- 1Department of Postgraduate, Jiangxi University of Chinese Medicine, Nanchang, Jiangxi, China
- 2Department of Cardiology, Affiliated Hospital of Jiangxi University of Chinese Medicine, Nanchang, Jiangxi, China
- 3Department of Traditional Chinese Medicine, Shangrao Municipal Hospital, Shangrao, Jiangxi, China
- 4National Pharmaceutical Engineering Center, Jiangxi University of Chinese Medicine, Nanchang, Jiangxi, China
Background: Prior research has established a correlation between immune cell activity and heart failure (HF), but the causal nature of this relationship remains unclear. Furthermore, the potential influence of metabolite levels on this interaction has not been comprehensively explored. To address these gaps, we employed a bidirectional Mendelian randomization (MR) approach in two stages to examine whether metabolite levels can mediate the causal relationship between immune cells and HF.
Methods: Genetic information was extracted from summary data of genome-wide association studies. By applying a two-sample, two-step MR approach, we investigated the causal relationships among immune cells, metabolite levels, and HF, with a specific focus on the mediating effects of metabolites. Sensitivity analysis techniques were implemented to ensure the robustness of our findings.
Results: MR analysis revealed significant causal associations between HF and eight specific immune cells and five metabolites. Mediation analysis further identified three mediated relationships. Particularly, hexadecenedioate (C16:1-DC) mediated the influence of both the CD28- CD127- CD25++ CD8br%CD8br (mediation proportion: 19.2%) and CD28+ CD45RA + CD8br%T cells (mediation proportion: 11.9%) on HF. Additionally, the relationship between IgD + CD38br AC cells and HF appeared to be mediated by the phosphate to alanine ratio (mediation proportion: 16.3%). Sensitivity analyses validated that the used instrumental variables were free from pleiotropy and heterogeneity.
Conclusion: This study provides evidence that certain immune cell levels are associated with the risk of HF and that metabolite levels may mediate these relationships. However, to strengthen these findings, further validation using MR analyses with larger sample sizes is essential.
1 Introduction
Heart failure (HF) is a clinical syndrome resulting from impaired cardiac structure or function, representing the severe and final stages of various heart diseases. It carries a high prevalence, mortality, and significant economic burden, making it one of the foremost cardiovascular conditions of the 21st century (1). Studies in epidemiology have revealed that there are currently 64.3 million individuals with HF worldwide, with approximately 3 million new cases annually (2). In China, over 12.1 million individuals aged 25 and older are affected by HF (3). A meta-analysis of 1.5 million patients with chronic HF revealed survival rates of 87%, 73%, 57%, and 35% at 1, 2, 5, and 10 years, respectively (4). HF severely affects the health and quality of life of patients, positioning it as a global priority in chronic disease prevention and research.
As the understanding of the pathophysiological mechanisms underlying HF advances, there is growing evidence supporting the involvement of immune activation in the disease development (5, 6). Both innate (monocytes and macrophages) and adaptive (T lymphocytes) immune responses can intensify HF progression by releasing pro-inflammatory factors. Macrophages, the predominant immune cells in the myocardium, are integral in mediating inflammatory responses, maintaining cardiac stability, and promoting tissue repair (7). During the initial stages of HF, macrophages can polarize into the M1 phenotype, exacerbating myocardial damage through the secretion of pro-inflammatory cytokines and chemokines (8). Furthermore, various triggers associated with cardiac injury can activate effector T cells, which infiltrate the vascular walls. The diverse cytokines released by different T cell subsets can then accelerate vascular aging, degrade the elastic lamina, and promote myocardial fibrosis, ultimately altering cardiovascular structure and function (9).
Emerging in the post-genomic era, metabolomics offers a novel perspective by examining the links between metabolites or metabolic pathways and physiological as well as pathological changes, thereby providing new insights into disease mechanisms (10). Compelling evidence indicates that metabolites and metabolic pathways are intricately linked with HF. For example, a targeted serum metabolomic study has identified several metabolites, including octadecanoic acid, tyrosine, and catecholamines, which are significantly associated with HF severity (11). Additionally, metabolites found in plasma, such as ketone bodies and branched-chain amino acids, might play a mediating role in the interaction between immune cells and HF (12, 13).
Although prior research has established links among immune cells, metabolomics, and HF, the precise causal relationships and the mediating effects of plasma metabolites have yet to be clarified. Utilizing Mendel's Second Law, or the Law of Independent Assortment, Mendelian randomization (MR) is an innovative genetic statistical technique that uses genetic variants linked to exposure factors as instrumental variables (IVs) for determining causal links between exposures and outcomes. This method, which relies on the random allocation determined by DNA genotypes, significantly reduces external influences on these causal relationships (14, 15). Mediation analysis further aids in evaluating how exposure affects outcomes through mediators (16). Thus, utilizing publicly available summary data from genome-wide association studies (GWAS), we executed MR analysis to evaluate the causal relationships among immune cells, plasma metabolites, and HF and to elucidate the mediating role of plasma metabolites.
2 Research methods and materials
2.1 Study design
Initially, we accessed genome-wide data on immune cells, plasma metabolites, and HF from publicly available GWAS summary datasets. A two-sample MR analysis was conducted to investigate the causal interactions among immune cells, plasma metabolites, and HF. Subsequently, a two-step MR analysis was undertaken to determine the mediating influence of plasma metabolites on the interaction between immune cells and HF. Figure 1 depicts the schematic representation of the overall design of our study.
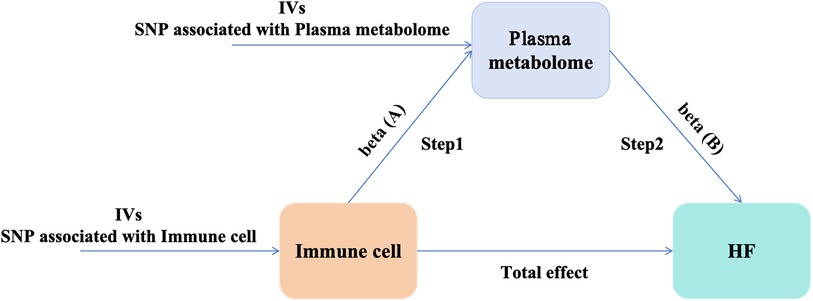
Figure 1. Study design: a two-step MR study of immune cells on HF mediated by plasma metabolome. HF, heart failure; IVs, instrumental variables.
2.2 Data sources
Summary statistics for immune cells were extracted from the GWAS summary dataset hosted at https://www.ebi.ac.uk/gwas/, encompassing study access numbers GCST90001391 to GCST90002121. This dataset included data on 731 immune phenotypes derived from 3,757 European individuals, covering a wide range of cell types such as T, B, and natural killer cells (TBNK), B cells, CDC, Treg, monocytes, various T cell maturation stages, and a bone marrow cell panel, including absolute cell counts (118), relative counts (192), median fluorescence intensities of surface antigens (389), and morphological parameters (32) (17, 18).
For plasma metabolomics, summary statistics were sourced from the same database, with study access numbers GCST90199621 to GCST90201020. This dataset comprised 1,091 plasma metabolites and 309 metabolite ratios from 8,299 European individuals, spanning categories such as 395 types of lipids, 210 types of amino acids, 22 types of carbohydrates, 33 types of nucleotides, 31 types of vitamins, 21 types of peptides, 8 types of energies, 21 types of characteristic molecules, and 220 types of unknown metabolites, etc. (19).
HF GWAS data were obtained from https://gwas.mrcieu.ac.uk/, under the data ID “ebi-a-GCST009541,” involving a cohort of 977,323 participants, which includes 47,309 HF patients and 930,014 controls. HF GWAS data from 26 cohort studies, with cases including subjects clinically diagnosed with HF of any etiology (20).
2.3 Selection of IVs
The selection of IVs must adhere to three core assumptions (16): (1) Single nucleotide polymorphisms (SNPs) utilized as IVs should exhibit a strong association with the exposure factor; (2) SNPs used as IVs must be independent of any confounding factors; (3) SNPs should solely influence the outcome through the exposure factor. To ensure the accuracy and efficacy of the causal links among immune cells, plasma metabolites, and HF risk, we used a genome-wide significance threshold for exposure-related SNPs of P < 1 × 10−5, following guidelines from earlier MR studies (21, 22). To circumvent bias from linkage disequilibrium within SNPs, we used the PLINK clumping method (r2 < 0.001, kb = 10,000) based on data from the 1,000 Genomes Project for Europeans to isolate independent SNPs. We then calculated the F-statistics for each SNP, discarding those with F < 10 to prevent weak IVs from influencing our results.
2.4 MR analysis
2.4.1 Primary analysis
To evaluate the causal impact of immune cells and plasma metabolites on HF, we implemented several MR techniques, encompassing the inverse variance weighted (IVW), MR-Egger regression, weighted median, weighted mode, and simple mode methods. The IVW method is widely acknowledged for its robustness in MR studies for estimating the causal effects of exposure factors on outcomes (23). Consequently, IVW served as the primary analytical tool in our research. The additional methodologies—MR-Egger regression, weighted median, weighted mode, and simple mode—were used to corroborate the primary findings and evaluate the stability of the results.
2.4.2 Mediation analysis
We used a two-step MR approach for mediation analysis to investigate whether plasma metabolites serve as mediators in the disease pathway between immune cells and HF. The total effect was parsed into direct effects (the influence of immune cells on HF) and mediating effects. The causal relationship between immune cells and plasma metabolites was evaluated using the two-sample MR approach, yielding β (A); β (B) was derived from plasma metabolites causally linked to HF. The mediation analysis employed the following formula: Mediating Effect = β (A) × β (B), with the Mediation Proportion calculated as (Mediating Effect/Total Effect) × 100%. The delta method was used to determine the 95% confidence intervals (CI) for both the mediating effect and the mediation proportion.
2.5 Sensitivity analysis
We conducted sensitivity analyses using three distinct approaches, namely the MR-Egger intercept, Cochran's Q test, and the leave-one-out method, to determine the potential effects of heterogeneity and horizontal pleiotropy on our findings. Heterogeneity among the SNPs was assessed using Cochran's Q test, where P < 0.05 was considered indicative of significant heterogeneity. Horizontal pleiotropy was globally evaluated using the MR-Egger intercept, with P < 0.05 suggesting the presence of this effect. Additionally, the leave-one-out method involved sequentially excluding each SNP to observe any resultant variations in the analysis, thereby assessing the robustness of our data.
2.6 Statistical analysis
All MR analyses were conducted using R software (version 4.3.1). For estimating causal effects and identifying outliers, we used the “TwoSampleMR” (version 0.5.8) and “MR-PRESSO” (version 1.0) packages. The results are presented as odds ratios (OR) with 95% CI for each standard deviation. Statistical significance for the MR outcomes was set at P < 0.05. Finally, multiple testing correction was used to eliminate the increase in Type I error caused by multiple testing, where a resultant false discovery rate (FDR) <0.05 was considered causally related; FDR >0.05 but a P value < 0.05 suggests a potential causal relationship; and a P value > 0.05 was considered no causal relationship. Using the online power calculation tool (mRnd) (https://cnsgenomics.com/shiny/mRnd/) to calculate the statistical power of the causal effect estimation (24).
3 Results
3.1 MR analysis of immune cells and HF
Our analysis revealed that among 731 immune cell phenotypes, 8 demonstrate a significant causal relationship with HF (P < 0.01). The IVW results for these immune cells are as follows: IgD + CD38br AC [P = 0.001; FDR = 0.028; OR 95% CI = 1.03 (1.01, 1.05)], CD39+ CD8br%CD8br [P = 0.004; FDR = 0.030; OR 95% CI = 1.03 (1.01, 1.05)], CD28- CD127- CD25++ CD8br% T cell [P = 0.004; FDR = 0.022; OR 95% CI = 1.04 (1.01, 1.07)], CD28- CD127- CD25++ CD8br%CD8br [P = 0.004; FDR = 0.025; OR 95% CI = 1.04 (1.01, 1.06)], CD28+ CD45RA + CD8br% T cell [P = 0.001; FDR = 0.015; OR 95% CI = 1.01 (1.00, 1.01)], and CD28+ CD45RA + CD8br AC [P = 0.007; FDR = 0.023; OR 95% CI = 1.01 (1.00, 1.01)], CD27 on IgD- CD38- [P = 0.007; FDR = 0.026; OR 95% CI = 1.03 (1.01, 1.05)], and CD45 on lymphocytes [P = 0.006; FDR = 0.026; OR 95% CI = 1.04 (1.01, 1.07)]. Each phenotype showed a positive correlation with HF risk (Figure 2). Sensitivity analyses using the MR-Egger intercept and Cochran's Q test yielded P-values greater than 0.05, suggesting no evidence of horizontal pleiotropy or heterogeneity among the IVs. The leave-one-out analysis indicated no significant outliers, validating the stability and reliability of the MR findings. Detailed results of the MR analysis of immune cells and HF are available in Supplementary Tables S3, S4 and Supplementary Figures 1–3.
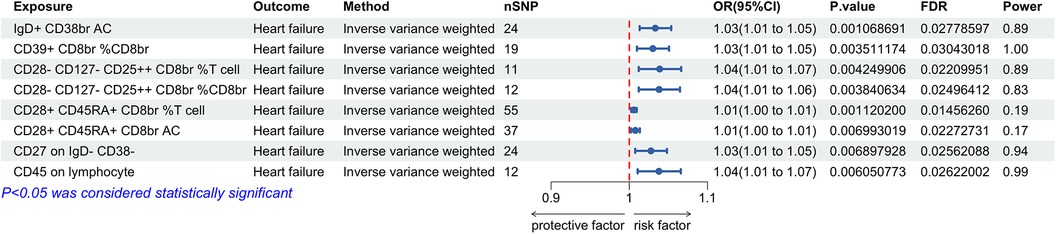
Figure 2. Forest plot for the Mendelian randomization analysis between immune cells and heart failure.
3.2 MR analysis of plasma metabolites and HF
The findings indicated that among 1,400 plasma metabolites, 5 exhibited a significant causal relationship with HF (P < 0.01). The IVW results for these metabolites were as follows: N-acetylglycine levels [P = 0.006; FDR = 0.045; OR 95% CI = 1.18 (1.05, 1.32)], N-acetylalliin levels [P < 0.001; FDR = 0.003; OR 95% CI = 0.82 (0.74, 0.90)], hexadecenedioate (C16:1-DC) levels [P = 0.005; FDR = 0.041; OR 95% CI = 1.15 (1.04, 1.26)], phosphate to alanine ratio [P = 0.007; FDR = 0.047; OR 95% CI = 0.86 (0.76, 0.96)], and bilirubin (Z,Z) to glucuronate ratio [P = 0.003; FDR = 0.036; OR 95% CI = 0.82 (0.72, 0.94)]. N-acetylglycine and hexadecenedioate (C16:1-DC) levels positively correlated with HF risk, whereas N-acetylalliin levels, phosphate to alanine ratio, and bilirubin (Z,Z) to glucuronate ratio negatively correlated with HF risk (Figure 3). The MR-Egger intercept and Cochran's Q tests revealed no significant horizontal pleiotropy or heterogeneity. Leave-one-out analysis showed no biased SNPs, indicating robust results. A detailed discussion of the MR analysis of plasma metabolites and HF is provided in Supplementary Tables S5, S6 and Supplementary Figures S4–S6.

Figure 3. Forest plot for the Mendelian randomization analysis between plasma metabolites and heart failure.
3.3 Mediation analysis results
To explore the potential mechanisms driving the onset and progression of HF, we conducted a mediation analysis to identify the causal pathways mediated by plasma metabolites from immune cells to HF. Initially, the causal relationships between immune cells and plasma metabolites were evaluated using two-sample MR. The IVW results showed positive correlations of CD28+ CD45RA + CD8br%T [P = 0.049; OR 95% CI = 1.01 (1.00, 1.01)] and CD28- CD127- CD25++ CD8br%CD8br cells [P = 0.014; OR 95% CI = 1.05 (1.01, 1.10)] with hexadecenedioate (C16:1-DC) levels. In contrast, IgD + CD38br AC cells [P = 0.003; OR 95% CI = 0.97 (0.95, 0.99)] were negatively correlated with the phosphate to alanine ratio (Figure 4). Subsequent mediation analysis revealed that hexadecenedioate (C16:1-DC) mediated the pathways from CD28+ CD45RA + CD8br%T and CD28- CD127- CD25++ CD8br%CD8br cells to HF, with mediation proportions of 11.9% and 19.2%, respectively. Additionally, the phosphate to alanine ratio was found to mediate the link between IgD + CD38br AC cells and HF, with a mediation proportion of 16.3% (Table 1). Further details are provided in Supplementary Table S7 and Supplementary Figures S7–S9.

Figure 4. Forest plot for the Mendelian randomization analysis between immune cells and plasma metabolites.
4 Discussion
In this study, we utilized a two-sample MR analysis to investigate the causal relationships among immune cells, plasma metabolites, and HF. Our findings demonstrate that IgD + CD38br AC, CD39+ CD8br%CD8br, CD28- CD127- CD25++ CD8br%T, CD28- CD127- CD25++ CD8br%CD8br, CD28+ CD45RA + CD8br%T, and CD28+ CD45RA + CD8br AC cells, CD27 on IgD- CD38- cells, and CD45 on lymphocytes contribute to an increased risk of HF. Similarly, elevated levels of N-acetylglycine and hexadecenedioate (C16:1-DC) are associated with a higher risk of HF, whereas lower risks are linked to N-acetylalliin levels, phosphate to alanine ratio, and bilirubin (Z,Z) to glucuronate ratio. Further analysis reveals that the increased HF risk associated with CD28+ CD45RA + CD8br%T and CD28- CD127- CD25++ CD8br%CD8br cells is mediated by hexadecenedioate (C16:1-DC). Moreover, the heightened HF risk linked to IgD + CD38br AC cells is mediated by the phosphate to alanine ratio.
CD28+ CD45RA + CD8br%T and CD28- CD127- CD25++ CD8br%CD8br cells are classified under the Treg panel, whereas IgD + CD38br AC cells fall under the B cell panel. T lymphocytes, the pivotal immune cells in the development and progression of HF, are categorized into CD4 + and CD8 + subgroups based on surface markers. Once activated, CD4 + cells can differentiate into regulatory and effector T cell subgroups, which include Th1, Th2, Th17, and Tregs (25). Tregs, comprising approximately 5%–10% of all peripheral CD4+ T cells, play a pivotal role in maintaining internal equilibrium, immune homeostasis, and peripheral immune tolerance through the production of anti-inflammatory cytokines such as transforming growth factor β and interleukin 10. These cytokines inhibit the activity of other immune cells, including antigen-presenting cells like macrophages and CD8 + effector T cells, thereby reducing inflammation and preventing hyperactive immune responses (26, 27). Emerging research suggests that a decrease in circulating Treg numbers is associated with an increased risk of cardiovascular diseases. Moreover, Treg counts may serve as valuable biomarkers for predicting exacerbations of HF and the likelihood of rehospitalization (28, 29). In the early stages of cardiac injury, Tregs can mitigate inflammatory responses, generate repair-associated molecules, and directly facilitate repair. However, as HF progresses, Tregs may alter their phenotype and functionality, exacerbating HF (30, 31). CD28, a co-stimulatory molecule expressed on T lymphocytes, is crucial for T cell activation. Lack of CD28 can diminish systemic and cardiac inflammation, suppress T cell activation, and slow the progression of HF (32). In contrast, B cells produce pro-inflammatory factors early in the injury process, recruit monocytes to the heart, and aggravate acute cardiac injury (31). CD38 is a type II transmembrane glycoprotein vital for maintaining intracellular NAD levels. Evidence suggests that a deficiency in CD38 significantly boosts intracellular nicotinamide adenine dinucleotide (NAD) levels across various tissues, curtails oxidative stress pathways, and ameliorates cardiac hypertrophy and myocardial fibrosis (33).
Hexadecenedioate (C16:1-DC), as a lipid, plays a pivotal role in fatty acid metabolism (34). Research indicates that elevated plasma free fatty acid levels can enhance myocardial free fatty acid uptake, thereby augmenting intramyocardial lipid storage and contributing to left ventricular dysfunction (35). Alanine, classified as a non-essential amino acid, is predominantly synthesized through glycolysis and other metabolic pathways (36). Metabolomic studies have demonstrated reductions in alanine levels in the serum of HF models induced by oxidative stress (37). Strong evidence indicates that alterations in energy metabolism exacerbate the severity of HF. Stimulating glucose metabolism or inhibiting fatty acid oxidation can alleviate the effects of decreased mitochondrial oxidative capacity and improve cardiac function (38). Phosphate, a constituent of phospholipids, is crucial for cellular energy metabolism and participates in glycolysis, ammoniagenesis, and oxidative phosphorylation. Persistently high levels of plasma phosphate can elicit inflammatory responses and vascularization, thereby increasing cardiovascular disease morbidity and mortality (39, 40). Thus, for HF patients, monitoring hexadecenedioate (C16:1-DC), alanine, and phosphate levels can aid in disease prevention and early diagnosis, reducing the risk of developing HF. Furthermore, previous research has shown that cellular metabolism regulates immune cell function and differentiation, influencing outcomes in adaptive and innate immune responses. Effector T and Th17 cells rely on aerobic glycolysis, whereas memory T cells and Tregs primarily depend on fatty acid oxidation for energy (41). Our findings affirm the mediating roles of hexadecenedioate (C16:1-DC) and the phosphate to alanine ratio in the associations of CD28+ CD45RA + CD8br%T, CD28- CD127- CD25++ CD8br%CD8br, and IgD + CD38br AC cells with HF. Our mediation analysis indicated that hexadecenedioate (C16:1-DC) contributed to 11.9% and 19.2% of the mediation in the effects of CD28+ CD45RA + CD8br%T and CD28- CD127- CD25++ CD8br%CD8br cells on HF, respectively. The phosphate to alanine ratio mediated 16.3% of the effect of IgD + CD38br AC cells on HF.
Our research boasts several strengths. First, utilizing a two-sample, two-step MR analysis, we examined the causal links and mediating roles of plasma metabolites between immune cells and HF. Second, our study relied on a comprehensive population genetic database, enhancing the reliability of our findings. Additionally, extensive sensitivity analyses were conducted to bolster the robustness of our MR outcomes. Despite these strengths, our study has certain limitations. The data originates from a European cohort, and there may be interactions between diet, genes, and the environment (42), which could constrain the relevance of our findings to other demographic groups. Furthermore, the impact of age varies across the exposure and outcome variables studied. Although HF demonstrates a stronger correlation with age, our data lacks age-specific screening. Future research obtaining age-specific GWAS data could further refine these results through more targeted MR analyses.
5 Conclusion
Our MR study has established causal connections between eight specific immune cells, five metabolites, and HF. Additionally, findings from mediation analysis suggest that hexadecenedioate (C16:1-DC) influences HF regulation through CD28+ CD45RA + CD8br%T and CD28- CD127- CD25++ CD8br%CD8br cells. Similarly, the phosphate to alanine ratio affects HF regulation through IgD + CD38br AC cells. These identified immune cells and plasma metabolites can act as valuable biomarkers for diagnosing and treating HF and help in understanding its pathophysiological mechanisms.
Data availability statement
The original contributions presented in the study are included in the article/Supplementary Material, further inquiries can be directed to the corresponding authors.
Author contributions
TL: Methodology, Writing – original draft. YL: Data curation, Funding acquisition, Writing – review & editing. JF: Software, Writing – review & editing. LH: Data curation, Funding acquisition, Methodology, Writing – original draft, Writing – review & editing. ZL: Funding acquisition, Supervision, Writing – review & editing.
Funding
The author(s) declare financial support was received for the research, authorship, and/or publication of this article. This study was funded by several prestigious grants, including the National Natural Science Foundation of China (82460914, 82260918), National Traditional Chinese Medicine Expert Inheritance Studio Construction Project [Ministry of Traditional Chinese Medicine Education Letter (2022) No. 75], NATCM's Project of High-level Construction of Key TCM Disciplines (zyyzdxk-2023113), Natural Science Foundation of Jiangxi Province (20242BAB20439, 20232BAB206146), Science and Technology Project of Jiangxi Provincial Health Commission (202410045), Key Discipline Construction Fund of Jiangxi University of Chinese Medicine (2023jzzdxk033), and Jiangxi University of Chinese Medicine College Student Innovation and Entrepreneurship Training Program project (202410412267).
Conflict of interest
The authors declare that the research was conducted in the absence of any commercial or financial relationships that could be construed as a potential conflict of interest.
Publisher's note
All claims expressed in this article are solely those of the authors and do not necessarily represent those of their affiliated organizations, or those of the publisher, the editors and the reviewers. Any product that may be evaluated in this article, or claim that may be made by its manufacturer, is not guaranteed or endorsed by the publisher.
Supplementary material
The Supplementary Material for this article can be found online at: https://www.frontiersin.org/articles/10.3389/fcvm.2024.1430477/full#supplementary-material
References
2. Groenewegen A, Rutten FH, Mosterd A, Hoes AW. Epidemiology of heart failure. Eur J Heart Fail. (2020) 22:1342–56. doi: 10.1002/ejhf.1858
3. Wang H, Chai K, Du M, Wang S, Cai JP, Li Y, et al. Prevalence and incidence of heart failure among urban patients in China: a national population-based analysis. Circ Heart Fail. (2021) 14(e008406):1127–30. doi: 10.1161/CIRCHEARTFAILURE.121.008406
4. Jones NR, Roalfe AK, Adoki I, Hobbs FDR, Taylor CJ. Survival of patients with chronic heart failure in the community: a systematic review and meta-analysis. Eur J Heart Fail. (2019) 21:1306–25. doi: 10.1002/ejhf.1594
5. Rurik JG, Aghajanian H, Epstein JA. Immune cells and immunotherapy for cardiac injury and repair. Circ Res. (2021) 128:1766–79. doi: 10.1161/CIRCRESAHA.121.318005
6. Swirski FK, Nahrendorf M. Cardioimmunology: the immune system in cardiac homeostasis and disease. Nat Rev Immunol. (2018) 18:733–44. doi: 10.1038/s41577-018-0065-8
7. DeBerge M, Shah SJ, Wilsbacher L, Thorp EB. Macrophages in heart failure with reduced versus preserved ejection fraction. Trends Mol Med. (2019) 25:328–40. doi: 10.1016/j.molmed.2019.01.002
8. Halade GV, Norris PC, Kain V, Serhan CN, Ingle KA. Splenic leukocytes define the resolution of inflammation in heart failure. Sci Signal. (2018) 11:eaao1818. doi: 10.1126/scisignal.aao1818
9. Rai A, Narisawa M, Li P, Piao L, Li Y, Yang G, et al. Adaptive immune disorders in hypertension and heart failure: focusing on T-cell subset activation and clinical implications. J Hypertens. (2020) 38:1878–89. doi: 10.1097/HJH.0000000000002456
10. Johnson CH, Ivanisevic J, Siuzdak G. Metabolomics: beyond biomarkers and towards mechanisms. Nat Rev Mol Cell Biol. (2016) 17:451–9. doi: 10.1038/nrm.2016.25
11. Guo S, Kong J, Zhou D, Lai M, Chen Y, Xie D, et al. Serum metabolic characteristics and biomarkers of early-stage heart failure. Biomark Med. (2020) 14:119–30. doi: 10.2217/bmm-2019-0176
12. Sun H, Olson KC, Gao C, Prosdocimo DA, Zhou M, Wang Z, et al. Catabolic defect of branched-chain amino acids promotes heart failure. Circulation. (2016) 133:2038–49. doi: 10.1161/CIRCULATIONAHA.115.020226
13. Matsuura TR, Puchalska P, Crawford PA, Kelly DP. Ketones and the heart: metabolic principles and therapeutic implications. Circ Res. (2023) 132:882–98. doi: 10.1161/CIRCRESAHA.123.321872
14. Habibi D, Daneshpour MS, Asgarian S, Kohansal K, Hadaegh F, Mansourian M, et al. Effect of C-reactive protein on the risk of heart failure: a Mendelian randomization study. BMC Cardiovasc Disord. (2023) 23(112). doi: 10.1186/s12872-023-03149-3
15. Liu N, Tan JS, Liu L, Li H, Wang Y, Yang Y, et al. Roles of obesity in mediating the causal effect of attention-deficit/hyperactivity disorder on diabetes. Epidemiol Psychiatr Sci. (2023) 32(e32). doi: 10.1017/S2045796023000173
16. Carter AR, Sanderson E, Hammerton G, Richmond RC, Davey Smith G, Heron J, et al. Mendelian randomisation for mediation analysis: current methods and challenges for implementation. Eur J Epidemiol. (2021) 36:465–78. doi: 10.1007/s10654-021-00757-1
17. Orrù V, Steri M, Sidore C, Marongiu M, Serra V, Olla S, et al. Complex genetic signatures in immune cells underlie autoimmunity and inform therapy. Nat Genet. (2020) 52:1036–45. doi: 10.1038/s41588-020-0684-4
18. Wang C, Zhu D, Zhang D, Zuo X, Yao L, Liu T, et al. Causal role of immune cells in schizophrenia: Mendelian randomization (MR) study. BMC Psychiatry. (2023) 23(590). doi: 10.1186/s12888-023-05081-4
19. Chen Y, Lu T, Pettersson-Kymmer U, Stewart ID, Butler-Laporte G, Nakanishi T, et al. Genomic atlas of the plasma metabolome prioritizes metabolites implicated in human diseases. Nat Genet. (2023) 55:44–53. doi: 10.1038/s41588-022-01270-1
20. Shah S, Henry A, Roselli C, Lin H, Sveinbjörnsson G, Fatemifar G, et al. Genome-wide association and Mendelian randomisation analysis provide insights into the pathogenesis of heart failure. Nat Commun. (2020) 11(163). doi: 10.1038/s41467-019-13690-5
21. Xu S, Liu Y, Wang Q, Liu F, Xian Y, Xu F, et al. Gut microbiota in combination with blood metabolites reveals characteristics of the disease cluster of coronary artery disease and cognitive impairment: a Mendelian randomization study. Front Immunol. (2024) 14(1308002). doi: 10.3389/fimmu.2023.1308002
22. Hu X, Binxu Q, Shao GZ, Huang Y, Qiu W. Gut microbiota, circulating metabolites, and gallstone disease: a Mendelian randomization study. Front Microbiol. (2024) 15(1336673). doi: 10.3389/fmicb.2024.1336673
23. Sheng D, Wang S, Li P, Li J, Xiao Z, Lv H, et al. Evidence for genetic causal relationships between gut microbiome, metabolites, and myasthenia gravis: a bidirectional Mendelian randomization study. Front Immunol. (2023) 14(1279845). doi: 10.3389/fimmu.2023.1279845
24. Brion MJ, Shakhbazov K, Visscher PM. Calculating statistical power in Mendelian randomization studies. Int J Epidemiol. (2013) 42:1497–501. doi: 10.1093/ije/dyt179
25. Cai YH, Ma ZJ, Lu XY, He EL, You MY. Study on the effect and mechanism of the dysfunction of CD4(+) T cells in the disease process of chronic cardiac failure. Asian Pac J Trop Med. (2016) 9:682–7. doi: 10.1016/j.apjtm.2016.05.006
26. Xia Y, Gao D, Wang X, Liu B, Shan X, Sun Y, et al. Role of Treg cell subsets in cardiovascular disease pathogenesis and potential therapeutic targets. Front Immunol. (2024) 15(1331609). doi: 10.3389/fimmu.2024.1331609
27. Proto JD, Doran AC, Gusarova G, Jr YA, Sozen E, Subramanian M, et al. Regulatory T cells promote macrophage efferocytosis during inflammation resolution. Immunity. (2018) 49:666–77.e6. doi: 10.1016/j.immuni.2018.07.015
28. Wigren M, Bjorkbacka H, Andersson L, Ljungcrantz I, Fredrikson GN, Persson M, et al. Low levels of circulating CD4+FoxP3+ T cells are associated with an increased risk for development of myocardial infarction but not for stroke. Arterioscler Thromb Vasc Biol. (2012) 32:2000–4. doi: 10.1161/ATVBAHA.112.251579
29. Okamoto N, Noma T, Ishihara Y, Miyauchi Y, Takabatake W, Oomizu S, et al. Prognostic value of circulating regulatory T cells for worsening heart failure in heart failure patients with reduced ejection fraction. Int Heart J. (2014) 55:271–7. doi: 10.1536/ihj.13-343
30. Bansal SS, Ismahil MA, Goel M, Zhou G, Rokosh G, Hamid T, et al. Dysfunctional and proinflammatory regulatory T-lymphocytes are essential for adverse cardiac remodeling in ischemic cardiomyopathy. Circulation. (2019) 139:206–21. doi: 10.1161/CIRCULATIONAHA.118.036065
31. Lu Y, Xia N, Cheng X. Regulatory T cells in chronic heart failure. Front Immunol. (2021) 12(732794). doi: 10.3389/fimmu.2021.732794
32. Wang H, Kwak D, Fassett J, Hou L, Xu X, Burbach BJ, et al. CD28/B7 deficiency attenuates systolic overload-induced congestive heart failure, myocardial and pulmonary inflammation, and activated T cell accumulation in the heart and lungs. Hypertension. (2016) 68:688–96. doi: 10.1161/HYPERTENSIONAHA.116.07579
33. Guan XH, Hong X, Zhao N, Liu XH, Xiao YF, Chen TT, et al. CD38 promotes angiotensin II-induced cardiac hypertrophy. J Cell Mol Med. (2017) 21:1492–502. doi: 10.1111/jcmm.13076
34. Ren T, Jones RS, Morris ME. Untargeted metabolomics identifies the potential role of monocarboxylate transporter 6 (MCT6/SLC16A5) in lipid and amino acid metabolism pathways. Pharmacol Res Perspect. (2022) 10(e00944). doi: 10.1002/prp2.944
35. Djoussé L, Benkeser D, Arnold A, Kizer JR, Zieman SJ, Lemaitre RN, et al. Plasma free fatty acids and risk of heart failure: the cardiovascular health study. Circ Heart Fail. (2013) 6:964–9. doi: 10.1161/CIRCHEARTFAILURE.113.000521
36. Wei X, Jia X, Liu R, Zhang S, Liu S, An J, et al. Metabolic pathway analysis of hyperuricaemia patients with hyperlipidaemia based on high-throughput mass spectrometry: a case‒control study. Lipids Health Dis. (2022) 21(151). doi: 10.1186/s12944-022-01765-0
37. Spyropoulos F, Sorrentino A, van der Reest J, Yang P, Waldeck-Weiermair M, Steinhorn B, et al. Metabolomic and transcriptomic signatures of chemogenetic heart failure. Am J Physiol Heart Circ Physiol. (2022) 322:H451–65. doi: 10.1152/ajpheart.00628.2021
38. Fillmore N, Levasseur JL, Fukushima A, Wagg CS, Wang W, Dyck JRB, et al. Uncoupling of glycolysis from glucose oxidation accompanies the development of heart failure with preserved ejection fraction. Mol Med. (2018) 24(3). doi: 10.1186/s10020-018-0005-x
39. Wagner CA. The basics of phosphate metabolism. Nephrol Dial Transplant. (2024) 39:190–201. doi: 10.1093/ndt/gfad188
40. Ess M, Heitmair-Wietzorrek K, Frick M, Umlauf N, Ulmer H, Poelzl G. Serum phosphate and long-term outcome among patients with stable heart failure. J Card Fail. (2013) 19:25–30. doi: 10.1016/j.cardfail.2012.11.008
41. Palmer CS, Ostrowski M, Balderson B, Christian N, Crowe SM. Glucose metabolism regulates T cell activation, differentiation, and functions. Front Immunol. (2015) 6(1). doi: 10.3389/fimmu.2015.00001
Keywords: plasma metabolome, immune cells, heart failure, Mendelian randomization, mediate
Citation: Li T, Liu Y, Fu J, Huang L and Liu Z (2024) Plasma metabolome mediates the causal relationship between immune cells and heart failure: a two-step bidirectional Mendelian randomization study. Front. Cardiovasc. Med. 11:1430477. doi: 10.3389/fcvm.2024.1430477
Received: 10 May 2024; Accepted: 20 September 2024;
Published: 9 October 2024.
Edited by:
Jian Cao, Chinese PLA General Hospital, ChinaReviewed by:
Jiang-Shan Tan, Chinese Academy of Medical Sciences and Peking Union Medical College, ChinaTong Yin, Medical School of Chinese PLA and Chinese PLA General Hospital, China
Sharath Kommu, Marshfield Clinic, United States
Copyright: © 2024 Li, Liu, Fu, Huang and Liu. This is an open-access article distributed under the terms of the Creative Commons Attribution License (CC BY). The use, distribution or reproduction in other forums is permitted, provided the original author(s) and the copyright owner(s) are credited and that the original publication in this journal is cited, in accordance with accepted academic practice. No use, distribution or reproduction is permitted which does not comply with these terms.
*Correspondence: Langlang Huang, MTI1NTI4MzM3NUBxcS5jb20=; Zhongyong Liu, bHp5b25nbWFpbEAxNjMuY29t