- 1Department of the Geophysical Sciences, The University of Chicago, Chicago, IL, United States
- 2Grupo de Ingeniería y Gestión Ambiental, Escuela Ambiental, Facultad de Ingeniería, Universidad de Antioquia, Medellín, Colombia
- 3Department of Mathematics and Statistics, University of Exeter, Exeter, United Kingdom
- 4Department of Water and Climate Risk, Institute for Environmental Studies, VU Amsterdam, Amsterdam, Netherlands
- 5Institute of Environmental Geosciences, University Grenoble Alpes, Grenoble, France
- 6The Fredy and Nadine Herrmann Institute of Earth Sciences, The Hebrew University of Jerusalem, Jerusalem, Israel
- 7Institute for Risk and Disaster Reduction, University College London, London, United Kingdom
- 8Centre for Climate Research Singapore, Singapore, Singapore
- 9Centre for Climate Change Research, Indian Institute of Tropical Meteorology, Ministry of Earth Sciences, Pune, India
- 10Leipzig Institute for Meteorology, University of Leipzig, Leipzig, Germany
- 11Department of Meteorology, University of Reading, Reading, United Kingdom
- 12Pacific Northwest National Laboratory, Richland, WA, United States
- 13Australian Bureau of Meteorology, Melbourne, VIC, Australia
- 14Oeschger Centre for Climate Change Research, Institute of Geography, University of Bern, Bern, Switzerland
- 15Lamont Doherty Earth Observatory of Columbia University, Palisades, New York, NY, United States
- 16Jülich Supercomputing Centre, Forschungszentrum Jülich, Jülich, Germany
- 17Facultad de Ciencias Exactas y Naturales, Universidad de Buenos Aires, Buenos Aires, Argentina
- 18Centro de Investigaciones del Mar y la Atmósfera (CIMA), CONICET–Universidad de Buenos Aires, Buenos Aires, Argentina
- 19Instituto Franco-Argentino Para el Estudio del Clima y sus Impactos (UMI 3351 IFAECI), CNRS–IRD–CONICET–UBA, Buenos Aires, Argentina
- 20Department of Physics, The University of the West Indies, Kingston, Jamaica
- 21Institute of Atmospheric Physics, Chinese Academy of Sciences, Beijing, China
Climate change has emerged across many regions. Some observed regional climate changes, such as amplified Arctic warming and land-sea warming contrasts have been predicted by climate models. However, many other observed regional changes, such as changes in tropical sea surface temperature and monsoon rainfall are not well simulated by climate model ensembles even when taking into account natural internal variability and structural uncertainties in the response of models to anthropogenic radiative forcing. This suggests climate model predictions may not fully reflect what our future will look like. The discrepancies between models and observations are not well understood due to several real and apparent puzzles and limitations such as the “signal-to-noise paradox” and real-world record-shattering extremes falling outside of the possible range predicted by models. Addressing these discrepancies, puzzles and limitations is essential, because understanding and reliably predicting regional climate change is necessary in order to communicate effectively about the underlying drivers of change, provide reliable information to stakeholders, enable societies to adapt, and increase resilience and reduce vulnerability. The challenges of achieving this are greater in the Global South, especially because of the lack of observational data over long time periods and a lack of scientific focus on Global South climate change. To address discrepancies between observations and models, it is important to prioritize resources for understanding regional climate predictions and analyzing where and why models and observations disagree via testing hypotheses of drivers of biases using observations and models. Gaps in understanding can be discovered and filled by exploiting new tools, such as artificial intelligence/machine learning, high-resolution models, new modeling experiments in the model hierarchy, better quantification of forcing, and new observations. Conscious efforts are needed toward creating opportunities that allow regional experts, particularly those from the Global South, to take the lead in regional climate research. This includes co-learning in technical aspects of analyzing simulations and in the physics and dynamics of regional climate change. Finally, improved methods of regional climate communication are needed, which account for the underlying uncertainties, in order to provide reliable and actionable information to stakeholders and the media.
1 Introduction
Global-mean warming, which is now approaching 1.5°C, was detected decades ago and is reliably attributed to human activity (IPCC, 2021). Yet, weather and climate are experienced at the regional or local scale, where the internal variability (i.e., noise) is much larger than at the global scale, and it takes longer for the ‘forced’ climate change signal, e.g., a statistically significant linear trend, to emerge from the noise (Hawkins and Sutton, 2009). Even so, the regional impacts of global warming, such as increases in hot extremes, have now been detected and attributed across most of the Earth’s land surface (see IPCC, 2021, Figure SPM.3A). In general, thermodynamic (temperature-related) aspects of regional change are supported by theory, observation-based data, paleoclimate reconstructions, and climate models, which tell a consistent story, and can be related to extremes such as heatwaves, heavy precipitation, and evaporatively-driven drought. However, even in this thermodynamic context models are faced with challenges including model-observation discrepancies of trends in regional sea surface temperature (SST), heat extremes and record-shattering extremes (Perkins-Kirkpatrick et al., 2024).
The dynamical aspects of climate change, that is those associated with changes in atmospheric or oceanic circulation, which also significantly impact regional climate change, have long been more difficult to ascertain due to competing theories and the significant influence of internal variability (Shaw et al., 2016, Shaw 2019). However, some signals, such as a weakening trend of the summertime circulation in the Northern Hemisphere (Coumou et al., 2015; Kang et al., 2023a) and strengthening trend of storminess in the Southern Hemisphere (Chemke et al., 2022; Shaw et al., 2022), have already emerged in observations and, as global warming continues to increase, we can expect even more dynamical signals to emerge clearly from the noise in observations. This will test theoretical expectations, and the climate science community must be ready to provide meaningful interpretations of the observed trends in the face of what is still considerable uncertainty.
At this point, some of the emerging signals seem consistent with climate model predictions, but others are not, and there are concerns being raised that climate models may underestimate key aspects of the forced response, including unprecedented extremes as discussed below. The purpose of this perspective article is to summarize where there is agreement on aspects of regional climate change, where there are discrepancies, and to suggest ways forward.
2 Model-observation discrepancies, confounding puzzles and limitations
Generations of models have predicted changes in regional climate under anthropogenic forcing with strong consensus (see Doblas-Reyes et al., 2021). Several of these regional changes have already emerged in observations and have been attributed to human activities, e.g., land warming more than ocean, Arctic amplification of surface temperature increase, more intense heat waves, heavy precipitation increase, and delayed monsoon onset. As climate change continues unabated, we are now entering a time where we are expecting to be able to detect many more of those predicted changes. These changes include, e.g., weakening tropical circulation, SST pattern trends in the tropics, shifts in jets, strengthening of midlatitude storms in the Southern Hemisphere across seasons and weakening during Northern Hemisphere summer, and a slowdown of the Atlantic Meridional Overturning Circulation (AMOC). A recent assessment of the above-mentioned regional changes can be found in the 6th Assessment Report of the IPCC (Doblas-Reyes et al., 2021; Eyring et al., 2021). Models have shown fidelity in capturing some of the emerging regional signals, however, model-observation discrepancies have been accumulating across different regions. We outline several examples of the discrepancies below that are summarized in Figure 1. The different outcomes of model-observation comparisons are provided in Figure 2.
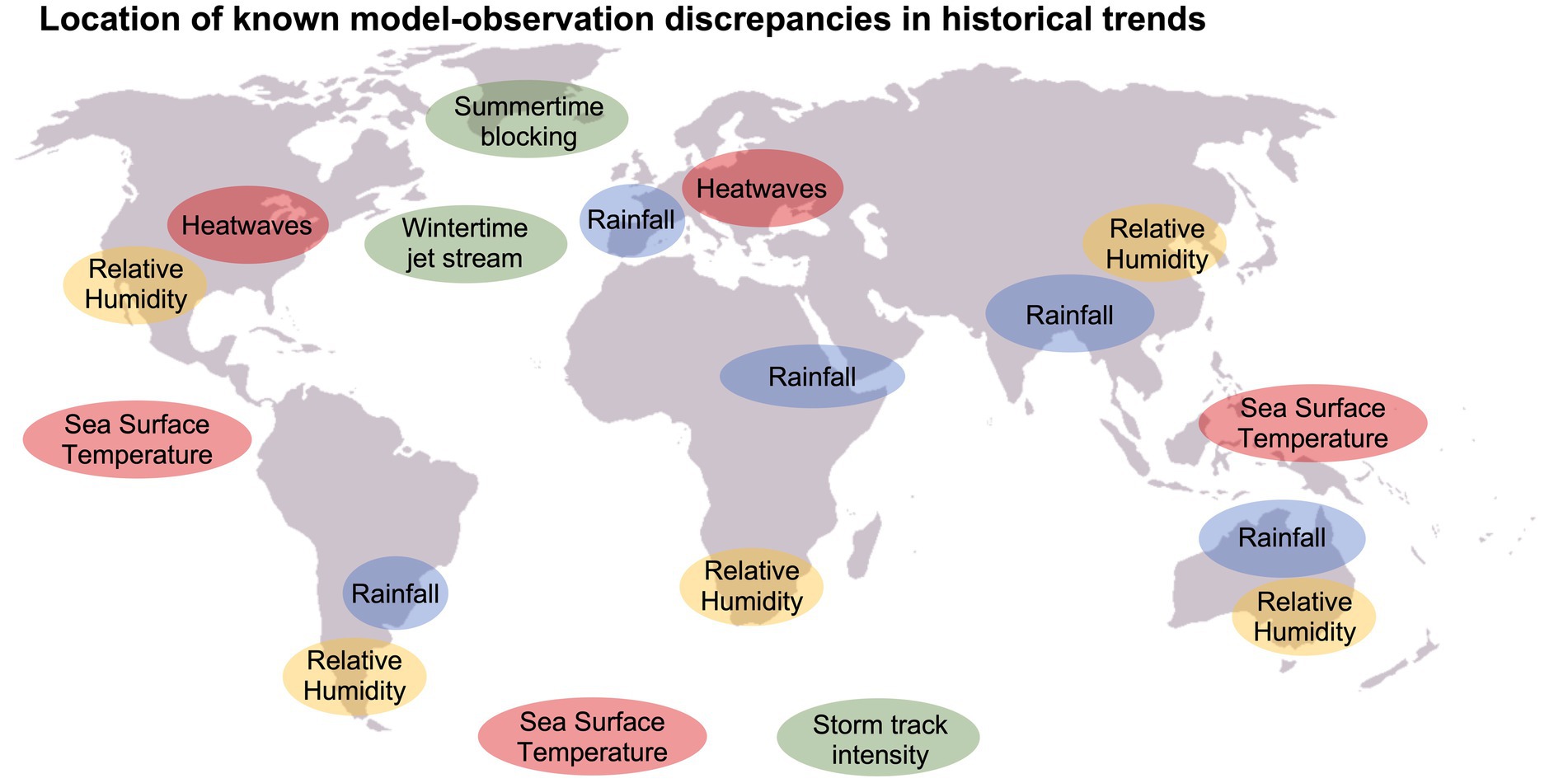
Figure 1. The location of known model-observation discrepancies in historical trends discussed in section 3.
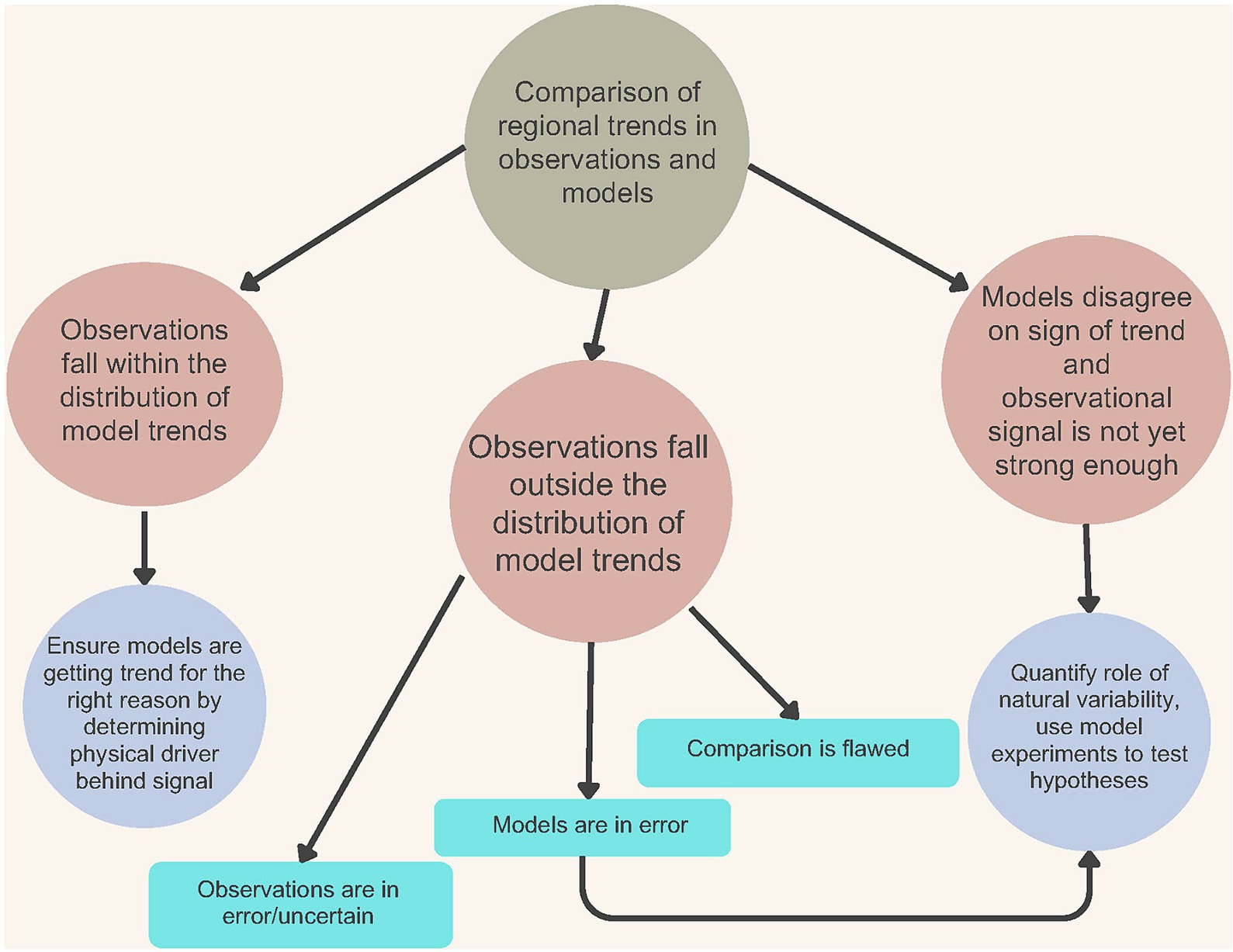
Figure 2. The logical flow chart of possibilities when confronting climate model trends with observations. Based on Schmidt (2013). Image credit: S. Sehrawat.
The accumulating discrepancies are not well understood and confounded by several real and apparent puzzles (e.g., “signal-to-noise paradox,” real-time record-shattering extremes falling outside of the possible range predicted by models) and limitations (e.g., disentangling the role of forcing versus internal climate variability, impact of physical processes not included in the models, forcing uncertainty, persistent model biases, and the limits of instrumental observations), which are also outlined below.
2.1 Discrepancies between modeled and observed regional climate changes
2.1.1 Asian-Australian monsoon rainfall trends
Observed mean summer monsoon rainfall over central-north India exhibited a significant reduction since the 1950s, while the extreme rainfall events manifested a threefold rise (Roxy et al., 2015, 2017; Wang et al., 2021). However, historical simulations across Coupled Model Intercomparison Project (CMIP) generations show an increase in the mean summertime monsoon rainfall over the same region in response to anthropogenic forcing from increasing greenhouse gasses (GHGs) (Saha et al., 2014). IPCC AR6 Chapter 10 concluded multidecadal internal variability and time-evolving aerosol forcing as key reasons for the mismatch in monsoon rainfall (Doblas-Reyes et al., 2021), however, other factors such as observational uncertainty, ocean warming, and anthropogenic changes in land use/land cover have also been proposed to explain this discrepancy (Roxy et al., 2015; Paul et al., 2016; Singh et al., 2019; Jain et al., 2023). These factors are found to have systematic impacts on monsoon rainfall and it is also found that the decadal variability is large enough to easily override the underlying trends due to anthropogenic changes to give temporary trends of opposite sign in rainfall (Huang et al., 2020; Jain and Scaife, 2022). This emphasizes that internal variability can explain the mismatch in observed and model simulated trends (Carvalho et al., 2024).
In contrast, the Australian Summer monsoon has experienced a trend toward higher rainfall since the beginning of the observational record (Dey et al., 2019). At present, the observed trend is not adequately explained, although there are several plausible explanations proposed in the literature, including multidecadal internal variability, GHG forcing, and changes due to remote atmospheric aerosol effects on circulation (Dey et al., 2019). Climate change projections of the Australian monsoon have remained uncertain for multiple successive generations of climate models, with models failing to agree on both the magnitude and direction of changes in monsoon rainfall with global warming (Colman et al., 2011; Brown et al., 2016; Narsey et al., 2020).
2.1.2 Eastern African paradox in rainfall trends
The “East African Climate Paradox” refers to the observed decline in “long rains” (March to May) precipitation in coastal East Africa, originally identified by Rowell et al. (2015) for 1986–2004 but valid for trends beginning since 1950 (Yang et al., 2014). This drying trend contradicts both the precipitation increase simulated in response to changes in radiative forcing to date and the future predictions of wetter long rains in multiple generations of CMIP models (Yang et al., 2014; Rowell et al., 2015; Makula and Zhou, 2022). Wainwright et al. (2019) showed that the reduced precipitation in the long rains comes about from a shortened rainy season as opposed to a reduction of precipitation intensity. Figure 3 shows the model-observation trend discrepancy in long rains for the CMIP6 models from Makula and Zhou (2022).
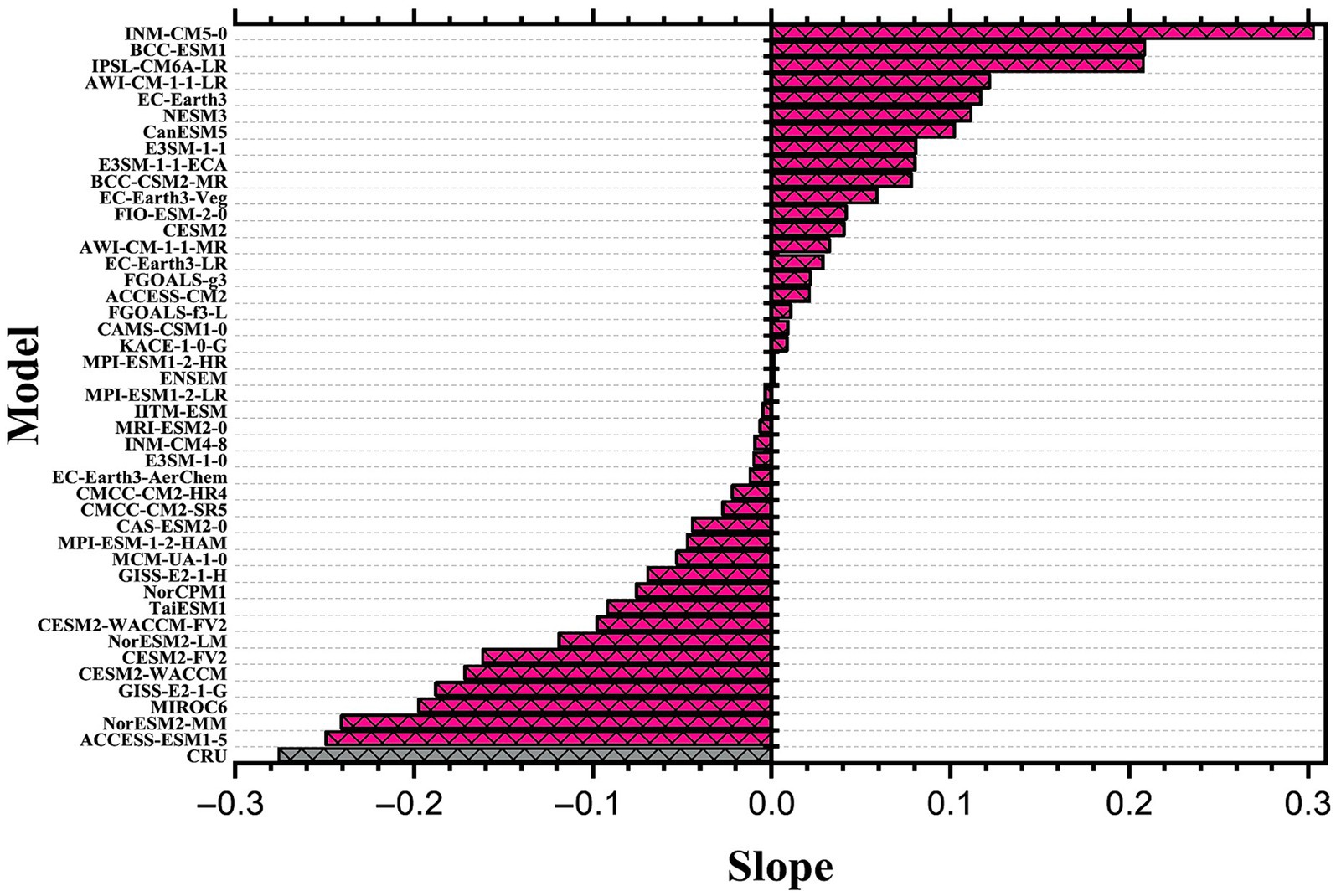
Figure 3. From Makula and Zhou (2022) Sen’s slope (unit mm month−1 year−1) of MAM precipitation averaged over East Africa (area comprising Kenya, Tanzania, and Uganda) for 43 CMIP6 models, the ensemble mean (ENSEM) and CRU from 1950 to 2014.
Only a very small number of individual coupled runs in the multi-model ensemble are able to reproduce the drying recorded in multiple observational data sets while the ensemble mean shows a modest wetting trend (Yang et al., 2014; Rowell et al., 2015). Since the time of “peak paradox,” long rains precipitation totals have increased (Wainwright et al., 2019) and the trend from 1983 to 2021 actually shows a modest wetting in some data sets and little change in others (Palmer et al., 2023). The current consensus is that the observed drying was forced by decadal variations in SSTs in the tropical Indo-Pacific Oceans, presumed natural (Yang et al., 2014; Funk et al., 2018), with a likely additional component of Indo-west Pacific warming driven by rising GHGs (Hoell et al., 2017).
The fact that the observed trends during the “peak paradox” period are almost entirely outside the coupled model ensemble spread, and can be better simulated by SST-forced models, suggests that the coupled models cannot simulate the appropriate decadal ocean variability that drives long rains precipitation over East Africa. At this point we do not know if models are biased in how the rains in East Africa respond to radiative forcing, but the sensitivity to tropical Pacific SSTs suggests the reliability of projections in this region could be a casualty of model biases in simulating both decadal variability and the forced response of the tropical Pacific Ocean (cf. section 2.1.6).
2.1.3 South East South America rainfall trends
Several studies have identified a positive trend in austral summer precipitation over Southeastern South America (SESA) since the beginning of the 20th century, according to different datasets (Gonzalez et al., 2014; Vera and Díaz, 2015; Zhang et al., 2016; Díaz and Vera, 2017; Saurral et al., 2017; Wu and Polvani, 2017; Montini et al., 2019). The trend from the 1950s onwards is explained by the interplay of the Atlantic Multidecadal Variability (AMV), and anthropogenic influence associated with GHG emissions and stratospheric ozone depletion (Doblas-Reyes et al., 2021). Although global models in general simulate positive trends when forced with GHG and stratospheric ozone depletion, they reproduce weaker trends than the observed. The CMIP6 model-observation precipitation trend discrepancy over SESA is shown in Figure 4 from Varuolo-Clarke et al. (2021). To capture the magnitude of the observed trend within an ensemble range, a multi-model SMILE ensemble is needed (Díaz et al., 2021). This is partly due to the biases of CMIP6 models in simulating summer mean precipitation and its interannual variability in the region (Díaz et al., 2021). Internal decadal-to-centennial variability not present in state-of-the-art models, perhaps related to missing ocean–atmosphere or land-ice coupling, may have also played a large role in generating the twentieth-to-twenty-first-century SESA precipitation trend (Varuolo-Clarke et al., 2021).
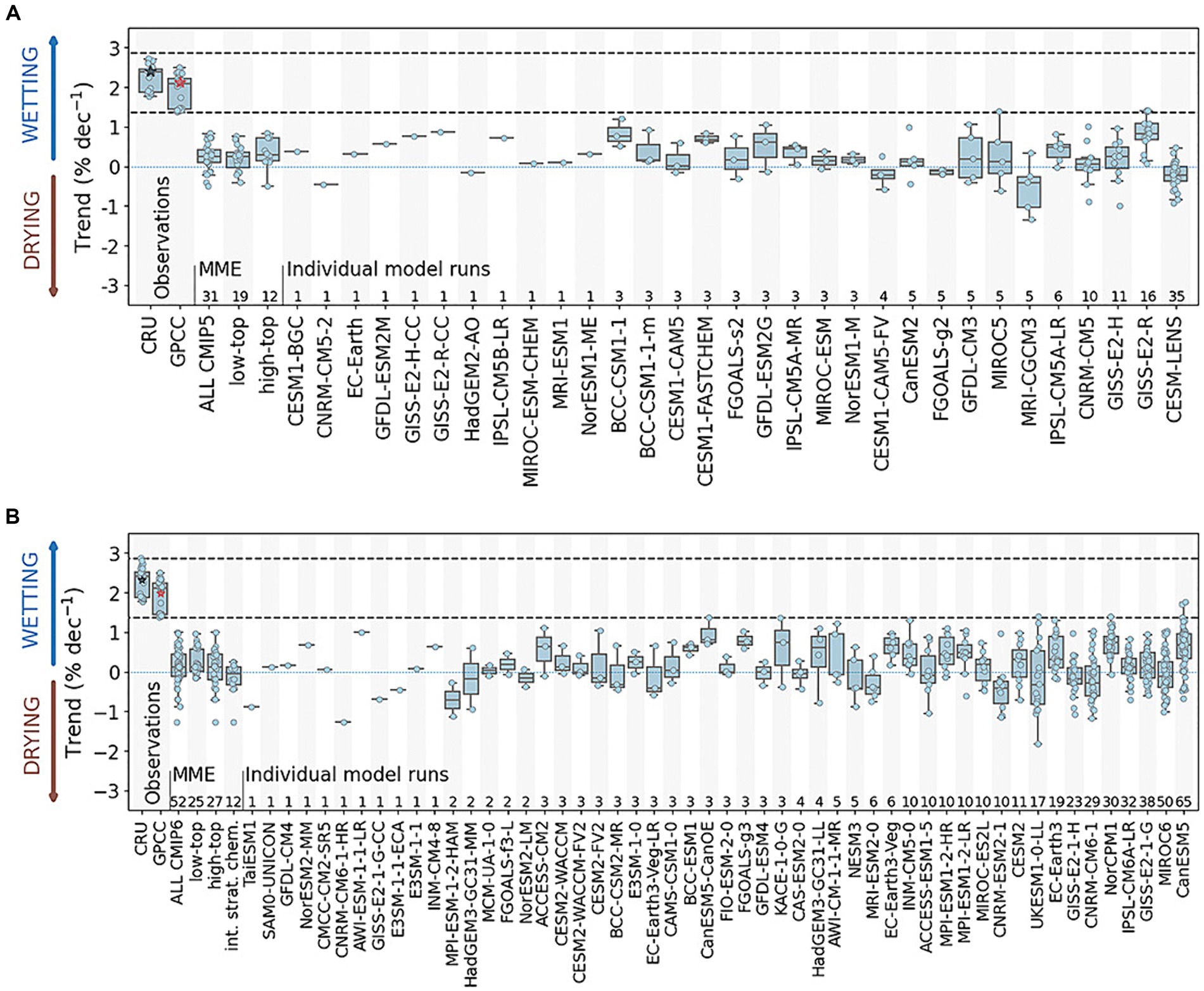
Figure 4. From Varuolo-Clarke et al. (2021). Boxplots of linear trends in DJF precipitation in South East South America calculated as the relative change (%/decade) for historical simulations in (A) CMIP5 and (B) CMIP6 from 1921 to 2005. The observed trends from 1921 to 2005 are shown for CRU (black) and GPCC (red). The black dashed horizontal lines represent the minimum and maximum thresholds of the observational uncertainty based on the full spread of observations between the minimum GPCC trend and the maximum CRU trend, which are 1.36 and 2.86%/decade for the 1902–2019 or 1902–2016 periods. Internal black lines in each boxplot represent the median value of the distribution, the box outlines the interquartile range, and the whiskers are defined as 1.5 times the interquartile range; trends that fall outside this latter range are considered to be outliers. The numbers above each model name indicate the number of models included in the multimodel ensemble (MME) or the number of members included in an individual model ensemble. The gray-and-white-striped background is for visualization only. © American Meteorological Society. Used with permission.
2.1.4 Northern hemisphere extratropical circulation, extreme and hydroclimate trends
Recent trends in Northern Hemisphere wintertime extratropical zonal-mean zonal winds and temperatures are captured reasonably well by the latest generation of coupled models (Woollings et al., 2023). The trends include an upward shift and acceleration of the subtropical jet and an emerging poleward shift. However, regional trends including the strengthening of the wintertime North Atlantic jet from 1951 to 2020, are not well-captured by the recent generation of coupled models (Blackport and Fyfe, 2022). The models similarly have difficulty capturing the observed precipitation trends over Europe (Blackport and Fyfe, 2022). Multi-decadal variability in the North Atlantic is large and models struggle to capture it (Eade et al., 2022); consistently, trends in individual models differ considerably from the multi-model mean trend and from each other (Blackport and Fyfe, 2022).
The Northern Hemisphere summertime circulation, including the jet stream and storm tracks, has weakened significantly over the satellite era since the late 1970s. While only a few select models of the previous generation (CMIP5) captured the weakening in reanalysis data (Kang J. et al., 2023), the most recent generation of coupled models show good agreement with the observed trends. Previous work hypothesized a role of Arctic Amplification and Arctic Sea ice loss (Coumou et al., 2018), however recent work suggests they do not appear to be driving the weakening (Kang J. et al., 2023). Instead, Dong et al. (2022) have highlighted a role for aerosol emissions for the circulation weakening.
While some fidelity has been shown for the weakening of the summertime circulation, models fail to capture an emerging upper-level geopotential height trend that has been suggested to be a circumglobal Rossby wave train (Teng et al., 2022). Similarly, summer blocking frequencies have almost doubled over the last years, primarily due to increasing blocking duration, a trend that is also not captured by CMIP5 climate models (Hanna et al., 2018). Potentially associated with these discrepancies in circulation trends, CMIP6 models fail to capture the rapid rise in heat extremes over western Europe (Van Oldenburg et al., 2022). Recent work indicates that the faster observed increase in heat extremes over Western Europe, as compared to simulations, is due to missed atmospheric circulation trends in climate models (Patterson, 2023; Vautard et al., 2023). Figure 5 shows the model-observation discrepancy in the trend in maximum minus trend in mean index from Patterson (2023). Finally, observed midlatitude summertime drying (relative humidity) trends over land in several regions, including the US South West, is not well captured in CMIP5 and CMIP6 models for reasons that are unclear (Douville and Plazzotta, 2017; Dunn et al., 2017; Douville and Willett, 2023; Simpson et al., 2023).
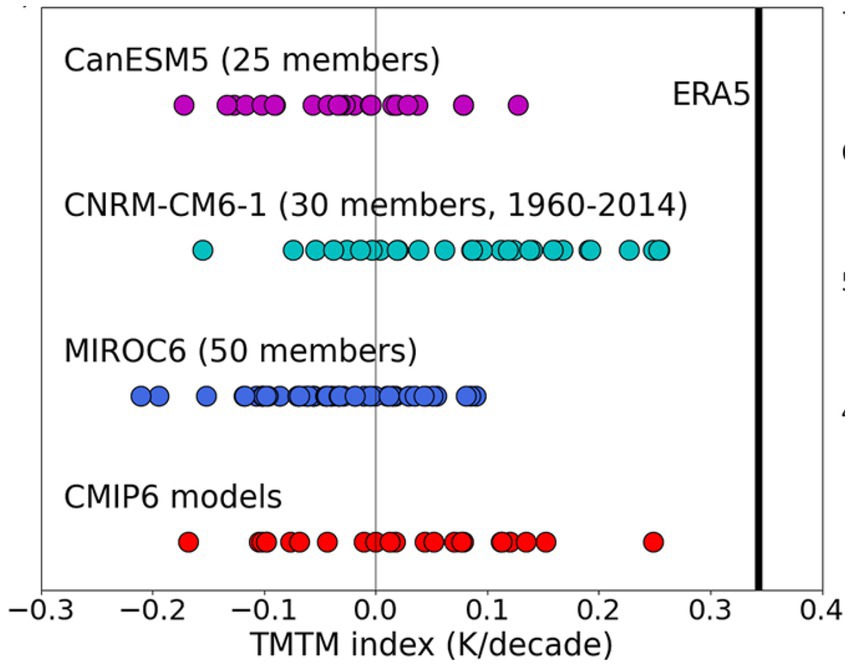
Figure 5. From Patterson (2023). The multi-model mean of the JJA-mean trend of maximum surface air temperature minus the trend in the mean surface air temperature (TMTM) index for CMIP6 models (colors) and ERA5 (thick, black line).
2.1.5 Southern hemisphere extratropical circulation and SSTs
Statistically significant trends have emerged across the Southern Hemisphere extratropics, including a cooling trend over the Southern Ocean (Armour et al., 2016; Wills et al., 2022) and an intensification of the Southern storm tracks (Chemke et al., 2022; Shaw et al., 2022). While both signals have been predicted by climate models (Manabe and Weatherald, 1975; Stouffer et al., 1989), trends in the most recent generation of models exhibit significant discrepancies with observations. In particular, CMIP6 models predict the opposite sign (warming) trend over the Southern Ocean (Wills et al., 2022) and models underpredict the intensification of the storm tracks by a factor of 2–4 depending on the reanalysis product used (Chemke et al., 2022, Shaw et al., 2022). Recent work shows that the inability of models to predict SST trends over the Southern Ocean has implications for regional temperature trends across the globe (Kang S. et al., 2023).
2.1.6 Tropical Pacific SST trends
There is a significant discrepancy between observations and models in recent multi-decadal trends in the spatial pattern of tropical Pacific SSTs and the strength of the Pacific Walker Circulation (Wills et al., 2022). Observations show a strengthening of the east–west (E-W) gradient and Walker Circulation (i.e., La Niña-like) whereas models simulate a weakening of this gradient (i.e., El Niño-like) in both historical and future trends. Such SST trends are expected to impact climate around the Pacific Rim and elsewhere and are linked in models to cloud feedbacks and the global climate sensitivity (e.g., Rugenstein et al., 2023 and references therein). Several mechanisms have been proposed for weakening: constraints on changes in the global hydrological cycle and weakening tropical overturning, differential evaporation damping, rainfall-induced freshwater forcing. In contrast, mechanisms for strengthening focus on the ocean dynamical thermostat, which might be transient and only operative until subsurface and upwelling waters have warmed, warming in the tropical Indian/Atlantic Oceans, aerosol forcing and Southern Ocean cooling. A recent review by Lee et al. (2022) summarizes the different mechanisms. Further, over recent decades (i.e., about 1980 to now) the discrepancy could be a consequence of high amplitude natural decadal variability that CMIP-class models cannot simulate. However, even on longer multidecadal and century timescales the E-W gradient has strengthened in observations and is essentially outside the range of the individual runs in the CMIP6 and Large Ensemble projects (Seager et al., 2022). The challenge is to reconcile and quantify these different trends, the mechanisms involved, and model biases and use this information to predict how the gradient will evolve into the future, including the implications for regional climate.
2.2 Climate model fidelity
The accumulating model-observation discrepancies in historical trends raise many questions related to climate model fidelity and fitness for purpose. While climate model fidelity related to limited resolution is a known concern, several other puzzles have emerged. For example, initial condition ensemble predictions using climate models sometimes show higher correlation with observed variability than with a randomly selected individual member of their own simulation (Scaife and Smith, 2018). Another way of putting this is that the correlations with observations are higher than would be expected from the small signal-to-noise ratios evident in models, even as the total variance in the models is often close in magnitude to the observed variance. These perplexing results are referred to as a ‘signal-to-noise (S/N) paradox’ (c.f. Scaife and Smith, 2018, Smith et al., 2020). It was shown to be particularly pronounced in the Atlantic sector, where the variance in atmospheric circulation measures that is predictable in climate models appears to be too weak in amplitude by a factor of two, or perhaps more (Smith et al., 2020). This implies the North Atlantic Oscillation to be more strongly forced by surface boundary conditions and by solar and volcanic variability in nature than in models. The (S/N) paradox also highlights that one cannot simply use model projections at face value: if the true magnitude of the forced trend is stronger than models imply, then future regional climate change could be well outside the range predicted by models. Given the S/N paradox apparently applies to the North Atlantic Oscillation (NAO), this presents a problem for climate prediction in regions affected by it, including the Mediterranean and Middle East and North Africa, where it exerts a strong influence. At least four (likely complementary and inter-related) explanations have been proposed to explain the S/N paradox - overly weak atmospheric eddy feedback, poor representation of air-sea coupling, poor representation of tropical-extratropical and stratosphere-troposphere coupling processes, and insufficient resolution - and testing these explanations remains an active research area.
Another puzzle related to climate model fidelity is that pacemaker simulations, whereby coupled model SST anomalies are nudged to observations, suggest coupled models can capture regional climate change when SSTs are nudged but cannot capture the trends when free-running (O’Reilly et al., 2023). If this means coupled models are misrepresenting the forced trend, then what are they missing?
2.3 Time evolving anthropogenic forcing
When comparing regional predictions from climate models with observations it is important to assess which emission scenario aligns best with the real world. Indeed, a well-known challenge of climate prediction is scenario uncertainty (Hawkins and Sutton, 2009). Recent work suggests cumulative CO2 emissions are not consistent with the RCP8.5 emission scenario (Hausfather and Peter, 2020). This highlights the importance of weighting model predictions per degree of global warming. In addition to the uncertainty of CO2 emissions, the time evolution of anthropogenic aerosols can be up to a factor of four different across scenarios (Persad et al., 2022) and compared to the pre-2000 era we are now in a period of stratospheric ozone recovery (Banerjee et al., 2020).
Anthropogenic aerosol emissions have been shown to be as important as GHG emissions for recent climate change across many regions. In particular, aerosols have played an important role in the recent decrease in summer monsoon precipitation and circulation in South Asia (e.g., Krishnan et al., 2016; Li et al., 2016; Liu et al., 2016; Lau and Kim, 2017; Lin et al., 2018; Singh et al., 2020). Also, the transition of a wet period in the mid-20th century to a very dry period in the 1970s-1980s over West Africa is attributed to cooling from Northern Hemisphere aerosols (Giannini and Kaplan, 2019; Hirasawa et al., 2020). The recent recovery of the West African monsoon since the mid-1990s is due to the combined effects of increasing GHG and decreasing anthropogenic aerosols (e.g., Dong and Sutton, 2015; Undorf et al., 2018). Aerosol forcing also dominates the recent weakening of the Pacific jet stream (Dong et al., 2022). In many of these cases, radiative forcing associated with decreasing aerosol and increasing GHG drive a similar response, and hence constructively interfere. Thus, the signal emerging in observations is twice as large as it would be in response to a single forcing alone.
Ultimately, the evolution of regional climate change over the next several decades will depend on how GHG, aerosol and stratospheric ozone forcing evolve and interact, in particular whether the forcings constructively or destructively interfere regionally. For example, radiative forcing associated with increasing aerosols has led to a decrease in Northern Hemisphere land monsoon precipitation over the past century, but this link is expected to disappear as anthropogenic aerosol emissions decrease in the future (Jiang et al., 2023). This time-evolving response represents a significant source of uncertainty for regional climate change projections and should be factored into climate risk assessments (Persad et al., 2022). In addition to the time-evolving impact of atmospheric composition another important and underappreciated time evolving anthropogenic forcing is land use change. For example, uncertainty in land use scenarios could translate to large uncertainty in CO2 emissions, which may lead to discrepant climate projections in emissions-driven climate simulations (Pongratz et al., 2021). At the same time, models tend to produce diverse physical responses to land use change, which may also result in uncertainty in projecting regional climate change (Tebaldi et al., 2023).
2.4 The “known-unknown” drivers of regional climate
Regional climate prediction is subject to a number of known-unknown unknowns related to physics that are missing from climate models that may impact the overall prediction skill. For example, the current generation of coupled climate models miss important physical processes such as changes in Antarctic meltwater, mesoscale processes, irrigation and groundwater (McDermid et al., 2023) and complex terrestrial and coastal ecosystem dynamics and feedback cycles.
While some studies have attempted to include missing physics, e.g., by imposing Antarctic meltwater trends missing from the models (Roach et al., 2023) or resolving mesoscale ocean eddies (Yeager et al., 2023) to improve Southern Ocean and Pacific SST observation-model discrepancy, their quantitative impact and robustness across models are largely unknown. Furthermore, mesoscale processes, e.g., tropical cyclones like Storm Daniel (Copernicus, 2023a) and severe convective storms, have not been accessible with current computing power, representing another “known-unknown,” potentially introducing significant sources of uncertainty for regional climate change predictions. As discussed below, the ongoing push toward higher resolution models may reveal previously unrecognized drivers of regional climate related to coupling between scales (mountain regions, coastal regions, small scale storms) and potential upscale impacts. The known missing processes in climate models loom and beg the question what else might climate models be missing? In other words, what are the “unknown unknowns”?
2.5 Communicating regional climate change, including record-shattering extremes
By far, 2023 was the hottest year on record and over the past several years, such record-shattering extremes have been accumulating regionally. Observed extremes are generally a manifestation of internal variability acting together with the response to anthropogenic forcing. However, communicating these complex interactions to the public is challenging. In the media, the question whether an event is due to climate change is often asked, but this question does not usually have a clear answer. Framing the question in terms of attribution, e.g., how frequent or how much more intense such events have become due to climate change, is more meaningful (Lloyd and Shepherd, 2023).
In addition, as noted above, there are multiple dimensions to consider when answering questions related to emerging regional climate changes: role of different forcings, role of internal variability and model fidelity in representing the underlying mechanisms. CO2 forcing may not be the sole contributor to emerging regional climate changes and individual record-shattering events. For example, trends in European heatwaves have been attributed to both GHG and aerosol forcing (Dong et al., 2017; Freychet et al., 2019) and the 2023 record-shattering marine heat wave over the North Atlantic basin may have been boosted by Saharan dust (Copernicus, 2023b), though no formal attribution has been reported. Indeed, it seems that in many regions the magnitude of emerging trends is related to the additivity of forcings. Along similar lines, record-shattering extremes and emerging regional climate trends can be boosted because internal variability and anthropogenic forcing constructively interfere. In addition, internal variability could interact with anthropogenic forcing to boost the signal.
Model fidelity in representing extremes is also important. Recent research indicates heat wave trends across Europe are underestimated in coupled models (Patterson, 2023; Vautard et al., 2023), raising questions about model fidelity for predicting future changes in heatwaves. Furthermore, recent record-shattering events, e.g., 2021 Pacific Northwest heat wave (White et al., 2023), 2023 North Atlantic marine heat wave and record low Antarctic sea ice (Purich and Doddridge, 2023) were not predicted in advance by climate models. There is also a misconception that the models cannot capture record-breaking extremes. However, there are events that models did capture (e.g., Fischer et al., 2023) but due to smaller/limited ensemble size of the climate simulations, it was not possible to sample the full range of internal variability that is needed to boost the signal. Methodologies like ensemble boosting (Fischer et al., 2023) can be useful to use climate models to predict extremes. However, there are still important questions regarding whether the probability of such rare events in current models is the same as that in the real world and ultimately whether models are fit for purpose (capture the underlying physical mechanisms).
3 Ways forward
3.1 Prioritize resources for understanding regional climate projections and analyzing where and why models go wrong
Historically, the key objective of community efforts like the CMIP and the Coordinated Regional Climate Downscaling Experiment (CORDEX) are to provide sets of standardized simulations for a systematic evaluation and benchmarking of climate models. Over the successive generations of models, much has been learned about how regional climate will unfold including reliable predictions and consensus (see the 2021 Nobel prizes in Physics). CMIP has also been used to detect the fingerprint of climate change in the temperature record (Santer et al., 1996). The learning from this project was immense. Three decades later, with CMIP in its 6th cycle, encompassing ∼120 models, over 300 experiments (Petrie et al., 2021), and 23 endorsed MIPs (Eyring et al., 2016), the project has grown exponentially in scale.
Similarly, the CORDEX framework has enabled an unprecedented number of coordinated regional climate model simulations that cover most continental areas. CORDEX has enabled the identification of robust climate change signals over different CORDEX domains (e.g., Jacob et al., 2020 and Dosio 2016 for the EURO-CORDEX domain; Sanjay et al., 2017 for the South Asia domain; Maúre et al. (2018) for the African domain). CORDEX Flagship Pilot Studies (FPSs) were initiated in 2016 to specifically tackle scientific questions for any given region of the world for which current RCMs are still unable to reproduce the regional climate features adequately. As of 2021, there were 13 FPSs over 5 regions and an additional 4 FPSs having been endorsed. Recent studies are tackling topics that require coordinated convection permitting simulations over several sub-continental regions, e.g., over Europe and South America (Coppola et al., 2020; Bettolli et al., 2021).
Massive endeavors like CMIP and CORDEX not only consume research time and resources but also play a crucial role in determining the scientific direction of our field, as they decide which model data are available and considered relevant. Therefore, it is necessary to revisit and potentially redefine the objectives of such large-scale projects to ensure that they continue to meet the research needs of our community as well as society. In a recent paper, a group of early-mid career researchers have raised a call on the risk of model-intercomparison becoming a quality-control activity in place of a creative research endeavor (Jain et al., 2022). Others have argued that CMIP should be operationalized to better support decision making (Jakob et al., 2023; Stevens 2024).
Analysis from the previous versions of CMIP have unveiled a number of discrepancies. Many of those are long-standing for about two decades (see examples in Section 2). More coordinated and targeted efforts are needed toward solving these stubborn discrepancies and biases in the models. It is important to take a balanced approach. In particular, not only push the model frontier in terms of properly resolving small-scale processes via high resolution modeling (as discussed in Section 3.2) but also invest more time and effort in understanding what is going wrong in the models since the discrepancies may not disappear solely by increasing horizontal resolution. Given the uncertainties in the forced circulation response and the discrepancies between modeled and observed trends discussed here, moving beyond the classical Detection and Attribution (D&A) paradigm — i.e. first detect a statistically significant trend in observations, then attribute it to anthropogenic forcing using the consensus from climate models is important. Instead, it would be valuable to articulate physically plausible hypotheses for the observed changes (in terms of causal processes that are well established by the relevant expert groups, e.g., physical drivers), which can be then assessed using models (Lloyd and Shepherd, 2023).
3.2 Leverage tools to understand model-observation discrepancies and reveal new drivers
Several new tools are emerging that provide new opportunities to potentially address climate model-observation discrepancies as well as reveal new local drivers of regional climate that are not included in lower resolution models. Storm resolving model data is now becoming regionally available, for example over Europe through CORDEX FPS convection (Coppola et al., 2020) or for the Lake Victoria area through CORDEX FPS Lake Victoria (van Lipzig et al., 2023), and globally in the next years through the digital twin developed within the Destination Earth project (Hoffmann et al., 2023). A few examples for processes where storm resolving simulations bring demonstrated benefit are the dynamics of the North Atlantic jet (Schemm, 2023), precipitation in complex orography (Ban et al., 2021), convection and circulation over Lake Victoria (Van de Walle et al., 2020), local features around islands in the Caribbean (Martinez et al., 2024), the subtropical Atlantic (Gao et al., 2023) and modeling lake-effect snow around the Tibetan Plateau (Lin et al., 2023). Higher resolution models, particularly for the ocean, have also been shown to have more fidelity in capturing SST trends than lower resolution models (Yeager et al., 2023). The push to higher resolution modeling will help the community better understand which discrepancies are connected to drivers that are not included in lower resolution models.
The current generation of coupled models also suffer from significant structural uncertainty related to the empirical parameterizations of subgrid scale physics. Machine learning methods have emerged as a promising new tool for parameterization development (e.g., Rasp et al., 2018; Wang P. et al., 2022; Yuval and O’Gorman, 2023; Beucler et al., 2024), for correcting (Bretherton et al., 2022) and understanding (Silva et al., 2022; Wang S. S. C. et al., 2022) model biases, and for downscaling (e.g., Leinonen et al., 2021; Miralles et al., 2022; Sekiyama et al., 2023). Furthermore, causal discovery and interpretable and explainable Artificial Intelligence (XAI) are also potentially useful for identifying drivers of the discrepancies and understanding where and why models are deviating from observations (e.g., Gregory et al., 2023). However, the interpretability of and trust in these data-driven approaches remains challenging (Rudin, 2019; Barnes et al., 2022).
Discrepancies in the current generation of models could also be better understood by leveraging perturbed parameter ensembles, mechanism denial and nudging experiments. Perturbed parameter ensembles for the historical period could reveal what parameters exert significant control on regional climate trends. The most significant parameters could then be targets for new observational campaigns. Mechanism denial experiments, whereby a physical process is disabled or held fixed in the model, could be used to understand the role of specific mechanisms underlying the discrepancy. Numerical and statistical models also allow removing processes or variables to explore their role in forming an extreme event or a trend and their interactions with other processes (e.g., Wehrli et al., 2022). Finally, nudging experiments in which mean-state biases are removed in run-time can lead to more reliable predictions (Krinner et al., 2020). These approaches could be used to better understand outstanding discrepancies, puzzles and limitations, but require time and investment from modeling centers.
3.3 More involvement of regional scientists in studying regional climate change
Making progress on regional climate change research requires the involvement of scientists from the region under study. Scientists working on a regional scale are usually better acquainted with the knowledge that is needed to address climate change in their region of study. This may include identification of the most relevant or impactful climate risks (e.g., wet getting wetter or wet getting drier), challenges and limitations of observational data, possible sources of errors in the modeling systems, or capacity to deliver services.
Observations sit at the heart of model improvement and scientists working on a regional scale are more familiar with the historical records (e.g., what changes were made to the instruments and climate stations, how the quality control of the data was done), which can be crucial for interpreting local record-shattering events and impact of different forcings. They usually also have a better understanding of the different cultural and institutional dynamics, and wider networks across different sectors (such as governmental and industrial) within their region, and therefore have access to exclusive observational data (e.g., radar data, coastal data in the exclusive economic zone). This could be useful to resolve some of the observational discrepancies and help in the translation of climate information to policy. In summary, this knowledge and understanding of the region brings value which is irreplaceable.
A greater involvement of scientists from the region in studies of regional climate change would automatically increase the involvement of scientists from the Global South. However, our continued reluctance in the international climate science community to include more climate scientists from the Global South in international scientific endeavors, especially in leading positions, is reflected in multiple ways. A clear example of how the scientific literature is dominated by the Global North is the Contribution of Working Group I (The Physical Basis) to the Sixth Assessment Report of the Intergovernmental Panel on Climate Change, which relied on the assessment of about 13,500 citations from which 99.95% were written in English and 75% included at least one author based in either the United States or the United Kingdom (Carbon Brief, 2023). There are multiple barriers for climate scientists from the Global South to publish in recognized journals, ranging from barriers to conduct science because of lack of funding to divergence from western academic culture of redaction of papers given the anglocentric language (Carbon Brief, 2021). There is also a perception among scientists from the Global South conducting regional research that their submissions are often rejected without revision with the argument that the study is too regional, even while regional studies from Global North scientists are deemed publishable (e.g., Brainard, 2022).
Historically, the leadership within the World Climate Research Program (WCRP) and its projects has also been dominated by Global North scientists. However, top-down efforts are now being made within the WCRP to improve the diversity and bring more scientists from the Global South on board. One such example is WCRP My Climate Risk Lighthouse Activity, which involves scientists from different regions, particularly the Global South, taking scientific leadership roles and designing the scientific course of their projects. This also highlights that structural changes initiated top-down are important to include scientists from the regions of interest. Initiatives such as the WCRP My Climate Risk Lighthouse Activity make it possible to develop a ‘bottom-up’ approach to regional climate risk, which considers the synergies, interactions and contributions from regional scientists, communities, governments, and decision makers (e.g., Rodrigues and Shepherd, 2022).
Capacity building, and in particular providing resources for scientists at regional institutions in the Global South to have as productive a career as they would otherwise have were they to relocate, is an urgent task for the Global South. This may require establishing equitable global partnerships, enforcing the principles and practices of open science and data, adequate financing, including national funding agencies supporting projects that focus on capacity building in the Global South through collaborative projects and technology transfer (e.g., Schipper et al., 2021; Else, 2022; Gewin, 2023).
3.4 Improved regional climate communication
Communicating regional climate change to the public, media and stakeholders is challenging because of the conflating influences of different forcings, internal variability, and limitations of model fidelity, as discussed above. Storylines of the forced circulation response developed based on physical hypotheses of how different dynamical drivers respond to climate change have emerged as one approach for communicating regional climate change (Lloyd and Shepherd, 2023). In this way, uncertainties around the regional circulation response to climate change can be represented in a physically interpretable fashion which can be reduced as knowledge advances in the future.
More generally, in order to provide actionable information to stakeholders and the media, climate scientists need more support (Swain, 2023) and improved methods of regional climate communication, e.g., Copernicus, Climate ADAPT, Findlater et al. (2021). It is important to take stock and leverage what is being learned from climate services (Boon et al., 2022). While climate services are largely English-dominated, it may be important to ensure that regional climate communication is also delivered in regional languages to break through the language barriers and to make sure that climate services and engagement happen at a grassroot level (Hall et al., 2022).
4 Recommendations
We are in a critical time for regional climate change research and development. The climate change signal has emerged across different variables, regions, and seasons and there is in general a consensus in terms of thermodynamic responses. At the same time, uncertainties regarding the hydrological cycle and dynamic responses remain, and discrepancies between model predicted and observed climatic changes (such as regional trends) are also accumulating. We need to find ways to represent and communicate this web of certainty and uncertainty in a way that can be used in decision-making, which is not an uncommon situation (Marchau et al., 2019). Uncertainty does not necessarily mean a lack of information: for example, even if mean precipitation trends in a region may be uncertain, the region may nevertheless face both heavier precipitation and more severe agricultural drought. Based on physical understanding, informative and decision-relevant statements can be made in a conditional manner, given the present state of knowledge.
Addressing the puzzles and discrepancies outlined in this perspective article should be a priority of the global climate science community and funding agencies. It would involve a balanced approach involving the mass production of climate scenario runs and in addition a serious community-based effort toward addressing the discrepancies between observations and models on a regional scale, and advancing our understanding of regional climate change signals. This work to understand, address, and hopefully resolve discrepancies should be built into the cycle of designing and running scenarios. Many existing and emerging tools, e.g., storm resolving models, models of intermediate complexity, and AI/ML methods, can be leveraged to address these discrepancies. It is important to involve scientists from the region of interest in initiatives targeted toward studying regional climate change, as they are better acquainted with particular regional challenges, for example, the quality of regional observational and monitoring networks and the dynamical and physical processes responsible for variability and change in the region.
Targeted capacity building on the technical aspects of handling big climate data (e.g., from CMIP or CORDEX and Destination Earth), e.g., using Cloud-based services, advanced computation and statistical analysis, and process-based understanding of regional climate change, would be some steps to support regional science communities. It is equally important to ensure continued access to data and data analysis facilities. The WCRP Academy could support the global climate science community and its goal to understand regional climate change through co-learning initiatives, e.g., regional climate analysis including technical aspects of analyzing CMIP/CORDEX simulations, accessing and analyzing very high resolution global climate data, AI based data and the physics and dynamics of regional drivers. The next several decades represent a pivotal time for building a more regionally inclusive climate science community that is well equipped to address regional climate change discrepancies, improve regional climate change predictions and reduce uncertainties.
Author contributions
TAS: Conceptualization, Writing – original draft, Writing – review & editing. PA: Conceptualization, Writing – original draft, Writing – review & editing. MC: Conceptualization, Writing – original draft, Writing – review & editing. DC: Conceptualization, Writing – original draft, Writing – review & editing. AD: Conceptualization, Writing – original draft, Writing – review & editing. CG: Conceptualization, Writing – original draft, Writing – review & editing. SJ: Conceptualization, Writing – original draft, Writing – review & editing. MR: Conceptualization, Writing – original draft, Writing – review & editing. MK: Conceptualization, Writing – original draft, Writing – review & editing. LL: Conceptualization, Writing – original draft, Writing – review & editing. SN: Conceptualization, Writing – original draft, Writing – review & editing. OM: Conceptualization, Writing – original draft, Writing – review & editing. RS: Conceptualization, Writing – original draft, Writing – review & editing. TGS: Conceptualization, Writing – original draft, Writing – review & editing. AS: Conceptualization, Writing – original draft, Writing – review & editing. TS: Conceptualization, Writing – original draft, Writing – review & editing. MT: Conceptualization, Writing – original draft, Writing – review & editing. LW: Writing – review & editing, Conceptualization, Writing – original draft.
Funding
The author(s) declare that financial support was received for the research, authorship, and/or publication of this article. TS acknowledges support from NSF (AGS-2300037) and NOAA (NA23OAR4310597). RS was supported by NSF awards AGS-2127684 and OCE-2219829. CG acknowledges the support of the Israel Science Foundation (grant agreement 1727/21).
Acknowledgments
The authors acknowledge the participants of the WCRP Open Science Conference that took place 23-27 October 2023 in Kigali, Rwanda.
Conflict of interest
The authors declare that the research was conducted in the absence of any commercial or financial relationships that could be construed as a potential conflict of interest.
The author(s) declared that they were an editorial board member of Frontiers, at the time of submission. This had no impact on the peer review process and the final decision.
Publisher’s note
All claims expressed in this article are solely those of the authors and do not necessarily represent those of their affiliated organizations, or those of the publisher, the editors and the reviewers. Any product that may be evaluated in this article, or claim that may be made by its manufacturer, is not guaranteed or endorsed by the publisher.
References
Armour, K., Marshall, J., Scott, J., Donohoe, A., and Newsom, E. R. (2016). Southern Ocean warming delayed by circumpolar upwelling and equatorward transport. Nat. Geosci. 9, 549–554. doi: 10.1038/ngeo2731
Ban, N., Caillaud, C., Coppola, E., Pichelli, E., Sobolowski, S., Adinolfi, M., et al. (2021). The first multi-model ensemble of regional climate simulations at kilometer-scale resolution, part I: evaluation of precipitation. Clim. Dyn. 57, 275–302. doi: 10.1007/s00382-021-05708-w
Banerjee, A., Fyfe, J. C., Polvani, L. M., Waugh, D., and Chang, K. L. (2020). A pause in southern hemisphere circulation trends due to the Montreal protocol. Nature 579, 544–548. doi: 10.1038/s41586-020-2120-4
Barnes, E. A., Barnes, R. J., Martin, Z. K., and Rader, J. K. (2022). This looks like that there: Interpretable neural networks for image tasks when location matters. Art. Intell. Earth Syst. 2022:1. doi: 10.1175/AIES-D-22-0001.1
Bettolli, M. L., Solman, S. A., da Rocha, R. P., Llopart, M., Gutierrez, J. M., Fernández, J., et al. (2021). The CORDEX flagship pilot study in southeastern South America: a comparative study of statistical and dynamical downscaling models in simulating daily extreme precipitation events. Clim. Dyn. 56, 1589–1608. doi: 10.1007/s00382-020-05549-z
Beucler, T., Gentine, P., Yuval, J., Gupta, A., Peng, L., Lin, J., et al. (2024). Climate-invariant machine learning. Science. Advances 10:250. doi: 10.1126/sciadv.adj7250
Blackport, R., and Fyfe, J. (2022). Climate models fail to capture strengthening wintertime North Atlantic jet and impacts on Europe. Sci. Adv. 8:3112. doi: 10.1126/sciadv.abn3112
Boon, E., Wright, S. J., Biesbroek, R., Goosen, H., and Ludwig, F. (2022). Successful climate services for adaptation: what we know, don’t know and need to know. Clim. Serv. 27:100314. doi: 10.1016/j.cliser.2022.100314
Brainard, J. (2022). Reviewers award higher marks when a paper’s author is famous: “Matthew effect” is powerful, unusually large study finds. Science 377:1251. doi: 10.1126/science.ade8721
Bretherton, C. S., Henn, B., Kwa, A., Brenowitz, N. D., Watt-Meyer, O., McGibbon, J., et al. (2022). Correcting coarse-grid weather and climate models by machine learning from global storm-resolving simulations. J. Adv. Earth Sys. Mod. 2022:2794. doi: 10.1029/2021MS002794
Brown, J. R., Moise, A. F., Colman, R., and Zhang, H. (2016). Will a warmer world mean a wetter or drier Australian monsoon? J. Clim. 29, 4577–4596. doi: 10.1175/JCLI-D-15-0695.1
Carbon Brief . (2021). Analysis: The lack of diversity in climate-science research. Available at: https://www.carbonbrief.org/analysis-the-lack-of-diversity-in-climate-science-research/.
Carbon Brief . (2023). Guest post: What 13,500 citations reveal about the IPCC’s climate science report. Available at: https://www.carbonbrief.org/guest-post-what-13500-citations-reveal-about-the-ipccs-climate-science-report/.
Carvalho, L., Suryachander, R., et al. (2024). Global and regional monsoons, Frontiers in climate, to be submitted.
Chemke, R., Yuval, J., and Ming, Y. (2022). The intensification of winter mid-latitude storm tracks in the southern hemisphere. Nat. Clim. Chang. 12, 553–557. doi: 10.1038/s41558-022-01368-8
Colman, R. A., Moise, A. F., and Hanson, L. I. (2011). Tropical Australian climate and the Australian monsoon as simulated by 23 CMIP3 models. J. Geophys. Res. 116:149. doi: 10.1029/2010JD015149
Copernicus . (2023a). Storm Daniel causes flooding in Libya. Available at: https://www.copernicus.eu/en/media/image-day-gallery/storm-daniel-causes-flooding-libya.
Copernicus . (2023b). Aerosols: Are SO2 emissions reductions contributing to global warming? Available at: https://atmosphere.copernicus.eu/aerosols-are-so2-emissions-reductions-contributing-global-warming.
Coppola, E., Sobolowski, S., Pichelli, E., Raffaele, F., Ahrens, B., Anders, I., et al. (2020). A first-of-its-kind multi-model convection permitting ensemble for investigating convective phenomena over Europe and the Mediterranean. Clim. Dyn. 55, 3–34. doi: 10.1007/s00382-018-4521-8
Coumou, D., di Capua, G., Vavrus, S., Wang, L., and Wang, S. (2018). The influence of Arctic amplification on mid-latitude summer circulation. Nat. Commun. 9:2959. doi: 10.1038/s41467-018-05256-8
Coumou, D., Lehmann, J., and Beckmann, J. (2015). The weakening summer circulation in the northern hemisphere mid-latitudes. Science 348, 324–327. doi: 10.1126/science.1261768
Dey, R., Lewis, S. C., Arblaster, J. M., and Abram, N. J. (2019). A review of past and projected changes in Australia’s rainfall. WIREs Clim. Change 10:577. doi: 10.1002/wcc.577
Díaz, L. B., Saurral, R. I., and Vera, C. S. (2021). Assessment of South America summer rainfall climatology and trends in a set of global climate models large ensembles. Int. J. Climatol. 41, E59–E77. doi: 10.1002/joc.6643
Díaz, L. B., and Vera, C. S. (2017). Austral summer precipitation interannual variability and trends over southeastern South America in CMIP5 models. Int. J. Climatol. 37, 681–695. doi: 10.1002/joc.5031
Doblas-Reyes, F. J., Sörensson, A. A., Almazroui, M., Dosio, A., Gutowski, W. J., Haarsma, R., et al. (2021). “Linking global to regional climate change” in Climate change 2021: The physical science basis. eds. V. Masson-Delmotte, P. Zhai, A. Pirani, S. L. Connors, C. Péan, and S. Berger, et al. (Cambridge, UK: Cambridge University Press), 1363–1512.
Dong, B., and Sutton, R. (2015). Dominant role of greenhouse-gas forcing in the recovery of Sahel rainfall. Nat. Clim. Change 5, 757–760. doi: 10.1038/nclimate2664
Dong, B., Sutton, R. T., and Shaffrey, L. (2017). Understanding the rapid summer warming and changes in temperature extremes since the mid-1990s over Western Europe. Clim. Dyn. 48, 1537–1554. doi: 10.1007/s00382-016-3158-8
Dong, B., Sutton, R. T., Shaffrey, L., and Harvey, B. (2022). Recent decadal weakening of the summer Eurasian westerly jet attributable to anthropogenic aerosol emissions. Nat. Commun. 13:1148. doi: 10.1038/s41467-022-28816-5
Douville, H., and Plazzotta, M. (2017). Midlatitude summer drying: an underestimated threat in CMIP5 models. Geophys. Res. Lett. 44, 9967–9975. doi: 10.1002/2017GL075353
Douville, H., and Willett, K. M. (2023). A drier than expected future, supported by near-surface relative humidity observations. Sci. Adv. 9:eade6253. doi: 10.1126/sciadv.ade6253
Dunn, R. J. H., Willett, K. M., Ciavarella, A., and Stott, P. A. (2017). Comparison of land surface humidity between observations and CMIP5 models. Earth Syst. Dynam. 8, 719–747. doi: 10.5194/esd-8-719-2017
Eade, R., Stephenson, D. B., Scaife, A. A., and Smith, D. M. (2022). Quantifying the rarity of extreme multi-decadal trends: how unusual was the late twentieth century trend in the North Atlantic oscillation? Clim. Dyn. 58, 1555–1568. doi: 10.1007/s00382-021-05978-4
Else, H. (2022). African researchers lead campaign for equity in global collaborations. Nature 606:636. doi: 10.1038/d41586-022-01604-3
Eyring, V., Bony, S., Meehl, G. A., Senior, C. A., Stevens, B., Stouffer, R. J., et al. (2016). Overview of the coupled model Intercomparison project phase 6 (CMIP6) experimental design and organization. Geosci. Model Dev. 9, 1937–1958. doi: 10.5194/gmd-9-1937-2016
Eyring, V., Gillett, N. P., Rao, K. M. A., Barimalala, R., Parrillo, M. B., Bellouin, N., et al. (2021). “Human influence on the climate system” in Climate change 2021: The physical science basis. eds. V. Masson-Delmotte, P. Zhai, A. Pirani, S. L. Connors, C. Péan, and S. Berger (Cambridge, UK: Cambridge University Press), 1363–1512.
Findlater, K., Webber, S., Kandlikar, M., and Donner, S. (2021). Climate services promise better decisions but mainly focus on better data. Nat. Clim. Chang. 11, 731–737. doi: 10.1038/s41558-021-01125-3
Fischer, E. M., Beyerle, U., Bloin-Wibe, L., Gessner, C., Humphrey, V., Lehner, F., et al. (2023). Storylines for unprecedented heatwaves based on ensemble boosting. Nat. Commun. 14:4643. doi: 10.1038/s41467-023-40112-4
Freychet, N., Tett, S. F. B., Bollasina, M., Kang, K. C., and Hergel, G. C. (2019). The local aerosol emission effect on surface shortwave radiation and temperatures. J. Adv. Earth Syst. Mod. 11:1530. doi: 10.1029/2018MS001530
Funk, C., Harrison, L., Shukla, S., Pomposi, C., Galu, G., Korecha, D., et al. (2018). Examining the role of unusually warm indo-Pacific Sea-surface temperatures in recent African droughts. Quart. J. Roy. Met. Soc 144, 360–383. doi: 10.1002/qj.3266
Gao, K., Harris, L., Bender, M., Chen, J.-H., Zhou, L., and Knutson, T. (2023). Regulating fine-scale resolved convection in high-resolution models for better hurricane track prediction. Geophys. Res. Lett. 50:329. doi: 10.1029/2023GL103329
Gewin, V. (2023). Pack up the parachute: why global north–south collaborations need to change. Nature 619, 885–887. doi: 10.1038/d41586-023-02313-1
Giannini, A., and Kaplan, A. (2019). The role of aerosols and greenhouse gases in Sahel drought and recovery. Clim. Chang. 152, 449–466. doi: 10.1007/s10584-018-2341-9
Gonzalez, P. L. M., Polvani, L. M., Seager, R., and Correa, G. J. P. (2014). Stratospheric ozone depletion: a key driver of recent precipitation trends in south eastern South America. Clim. Dyn. 42, 1775–1792. doi: 10.1007/s00382-013-1777-x
Gregory, W., Bushuk, M., Adcroft, A., Zhang, Y., and Zanna, L. (2023). Deep learning of Systematic Sea ice model errors from data assimilation increments. J. Adv. Earth Syst. Model. 15:3757. doi: 10.1029/2023MS003757
Hall, C. A., Illingworth, S., Mohadjer, S., Roxy, M. K., Poku, C., Otu-Larbi, F., et al. (2022). GC insights: diversifying the geosciences in higher education: a manifesto for change. Geosci. Commun. 5, 275–280. doi: 10.5194/gc-5-275-2022
Hanna, E., Fettweis, X., and Hall, R. J. (2018). Brief communication: recent changes in summer Greenland blocking captured by none of the CMIP5 models. Cryosphere 12, 3287–3292. doi: 10.5194/tc-12-3287-2018
Hausfather, Z., and Peter, G. P. (2020). Emissions - the ‘business as usual’ story is misleading. Nature 577, 618–620. doi: 10.1038/d41586-020-00177-3
Hawkins, E., and Sutton, R. (2009). The potential to narrow uncertainty in regional climate predictions. Bull. Am. Met. Soc. 90, 1095–1108. doi: 10.1175/2009BAMS2607.1
Hirasawa, H., Kushner, P. J., Sigmond, M., Fyfe, J., and Deser, C. (2020). Anthropogenic aerosols dominate forced multidecadal Sahel precipitation change through distinct atmospheric and oceanic drivers. J. Clim. 33:10187. doi: 10.1175/JCLI-D-19-0829.1
Hoell, A., Hoerling, M., Eischeid, J., Quan, X.-W., and Liebmann, B. (2017). Reconciling theories for human and natural attribution of recent East Africa drying. J. Clim. 30, 1939–1957. doi: 10.1175/JCLI-D-16-0558.1
Hoffmann, J., Bauer, P., Sandu, I., Wedi, N., Geenen, T., and Thiemert, D. (2023). Destination earth – A digital twin in support of climate services. Clim. Serv. 30:100394. doi: 10.1016/j.cliser.2023.100394
Huang, X., Zhou, T., Turner, A., Dai, A., Chen, X., Clark, R., et al. (2020). The recent decline and recovery of Indian summer monsoon rainfall: relative roles of external forcing and internal variability. J. Clim. 33, 5035–5060. doi: 10.1175/JCLI-D-19-0833.1
IPCC (2021). “Summary for policymakers” in Climate change 2021: The physical science basis. Contribution of working group I to the sixth assessment report of the intergovernmental panel on climate change. eds. V. Masson-Delmotte, P. Zhai, A. Pirani, S. L. Connors, C. Péan, and S. Berger (Cambridge, UK: Cambridge University Press), 3–32.
Jacob, D., Teichmann, C., Sobolowski, S., Katragkou, E., Anders, I., Belda, M., et al. (2020). Regional climate downscaling over Europe: perspectives from the EURO-CORDEX community. Reg. Environ. Chang. 20:51. doi: 10.1007/s10113-020-01606-9
Jain, S., Mindlin, J., Koren, G., Gulizia, C., Steadman, C., Langendijkr, G. S., et al. (2022). Are we at risk of losing the current generation of climate researchers to data science. AGU Adv. 3:676. doi: 10.1029/2022AV000676
Jain, S., and Scaife, A. A. (2022). How extreme could the near term evolution of the Indian Summer Monsoon rainfall be? Environ. Res. Lett. 17:034009. doi: 10.1088/1748-9326/ac4655
Jain, S., Scaife, A. A., Shepherd, T. G., Deser, C., Dunstone, N., Schmidt, G. A., et al. (2023). Importance of internal variability for climate model assessment. NPJ Clim. Atmos. Sci. 6:68. doi: 10.1038/s41612-023-00389-0
Jakob, C., Gettelman, A., and Pitman, A. (2023). The need to operationalize climate modelling. Nat. Clim. Chang. 13, 1158–1160. doi: 10.1038/s41558-023-01849-4
Jiang, Y., Li, J., Wang, B., Yang, Y., and Zhu, Z. (2023). Weakening of decadal variation of northern hemisphere land monsoon rainfall under global warming. NPJ Clim. Atmos. Sci. 6:115. doi: 10.1038/s41612-023-00441-z
Kang, J., Shaw, T. A., and Sun, L. (2023). Arctic Sea ice loss weakens northern hemisphere summertime storminess but not until the late 21st century. Geophys. Res. Lett. 50:2301. doi: 10.1029/2022GL102301
Kang, S., Yu, Y., Deser, C., Zhang, X., Kang, I.-S., Lee, S.-S., et al. (2023). Global impacts of recent Southern Ocean cooling. Proc. Natl. Acad. Sci. 120:e2300881120. doi: 10.1073/pnas.2300881120
Krinner, G., Kharin, V., Roehrig, R., Scinocca, J., and Codron, F. (2020). Historically-based run-time bias corrections substantially improve model projections of 100 years of future climate change. Commun. Earth Environ. 1:29. doi: 10.1038/s43247-020-00035-0
Krishnan, R., Sabin, T. P., Vellore, R., Mujumdar, M., Sanjay, J., Goswami, B. N., et al. (2016). Deciphering the desiccation trend of the south Asian monsoon hydroclimate in a warming world. Clim. Dyn. 47, 1007–1027. doi: 10.1007/s00382-015-2886-5
Lau, W. K. M., and Kim, K. M. (2017). Competing influences of greenhouse warming and aerosols on Asian summer monsoon circulation and rainfall. Asia-Pac. J. Atmos. Sci. 53, 181–194. doi: 10.1007/s13143-017-0033-4
Lee, S., L’Heureux, M., Wittenberg, A. T., Seager, R., O’Gorman, P. A., and Johnson, N. C. (2022). On the future zonal contrasts of equatorial Pacific climate: perspectives from observations, simulations, and theories. NPJ Clim. Atmos. Sci. 5:82. doi: 10.1038/s41612-022-00301-2
Leinonen, J., Nerini, D., and Berne, A. (2021). Stochastic super-resolution for downscaling time-evolving atmospheric fields with a generative adversarial network. IEEE Trans. Geosci. Remote Sens. 59, 7211–7223. doi: 10.1109/TGRS.2020.3032790
Li, X., Hu, Z. Z., Jiang, X., Li, Y., Gao, Z., Yang, S., et al. (2016). Trend and seasonality of land precipitation in observations and CMIP5 model simulations. Int. J. Climatol. 36, 3781–3793. doi: 10.1002/joc.4592
Lin, Q., Chen, J., Ou, T., Lai, H.-W., Prein, A., and Chen, D. (2023). Performance of the WRF model at the convection-permitting scale in simulating snowfall and Lake-effect snow over the Tibetan plateau. J. Geophys. Res. 128:38433. doi: 10.1029/2022JD038433
Lin, L., Wang, Z., Xu, Y., Fu, Q., and Dong, W. (2018). Larger sensitivity of precipitation extremes to aerosol than greenhouse gas forcing in CMIP5 models. J. Geophys. Res. Atmos. 123, 8062–8073. doi: 10.1029/2018jd028821
Lipzig, N. P. M., Walle, J. V., Belušić, D., Berthou, S., Coppola, E., Demuzere, M., et al. (2023). Representation of precipitation and top-of-atmosphere radiation in a multi-model convection-permitting ensemble for the Lake Victoria Basin (East-Africa). Clim. Dyn. 60, 4033–4054. doi: 10.1007/s00382-022-06541-5
Liu, F., Chai, J., Wang, B., Liu, J., Zhang, X., and Wang, Z. (2016). Global monsoon precipitation responses to large volcanic eruptions. Sci. Rep. 6:24331. doi: 10.1038/srep24331
Lloyd, E. A., and Shepherd, T. G. (2023). Foundations of attribution in climate-change science. Environ. Res. Clim. 2:035014. doi: 10.1088/2752-5295/aceea1
Makula, E. K., and Zhou, B. (2022). Coupled model intercomparison project phase 6 evaluation and projection of east African precipitation. Int. J. Climatol. 42, 2398–2412. doi: 10.1002/joc.7373
Manabe, S., and Weatherald, R. T. (1975). The effects of doubling the CO2 concentration on the climate of a general circulation model. J. Clim. 32, 3–15.
Marchau, V. A. W., Walker, W. E., Bloemen, P. J. T., and Popper, S. W. (2019). Decision making under deep uncertainty. Berlin: Springer, p. 405.
Martinez, C. J., Simpson, I. R., Fasullo, J. T., and Prein, A. F., (2024). An evaluation of the seasonal Caribbean hydroclimate in low and high-resolution CESM and other CMIP6 models.
Maúre, G., Pinto, I., Ndebele-Murisa, M., Muthige, M., Lennard, C., Nikulin, G., et al. (2018). The southern African climate under 1.5 °C and 2 °C of global warming as simulated by CORDEX regional climate models. Environ. Res. Lett. 13:065002. doi: 10.1088/1748-9326/aab190
McDermid, S., Nocco, M., Lawston-Parker, P., Keune, J., Pokhrel, Y., Jain, M., et al. (2023). Irrigation in the earth system. Nat. Rev. Earth Environ. 4, 435–453. doi: 10.1038/s43017-023-00438-5
Miralles, O., Steinfeld, D., Martius, O., and Davison, A. C. (2022). Downscaling of historical wind fields over Switzerland using generative adversarial networks. Artif. Intell. Earth Syst. 1:e220018. doi: 10.1175/AIES-D-22-0018.1
Montini, T. L., Jones, C., and Carvalho, L. M. V. (2019). The south American low-level jet: A new climatology, variability, and changes. J. Geophys. Res. Atmos. 124, 1200–1218. doi: 10.1029/2018JD029634
Narsey, S. Y., Brown, J. R., Colman, R. A., Delage, F., Power, S. B., Moise, A. F., et al. (2020). Climate change projections for the Australian monsoon from CMIP6 models. Geophys. Res. Lett. 47:816. doi: 10.1029/2019GL086816
O’Reilly, C. H., Patterson, M., Robson, J., Monerie, P. A., Hodson, D., and Ruprich-Robert, Y. (2023). Challenges with interpreting the impact of Atlantic multidecadal variability using SST-restoring experiments. NPJ Clim. Atmos Sci. 6:14. doi: 10.1038/s41612-023-00335-0
Palmer, P. I., Wainwright, C. M., Dong, B., Maidment, R. I., Wheeler, K. G., Gedney, N., et al. (2023). Drivers and impacts of eastern African rainfall variability. Nat. Rev. Earth Environ. 4, 254–270. doi: 10.1038/s43017-023-00397-x
Patterson, M. (2023). North-West Europe hottest days are warming twice as fast as mean summer days. Geophys. Res. Lett. 50:757. doi: 10.1029/2023GL102757
Paul, S., Ghosh, S., Oglesby, R., Pathak, A., Chandrasekharan, A., and Ramsankaran, R. A. A. J. (2016). Weakening of Indian summer monsoon rainfall due to changes in land use land cover. Sci. Rep. 6:32177. doi: 10.1038/srep32177
Perkins-Kirkpatrick, S., et al. (2024). New challenges in attributing climate extremes and associated impacts, Frontiers in climate, to be submitted.
Persad, G. G., Samset, B. H., and Wilcox, L. J. (2022). Aerosols must be part of climate risk assessments. Nature 611, 662–664. doi: 10.1038/d41586-022-03763-9
Petrie, R., Denvil, S., Ames, S., Levavasseur, G., Fiore, S., Allen, C., et al. (2021). Coordinating an operational data distribution network for CMIP6 data. Geosci. Model Dev. 14, 629–644. doi: 10.5194/gmd-14-629-2021
Pongratz, J., Schwingshackl, C., Bultan, S., Obermeier, W., Havermann, F., and Guo, S. (2021). Land use effects on climate: current state, recent Progress, and emerging topics. Curr. Clim. Chang. Rep. 7, 99–120. doi: 10.1007/s40641-021-00178-y
Purich, A., and Doddridge, E. W. (2023). Record low Antarctic Sea ice coverage indicates a new sea ice state. Commun. Earth Environ. 4:314. doi: 10.1038/s43247-023-00961-9
Rasp, S., Pritchard, M. S., and Gentine, P. (2018). Deep learning to represent subgrid processes in climate models. Proc. Natl. Acad. Sci. 115, 9684–9689. doi: 10.1073/pnas.1810286115
Roach, L. A., Mankoff, K. D., Romanou, A., Blanchard-Wrigglesworth, E., Haine, T. W. H., and Schmidt, G. A. (2023). Winds and meltwater together Lead to Southern Ocean surface cooling and sea ice expansion. Geophys. Res. Lett. 50:948. doi: 10.1029/2023GL105948
Rodrigues, R. R., and Shepherd, T. G. (2022). Small is beautiful: climate-change science as if people mattered. PNAS Nexus 1:pgac009. doi: 10.1093/pnasnexus/pgac009
Rowell, D. P., Booth, B. B. B., Nicholson, S. E., and Good, P. (2015). Reconciling past and future rainfall trends over East Africa. J. Clim. 28, 9768–9788. doi: 10.1175/JCLI-D-15-0140.1
Roxy, M. K., Ghosh, S., Pathak, A., Athulya, R., Mujumdar, M., Murtugudde, R., et al. (2017). A threefold rise in widespread extreme rain events over Central India. Nat. Commun. 8:708. doi: 10.1038/s41467-017-00744-9
Roxy, M. K., Ritika, K., Terray, P., Murtugudde, R., Ashok, K., and Goswami, B. N. (2015). Drying of Indian subcontinent by rapid Indian Ocean warming and a weakening land-sea thermal gradient. Nat. Commun. 6:7423. doi: 10.1038/ncomms8423
Rudin, C. (2019). Stop explaining black box machine learning models for high stakes decisions and use interpretable models instead. Nat. Mach. Intell. 1, 206–215. doi: 10.1038/s42256-019-0048-x
Rugenstein, M., Dhame, S., Olonscheck, D., Wills, R. J., Watanabe, M., and Seager, R. (2023). Connecting the SST pattern problem and the hot model problem. Geophys. Res. Lett. 50:e2023GL105488. doi: 10.1029/2023GL105488
Saha, A, Ghosh, S, Sahana, A S, and Rao, E P. (2014). Failure of CMIP5 climate models in simulating post-1950 decreasing trend of Indian monsoon Geophys. Res. Lett. 41 7323–30
Sanjay, J., Krishnan, R., Shrethra, A. B., Rajbhandari, R., and Ren, G.-Y. (2017). Downscaled climate change projections for the Hindu Kush Himalayan region using CORDEX South Asia regional climate models. Adv. Clim. Chang. Res. 8, 185–198. doi: 10.1016/j.accre.2017.08.003
Santer, B. D., Wigley, T. M. L., Barnett, T. P., and Anyamba, E. (1996). “Detection of climate change and attribution of causes” in Climate change 1995: The science of climate change, contribution of working group I to the second assessment report of the intergovernmental panel on climate change. eds. J. T. Houghton, L. G. Meira Filho, B. A. Callander, N. Harris, A. Kattenberg, and K. Maskell (Cambridge, UK: Cambridge University Press)
Saurral, R. I., Camilloni, I. A., and Barros, V. R. (2017). Low-frequency variability and trends in centennial precipitation stations in southern South America. Int. J. Climatol. 37, 1774–1793. doi: 10.1002/joc.4810
Scaife, A. A., and Smith, D. A. (2018). Signal-to-noise paradox in climate science. NPJ Clim. Atmos. Sci. 1:28. doi: 10.1038/s41612-018-0038-4
Schemm, S. (2023). Toward eliminating the decades-old “too zonal and too equatorward” storm-track Bias in climate models. J. Adv. Earth Syst. Model. 15:e2022MS003482. doi: 10.1029/2022MS003482
Schipper, E. F. L., Ensor, J., Mukherji, A., Mirzabaev, A., Fraser, A., Harveyb, B., et al. (2021). Equity in climate scholarship: a manifesto for action. Clim. Dev. 13, 853–856. doi: 10.1080/17565529.2021.1923308
Schmidt, G. (2013). On mismatches between models and observations. Available at: https://www.realclimate.org/index.php/archives/2013/09/on-mismatches-between-models-and-observations/.
Seager, R., Henderson, N., and Cane, M. (2022). Persistent discrepancies between observed and modeled trends in the tropical Pacific Ocean. J. Clim. 35, 4571–4584. doi: 10.1175/JCLI-D-21-0648.1
Sekiyama, T. T., Hayashi, S., Kaneko, R., and Fukui, K.-I. (2023). Surrogate downscaling of mesoscale wind fields using ensemble Superresolution convolutional neural networks. Artif. Intell. Earth Syst. 2:7. doi: 10.1175/AIES-D-23-0007.1
Shaw, T. A., Miyawaki, O., and Donohoe, A. (2022). Stormier southern hemisphere induced by topography and the ocean circulation. Proc. Natl. Acad. Sci. 119:e2123512119. doi: 10.1073/pnas.2123512119
Shaw, T. A. (2019). Mechanisms of Future Predicted Changes in the Zonal Mean Mid-Latitude Circulation. Curr Clim Change Rep 5, 345–357. doi: 10.1007/s40641-019-00145-8
Shaw, T., Baldwin, M., Barnes, E., et al. (2016). Storm track processes and the opposing influences of climate change. Nature Geosci 9, 656–664. doi: 10.1038/ngeo2783
Silva, S. J., Keller, C. A., and Hardin, J. (2022). Using an explainable machine learning approach to characterize earth system model errors: Application of SHAP analysis to modeling lightning flash occurrence. J. Adv. Earth Syst. Mod. 14:2881. doi: 10.1029/2021MS002881
Simpson, I., McKinnon, K., Kennedy, D., and Seager, R. (2023). Observed humidity trends in dry regions contradict climate models. Proc. Natl. Acad. Sci. 2023:12. doi: 10.1073/pnas.230248012
Singh, D., Ghosh, S., Roxy, M. K., and McDermid, S. (2019). Indian summer monsoon: extreme events, historical changes, and role of anthropogenic forcings. WIREs. Climate Change 10:571. doi: 10.1002/wcc.571
Singh, M., Krishnan, R., Goswami, B., Choudhury, A. D., Swapna, P., Vellore, R., et al. (2020). Fingerprint of volcanic forcing on the ENSO–Indian monsoon coupling. Science. Advances 6:eaba8164. doi: 10.1126/sciadv.aba8164
Smith, D. M., Scaife, A. A., Eade, R., Athanasiadis, P., Bellucci, A., Bethke, I., et al. (2020). North Atlantic climate far more predictable than models imply. Nature 583, 796–800. doi: 10.1038/s41586-020-2525-0
Stouffer, R., Manabe, S., and Bryan, K. (1989). Interhemispheric asymmetry in climate response to a gradual increase of atmospheric CO2. Nature 342, 660–662. doi: 10.1038/342660a0
Swain, D. (2023). Climate researchers need support to become scientist-communicators. Nature 624:9. doi: 10.1038/d41586-023-03436-1
Tebaldi, C., Wehner, M., Leung, L. R., and Lawrence, D. (2023). Is land use producing robust signals in future projections from earth system models, all else being equal? Environ. Res. Lett. 18:9326. doi: 10.1088/1748-9326/ace3da
Teng, H., Leung, R., Branstator, G., Lu, J., and Ding, Q. (2022). Warming pattern over the northern hemisphere midlatitudes in boreal summer 1979-2020. J. Clim. 35, 3479–3494. doi: 10.1175/JCLI-D-21-0437.1
Undorf, S., Polson, D., Bollasina, M. A., Ming, Y., Schurer, A., and Hegerl, G. C. (2018). Detectable impact of local and remote anthropogenic aerosols on the 20th century changes of West African and south Asian monsoon precipitation. Geophys. Res. Lett. 123, 4871–4889. doi: 10.1029/2017JD027711
Van de Walle, J., Thiery, W., Brousse, O., Souverijns, N., Demuzere, M., and van Lipzig, N. P. M. (2020). A convection-permitting model for the Lake Victoria Basin: evaluation and insight into the mesoscale versus synoptic atmospheric dynamics. Clim. Dyn. 54, 1779–1799. doi: 10.1007/s00382-019-05088-2
Van Oldenburg, G. J., Wehner, M. F., Vautard, R., Otto, F. E. L., Seneviratne, S. I., Stott, P. A., et al. (2022). Attributing and projecting heatwaves is hard: we can do better. Earth’s Future 10:271. doi: 10.1029/2021EF002271
Varuolo-Clarke, A. M., Smerdon, J. E., Williams, A. P., and Seager, R. (2021). Gross discrepancies between observed and simulated twentieth-to-twenty-first-century precipitation trends in southeastern South America. J. Clim. 34, 6441–6457. doi: 10.1175/JCLI-D-20-0746.1
Vautard, R., Cattiaux, J., Happé, T., Singh, J., Bonnet, R., Cassou, C., et al. (2023). Heat extremes in Western Europe increasing faster than simulated due to atmospheric circulation trends. Nat. Commun. 14:6803. doi: 10.1038/s41467-023-42143-3
Vera, C. S., and Díaz, L. (2015). Anthropogenic influence on summer precipitation trends over South America in CMIP5 models. Int. J. Climatol. 35, 3172–3177. doi: 10.1002/joc.4153
Wainwright, C. M., Marsham, J. H., Keane, R. J., Rowell, D. P., Finney, D. L., Black, E., et al. (2019). ‘Eastern African paradox’ rainfall decline due to shorter not less intense long rains. NPJ Clim. Atmos. Sci. 2:34. doi: 10.1038/s41612-019-0091-7
Wang, B., Biasutti, M., Byrne, M. P., Castro, C., Chang, C.-P., Cook, K., et al. (2021). Monsoons climate change assessment. Bull. Am. Met. Soc. 102, E1–E19. doi: 10.1175/BAMS-D-19-0335.1
Wang, S. S.-C., Qian, Y., Leung, R. L., and Zhang, Y. (2022). Interpreting machine learning prediction of fire emissions and comparison with FireMIP process-based models. Atmos. Chem. Phys. 22, 3445–3468. doi: 10.5194/acp-22-3445-2022
Wang, P., Yuval, J., and O’Gorman, P. A. (2022). Non-local parameterization of atmospheric subgrid processes with neural networks. J. Adv. Modell. Earth Syst. 14:2984. doi: 10.1029/2022MS002984
Wehrli, K., Luo, F., Hauser, M., Shiogama, H., Tokuda, D., Kim, H., et al. (2022). The ExtremeX global climate model experiment: investigating thermodynamic and dynamic processes contributing to weather and climate extremes. Earth Syst. Dynam. 13, 1167–1196. doi: 10.5194/esd-13-1167-2022
White, R. H., Anderson, S., Booth, J. F., Braich, G., Draeger, C., Fei, C., et al. (2023). The unprecedented Pacific northwest heatwave of June 2021. Nat. Commun. 14:727. doi: 10.1038/s41467-023-36289-3
Wills, R. C. J., Dong, Y., Proistosecu, C., Armour, K., and Battisti, D. S. (2022). Systematic climate model biases in the large-scale patterns of recent sea-surface temperature and sea-level pressure change. Geophys. Res. Lett. 49:e2022GL100011. doi: 10.1029/2022GL100011
Woollings, T., Drouard, M., O’Reilly, C. H., Sexton, D. M. H., and McSweeney, C. (2023). Trends in the atmospheric jet streams are emerging in observations and could be linked to tropical warming. Commun. Earth Environ. 4:125. doi: 10.1038/s43247-023-00792-8
Wu, Y., and Polvani, L. M. (2017). Recent trends in extreme precipitation and temperature over southeastern South America: the dominant role of stratospheric ozone depletion in the CESM large ensemble. J. Clim. 30, 6433–6441. doi: 10.1175/jcli-d-17-0124.1
Yang, W., Seager, R., Cane, M., and Bradfield, L. (2014). The annual cycle of east African precipitation. J. Clim. 28:6. doi: 10.1175/JCLI-D-14-00484.1
Yeager, S. G., Chang, P., Danabasoglu, G., Rosenbloom, N., Zhang, Q., Castruccio, F. S., et al. (2023). Reduced Southern Ocean warming enhances global skill and signal-to-noise in an eddy-resolving decadal prediction system. NPJ Clim. Atmos. Sci. 6:107. doi: 10.1038/s41612-023-00434-y
Yuval, J., and O’Gorman, P. A. (2023). Neural-network parameterization of subgrid momentum transport in the atmosphere. J. Adv. Earth Syst. Model. 15:606. doi: 10.1029/2023MS003606
Keywords: regional climate change, climate dynamics, climate modeling, climate communication, climate prediction and projection
Citation: Shaw TA, Arias PA, Collins M, Coumou D, Diedhiou A, Garfinkel CI, Jain S, Roxy MK, Kretschmer M, Leung LR, Narsey S, Martius O, Seager R, Shepherd TG, Sörensson AA, Stephenson T, Taylor M and Wang L (2024) Regional climate change: consensus, discrepancies, and ways forward. Front. Clim. 6:1391634. doi: 10.3389/fclim.2024.1391634
Edited by:
Fernando Manuel Raposo Morgado, University of Aveiro, PortugalReviewed by:
Guillaume Gastineau, Sorbonne Université, FranceTaketoshi Kodama, Japan Fisheries Research and Education Agency (FRA), Japan
Copyright © 2024 Shaw, Arias, Collins, Coumou, Diedhiou, Garfinkel, Jain, Roxy, Kretschmer, Leung, Narsey, Martius, Seager, Shepherd, Sörensson, Stephenson, Taylor and Wang. This is an open-access article distributed under the terms of the Creative Commons Attribution License (CC BY). The use, distribution or reproduction in other forums is permitted, provided the original author(s) and the copyright owner(s) are credited and that the original publication in this journal is cited, in accordance with accepted academic practice. No use, distribution or reproduction is permitted which does not comply with these terms.
*Correspondence: Tiffany A. Shaw, dGFzMUB1Y2hpY2Fnby5lZHU=