- 1RAND, Santa Monica, CA, United States
- 2Victoria University of Wellington, Wellington, New Zealand
- 3Department of Earth & Planetary Sciences and Rutgers Climate & Energy Institute, Rutgers University, New Brunswick, NJ, United States
- 4Deltares, Delft, Netherlands
- 5Utrecht University, Utrecht, Netherlands
- 6Institute of Climate, Energy and Disaster Solutions (ICEDS), Australian National University, Canberra ACT, Australia
- 7University College London, London, United Kingdom
The Intergovernmental Panel on Climate Change (IPCC) exists to provide policy-relevant assessments of the science related to climate change. As such, the IPCC has long grappled with characterizing and communicating uncertainty in its assessments. Decision Making under Deep Uncertainty (DMDU) is a set of concepts, methods, and tools to inform decisions when there exist substantial and significant limitations on what is and can be known about policy-relevant questions. Over the last twenty-five years, the IPCC has drawn increasingly on DMDU concepts to more effectively include policy-relevant, but lower-confidence scientific information in its assessments. This paper traces the history of the IPCC’s use of DMDU and explains the intersection with key IPCC concepts such as risk, scenarios, treatment of uncertainty, storylines and high-impact, low-likelihood outcomes, and both adaptation and climate resilient development pathways. The paper suggests how the IPCC might benefit from enhanced use of DMDU in its current (7th) assessment cycle.
1 Introduction
The Intergovernmental Panel on Climate Change (IPCC) exists to provide policy-relevant assessments of the science related to climate change, its impacts, risks, and potential adaptation and mitigation responses (IPCC, 2021a). As such, the IPCC has always grappled with characterizing and communicating uncertainty in its assessments. Decision Making under Deep Uncertainty (DMDU) is a set of concepts, methods, and tools to inform decisions when there exist substantial and significant limitations on what is and can be known about policy-relevant questions (Marchau et al., 2019a). The IPCC has drawn increasingly on DMDU in recent assessment cycles, but not always explicitly, clearly, or comprehensively. The IPCC now faces a fast-changing climate and an increasing focus on solutions that take place in diverse situations and evolving societal contexts, so that DMDU could prove even more useful in the future. This paper reviews the evolving use of DMDU by the IPCC and suggests how the organization can more fully incorporate these concepts in its next assessment cycle.
The IPCC was established in 1988 by the World Meteorological Organization (WMO) and United Nations Environment Programme (UNEP); since that time, it has conducted six assessment cycles and is now beginning a seventh. The IPCC membership consists of 195 national governments, who elect the IPCC’s scientific leadership. The IPCC is organized around three working groups (WG). Since the Third Assessment Report WGI has assessed climate science; WGII has assessed the impacts of climate change, vulnerability to those impacts, and adaptation solutions; and WGIII has assessed ways to reduce the greenhouse gas forcings that contribute to climate change, as described in Box 1. Assessment cycles have produced Special Reports on specific topics, a comprehensive Assessment Report from each Working Group, and a Synthesis Report by all three Working Groups. Scientists from around the world volunteer their time to write these IPCC reports. Most of the authors of this paper had the privilege to serve as authors in the IPCC Sixth Assessment and previous cycles.
BOX 1. Structure of the IPCC
The IPCC process unfolds over assessment cycles, each of which is launched by the governments and then runs for five to seven years. The IPCC has for the last few assessment cycles been organized around three working groups (WG): The Physical Science Basis of Climate Change (WG I), Climate Change Impacts, Adaptation, and Vulnerability (WG II), and The Mitigation of Climate Change (WGIII).
Each assessment cycle produces an assessment report from each working group. The WGI report is issued first, followed by the WGII and WGIII reports. Each working groups’ assessment report has an extensive main report written by the authors and a much shorter Summary for Policymakers (SPM) drafted by the authors and approved line-by-line by the governments.
Prior to the assessment reports, the IPCC may also produce several Special Reports on specific topics. For example, the Fourth Assessment Report (AR4) produced, amongst others, the Special Report on Emissions Scenarios (SRES) and the Fifth Assessment Report (AR5) produced the Special Report on Managing the Risks of Extreme Events and Disasters to Advance Climate Change Adaptation (SREX). The Sixth Assessment Report (AR6) produced special reports on the oceans and cryosphere in a changing climate, climate change and land, and global warming of 1.5°C. Two or three of the working groups collaborate to produce these Special Reports.
Each assessment cycle culminates in a Synthesis Report in which authors from all three working groups collaborate to summarize and integrate the findings from the assessment and special reports.
Whilst the fact of human-induced climate change is “virtually certain” (IPCC WG1), uncertainties play a central role in IPCC assessments and decision-making. The IPCC now commonly engages with policy questions for which the underlying science or the real-world efficacy of specific responses is less clear. For instance, climate scientists can project increases in global mean temperature far more accurately than they can project precipitation extremes in a particular watershed. But policymakers concerned about flooding care greatly about future local precipitation extremes. Similarly, technologists can estimate the average cost, performance, and uptake of well-established technologies with far more accuracy than for novel technologies, or the barriers to uptake of existing technologies within a novel societal context. Policymakers aiming to meet decarbonization goals may care greatly about the future properties of novel technologies and any new barriers to existing ones.
The IPCC Seventh Assessment cycle, which is just getting underway, will also face significant uncertainty challenges. One reason is that an increased focus on solutions greatly expands the questions the IPCC needs to address. The 1992 United Nations Framework Convention on Climate Change (UNFCCC) called for preventing dangerous anthropogenic interference in the climate system. In its early years, the IPCC focused primarily on informing the global-scale contours of this challenge – what amount of warming is and will be caused by rising greenhouse gas concentrations, what level of atmospheric greenhouse gas concentrations might constitute dangerous interference and how rapidly must emissions decline in order to prevent such interference? Today, two international agreements, informed in part by answers to these questions – the Paris Agreement building on the UNFCCC, and the Sustainable Development Goals (SDGs) as set out in the 2030 Agenda for Sustainable Development – provide overarching goals for climate action (Ara Begum et al., 2022). Achieving these goals requires a multiplicity of mitigation and adaptation actions at the international, national, regional, and local levels by numerous actors including governments, the private sector, and civil society.
The Sixth Assessment Report (AR6) advanced analysis of the feasibility and effectiveness of many potential mitigation and adaptation solutions such actors might undertake, drawing on climate science, engineering, economics, sociology, political science, geography and numerous other disciplines. Each introduces different dimensions of uncertainty. In addition, the Paris Agreement and SDGs reflect demands for recognition and justice from many groups suggesting that the Seventh Assessment cycle will increasingly need to assess interdependencies across sectors and across climate and non-climate domains, with more central roles for innovation and a wide range of economic and non-economic co-benefits. Decision makers considering climate action care not only about the risks generated by climate change, but the safety, economic, political, and other risks potentially generated by taking action, which introduces additional and significant policy-relevant uncertainties.
The IPCC will also increasingly grapple with both the challenges of understanding climate changes that push systems outside their range of previous behavior as well as of informing transformational mitigation and adaptation solutions. Climate change is already driving many regional climates beyond well-understood regimes (IPCC, 2021b) while current climate action is insufficient to meet the Paris goals, or SDGs (IPCC, 2022; Malekpour et al., 2023). Increasing climate action would require unprecedented expansion of novel policies, practices, behaviors, and technologies. Failure to increase climate action will increasingly result in a climate, interlinked with many human and natural systems, pushed into poorly understood regimes.
AR6 notes the inherently complex nature of the risks generated by large changes in climate and human systems, which include “multiple stressors unfolding together, cascading or compounding interactions, and non-linear responses and the potential for surprise” (Ara Begum et al., 2022, Sect 1.3.1.2). AR6 defines the concept of transformation as a “change in the fundamental attributes of natural and human systems” (IPCC, 2023) and finds that some type of transformation of natural or human systems is now inevitable, given the combination of rapid changes in the climate and the rapid socio-economic changes needed to slow climate change and adapt to its effects (Ara Begum et al., 2022, Sect 1.5.1). Complex systems can be understood but are often hard to predict with any confidence (Mitchell, 2009).
A heightened salience of solutions, cascading risks, complex systems, and systems transformation thus increases the relevance of DMDU. The IPCC defines deep uncertainty as existing “when experts or stakeholders do not know or cannot agree on: (1) appropriate conceptual models that describe relationships among key driving forces in a system, (2) the probability distributions used to represent uncertainty about key variables and parameters and/or (3) how to weigh and value desirable alternative outcomes” (IPCC, 2023). The DMDU field consists of a set of concepts, methods, and approaches designed to inform and improve decisions that face such conditions. While these methods are varied, they all emphasize multi-scenario, multi-objective decision analyses, considering a wide range of plausible futures; seeking policies which are robust over these futures rather than optimal for any best estimate; addressing multiple rather than single policy objectives in order to reflect a diversity of values; and explicitly designing policies to adjust over time in response to new information.
The literature contrasts DMDU with “predict-then-act” analyses. The latter base policy advice on consensus predictions of the future and, in the DMDU critique, can create incentives for underestimating uncertainty and exacerbate controversy by encouraging those making science-based policy recommendations to appear more confident in the supporting evidence than they actually are (Kalra et al., 2014; Marchau et al., 2019b). In response, DMDU methods embrace two key ideas. First, science-based analysis should seek to facilitate human creativity, deliberation, and judgment in solving complex problems rather than aspire to proscribe the best decisions. Second, science can help decision-makers manage deep uncertainty, not just reduce it.
Decision makers in national and sub-national governments have successfully employed DMDU to help manage climate risk. To give just a few examples, the United States Bureau of Reclamation worked with seven U.S. states using Robust Decision Making (RDM) to identify water management strategies that could reduce vulnerabilities in the Colorado River Basin (Groves et al., 2019). Regional flood risk managers in New Zealand used Dynamic Adaptive Policy Pathways (DAPP) to develop a long-term plan given changing flood frequencies (Lawrence et al., 2019) and in the Netherlands the national government uses this approach for flood risk and water security (Bloemen et al., 2019; van Alphen et al., 2022). DAPP has similarly been used at the city level (see, e.g., for Pacific region, Ocean and Climate Platform, 2023, for London, Kingsborough et al. (2017) and for Ho Chi Min City, Scussolini et al., 2017). Costa Rica used RDM to evaluate its Nationally Determined Contributions (NDC) under the Paris Accord (Groves et al., 2020).
Over its last two assessment cycles, the IPCC has offered a risk-management framing for the policy challenges posed by climate change. As described in more detail below, DMDU ideas infuse the IPCC risk framework. Risk is in some disciplines defined narrowly as the probability of an event multiplied by its consequences. The IPCC uses a broader definition, which includes well-known probabilities and consequences as a special case, but also acknowledges the possibility of deep uncertainty, in which the probabilities and consequences associated with many climate-related risks are imprecise, contested, or unknown.
Climate change is an archetypal example of a deeply uncertain policy challenge. While DMDU has found its most explicit applications in WGI and WGII, DMDU concepts also infuse WGIII. This paper reviews the history of how the IPCC has used DMDU concepts over its previous six assessment cycles and suggests how DMDU has been and will become increasingly useful for the IPCC. The next section reviews this history. The third section clarifies the relationship among DMDU and related IPCC key concepts, including risk, scenarios, low confidence, storylines, low-likelihood high impact outcomes, and adaptation and climate resilient development pathways. The final section offers guidance for the leadership and authors of the Seventh Assessment cycle in the use of DMDU.
2 DMDU in the first six IPCC assessment cycles
The representation and communication of uncertainty has proven a central and enduring theme throughout the history of the IPCC. Science operates at the edge of uncertainty, so any comprehensive assessment of the state of the science necessarily engages with unresolved questions and incomplete data and knowledge. Clear and consistent language is important for effective risk communication (Pidgeon and Fischhoff, 2011). The IPCC’s breadth complicates such clear and consistent language, since the organization encompasses many scientific disciplines each with their own approaches to representing uncertainty (Adler and Hirsch Hadorn, 2014). This challenge has grown as the IPCC encompasses an even wider range of disciplines and uncertainty approaches as it shifts from a previous focus on explicating the risks of climate change to an increased focus on informing solutions (Magnan et al., 2020).
As an even more significant challenge, the IPCC is tasked with providing scientific input into what are necessarily value-laden and politically fraught climate-related decisions for which both action and inaction can generate risks for decision makers. Climate change is often described as a (super) wicked problem with contested framings, deep uncertainty, unequal power relationships, vested interests, and no central authority (Levin et al., 2012). In such situations, uncertainty can complicate effective provision of scientific information. Political actors can highlight uncertainty to delay action or to contest the legitimacy of scientists whose claims they find inconvenient. Conversely, scientists claiming they know more than they do can also undermine public trust (Sarewitz and Pielke, 2000).
DMDU was designed to help ameliorate such challenges by providing a structure for informing contested decisions with deeply uncertain information (Popper, 2019). Its multi-scenario, multi-objective framing enhances transparency regarding key assumptions and the tradeoffs among the objectives of different groups (Lempert et al., 2013) as well as facilitates the evaluation of risk from the perspective of diverse values and worldviews (Jafino et al., 2021; Lempert and Turner, 2021). Consistent with the understanding of many political theorists, DMDU seeks to inform agreement on actions to take rather than on the often more difficult task of generating consensus on the underlying truths that might support such actions (Lempert, 2013, Sect 4).
2.1 IPCC treatment of uncertainty
Over the course of six assessment cycles, the IPCC has sought to develop and adopt a common framework for representing uncertainty, to emphasize what is known with certainty, and to represent clearly what is known with less confidence. The IPCC has used scenarios to characterize uncertainties, in particular those associated with greenhouse emissions trajectories and their socio-economic drivers. Many of these IPCC practices reflect concepts consistent with DMDU.
The first two IPCC reports, the First Assessment Report (FAR) in 1990 and the Second Assessment Report (SAR) in 1995, had rudimentary treatments of uncertainty. The FAR acknowledged uncertainties in forecasting future climate but expressed confidence that these uncertainties would be reduced. The SAR acknowledged the potential for ‘surprises’ arising from non-linearities in the climate system which might make some predictions inherently unreliable (Meah, 2019).
The FAR also used four relatively simple emissions scenarios, a business-as-usual reflecting relatively high emissions and three mitigation scenarios in which policy choices led to lower emissions, as shown in Figure 1. For the SAR, the IPCC developed a more sophisticated set of six emissions scenarios, called IS92, that explored the impact of various exogenous and uncertain socio-economic drivers, not explicitly policy driven (Leggett et al., 1992).
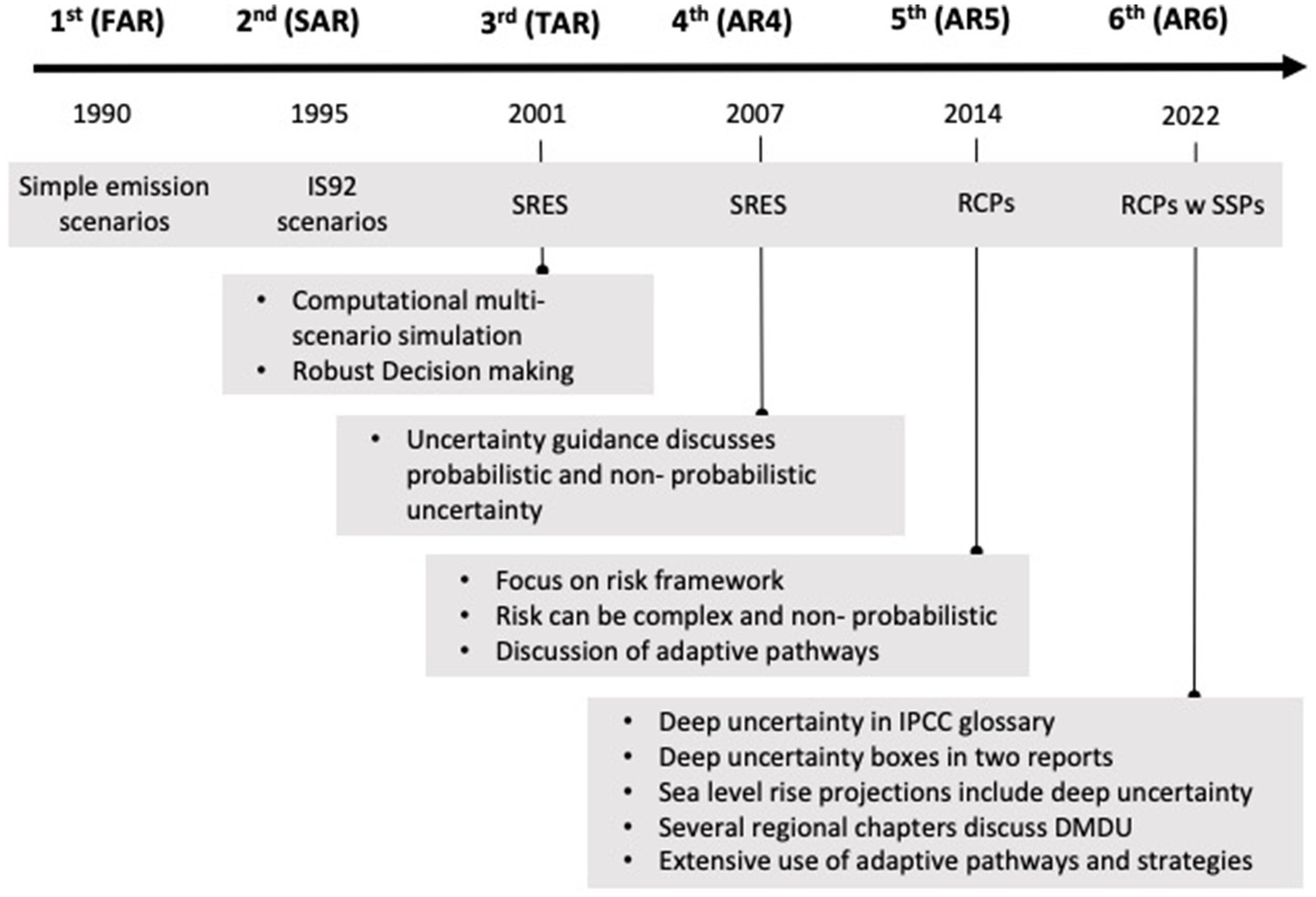
Figure 1. DMDU in the IPCC Timeline, showing use of scenarios (upper row) and introduction of other DMDU concepts across the assessment cycles.
The Third Assessment Report (TAR) in 2001 included several major advances in the treatment of uncertainty, including an uncertainty guidance, “burning embers” diagrams illustrating key risks associated with climate change, and a well-developed set of emissions scenarios.
The TAR was the first with an uncertainty guidance intended for all three working groups. In a chapter of the IPCC’s Guidance Papers on Cross Cutting Issues, Moss and Schneider (2000) provide recommendations for improving the consistency and clarity of uncertainty communications, particularly in relation to WGI (Science) and WGII (Impacts). They introduced the idea of levels of confidence, which they tied to ranges of probabilities (e.g., very low confidence is 0 to 5% while medium confidence is 33 to 67%) and the concept of evaluating certainty according to the amount of evidence available and the level of agreement among experts. Consistent with this guidance, the TAR provided the IPCC’s first attempt at a comprehensive assessment of uncertainties in climate projections and quantification of levels of confidence in predictions of climate change impacts.
The TAR included the so-called ‘burning embers’ diagrams to summarize and communicate information on key risks from climate change to human and natural systems (O’Neill et al., 2017; Zommers et al., 2020). The embers are a series of vertical rectangles color-coded to indicate increasing risk as a function of the increase in global mean temperature. The colors run from cool at the bottom (lower risk) to hot at the top (higher risk), hence the name burning embers. The embers diagrams are intended to help inform judgments about what constitutes dangerous anthropogenic interference in the climate system and have proved an enduring and powerful risk communication format for the IPCC. The TAR aggregated risk information from WGII into five categories, risks associated with: (1) unique and threatened systems, (2) extreme weather events, (3) distribution of impacts, (4) global aggregate impacts, and (5) large-scale singular events. The five categories, also known as “Reasons for Concern”, acknowledge that climate risk is inherently a multi-objective phenomenon, not appropriately aggregated into any single metric.
The Third Assessment Cycle also included the IPCC Special Report on Emissions Scenarios (Nakicenovic et al., 2000). This new set of so-called SRES emissions scenarios introduced two important and reoccurring themes into the IPCC discourse: storylines and the question of placing probabilities on scenarios. The SRES scenarios used storylines to combine both narrative and quantitative elements (the FAR and SAR scenarios were entirely quantitative). Narrative storylines can enhance communication and understanding of policy-relevant uncertainties, but it is often difficult to assess the self-consistency of individual scenario narratives or the extent to which a set of narratives span the full range of uncertainty. Using quantitative scenarios helps ensure self-consistency and provides a means to evaluate how well the scenarios span the uncertainty. But the Integrated Assessment Models (IAMs) used to create quantitative scenarios often contain biased assumptions and represent incomplete representations of reality, which constrain what uncertainties can be considered (Köberle et al., 2021). To balance among these challenges, the SRES process began with qualitative storylines which were then used to guide the development of quantitative scenarios by six leading IAMs (Nakicenovic et al., 2014).
Storylines also helped address a debate over the appropriateness of assigning probabilities to the scenarios. Despite pressures to assign such probabilities or identify “central” or “most likely” emission scenarios (Schneider, 2001; Morgan and Keith, 2008), the authors insisted that this would be misleading, given the intrinsic uncertainties and reflexive nature of technological and socio-economic systems (Grubler and Nakicenovic, 2001; Dessai and Hulme, 2004). Hence the SRES insisted on scenarios as expressing the plausible emission implications of different socio-economic “storylines”—with each storyline itself then encompassing a spread of emission trends associated with different numerical assumptions in the associated modeling.
The Fourth Assessment Report (AR4) in 2007 also used the SRES scenarios but featured an updated uncertainty guidance that aimed to ‘define common approaches and language that can be used broadly across working groups’ (IPCC, 2005). The guidance presented an uncertainty typology, consisting of unpredictability, structural uncertainty, and value uncertainty. The guidance also recommended the use of calibrated uncertainty language with specific terms (e.g., likely, virtually certain) assigned to specific probability ranges where the available evidence allowed such quantification. The guidance was permissive rather than prescriptive, however, and the three IPCC working groups continued to take different approaches to the treatment and communication of uncertainty reflecting both different types of evidence but also different approaches to uncertainty associated with different scientific disciplines.
The Fifth Assessment Report (AR5) in 2014 built on the AR4 guidance and sought to strengthen a common uncertainty guidance for all three working groups (Mach et al., 2017). Mastrandrea et al. (2010), the new AR5 guidance, established separate, calibrated scales for probabilistic estimates and for a non-probabilistic level of confidence in assessment findings. The guidance also provided a scale for reporting the level of evidence and agreement supporting assessment findings, in cases where the authors lacked sufficient information to judge confidence or probabilities. AR6 adopted the same approach (Ara Begum et al., 2022, Section 1.3.4; Chen et al., 2021, Box 1.1).
AR5 and AR6 also employed a new set of scenarios, organized around Representative Concentration Pathways (RCPs), which described alternative trajectories of radiative forcing over the 21st century (Nakicenovic et al., 2014). AR6 also employed Shared Socio-Economic Pathways (SSPs), which describe alternative socio-economic futures associated with the RCPs. These new scenarios aimed to address at least two challenges. First, the SSPs aimed to provide both WGII and WGIII with common set of more detailed socio-economic storylines in order to inform their assessments, in particular as the consideration of policy responses became more focused and regionally and sectorally detailed than had been the case in previous IPCC assessment cycles. Second, the RCPs aimed to shorten the timelines of the multi-year IPCC scenario process. Previously WGIII would develop emissions scenarios based on socio-economic modeling, pass the results along to the WGI climate modelers, who would then pass climate projections to WGII to examine impacts. In contrast, the RCPs were simultaneously passed to WGIII, who developed socio-economic scenarios consistent with various combinations of RCPs and the SSP storylines, and at the same time to WGI, who generated future climate trajectories.
2.2 Explicit IPCC treatment of DMDU
The TAR was the first to make explicit mention of DMDU-related concepts. The WGIII SPM (para 21) offers a statement that could come straight out of the DMDU literature: “Climate change decision-making is essentially a sequential process under general uncertainty…The relevant question is not “what is the best course for the next 100 years,” but rather “what is the best course for the near term given the expected long-term climate change and accompanying uncertainties.” As noted above, the TAR explicitly eschewed placing probabilities on the SRES scenarios for reasons consistent with DMDU. In addition, the WGIII chapter on decision making frameworks (Toth et al., 2001) includes a discussion of RDM (Sect 10.1.5) and multi-scenario simulation (Sect 10.1.4.4), as shown in Figure 1. The AR4 uncertainty guidance recommends considering all sources of uncertainty, paying attention to the tendency of experts to underestimate structural uncertainty, and using probabilistic and non-probabilistic representations as appropriate to represent the full range of uncertainties. The guidance also notes the approach of providing “information for decisions that would be robust in the sense of avoiding adverse outcomes for a wide range of future possibilities” IPCC, 2005), but without offering any suggestions for how to do so.
AR5 highlights several themes consistent with DMDU. The assessment cycle emphasized climate change as a risk management challenge and highlighted the concept of risk throughout the three working groups. Both the Working Group II and Working Group III reports had chapters focused on risk and decision making (Jones et al., 2014; Kunreuther et al., 2014), with the latter (WGIII) focused more on individual decision making, and the former (WGII) more focused on institutional decision-making. All three AR5 working group reports used a broad definition of risk as “the potential for consequences where something of value is at stake and where the outcome is uncertain, recognizing the diversity of values” (IPCC, 2014). The reports highlighted the narrow definition of (well-characterized) risk as the product of probability of occurrence and the impact of an event but acknowledged that it is not always possible to represent risks in this way (e.g., Jones et al., 2014).
The Fifth Assessment cycle began with the Special Report on Managing the Risks of Extreme Events and Disasters to Advance Climate Change Adaptation (SREX) (IPCC, 2012), a WGI and WGII collaboration which emphasized the importance of providing information on extreme events even when that information was not of high confidence. Accordingly, AR5 authors, relative to AR4, opened the aperture and offered far more statements with important, decision-relevant information that could only be provided at lower levels of confidence (Mach et al., 2017). In WGII Chapters 5, 16, 23, and 25 AR5 also introduces the idea of adaptation pathways (Haasnoot et al., 2019), an important DMDU method, as one way to manage risks even when they are not well-characterized. AR5 WGII Chapter 17 on the Economics of Adaptation also includes a discussion of non-probabilistic decision methodologies, with an explicit mention of robust decision making (Section 17.3.2.3).
AR6 was the first to explicitly highlight the term deep uncertainty. For the first time, the concept appeared in the IPCC glossary. Reports in the AR6 assessment cycle included two cross-chapter boxes focused on deep uncertainty including examples of how decision makers are using deep uncertainty management decision tools (Adler et al., 2022; Cozannet et al., 2022). The Special Report on Oceans and Cryosphere in a Changing Climate (SROCC) featured a cross-chapter box (Adler et al., 2019) which provided context on past IPCC uses of deep uncertainty and explored three case studies in which the scientific community had assessed and, in some cases, reduced deep uncertainties in order to advance risk management. The WGII Assessment Report featured a cross-chapter box focused on approaches and information requirements for managing climate change adaptation under conditions of deep uncertainty (Adler et al., 2022). The box identifies low regrets, adaptation pathways, and keeping options open as appropriate approaches as well as highlighting the value of monitoring to detect signals of change and the relevance of decision-making under deep uncertainty for navigating climate resilient development pathways. The box offers examples of deep uncertainty methods in the management of climate risks associated with landslides, sea level rise, flooding, and threats to biodiversity. The box concludes that “it is evident that the application of deep uncertainty methods is enabling decisions to be made in a timely manner that avoid foreseeable and undesirable outcomes and take opportunities as they arise (high confidence)”.
In a novel development, the presentation of sea level rise projections in AR6 WGI are organized to facilitate the development of robust adaptive strategies in WGII (Kopp et al., 2023). The SLR projections include a probabilistic description of the components of global and regional mean sea level rise driven by processes in which there is at least medium confidence, as well as quantitative assessments of sea-level rise projections incorporating ice-sheet processes in which there is low confidence. The latter are described with storylines that identify these physical processes in such a way as to facilitate the development of adaptive decision response strategies. In addition, WGI presented its sea-level rise projections both in the traditional form estimating the range of rise as a function of time, as well as in a new format showing the range of times at which a particular level of sea level rise might be experienced depending on the scenario. This later format is often viewed as more useful for policymakers seeking to craft adaptation strategies.
2.3 DMDU and WGIII
While WGIII has made less explicit use than WGII of the terms “deep uncertainty” and DMDU, the concepts have strongly influenced the evolution of the IPCC’s treatment of greenhouse gas mitigation. Intense debates and confrontations that first appeared in the SAR have only recently been addressed as AR6 adopted framings more consistent with DMDU.
The SAR structured its working groups so that WGII focused on technical and engineering dimensions of both adaptation and mitigation. WGIII focused on cross-cutting ‘Economic and Social Dimensions of Climate Change’ with the aim of addressing some governments’ interest in a cost–benefit analysis of the case for climate action.
The resulting effort to “monetize” damages and compare them against estimated cost of mitigation proved contentious and disruptive within the IPCC. There were widespread disagreements about assigning dollar values to risk and IPCC economists were unable to agree on appropriate discount rates for weighting future damages. The crux of the confrontations centered on equity, in particular how to assign a value of a statistical life in countries with vastly different levels of national wealth (Grubb et al., 2018).
The SAR convinced both governments and IPCC authors that global cost–benefit assessment was for the time being beyond the feasible scope of objective analysis. For the next four assessment cycles, interpretation of the overall UNFCCC objective of “avoiding dangerous interference” was largely left to the political process, informed by WGI and WGII. The TAR’s economic assessments focused on the cost effectiveness of emission reduction pathways consistent with the Kyoto Protocol’s targets, but also made explicit mention of DMDU concepts as noted above. AR4 WGIII further explored the many dimensions of such “global decision-making strategies.” Following introduction and a chapter devoted to “Frameworks,” Chapter 3 offered a brief and mainly qualitative discussion of the complexities, and a wide range of quantitative estimates of the social cost of carbon by 2030 (8-189 US$/tC02: p.233). At the same time, Weitzman (2011) argued that there could be no objective, evidence-based anticipatory estimate of climate impacts because any statistical cost–benefit would be dominated by tail risks, which could only be quantified when it was too late to avoid the consequences.
AR5 delved deeper into the fundamentals of decision-making. Chapter 2 was devoted to “integrated risk and uncertainty assessment of climate change response policies.” Chapter 3 covered Social, Economic, and Ethical Concepts and Methods. Together these chapters contained probably the most extensive discussion of decision-making literature to date, but notably lacked any clear policy-relevant conclusions.
AR6 WGIII offered several developments. For the first time, Chapter 1 was tasked to cover Introduction and Frameworks. Whereas previous reports addressed uncertainty primarily with decision and risk analytic frameworks, the AR6 WGIII frameworks section considered multiple analytic frameworks: economics, equity, transitions, and psychology and politics.
Second, the dramatic drop in the cost of wind, solar, and other low carbon technologies highlighted the importance of policy-driven innovation, along with the need and potential for major socio-economic transformation in multiple sectors (Grubb et al., 2021). Such transformation in complex systems both expands the challenge of addressing uncertainty and also highlights that uncertainty includes potential benefits, suggesting a focus on both risks and opportunities (Mercure et al., 2021).
Third, AR6 embedded co-benefits more deeply in the narrative, though only very partially in the modeling. This disjuncture owed to the diversity of perspectives (co-benefits are often non-monetary and vary according to particular national contexts and policies) and the intrinsic uncertainty. Nonetheless, the risk and opportunity framing helped to defuse aspects of the traditional north–south dialogue around costs and burden-sharing, by focusing on the emerging opportunities for shifting development pathways towards sustainability, and the enabling conditions required for this, with a move away from focus purely on policy instruments to the integrated governance required to make smart mitigation decisions in this more complex world (notably AR6 WGIII, Chapters 4, 13 and 14).
AR6, therefore, itself marked a point of transition in how to approach DMDU for mitigation in a world where the major uncertainties were not just exogenous but comprise endogenous discovery and creation of opportunities to cut emissions, and how this may also help to overcome the political obstacles to low carbon development for countries at all stages of development.
3 DMDU and IPCC key concepts
Several key concepts in the IPCC Sixth Assessment report intersect with DMDU. These include risk; scenarios; treatment of uncertainty in IPCC uncertainty guidances; storylines and low likelihood, high impact (LLHI) outcomes; and both adaptation and climate resilient development pathways. This section will address each in turn.
3.1 Risk
The IPCC views risk management as a central framing for the climate change challenge (Ara Begum et al., 2022, Sect 1.2.1; Grubb et al., 2022, Sect 1.2.2). The IPCC highlights risk because it provides a useful frame for linking scientific and technical assessment to consequences of concern to people and natural systems, characterizing the uncertainty in such assessments, and linking these understandings to potential solutions and decision processes.
AR5 introduced the risk propeller graphic to integrate the WGI and WGII views of risk (Lavell et al., 2012, Figure 1-1). The risk propeller shows risk emerging from the interaction of hazard, exposure, and vulnerability; that climate change directly affects hazards; and that exposure and vulnerability arise from socioeconomic processes which in turn are affected by climate change.
The original propeller diagram thus focused largely on what the financial literature calls “physical risks”. However, as noted above, decision makers care not only about the risks caused by climate change but also the risk generated by climate action. In responding to physical risks, humans can create new risks or exacerbate existing ones. Human response may also fail to achieve their objectives, and thus climate action may pose economic, reputational and political risks. AR6 thus added human responses as a fourth component of climate-related risk (Ara Begum et al., 2022, Figure 1.5). This four-blade risk propeller also aims to better incorporate the multiple WGIII dimensions of risk that typically are not directly influenced by the three classical drivers of hazards, exposure and vulnerability, which the financial literature broadly refers to as “transition risks” (Reisinger et al., 2020; Simpson et al., 2021).
AR6 employs risk consistent with the concept of deep uncertainty, adopting a broad core definition of risk as IPCC (2023):
“The potential for adverse consequences for human or ecological systems, recognizing the diversity of values and objectives associated with such systems. Risks can arise from potential impacts of climate change as well as human responses to climate-related risks.”
This broad definition explicitly includes but goes beyond a narrow definition of risk as the numeric product of an event’s likelihood and its consequence, acknowledging that both an events’ magnitude and likelihood may be uncertain and that risk in some cases cannot be quantified (Reisinger et al., 2020, p. 5). AR6 also explicitly adopts a multi-scenario, multi-objective view of risk, noting that it can affect a diversity of values and emphasizes that different individuals will view these consequences from different points of view (Reisinger et al., 2020, p. 6).
The AR6 understanding of climate-related risk highlights conditions consistent with deep uncertainty. To represent the deep uncertainty in many of the burning embers diagrams, the transitions among different levels are shown as blurry boundaries and the diagrams indicate the level of confidence associated with the imprecise location of each boundary (Zommers et al., 2020). As discussed below, the reports describe low-likelihood, high-impact outcomes, such as higher than expected climate sensitivity or rapid polar ice-sheet loss, whose probability of occurrence and timing are either not well-known or assessed to be low. The AR6 guidance on risk (Reisinger et al., 2020, p. 5) notes that risk arises from dynamic interactions among biophysical and human systems and may change over time and space due to each of these hard-to-predict factors. WGII emphasizes the importance of non-linear system behaviors and of complex, compound, and cascading climate risks (Simpson et al., 2021) such that “the challenge of assessing risks of climate change is not well bounded, will be framed differently by individuals and groups, involves large and deep uncertainties, and will have unclear solutions and pathways to solutions” (Ara Begum et al., 2022, Section 1.3.1.2). That some type of transformation of natural or human systems is now inevitable also heightens the salience of deep uncertainty to the assessment and management of climate risk.
Critiques of IPCC risk assessment argue that there has been too much focus on quantified probabilities, even when the evidence does not support such judgments; insufficient use of imprecise probabilities; too much focus on mean estimates rather than information on tails of distributions; and insufficiently described scientific knowledge that supports low-confidence judgments (Aven and Renn, 2015; Aven, 2019). Others criticize the IAMs used to inform WGIII assessments for their focus on techniques that are mostly based on changes from an assumed “optimal” reference trajectory, using concepts derived from equilibrium theory, with little representation of the actual process and dynamics of economic evolution and transformational change - and thereby tend to underplay the significance of heavy-tailed distributions of probability or systemic change (Grubb et al., 2021; Mercure et al., 2021). As practiced, climate risk management can evolve into an overly rationalist, expert-driven view of decision making (Tangney, 2019a,b). In such cases, risk assessment can hide value-laden judgments within what is claimed as expert judgment and can fail to either describe policy-makers actual decision processes or provide them the information they need most to make good decisions (Jones et al., 2014). DMDU concepts are designed to address such critiques, which may be one of the reasons for DMDU’s increasing adoption within the AR6 treatment of risk.
3.2 Scenarios
Since the first assessment cycle, the IPCC has used scenarios to characterize socio-economic uncertainties. The radiative forcing that drives climate change depends on anthropogenic emissions of greenhouse gases, which in turn depend on hard-to-predict human behaviors over long periods of time. To characterize these socio-economic uncertainties, the IPCC has used sets of emissions scenarios, which then serve as primary inputs into the general circulation climate models that project future climate and support evaluation of human actions that affect future climate.
The AR6 glossary defines a “scenario” as “a plausible description of how the future may develop based on a coherent and internally consistent set of assumptions about key driving forces (e.g., rate of technological change, prices) and relationships.” The definition goes on to note that “scenarios are neither predictions nor forecasts but are used to provide a view of the implications of developments and actions.” There exist many definitions of scenarios (Parson et al., 2007), but this current definition is consistent with how the IPCC has used the concept over six assessment cycles.
Scenarios also play an important role in the DMDU literature. There is significant alignment, though some differences in how the IPCC and DMDU use scenarios.
The DMDU literature draws from scenario analysis two key concepts: choosing scenarios to stress test proposed policies (Lempert, 2013) and using a multiplicity of plausible futures as a means to characterize and communicate deep uncertainty (Lempert et al., 2003; Lempert, 2019). For instance, DMDU methods often use scenario approaches to enhance stakeholder engagement, even when such analysis ultimately presents probabilities at the end of the analysis (Parker et al., 2015).
Similarly to the scenario literature, DMDU argues that scenarios can facilitate communication with information users, in particular when policies or the science is contested (Lempert, 2013). By focusing on plausibility rather than probability, scenarios can help users expand the range of futures they consider, allowing them to contemplate their choices from a wider range of views and vantages, including uncomfortable or unexpected futures (Wack, 1985; Schoemaker, 1993; Gong et al., 2017). Focusing on plausibility rather than probability also helps scenarios engage with audiences not necessarily eager to have their vantage expanded. By representing different visions of the future without privileging among them, scenarios can offer comfortable entry points that are more resonant with users before contemplating scenarios that they find more dissonant. As noted above, the IPCC has generally eschewed placing probabilities on scenarios. When DMDU employs probabilities on scenarios, it generally does so at the end of the analysis, rather than the beginning, and only at the point when decision makers are ready to make a final choice among decision options (Lempert, 2019).
As a key example of the scenario stress test concept, Dynamic Adaptive Policy Pathways (DAPP) identifies the general conditions under which a proposed policy might fail, called an “adaptation tipping point” or “adaptation threshold” (Haasnoot et al., 2013, 2019). For instance, a coastal management plan might fail if global sea level rise exceeds some threshold (e.g., 30 cm or 1 meter). Scenarios are then used to identify the factors that might lead to reaching a threshold at an earlier or later point in time. More generally, such scenarios can be used to stress-test a range of near-term actions and longer-term adaptation options for the conditions under which they fail to deliver on objectives and methods to consider lead time needed for decision makers (Slangen et al., 2022).
RDM generates scenarios as one product of the analysis, rather than as initial inputs into the analysis. It draws from scenario analysis the concept of organizing information about the future into a small number of distinct, decision-relevant cases (Lempert, 2019). The Intuitive Logics school of scenario analysis (Schwartz, 1996) uses qualitative methods to craft a handful of scenarios, each distinguished by a small number of key uncertain factors. Similarly, RDM uses quantitative “scenario discovery” algorithms applied to large databases of simulation model results to identify the handful of uncertain parameters that best distinguish future conditions in which a proposed meets and misses its goals (Bryant and Lempert, 2010; Kwakkel, 2019). Such clusters can illuminate the vulnerabilities of proposed policies (Lempert, 2013), suggest a handful of diverse cases against which to stress test proposed policies (Carlsen et al., 2016), or suggest a small set of cases that reveal different and policy-relevant behaviors of the system (Lamontagne et al., 2018).
Risk-Opportunity Analysis, a DMDU-adjacent approach for evaluating policy-driven, low-carbon innovation, adopts scenarios as a means to explore risks and opportunities that might emerge from such policy action (Grubb et al., 2021; Mercure et al., 2021). The approach identifies scenarios using a cross-over analysis (Guillaume et al., 2016) that seeks to identify thresholds where the combination of technology and policies (e.g., subsidies) create cost-parity between one technology pathway and another. Reaching such a threshold can catalyze reinforcing change via pushing (e.g., R&D investments to decrease cost) and then market pull (e.g., learning by doing), launching a transition towards a low carbon lower cost society.
AR6 uses scenarios consistently with DMDU but does not employ the full breadth of DMDU scenario approaches. The WGI and WGII assessments largely focused on the idea of choosing a small set of diverse cases designed to facilitate exploration and comparisons among multiple studies. The resulting scenarios are used as inputs to analyses rather than products of analysis that have explored a wide range of relevant futures.
To explore the implications of alternative emission trajectories and associated socio-economic futures, AR6 WGI and WGII employed a standard ‘core set’ of five scenarios, each consisting of an SSP paired with an RCP (Rose et al., 2022). These combinations are SSP1-1.9, SSP1-2.6, SSP2-4.5, SSP3-7.0, and SSP5-8.5, where the first number labels the SSP and the second labels the RCP. The WGI report selected these combinations to fill gaps identified in the RCPs used in AR5. WG1 reports projections of future climate conditions contingent on these five scenarios, mostly for specific points in the future (2050 and 2100) but also as time series with sometimes longer time horizons as done for sea-level rise. Uncertainties within a scenario are represented as bandwidth using the likely range (33–66% probability). For sea level rise, uncertainties were also expressed in terms of a time period at which a specific sea level rise value will be exceeded under a specific scenario (based on Slangen et al., 2022). WGII employs these scenarios and associated climate projections to explore impacts and adaptation strategies over a range of conditions and to enhance the ability to compare and contrast a multiple analysis conducted by different research groups.
WGIII considered a much larger set of scenarios, developed by different modeling teams, to explore a wide range of potential pathways for energy, land use, and other systems and to understand mitigation options and milestones to limit global warming to specific levels. In total, more than 1,200 individual scenarios were assessed in the AR6 WGIII report for their quantitative climate outcomes and used to identify common features as well as unique, scenario-specific aspects of different mitigation pathways such as reliance on carbon dioxide removal versus the pace of emission reductions in specific sectors. To complement this broad scenario approach, the WGIII assessment also employed what it calls Illustrative Mitigation Pathways (IMPs). This small sample of scenarios was chosen to illustrate a policy-relevant and contrasting range of systems behaviors (Grubb et al., 2022, Sect 1.5; Riahi et al., 2022, Sect 3.1.3), similar to the DMDU approach of scenario discovery. But the IMPs were chosen by expert judgment to illustrate specific alternative policy narratives rather than with the assistance of DMDU statistical tools that would cluster these many futures into what DMDU analyses would consider policy-relevant scenarios (Lamontagne et al., 2018).
3.3 Uncertainty guidance
The IPCC uncertainty guidance aims to ensure a consistent treatment of uncertainties in and across IPCC reports by providing authors with a calibrated language for evaluating and communicating the degree of certainty in assessment findings (Mastrandrea et al., 2010). As such, the degrees of certainty in the guidance are related to, but not identical with the levels of uncertainty in the deep uncertainty literature.
The AR5 guidance, which was also used in AR6, defines a three-stage process for representing and communicating the degree of certainty and confidence. In ascending order these are: evidence and agreement, level of confidence, and probabilistic representations. As illustrated in the upper panel of Figure 2, authors are instructed to use the highest-level description appropriate for the available evidence, which includes the literature and elicited expert judgment of the authors (Ara Begum et al., 2022, Sect 1.3.4).
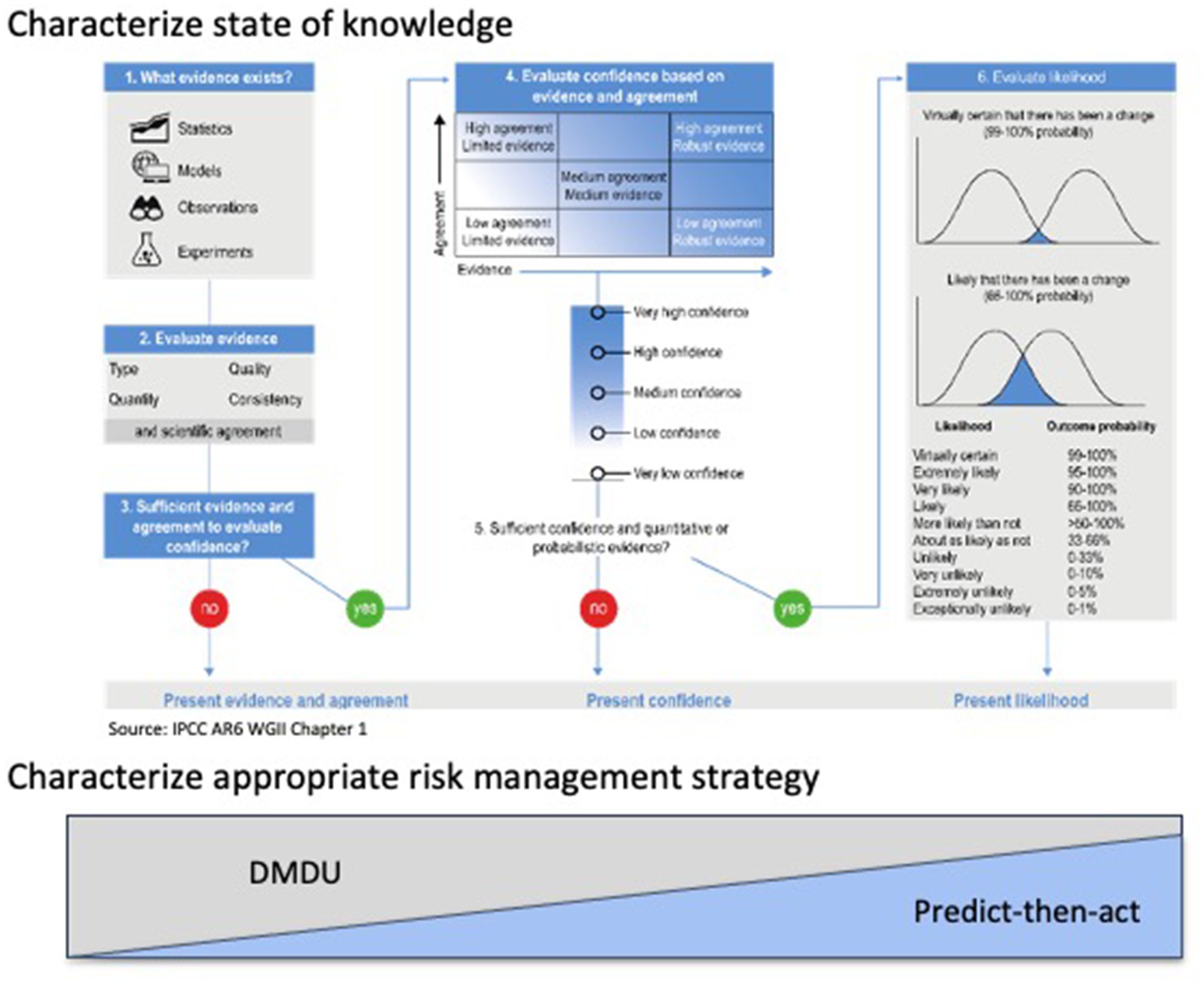
Figure 2. DMDU and IPCC uncertainty guidance, showing AR6 guidance for characterizing the state of knowledge (upper panel) and appropriate type of risk management strategies for various states of knowledge (lower panel).
As shown in Figure 2, the assessment of scientific evidence begins with the evaluation of the type of evidence available and the degree of agreement in the literature. Authors should rank evidence as more robust when there exist multiple lines of consistent, independent, and high-quality evidence and larger amount of literature. Authors should rank higher agreement when there exist a single, rather than multiple, competing explanations in the literature. If sufficient evidence and agreement exists, authors can choose to integrate this information into a level of confidence, ranging from very high to very low, using a five-point scale. If uncertainties can be quantified, the guidance provides calibrated uncertainty language for ten different probability intervals, including virtually certain (99–100%), likely (66–100%), and about as likely as not (33–66%).
In practice, likelihood judgments were most common in AR5 WGI, confidence most common in AR5 WGII, and evidence/agreement most common in AR5 WGIII (Mach et al., 2017). AR6 achieved greater harmonization, with confidence levels employed across all three Working Groups where probabilistic statements were not possible. In practice however, following the uncertainty guidance is becoming increasingly challenging given the rapidly increasing amount of literature that makes a transparent assessment of the quality of each individual study and its contribution to the overall evidence base difficult to achieve.
Importantly, levels of confidence are not simply a qualitative proxy for levels of certainty. If a given finding is judged to be “likely” (judged to be true with >66% probability), then by definition the opposite outcome is unlikely (<33% probability). By contrast, if a finding is judged to have “low confidence”, this does not imply that the opposite outcome has “high confidence”. A “low confidence” finding still implies that this is the best statement that can be made based on current knowledge, but it signals explicitly that this knowledge is not solid and thus could change in light of future discoveries. Levels of confidence and probabilistic statements are thus complementary rather than a strictly hierarchical means of communicating degrees of scientific understanding and robustness of conclusions. The use of probabilistic statements generally implies at least a high level of confidence in the scientific understanding of relevant processes and drivers of future change. WGI did, however, report probabilistic sea level rise projections with only medium confidence. This lower level of confidence was stated explicitly alongside the probabilistic projection.
There exist several uncertainty taxonomies in the literature, but they all describe a continuum from well-characterized or shallow uncertainty to deep uncertainty to total ignorance (Walker et al., 2003; Kwakkel et al., 2010; Janzwood, 2022). Well-characterized uncertainty can be confidently represented by single joint probability distributions over a known set of future states of the world. Deep uncertainty includes cases in which it is not possible to define probabilities with any confidence as well as cases in which probabilities estimates are sufficiently imprecise to suggest a wide range of policy responses. Ignorance is an extreme case of deep uncertainty, when very little is known about future states of the world, the consequences of actions, and there is little or no evidence to support probabilistic estimates. The Risk-Opportunity Analysis literature highlights systemic risk (discussed above), fundamental uncertainty, and heavy-tailed distributions (Mercure et al., 2021). Fundamental uncertainty is similar to ignorance in the deep uncertainty literature. Heavy-tailed distributions involve events with very high impacts which are sufficiently likely so that the variance of the distribution increases with sample size and the average converges only slowly, if at all and often introduce deep uncertainty because optimal policy choices may be so sensitive to even small amounts of imprecision in the probability estimates of the tails.
The deep uncertainty literature’s taxonomies of uncertainty, in particular the distinction between DMDU and predict-then-act approaches to decision-making, correlate with but are not identical to the distinction between confidence and likelihood in the IPCC uncertainty guidance. Predict-then-act risk management – in particular optimum policies – will often be more appropriate in situations in which the relevant information is expressed as probabilities. DMDU approaches to risk management – such as low regret options and adaptive pathways – will often be more appropriate when the relevant information is expressed as evidence/agreement or levels of confidence.
However, these distinctions are not identical because they have different purposes. The uncertainty guidance in the IPCC aims to differentiate among states of knowledge. As noted in the lower panel of Figure 2, deep uncertainty taxonomies aim to differentiate among conditions best addressed with alternative risk management strategies (also see Marchau et al., 2019b, Figure 1.3). The correlation is therefore not perfect. In some cases, a DMDU response may be appropriate even when knowledge is communicated with likelihoods. This can occur when the probability intervals are sufficiently large relative to the available policy responses. For instance, scientists may judge a certain extreme precipitation event in a particular river basin to be extremely unlikely, that is, to have a probability between 0 to 5% using the IPCC guidance’s calibrated uncertainty language. Decision makers involved with flood management in that river basin may determine that they would respond very differently if they were confident that the likelihood of the extreme event was 1% rather than 5%, and thus pursue a DMDU rather than a predict-then-act approach.
Conversely, a predict-then-act approach may be appropriate even in some low impact situations where knowledge is communicated only with levels of confidence. For instance, scientists may have high confidence that rainfall intensities and wind speeds in the extreme event in the river basin would not change significantly compared to the historic climate record, but insufficient evidence to create new probability distributions for those climate parameters. The structural engineers might nonetheless use predict-then-act analyses where the risk of lock-in is low, using whatever future climate parameters are available for their region.
It is useful to note two other differences between the IPCC guidance degrees of certainty and DMDU uncertainty taxonomies. First, the DMDU literature often characterizes uncertainty by identifying policy-relevant thresholds in combinations of key parameters, as noted in the scenario discussion above (also, Brown et al. 2019). The IPCC guidance on degrees of certainty can still be used to characterize knowledge about the location of such thresholds and whether the threshold may be exceeded and when (Sriver et al., 2018). But these judgments are contextualized by their implications for alternative decisions. Second, recent literature has suggested adding reducibility of deep uncertainty as a second dimension of the deep uncertainty taxonomy. One such scale differentiates among practically reducible, practically irreducible, and irreducible deep uncertainties (Janzwood, 2022). Practically reducible describes the situation in which it is possible to define the evidence needed to move from deep to well-characterized uncertainty and to obtain that evidence with reasonable effort and in reasonable time. Practically irreducible describes the situation in which the needed evidence can be defined but it is not possible to obtain it with reasonable effort or in reasonable time. Irreducible deep uncertainty describes the situation in which it is not possible to define or obtain evidence that would reduce deep to well-characterized uncertainty, such as is the case with many complex biophysical or socioeconomic systems whose behaviors may be understood but not predicted (Mitchell, 2009). As noted in Section IV, these differences between the AR5 IPCC guidance and DMDU taxonomies may have implications for AR7.
3.4 Storylines and low-likelihood, high impact outcomes
The AR6 WGI report employs two framing concepts related to deep uncertainty: storylines and LLHI outcomes. Similarly to the storylines in the SRES scenarios, physical climate storylines (Shepherd et al., 2018) are narratives with unquantified likelihood describing the “self-consistent and possible unfolding of a physical trajectory of the climate system” (Chen et al., 2021). One WGI application of storylines is the description of low-likelihood, high-impact outcomes, described as outcomes “whose probability of occurrence is low or not well known (as in the context of deep uncertainty) but whose potential impacts on society and ecosystems could be high” (IPCC, 2021a,b). LLHI storylines are used to describe climatic conditions in a world with climate sensitivity above the very likely range (Lee et al., 2021) and in an unknown-likelihood world with rapid polar ice-sheet losses and consequently high-end sea-level rise (Fox-Kemper et al., 2021).
The high-end sea-level rise case is particularly well developed for the purpose of informing adaptation decision-making under deep uncertainty (Kopp et al., 2023). As in AR5, AR6 provides projections of likely future sea-level change incorporating only physical processes with respect to which there is a medium level of agreement and thus medium confidence. But alongside these probabilistic projections AR6 also provides quantitative projections that use the limited evidence available to assess potential contributions from ice-sheet processes about which there is a low level of agreement, as shown in Figure 3. These processes include “earlier-than-projected disintegration of marine ice shelves, the abrupt, widespread onset of marine ice sheet instability and marine ice cliff instability around Antarctica, and faster-than-projected changes in the surface mass balance and discharge from Greenland” (Fox-Kemper et al., 2021). AR6 used DMDU to avoid neglect of these unknown-likelihood, high-impact outcomes by providing in key figures and tables its low confidence projections alongside projections of the likely contributions from medium confidence processes, both in the relevant WGI report chapters and the WGI SPM, which was then carried forward into the WGII assessment. The WGII assessment used these projections to stress test and design options and pathways shown in Figure 3 (lower right panel) for their robustness (Cozannet et al., 2022; Glavovic et al., 2022). The figure illustrates how adaptation options can be sequenced and combined as sea level rises and options may not sufficiently reduce risk or may encounter other soft or hard thresholds (e.g., resources, space, time limits). Based on the literature different reasons to further adapt have been indicated (numbers). Some pathways are more appropriate to mega-cities while others fit better to rural areas.
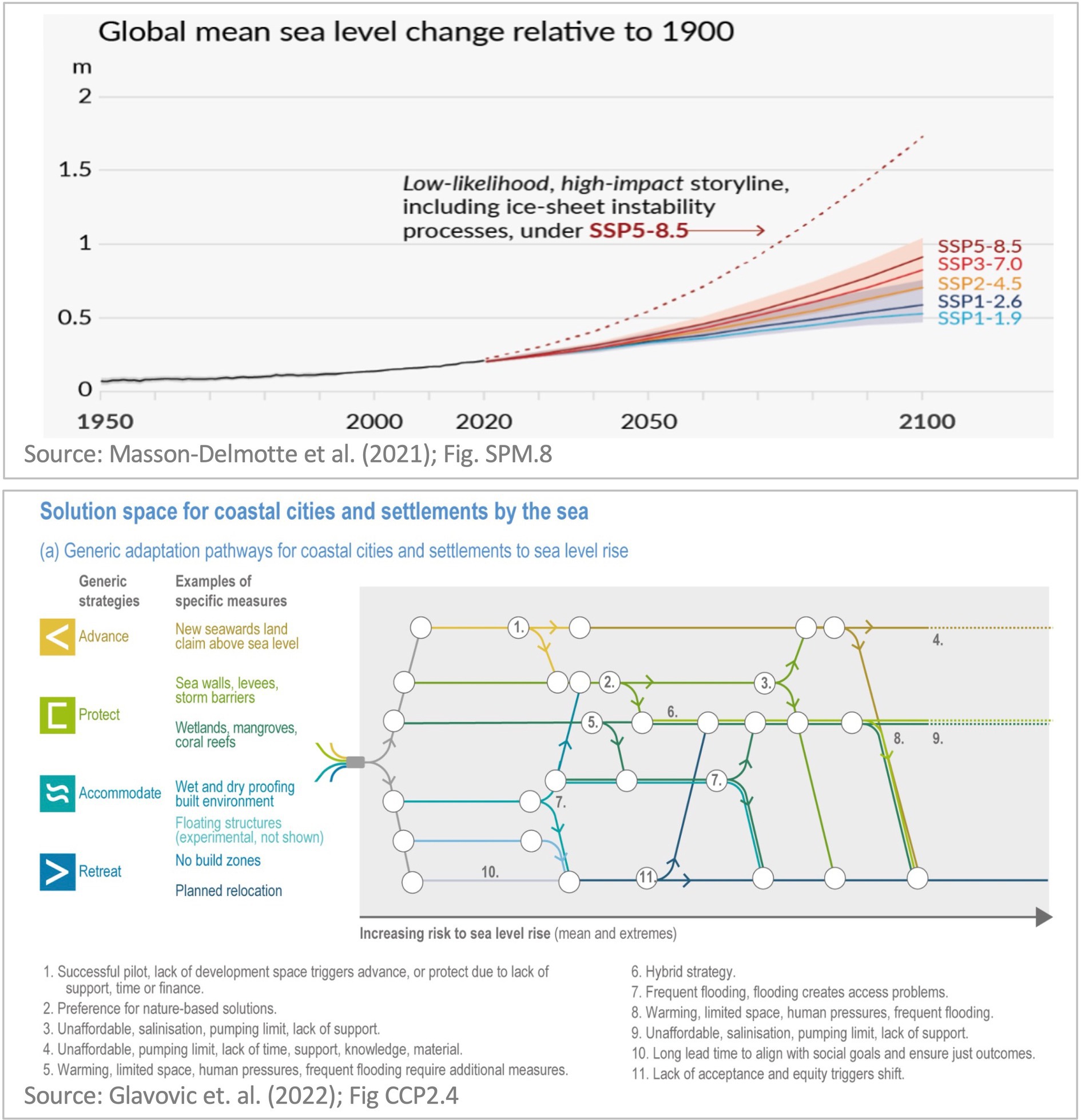
Figure 3. IPCC sea level risk projections and adaptive pathways, showing AR6 WGI sea level rise projections with a low-likelihood, high-impact storyline (upper left) and illustrative adaptive pathways for coastal cities and settlements designed to employ such information to stress-test the options and pathways for robust decision making under uncertainty. As sea levels rise solutions need to be sequenced or combined and may reach soft or hard thresholds and limits which are reasons to further adapt (indicated with numbers) (lower right).
AR6 WGIII uses storylines associated with its IMP scenarios to describe the particular sets of drivers or policy preferences needed to achieve an overall mitigation outcome. Examples include pathways that: rely on reduction of demand and increased efficiency; prioritize the deployment of renewable energy to meet rising demand; accelerate actions to reduce emissions of non-CO2 emissions; or rely on carbon dioxide removal to compensate for on-going emissions. Similarly, different scenario categories explore options to limit warming to 1.5°C either with only limited overshoot (implying greater near-term actions to reduce emissions), or with higher overshoot (implying less near-term actions but greater deployment of existing and novel technologies to remove carbon dioxide from the atmosphere after 2050, along with risks and adverse side-effects associated with such approaches). The use of different mitigation pathways and storylines also serves to illustrate the extent to which non-achievement of a particular mitigation option in one sector would require enhanced action in other sectors or by other actors to achieve the same overall mitigation outcome. These approaches were used both in the assessment of global scenarios (Riahi et al., 2022) and in sectoral chapters that evaluated the potential for specific mitigation technologies within a given sector, or the complementary roles of demand-management (Creutzig et al., 2022).
Alongside the use of scenarios and storylines, WGIII also adopted a more structured approach to assessing the feasibility of different mitigation strategies and pathways, to highlight risks arising to policies that rely on the successful deployment of various mitigation options. This feasibility assessment systematically evaluates barriers and enablers of implementing mitigation options across six dimensions of feasibility (geophysical, environmental-ecological, technological, economic, socio-cultural, and institutional). While this approach does not avoid the reliance on expert judgment, it forces a more systematic and transparent evaluation across a consistent set of indicators that reduces bias and enhances comparability (Pathak et al., 2022, Box TS.15; Riahi et al., 2022).
3.5 Adaptation and climate resilient development pathways
DMDU emphasizes the importance of strategies designed to balance among competing objectives while adjusting over time to new information. DMDU also aims to provide concepts and tools for the management of complex systems (Lempert, 2002; Mitchell, 2009). AR6 engages with these themes in its discussions of adaptation pathways, solution spaces, and climate resilient development pathways.
AR6 WGII describes adaptation pathways as breaking “adaptation planning into manageable steps based on near-term, low-regret actions and aligning adaptation choices with societal goals that account for changing risk, interests and values, uncertain futures,” and long-term commitments (Technical Summary, D.7). While AR5 included this adaptation pathways concept, AR6 presents illustrative/generic adaptation pathways in several WGII chapters including Europe (Chap 13), Australasia (Chap 11), Oceans (Chap 3), Cities and Settlements by the Sea (Cross Chapter Paper 2), and in the Technical Summary. The WGII report presents adaptation pathways for heat, sea level rise, drought, river flooding and ecosystems, demonstrating how pathways can diverge into completely different futures as global warming increases and that measures for different risks interact resulting in trade-offs (Muccione et al., 2024). As shown in Figure 3, these pathways highlight how DMDU methods can link uncertainty considerations between WGI and WGII’s areas of concern. In some cases the pathways are laid out against warming level (similar to burning ember diagrams), which can be mapped against scenarios to assess the timing, similar to the DMDU approaches of stress-testing (e.g., scenario discovery, decision scaling and adaptation tipping points).
AR6 WGII also introduces the concept of solution space to help assess the role of path dependence in complex systems and, in particular, how near-term actions can expand or contract the options available to future decision makers (Haasnoot et al., 2020). Defined “as the space within which opportunities and constraints determine why, how, when and who adapts to climate risks” (Ara Begum et al., 2022, Section 1.4.2), the solution space is related to the idea of keeping options open and describes how human choices and exogenous changes expand and contract the set of future available effective, feasible, and just decision options. Mapping the solution space over time helps to illuminate the long-term effectiveness of measures which in some cases is increasingly challenged by the rate and magnitude of climate change impacts and in some cases by a reinforcing path dependency. For instance, flood defense and water supply attract new human settlements which require further protection and supply (Bednar-Friedl et al., 2022). As such the pathways concept also helps describe both maladaptation, in which lock-in of decisions creates greater residual risk in the future, and the limits and lifetimes of different adaptation options. The WGII SPM states that adaptation can be more effective if combined, sequenced, planned well ahead, aligned with sociocultural values and development priorities, and underpinned by inclusive community engagement processes. The SPM explicitly mentions DMDU approaches as means to minimize maladaptation through flexible pathways planning that accounts for the uncertainty about the rate and magnitude of climate risk.
AR6 employs the concepts of climate resilient development (CRD) and climate resilient development pathways (CRDP) to emphasize the importance of aligning with principles of justice; the urgency of climate action in this next decade; and that mitigation, adaptation, and sustainable development are more effective if pursued together with integrated solutions (WGII SPM.D). AR5 first introduced the concept of climate resilient pathways, which was further addressed in SR1.5. AR6 makes more extensive use of the concept, emphasizing that achieving CRD requires large and equitable transitions of human and natural systems and that the process of achieving such transitions requires effective management of complex systems under conditions of deep uncertainty. AR6 draws on DMDU concepts to assess how this might be achieved.
AR6 defines CRD as “the process of implementing greenhouse gas mitigation and adaptation measures to support sustainable development for all” (IPCC, 2023). AR6 defines CRDP as trajectories in time reflecting a particular sequence of actions and consequences against background of autonomous developments leading to a specific future situation (Schipper et al., 2022, Sect 18.1.2). The SGII SPM (Figure SPM.5) illustrates potential CRDPs with a decision tree whose branches emerge as the result of cumulative societal choices over time by many different actors in government, business, civic organizations and households at the individual, community, national and international levels. All pathways are subject to hard-to-predict shocks, both adverse (e.g., climate disasters) and beneficial (e.g., new technologies or shifts in human behaviors) (Ara Begum et al., 2022, Sect 1.5.3). Successful pathways pursue integrative and transformative solutions and include near-term actions which expand the future solution space for mitigation and adaptation. An important enabler for CRD is the capacity of iterative risk management and the ability to “identify ‘low regret’ options that enable mitigation and adaptation in the face of change, complexity, deep uncertainty, and divergent views” (WGII SPM.D.2.1).
4 Guidance for AR7
AR6 benefited from significant engagement with DMDU. Consistent with DMDU concepts and methods, the Sixth Assessment cycle adopted a broad, multi-scenario and multi-objective view of risk in which the magnitude and likelihood of an event and its potential consequences may be uncertain and, in some cases, cannot be quantified with any confidence. AR6 also emphasizes that risk is often best evaluated from the perspective of a diversity of values and thus inappropriately represented by any single framing. Employing DMDU concepts enabled AR6 to provide policy-relevant assessments of phenomena about which only low confidence information exists, such as high-end sea level rise, as well as better address climate policy challenges such as low probability high impact events; complex and cascading risks, systems transitions and transformations; and the wide range of perspectives that different communities bring to questions of justice and the climate challenge. AR6 used a DMDU lens to assess risk management approaches appropriate for conditions of deep uncertainty, including low regrets options and adaptive pathways; to use scenarios to stress test proposed policies and highlight key drivers of policy success or failure; and to organize scientific information to facilitate the development of such DMDU-consistent strategies.
AR7 will face challenges even more daunting than those of AR6, including a more extensive focus on solutions, both in planning and implementation; reaching a wider policy audience; and engaging more fully with transitions and transformation of complex systems. Fortunately, AR6 provides AR7 with a firm foundation to expand the use of DMDU to help address these challenges. Here we offer some suggestions, based on our experience as AR6 authors, on how AR7 might best employ DMDU.
First, AR7 could update its uncertainty and risk guidance with a more explicit focus on DMDU, building on AR6 risk guidance and the DMDU-focused cross chapter boxes in the AR6 special and assessment reports. Such an AR7 guidance note could more clearly distinguish between uncertainty characterization, the focus of the AR5 uncertainty guidance (Mastrandrea et al., 2010), and the assessment of risk management strategies appropriate for conditions of deep uncertainty, a subject of the DMDU cross chapter box in AR6 WGII (Adler et al., 2022). The new guidance could describe low-regrets, keeping options open, adaptive pathways, and adaptive management and other strategies focused on policy experimentation; give examples of such strategies; and provide suggestions for how to evaluate them. A challenge will be to ensure such a guidance is applicable across both the WGII and WGIII domains, where both the nature of uncertainty and risk can differ markedly and are also treated differently in the underlying literature.
A more DMDU-focused guidance note could also help the IPCC refocus from an assessment of risk towards an assessment designed to inform appropriate risk management strategies. Rather than focus on assessing all new climate-related science, DMDU suggests focusing on decision-relevant information that can inform the most robust actions given what is and is not known. For instance, the storyline focus for high-end sea level rise adopted by AR6 WGI and WGII aimed to inform adaptive pathways and the WGIII scenario approaches were designed to illuminate key decarbonization policy choices. While the IPCC should remain policy-relevant, not policy-prescriptive, much can be done to organize available information to assist policy-makers craft appropriate risk management strategies especially when uncertainties are deep. The DMDU guidance could support all working groups in discussing the certainties and uncertainties. For example, while the science may be uncertain regarding impacts at a particular time-horizon, some impacts are committed so that there is high confidence these impacts will occur, even if the timing remains uncertain. Similarly, while policymakers have choices about their preferred portfolio of mitigation approaches, some types of mitigation are necessary to stay below 2°C, let alone 1.5°C. This helps to present not only uncertainties and related solutions, but also certainties and consequences for solutions.
A focus on informing risk management strategies under deep uncertainty may also help to prioritize topics that AR7 should address. For WGII, robust strategies often involve low regrets options that perform well over a wide range of uncertainty and adaptive pathways designed to be monitored and adjusted over time, and taking near-term actions designed to expand the future solution space (Haasnoot et al., 2020; Adler et al., 2022). Some parts of WGIII have emphasized experimentation that promotes both technological and institutional learning as a crucial risk management strategy under deep uncertainty, and to emphasize the range of options for action as well as their interdependencies. However, in AR7, WGIII may also have to confront the consequences of actions not taken. For instance, limiting warming to 1.5°C may no longer be feasible without temporary overshoot, given the lack of mitigation actions to date. While DMDU approaches often seek pathways that keep options open for as long as possible, a full assessment should also clearly indicate where options are no longer open and specific DMDU options have effectively been taken off the table (Reisinger and Geden, 2023).
AR6 addressed the need for experimentation and learning under conditions of deep uncertainty, a common DMDU theme. Evaluation is one important learning strategy. In its most straight-forward implementation, evaluation supports a process of unplanned learning in which actions are taken, their successes and failures documented and understood, this information is assessed by groups such as the IPCC, and these assessments inform future policy decisions. AR6 highlights the importance of monitoring and evaluation but WGII finds that its implementation is currently limited worldwide and insufficiently focused on the outcomes of adaptation actions (WGII SPMC.5.5). AR7 could focus on literature evaluating both mitigation and adaptation outcomes and on actions to promote more evaluation. However, the growing amount and diversity of literature will make it increasingly challenging for IPCC authors to undertake such an assessment in a transparent and traceable manner.
Learning can also be planned so that policy actions are explicitly designed to generate new information to enable adjustments over time. Planned learning can include strategies designed to act-monitor-adjust, but with anticipatory foresight designed to avoid lock-in, that are initial actions that preclude later adjustment (Haasnoot et al., 2019). Such foresight could benefit from information about when learning can be expected (Haasnoot et al., 2018; Hinkel et al., 2019) and about monitoring and analysis to derive signals (Stephens et al., 2017; Haasnoot et al., 2018; Lawrence et al., 2018). Planned learning also includes institutional designs that facilitate experimentation. For instance, the experimentalist governance framework of Sabel and Victor (2022), focused on greenhouse gas mitigation, recommends setting ambitious goals, empowering technical experts from government and industry to collaborate towards achieving those goals, with the threat of penalty defaults if sufficient progress is not made. AR7 could assess literature on experimental governance and planned learning processes, including where they have been tried, evaluation of their successes and failures, approaches to ensure equitable participation and outcomes, and the information needs of such processes and on the institutional enablers that empower such approaches.
DMDU also emphasizes the importance of stress tests that suggest the conditions under which policies will no longer meet their goals. Amidst deep uncertainty DMDU stress tests can provide higher confidence information than can predictions of technology trends, climate changes, and their impacts; promote shared understanding among stakeholders with differing expectations and values; and help inform the development of robust and resilient strategies.
The IPCC has employed stress test concepts, including that of temperature thresholds and limits to adaptation; benchmarks for near-term mitigation actions by 2030 to keep limiting warming to 1.5°C within reach; and societal coping thresholds for heat stress on humans and SLR thresholds determined by exposure and vulnerability. DMDU brings the stress test idea to specific contexts and policy alternatives and includes consideration of response risks, which can also cause policies to fail to meet their goals, as well as opportunities that might arise from favorable combinations of synergic policies. AR7 could assess literature reporting on stress tests of alternative climate-related policies, and how these relate to adaptation enablers as well as the cost-parity thresholds for low carbon technologies examined in risk-opportunity analysis.
These DMDU characteristics suggest some specific guidance for each of the AR7 working groups. A major challenge is that the IPCC has a global scope, whereas most DMDU applications have a much more local spatial focus. To balance between the need for specific context and generalizability, WGII might identify specific decision-relevant archetypes for users, perhaps about a dozen, to represent the range of worldwide contexts. Authors could then use the literature to conduct stress tests for a range of adaptation actions, such as shown in AR6 WGII Figures 13.29 and 13.30 in each of these decision contexts to suggest the conditions under which each action might begin to fail and the key factors affecting such an assessment. The authors could then identify and evaluate potential responses to these vulnerabilities and lay them out in adaptation pathways. AR6 identified governance as a critical risk to adaptation implementation using examples from regional assessments such as in WGII Chapters 11 and 13. Pathways might address vulnerabilities and the actions needed to address them by jurisdictions and other actors at multiple scales.
WGIII might bridge global and narrower scales by continuing with its focus on scenarios, illustrative mitigation pathways and storylines, actions within and across sectors. WGIII could also emphasize DMDU-aligned risk-opportunity analysis (Mercure et al., 2021) and a least-risk rather than least-cost framework (Gambhir and Lempert, 2023) at multiple scales. The assessment of integrated assessment modeling in WGIII could focus more on identifying positive and negative feedbacks, trade-offs and synergies between actions taken or not taken in different sectors, and policy interventions that enhance or move towards positive feedbacks and diminish or move away from negative ones (David et al., 2018).
These topics for WGII and WGIII would create information needs for WGI, such as using a storyline format for high-level sea level rise projections as discussed above. AR6 also used such storylines when considering extremely high climate sensitivity futures, as well as the potential for a large volcanic eruption to affect the climate.
Embracing DMDU concepts might thus suggest changes in the IPCC’s workflow across the assessment cycle and in the information flows among working groups. Information currently flows primarily from WGI to WGII and WGIII. The three working groups then combine toward the end of the assessment cycle to produce a single Synthesis Report (see Box 1). The chapter outlines for the three working group reports are scoped years before the Synthesis Report, which is begun only after the Working Group reports are underway. This process is akin to a predict-then-act framework for informing policy choices.
DMDU envisions a different information flow – often conducting the analysis backwards, that is, starting from policy goals and then considering the actions and uncertainties which affect the pathways to these goals. AR7 might begin with a preliminary scoping of the synthesis report in close collaboration with policy makers, and then constructing the WG outlines to inform the synthesis report. Previous assessments have used loose arrangements such as handshakes and meetings to facilitate information flows among working groups. In particular, AR6 has seen increasing integration and joint scoping, definition and assessment processes. WGI informed WGII of WGI outcomes well before they were finalized, WGII informed WGI of its information needs, and WGI harmonized its climate emulators for use in WGIII. WGII and WGIII also explored opportunities for linkages in risk definitions, CRDPs, and other topics. AR7 could enhance such linkages by having a preliminary Synthesis Report outline as a clearly defined target throughout the WG report development process.
DMDU methods can support such analysis through (a) assessing system vulnerabilities informing the needs for climate information which trigger these vulnerabilities, (b) identifying relevant uncertainties for adaptation, mitigation and decision making, (c) synthesizing literature to support decision making despite and because of uncertainties. Policy makers are faced with decisions that intertwine adaptation, mitigation, and sustainable development. Ideally such decisions would be informed by scientific understanding regarding what is changing, why, how fast and when both mitigation and adaptation is needed. The need for transformative adaptation and mitigation further requires knowledge on how to integrate and achieve system change that addresses these goals sustainably for all, which in turn requires a comprehensive assessment of synergies and trade-offs between adaptation and mitigation at local scale and global scale. Extending the CRDP concept could help specify CRD pathways, key decision points, and their consequences for near term decision making, building on the adaptation pathways for adaptation in WGII AR6. This would require close collaboration between WGII and WGIII.
AR7 might implement these ideas by including more policy practitioners on the author teams, creating a new uncertainty guidance, and highlighting research questions the literature might address before the AR7 literature cutoff.
AR7 will conduct its work in a world undergoing profound change. What was once considered business as usual is no longer descriptive of current reality, no longer ethically acceptable, and in many cases no longer least cost. The contours and effectiveness of many of the solutions that comprise the needed transformational mitigation and adaptation are well-understood. But realizing these transformations in interconnected, complex systems through the actions of multiple decision makers in the face of vested interests, existing power structures, contested values, and many significant risks is a process teaming with deep uncertainties. DMDU is no panacea (Stanton and Roelich, 2021), but is designed to bring uncertain scientific information more effectively into such decision processes. IPCC AR7 may find DMDU increasingly useful as it strives to help decision-makers navigate these opportunities and challenges.
Data availability statement
Publicly available datasets were analyzed in this study. This data can be found here: we report on six assessment cycles of IPCC reports all of which are publicly available.
Author contributions
RL: Writing – original draft, Writing – review & editing. JL: Writing – original draft, Writing – review & editing. RK: Writing – original draft, Writing – review & editing. MH: Writing – original draft, Writing – review & editing. AR: Writing – original draft, Writing – review & editing. MG: Writing – original draft, Writing – review & editing. RP: Writing – review & editing.
Funding
The author(s) declare financial support was received for the research, authorship, and/or publication of this article. RL was supported by the U.S. National Science Foundation (award number 2052972) and the RAND Frederick S. Pardee Center for Longer Range Global Policy and the Future Human Condition. RK was supported by the U.S. National Aeronautics and Space Administration (80NSSC20K1724 and JPL task 105393.509496.02.08.13.31) and by the U.S. National Science Foundation (grant ICER-2103754), as part of the Megalopolitan Coastal Transformation Hub. JL was supported by MBIE grants from the Deep South National Science Challenge Adaptive Tools project (CO1X1412); the Resilience to Nature’s Challenges National Science Challenge Enabling Coastal Adaptation project (GNS-RNC040); and Endeavour Grant (RTVU2206) Our changing coast – Sea-level rise. MG and RP were funded by the Department for Energy Security and Net Zero (DESNZ) and the Children Investment Fund Foundation (CIFF) – United Kingdom, project consortium Economics of Energy Innovation Systems Transition (EEIST).
Conflict of interest
The authors declare that the research was conducted in the absence of any commercial or financial relationships that could be construed as a potential conflict of interest.
Publisher’s note
All claims expressed in this article are solely those of the authors and do not necessarily represent those of their affiliated organizations, or those of the publisher, the editors and the reviewers. Any product that may be evaluated in this article, or claim that may be made by its manufacturer, is not guaranteed or endorsed by the publisher.
References
Adler, C. E., and Hirsch Hadorn, G. (2014). The IPCC and treatment of uncertainties: topics and sources of dissensus. Wiley Interdiscip. Rev. Clim. Chang. 5, 663–676. doi: 10.1002/wcc.297
Adler, C., Lempert, R., Constable, A., Haasnoot, M., Lawrence, J., Mach, K. J., et al. (2022). “Effective adaptation and decision-making under deep uncertainties” in Climate change 2022: Impacts, adaptation and vulnerability. Contribution of working group II to the sixth assessment report of the intergovernmental panel on climate change. eds. H.-O. Pörtner, D. C. Roberts, and M. Tignor (Cambridge, UK and New York, NY, USA: Cambridge University Press), 2578–2580.
Adler, C., Oppenheimer, M., Abram, N., McInnes, K., and Schuur, T. (2019). “Cross chapter box 5: confidence and deep uncertainty” in IPCC special report on the ocean and cryosphere in a changing climate. eds. H.-O. Pörtner, D. C. Roberts, and V. Masson-Delmotte (Cambridge, UK and New York, NY, USA: Cambridge University Press).
Ara Begum, R., Lempert, R., Ali, E., Benjaminsen, T. A., Bernauer, T., Cramer, W., et al. (2022). “Point of departure and key concepts” in Climate change 2022: Impacts, adaptation, and vulnerability. eds. H.-O. Pörtner, D. C. Roberts, and E. S. Poloczanska (Cambridge, UK and New York, NY, USA: Cambridge University Press).
Aven, T. (2019). Climate change risk–what is it and how should it be expressed? J. Risk Res., 23, 1–18. doi: 10.1080/13669877.2019.1687578
Aven, T., and Renn, O. (2015). An evaluation of the treatment of risk and uncertainties in the IPCC reports on climate change. Risk Anal. 35, 701–712. doi: 10.1111/risa.12298
Bednar-Friedl, B., Biesbroek, R., Schmidt, D. N., Alexander, P., Børsheim, K. Y., Carnicer, J., et al. (2022). “Europe” in Climate change 2022: Impacts, adaptation and vulnerability. Contribution of working group II to the sixth assessment report of the intergovernmental panel on climate change. eds. H.-O. Pörtner, D. C. Roberts, M. Tignor, E. S. Poloczanska, K. Mintenbeck, and A. Alegría, et al. (Cambridge, UK and New York, NY, USA: Cambridge University Press), 1817–1927.
Bloemen, P. J. T. M., Hammer, F., van der Vlist, M. J., Grinwis, P., and van Alphen, J. (2019). “DMDU into practice: Adaptive Delta Management in the Netherlands” in Decision making under deep uncertainty. eds. V. Marchau, W. Walker, P. Bloemen, and S. Popper (Cham: Springer).
Brown, C., Steinschneider, S., Ray, P. A., Wi, S., Basdekas, L., and Yates, D. (2019). “Decision scaling (DS): decision support for climate change” in Decision making under deep uncertainty: from theory to practice. eds. V. A. W. J. Marchau, W. E. Walker, P. J. T. M. Bloemen, and S. W. Popper (Springer), 255–287.
Bryant, B. P., and Lempert, R. J. (2010). Thinking inside the box: a participatory, computer-assisted approach to scenario discovery. Technol. Forecast. Soc. Chang. 77, 34–49. doi: 10.1016/j.techfore.2009.08.002
Carlsen, H., Lempert, R., Wikman-Svahn, P., and Schweizer, V. (2016). Choosing small sets of policy-relevant scenarios by combining vulnerability and diversity approaches, Environmental Modelling & Software. 84, 155–164. doi: 10.1016/j.envsoft.2016.06.011
Chen, D., Rojas, M., Samset, B. H., Cobb, K., Niang, A. D., Edwards, P., et al. (2021). “Framing, context, and methods” in climate change (2021): the physical science basis. Contribution of working group I to the sixth assessment report of the intergovernmental panel on climate change. eds. V. Masson-Delmotte, P. Zhai, A. Pirani, S. L. Connors, C. Péan, and S. Berger, et al. (Cambridge, United Kingdom and New York, NY, USA: Cambridge University Press), 147–286.
Cozannet, L., Lawrence, J., Schoeman, D. S., Adelekan, I., Cooley, S. R., Glavovic, B., et al. (2022). Cross-chapter box SLR | sea level rise. In S. Cooley and D. Schoeman. In: Climate change 2022: impacts, adaptation, and vulnerability. Contribution of working group II to the sixth assessment report of the intergovernmental panel on climate change H. O. Pörtner, D. C. Roberts, M. Tignor, E. S. Poloczanska, K. Mintenbeck, and A. Alegría, et al. Cambridge University Press, Cambridge, UK and New York, NY, USA, pp. 379–550.
Creutzig, F., Niamir, L., Bai, X., Callaghan, M., Cullen, J., Díaz-José, J., et al. (2022). Demand-side solutions to climate change mitigation consistent with high levels of well-being. Nat. Clim. Chang. 12, 36–46. doi: 10.1038/s41558-021-01219-y
David, T. J. N., Frantzeskaki, K. H., Pedde, S., Kok, K., Lamperti, F., Christensen, J. H., et al. (2018). Positive tipping points in a rapidly warming world. Curr. Opin. Environ. Sustain. 31, 120–129. doi: 10.1016/j.cosust.2018.01.012
Dessai, S., and Hulme, M. (2004). Does climate adaptation policy need probabilities? Clim. Pol. 4, 107–128. doi: 10.1080/14693062.2004.9685515
Fox-Kemper, B., Hewitt, H. T., Xiao, C., Aðalgeirsdóttir, G., Drijfhout, S. S., Edwards, T. L., et al. (2021). “Ocean, cryosphere, and sea level change. Chapter 9 in climate change 2021: the physical science basis” in Contribution of working group I to the sixth assessment report of the intergovernmental panel on climate change. eds. V. Masson-Delmotte, P. Zhai, A. Pirani, S. L. Connors, C. Péan, and S. Berger, et al. (Cambridge, UK, New York, NY USA: Cambridge University Press).
Gambhir, A., and Lempert, R. (2023). From least cost to least risk: producing climate change mitigation plans that are resilient to multiple risks. Front. Clim. 5:1149309. doi: 10.3389/fclim.2023.1149309
Glavovic, B. C., Dawson, R., Chow, W., Garschagen, M., Haasnoot, M., Singh, C., et al. (2022). “Cross-chapter paper 2: Cities and settlements by the sea” in Climate change 2022: Impacts, adaptation and vulnerability. Contribution of working group II to the sixth assessment report of the intergovernmental panel on climate change. eds. H.-O. Pörtner, D. C. Roberts, M. Tignor, E. S. Poloczanska, K. Mintenbeck, and A. Alegría, et al. (Cambridge, UK and New York, NY, USA: Cambridge University Press), 2163–2194.
Gong, M., Lempert, R., Parker, A., Mayer, L. A., Fischbach, J., Sisco, M., et al. (2017). Testing the scenario hypothesis: an experimental comparison of scenarios and forecasts for decision support in a complex decision environment. Environ. Model Softw. 91, 135–155. doi: 10.1016/j.envsoft.2017.02.002
Groves, D. G., Molina Perez, E., Bloom, E., and Fischbach, J. R. (2019). “Robust decision making (RDM): application to water planning and climate policy” in Decision making under deep uncertainty: From theory to practice. eds. V. A. W. J. Marchau, W. E. Walker, P. J. T. M. Bloemen, and S. W. E. Popper, vol. 329 (Cham, Switzerland: Springer).
Groves, D. G., Syme, J., Molina-Perez, E., Hernandez, C. C., Víctor-Gallardo, L. F., Godinez-Zamora, G., et al. (2020). The benefits and costs of decarbonizing Costa Rica's economy: informing the implementation of Costa Rica's National Decarbonization Plan under Uncertainty. I.-A. D.: Santa Monica, CA USA: Bank, RAND.
Grubb, M., Drummond, P., Mercure, J.-F., Popp, D., Samadi, S., Penasco, C., et al. (2021) The new economics of innovation and transition: Evaluating opportunities and risks. Economics of Energy Innovation and System Transition. Exeter University, Exeter, UK. Available at: https://eeist.co.uk/eeist-reports/
Grubb, M., Okereke, C., Arima, J., Bosetti, V., Chen, Y., Edmonds, J., et al., (2022): Introduction and framing. In IPCC, 2022: climate change 2022: mitigation of climate change. Contribution of working group III to the sixth assessment report of the intergovernmental panel on climate change [P.R. Shukla, J. Skea, R. Slade, A. KhourdajieAl, R. Diemenvan, and D. McCollum, et al., (eds.)]. Cambridge University Press, Cambridge, UK and New York, NY, USA
Grubb, M., Vrolijk, C., and Brack, D. (2018). Routledge revivals: Kyoto protocol (1999): A guide and assessment. Abingdon, Oxfordshire UK: Routledge.
Grubler, A. N., and Nakicenovic, N. (2001). Identifying dangers in an uncertain climate. Nature 412:15. doi: 10.1038/35083752
Guillaume, J. H. A., Arshad, M., Jakeman, A. J., Jalava, M., and Kummu, M. (2016). Robust discrimination between uncertain management alternatives by iterative reflection on crossover point scenarios: principles, design and implementations. Environ. Model Softw. 83, 326–343. doi: 10.1016/j.envsoft.2016.04.005
Haasnoot, M., Biesbroek, R., Lawrence, J., Muccione, V., Lempert, R., and Glavovic, B. (2020). Defining the solution space to accelerate climate change adaptation. Reg. Environ. Chang. 20, 1–5. doi: 10.1007/s10113-020-01623-8
Haasnoot, M., Kwakkel, J. H., Walker, W. E., and ter Maat, J. (2013). Dynamic adaptive policy pathways: a New method for crafting robust decisions for a deeply uncertain world. Glob. Environ. Chang. 23, 485–498. doi: 10.1016/j.gloenvcha.2012.12.006
Haasnoot, M., Van’t Klooster, S., and Van Alphen, J. (2018). Designing a monitoring system to detect signals to adapt to uncertain climate change. Glob. Environ. Chang. 52, 273–285. doi: 10.1016/j.gloenvcha.2018.08.003
Haasnoot, M., Warren, A., and Kwakkel, J. H. (2019). “Dynamic adaptive policy pathways (DAPP)” in Decision making under deep uncertainty: From theory to practice. eds. V. A. W. J. Marchau, W. E. Walker, P. J. T. M. Bloemen, and S. W. E. Popper (Cham, Switzerland: Springer).
Hinkel, J., Church, J. A., Gregory, J. M., Lambert, E., Le Cozannet, G., Lowe, J., et al. (2019). Meeting user needs for sea level rise information: a decision analysis perspective. Earth's Future 7, 320–337. doi: 10.1029/2018EF001071
IPCC (2005). “Guidance notes for lead authors of the IPCC fourth assessment report on addressing uncertainties” in AR4 climate change 2007: the physical science basis. Eds. Bert Metz, Ogunlade Davidson, Heleen de Coninck, Manuela Loos, Leo Meyer (Cambridge University Press).
IPCC (2012). “Managing the risks of extreme events and disasters to advance climate change adaptation” in A special report of working groups I and II of the intergovernmental panel on climate change. Eds. Christopher B. Field, Vicente Barros, Thomas F. Stocker, Qin Dahe, David Jon Dokken, Kristie L. Ebi, et al. (Cambridge, UK, and New York, NY, USA, IPCC: Cambridge University Press).
IPCC (2014). “Climate change 2014: synthesis report” in Contribution of working groups I, II and III to the fifth assessment report of the intergovernmental panel on climate change. eds. C. W. Team, R. K. Pachauri, and L. A. Meyer (Geneva, Switzerland: IPCC), 151.
IPCC (2021a) FactSheet. Available at: https://www.ipcc.ch/site/assets/uploads/2021/07/AR6_FS_What_is_IPCC.pdf
IPCC (2021b). “Summary for policymakers” in Climate change 2021: the physical science basis. Contribution of working group I to the sixth assessment report of the intergovernmental panel on climate change. eds. V. Masson-Delmotte, P. Zhai, A. Pirani, S. L. Connors, C. Péan, and S. Berger, et al. (Cambridge, United Kingdom and New York, NY, USA: Cambridge University Press), 3–32.
IPCC (2022) Summary for policymakers. In: Climate change 2022: Mitigation of climate change. Contribution of working group III to the sixth assessment report of the intergovernmental panel on climate change [P.R. Shukla, J. Skea, R. Slade, A. KhourdajieAl, R. Diemenvan, and D. McCollum, et al., (eds.)]. Cambridge University Press, Cambridge, UK and New York, NY, USA.
IPCC (2023). “Annex I: glossary” in Climate change 2023: Synthesis report. Contribution of working groups I, II and III to the sixth assessment report of the intergovernmental panel on climate change. eds. A. Reisinger, D. Cammarano, A. Fischlin, J. S. Fuglestvedt, G. Hansen, and Y. Jung, et al. (Geneva, Switzerland: IPCC), 119–130.
Jafino, B. A., Kwakkel, J. H., and Taebi, B. (2021). Enabling assessment of distributive justice through models for climate change planning: a review of recent advances and a research agenda. Wiley Interdiscip. Rev. Clim. Chang. 12:721. doi: 10.1002/wcc.721
Janzwood, S. (2022). Confidence deficits and reducibility: toward a coherent conceptualization of uncertainty level. Risk Anal. 43, 2004–2016. doi: 10.1111/risa.14008
Jones, R. N., Patwardhan, A., Cohen, S., Dessai, S., Lammel, A., Lempert, R., et al. (2014). “Foundations for decision making” in Climate change 2014: Impacts, adaptation, and vulnerability. Rajendra K. Pachauri, Myles R. Allen, Vicente R. Barros, John Broome, Wolfgang Cramer, Renate Christ, et al. (Geneva, Switzerland: Intergovernmental Panel on Climate Change (IPCC)).
Kalra, N., Hallegatte, S., Lempert, R., Brown, C., Fozzard, A., Gill, S., et al. (2014). Agreeing on robust decisions: A New process fo decision making under deep uncertainty : Policy Research Working Paper. Washington, D.C. USA: World Bank.
Kingsborough, A., Katie, J., and Hall, J. W. (2017). Development and appraisal of long-term adaptation pathways for managing heat-risk in London Climate Risk Management, 16, 73–92. doi: 10.1016/j.crm.2017.01.001
Köberle, A. C., Vandyck, T., Guivarch, C., Macaluso, N., Bosetti, V., Gambhir, A., et al. (2021). The cost of mitigation revisited. Nat. Clim. Chang. 11, 1035–1045. doi: 10.1038/s41558-021-01203-6
Kopp, R. E., Oppenheimer, M., O’Reilly, J. L., Drijfhout, S. S., Edwards, T. L., Fox-Kemper, B., et al. (2023). Communicating future sea-level rise uncertainty and ambiguity to assessment users. Nat. Clim. Chang. 13, 648–660. doi: 10.1038/s41558-023-01691-8
Kunreuther, H., Gupta, S., Bosetti, V., Cooke, R., Dutt, V., Ha-Duong, M., et al. (2014). “Integrated risk and uncertainty assessment of climate change response policies” in Climate change 2014: Mitigation of climate change. Contribution of working group III to the fifth assessment report of the intergovernmental panel on climate change. eds. O. Edenhofer, R. Pichs-Madruga, and E. F. Y. Sokona (Cambridge, United Kingdom and New York, NY, USA: Cambridge University Press).
Kwakkel, J. H. (2019). A generalized many-objective optimization approach for scenario discovery. Fut. Fores. Sci. 1:e8. doi: 10.1002/ffo2.8
Kwakkel, J., Walker, W., and Marchau, V. (2010). Classifying and communicating uncertainties in model-based policy analysis. Int. J. Technol. Manag. 10, 299–315. doi: 10.1504/IJTPM.2010.036918
Lamontagne, J. R., Reed, P. M., Link, R., Calvin, K. V., Clarke, L. E., and Edmonds, J. A. (2018). Large ensemble analytic framework for consequence-driven discovery of climate change scenarios. Earth's Future 6, 488–504. doi: 10.1002/2017EF000701
Lavell, A., Oppenheimer, M., Diop, C., Hess, J., Lempert, R., Li, J., et al. (2012). Climate change: New dimensions in disaster risk, exposure, vulnerability, and resilience. Managing the risks of extreme events and disasters to advance climate change adaptation. Cambridge, UK and New York, NY USA: IPCC, Cambridge University Press.
Lawrence, J., Bell, R., Blackett, P., Stephens, S., and Allan, S. (2018). National guidance for adapting to coastal hazards and sea-level rise: anticipating change, when and how to change pathway. Environ. Sci. Pol. 82, 100–107. doi: 10.1016/j.envsci.2018.01.012
Lawrence, J., Haasnoot, M., McKim, L., Atapattu, D., Campbell, G., and Stroombergen, A. (2019). “From theory to practice: a timeline of interventions by a change agent with the developers and users of dynamic adaptive policy pathways (DAPP)” in Decisionmaking under deep uncertainty: From theory to practice. eds. V. Marchau, W. Walker, and P. Bloeman, 187. Available at: https://www.springer.com/us/book/9783030052515
Leggett, J., Pepper, W. J., Swart, R. J., Edmonds, J., Meira Filho, L. G., Mintzer, I., et al. (1992). Emissions scenarios for the IPCC: an update. Climate Change 1040, 75–95.
Lempert, R. J. (2002). A new decision sciences for complex systems. Proc. Natl. Acad. Sci. 99, 7309–7313. doi: 10.1073/pnas.082081699
Lempert, R. J. (2013). Scenarios that illuminate vulnerabilities and robust responses. Clim. Chang. 117, 627–646. doi: 10.1007/s10584-012-0574-6
Lempert, R. (2019). “Robust decision making (RDM)” in Decision making under deep uncertainty: From theory to practice. eds. V. A. W. J. Marchau, W. E. Walker, P. J. T. M. Bloemen, and S. W. E. Popper, vol. 329 (Cham, Switzerland: Springer).
Lempert, R., Groves, D. G., and Fischbach, J. (2013). “Is it ethical to use a single probability density function?” RAND-WR992. RAND Corporation.
Lempert, R. J., Popper, S. W., and Bankes, S. C. (2003). Shaping the next one hundred years: New methods for quantitative. Long-term Policy Analysis: Santa Monica, CA, RAND Corporation.
Lempert, R. J., and Turner, S. (2021). Engaging multiple worldviews with quantitative decision support: a robust decision-making demonstration using the Lake model. Risk Anal. 41, 845–865. doi: 10.1111/risa.13579
Levin, K., Cashore, B., Bernstein, S., and Auid, G. (2012). Overcoming the tragedy of super wicked problems: constraining our future selves to ameliorate global climate change. Policy. Sci. 45:152. doi: 10.1007/s11077-012-9151-0
Mach, K. J., Mastrandrea, M. D., Freeman, P. T., and Field, C. B. (2017). Unleashing expert judgment in assessment. Glob. Environ. Chang. 44, 1–14. doi: 10.1016/j.gloenvcha.2017.02.005
Magnan, A. K., Schipper, E. L. F., and Duvat, V. K. (2020). Frontiers in climate change adaptation science: advancing guidelines to design adaptation pathways. Curr. Clim. Chang. Rep. 6, 166–177. doi: 10.1007/s40641-020-00166-8
Malekpour, S., Allen, C., Sagar, A., Scholz, I., Persson, Å., Miranda, J., et al. (2023). What scientists need to do to accelerate progress on the SDGs. Nature 621, 250–254. doi: 10.1038/d41586-023-02808-x
Marchau, V. A., Walker, W. E., Bloemen, P. J., and Popper, S. W. (2019a). Decision making under deep uncertainty: From theory to practice. Cham, Switzerland: Springer Nature, 405.
Marchau, V. A., Walker, W. E., Bloemen, P. J., and Popper, S. W. (2019b). “Introduction” in Decision making under deep uncertainty: From theory to practice, Eds. Vincent A. W. J. Marchau, Warren E. Walker, Pieter J.T.M. Bloemen, Steven W. Popper 1–20.
Mastrandrea, M. D., Field, C. B., Stocker, T. F., Edenhofer, O., Ebi, K. L., Frame, D. J., et al. (2010). Guidance note for Lead authors of the IPCC fifth assessment report on consistent treatment of uncertainties. Intergovernmental Panel on Climate Change. Available at: http://www.ipcc.ch
Meah, N. (2019). Climate uncertainty and policy making—what do policy makers want to know? Reg. Environ. Chang. 19, 1611–1621. doi: 10.1007/s10113-019-01492-w
Mercure, J.-F., Sharpe, S., Vinuales, J., Grubb, M., and Knobloch, F. (2021). Risk-opportunity analysis for low-carbon policy design and appraisal. Glob. Environ. Chang. 70:102359. doi: 10.1016/j.gloenvcha.2021.102359
Mitchell, S. (2009). Unsimple truths: Science, complexity, and policy. Chicago: IL, University of Chicago Press.
Morgan, M. G., and Keith, D. W. (2008). Improving the way we think about projecting future energy use and emissions of carbon dioxide. Clim. Chang. 90, 189–215. doi: 10.1007/s10584-008-9458-1
Moss, R. H., and Schneider, S. H. (2000). “Uncertainties in the IPCC TAR: recommendations to lead authors for more consistent assessment and reporting” in Guidance papers on the cross cutting issues of the third assessment report of the IPCC. eds. R. Pachauri, T. Taniguchi, and K. Tanaka (Geneva: World Meteorological Organization), 33–51.
Muccione, V., Haasnoot, M., Alexander, P., Bednar-Friedl, B., Biesbroek, R., Georgopoulou, E., et al. (2024). Adaptation pathways for effective responses to climate change risks. Wiley Interdiscip. Rev. Clim. Chang. :e883. doi: 10.1002/wcc.883
Nakicenovic, N., Alcamo, J., Davis, G., Vries, B. D., Fenhann, J., Gaffin, S., et al. (2000). Special report on emissions scenarios. Cambridge University Press.
Nakicenovic, N., Lempert, R. J., and Janetos, A. C. (2014). A framework for the development of New socio-economic scenarios for climate change research: introductory essay, a forthcoming special issue of climatic change. Clim. Chang. 122, 351–361. doi: 10.1007/s10584-013-0982-2
Ocean and Climate Platform (2023). Adapting coastal cities and territories to sea level rise in North America – U.S. West coast: challenges and leading practices, vol. 56: Ocean and Climate Platform.
O’Neill, B. C., Oppenheimer, M., Warren, R., Hallegatte, S., Kopp, R. E., Pörtner, H. O., et al. (2017). IPCC reasons for concern regarding climate change risks. Nat. Clim. Chang. 7, 28–37. doi: 10.1038/nclimate3179
Parker, A. M., Srinivasan, S., Lempert, R. J., and Berry, S. (2015). Evaluating simulation-derived scenarios for effective decision support. Technol. Forecast. Soc. Chang. 91, 64–77. doi: 10.1016/j.techfore.2014.01.010
Parson, E. A., Burkett, V., Fischer-Vanden, K., Keith, D., Mearns, L. O., Pitcher, H., et al. (2007). Global-change scenarios: Their development and use, synthesis and assessment product 2.1b. Lincoln, NE USA: US Climate Change Science Program.
Pathak, M. R. S., Shukla, P. R., Skea, J., Pichs-Madruga, R., and Ürge-Vorsatz, D. (2022): Technical summary. In: Climate change 2022: Mitigation of climate change. Contribution of working group III to the sixth assessment report of the intergovernmental panel on climate change [P. R. Shukla, J. Skea, R. Slade, A. KhourdajieAl, R. Diemenvan, and D. McCollum, et al., (eds.)]. Cambridge University Press, Cambridge, UK and New York, NY, USA.
Pidgeon, N., and Fischhoff, B. (2011). The role of social and decision sciences in communicating uncertain climate risks. Nat. Clim. Chang. 1, 35–41. doi: 10.1038/nclimate1080
Popper, S. W. (2019). “Reflections: DMDU and public policy for uncertain times” in Decision making under deep uncertainty: from theory to practice. eds. V. A. W. J. Marchau, W. E. Walker, P. J. T. M. Bloemen, and S. W. Popper (Cham, Switzerland: Springer).
Reisinger, A., and Geden, O. (2023). “Temporary overshoot: Origins, prospects, and a long path ahead.” One Earth 6, no 12, 1631–1637. doi: 10.1016/j.oneear.2023.11.008
Reisinger, A., Howden, M., Vera, C., Garschagen, M., Mach, K. J., Pathak, M., et al. (2020). The concept of risk in the IPCC sixth assessment report: A summary of cross-working group discussions. Geneva, Switzerland: Intergovernmental Panel on Climate Change, 15.
Riahi, K., Schaeffer, R., Arango, J., Calvin, K., Guivarch, C., Hasegawa, T., et al. (2022): Mitigation pathways compatible with long-term goals. In IPCC, 2022: Climate change 2022: Mitigation of climate change. Contribution of working group III to the sixth assessment report of the intergovernmental panel on climate change [P. R. Shukla, J. Skea, R. Slade, A. KhourdajieAl, R. Diemenvan, and D. McCollum, et al., (eds.)]. Cambridge University Press, Cambridge, UK and New York, NY, USA.
Rose, S., Betts, R., Wester, P., and Koutroulis, A. (2022). “Cross-chapter box: climate reference periods, global warming levels and common climate dimensions” in Climate change 2022: Impacts, adaptation and vulnerability. Contribution of working group II to the sixth assessment report of the intergovernmental panel on climate change H.-O. Pörtner. eds. D. C. Roberts and M. Tignor (Cambridge, UK and New York, NY, USA: Cambridge University Press), 135–136.
Sabel, C. F., and Victor, D. G. (2022). Fixing the climate: Strategies for an uncertain world. Princeton, NJ USA: Princeton University Press.
Sarewitz, D., and Pielke, R. A. (2000). Science, prediction: Decisionmaking, and the future of nature. Washington, DC: Island Press.
Schipper, E. L. F., Revi, A., Preston, B. L., Carr, E. R., Eriksen, S. H., Fernandez-Carril, L. R., et al. (2022). “Climate resilient development pathways” in Climate change 2022: Impacts, adaptation and vulnerability. Contribution of working group II to the sixth assessment report of the intergovernmental panel on climate change. eds. H.-O. Pörtner, D. C. Roberts, M. Tignor, E. S. Poloczanska, K. Mintenbeck, and A. Alegría, et al. (Cambridge, UK and New York, NY, USA: Cambridge University Press), 2655–2807.
Schneider, S. H. (2001). What is ‘dangerous’ climate change? Nature 411, 17–19. doi: 10.1038/35075167
Schoemaker, P. J. H. (1993). Multiple scenario development: its conceptual and behavioral foundation. Strateg. Manag. J. 14, 193–213. doi: 10.1002/smj.4250140304
Schwartz, P. (1996). The art of the long view-planning for the future in an uncertain world. New York: NY, Currency-Doubleday.
Scussolini, P., Tran, T. V. T., Koks, E., Diaz-Loaiza, A., Ho, P. L., and Lasage, R. (2017). Adaptation to sea level rise: a multidisciplinary analysis for Ho Chi Minh City, Vietnam. Water Resour. Res. 53, 10841–10857. doi: 10.1002/2017WR021344
Shepherd, T. G., Boyd, E., Calel, R. A., Chapman, S. C., Dessai, S., Dima-West, I. M., et al. (2018). Storylines: an alternative approach to representing uncertainty in physical aspects of climate change. Clim. Chang. 151, 555–571. doi: 10.1007/s10584-018-2317-9
Simpson, N. P., Mach, K. J., Constable, A., Hess, J., Hogarth, R., Howden, M., et al. (2021). A framework for complex climate change risk assessment. One Earth 4, 489–501. doi: 10.1016/j.oneear.2021.03.005
Slangen, A. B. A., Haasnoot, M., and Winter, G. (2022). Rethinking Sea-level projections using families and timing differences. Earth’s Fut. 10:e2021EF002576. doi: 10.1029/2021EF002576
Sriver, R. L., Lempert, R. J., Wikman-Svahn, P., and Keller, K. (2018). Characterizing uncertain sea-level rise projections to support investment decisions. PLoS One 13:e0190641. doi: 10.1371/journal.pone.0190641
Stanton, M. C., and Roelich, K. (2021). Decision making under deep uncertainties: a review of the applicability of methods in practice. Technol. Forecast. Soc. Chang. 171:120939. doi: 10.1016/j.techfore.2021.120939
Stephens, S. A., Bell, R. G., and Lawrence, J. (2017). Applying principles of uncertainty within coastal hazard assessments to better support coastal adaptation. J. Mar. Sci. Eng. 5:40. doi: 10.3390/jmse5030040
Tangney, P. (2019a). Does risk-based decision-making present an 'epistemic trap' for climate change policymaking? Evid. Policy J. Res. Debate Pract., 16, 1–12.
Tangney, P. (2019b). Understanding climate change as risk: a review of IPCC guidance for decision-making. J. Risk Res., 23, 1–16. doi: 10.1080/10361146.2018.1551482
Toth, F. L., Mwandosya, M., Carraro, C., Christensen, J., Edmonds, J., Flannery, B., et al. (2001). “Chapter 10 decision-making frameworks” in Climate change 2001: Mitigation: Contribution of working group III to the third assessment report of the intergovernmental panel on climate change. Cambridge University Press.
van Alphen, J., Haasnoot, M., and Diermanse, F. (2022). Uncertain Accelerated Sea-level rise, potential consequences, and adaptive strategies in the Netherlands. Water 14:1527. doi: 10.3390/w14101527
Wack, P. (1985). The gentle art of reperceiving–scenarios: uncharted waters ahead (part 1 of a two-part article). Harv. Bus. Rev., 85516, 73–89.
Walker, W. E., Harremoës, P., Rotmans, J., van der Sluijs, J. P., van Asselt, M. B. A., Janssen, P., et al. (2003). Defining uncertainty: a conceptual basis for uncertainty Management in Model-Based Decision Support. Integr. Assess. 4, 5–17. doi: 10.1076/iaij.4.1.5.16466
Weitzman, M. L. (2011). Fat-tailed uncertainty in the economics of catastrophic climate change. Rev. Environ. Econ. Policy 5, 275–292. doi: 10.1093/reep/rer006
Keywords: DMDU, IPCC, AR7, deep uncertainty, risk management, dynamic adaptive policy pathways, robust decision making
Citation: Lempert RJ, Lawrence J, Kopp RE, Haasnoot M, Reisinger A, Grubb M and Pasqualino R (2024) The use of decision making under deep uncertainty in the IPCC. Front. Clim. 6:1380054. doi: 10.3389/fclim.2024.1380054
Edited by:
Peter Haas, University of Massachusetts Amherst, United StatesReviewed by:
Jonathan Lamontagne, Tufts University, United StatesDetlef Sprinz, Potsdam Institute for Climate Impact Research (PIK), Germany
Copyright © 2024 Lempert, Lawrence, Kopp, Haasnoot, Reisinger, Grubb and Pasqualino. This is an open-access article distributed under the terms of the Creative Commons Attribution License (CC BY). The use, distribution or reproduction in other forums is permitted, provided the original author(s) and the copyright owner(s) are credited and that the original publication in this journal is cited, in accordance with accepted academic practice. No use, distribution or reproduction is permitted which does not comply with these terms.
*Correspondence: Robert J. Lempert, bGVtcGVydEByYW5kLm9yZw==