- 1Department of Spinal Surgery, Longyan First Affiliated Hospital of Fujian Medical University, Longyan, China
- 2Department of Pathology, Zhongshan Hospital Affiliated to Xiamen University, Xiamen, China
- 3Department of Cardiology, Shengli Clinical Medical College of Fujian Medical University, Fuzhou, Fujian, China
- 4Department of Cardiology, Longyan First Affiliated Hospital of Fujian Medical University, Longyan, China
- 5Department of Cardiology, The Second Affiliated Hospital, School of Medicine, Zhejiang University, Hangzhou, China
- 6State Key Laboratory of Transvascular Implantation Devices, Hangzhou, China
- 7Department of Intensive Care Unit, Longyan First Affiliated Hospital of Fujian Medical University, Longyan, China
Background: The identification of efficient predictors for short-term mortality among patients with myocardial infarction (MI) in coronary care units (CCU) remains a challenge. This study seeks to investigate the potential of machine learning (ML) to improve risk prediction and develop a predictive model specifically tailored for 30-day mortality in critical MI patients.
Method: This study focused on MI patients extracted from the Medical Information Mart for Intensive Care-IV database. The patient cohort was randomly stratified into derivation (n = 1,389, 70%) and validation (n = 595, 30%) groups. Independent risk factors were identified through eXtreme Gradient Boosting (XGBoost) and random decision forest (RDF) methodologies. Subsequently, multivariate logistic regression analysis was employed to construct predictive models. The discrimination, calibration and clinical utility were assessed utilizing metrics such as receiver operating characteristic (ROC) curve, calibration plot and decision curve analysis (DCA).
Result: A total of 1,984 patients were identified (mean [SD] age, 69.4 [13.0] years; 659 [33.2%] female). The predictive performance of the XGBoost and RDF-based models demonstrated similar efficacy. Subsequently, a 30-day mortality prediction algorithm was developed using the same selected variables, and a regression model was visually represented through a nomogram. In the validation group, the nomogram (Area Under the Curve [AUC]: 0.835, 95% Confidence Interval [CI]: [0.774–0.897]) exhibited superior discriminative capability for 30-day mortality compared to the Sequential Organ Failure Assessment (SOFA) score [AUC: 0.735, 95% CI: (0.662–0.809)]. The nomogram (Accuracy: 0.914) and the SOFA score (Accuracy: 0.913) demonstrated satisfactory calibration. DCA indicated that the nomogram outperformed the SOFA score, providing a net benefit in predicting mortality.
Conclusion: The ML-based predictive model demonstrated significant efficacy in forecasting 30-day mortality among MI patients admitted to the CCU. The prognostic factors identified were age, blood urea nitrogen, heart rate, pulse oximetry-derived oxygen saturation, bicarbonate, and metoprolol use. This model serves as a valuable decision-making tool for clinicians.
Introduction
Myocardial infarction (MI) represents a widespread and severe cardiovascular ailment, characterized by substantial global morbidity and mortality. In the coronary care unit (CCU), complications such as cardiogenic shock, cardiac rupture and in-hospital cardiac arrest contribute to elevated mortality among MI patients (1–3). Robust risk prediction models are imperative for acute cardiac conditions. Currently utilized intensive care unit (ICU) risk scores, such as the Acute Physiology and Chronic Health Evaluation and Sequential Organ Failure Assessment (SOFA), play a crucial role in stratifying mortality risk (4–6). These ICU risk scores exhibit commendable discrimination, reflecting their capacity to effectively distinguish between survivors and fatalities within unselected CCU cohorts. However, it is noteworthy that calibration has been consistently suboptimal (7–10). Admission diagnoses, particularly critical care conditions like cardiac arrest, respiratory failure and shock, can significantly influence the precision of risk prediction by ICU risk scores (11, 12). The impact of admission diagnoses on the performance of risk scores in CCU patients, especially those with common cardiac diagnoses such as MI, remains underexplored. Given the grim prognosis associated with MI, an investigation into the risk factors associated with mortality becomes imperative. Accurate prediction of short-term mortality in CCU-admitted patients with MI has the potential to enhance the management and prognosis of complications related to MI.
Incorporating newer analytical methods has the potential to enhance risk prediction beyond the scope of conventional statistical approaches using existing data. Machine learning (ML) is a promising avenue for improving accuracy in predicting short-term mortality post-MI (13–15). Predominant supervised ML algorithms, including decision trees, random decision forest (RDF) and gradient boosting (GB), each possess distinct characteristics. Despite the demonstrated efficacy of multiple ML methods in the field of medicine, it is noteworthy that only a limited number of constructed models have found practical implementation in clinical settings (16). Additionally, the comparative prognostic performance of ML in predicting outcomes for patients with MI admitted to the CCU remains unknown, especially with conventional critical scores.
This study endeavors to leverage a comprehensive dataset encompassing MI patients to explore the viability and precision of ML in predicting 30-day mortality. Additionally, the objective is to develop and validate a mortality prediction model specifically tailored for MI patients admitted to the CCU utilizing the Medical Information Mart for Intensive Care-IV (MIMIC-IV) database. The anticipated outcome of this research is to contribute valuable insights that could potentially enhance medical prognosis and facilitate informed decision-making in the context of CCU-admitted MI patients.
Method
Data sources
The data utilized in this study were sourced from the MIMIC-IV database (https://physionet.org/content/mimiciv/0.3/), a substantial and publicly accessible resource developed and managed by the Massachusetts Institute of Technology (MIT) Computational Physiology Laboratory. Spanning the years 2008 to 2019, this database aggregates patient information derived from hourly physiological readings recorded by bedside monitors and cross-validated by ICU nurses. It encompasses a diverse and extensive cohort of ICU patients, providing comprehensive data on demographic characteristics, medical history, laboratory test and medications. Access to the database was granted upon the successful completion of the National Institutes of Health's online course, “Protecting Human Research Participants,” designed to ensure ethical research conduct involving human subjects. Specifically, individuals who passed the Collaborative Institutional Training Initiative examination, including author Lu (Certification number 10624278), were authorized to access the database. The project obtained approval from the institutional review boards of both MIT and Beth Israel Deaconess Medical Center (BIDMC), securing a waiver of informed consent for the study.
Study population
The MIMIC-IV database includes comprehensive and high-quality data of critically ill patients admitted to the ICU at the Beth Israel Deaconess Medical Center between 2008 and 2019. The selected population must be adults (age ≥ 18 years) diagnosed with MI, verified by manual review of ICD-9/10 codes, and with a first-time CCU admission. ICD-9 and -10 codes were documented for specific disease diagnosis in the MIMIC-IV database. We used the following ICD codes to define and extract MI patients: ICD-9: 410.0 (410.00, 410.01, and 410.02), 410.1 (410.10, 410.11, and 410.12), 410.2 (410.20, 410.21, and 410.22), 410.3 (410.30, 410.31, and 410.32), 410.4 (410.40, 410.41, and 410.42), 410.5 (410.50, 410.51, and 410.52), 410.6 (410.60, 410.61, and 410.62), 410.7 (410.70, 410.71, and 410.72), 410.8 (410.80, 410.81, and 410.82), and 410.9 (410.90, 410.91, and 410.92), and ICD-10: I21.0 (I21.01, I21.02, and I21.09), I21.1 (I21.11 and I21.19), I21.2 (I21.21 and I21.29), I21.3, and I21.4. This yielded 4,139 patients with MI from the first admission, which were then merged based on their hospital admission number. Finally, 1,984 critically ill MI patients were included. The patient selection process, outlined in Figure 1A, delineates the sequential steps leading to the final cohort of 1,984 patients extracted from the MIMIC-IV database. Rigorous measures were undertaken to preserve patient privacy, necessitating the removal of all personally identifiable information from the analytical dataset.
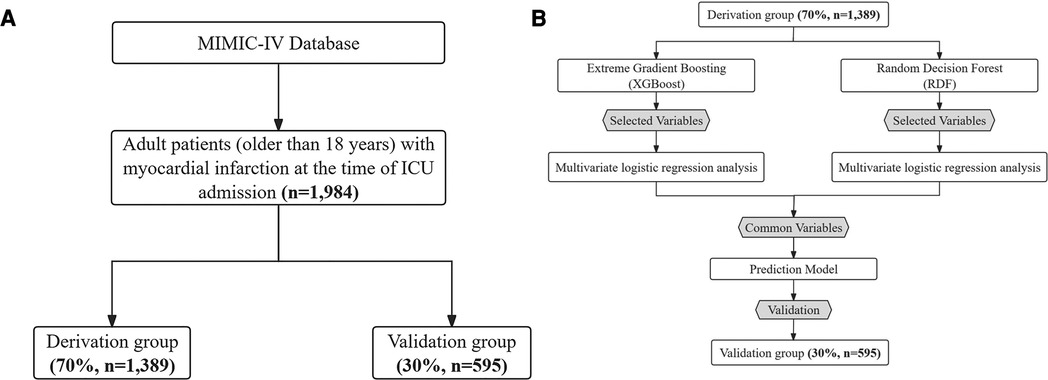
Figure 1. (A) Patient flow diagram. MIMIC-IV, medical information mart for intensive care-IV; ICU, intensive care unit. (B) Model development flowchart.
Data extraction
We collected the following data, considering their clinical relevance and overall availability when patients were admitted: Demographic characteristics consisted of age, gender, race, systolic blood pressure (SBP), diastolic blood pressure (DBP), mean blood pressure (MBP), heart rate, temperature, pulse oximetry-derived oxygen saturation (SpO2) and glucose. Medical history comprised congestive heart failure (CHF), peripheral vascular disease (PVD), cerebrovascular disease (CVD), chronic obstructive pulmonary disease (COPD), diabetes mellitus (DM), renal disease, coronary artery bypass grafting (CABG), percutaneous coronary Intervention (PCI) and continuous renal replacement therapy (CRRT). Laboratory tests included blood urea nitrogen (BUN), hematocrit, hemoglobin, platelet, white blood cell count (WBC), red blood cell count (RBC), mean corpuscular hemoglobin (MCH), mean corpuscular hemoglobin concentration (MCHC), mean corpuscular volume (MCV), red blood cell distribution width (RDW), neutrophils, lymphocytes, international normalized ratio (INR), prothrombin time (PT), bicarbonate, potassium, calcium, sodium, chloride, anion gap and creatinine (17). Medications were also collected, including aspirin, clopidogrel, statin, metoprolol and vasoactive agents. Laboratory parameters were systematically collected within the initial 24 h of admission to the CCU, adhering to the procedures outlined by Jin Lu et al. in 2022 (18). The documentation of specific diseases using ICD-9/10 codes was carried out by hospital staff during patients’ discharge, and this information was extracted from the database through the utilization of Structure Query Language (SQL).
Outcomes and definitions
The primary outcome of the study was all-cause mortality within 30 days of the date of admission. Monitoring of this endpoint event will involve extracting the survival status within 30 days post-admission for ICU patients from the MIMIC-IV database.
Missing data handling
The MIMIC-IV database frequently contains variables with missing data. However, excluding patients with incomplete data introduces inherent bias into the study. Therefore, imputation becomes a critical step in data pre-processing. Variables with missing values surpassing 30% were excluded from the analysis. For the remaining missing data, imputation was performed using the mice package with random forests in R, a method known as Multivariate Imputation by Chained Equations (MICE) in R.
Model development
A comprehensive set of 45 demographic, clinical, biochemical variables and medications were identified as potential predictors through a thorough review of current literature, expert knowledge and consideration of their applicability in clinical practice. The Extreme Gradient Boosting (XGBoost) and RDF algorithms were employed to discern the contributions of each predictor, facilitating the selection of the most relevant variables (19). From the results, the top 15 variables were chosen for subsequent analysis. To identify independent risk factors associated with 30-day mortality, univariate logistic regression analysis was conducted to assess the significance of variables selected by each method in the derivation group. Variables demonstrating a significant association with 30-day mortality were considered potential candidates for inclusion in the multivariate logistic regression model, as depicted in Figure 1B.
SOFA score
The SOFA score, in existence for over 25 years, was devised as a succinct method for assessing and monitoring organ dysfunction in critically ill patients. Swiftly gaining prominence, the SOFA score has become a cornerstone in adult intensive care settings, finding widespread use in both clinical applications and research endeavors (20, 21).
Displaying risk assessment using nomogram
The predictive model is designed to provide estimates of the probability or risk associated with the prospective occurrence of a specific outcome or event in individuals who are at risk of experiencing such an event. Within this framework, a nomogram serves as a visual and intuitive tool for calculating risk scores across various outcomes. By presenting a tangible representation, nomograms play a pivotal role in guiding the selection of appropriate interventions or treatments tailored to an individual's risk profile.
Discrimination assessment
The dataset underwent random allocation into derivation (n = 1,389, 70%) and validation (n = 595, 30%) groups. Receiver Operating Characteristic (ROC) curve analysis was employed as a metric for optimizing model parameters. Discrimination performance was evaluated through the calculation of the Area Under the ROC Curve (AUC), a metric that ranges from 0.5 to 1.0. Higher AUC values signify an enhanced discriminatory ability of the model.
Calibration assessment
Calibration characterizes the concordance between the anticipated and observed probabilities of 30-day mortality. To gauge the model's goodness-of-fit, the Hosmer-Lemeshow chi-square test was employed. This statistical test assesses the adequacy of fit between the observed and predicted outcomes from the model.
Decision curve analysis
Decision Curve Analysis (DCA) (22) was employed to assess and compare the clinical net benefits associated with the models. The performance of the model was comprehensively evaluated by computing various metrics, encompassing AUC, sensitivity, specificity, positive predictive value, negative predictive value, positive likelihood ratio, negative likelihood ratio and accuracy. These metrics collectively offer insights into the model's discriminative ability, diagnostic accuracy and predictive value.
Statistical analysis
To evaluate the normality of continuous variables, a normality test was executed. Continuous variables exhibiting a normal distribution were expressed as mean ± standard deviation, while those with a non-normal distribution were represented as median [interquartile range (IQR)]. Categorical data were presented as numbers (percent). Group comparisons for normally distributed continuous data employed Student's t-test, whereas the Kruskal–Wallis test was utilized for non-normally distributed data. Categorical data were compared using either χ2 or Fisher's exact test, facilitated by the tableone package in R 4.3.1. The risk score for each predictor was computed based on the beta (β) coefficient of the re-evaluated model (23). A two-tailed p-value of <0.05 indicated statistical significance in all analyses. The entire analysis was conducted using R software (V.4.3.1; https://www. R-project.org).
Result
Patient characteristics
Table 1 presents a comprehensive comparison of demographics and variables between deceased and surviving patients during hospitalization. The mean age of the cohort was 69.4 ± 13.0 years, with females constituting 33.2% (659 individuals). Deceased patients exhibited advanced age, a higher proportion of females, elevated heart rates and increased glucose levels in comparison to survivors. Furthermore, individuals who succumbed to their condition demonstrated a higher incidence of complications, including CHF and renal disease, while being less likely to undergo CABG and PCI in contrast to their surviving counterparts. Additionally, non-surviving patients exhibited lower lymphocyte counts and bicarbonate levels. Conversely, BUN, platelet count, WBC, MCV, neutrophil percentage, potassium levels and the proportion of vasoactive agent use were significantly higher among non-survivors. Factors such as SBP, MBP, temperature, SpO2, calcium levels and the proportion of metoprolol use were lower in deceased patients. Notably, there was no statistically significant difference in hematocrit levels between the survival and non-survival groups.
Selected variables
Utilizing XGBoost and RDF algorithms, the set of 45 selected variables was employed to discern patients at risk of mortality within the derivation group. The relative importance of the top 15 variables utilized in the XGBoost model is depicted in Figure 2A, while Figure 2B illustrates the predictors chosen by RDF.
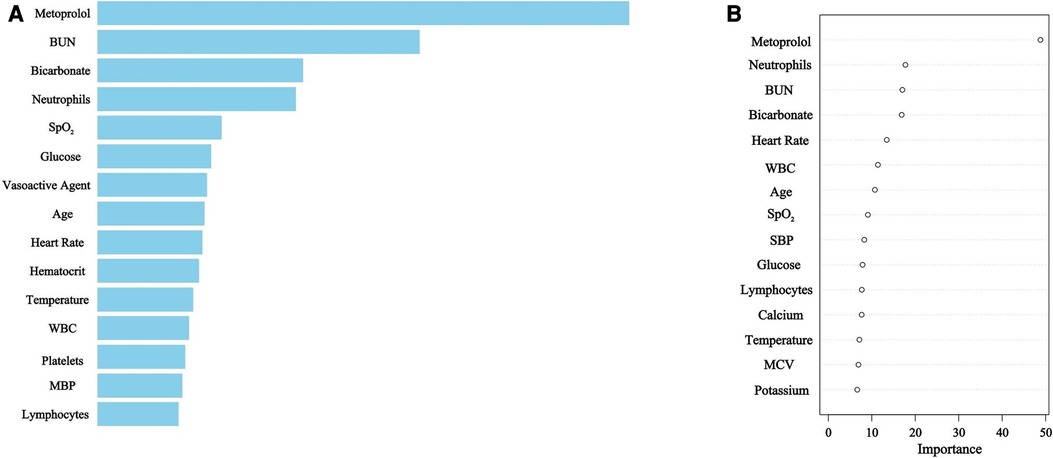
Figure 2. (A) XGBoost selected predictors. BUN, blood urea nitrogen; SpO2, pulse oximetry-derived oxygen saturation; WBC, white blood cell count; MBP, mean blood pressure. (B) Random Decision Forest selected predictors. BUN, blood urea nitrogen; WBC, white blood cell count; SpO2, pulse oximetry-derived oxygen saturation; SBP, systolic blood pressure; MCV, mean corpuscular volume.
Model development
For the derivation group, Tables 2, 3 present the variables selected by XGBoost and RDF, respectively, that exhibited a significant association with 30-day mortality in the univariate analysis. Variables demonstrating statistical significance in the multivariate logistic analysis were incorporated for model construction. Both the XGBoost and RDF models, exhibiting similar predictive performances (Supplementary Material) and variable counts, identified the same six variables: age, BUN, heart rate, SpO2, bicarbonate and metoprolol, as illustrated in Figure 3. Subsequently, a 30-day mortality prediction algorithm (PA) was formulated employing the selected variables as follows: log odds of mortality = 5.69633−2.03479 × Metoprolol−0.07936 × SpO2 + 0.01442 × BUN + 0.02831 × Age—0.10985 × Bicarbonate + 0.01688 × Heart rate.
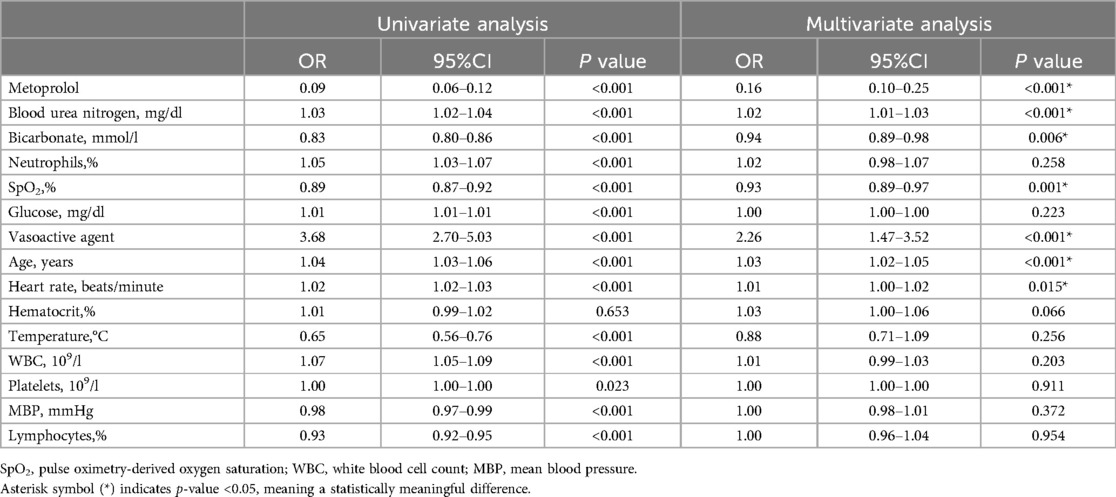
Table 2. Univariate and multivariate logistic regression analysis variables screened by extreme gradient boosting in the derivation group.
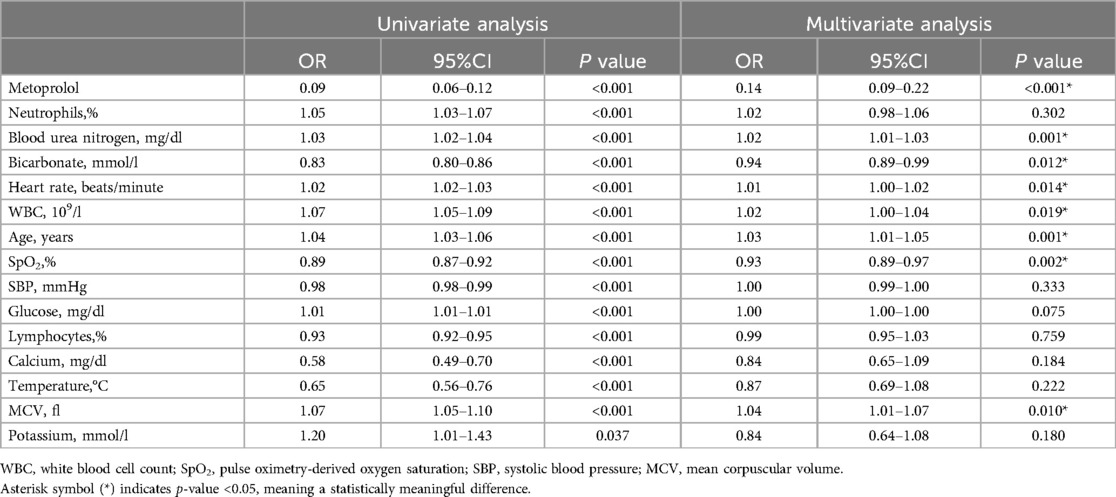
Table 3. Univariate and multivariate logistic regression analysis variables screened by random decision forest in the derivation group.
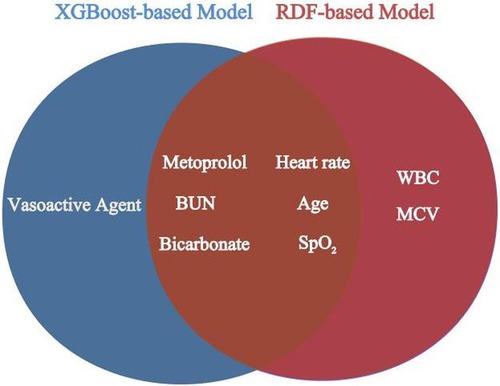
Figure 3. Overlapping variables of the XGBoost-based and RDF-based model were obtained in veen analysis. BUN, blood urea nitrogen; SpO2, pulse oximetry-derived oxygen saturation; WBC, white blood cell count; MCV, mean corpuscular volume.
Model building
Given the comparable predictive performance and the identical number of variables in both the XGBoost and RDF models, the final predictive model was constructed using the same set of six variables. This model is visually represented by the nomogram in Figure 4.
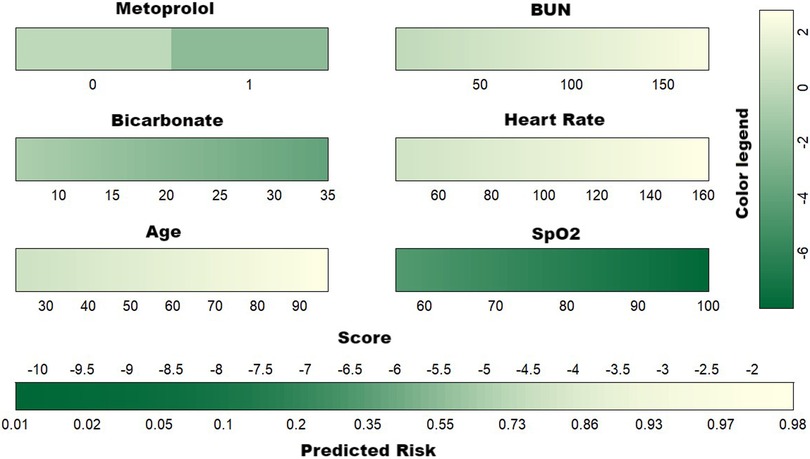
Figure 4. Developed nomogram for the risk of 30-day mortality. BUN, blood urea nitrogen; SpO2, pulse oximetry-derived oxygen saturation.
Model comparison
The predictive efficacy of the nomogram and SOFA score models was evaluated by comparing the ROC curves of the two models (Figure 5). The calculated area under the ROC curve values for the nomogram and the SOFA score model were 0.835 (95%CI 0.774–0.897) and 0.735 (95%CI: 0.662–0.809) in the validation group, respectively. Figure 6 illustrates the calibration of both models in the derivation and validation groups, indicating robust concordance performance in both cohorts. The DCA for the nomogram and SOFA score is presented in Figure 7. Both models demonstrated a higher net benefit than either the “treat all” or “treat none” strategy, with the nomogram consistently improving the net benefit for predicting 30-day mortality compared to the SOFA score. The predictive performance of the nomogram was significantly superior to that of the SOFA risk score (p < 0.05), as summarized in Table 4.
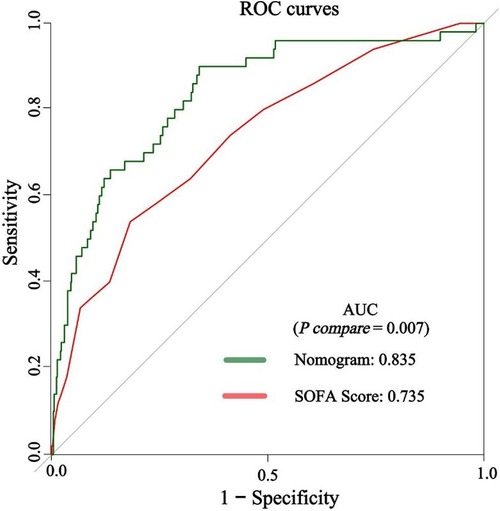
Figure 5. The discrimination performance of the nomogram and SOFA score in the validation group. ROC, receiver operating characteristic; AUC, area under the curve; SOFA, sequential organ failure assessment.
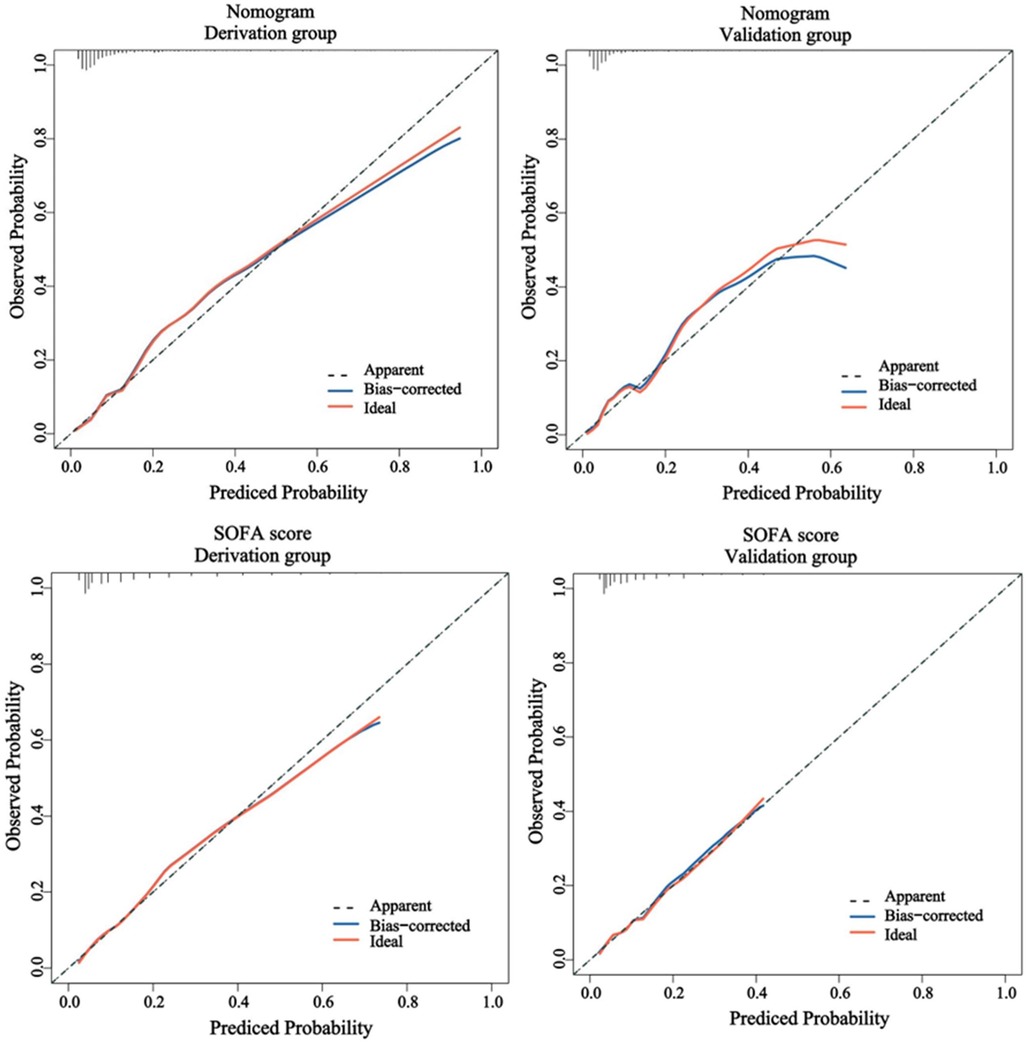
Figure 6. The calibration performance of the nomogram and SOFA score in the derivation and validation group. SOFA, sequential organ failure assessment.
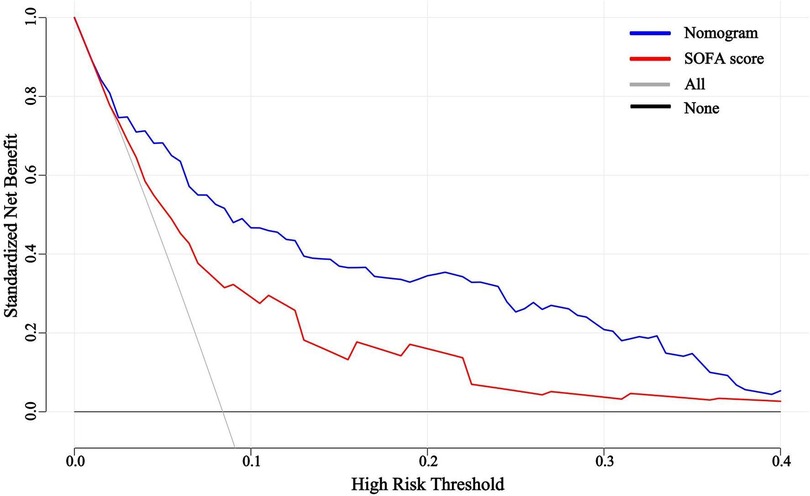
Figure 7. Decision curve analysis for the nomogram and SOFA score in the validation group. SOFA, sequential organ failure assessment.
Discussion
By leveraging data extracted from the MIMIC-IV database, we applied ML techniques, specifically XGBoost and RDF algorithms, to discern independent risk factors among critically ill patients with MI. Additionally, we pioneered the development of a 30-day mortality prediction model, aiming to offer valuable insights for clinical decision-making in advanced management strategies.
In recent years, ML has garnered widespread attention for its applications in risk prediction and disease screening, exhibiting remarkable performance. Lee et al. successfully developed an ML model for predicting 1-year mortality, showcasing excellent discriminatory ability, superior performance and good calibration (24). Oliveira et al. demonstrated the valuable role of ML methods in clinical decision-making for MI patients (14). Moreover, existing survival scoring systems following ICU admission, such as the SOFA score, have demonstrated limited efficacy, particularly in the context of MI admission. In a real-world cohort of patients diagnosed with cardiogenic shock, prevailing risk scores exhibited modest prognostic accuracy without clear indications of superiority. Further exploration is imperative to enhance the discriminative capacities of existing models or develop novel methodologies (25). Hence, this study leveraged XGBoost and RDF methodologies to uncover the intricate relationship between poor prognosis and clinical variables. Beyond conventional risk factors, XGBoost and RDF ML methods identified several novel factors, highlighting ML's advantage in enhancing classification accuracy and identification efficiency.
The most prominent feature identified by ML methods was metoprolol. Our study reveals a correlation between metoprolol administration and the prognosis of critical MI patients. Specifically, in patients with ST-elevation myocardial infarction (STEMI) undergoing primary PCI, early intravenous metoprolol administration before reperfusion has been associated with higher long-term Left Ventricular Ejection Fractions (LVEF), reduced incidence of severe left ventricular (LV) systolic dysfunction, fewer indications for implantable cardioverter defibrillator (ICD), and decreased admissions for HF (26). Early-blocker therapy has been widely recommended as an integral component of emergency treatment for suspected MI (27, 28). However, ongoing ambiguity regarding the appropriate use of intravenous-blocker therapy has persisted for several years (29–31). Our model illustrates that metoprolol significantly contributes to predicting the 30-day mortality risk in patients with critical MI.
In alignment with previous studies, our research identified the acid–base balance as a robust prognostic predictor. Zhu et al. demonstrated that the L/A ratio, bicarbonate concentration and hemoglobin level possess predictive value for 30-day mortality in patients with MI (32). Furthermore, lower serum bicarbonate levels upon admission were found to independently predict mortality in a substantial cohort of consecutive patients with cardiogenic shock hospitalized in the CCU. With the availability of point-of-care blood gas and electrolyte analyzers in the CCU, we propose that baseline serum bicarbonate levels could serve as an additional biomarker for risk identification and stratification in critical MI patients.
BUN levels also exerted a significant impact on the total point score in our model, with elevated BUN levels associated with increased 30-day mortality. The present findings align with previous studies, suggesting that a higher BUN/Cr ratio is linked to an increased risk of in-hospital mortality in patients with non-STEMI (33). Moreover, elevated levels of BUN and BUN/creatinine ratio upon admission have been identified as independent predictors of long-term mortality in patients diagnosed with STEMI (34). These consistent findings underscore the relevance of incorporating BUN levels into prognostic models for predicting the outcomes of patients with MI.
In addition to metoprolol, bicarbonate and BUN, other variables such as age and SpO2 hold relative importance in stratifying a patient's risk. Age is universally acknowledged as a critical risk factor for poor prognosis in CAD, and the mortality risk in MI patients escalates with advancing age (35). Moreover, with increasing age, bodily functions deteriorate, and physiological compensatory functions diminish, all of which contribute to an elevated risk of MI events (36, 37). Meanwhile, low-normal baseline oxygen saturation or the onset of hypoxemia has been identified as an independent indicator of poor prognosis (38). For patients experiencing hypoxia, personalized oxygen treatment guided by dynamic oxygen saturation is recommended.
Limitation
Nevertheless, this study has several limitations. Firstly, the decision to admit patients to a CCU may be influenced by various factors, including practitioner judgment, institutional policies and hospital capacity, potentially introducing bias to our prediction scores. In addition, certain variables such as insurance status, marital status, troponins and natriuretic peptides were not included in the study, which may have impacted the accuracy of the results. We fully recognize the critical role of biomarkers such as troponins and natriuretic peptides in the diagnosis and treatment of myocardial infarction, and we acknowledge their absence as a significant limitation of our study. This also reflects the constraints of the database utilized, where these markers were missing at a rate exceeding 30%, precluding further in-depth analysis. Secondly, the retrospective nature of this research may introduce selective bias, and we rigorously included eligible patients according to predefined criteria to mitigate this potential bias. Thirdly, being a single-center study, the sample size was relatively modest. While the robustness of our risk model was rigorously tested through internal validation using bootstrap testing, the generalizability of these study results to other populations remains uncertain. Lastly, our model may primarily serve to promptly recognize critical clinical situations at the bedside and might not offer additional insights into potential life-threatening pathophysiological mechanisms.
Conclusion
We developed the 30-day mortality prediction model for CCU-admitted MI patients. This tool incorporates a concise set of routinely collected variables, facilitating ease of use at the bedside. Moreover, it can be seamlessly integrated into CCU monitoring technology, enabling automated notifications to CCU staff at various stages of the illness.
Data availability statement
The datasets presented in this study can be found in online repositories. The names of the repository/repositories and accession number(s) can be found below: MIMIC-IV database (https://physionet.org/content/mimiciv/0.3/) Certification number 10624278.
Ethics statement
The studies involving humans were approved by institutional review boards of both MIT and Beth Israel Deaconess Medical Center (BIDMC). The studies were conducted in accordance with the local legislation and institutional requirements. The participants provided their written informed consent to participate in this study.
Author contributions
XL: Conceptualization, Data curation, Formal Analysis, Funding acquisition, Writing – original draft, Writing – review & editing. XP: Formal Analysis, Validation, Writing – review & editing. YY: Investigation, Methodology, Project administration, Writing – original draft, Writing – review & editing. WY: Data curation, Funding acquisition, Investigation, Validation, Writing – original draft. XW: Conceptualization, Data curation, Formal Analysis, Funding acquisition, Writing – review & editing. KZ: Conceptualization, Methodology, Project administration, Resources, Writing – original draft. YW: Data curation, Investigation, Project administration, Resources, Writing – review & editing. JX: Conceptualization, Formal Analysis, Methodology, Writing – original draft. PY: Software, Supervision, Visualization, Writing – review & editing. JL: Conceptualization, Formal Analysis, Supervision, Visualization, Writing – original draft, Writing – review & editing. YZ: Conceptualization, Methodology, Validation, Visualization, Writing – original draft. HL: Data curation, Project administration, Resources, Writing – original draft.
Funding
The author(s) declare financial support was received for the research, authorship, and/or publication of this article. This research was funded and supported by Project of Scientific Research and Development Fund of Fujian Medical University (2022QH1491). The funders had no role in the study design, data collection and analysis, decision to publish, or preparation of the manuscript; the work was not funded by any industry sponsors.
Conflict of interest
The authors declare that the research was conducted in the absence of any commercial or financial relationships that could be construed as a potential conflict of interest.
Publisher's note
All claims expressed in this article are solely those of the authors and do not necessarily represent those of their affiliated organizations, or those of the publisher, the editors and the reviewers. Any product that may be evaluated in this article, or claim that may be made by its manufacturer, is not guaranteed or endorsed by the publisher.
Supplementary material
The Supplementary Material for this article can be found online at: https://www.frontiersin.org/articles/10.3389/fcvm.2024.1368022/full#supplementary-material
References
1. Thang ND, Karlson BW, Karlsson T, Herlitz J. Characteristics of and outcomes for elderly patients with acute myocardial infarction: differences between females and males. Clin Interv Aging. (2016) 11:1309–16. doi: 10.2147/CIA.S110034
2. Yamamoto T, Yoshida N, Takayama M. Temporal trends in acute myocardial infarction incidence and mortality between 2006 and 2016 in Tokyo—report from the Tokyo CCU network. Circ J. (2019) 83(6):1405–9. doi: 10.1253/circj.CJ-19-0187
3. Higuchi S, Suzuki M, Horiuchi Y, Tanaka H, Saji M, Yoshino H, et al. Higher non-cardiac mortality and lesser impact of early revascularization in patients with type 2 compared to type 1 acute myocardial infarction: results from the Tokyo CCU network registry. Heart Vessels. (2019) 34(7):1140–7. doi: 10.1007/s00380-019-01350-z
4. Knaus WA, Wagner DP, Draper EA, Zimmerman JE, Bergner M, Bastos PG, et al. The APACHE III prognostic system. Risk prediction of hospital mortality for critically ill hospitalized adults. Chest. (1991) 100(6):1619–36. doi: 10.1378/chest.100.6.1619
5. Vincent JL, Moreno R, Takala J, Willatts S, De Mendonça A, Bruining H, et al. The SOFA (sepsis-related organ failure assessment) score to describe organ dysfunction/failure. On behalf of the working group on sepsis-related problems of the European Society of Intensive Care Medicine. Intensive Care Med. (1996) 22(7):707–10. doi: 10.1007/BF01709751
6. Zimmerman JE, Kramer AA, McNair DS, Malila FM. Acute physiology and chronic health evaluation (APACHE) IV: hospital mortality assessment for today’s critically ill patients. Crit Care Med. (2006) 34(5):1297–310. doi: 10.1097/01.CCM.0000215112.84523.F0
7. Jentzer JC, Bennett C, Wiley BM, Murphree DH, Keegan MT, Gajic O, et al. Predictive value of the sequential organ failure assessment score for mortality in a contemporary cardiac intensive care unit population. J Am Heart Assoc. (2018) 7(6):e008169. doi: 10.1161/JAHA.117.008169
8. Jentzer JC, Murphree DH, Wiley B, Bennett C, Goldfarb M, Keegan MT, et al. Comparison of mortality risk prediction among patients ≥70 versus <70 years of age in a cardiac intensive care unit. Am J Cardiol. (2018) 122(10):1773–8. doi: 10.1016/j.amjcard.2018.08.011
9. Bennett CE, Wright RS, Jentzer J, Gajic O, Murphree DH, Murphy JG, et al. Severity of illness assessment with application of the APACHE IV predicted mortality and outcome trends analysis in an academic cardiac intensive care unit. J Crit Care. (2019) 50:242–6. doi: 10.1016/j.jcrc.2018.12.012
10. Argyriou G, Vrettou CS, Filippatos G, Sainis G, Nanas S, Routsi C. Comparative evaluation of acute physiology and chronic health evaluation II and sequential organ failure assessment scoring systems in patients admitted to the cardiac intensive care unit. J Crit Care. (2015) 30(4):752–7. doi: 10.1016/j.jcrc.2015.04.014
11. Chandra S, Kashyap R, Trillo-Alvarez CA, Tsapenko M, Yilmaz M, Hanson AC, et al. Mapping physicians’ admission diagnoses to structured concepts towards fully automatic calculation of acute physiology and chronic health evaluation score. BMJ Open. (2011) 1(2):e000216. doi: 10.1136/bmjopen-2011-000216
12. Holland EM, Moss TJ. Acute noncardiovascular illness in the cardiac intensive care unit. J Am Coll Cardiol. (2017) 69(16):1999–2007. doi: 10.1016/j.jacc.2017.02.033
13. Deng L, Zhao X, Su X, Zhou M, Huang D, Zeng X. Machine learning to predict no reflow and in-hospital mortality in patients with ST-segment elevation myocardial infarction that underwent primary percutaneous coronary intervention. BMC Med Inform Decis Mak. (2022) 22(1):109. doi: 10.1186/s12911-022-01853-2
14. Oliveira M, Seringa J, Pinto FJ, Henriques R, Magalhães T. Machine learning prediction of mortality in acute myocardial infarction. BMC Med Inform Decis Mak. (2023) 23(1):70. doi: 10.1186/s12911-023-02168-6
15. Xi H, Langerman J, Sabri S, Chien P, Young CS, Younesi S, et al. A human skeletal muscle atlas identifies the trajectories of stem and progenitor cells across development and from human pluripotent stem cells. Cell Stem Cell. (2020) 27(1):181–5. doi: 10.1016/j.stem.2020.06.006
16. Deo RC. Machine learning in medicine. Circulation. (2015) 132(20):1920–30. doi: 10.1161/CIRCULATIONAHA.115.001593
17. Vincent J-L, Moreno R. Clinical review: scoring systems in the critically ill. Crit Care. (2010) 14(2):207. doi: 10.1186/cc8204
18. Lu J, Huang Z, Wang J, Zhao X, Yang Y, Wu B, et al. Prevalence and prognostic impact of malnutrition in critical patients with acute myocardial infarction: results from Chinese CIN cohort and American MIMIC-III database. Front Nutr. (2022) 9:890199. doi: 10.3389/fnut.2022.890199
19. Plagnol V, Curtis J, Epstein M, Mok KY, Stebbings E, Grigoriadou S, et al. A robust model for read count data in exome sequencing experiments and implications for copy number variant calling. Bioinformatics. (2012) 28(21):2747–54. doi: 10.1093/bioinformatics/bts526
20. Khanna A, English SW, Wang XS, Ham K, Tumlin J, Szerlip H, et al. Angiotensin II for the treatment of vasodilatory shock. N Engl J Med. (2017) 377(5):419–30. doi: 10.1056/NEJMoa1704154
21. van Zanten ARH, Sztark F, Kaisers UX, Zielmann S, Felbinger TW, Sablotzki AR, et al. High-protein enteral nutrition enriched with immune-modulating nutrients vs standard high-protein enteral nutrition and nosocomial infections in the ICU: a randomized clinical trial. JAMA. (2014) 312(5):514–24. doi: 10.1001/jama.2014.7698
22. Vickers AJ, Elkin EB. Decision curve analysis: a novel method for evaluating prediction models. Med Decis Making. (2006) 26(6):565–74. doi: 10.1177/0272989X06295361
23. Sullivan LM, Massaro JM, D'Agostino RB. Presentation of multivariate data for clinical use: the framingham study risk score functions. Stat Med. (2004) 23(10):1631–60. doi: 10.1002/sim.1742
24. Lee HC, Park JS, Choe JC, Ahn JH, Lee HW, Oh J-H, et al. Prediction of 1-year mortality from acute myocardial infarction using machine learning. Am J Cardiol. (2020) 133:23–31. doi: 10.1016/j.amjcard.2020.07.048
25. Miller RJH, Southern D, Wilton SB, James MT, Har B, Schnell G, et al. Comparative prognostic accuracy of risk prediction models for cardiogenic shock. J Intensive Care Med. (2020) 35(12):1513–9. doi: 10.1177/0885066619878125
26. Pizarro G, Fernández-Friera L, Fuster V, Fernández-Jiménez R, García-Ruiz JM, García-Álvarez A, et al. Long-term benefit of early pre-reperfusion metoprolol administration in patients with acute myocardial infarction: results from the METOCARD-CNIC trial (effect of metoprolol in cardioprotection during an acute myocardial infarction). J Am Coll Cardiol. (2014) 63(22):2356–62. doi: 10.1016/j.jacc.2014.03.014
27. Smith SC, Blair SN, Bonow RO, Brass LM, Cerqueira MD, Dracup K, et al. AHA/ACC guidelines for preventing heart attack and death in patients with atherosclerotic cardiovascular disease: 2001 update. A statement for healthcare professionals from the American Heart Association and the American College of Cardiology. J Am Coll Cardiol. (2001) 38(5):1581–3. doi: 10.1016/S0735-1097(01)01682-5
28. De Backer G, Ambrosioni E, Borch-Johnsen K, Brotons C, Cifkova R, Dallongeville J, et al. European Guidelines on cardiovascular disease prevention in clinical practice. Third joint task force of European and other societies on cardiovascular disease prevention in clinical practice. Eur Heart J. (2003) 24(17):1601–10. doi: 10.1016/S0195-668X(03)00347-6
29. Pfisterer M, Cox JL, Granger CB, Brener SJ, Naylor CD, Califf RM, et al. Atenolol use and clinical outcomes after thrombolysis for acute myocardial infarction: the GUSTO-I experience. Global utilization of streptokinase and TPA (alteplase) for occluded coronary arteries. J Am Coll Cardiol. (1998) 32(3):634–40. doi: 10.1016/S0735-1097(98)00279-4
30. Gurwitz JH, Goldberg RJ, Chen Z, Gore JM, Alpert JS. Beta-blocker therapy in acute myocardial infarction: evidence for underutilization in the elderly. Am J Med. (1992) 93(6):605–10. doi: 10.1016/0002-9343(92)90192-E
31. Rogers WJ, Canto JG, Lambrew CT, Tiefenbrunn AJ, Kinkaid B, Shoultz DA, et al. Temporal trends in the treatment of over 1.5 million patients with myocardial infarction in the US from 1990 through 1999: the national registry of myocardial infarction 1, 2 and 3. J Am Coll Cardiol. (2000) 36(7):2056–63. doi: 10.1016/S0735-1097(00)00996-7
32. Zhu J-L, Liu H, Wang L-L, Lu X-H, Yin H-Y, Lyu J, et al. Association of lactate to albumin ratio and bicarbonate with short-term mortality risk in patients with acute myocardial infarction. BMC Cardiovasc Disord. (2022) 22(1):490. doi: 10.1186/s12872-022-02902-4
33. Huang S, Guo N, Duan X, Zhou Q, Zhang Z, Luo L, et al. Association between the blood urea nitrogen to creatinine ratio and in-hospital mortality among patients with acute myocardial infarction: a retrospective cohort study. Exp Ther Med. (2023) 25(1):36. doi: 10.3892/etm.2022.11735
34. Aronson D, Hammerman H, Beyar R, Yalonetsky S, Kapeliovich M, Markiewicz W, et al. Serum blood urea nitrogen and long-term mortality in acute ST-elevation myocardial infarction. Int J Cardiol. (2008) 127(3):380–5. doi: 10.1016/j.ijcard.2007.05.013
35. Chen Y, Chen S, Han Y, Xu Q, Zhao X. Neutrophil-to-Lymphocyte ratio and platelet-to-lymphocyte ratio are important indicators for predicting in-hospital death in elderly AMI patients. J Inflamm Res. (2023) 16:2051–61. doi: 10.2147/JIR.S411086
36. McHugh D, Gil J. Senescence and aging: causes, consequences, and therapeutic avenues. J Cell Biol. (2018) 217(1):65–77. doi: 10.1083/jcb.201708092
37. Meng C, Zhang J, Ye X, Guo F, Zou Q. Review and comparative analysis of machine learning-based phage virion protein identification methods. Biochim Biophys Acta Proteins Proteom. (2020) 1868(6):140406. doi: 10.1016/j.bbapap.2020.140406
Keywords: machine learning, 30-day mortality, myocardial infarction, MIMIC-IV, coronary care unit
Citation: Lin X, Pan X, Yang Y, Yang W, Wang X, Zou K, Wang Y, Xiu J, Yu P, Lu J, Zhao Y and Lu H (2024) Machine learning models to predict 30-day mortality for critical patients with myocardial infarction: a retrospective analysis from MIMIC-IV database. Front. Cardiovasc. Med. 11:1368022. doi: 10.3389/fcvm.2024.1368022
Received: 9 January 2024; Accepted: 9 September 2024;
Published: 20 September 2024.
Edited by:
Sascha Treskatsch, Charité University Medicine Berlin, GermanyReviewed by:
Lan Shen, Shanghai Jiao Tong University, ChinaBojan Staneticc, University Clinical Center of Republika Srpska, Bosnia and Herzegovina
Natallia Maroz-Vadalazhskaya, Belarusian State Medical University, Belarus
Copyright: © 2024 Lin, Pan, Yang, Yang, Wang, Zou, Wang, Xiu, Yu, Lu, Zhao and Lu. This is an open-access article distributed under the terms of the Creative Commons Attribution License (CC BY). The use, distribution or reproduction in other forums is permitted, provided the original author(s) and the copyright owner(s) are credited and that the original publication in this journal is cited, in accordance with accepted academic practice. No use, distribution or reproduction is permitted which does not comply with these terms.
*Correspondence: Haichuan Lu, MTgzOTYzMTQwODBAMTYzLmNvbQ==; Yukun Zhao, eWltdWthMTcxOTU2NzA1QDE2My5jb20=
†These authors have contributed equally to this work