below X2-T. This phenomenon suggested that during the process, a faster growth rate in the subculture leads to sparser mycelium texture, darker coloration, and shallower grooves. On the contrary, X2-T exhibited the lowest coverage due to its extremely slow growth rate but maintained relatively completed roundness, and the mycelial texture was still white and dense with obvious grooves.
3.3 Results of enzyme activity
In this study, 30 samples were selected from X2-N, X2-5, X2-14, and X2-T. The anthrone colorimetric method was used to calculate the index of cellulase activity in the unit of mycelium mass using Formula 11. The comprehensive statistical results of these indexes through one-way ANOVA showed that there were statistical differences in cellulase activity among the four groups (
Comparing Figures 5–7 shows that the cellulase activity trend of the mycelium was basically consistent with the analysis results of five indices related to its mycelium outline, as well as the analysis results of the two indices of mycelium coverage and roundness in its texture morphology. However, the laccase activity of the strain was different from the 10 indices in the outline and texture of the mycelium, and contrarily, the laccase activity of the degraded mycelium was particularly significant. Therefore, from the physiological perspective of the mycelium, the cellulase activity of this mycelium can be consistent with the phenotypic characteristic indices of the mycelium. Cellulase activity can be well used to verify the accuracy of digital image processing technology in the analysis of phenotypic characteristics.
4 Discussion
As a species of edible fungi with high yield and high economic value, the analysis of the phenotypic characteristics of P. geesteranus currently involves a lot of manual repeated operations, mainly including the average growth rate, color, texture, density, and edge uniformity, measured and calculated by a manual ruler or experience (Cardini et al., 2020). By applying digital image technology to the analysis of the mycelium, its phenotypic data can not only improve the accuracy of phenotypic characteristics obtained by manual measurement but also convert qualitative phenotypic characteristics into objective ones that can be qualitatively and quantitatively analyzed (Hardy et al., 2017). It has been shown that the use of digital image processing technology is regarded as an automated mean to extract and analyze the phenotypic characteristics of the mycelium, which has obvious advantages (Phoulady et al., 2016).
In order to better analyze the phenotypic characteristics of P. geesteranus, digital image processing technology has made certain progress in the following aspects (Alkhudaydi et al., 2019):
1) Image acquisition and preprocessing: High-quality image acquisition equipment and preprocessing techniques are used to obtain the enhanced images of the growth process of P. geesteranus.
2) Feature extraction: Relevant features related to the phenotype of P. geesteranus are extracted from the preprocessed images. These features can include shape, color, and texture (Yang et al., 2024).
3) Algorithm design and analysis: Utilizing algorithms and mathematical formulas to digitally calculate the phenotypic characteristics of P. geesteranus (Zhai et al., 2019).
4) Application and expansion: Combining technologies from other fields with digital image processing to achieve more efficient and accurate phenotypic analysis of P. geesteranus (Wei et al., 2021).
In our study, the statistical analysis results of the above phenotypic characteristics showed that during the subculture process of the mycelium, the growth rate of the mycelium showed a trend of slowing down at first and then accelerating, and the texture quality of the mycelium decreased with the acceleration of their growth rate. At the same time, the growth ability of the degraded mycelium was definitely the weakest. Therefore, in the early breeding stage of the mycelium, we could efficiently eliminate those degraded mycelia with slow production speed from the outline indices, and we also eliminated those mycelia with uneven texture and sparse density from the texture indices. Thus, among these two types of phenotypic characteristics, the mycelium with better growth, higher quality, and stronger vitality could be quickly identified for cultivating P. geesteranus.
In summary, there is still considerable room for development in the digital image processing research of P. geesteranus phenotypic analysis. In this paper, four groups of mycelia, X2-N, X2-5, X2-14, and X2-T, were formulated by using digital image processing technology from its outline and texture morphology. However, different edible fungus species had individual differences, and their phenotypic characteristics were also different in the macroscopic view. Therefore, in the field of research on the phenotypic characteristics of edible fungi, it is necessary to combine the characteristics of different mycelia to address proper algorithms and analysis schemes of phenotypic characteristics (Zhang et al., 2020).
5 Conclusion
This paper studied the phenotypic characteristics of the mycelium of P. geesteranus by using image recognition technology. We found that the mycelium was analyzed from the outline and texture shapes. In the outline shape, five indexes, namely, mycelium radius, perimeter, area, growth rate, and change speed, were calculated to obtain the results of quantitative analysis. In texture shape, five indices, namely, mycelial coverage, roundness, groove depth, density, and density change, were calculated to obtain the results of qualitative analysis. All indices of the degraded mycelium were significantly lower than those of the non-degraded mycelium, and the obtained analysis results showed large statistical differences (
Data availability statement
The original contributions presented in the study are included in the article/Supplementary Material; further inquiries can be directed to the corresponding author.
Author contributions
XiW: data curation, formal analysis, methodology, and writing–original draft. YX: software and writing–review and editing. XuW: funding acquisition and writing–review and editing.
Funding
The author(s) declare financial support was received for the research, authorship, and/or publication of this article. This research was funded by the Outstanding Young Research Talents Program of Fujian Agriculture and Forestry University (xjq202116); the Fujian Province Science and Technology Plan Project (2021J01131024).
Acknowledgments
The authors thank Prof. Zhixiong Zhong (College of Computer and Control Engineering, Minjiang University) for his comments on the early drafts of this paper. The authors thank editors and reviewers for their constructive comments.
Conflict of interest
The authors declare that the research was conducted in the absence of any commercial or financial relationships that could be construed as a potential conflict of interest.
Publisher’s note
All claims expressed in this article are solely those of the authors and do not necessarily represent those of their affiliated organizations, or those of the publisher, the editors, and the reviewers. Any product that may be evaluated in this article, or claim that may be made by its manufacturer, is not guaranteed or endorsed by the publisher.
References
Alkhudaydi, T., Reynolds, D., Griffiths, S., Zhou, J., and de la Iglesia, B. (2019). An exploration of deep-learning based phenotypic analysis to detect spike regions in field conditions for UK bread wheat. Plant Phenomics 2019, 7368761. doi:10.34133/2019/7368761
Bae, E., Bai, N., Aroonnual, A., Bhunia, A. K., and Hirleman, E. D. (2011). Label-free identification of bacterial microcolonies via elastic scattering. Biotechnol. Bioeng. 108 (3), 637–644. doi:10.1002/bit.22980
Basak, A. K., Mirzaei, M., Strzałka, K., and Yamada, K. (2021). Texture feature extraction from microscope images enables a robust estimation of ER body phenotype in Arabidopsis. Plant Methods 17, 109–116. doi:10.1186/s13007-021-00810-w
Cardini, A., Pellegrino, E., Del Dottore, E., Gamper, H. A., Mazzolai, B., and Ercoli, L. (2020). HyLength: a semi-automated digital image analysis tool for measuring the length of roots and fungal hyphae of dense mycelia. Mycorrhiza 30 (2-3), 229–242. doi:10.1007/s00572-020-00956-w
Chen, D., Neumann, K., Friedel, S., Kilian, B., Chen, M., Altmann, T., et al. (2014). Dissecting the phenotypic components of crop plant growth and drought responses based on high-throughput image analysis. plant Cell. 26 (12), 4636–4655. doi:10.1105/tpc.114.129601
Chen, Q., Sun, Q., Heng, P. A., and Xia, D. S. (2008). A double-threshold image binarization method based on edge detector. Pattern Recognit. 41 (4), 1254–1267. doi:10.1016/j.patcog.2007.09.007
Danner, C., Mach, R. L., and Mach-Aigner, A. R. (2023). The phenomenon of strain degeneration in biotechnologically relevant fungi. Appl. Microbiol. Biotechnol. 107, 4745–4758. doi:10.1007/s00253-023-12615-z
Furbank, R. T., and Tester, M. (2011). Phenomics–technologies to relieve the phenotyping bottleneck. Trends plant Sci. 16 (12), 635–644. doi:10.1016/j.tplants.2011.09.005
Ghose, T. K. (1987). Measurement of cellulase activities. Pure Appl. Chem. 59 (2), 257–268. doi:10.1351/pac198759020257
Guo, Z., Yang, W., Chang, Y., Ma, X., Tu, H., Xiong, F., et al. (2018). Genome-wide association studies of image traits reveal genetic architecture of drought resistance in rice. Mol. Plant 11 (6), 789–805. doi:10.1016/j.molp.2018.03.018
Hardy, N., Moreaud, M., Guillaume, D., Augier, F., Nienow, A., Béal, C., et al. (2017). Advanced digital image analysis method dedicated to the characterization of the morphology of filamentous fungus. J. Microsc. 266 (2), 126–140. doi:10.1111/jmi.12523
Helgason, T., and Fitter, A. H. (2009). Natural selection and the evolutionary ecology of the arbuscular mycorrhizal fungi (Phylum Glomeromycota). J. Exp. Bot. 60 (9), 2465–2480. doi:10.1093/jxb/erp144
Hu, D., Yuan, T., Yu, H. L., Ma, C., Tian, M., and Li, L. (2022). Digital image processing assisted phenotypic analysis of Pleurotus giganteus colony. Acta Edulis Fungi 29 (3), 32–40. doi:10.16488/j.cnki.1005-9873.2022.03.005
James, J. E., Santhanam, J., Zakaria, L., Mamat Rusli, N., Abu Bakar, M., Suetrong, S., et al. (2022). Morphology, phenotype, and molecular identification of clinical and environmental Fusarium solani species complex isolates from Malaysia. J. Fungi 8 (8), 845. doi:10.3390/jof8080845
Khan, G. M. A., Hasan, M. S., Rahaman, M. H., Aydid, A., Rahman, M. M., Hasanuzzaman, M., et al. (2023) Cellulose and its composites in textiles and food industry. Regenerated Cellulose and composites: morphology-property relationship. Singapore: Springer Nature Singapore, 223–264.
Le, C. N., Mendes, R., Kruijt, M., and Raaijmakers, J. M. (2012). Genetic and phenotypic diversity of Sclerotium rolfsii in groundnut fields in central Vietnam. Plant Dis. 96 (3), 389–397. doi:10.1094/pdis-06-11-0468
Lehmann, A., Zheng, W., Soutschek, K., Roy, J., Yurkov, A. M., and Rillig, M. C. (2019). Tradeoffs in hyphal traits determine mycelium architecture in saprobic fungi. Sci. Rep. 9 (1), 14152. doi:10.1038/s41598-019-50565-7
Li, B., Chen, L., Sun, W., Wu, D., Wang, M., Yu, Y., et al. (2020a). Phenomics-based GWAS analysis reveals the genetic architecture for drought resistance in cotton. Plant Biotechnol. J. 18 (12), 2533–2544. doi:10.1111/pbi.13431
Li, H., Feng, H., Guo, C., Yang, S., Huang, W., Xiong, X., et al. (2020b). High-throughput phenotyping accelerates the dissection of the dynamic genetic architecture of plant growth and yield improvement in rapeseed. Plant Biotechnol. J. 18 (11), 2345–2353. doi:10.1111/pbi.13396
Liu, F., Wang, S. H., Jia, D. H., Tan, H., Wang, B., and Zhao, R. L. (2023). Development of multiple nucleotide polymorphism molecular markers for enoki mushroom (flammulina filiformis) cultivars identification. J. Fungi 9 (3), 330. doi:10.3390/jof9030330
Mahajan, S., Das, A., and Sardana, H. K. (2015). Image acquisition techniques for assessment of legume quality. Trends Food Sci. Technol. 42 (2), 116–133. doi:10.1016/j.tifs.2015.01.001
Marei, G. I. K., Rasoul, M. A. A., and Abdelgaleil, S. A. M. (2012). Comparative antifungal activities and biochemical effects of monoterpenes on plant pathogenic fungi. Pesticide Biochem. physiology 103 (1), 56–61. doi:10.1016/j.pestbp.2012.03.004
Minervini, M., Abdelsamea, M. M., and Tsaftaris, S. A. (2014). Image-based plant phenotyping with incremental learning and active contours. Ecol. Inf. 23, 35–48. doi:10.1016/j.ecoinf.2013.07.004
Omari, M. K., Lee, J., Faqeerzada, M. A., Joshi, R., Park, E., and Cho, B. K. (2020). Digital image-based plant phenotyping: a review. Korean J. Agric. Sci. 47 (1), 119–130.
Pérez, G., Lopez-Moya, F., Chuina, E., Ibañez-Vea, M., Garde, E., López-Llorca, L. V., et al. (2021). Strain degeneration in Pleurotus ostreatus: a genotype dependent oxidative stress process which triggers oxidative stress, cellular detoxifying and cell wall reshaping genes. J. Fungi 7 (10), 862. doi:10.3390/jof7100862
Phoulady, H. A., Goldgof, D. B., Hall, L. O., and Mouton, P. R. (2016). Nucleus segmentation in histology images with hierarchical multilevel thresholding. Med. Imaging 2016 Digit. Pathol. SPIE 9791, 280–285. doi:10.1117/12.2216632
Samy, M. M., Almamlook, R. E., Elkhouly, H. I., and Barakat, S. (2022). Decision-making and optimal design of green energy system based on statistical methods and artificial neural network approaches. Sustain. Cities Soc. 84, 104015. doi:10.1016/j.scs.2022.104015
Shakoor, N., Lee, S., and Mockler, T. C. (2017). High throughput phenotyping to accelerate crop breeding and monitoring of diseases in the field. Curr. Opin. plant Biol. 38, 184–192. doi:10.1016/j.pbi.2017.05.006
Singh, M., Kamal, S., and Sharma, V. P. (2021). Species and region-wise mushroom production in leading mushroom producing countries-China, Japan, USA, Canada and India. Mushroom Res. 30 (2), 99–108. doi:10.36036/mr.30.2.2021.119394
Underwood, J., Wendel, A., Schofield, B., McMurray, L., and Kimber, R. (2017). Efficient in-field plant phenomics for row-crops with an autonomous ground vehicle. J. Field Robotics 34 (6), 1061–1083. doi:10.1002/rob.21728
Wei, Y., He, Y., and Li, X. (2021). Tea moisture content detection with multispectral and depth images. Comput. Electron. Agric. 183, 106082. doi:10.1016/j.compag.2021.106082
Wei, X., Liu, S., Xie, C., Fang, W., Deng, C., Wen, Z., et al. (2023). Nondestructive detection of pleurotus geesteranus strain degradation based on micro-hyperspectral imaging and machine learning. Front. Plant Sci. 14. doi:10.3389/fpls.2023.1260625
Williams, K., Munkvold, J., and Sorrells, M. (2013). Comparison of digital image analysis using elliptic Fourier descriptors and major dimensions to phenotype seed shape in hexaploid wheat (Triticum aestivum L.). Euphytica 190, 99–116. doi:10.1007/s10681-012-0783-0
Wood, T. M., and Bhat, K. M. (1988). Methods for measuring cellulase activities. Methods Enzym. 160, 87–112. doi:10.1016/0076-6879(88)60109-1
Wu, G., Fang, Y., Jiang, Q., Cui, M., Li, N., Ou, Y., et al. (2023). Early identification of strawberry leaves disease utilizing hyperspectral imaging combing with spectral features, multiple vegetation indices and textural features. Comput. Electron. Agric. 204, 107553. doi:10.1016/j.compag.2022.107553
Xuan, G., Li, Q., Shao, Y., and Shi, Y. (2022). Early diagnosis and pathogenesis monitoring of wheat powdery mildew caused by blumeria graminis using hyperspectral imaging. Comput. Electron. Agric. 197, 106921. doi:10.1016/j.compag.2022.106921
Yang, C., Ma, X., Guan, H., and Fan, B. (2022). Rapid detection method of Pleurotus eryngii mycelium based on near infrared spectral characteristics. Spectrochimica Acta Part A Mol. Biomol. Spectrosc. 271, 120919. doi:10.1016/j.saa.2022.120919
Yang, N., Qian, Y., el-Mesery, H. S., Zhang, R., Wang, A., and Tang, J. (2019). Rapid detection of rice disease using microscopy image identification based on the synergistic judgment of texture and shape features and decision tree–confusion matrix method. J. Sci. Food Agric. 99 (14), 6589–6600. doi:10.1002/jsfa.9943
Yang, T., Zhu, S., Zhang, W., Zhao, Y., Song, X., Yang, G., et al. (2024). Unmanned aerial vehicle-scale weed segmentation method based on image analysis technology for enhanced accuracy of maize seedling counting. Agriculture 14 (2), 175. doi:10.3390/agriculture14020175
Yin, H., Yi, W., and Hu, D. (2022). Computer vision and machine learning applied in the mushroom industry: a critical review. Comput. Electron. Agric. 198, 107015. doi:10.1016/j.compag.2022.107015
Yoo, S., Lee, H. Y., Markkandan, K., Moon, S., Ahn, Y. J., Ji, S., et al. (2019). Comparative transcriptome analysis identified candidate genes involved in mycelium browning in Lentinula edodes. BMC genomics 20, 1–13. doi:10.1186/s12864-019-5509-4
Zhai, Z., Jin, Z., and Zhang, R. (2019). Information integration of force sensing and machine vision for in-shell shrivelled walnut detection based on the golden-section search optimal discrimination threshold. J. Sci. Food Agric. 99 (8), 3941–3949. doi:10.1002/jsfa.9618
Zhang, H., Zhan, B., Pan, F., and Luo, W. (2020). Determination of soluble solids content in oranges using visible and near infrared full transmittance hyperspectral imaging with comparative analysis of models. Postharvest Biol. Technol. 163, 111148. doi:10.1016/j.postharvbio.2020.111148
Zhang, J., Li, X., Ying, Y., and Yao, X. (2019). Effects of the camellia oleifera shell substrate on the yield and nutritional composition of Pleurotus geesteranus. Agric. Sci. 10 (10), 1298–1311. doi:10.4236/as.2019.1010096
Keywords: image recognition, mycelial quality, outline, phenotypic characteristics, Pleurotus geesteranus, texture feature
Citation: Wang X, Xu Y and Wei X (2024) Phenotypic characteristics of the mycelium of Pleurotus geesteranus using image recognition technology. Front. Bioeng. Biotechnol. 12:1338276. doi: 10.3389/fbioe.2024.1338276
Received: 14 November 2023; Accepted: 14 May 2024;
Published: 17 June 2024.
Edited by:
Zhixiong Zhong, Minjiang University, ChinaReviewed by:
Maura Téllez Téllez, Autonomous University of the State of Morelos, MexicoMiha Humar, University of Ljubljana, Slovenia
Baby Summuna, Sher-e-Kashmir University of Agricultural Sciences and Technology, India
Copyright © 2024 Wang, Xu and Wei. This is an open-access article distributed under the terms of the Creative Commons Attribution License (CC BY). The use, distribution or reproduction in other forums is permitted, provided the original author(s) and the copyright owner(s) are credited and that the original publication in this journal is cited, in accordance with accepted academic practice. No use, distribution or reproduction is permitted which does not comply with these terms.
*Correspondence: Xuan Wei, eHVhbndlaUBmYWZ1LmVkdS5jbg==