- 1Central Diagnostics Laboratory, University Medical Center Utrecht, University Utrecht, Utrecht, Netherlands
- 2Laboratory of Experimental Cardiology, Department of Cardiology, University Medical Center Utrecht, University Utrecht, Utrecht, Netherlands
Atherosclerosis still is the primary cause of death worldwide. Our characterization of the atherosclerotic lesion is mainly rooted in definitions based on pathological descriptions. We often speak in absolutes regarding plaque phenotypes: vulnerable vs. stable plaques or plaque rupture vs. plaque erosion. By focusing on these concepts, we may have oversimplified the atherosclerotic disease and its mechanisms. The widely used definitions of pathology-based plaque phenotypes can be fine-tuned with observations made with various -omics techniques. Recent advancements in single-cell transcriptomics provide the opportunity to characterize the cellular composition of the atherosclerotic plaque. This additional layer of information facilitates the in-depth characterization of the atherosclerotic plaque. In this review, we discuss the impact that single-cell transcriptomics may exert on our current understanding of atherosclerosis.
Introduction
The classical concept of a “vulnerable plaque” originates from the 1980s and depicts plaque rupture in patients who died from coronary syndromes as the major pathological cause of acute myocardial infarction (1). The concept describes a lipid-rich, atheromatous plaque with a thin fibrous cap and local infiltration of inflammatory cells that cause proteolytic activity and degradation of the stabilizing extracellular matrix (ECM). Genome-wide association studies (GWAS) and lesion-based transcriptomic studies point to a diverse landscape of mechanisms leading to atherosclerotic lesion initiation and progression. Vascular obstructive lesions are characterized by significant variability in pathology-based characteristics. Thrombotic occlusions may arise from traditionally described ruptured vulnerable plaques, but also unruptured eroded plaques and microcalcifications can be complicated by destabilisation (2).
Bulk micro-array and sequencing of atherosclerotic plaques obtained from human patients (3–6) or animals (7) highlight networks and pathways that could accelerate lesion progression. The heterogeneous nature of the tissue complicates the interpretation and deconvolution of the transcriptomic signal. Therefore, it is difficult to pinpoint which cells are responsible for this signal, and the effect of rare but crucial cells is lost in the noise. Fine-tuning the representation of the cellular composition and concomitant gene expression profiles is facilitated by single-cell RNA sequencing (scRNA-seq). The research field is experiencing a rapid increase in scRNA-seq efforts in the domain of atherosclerosis (7–15). Numerous scRNA-seq datasets are already available for the broad scientific community for reanalysis or via online tools like PlaqView (16). For both mice and humans, the present cell (sub-)populations have been meticulously described. These studies of atherosclerotic tissue may hold promise in the translation of mouse-to-human findings and provide clues to phenotypic changes in human cell populations that have so far only been described in animal models and cell cultures. In this review, we provide an overview of the findings made with scRNA-seq in atherosclerotic tissue. In addition, we discuss the possible answers that scRNA-seq will potentially provide to long-standing questions that have remained unanswered to date (Figure 1).
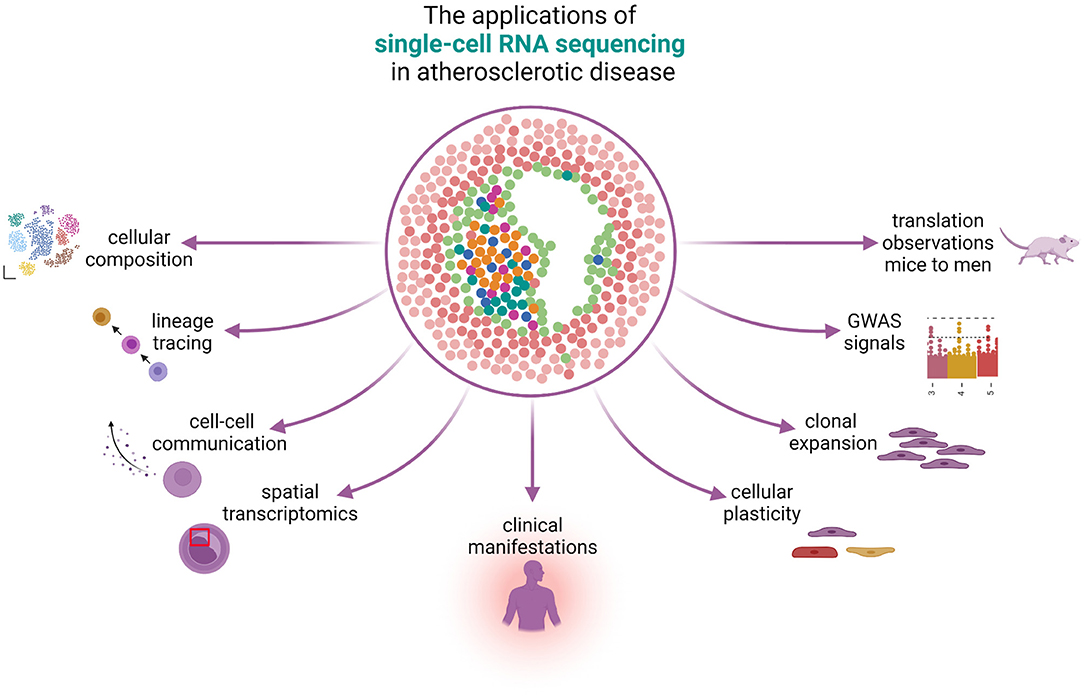
Figure 1. The applications of single-cell RNA sequencing in atherosclerotic disease. scRNA-seq can be used to study many different aspects of atherosclerotic disease. The knowledge on cellular composition can give insights on lineage tracing and can be used to study cellular plasticity, clonal expansion, and reveal cell-gene pairs from GWAS signals. Spatial transcriptomics reveals the physical locations of different cell populations in the plaque and can additionally be used to study cell-cell communication. scRNA-seq can reveal differences in cells under the influence of different clinical presentations and bridge the gap between mice and men.
Going Solo
Exploring the cellular diversity of plaques is hindered by tissue heterogeneity. The broad spectrum of cells present in plaque tissue has been unraveled via histology, highlighting the classical image of the atherosclerotic plaque with the thin cap comprised of smooth muscle cells (SMCs) and infiltrating immune cells (1). Progress in various –omics techniques has elucidated protein content (17, 18) and transcriptomic content of plaque cells via DNA microarrays (3, 4, 6) and bulk RNA-seq (5, 19), providing more insight into the various processes. However, whole tissue (bulk) transcriptomics provides the sum of the transcriptome for all the cells in the tissue and provides no direct information about cell composition. Consequently, the most prevalent cell types dominate the outcomes and subsequent interpretations of bulk transcriptomics efforts, hiding the biology, and heterogeneity of individual cells in their numbers. Each cell type has its unique contribution to plaque manifestation, which is affected by both intrinsic and environmental factors -such as risk factors and medication. Therefore, it is not surprising that the current trend is leaning toward methods that allow for more optimal resolution at the cellular level, evident from the rise in papers using single-cell methods. Flow cytometry and mass cytometry can provide single-cell resolution. However, they are biased as they are dependent on prior knowledge about the phenotype and markers of the cells present in the sample. Predetermined antibodies have to be added to the cell suspension, which can subsequently be analyzed. These methods require in-depth knowledge of the available cell types and sparsely allow for discovering unexpected new or rare cell populations.
In contrast to flow cytometry, scRNA-seq does not require any prior knowledge of cell composition. Rapid developments in the–omics field have benefitted the progression from bulk RNA-seq to single-cell (sc)RNA-seq. The first scRNA-seq effort in 2009 (20) has opened the gates to a rapid increase in applying this technique to various fields, with no exception to atherosclerotic research (8–11, 21, 22). The main advantages are that it can be applied unbiasedly, highlighting new and known cell populations from heterogenic tissue (23). At the same time, they simultaneously uncover the (often subtle) transcriptomic differences created by their unique environment. The rising popularity of scRNA-seq has resulted in efforts to describe the transcriptome of heterogenic tissues and drove the development of bioinformatics tools to analyse the data that follows—ranging from tools to pre-process, analyse and visualize data (24–26), to in-depth specialized follow-up experiments such as identifying rare cell types (27), cell trajectory analysis [evaluated by Saelens et al. (28)], RNA velocity (29) and cell-cell communication [reviewed by Armingol et al. (30)], amongst others. In the following text, we will focus on the discoveries made through scRNA-seq in atherosclerotic research and their implications for plaque characterization. We will discuss the current knowledge on cellular composition of lesions, lineage tracing experiments in mice, cell-cell communication, the importance of sex-stratified research, and examine the technical considerations of scRNA-seq research. For the future perspectives we examine spatial scRNA-seq, the impact of scRNA-seq on pathological specification of atherosclerosis, cell plasticity, clonal expansion, integration of genetic and transcriptomic research, and finally the translation from mice to men.
Current Knowledge About Cellular Composition: Insights From scRNA-seq
Cellular Composition in Human and Mouse Plaques
The most significant impact that scRNA-seq has on our understanding of the atherosclerotic plaque is the meticulous dissection of cell populations that are present (8–14)—including multiple types of SMCs, endothelial cells (ECs), and immune cell sub-sets. The cell composition and cell state affect the plaque stability and may lead to different clinical manifestations. The bulk RNA-seq analysis of 654 human lesions uncovered five main plaque types that correlate with symptoms at admission (19). Deconvolution of this bulk data revealed that these plaque types have different underlying cell compositions. Indicating that underlying clinical symptoms can potentially be detected in individual cell populations using scRNA-seq. Here we will briefly discuss findings on the major cell populations of lesions reported in mice and humans. More comprehensive descriptions of the cellular landscape of atherosclerotic lesions are discussed elsewhere (31–33).
The vascular SMCs are located in the vessel wall. They provide structural support and regulate blood flow and blood pressure. Although vascular SMCs are omnipresent in the vasculature, in atherosclerosis, they exhibit distinct phenotypes. In human plaques, we (10) found two major subclasses of SMCs, one with contractile and the other showcasing synthetic characteristics. The synthetic phenotype showed low expression of typical SMC markers and upregulation of ECM genes, suggesting that these cells are cap-derived. In a recent review, authors challenge the black and white division between contractile and synthetic SMCs in the vasculature (34). Pan et al. (11) report SMCs (corresponding to a contractile phenotype), fibrochondrocytes, and an intermediate cell population named the intermediate cell state. They also report the presence of fibroblasts in carotid arteries. In aortic arteries and mice, Wirka et al. (8) report fibroblasts and phenotypically modulated SMCs. The value of scRNA-seq in unraveling the fate and origin of transdifferentiating SMCs is underlined by the association of TCF21 expression with the phenotypic switch of SMC into fibrocytes. With the subsequent strengthening of the fibrous cap and stabilization of the lesion (8).
A thin layer of ECs coats the inner layer of the artery, forming a barrier between the lumen and the artery. Endothelial dysfunction aggravates vascular diseases such as atherosclerosis (35). The role of these cells in disease progression ranges from athero-protective to inflammatory. Our group (10) and others (11) report two main distinct EC populations. Hidden within are also cells undergoing active angiogenesis or those that display the signs of epithelial to mesenchymal transition (10).
The infiltration of inflammatory cells into the intima is a hallmark of disease progression. Macrophages (Mϕs) can be observed during all phases of atherosclerotic lesion formation. Monocytes are rapidly recruited into the lesion and can differentiate into Mϕs or dendritic cells. In a recent meta- analysis (31), authors discriminate between five sub-populations of Mϕs in murine atherosclerosis: resident-like macrophages, foamy Trem2 macrophages, inflammatory macrophages, IFNIC macrophages, and cavity macrophages. Of note, not all Mϕs found in lesions are of monocyte origin (36, 37). In human lesions, Fernandez et al. (9) reported classically activated M1 macrophages. Further transcriptional analysis identified four different Mϕ populations with distinct functions. They reported activated Mϕs, and a population expressing inflammatory genes, and an MMP inhibitor, which could impair ECM degradation and subsequently a more stable plaque. The third population was pro-inflammatory, and the final population showed the transcriptional signature of foam cells with anti-inflammatory signaling. We (10), found three Mϕ populations. Two of which were pro-inflammatory and expressed IL-1β or TNF. The third population was foam cell-like and exhibited a fibrosis promoting phenotype. This population also showed alpha-actin expression, which potentially indicates a shift to SMC or from SMC to Mϕs. Cochain et al. (13) reported three Mϕ populations in mice. The first population represented a resident-like Mϕ, which is also present in healthy aortic tissue. The other two populations were atherosclerosis specific. The presence of these Mϕ populations was confirmed in human tissue (10).
T cells exhibit significant heterogeneity within the plaque. Fernandez et al. (9) reported that T cells in the plaque demonstrate cytotoxicity, activation, and exhaustion, whereas T cells in the blood circulation show cytokine inhibition, active RNA synthesis, and metabolic reprogramming. They also reported that T cells from symptomatic patients differ from symptomatic patients. However, the number of patients used is small (n = 3). Similarly, we (10) found a diverse landscape of CD4+ and CD8+ T cells populations, the main difference being their activation state. The subclass phenotypes varied from cytotoxic to more quiescent. scRNA-seq of plaques has established the existence of unforeseen T cell clusters with mixed Th1 and Treg cell transcriptional programs such as ApoB reactive T cells (38). In mice, single-cell RNA-sequencing of plaque immune cells revealed differential expression patterns of Tregs in progressing vs. regressing plaques (39).
The spectrum of cell populations uncovered with scRNA-seq is complex and, without a doubt, still incomplete. The community will significantly benefit from a unified nomenclature of cell populations since transcriptome-based names of cell populations vary across multiple studies (40). The aggregation of this knowledge would simplify further research into understanding the molecular mechanisms in atherosclerosis.
Lineage Tracing
The cells in human atherosclerotic plaques have long been considered fully differentiated and non-plastic. However, in vitro and mice experiments have revealed the potential of vascular cell types to undergo phenotypic switching (a process also called dedifferentiation or phenotypic modulation) (36, 41, 42) and revealed the enormous differentiation potential of vascular cells. Endothelial cells can become mesenchymal cells by a process called EndoMT, and SMCs can undergo phenotypic modulation and differentiate into Mϕ-like, fibroblast-like, osteoblast-like, or chondrocyte-like cells. EndoMT can occur under the influence of disturbed blood flow (43, 44) and strongly affect the number of SMCs that stabilize a fibrous cap (45). However, the role and existence of EndoMT in disease is not without controversy (46). When the mechanisms are clear, the current definition of an atherosclerotic plaque eventually can be fine-tuned or altered. Determining the transcriptomic landscape of single cells in human atherosclerotic plaques with scRNA-seq may identify cells in a transitional state, and the trajectory and fate of phenotypic cell switching can be depicted.
One of the particular advantages of using mouse model systems is the ability to trace cells over time, providing a unique viewpoint on atherosclerotic lesion progression (47, 48). This is not directly possible in humans but can be inferred using the appropriate methods. For example, previous attempts at human lineage tracing involved the tracing of histone modifications on histological slides (49). Human atherosclerotic research is hampered by a cross-sectional design, but the (temporary) preservation of transcriptomic features may point to cells in transition. In addition, bioinformatics tools such as the inference of cell trajectories, also known as “pseudotime” may facilitate the capturing of cells in transition. Pseudotime is a computational technique used to determine the pattern of a dynamic process experienced by cells and then arrange the cells based on their progression through the process. Differences in gene expression between cells result from dynamic processes such as simple cell cycle progression or cell differentiation. In pseudotime such differences are depicted by placing cells along a continuous path that represents the evolution of the process rather than dividing cells into discrete clusters. To find evidence of EndoMT or phenotypic switching in heterogeneous tissue is not unambiguous, but knowledge from lineage tracing experiments in mice can be used to impute this (Figure 2). The assumption then is, that these processes are continuously active and cells from transient stages are always present. However, mice lesions are harvested in an earlier stage of atherosclerosis, whilst human plaques have progressed much further and are end-stage, often symptomatic, lesions. Naturally occurring markers fluctuate during cell transitions and cell fates are harder to trace without a reporter gene. For this reason, it is possible that the intermediate stages are not captured in either case and it is difficult to determine if they were present at all. Lineage tracing is a powerful tool to investigate putative cell transitions in time. A common practice is to label individual cells at an early or induced time point to trace the lineage of labeled daughter cells at a later time point. Lineage tracing is particularly insightful when the process at hand is less unambiguous to impute, such as phenotypic switching. The limitations of this analysis are reviewed elsewhere (50).
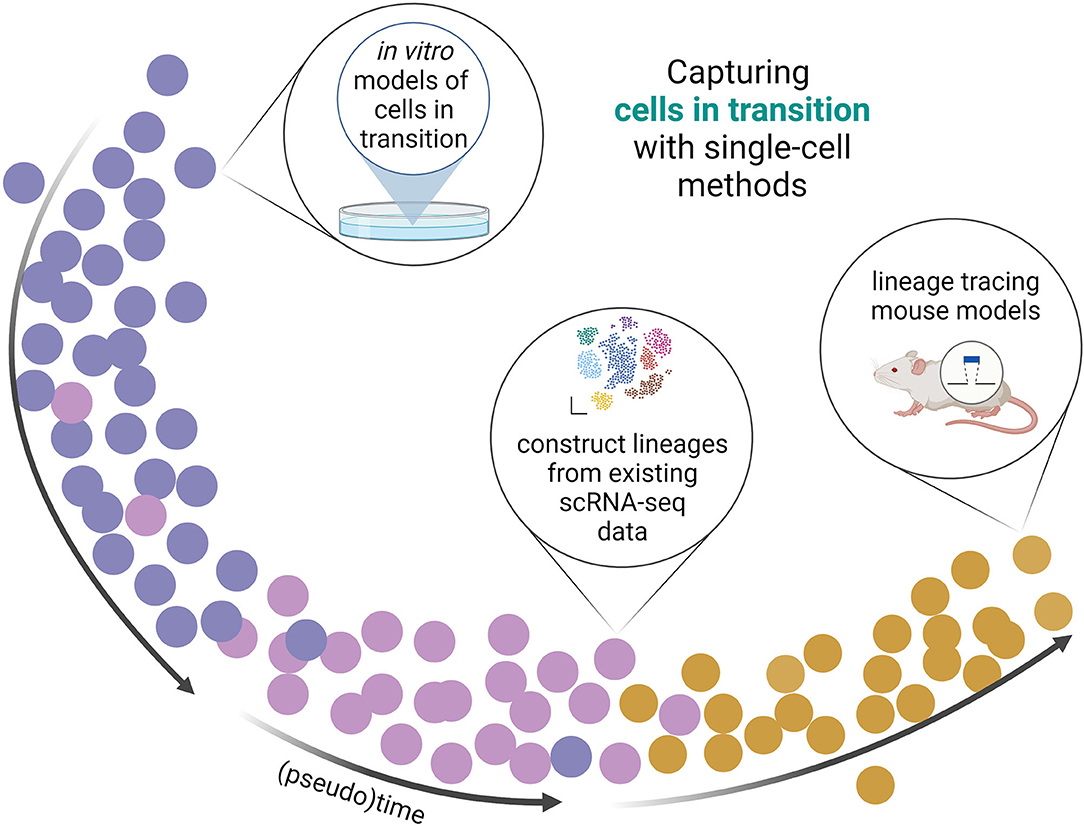
Figure 2. Capturing cells in transition with single-cell methods. Single-cell methods fascilitate the detection of cells in transition. scRNA and/or scATAC facilitate (re)constructing or confirmation of lineages of cells transitioning between phenotypes or cell states. With bioinformatical tools, data from in vitro, in silico, and in vivo experiments can be used to place cells on an artificial timeline (pseudotime) from which the cell transitions can be studied.
The fate of bone marrow derived cells can be followed in mice via bone marrow transplant. This method is often combined with lineage tracing techniques where the labeled bone marrow is grafted into wild-type or sex-mismatched mice. These studies show that bone marrow derived cells mainly give rise to inflammatory cells in the plaques and do not transdifferentiate into SMCs or ECs (51). However, supportive scRNA-seq evidence is lacking. A lineage tracing study following the fate of myeloid cells revealed that specialized aortic intima resident macrophages reside in the intima of the aortic arch (52). These resident macrophages give rise to early foam cells of the lesion but were not sustained during atherosclerotic progression. FoxP3 lineage tracing mice experiments showed that Treg cells switch to pro-atherogenic T follicular helper cells during atherosclerosis (53, 54). In order to determine which cells in plaque have SMC origin and how these cells contribute to disease, Shankman et al. (47) used an SMC YFP+/+Apoe−/− mouse model and labeled medial SMCs with YFP labels after tamoxifen injection. This revealed that 36% of LGALS3+ cells were of SMC origin and rather than of myeloid origin. Furthermore, they state that around 80% of cells with SMC origin are phenotypically modulated. A study from the same group with SMC−DualLineage tracing mouse models showed that the percentage of LGALS3+ cells was lower at around 25% (45). scRNA-seq data from these mouse models made it possible to compare cells across species. This revealed that all cell populations observed in the mice could be identified in human data, indicating that the transcriptional signatures can be used to identify cells that cannot be lineage traced in humans. The tamoxifen-induced ROSA26ZsGreen1/+; Myh11-CreERT2 mouse model of Pan et al. (11) revealed that SMCs in the lesion give rise to multiple cell-populations or cell states, including fibrochondrocytes and Mϕ-like cells. In a recent meta-analysis of scRNA-seq and scATAC-seq lineage tracing experiments, Conklin et al. (40) conclude that lipid loading of SMCs in vivo does not correlate to the changes observed in vitro. Highlighting the added value of lineage-tracing experiments compared to in vitro.
Cell-Cell Communication
Cell-cell communication (CCC) and cell-cell interactions are interactions between cells regulated by biochemical signaling. Molecules excreted by cells can be used to establish CCC or are part of the structural integrity of tissue, such as the extracellular matrix. scRNA-seq offers the opportunity to study the communicative links between cells in the lesions. Although a proxy for actual protein-protein interactions, the detailed RNA profile of cells can be leveraged to infer CCC from a transcriptomic viewpoint. The bioinformatical tools to impute CCC from this data have been rapidly maturing [reviewed by Almet et al. (55)], varying in their sensitivity and approach.
Previously, we examined the potential receptor-ligand interactions in carotid lesions. Overall, the majority of the interactions were between myeloid cells, SMCs, and ECs (10). The low number of potential interactions between immune cells by the lack of T Cell receptor-related genes can be possibly accounted to their absence in the receptor-ligand interaction database. The occurrence of false-negative or false-positive communication is not just a result of incomplete databases. For a large part, cells can communicate over a limited spatial distance, limiting the number of interactions that can physically take place within the plaque. Interactions by secreted molecules such as cytokines can, indeed, facilitate communications over larger distances. However, interactions where both ligand and receptor are present on the surface of the cells, such as the interaction between CD36 and THBS1, require physical proximity. This spatial information is lost with regular scRNA-seq. A novel sequencing technique makes use of doublets occurring in scRNA-seq data leveraging the idea that cells that are stuck together are likely to be physically interacting. By deconvoluting the single counterpart of doublet cells, the relationship between the physically interacting cells can be studied (56).
Single-Cell Sequencing and Understanding of Sex Differences in Atherosclerotic Disease
Next to the well-known risk factors for atherosclerotic disease [e.g., diabetes, smoking, hypertension, hypercholesterolemia, ethnicity (57) etc.] (58) also sex (59, 60) strongly associates with different clinical presentations and underlying atherosclerotic pathology. Understanding the underlying molecular and cellular mechanisms remains a significant challenge.
In murine BXH ApoE−/− model, the lesion size was increased in females (61). In humans, the sex-stratified gene regulatory networks derived from bulk RNA-sequencing data from atherosclerotic aortic root tissue showed large sex specific differences (62). The genes involved in female-specific networks have higher expression in SMCs, ECs, and Mϕs and are involved in epithelial to mesenchymal transition, KRAS signaling, and estrogen response, respectively. The next step will be to assess the cell types that are responsible for this transcriptomic diversity between sexes. This highlights the importance of sex stratification in studying risk factors.
Sex-specific atherosclerotic murine (12–14) or multiple samples of both sexes human scRNA-seq data (10) is available. However, for these analyses, a sufficient number of samples is required to reach statistical power and based on lessons from histopathological biobank studies, hundreds of individual samples might be required. Intuitively, this explains the limited amount of studies addressing this topic. It is not unlikely that the scRNA-seq field will face a déjà vu when researchers start merging data sets, just like the ongoing GWAS efforts to reach sufficient power for stratified analyses. If sample numbers increase, there are no doubt that the exploration of cell-based gene transcriptomes in humans may resolve many unanswered questions regarding the underlying mechanisms that explain the complex diversity and the mechanisms of how risk factors like sex modify the disease progression.
scRNA-seq Observations Associate With Hemodynamics
Blood flow is of major influence on the formation of atherosclerosis. Lesions develop primarily in vessel regions where blood flow is disturbed by branching or curvature of the artery. Disturbed flow affects the behavior of arterial cells, but how it affects their respective transcriptome was poorly investigated. In a study on flow effect, Andueza et al. (43) performed scRNA-seq and the single-cell assay for transposase accessible chromatin sequencing (scATAC-seq) in a partial carotid ligation mouse model. Trajectory analysis showed that ECs are capable of dramatic phenotypical changes. The already heterogeneous EC population was found to be transitioning to a mesenchymal, hematopoietic stem cell, endothelial stem/progenitor cell, and unexpected immune cell-like phenotypes (43).
Similarly, Li et al. (44) performed scRNA-seq on mice lesions after partial carotid ligation. Compared to the laminar flow condition, they found cell populations specific for both models. A disturbed flow EC population was found to be more enriched in processes such as epithelial to mesenchymal transition and TGF-β signaling. Disturbed flow-specific SMC and Mϕs were also reported (44). Current studies rarely take into account the influence of flow on the outcome of scRNA-seq experiments. However, with the notion that most studied plaque locations are subjected to disturbed flow (carotid artery and aortic arch), it is reasonable to assume that the disturbed flow could influence expression patterns of EC populations found in these lesions. In the future, spatial transcriptomics can help to resolve these questions.
Technical Considerations and Limitations
Source of Lesions
Atherosclerotic plaques develop in coronary, carotid, cerebral, aortic, iliac, and femoral arteries. The most commonly studied human plaques are located in the coronary and carotid arteries (32, 63). When it comes to defining the plaque on a cellular level, location matters (64). Although the arterial systems share characteristics in plaque morphology, there is a difference is the underlying substrate of the ischemic event. In coronary arteries, a local thrombosis caused by either plaque rupture or erosion leads to ischemia of downstream tissue. In the cerebral circulation, an embolization may take place from a plaque upstream of the cerebrovascular occlusion. In the carotid artery, intraplaque bleedings are observed more frequently, and in a significant number of cases, a fibrous lesion underlies the thrombotic event (65, 66). Human coronary plaque samples availability relies on the use of donor's hearts from recipients during transplantation. On the other hand, carotid plaques are dissected from living patients that can still be asymptomatic at the time of surgery. In murine research, aortic tissue is the most prevalent source (32, 63). Although these differences make comparing the coronary and carotid plaque and interspecies comparisons difficult (12), scRNA-seq can potentially unravel differential processes underlying a clinically relevant event. The common hurdle is tissue preservation prior to sequencing. In order to get the full potential from the lesion, sample handling has to be quick to keep it as fresh as possible, in contrast to histological samples where there is less time constraint for processing.
During carotid endarterectomy, the intima with the plaque is removed from the vessel while the tunica media stays majorly intact. Plaques derived from the aorta are likely clippings of diseased samples taken after heart transplants. This is ultimately reflected in the scRNA-seq data, where aortic plaques often show more significant SMCs numbers and phenotypic variety (8). Cochain et al. (13) observed that macrophages are the most dominant immune cell type in atherosclerotic plaques by scRNA-seq. But researchers using CD45+ positive selection with FACS will not report CD45− populations such as SMCs (9). Different digestion protocols can heavily skew cell selection and survival before sequencing, even if there is no prior selection. This can dramatically affect cells with poor survival rates ex-vivo, such as foam cells and neutrophils. This way, cell populations can become over and under-represented in scRNA-seq (40, 67). Therefore, caution is needed when interpreting results, and reports of cell ratios should always be carefully considered. Additionally, because of the need for digestion of solid tissues prior to scRNA-seq, the spatial location of cells is lost during the process.
Technical Limitations
A major limitation of scRNA-seq is the biases in transcript coverage and low capture efficiency, which additionally vary between protocols (33, 68, 69). Incomplete reverse transcription during second-strand synthesis and amplification of the samples can lead to fewer transcripts with the complete 5′ and/or 3′ ends. Gene length influences efficacy, and shorter genes generally have lower counts in single-cell sequencing compared to bulk RNA sequencing (70). As a consequence, genes with a low expression may not be detected due to dropouts and potentially create false negatives. A related obstacle is the use of poly(T) primer, resulting in only RNAs with a poly-A-tail being sequenced (68). Sequencing non-polyadenylated RNAs would be beneficial since these RNAs often serve regulatory functions.
The cost of scRNA-seq is another limiting factor in comparison to traditional bulk sequencing. Even though since 2017, the costs of single-cell sequencing have dropped significantly, single-cell sequencing is still 10 to 200 times more expensive per sample than bulk sequencing (52). Details on different methodologies are reviewed elsewhere (52).
A natural consequence of the growing popularity of scRNA-seq is the increasing need for specialized experimentalists, developers, and bioinformaticians as scRNA-seq gains popularity. However, finding the right tools to implement scRNA-seq into our research is not without caveats for experienced researchers. For example, the scRNA-tools database tracks 1,124 specialized tools for scRNA-seq analysis across 30 categories at the time of writing (71).
Future Perspectives
Spatial Location
One of the current limitations of single-cell transcriptomics is that spatial information of cells is lost, but therein also lies an opportunity for the future. Not only can spatial context provide insight into the mechanisms of atherosclerotic disease, but it also greatly influences our interpretation of cell-cell communication. Multiple ways can be used to visualize the spatial gene expression in plaque tissue. Spatial barcoding, in situ hybridization, and in situ sequencing can all visualize spatial gene expression. However, none of these techniques can cover the full spectrum of the transcriptome like RNA-seq can provide [reviewed by Longo et al. (72)]. Laser-capture microdissection coupled with RNA-seq and downstream analysis can give a detailed view of specific regions of interest like plaque cap or pinpoint to cells with specific phenotypes, under the condition that these cells can be located. This method has been applied successfully in the past on mouse tissue (73). However, the technical challenges to keep plaque tissue in a condition where it is possible to preserve, locate, isolate, and sequence cells of interest are limiting. The growing interest in these techniques is already reflected in implementing these techniques in the major sequencing platforms (74) and data analysis tools (71, 75). However, the current state of spatial technology sequencing does not fully allow for sequencing at individual cell resolution. Future advancements in spatial sequencing can possibly make it a powerful tool to shine new light on spatio-temporal gene expression in atherosclerotic disease.
Underlying Mechanisms Explaining the Diversity in Pathological Substrates of Clinically Relevant Plaques
One of the most prominent dogmas in atherosclerotic research is the concept of plaque rupture vs. plaque erosion. A concept well-described through histology and clinical presentation. The atherosclerotic plaque may rupture, which leads to red thrombosis due to blood coagulation components getting access to the core of the plaque. If the thrombus arises in coronary arteries or embolises the cerebral arteries, it results in a myocardial infarction or stroke. Plaques that rupture often have a large lipid core with a thin and weak fibrous cap and are called vulnerable plaques or thin-capped fibroatheromas (TCFAs). Besides plaque rupture, plaque erosion may arise (76, 77). These plaques are rich in ECM, have a thick cap and have little lipid deposits with superimposed (white) thrombi. The characteristics described in the TCFA model serve to date as the surrogate endpoints for a vulnerable plaque in animal models. Among other processes, this has led to valuable insights into the role of LDL oxidation, plaque hemorrhage, accumulation, SMC migration, proliferation and apoptosis, senescence, and the role of the variation of local inflammatory cells in the diseased vessel wall. The mechanisms leading to plaque erosion are less well-understood and are one of the major upcoming challenges of the vascular biology community. Animals models hardly develop a thrombotic response, and the lesion underlying the thrombus is thus far categorized as “stable.” For both processes, the mechanisms on a single cell level have not been revealed. The variety of sub-populations identified could be partly explained by these phenomena. Future research may reveal what cells contribute to a pro-thrombotic micro-environment and provide clues for underlying mechanisms.
Cell Plasticity
In lesions, cells can switch to a new phenotypical identity. These switches include EndoMT (78) and transition from SMC to a Mϕ-like phenotype or foam cells (36). scRNA-seq allows studying cellular plasticity in atherosclerosis in more detail and higher resolution (79). Tools such as the earlier-mentioned pseudotime can aid in studying these processes in mouse model systems and humans.
Dobnikar et al. (80) looked into the SMCs in healthy vessels and atherosclerotic plaque tissue from mice. A remarkable finding was a distinct SMC cluster that expressed Sca1—implying that these cells are transitioning from SMC to a Mϕ-like cell. Indications for the transitional capacity of vascular cells was also demonstrated in mice (45). Three SMC clusters expressed both endothelial cell and SMC marker genes, which suggests a transition between phenotypes. In addition, we found that a small subset of human SMCs was KLF4+ (10), which points to cells that differentiate into a cell with a more synthetic or Mϕ-like phenotype. The value of scRNA-seq to unravel the fate of and origin of transdifferentiating SMC was underlined by a study by Wirka et al. (8), who found that TCF21 expression was associated with the phenotypic switch of SMC into fibrocytes, with the subsequent strengthening of the fibrous cap and stabilization of the lesion (8). Another mouse study detected an unexpected cluster of proliferating monocytes with a stem cell-like signature, suggesting that monocytes may persist in a proliferating self-renewal state in inflamed tissue and that not all monocytes differentiate immediately into Mϕs after entering the vascular tissue (15). A recent meta-analysis (40) addressed the SMC heterogeneity between four different murine scRNA-seq datasets. Researchers conclude that SMC are capable of sourcing multiple different trajectories within lesions, with many of these transitioning through a de-differentiated SMC state defined by Pan et al. (11) as stem-cell, endothelial cell, monocyte (SEM) cells. The field would benefit from a coalescent review of plastic cells found in lesions to understand their source and impact on plaque development.
Attack of the Clones
Clonal expansion theory is derived from cancer research. It is a process where one parent cell multiplies as a consequence of acquired mutations. The notion of clonality in atherosclerosis is not a novel theory (81), but there are doubts on the true monoclonality and its consequence on atherosclerotic cells. The clonal environment is likely already present around the plaque, which contributes to the expansion of the lesion (82). Mice with a Jak2VF mutation showed increased inflammatory and proliferative myeloid populations in their lesions, as confirmed with scRNA-seq. In addition, these plaques show more pronounced necrotic cores (83). It is unclear whether these plaques exhibit clonal regions. Clonal expansion is mainly described for leukocytes, but in plaque, the concept applies similarly to SMCs. Mice fed with a high-fat diet showed an increase of dedifferentiated SMCs expressing the stem cell marker Sca1, forming hubs of clonally expanded cells that tended to form around the necrotic core. Researchers also report that clonally expanding SMCs aggravate atherosclerosis by activating the complement system (84). scRNA-seq confirmed that these cells exhibit a different phenotype from regular SMCs. Other findings in mice describe sheets of clonally expanded SMCs derived from the media rather than patches (85). The origin of these clonal progenitor cells giving rise to these clonal patches is still unclear, and the occurrence of clonal expansion is seemingly random. Whether or not this clonal expansion of SMCs is beneficial for plaque stability is still up for debate.
The use of lineage tracing techniques has been majorly beneficial for the research on clonality in plaque tissue. A privilege that cannot be extended to human research. The alternatives are tracing naturally occurring markers in know cell differentiation pathways or detecting somatic mutations (Figure 3). A novel approach integrates scRNA-seq and scATAC-seq of mitochondrial RNA for both clonal and lineage tracing (86). This method can be applied to already existing single-cell datasets of lesion tissue and can confirm these long-standing observations of clonal expansion in plaque.
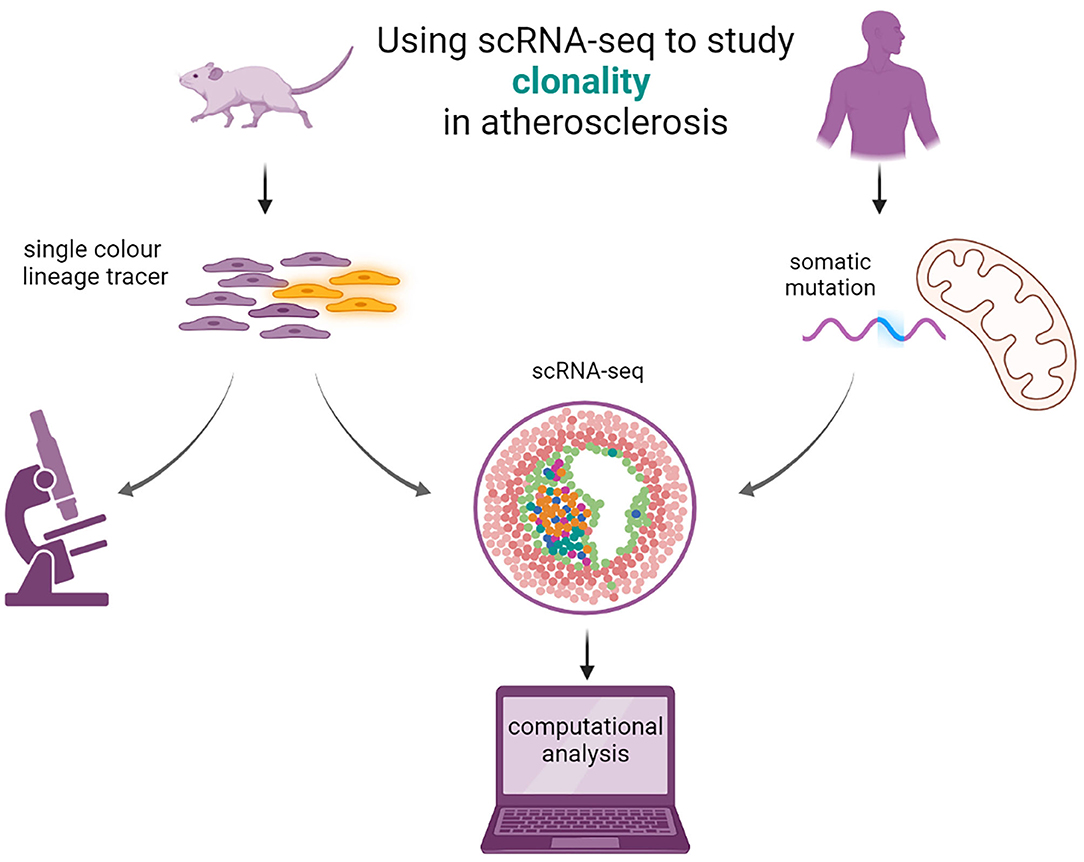
Figure 3. Using scRNA-seq to study clonal cell expansion in atherosclerosis. Single color lineage tracers are added to reporter genes to study the clonality in mice (left). The mice will develop atherosclerosis and patches of clonal cells are visible from microscopy images. scRNA seq can be performed, and computational analysis can elucidate the transcriptomic properties of the cells expressing the lineage tracer. In humans (right), clonality can be imputed from studying the somatic mutations in mitochondria with scRNA- or scATAC-seq from existing data with computational analysis.
Integrating scRNA-seq in Post-GWAS Analyses
The reported number of common genetic variants associated with coronary artery disease (87) ischemic stroke (88), and intermediate traits of atherosclerosis, such as carotid intima-media thickness (89), is increasing as the meta-analysis efforts of genome-wide association studies (GWAS) grow more prominent and larger (90). However, the functions of many genes that are considered to be causally related to cardiovascular diseases are unknown for roughly 50% of the loci discovered (90). The expression of these potentially causal genes or other downstream interacting genes can originate from different organs of which the (atherosclerotic) vasculature is one. Much research currently aims to prioritize the “right” genes underlying the GWAS loci through integration with molecular quantitative trait locus (molQTL) analyses in various tissues. In contrast, these efforts prioritize genes but they do not provide information on the cellular context (91). As we (92) and others (21) recently showed, the integration of GWAS summary statistics with plaque-derived scRNA-seq and/or snATAC-seq data aids in the prioritization of genes and cell populations relevant to disease, which in turn can be used as a guideline for in vitro and in vivo mechanistic research.
This could further be augmented by single-cell molQTL (sc-molQTL) analyses. Indeed, heritable genetic effects that modulate expression at a single-cell resolution may affect specific cellular processes in disease (93, 94). Studies focused on discovering genetic effects on proximal genes, cis-acting single-cell expression QTLs (cis-sc-eQTLs), across populations will face two challenges. First, most analytical tools are not designed to consider the sparsity (the zero-inflated expression) in single-cell data. New methods are developed, but this field has not matured (95). Second, expression and genetic variation is also population-specific, and many datasets are now mainly derived from European ancestral populations. An inclusive approach to population diversity will provide a fine-grained map of genetic variation affecting cellular processes at a single cell level in disease tissue.
From Mice to Men
The extrapolation of results from atherosclerotic mouse models to human advanced stages of atherosclerosis is still an ongoing matter of debate. Indeed, the translation from mouse to human regarding the mechanisms of initiation and progression of atherosclerosis has been shown to be complex. Small-scale comparison between specific murine and human cell-subsets have been attempted (8, 10, 11, 52), and to a smaller extent, larger scale comparison efforts have been made (16, 40). However, animals models hardly develop a thrombotic response, and the lesion underlying the thrombus is thus far categorized as “stable.” Nonetheless, the determinants of end-stage atherosclerosis in humans have been used as benchmarks in atherosclerotic mice. However, verification in human plaques was mainly performed by demonstrating gene or protein expression differences between symptomatic and asymptomatic plaques (9). This single gene/protein cross-sectional approach was difficult to translate into a specific role in either plaque stabilization or destabilization. While still observational, scRNA-seq could indicate in which cell a gene is expressed, given the transcriptomic context in which the gene is located. This location is not limited to cell-population-specific expression but extends to their position in networks and pathways. This could provide the opportunity to better translate and verify the value of the mouse models to human plaques. Further comparative research will reveal the extent to which the gene expressions found occurring from a genetically identical mouse strain can be translated to the genetically heterogeneous human population and whether expression patterns are generic or individual-specific. A recent study compared mice and human vascular SMCs and reported that the many similarities favor the continued use of mice models to extrapolate the fate of the human counterpart cells (40). However, in a systematic review, we reported that genes involved in human genetic variants for coronary artery disease, large artery ischemic stroke, or atherosclerotic plaque characteristics seldom show associations in mice (96). The vast body of work describing the cellular composition of mice compared to human findings offers the opportunity to investigate population similarities and could contribute to the translation from murine to human atherosclerosis.
Concluding Remarks
The value of scRNA-seq in elucidating the mechanisms of arteriosclerosis is beyond doubt. The current literature already indicates a much greater diversity of inflammatory and resident cell types than previously believed. The plasticity of vascular cells has been demonstrated thanks to scRNA-seq, and the first steps to spatial gene expression in plaque are taking place. However, the field would benefit from a consensus in cell-population nomenclature to match findings from different groups and facilitate inter-species comparisons. The rapid development of scRNA-seq will drive down the prices and therefore, more samples can be analyzed, which is currently the biggest limitation. A new limitation will arise, which is inevitably tied to the ever-growing popularity of big data: the expert (man) power to analyse and biologically interpret the data.
Author Contributions
LS, DT, and GP prepared the original draft. LS drafted the manuscript and designed figures. SL and MM contributed to manuscript extension and revision. All authors contributed to the article and approved the submitted version.
Funding
This work was supported by grants from: Fondation Leducq (PlaqOmics 18CVD02) to GP. SL was funded through grants from the Netherlands Cardiovascular Research Initiative of the Netherlands Heart Foundation (CVON 2011/B019 and CVON 2017-20: Generating the best evidence-based pharmaceutical targets for atherosclerosis [GENIUS I&II]). We are thankful for the support of the ERA-CVD program druggable-MI-targets (grant number: 01KL1802) and the EU H2020 TO_AITION (grant number: 848146).
Conflict of Interest
The authors declare that the research was conducted in the absence of any commercial or financial relationships that could be construed as a potential conflict of interest.
Publisher's Note
All claims expressed in this article are solely those of the authors and do not necessarily represent those of their affiliated organizations, or those of the publisher, the editors and the reviewers. Any product that may be evaluated in this article, or claim that may be made by its manufacturer, is not guaranteed or endorsed by the publisher.
Acknowledgments
Figures were created with BioRender.com.
References
1. Davies MJ, Thomas A. Thrombosis and acute coronary-artery lesions in sudden cardiac ischemic death. N Engl J Med. (1984) 310:1137–40. doi: 10.1056/NEJM198405033101801
2. Libby P, Buring JE, Badimon L, Hansson GK, Deanfield J, Bittencourt MS, et al. Atherosclerosis. Nat Rev Dis Primer. (2019) 5:56. doi: 10.1038/s41572-019-0106-z
3. Hägg S, Skogsberg J, Lundström J, Noori P, Nilsson R, Zhong H, et al. Multi-organ expression profiling uncovers a gene module in coronary artery disease involving transendothelial migration of leukocytes and LIM domain binding 2: the Stockholm Atherosclerosis Gene Expression (STAGE) study. PLOS Genet. (2009) 5:e1000754. doi: 10.1371/journal.pgen.1000754
4. Folkersen L, Persson J, Ekstrand J, Agardh HE, Hansson GK, Gabrielsen A, et al. Prediction of ischemic events on the basis of transcriptomic and genomic profiling in patients undergoing carotid endarterectomy. Mol Med Camb Mass. (2012) 18:669–75. doi: 10.2119/molmed.2011.00479
5. Franzén O, Ermel R, Cohain A, Akers NK, Di Narzo A, Talukdar HA, et al. Cardiometabolic risk loci share downstream cis- and trans-gene regulation across tissues and diseases. Science. (2016) 353:827–30. doi: 10.1126/sciencetalad6970
6. Perisic L, Aldi S, Sun Y, Folkersen L, Razuvaev A, Roy J, et al. Gene expression signatures, pathways and networks in carotid atherosclerosis. J Intern Med. (2016) 279:293–308. doi: 10.1111/joim.12448
7. Butcher MJ, Filipowicz AR, Waseem TC, McGary CM, Crow KJ, Magilnick N, et al. Atherosclerosis-driven treg plasticity results in formation of a dysfunctional subset of plastic IFNγ+ Th1/Tregs. Circ Res. (2016) 119:1190–203. doi: 10.1161/CIRCRESAHA.116.309764
8. Wirka RC, Wagh D, Paik DT, Pjanic M, Nguyen T, Miller CL, et al. Atheroprotective roles of smooth muscle cell phenotypic modulation and the TCF21 disease gene as revealed by single-cell analysis. Nat Med. (2019) 25:1280–9. doi: 10.1038/s41591-019-0512-5
9. Fernandez DM, Rahman AH, Fernandez NF, Chudnovskiy A, Amir E ad D, Amadori L, et al. Single-cell immune landscape of human atherosclerotic plaques. Nat Med. (2019) 25:1576–88. doi: 10.1038/s41591-019-0590-4
10. Depuydt MAC, Prange KHM, Slenders L, Örd T, Elbersen D, Boltjes A, et al. Microanatomy of the human atherosclerotic plaque by single-cell transcriptomics. Circ Res. (2020) 127:1437–55. doi: 10.1161/CIRCRESAHA.120.316770
11. Pan H, Xue C, Auerbach BJ, Fan J, Bashore AC, Cui J, et al. Single-cell genomics reveals a novel cell state during smooth muscle cell phenotypic switching and potential therapeutic targets for atherosclerosis in mouse and human. Circulation. (2020) 142:2060–75. doi: 10.1161/CIRCULATIONAHA.120.048378
12. Winkels H, Ehinger E, Vassallo M, Buscher K, Dinh HQ, Kobiyama K, et al. Atlas of the immune cell repertoire in mouse atherosclerosis defined by single-cell RNA-sequencing and mass cytometry. Circ Res. (2018) 122:1675–88. doi: 10.1161/CIRCRESAHA.117.312513
13. Cochain C, Vafadarnejad E, Arampatzi P, Pelisek J, Winkels H, Ley K, et al. Single-cell RNA-seq reveals the transcriptional landscape and heterogeneity of aortic macrophages in murine atherosclerosis. Circ Res. (2018) 122:1661–74. doi: 10.1161/CIRCRESAHA.117.312509
14. Kim K, Shim D, Lee JS, Zaitsev K, Williams JW, Kim K-W, et al. Transcriptome analysis reveals nonfoamy rather than foamy plaque macrophages are proinflammatory in atherosclerotic murine models. Circ Res. (2018) 123:1127–42. doi: 10.1161/CIRCRESAHA.118.312804
15. Lin J-D, Nishi H, Poles J, Niu X, Mccauley C, Rahman K, et al. Single-cell analysis of fate-mapped macrophages reveals heterogeneity, including stem-like properties, during atherosclerosis progression and regression. JCI Insight. (2019) 4:e124574. doi: 10.1172/jci.insight.124574
16. Ma WF, Hodonsky CJ, Turner AW, Wong D, Song Y, Mosquera JV, et al. Enhanced single-cell RNA-seq workflow reveals coronary artery disease cellular cross-talk and candidate drug targets. Atherosclerosis. (2021) 340:12–22. doi: 10.1016/j.atherosclerosis.2021.11.025
17. Bagnato C, Thumar J, Mayya V, Hwang S-I, Zebroski H, Claffey KP, et al. Proteomics analysis of human coronary atherosclerotic plaque: a feasibility study of direct tissue proteomics by liquid chromatography tandem mass Spectrometry*S. Mol Cell Proteomics. (2007) 6:1088–102. doi: 10.1074/mcp.M600259-MCP200
18. Vaisar T, Hu JH, Airhart N, Fox K, Heinecke J, Nicosia RF, et al. Parallel murine and human plaque proteomics reveals pathways of plaque rupture. Circ Res. (2020) 127:997–1022. doi: 10.1161/CIRCRESAHA.120.317295
19. Mokry M, Boltjes A, Cui K, Slenders L, Mekke JM, Depuydt MAC, et al. Transcriptomic-based clustering of advanced atherosclerotic plaques identifies subgroups of plaques with differential underlying biology that associate with clinical presentation. medRXiv. (2021). doi: 10.1101/2021.11.25.21266855
20. Tang F, Barbacioru C, Wang Y, Nordman E, Lee C, Xu N, et al. mRNA-Seq whole-transcriptome analysis of a single cell. Nat Methods. (2009) 6:377–82. doi: 10.1038/nmeth.1315
21. Örd T, Õunap K, Stolze LK, Aherrahrou R, Nurminen V, Toropainen A, et al. Single-cell epigenomics and functional fine-mapping of atherosclerosis GWAS Loci. Circ Res. (2021) 129:240–58. doi: 10.1161/CIRCRESAHA.121.318971
22. Williams JW, Zaitsev K, Kim K-W, Ivanov S, Saunders BT, Schrank PR, et al. Limited proliferation capacity of aortic intima resident macrophages requires monocyte recruitment for atherosclerotic plaque progression. Nat Immunol. (2020) 21:1194–204. doi: 10.1038/s41590-020-0768-4
23. Papalexi E, Satija R. Single-cell RNA sequencing to explore immune cell heterogeneity. Nat Rev Immunol. (2018) 18:35–45. doi: 10.1038/nri.2017.76
24. Satija R, Farrell JA, Gennert D, Schier AF, Regev A. Spatial reconstruction of single-cell gene expression data. Nat Biotechnol. (2015) 33:495–502. doi: 10.1038/nbt.3192
25. McCarthy DJ, Campbell KR, Lun ATL, Wills QF. Scater: pre-processing, quality control, normalization and visualization of single-cell RNA-seq data in R. Bioinformatics. (2017) 33:1179–86. doi: 10.1093/bioinformatics/btw777
26. Wolf FA, Angerer P, Theis FJ. SCANPY: large-scale single-cell gene expression data analysis. Genome Biol. (2018) 19:15. doi: 10.1186/s13059-017-1382-0
27. Grün D, Lyubimova A, Kester L, Wiebrands K, Basak O, Sasaki N, et al. Single-cell messenger RNA sequencing reveals rare intestinal cell types. Nature. (2015) 525:251–5. doi: 10.1038/nature14966
28. Saelens W, Cannoodt R, Todorov H, Saeys Y. A comparison of single-cell trajectory inference methods. Nat Biotechnol. (2019) 37:547–54. doi: 10.1038/s41587-019-0071-9
29. La Manno G, Soldatov R, Zeisel A, Braun E, Hochgerner H, Petukhov V, et al. RNA velocity of single cells. Nature. (2018) 560:494–8. doi: 10.1038/s41586-018-0414-6
30. Armingol E, Officer A, Harismendy O, Lewis NE. Deciphering cell–cell interactions and communication from gene expression. Nat Rev Genet. (2021) 22:71–88. doi: 10.1038/s41576-020-00292-x
31. Zernecke A, Winkels H, Cochain C, Williams JW, Wolf D, Soehnlein O, et al. Meta-analysis of leukocyte diversity in atherosclerotic mouse aortas. Circ Res. (2020) 127:402–26. doi: 10.1161/CIRCRESAHA.120.316903
32. Fu M, Song J. Single-cell transcriptomics reveals the cellular heterogeneity of cardiovascular diseases. Front Cardiovasc Med. (2021) 8:643519. doi: 10.3389/fcvm.2021.643519
33. Iqbal F, Lupieri A, Aikawa M, Aikawa E. Harnessing single-cell RNA sequencing to better understand how diseased cells behave the way they do in cardiovascular disease. Arterioscler Thromb Vasc Biol. (2021) 41:585–600. doi: 10.1161/ATVBAHA.120.314776
34. Yap C, Mieremet A, de Vries CJM, Micha D, de Waard V. Six shades of vascular smooth muscle cells illuminated by KLF4 (Krüppel-Like Factor 4). Arterioscler Thromb Vasc Biol. (2021) 41:2693–707. doi: 10.1161/ATVBAHA.121.316600
35. Paneni F, Costantino S, Castello L, Battista R, Capretti G, Chiandotto S, et al. Targeting prolyl-isomerase Pin1 prevents mitochondrial oxidative stress and vascular dysfunction: insights in patients with diabetes. Eur Heart J. (2015) 36:817–28. doi: 10.1093/eurheartj/ehu179
36. Bennett MR, Sinha S, Owens GK. Vascular smooth muscle cells in atherosclerosis. Circ Res. (2016) 118:692–702. doi: 10.1161/CIRCRESAHA.115.306361
37. Li Y, Zhu H, Zhang Q, Han X, Zhang Z, Shen L, et al. Smooth muscle-derived macrophage-like cells contribute to multiple cell lineages in the atherosclerotic plaque. Cell Discov. (2021) 7:1–4. doi: 10.1038/s41421-021-00328-4
38. Winkels H, Wolf D. Heterogeneity of T cells in atherosclerosis defined by single-cell RNA-sequencing and cytometry by time of flight. Arterioscler Thromb Vasc Biol. (2021) 41:549–63. doi: 10.1161/ATVBAHA.120.312137
39. Sharma M, Schlegel MP, Afonso MS, Brown EJ, Rahman K, Weinstock A, et al. Regulatory T cells license macrophage pro-resolving functions during atherosclerosis regression. Circ Res. (2020) 127:335–53. doi: 10.1161/CIRCRESAHA.119.316461
40. Conklin AC, Nishi H, Schlamp F, Örd T, Õunap K, Kaikkonen MU, et al. Meta-analysis of smooth muscle lineage transcriptomes in atherosclerosis and their relationships to in vitro models. Immunometabolism. (2021) 3:e210022. doi: 10.20900/immunometab20210022
41. Gomez D, Owens GK. Smooth muscle cell phenotypic switching in atherosclerosis. Cardiovasc Res. (2012) 95:156–64. doi: 10.1093/cvr/cvs115
42. Souilhol C, Harmsen MC, Evans PC, Krenning G. Endothelial–mesenchymal transition in atherosclerosis. Cardiovasc Res. (2018) 114:565–77. doi: 10.1093/cvr/cvx253
43. Andueza A, Kumar S, Kim J, Kang D-W, Mumme HL, Perez JI, et al. Endothelial reprogramming by disturbed flow revealed by single-cell RNA and chromatin accessibility study. Cell Rep. (2020) 33:108491. doi: 10.1016/j.celrep.2020.108491
44. Li F, Yan K, Wu L, Zheng Z, Du Y, Liu Z, et al. Single-cell RNA-seq reveals cellular heterogeneity of mouse carotid artery under disturbed flow. Cell Death Discov. (2021) 7:1–14. doi: 10.1038/s41420-021-00567-0
45. Alencar GF, Owsiany KM, Karnewar S, Sukhavasi K, Mocci G, Nguyen AT, et al. Stem cell pluripotency genes Klf4 and Oct4 regulate complex SMC phenotypic changes critical in late-stage atherosclerotic lesion pathogenesis. Circulation. (2020) 142:2045–59. doi: 10.1161/CIRCULATIONAHA.120.046672
46. Hong L, Du X, Li W, Mao Y, Sun L, Li X. EndMT: a promising and controversial field. Eur J Cell Biol. (2018) 97:493–500. doi: 10.1016/j.ejcb.2018.07.005
47. Shankman LS, Gomez D, Cherepanova OA, Salmon M, Alencar GF, Haskins RM, et al. KLF4-dependent phenotypic modulation of smooth muscle cells has a key role in atherosclerotic plaque pathogenesis. Nat Med. (2015) 21:628–37. doi: 10.1038/nm.3866
48. Bulut GB, Alencar GF, Owsiany KM, Nguyen AT, Karnewar S, Haskins RM, et al. KLF4 (Kruppel-Like Factor 4)-dependent perivascular plasticity contributes to adipose tissue inflammation. Arterioscler Thromb Vasc Biol. (2021) 41:284–301. doi: 10.1161/ATVBAHA.120.314703
49. Gomez D, Shankman LS, Nguyen AT, Owens GK. Detection of histone modifications at specific gene loci in single cells in histological sections. Nat Methods. (2013) 10:171–7. doi: 10.1038/nmeth.2332
50. Tritschler S, Büttner M, Fischer DS, Lange M, Bergen V, Lickert H, et al. Concepts and limitations for learning developmental trajectories from single cell genomics. Development. (2019) 146:dev170506. doi: 10.1242/dev.170506
51. Sun X, Lyu L, Zhong X, Ni Z, Xu Q. Application of genetic cell-lineage tracing technology to study cardiovascular diseases. J Mol Cell Cardiol. (2021) 156:57–68. doi: 10.1016/j.yjmcc.2021.03.006
52. Williams JW, Winkels H, Durant CP, Zaitsev K, Ghosheh Y, Ley K. Single cell RNA sequencing in atherosclerosis research. Circ Res. (2020) 126:1112–26. doi: 10.1161/CIRCRESAHA.119.315940
53. Gaddis DE, Padgett LE, Wu R, McSkimming C, Romines V, Taylor AM, et al. Apolipoprotein AI prevents regulatory to follicular helper T cell switching during atherosclerosis. Nat Commun. (2018) 9:1095. doi: 10.1038/s41467-018-03493-5
54. Wolf D, Gerhardt T, Winkels H, Michel NA, Pramod AB, Ghosheh Y, et al. Pathogenic autoimmunity in atherosclerosis evolves from initially protective apolipoprotein B100–Reactive CD4+ T-Regulatory cells. Circulation. (2020) 142:1279–93. doi: 10.1161/CIRCULATIONAHA.119.042863
55. Almet AA, Cang Z, Jin S, Nie Q. The landscape of cell–cell communication through single-cell transcriptomics. Curr Opin Syst Biol. (2021) 26:12–23. doi: 10.1016/j.coisb.2021.03.007
56. Giladi A, Cohen M, Medaglia C, Baran Y, Li B, Zada M, et al. Dissecting cellular crosstalk by sequencing physically interacting cells. Nat Biotechnol. (2020) 38:629–37. doi: 10.1038/s41587-020-0442-2
57. Gijsberts CM, Groenewegen KA, Hoefer IE, Eijkemans MJC, Asselbergs FW, Anderson TJ, et al. Race/ethnic differences in the associations of the framingham risk factors with carotid IMT and cardiovascular events. PLoS ONE. (2015) 10:e0132321. doi: 10.1371/journal.pone.0132321
58. Lechner K, von Schacky C, McKenzie AL, Worm N, Nixdorff U, Lechner B, et al. Lifestyle factors and high-risk atherosclerosis: pathways and mechanisms beyond traditional risk factors. Eur J Prev Cardiol. (2020) 27:394–406. doi: 10.1177/2047487319869400
59. Vrijenhoek JEP, Den Ruijter HM, De Borst GJ, de Kleijn DPV, De Vries J-PPM, Bots ML, et al. Sex is associated with the presence of atherosclerotic plaque hemorrhage and modifies the relation between plaque hemorrhage and cardiovascular outcome. Stroke. (2013) 44:3318–23. doi: 10.1161/STROKEAHA.113.002633
60. Vrijenhoek JEP, Haitjema S, de Borst GJ, de Vries J-PPM, Vaartjes I, Moll FL, et al. The impact of female sex on long-term survival of patients with severe atherosclerosis undergoing endarterectomy. Atherosclerosis. (2014) 237:521–7. doi: 10.1016/j.atherosclerosis.2014.10.010
61. Wang SS, Schadt EE, Wang H, Wang X, Ingram-Drake L, Shi W, et al. Identification of pathways for atherosclerosis in mice. Circ Res. (2007) 101:e11–30. doi: 10.1161/CIRCRESAHA.107.152975
62. Hartman RJG, Owsiany K, Ma L, Koplev S, Hao K, Slenders L, et al. Sex-stratified gene regulatory networks reveal female key driver genes of atherosclerosis involved in smooth muscle cell phenotype switching. Circulation. (2021) 143:713–26. doi: 10.1161/CIRCULATIONAHA.120.051231
63. Khan AW, Paneni F, Jandeleit-Dahm KAM. Cell-specific epigenetic changes in atherosclerosis. Clin Sci Lond Engl. (2021) 135:1165–87. doi: 10.1042/CS20201066
64. Espitia O, Chatelais M, Steenman M, Charrier C, Maurel B, Georges S, et al. Implication of molecular vascular smooth muscle cell heterogeneity among arterial beds in arterial calcification. PLoS ONE. (2018) 13:e0191976. doi: 10.1371/journal.pone.0191976
65. Kolodgie FD, Yahagi K, Mori H, Romero ME, Trout HH, Finn A V., et al. High-risk carotid plaque: lessons learned from histopathology. Semin Vasc Surg. (2017) 30:31–43. doi: 10.1053/j.semvascsurg.2017.04.008
66. Jashari F, Ibrahimi P, Nicoll R, Bajraktari G, Wester P, Henein MY. Coronary and carotid atherosclerosis: similarities and differences. Atherosclerosis. (2013) 227:193–200. doi: 10.1016/j.atherosclerosis.2012.11.008
67. Willemsen L, de Winther MPJ. Macrophage subsets in atherosclerosis as defined by single-cell technologies. J Pathol. (2020) 250:705–14. doi: 10.1002/path.5392
68. Kolodziejczyk AA, Kim JK, Svensson V, Marioni JC, Teichmann SA. The technology and biology of single-cell RNA sequencing. Mol Cell. (2015) 58:610–20. doi: 10.1016/j.molcel.2015.04.005
69. Haque A, Engel J, Teichmann SA, Lönnberg T. A practical guide to single-cell RNA-sequencing for biomedical research and clinical applications. Genome Med. (2017) 9:1–12. doi: 10.1186/s13073-017-0467-4
70. Phipson B, Zappia L, Oshlack A. Gene length and detection bias in single cell RNA sequencing protocols. F1000Research. (2017) 6:595. doi: 10.12688/f1000research.11290.1
71. Zappia L, Phipson B, Oshlack A. Exploring the single-cell RNA-seq analysis landscape with the scRNA-tools database. PLoS Comput Biol. (2018) 14:e1006245. doi: 10.1371/journal.pcbi.1006245
72. Longo SK, Guo MG, Ji AL, Khavari PA. Integrating single-cell and spatial transcriptomics to elucidate intercellular tissue dynamics. Nat Rev Genet. (2021) 22:627–44. doi: 10.1038/s41576-021-00370-8
73. Feig JE, Fisher EA. Laser capture microdissection for analysis of macrophage gene expression from atherosclerotic lesions. In: Freeman LA, redacteur, editors. Lipoproteins and Cardiovascular Disease: Methods and Protocols. Totowa, NJ: Humana Press (2013). p. 123–35. doi: 10.1007/978-1-60327-369-5_5
74. x Genomics. Spatial Transcriptomics. Beschikbaar op. Available online at: https://www.10xgenomics.com/spatial-transcriptomics (accessed November 2021).
75. Hao Y, Hao S, Andersen-Nissen E, Mauck WM, Zheng S, Butler A, et al. Integrated analysis of multimodal single-cell data. Cell. (2021) 184:3573–87.e29. doi: 10.1016/j.cell.2021.04.048
76. Libby P, Pasterkamp G, Crea F, Jang I-K. Reassessing the mechanisms of acute coronary syndromes: the “Vulnerable Plaque” and superficial erosion. Circ Res. (2019) 124:150–60. doi: 10.1161/CIRCRESAHA.118.311098
77. Quillard T, Franck G, Mawson T, Folco E, Libby P. Mechanisms of erosion of atherosclerotic plaques. Curr Opin Lipidol. (2017) 28:434–41. doi: 10.1097/MOL.0000000000000440
78. Chen P-Y, Schwartz MA, Simons M. Endothelial-to-mesenchymal transition, vascular inflammation, and atherosclerosis. Front Cardiovasc Med. (2020) 7:53. doi: 10.3389/fcvm.2020.00053
79. van Kuijk K, Kuppe C, Betsholtz C, Vanlandewijck M, Kramann R, Sluimer JC. Heterogeneity and plasticity in healthy and atherosclerotic vasculature explored by single-cell sequencing. Cardiovasc Res. (2019) 115:1705–15. doi: 10.1093/cvr/cvz185
80. Dobnikar L, Taylor AL, Chappell J, Oldach P, Harman JL, Oerton E, et al. Disease-relevant transcriptional signatures identified in individual smooth muscle cells from healthy mouse vessels. Nat Commun. (2018) 9:4567. doi: 10.1038/s41467-018-06891-x
81. Benditt EP, Benditt JM. Evidence for a monoclonal origin of human atherosclerotic plaques. Proc Natl Acad Sci USA. (1973) 70:1753–6. doi: 10.1073/pnas.70.6.1753
82. DiRenzo D, Owens GK, Leeper NJ. “Attack of the Clones”. Circ Res. (2017) 120:624–6. doi: 10.1161/CIRCRESAHA.116.310091
83. Fidler TP, Xue C, Yalcinkaya M, Hardaway B, Abramowicz S, Xiao T, et al. The AIM2 inflammasome exacerbates atherosclerosis in clonal haematopoiesis. Nature. (2021) 592:296–301. doi: 10.1038/s41586-021-03341-5
84. Wang Y, Nanda V, Direnzo D, Ye J, Xiao S, Kojima Y, et al. Clonally expanding smooth muscle cells promote atherosclerosis by escaping efferocytosis and activating the complement cascade. Proc Natl Acad Sci USA. (2020) 117:15818–26. doi: 10.1073/pnas.2006348117
85. Jacobsen K, Lund MB, Shim J, Gunnersen S, Füchtbauer E-M, Kjolby M, et al. Diverse cellular architecture of atherosclerotic plaque derives from clonal expansion of a few medial SMCs. JCI Insight. (2017) 2:e95890. doi: 10.1172/jci.insight.95890
86. Ludwig LS, Lareau CA, Ulirsch JC, Christian E, Muus C, Li LH, et al. Lineage tracing in humans enabled by mitochondrial mutations and single-cell genomics. Cell. (2019) 176:1325–39.e22. doi: 10.1016/j.cell.2019.01.022
87. Aragam KG, Jiang T, Goel A, Kanoni S, Wolford BN, Weeks EM, et al. Discovery and systematic characterization of risk variants and genes for coronary artery disease in over a million participants. medRXiv. (2021). doi: 10.1101/2021.05.24.21257377
88. Malik R, Chauhan G, Traylor M, Sargurupremraj M, Okada Y, Mishra A, et al. Multiancestry genome-wide association study of 520,000 subjects identifies 32 loci associated with stroke and stroke subtypes. Nat Genet. (2018) 50:524–37. doi: 10.1038/s41588-018-0058-3
89. Franceschini N, Giambartolomei C, de Vries PS, Finan C, Bis JC, Huntley RP, et al. GWAS and colocalization analyses implicate carotid intima-media thickness and carotid plaque loci in cardiovascular outcomes. Nat Commun. (2018) 9:5141. doi: 10.1038/s41467-018-07340-5
90. Erdmann J, Kessler T, Munoz Venegas L, Schunkert H. A decade of genome-wide association studies for coronary artery disease: the challenges ahead. Cardiovasc Res. (2018) 114:1241–57. doi: 10.1093/cvr/cvy084
91. Mountjoy E, Schmidt EM, Carmona M, Schwartzentruber J, Peat G, Miranda A, et al. An open approach to systematically prioritize causal variants and genes at all published human GWAS trait-associated loci. Nat Genet. (2021) 53:1527–33. doi: 10.1038/s41588-021-00945-5
92. Slenders L, Landsmeer LPL, Cui K, Depuydt MAC, Verwer M, Mekke J, et al. Intersecting single-cell transcriptomics and genome-wide association studies identifies crucial cell populations and candidate genes for atherosclerosis. Eur Heart J Open. (2021) oeab043. doi: 10.1093/ehjopen/oeab043
93. Petretto E. Single cell expression quantitative trait loci and complex traits. Genome Med. (2013) 5:72. doi: 10.1186/gm476
94. Wills QF, Livak KJ, Tipping AJ, Enver T, Goldson AJ, Sexton DW, et al. Single-cell gene expression analysis reveals genetic associations masked in whole-tissue experiments. Nat Biotechnol. (2013) 31:748–52. doi: 10.1038/nbt.2642
95. van der Wijst M, de Vries D, Groot H, Trynka G, Hon C, Bonder M, et al. The single-cell eQTLGen consortium. eLife. (2020) 9:e52155. doi: 10.7554/eLife.52155
Keywords: scRNA-sequencing, transcriptomics, -omics, single-cell, atherosclerosis
Citation: Slenders L, Tessels DE, van der Laan SW, Pasterkamp G and Mokry M (2022) The Applications of Single-Cell RNA Sequencing in Atherosclerotic Disease. Front. Cardiovasc. Med. 9:826103. doi: 10.3389/fcvm.2022.826103
Received: 30 November 2021; Accepted: 03 January 2022;
Published: 08 February 2022.
Edited by:
Holger Winkels, Universitätsklinikum Köln, GermanyReviewed by:
Judith Sluimer, Maastricht University, NetherlandsJesse W. Williams, University of Minnesota, United States
Copyright © 2022 Slenders, Tessels, van der Laan, Pasterkamp and Mokry. This is an open-access article distributed under the terms of the Creative Commons Attribution License (CC BY). The use, distribution or reproduction in other forums is permitted, provided the original author(s) and the copyright owner(s) are credited and that the original publication in this journal is cited, in accordance with accepted academic practice. No use, distribution or reproduction is permitted which does not comply with these terms.
*Correspondence: Gerard Pasterkamp, Zy5wYXN0ZXJrYW1wQHVtY3V0cmVjaHQubmw=; Michal Mokry, bS5tb2tyeUB1bWN1dHJlY2h0Lm5s
†These authors share last authorship