- 1Fenner School of Environment and Society, The Australian National University, Canberra, ACT, Australia
- 2ARC Centre of Excellence for Climate Extremes, The Australian National University, Canberra, ACT, Australia
- 3Climate Change Research Centre, University of New South Wales, Sydney, NSW, Australia
- 4ARC Centre of Excellence for Climate Extremes, University of New South Wales, Sydney, NSW, Australia
- 5School of Geography, Earth and Atmospheric Sciences, University of Melbourne, Melbourne, VIC, Australia
- 6ARC Centre of Excellence for 21st Century Weather, University of Melbourne, Melbourne, VIC, Australia
- 7Royal Netherlands Meteorological Institute (KNMI), De Bilt, Netherlands
- 8Grantham Institute for Climate Change and the Environment, Imperial College London, London, United Kingdom
- 9Wegener Center for Climate and Global Change, University of Graz, Graz, Austria
- 10Oxford Sustainable Law Programme, Smith School of Enterprise and the Environment, University of Oxford, Oxford, United Kingdom
- 11Oxford Sustainable Law Programme, University of Oxford, Oxford, United Kingdom
- 12École Nationale des Ponts et Chaussées, Champs sur Marne, France
- 13Laboratoire de Météorologie Dynamique/IPSL, ENS, PSL Research University, École Polytechnique, Institut Polytechnique de Paris, Sorbonne Université, CNRS, Paris, France
- 14Department of Compound Environmental Risks, Helmholtz Centre for Environmental Research—UFZ, Leipzig, Germany
- 15Copernicus Climate Change Service, European Centre for Medium-Range Weather Forecasts, Bonn, Germany
- 16Institute for Atmospheric and Climate Science, ETH Zürich, Zürich, Switzerland
- 17School of Geosciences, University of Edinburgh, Edinburgh, United Kingdom
- 18Kenya Meteorological Department, Nairobi, Kenya
- 19Copernicus Institute of Sustainable Development, Utrecht University, Utrecht, Netherlands
- 20African Centre of Meteorological Applications for Development (ACMAD), Niamey, Niger
- 21African Climate and Development Initiative (ACDI), University of Cape Town, Cape Town, South Africa
- 22African Aviation and Aerospace University, Abuja, Nigeria
- 23Division of Environmental Science and Engineering, Pohang University of Science and Technology, Pohang, Republic of Korea
- 24Institute for Convergence Research and Education in Advanced Technology, Yonsei University, Seoul, Republic of Korea
- 25African Climate and Development Initiative, University of Cape Town, Cape Town, South Africa
- 26Department of Environmental and Geographical Science, University of Cape Town, Cape Town, South Africa
- 27Cabot Institute for the Environment, University of Bristol, Bristol, United Kingdom
- 28Department of Geological and Atmospheric Sciences, Iowa State University, Ames, IA, United States
- 29Centre National de Recherches Météorologiques, Université de Toulouse, Météo-France, CNRS, Toulouse, France
- 30Department of the Geophysical Sciences, The University of Chicago, Chicago, IL, United States
- 31Department of Water and Climate, Vrije Universiteit Brussel, Brussels, Belgium
- 32Australian Bureau of Meteorology, Melbourne, VIC, Australia
- 33Institut Pierre-Simon Laplace, CNRS, Université Paris-Saclay, Sorbonne Université, Paris, France
- 34Lawrence Berkeley National Laboratory, Berkeley, CA, United States
- 35Department of Hydro Sciences, TUD Dresden University of Technology, Dresden, Germany
The field of extreme event attribution (EEA) has rapidly developed over the last two decades. Various methods have been developed and implemented, physical modelling capabilities have generally improved, the field of impact attribution has emerged, and assessments serve as a popular communication tool for conveying how climate change is influencing weather and climate events in the lived experience. However, a number of non-trivial challenges still remain that must be addressed by the community to secure further advancement of the field whilst ensuring scientific rigour and the appropriate use of attribution findings by stakeholders and associated applications. As part of a concept series commissioned by the World Climate Research Programme, this article discusses contemporary developments and challenges over six key domains relevant to EEA, and provides recommendations of where focus in the EEA field should be concentrated over the coming decade. These six domains are: (1) observations in the context of EEA; (2) extreme event definitions; (3) statistical methods; (4) physical modelling methods; (5) impact attribution; and (6) communication. Broadly, recommendations call for increased EEA assessments and capacity building, particularly for more vulnerable regions; contemporary guidelines for assessing the suitability of physical climate models; establishing best-practice methodologies for EEA on compound and record-shattering extremes; co-ordinated interdisciplinary engagement to develop scaffolding for impact attribution assessments and their suitability for use in broader applications; and increased and ongoing investment in EEA communication. To address these recommendations requires significant developments in multiple fields that either underpin (e.g., observations and monitoring; climate modelling) or are closely related to (e.g., compound and record-shattering events; climate impacts) EEA, as well as working consistently with experts outside of attribution and climate science more generally. However, if approached with investment, dedication, and coordination, tackling these challenges over the next decade will ensure robust EEA analysis, with tangible benefits to the broader global community.
1 Introduction
Extreme event attribution (EEA) seeks to determine whether there is a discernible effect on an observed extreme weather event’s characteristics due to a particular influence on the climate. In most EEA studies, the focus is on isolating anthropogenic influence. By this definition, EEA is an important tool in assessing how large-scale climate change interacts with events that will be most impactful—weather-scale extremes. EEA has rapidly expanded since initial formative studies by Allen (2003) and Stott et al. (2004) two decades ago. Part of this expansion was reviewed by the US National Academies of Sciences, Engineering, and Medicine (NASEM) (2016), introducing multiple methods underpinned by physical climate model simulations and/or sophisticated statistical analyses. More recently, the field of impact attribution has emerged, whereby various impacts of extreme events (e.g., on human health, agricultural yield, infrastructural damage, or financial losses) are also attributed to climate change (e.g., Vicedo-Cabrera et al., 2023). Moreover, the results of EEA are regularly and widely communicated to general audiences by many types of media, demonstrating the importance of these studies in shaping the conversation on how climate change is influencing the world we experience. For example, World Weather Attribution1 provides a rapid attribution analysis soon after the occurrence of an impactful extreme event, which is disseminated to, and often reported by, various media sources, particularly in the region where the extreme event occurred.
Whilst these developments are a testament to the power and reach of EEA and highlight scientists’ keen interest in advancing the field towards practical and operational applications, there remain challenges that should be addressed. Moreover, new challenges emerge as the likelihood of compound and record-shattering events increase (Zscheischler et al., 2018; Fischer et al., 2021), and new types of compound events emerge (Matthews et al., 2019). Importantly, these challenges are interdisciplinary and cannot be resolved by the climate community working in isolation. Ongoing and co-ordinated interactions between climate scientists and statisticians, impact modellers, lawyers, the financial sector, communication experts, and other stakeholders are important so that substantial constructive developments in EEA take place over the next decade.
This article addresses six key areas where principal developments in this field are required, chosen due to either their central role in the methodological execution of EEA assessments or the application of EEA outside of climate science:
1. The role and current limitations of observations used in EEA analysis;
2. How events are defined for EEA and why this is important for the interpretation of corresponding results;
3. Statistical methods commonly employed for undertaking EEA assessments, including developments for analysing compound and record-shattering events;
4. The use of physical climate models for EEA;
5. The emergence of impact attribution, and
6. Communication of the results of EEA to non-scientist audiences.
We discuss important developments in each of these areas, as well as current and future constraints that may hinder the development and usability of EEA if not properly addressed. We conclude this concept paper by suggesting focus topics to overcome these highlighted issues, such that EEA is propelled further towards robust assessments and the most relevant applications over the next decade. To help readers distil the considered topics and recommendations of this article, a schematic overview is provided in Figure 1.
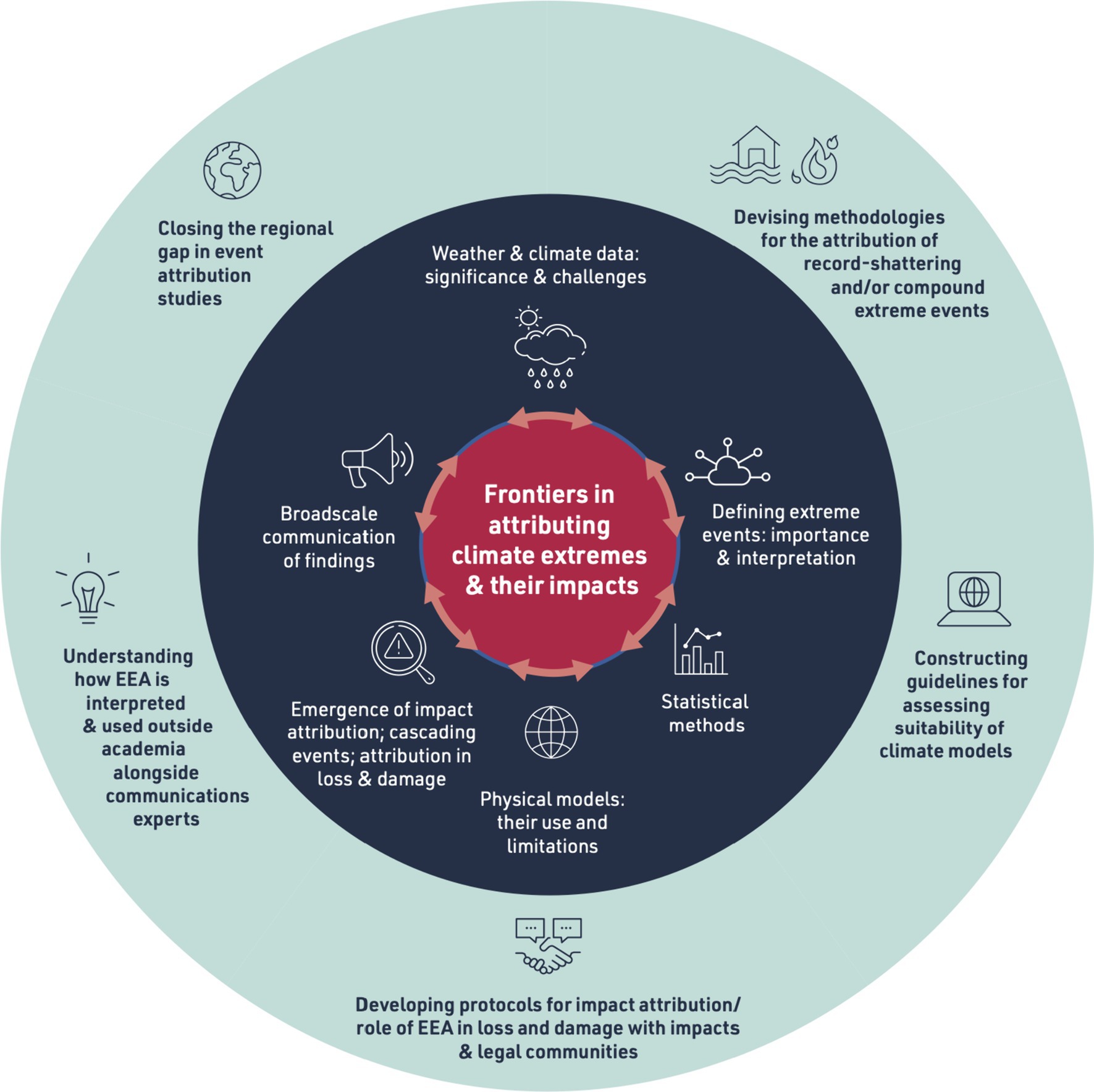
Figure 1. Schematic overview of the challenges and opportunities in extreme event attribution (EEA) this article addresses. The middle circle contains the six topics discussed in detail in the following sections, whereas the outer circle contains the recommendations listed in Section 8. Note that while the six topics of focus are separated for discussion in the following sections, they are ultimately interrelated and dependent on the current knowledge and limitations of one another, as highlighted by the circular arrows on the border of the inner circle.
2 Observational data for EEA
Trustworthy observations are critical for weather and climate extremes research and event attribution. However, high-quality data are often unavailable, inaccessible, and not long or dense enough to quantify and contextualise many extreme weather events (Doblas-Reyes et al., 2021). Here, we discuss some of the challenges in using observational data and approaches to make the most of these datasets for EEA studies.
The quality and length of observational data vary worldwide and are dependent on the climate/weather variable of interest. Weather station records remain the most trustworthy source of observational data, particularly if quality-controlled and/or homogenised. Long-running, quality-controlled station data or gridded data based on station data enable a more robust contextualisation of an extreme event in the historical record, both for the event itself, and for contributing factors such as regional warming and changes in the water cycle. However, weather station data are affected by various biases and uncertainties, particularly for extremes, due to human factors such as erroneous rainfall measurements (e.g., Viney and Bates, 2004) and other external factors (Trewin, 2010) such as site moves, changes in observing practices, and characteristics of instrument design (e.g., Harrison, 2010). For gridded data, the interpolation process also introduces uncertainties, particularly where the density of the underlying network is sparse or has changed over time (King et al., 2013).
There is a sparsity of current and historical station data in various parts of the world, particularly in many areas with high vulnerability to extreme events (Slivinski et al., 2019; Kimutai et al., 2023b). This may be because the observations were never made or were discontinuous, the observation network is not dense enough to resolve localised extreme events, or observations have not been digitised (Brunet and Jones, 2011). Substantial quantities of data measured at national levels are difficult to obtain, as they come from networks which do not report internationally. Historical data are also often not freely available for data policy reasons, although once implemented, the WMO’s Unified Data Policy (2022) should assist here. Finally, the nature of some extremes is such that the impact of the extreme itself may lead to the observation being lost (e.g., an observing site being inundated by flooding). Lack of accessible measurement data complicates quantifying the weather extreme (e.g., intense precipitation), but also the impact assessment (e.g., flooded area or damages; see Section 6). A further limitation for attribution studies of recent events is that recent station data are generally drawn from real-time synoptic data distributed through the WMO Global Telecommunication System (GTS). These encompass only a subset of all potentially available stations, have limited quality control, and sometimes inconsistent definitions (e.g., precipitation totals in a GTS report are sometimes only for part of a day, not a full 24 h). The dependence of recent data on GTS reports, and in particular the near-total lack of non-GTS precipitation data from many parts of the world, is illustrated in Figure 2.
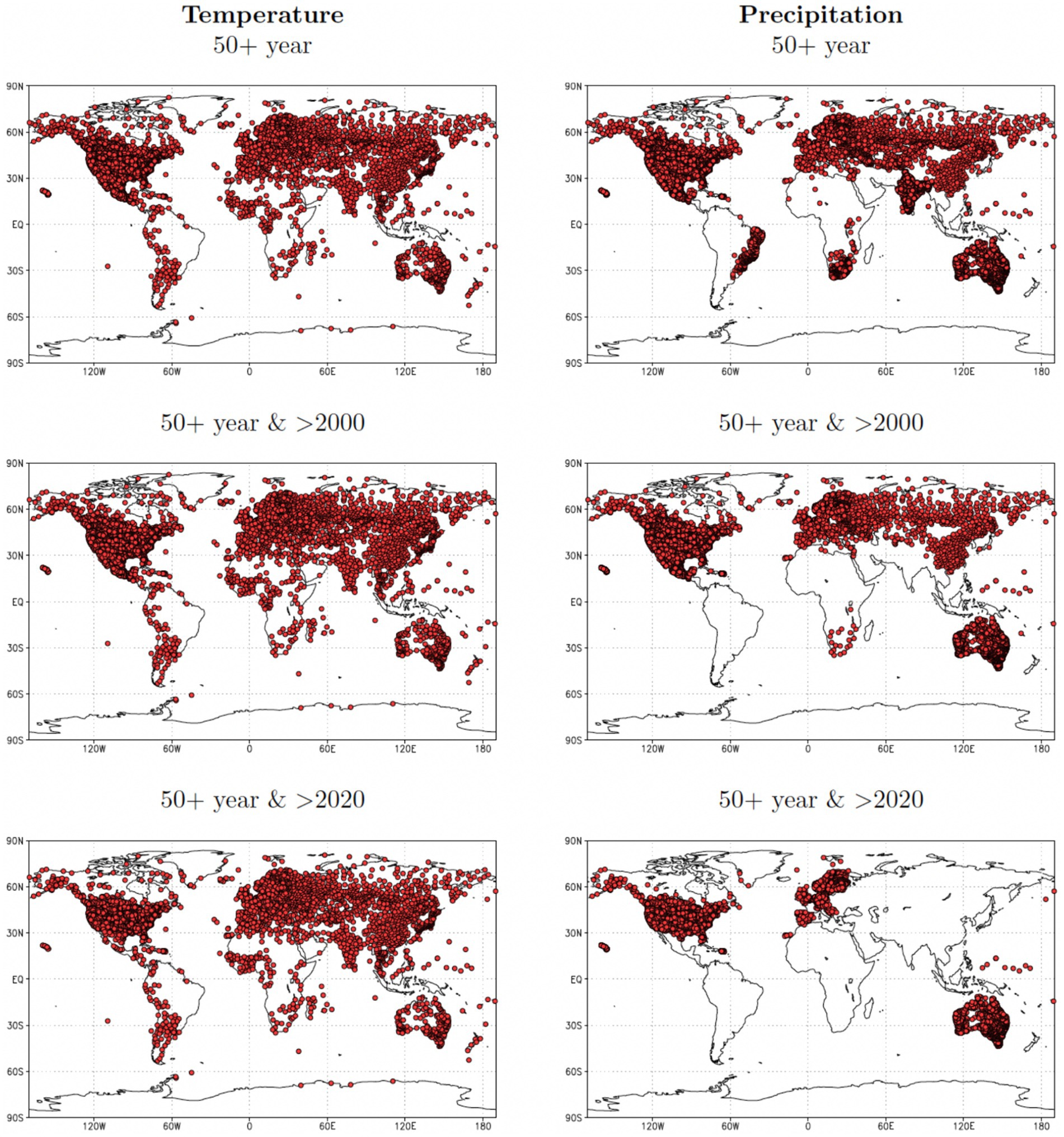
Figure 2. Observational stations from the Global Historical Climate Network daily (GHCNd) network where 50 or more years of data are available (top) and data ending after 2000 (centre) and 2020 (bottom) for temperature (left column) and precipitation data (right column; excluding GTS precipitation data). White land areas show the data-sparse regions. The figure does not take into account that there may be a significant amount of missing data within the 50-year time slot. Since precipitation data from the GTS are excluded, there are almost no stations with recent precipitation data outside Europe, Australia, Canada, and the United States and its territories.
While satellite data have helped fill gaps, uncertainties, and biases are best understood where there are long-term observations for comparison. Even in well-observed regions of the world, there are significant biases in satellite representation of especially precipitation extremes and associated climate processes (Hegerl et al., 2015; Hosseini-Moghari and Tang, 2022). Different products can exhibit large differences in precipitation extremes in data-sparse regions (Alexander et al., 2020; Bador et al., 2020), as was demonstrated with an attempted attribution analysis of flooding rains in parts of the Democratic Republic of Congo and Rwanda in 2023 (Figure 3), resulting in an inability to verify a gridded product suitable for the robust attribution of this event (Kimutai et al., 2023b). Africa, in particular, has very limited in situ observations and large uncertainties across products (Ayugi et al., 2024) to perform event attribution. A possible alternative is the use of reanalyses that are improving in quality for analysis of extremes (Donat et al., 2014; Dunn et al., 2022) and can shed light on historical extremes (Cowan et al., 2020), but for some variables, including precipitation, and/or in data-sparse regions, the spread across products remains large, inhibiting accurate event attribution (Alexander et al., 2020; Sun et al., 2018). Therefore, in places where in situ observations may be sparse and have other uncertainties, although reanalyses may be the best available option, they must be considered with caution (Kimutai et al., 2023b).
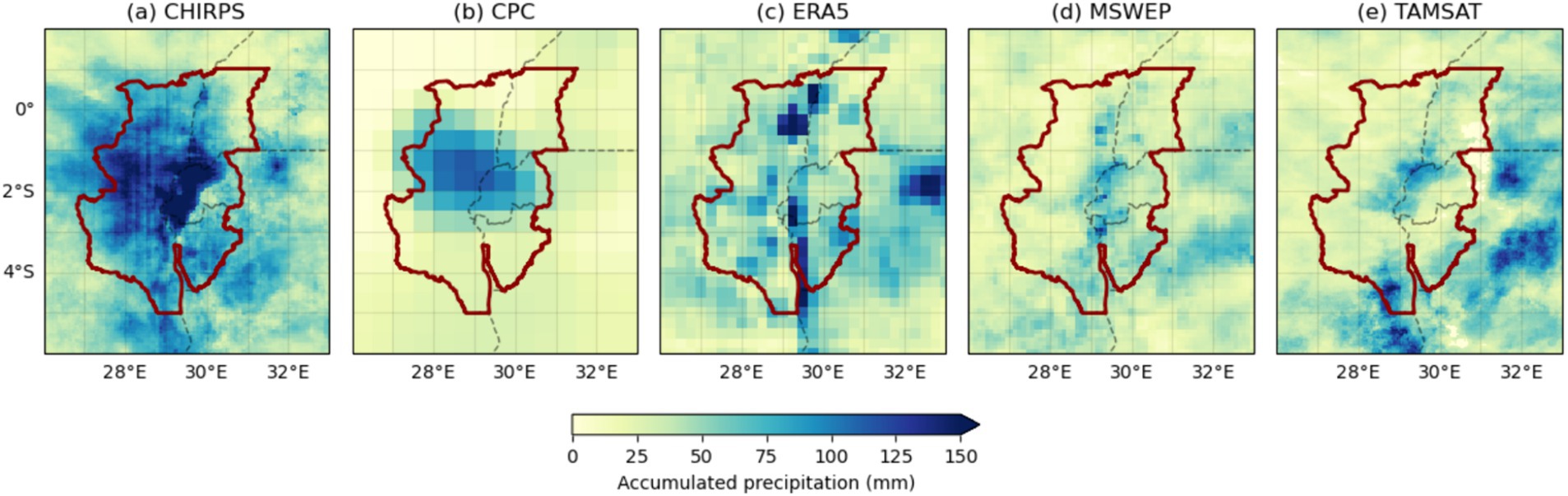
Figure 3. Five-day accumulated precipitation from 1 May to 5 May 2023 over the region surrounding Lake Kivu, in gridded data products using different sources of data: (a) CHIRPS (satellite and in situ), (b) CPC (analysis of in situ observations), (c) ERA5 (reanalysis), (d) MSWEP (reanalysis, satellite and in situ), and (e) TAMSAT (satellite and in situ). The red border indicates the larger region around Lake Kivu for which RX5day was analysed in Kimutai et al. (2023b); the figure is adapted from that paper’s Figure. The lack of sufficient regional measurement data complicates the selection of the most representative in situ, satellite, or reanalysis dataset for this specific case, and to establish why, for example, that CHIRPS is much wetter than the other products.
Many observational products do not include uncertainty estimates, which could hamper the examination of uncertainties in rapid analyses. However, utilising different observational products and reanalysis ensembles in the event definition (see Section 3) and model evaluation steps of EEA would be beneficial (Angélil et al., 2016). Variation due to choice of observational product and the subsequent model evaluation results should be incorporated in uncertainty statements on the overall attribution of extreme events to anthropogenic influences. This would be useful since the uncertainty associated with a lack of observations is greater in data-sparse regions, leading to wider confidence intervals in attribution statements.
Long and reasonably homogeneous records (such as temperature extremes after the introduction of Stevenson screens) contain past extreme events that improve sampling and allow examination of the distribution of extreme events (van Oldenborgh et al., 2021b). Regional historical extreme events can provide input to a storyline approach (see Section 5), from characteristics of the weather situation to its impact (Yule et al., 2023). Analogue methods, where events under common weather patterns may be identified and compared between these different climates (Cattiaux et al., 2010; Vautard et al., 2016; Faranda et al., 2022), allow for investigation of such events in present-day or future conditions, but this is challenging due to uncertainty from methodological choices (Harrington et al., 2019) and in the application to record-shattering events (see Section 5). Palaeoclimatic records provide additional context, with some past extreme drought events substantially stronger than recently observed ones, indicating the presence of exceptional climate conditions in the past (Cook et al., 2022). While generally, paleoclimate records do not have a high enough time and scale resolution for EEA, historical events captured in them provide context for modelling and understanding rare events in the present. In summary, long, reliable observational records support event attribution by improving sampling, allowing for better model evaluation, and supporting the development of storylines and analogues for impacts.
Ultimately, EEA will be best supported by regular observations with dense spatial coverage over all parts of the world, available in near real-time, and supported by high-quality historical data. WMO’s Global Basic Observing Network (GBON) and Systematic Observations Financing Facility (SOFF) aim to strongly enhance the real-time component. Quality reanalyses and remote sensing datasets will further strengthen this, as would a mechanism for more effective exchange of precipitation data. Technological developments, including advances in remote sensing capabilities and low-cost sensors, can benefit the most vulnerable communities. In addition, stricter requirements by research funding agencies on open data sharing have improved the overall availability of observed climate data for EEA, and the adoption of WMO’s Unified Data Policy is a further step in this direction, particularly for historical data. Data rescue initiatives are also important for the recovery of historical data. The continuation and development of these initiatives will pave the way for a larger and more spatially consistent global network of high observational quality that is essential for EEA.
3 Definition of events for EEA assessments
Quantitatively defining “an event” for attribution involves selecting a relevant weather phenomenon of some significance (e.g., with known impacts, and/or at or near a climatological record), and identifying the time scale and region over which it will be examined. This identification is important for detecting the event, both in the observational record and, depending on how attribution is carried out, using climate model simulations (Section 5). Great care must be taken to consider the duration, intensity, and spatial extent of the specific extreme event, whether necessary variables can reliably be obtained from observations (Section 2), and, in many cases, how the event relates to impacts. This process also requires consideration of possible compounding effects behind impacts (see Section 4.3; Bevacqua et al., 2020; Zscheischler et al., 2020). The definition of the event is a crucial element within event attribution procedures (Otto et al., 2016; Jézéquel et al., 2018; Leach et al., 2020) and is often the most time-intensive step; however, there is no single objective approach. Here we discuss various possibilities, along with challenges and recommendations.
In a broad sense, there are three approaches to event definition (van Oldenborgh et al., 2021b). These include a definition that will:
i. more clearly constrain any anthropogenic signal by averaging out variability over space and/or time. Opting for a large spatial scale can facilitate the detection of a trend and is a reason why large-scale precipitation events are more often attributable than small-scale convective precipitation storms (Kirchmeier-Young et al., 2019);
ii. as closely as possible focus on the observed event of interest by looking at the spatiotemporal window over which it has been particularly extreme. This can be done by maximising the return period of the event over all possible space-time domains (Cattiaux and Ribes, 2018);
iii. focus on the aspect of the extreme weather event that contributed most towards impacts on society and ecosystems. For example, local extreme temperatures during heatwaves are demonstrated to increase human mortality (Vicedo-Cabrera et al., 2023).
A simple example of approach (i) could be summer season temperatures averaged over Western Europe. For approach (ii) this could, for instance, be the annual peak 24-h precipitation extreme at the single station location exhibiting the highest return period for the region. Annual maximum wet-bulb temperature over a large city in India suffering from humid heat would illustrate the criteria of approach (iii). In some cases, these approaches are not mutually exclusive and the chosen definition may touch on aspects of each (see, e.g., Tradowsky et al., 2023). It is vital to communicate the variables and temporal and spatial definitions chosen (Philip et al., 2020), the rationale of the choice, and to make sure results are interpreted well and can be used as guidance (see Section 6). Both a storyline framing and a probabilistic framing can be applied to provide an answer to attribution questions (see Section 4; García-Portela and Maraun, 2023), and while the former tends to be the more common approach in attribution, both framings can complement each other.
It is important to make clear that the outcome of EEA depends strongly on the event definition and framing (see, e.g., Otto et al., 2012, 2016; van Oldenborgh et al., 2021b), and thus event definition is exceptionally non-trivial. However, this is not to say that attribution results are entirely subjective. As with any scientific analysis, EEA studies need to be precise about what is being assessed, and the event definition must be fit for purpose. It is therefore imperative to be clear about how and why the event was defined, and any potential influence these decisions might have on associated attribution results. Where an attribution analysis is motivated by providing information that is socially relevant, we advise that event definitions be selected to best capture corresponding and exacerbated impact(s) on society, whilst also quantifying the extreme meteorological nature of the event, temporal persistence, spatial domain affected, and where possible, the corresponding driving mechanisms. Indeed, conducting such analyses requires an understanding of the nature of the relationship between the physical hazard (temperature, precipitation, sea levels) and the resulting impact(s), which is not straightforward and requires a multidisciplinary approach (see Section 6).
When seeking to base an event definition on impacts, local knowledge is key. Wherever possible, local meteorological, climatological, and/or impact experts who can provide quantitative and lived experience information on the magnitude and scale of the event of interest should be part of the study (e.g., Philip et al., 2020; Kimutai et al., 2023a; Zachariah et al., 2023b; Junior et al., 2024). Their knowledge is essential in the initial discussions on event definition, to relay what the perceived impacts are, and to converge on an impact-relevant event definition. Currently, there are limitations to how close to impacts a definition can be. Often a compromise is made between the impact of interest and what measures can be robustly estimated from both observational and model climatological data, e.g., a Fire Weather Index (FWI) rather than fire extent (van Oldenborgh et al., 2021a) (see Section 5). In a rapid attribution study, metrics which are readily available or quickly computed are preferred (e.g., Philip et al., 2020; Zachariah et al., 2023b).
Disasters are often related to “compounding” factors such as multivariate, concurrent, preconditioned, or successive extremes in several variables or in several locations (Zscheischler et al., 2020). The definition of compound events for attribution typically involves a trade-off between the different relevant spatiotemporal scales of the contributing atmospheric variables (Zscheischler and Lehner, 2022). It may be possible to combine the contributing factors into a single index, for example, the fire weather index (van Oldenborgh et al., 2021a; Krikken et al., 2021), the heat index (Zachariah et al., 2023b; Mitchell, 2016; Wehner et al., 2016), or the spatial extent of a drought (Bevacqua et al., 2024). The preferred index may depend on the region under study, and there is sometimes a trade-off between an index being more suitable for either climatological or impact purposes. A bivariate approach can be used to define compound events where such indices do not exist (Zscheischler and Lehner, 2022) or to complement a univariate analysis by disentangling the contribution of related variables (e.g., Kimutai et al., 2023a). For a more in-depth discussion around compound events, see Section 4.3. Moreover, storyline approaches can include compounding factors, multivariate aspects and preconditioning, and cascading impacts of extreme events in a way that may provide insight into the specific role of the different contributing factors (Lloyd and Shepherd, 2021; Mishra et al., 2023; see Section 5).
Attribution studies may also be motivated by research questions more related to the description of climate change, or the understanding of its dynamics and feedback, rather than impacts. In these cases, the event definition will tend to focus on a meteorological or hydrological extreme. For example, there is a rapidly emerging challenge in conducting attribution of record-shattering events (Zhang et al., 2024; Bevacqua et al., 2024), which are a feature of escalating climate change (Fischer et al., 2021). In defining the event, these studies answer questions such as: How has climate change influenced this record-shattering weather extreme? Are we witnessing events that could not have happened without climate change? Are there new physical processes leading to these record-shattering events or a combination of existing extremes that we do not yet have the tools to define? What is the likelihood of an as-yet-unseen extremity occurring in a particular city? Can models reliably simulate the defined event and its underpinning physical mechanisms? Some of these questions lean towards the discussion in Sections 4 and 5.
Having outlined what is desirable for constructing a useful event definition, we acknowledge there are still obstacles that can lead to a compromised definition or a decision to discontinue a study. The choice of which variable to attribute may be influenced by data limitations, especially when evaluating impacts (Mitchell, 2016). Such limitations include short time series, missing data, changes in recording practices, and data being not publicly or freely available (Figure 2). Hence vulnerable countries that are likely also sparse in observational data (see Section 1) are also those for which attribution studies are most hindered since an extreme event cannot be suitably defined for an EEA assessment (Huggel et al., 2016; Kimutai et al., 2023b).
Attribution of the impacts of a given weather extreme event (see Section 6) poses further challenges for event definition. This is because the timing and scale of the impacts of interest likely differ from the causal weather event. For example, heatwaves generally cover large spatial domains over multiple political boundaries and may persist for weeks (e.g., Dole et al., 2011; Otto et al., 2012; Karmakar and Das, 2020), however, the impact of interest such as the mortality burden may be limited to just one political domain such as a small country or a large city (see Mitchell, 2016) over the hottest days. These considerations, as well as others, ultimately bear significant influence on how the impact is defined in the context of attribution. Moreover, the spatiotemporal differences between an impact and the causal event (Perkins-Kirkpatrick et al., 2022), and the complexity of these relationships, may require the use of separate attribution methods to assess the links between climate change and the hazard, and the hazard and the impact (e.g., Schaller et al., 2016). This is important to identify at the initial phase of the study when the event is defined and can be overcome by interdisciplinary collaboration between attribution scientists and impacts experts (Perkins-Kirkpatrick et al., 2022), such that the definition is underpinned by contemporary scientific understanding of the impact, as well as how it relates to weather and climate variables.
4 Statistical challenges in EEA
Once the event has been defined in terms of the variable of interest, duration, and spatial domain, an attribution analysis can be carried out to understand the effect of climate change on the event. Often, this is done in a probabilistic framework: the variable is aggregated over the specified time scale and region, and a time series representing that class of event in that area is obtained (for example, annual maxima of 3-day accumulated precipitation over a pre-defined domain). Here we discuss some commonly used probabilistic techniques, evolving challenges surrounding multivariate extreme events, and the emergence of record-shattering extremes.
4.1 Univariate probabilistic methods
Probabilistic event attribution uses a statistical model to represent the relationship between the variable of interest and the factors on which that variable depends—typically some measure of climate change, although other covariates may be included. A commonly used approach consists of modelling a variable’s annual maxima (or minima) by a generalised extreme value (GEV) distribution. This GEV is typically assumed to be non-stationary, using global or regional temperature as a covariate upon which the variable of interest depends (van Oldenborgh et al., 2021a; Robin and Ribes, 2020; Naveau et al., 2020), as in Figure 4a. For a given change in the covariate corresponding to the change between a factual and counterfactual climate of interest—for example, the present climate and a cooler climate representing the pre-industrial period—the statistical model can be used to estimate the expected change in frequency and intensity of the event due to climate change. The change in frequency is typically expressed as a change in the probability of exceeding a particular threshold in any year and is known as the probability ratio, given by the ratio p1/p0 in Figure 4b. For very extreme events, and particularly for heat extremes where the climate change signal is very strong, this ratio may approach infinity; Paciorek et al. (2018) discuss how to estimate one-sided confidence intervals for probability ratios using likelihood ratio tests when this occurs. While the GEV focuses on the most extreme event over a given time period, the generalised pareto distribution (GPD), another family of probability distributions that model the tail behaviour of a parent distribution, can be used in a similar way for all rare events above a chosen threshold. In cases where an average of a given climate variable is analysed, a normal distribution is likely to be a more appropriate choice (Schär et al., 2004; Ribes et al., 2020), and other distributions may be used in specific cases. Despite its apparent simplicity, univariate event attribution is still the subject of active methodological research, and several challenges are worth discussing.
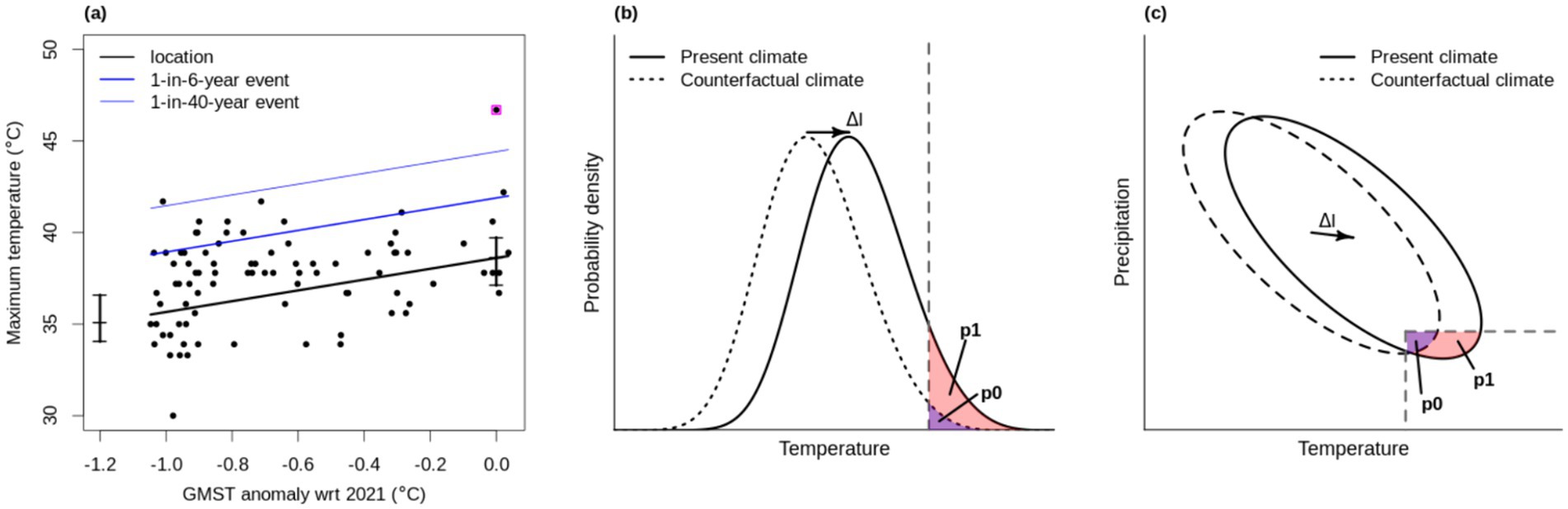
Figure 4. Plots illustrating probabilistic attribution of univariate and bivariate events. (a) Trend plot showing a non-stationary GEV distribution fitted to annual maximum temperatures at Portland International Airport (GHCNd). Points indicate observed annual maxima against global mean surface temperature (GMST), with the record-shattering 2021 event highlighted in pink. The black solid line indicates the centre of the distribution, which shifts with increasing GMST; the lines above represent expected 6- and 40-year return levels. Vertical bars represent uncertainty about the centre of the distribution in the 2021 climate and in a counterfactual 1.2°C cooler climate. (b) Schematic showing the modelled shift in temperatures between the present (solid line) and past climates (dotted line). The dashed vertical line indicates the magnitude of the event of interest; the shaded area underneath each curve represents the probability of observing an event at least as extreme as this in the counterfactual climate (p0) and the current climate (p1). The arrow labelled “I” shows the change in intensity of the event between the past and present climates. (c) As (b), but showing the shift in the bivariate probability distribution of temperature and precipitation. Ellipses represent contours of equal joint probability; the shaded area represents the probability of observing an event at least as extreme as the one represented by the dashed lines in the counterfactual (p0) and current climate (p1).
Studies with a focus on extreme temperature often assume that only the location parameter (characterising “typical” extremes) is shifting with the covariates—as in the example in Figures 4a,b—while others also allow the scale of the distribution to vary. Most studies assume that the tail shape of the distribution—critical for assessing the return period of extremely rare events—is constant, but this may not always be the case (e.g., Slater et al., 2021). Furthermore, accurately estimating the shape parameter of statistical distributions requires a large sample size, which is often a limiting factor in climate datasets (Fischer et al., 2021). Various studies have highlighted the benefits of incorporating additional covariates to account for physical processes known to affect the index of interest (e.g., large-scale modes of variability). Assuming that the underlying physical processes can be identified and captured, including process-based covariates, may improve the quality of the fit, allowing conditional attribution statements such as changes in the return levels and return periods given a certain state of internal variability (e.g., Sillmann et al., 2011; Sippel et al., 2020; Zeder and Fischer, 2023). The parameters of the statistical model may be estimated via frequentist approaches (e.g., maximum likelihood, van Oldenborgh et al., 2021b) or Bayesian techniques (e.g., Zeder and Fischer, 2023; Auld et al., 2023). Recent research suggests that Bayesian parameter estimates, which incorporate parameter uncertainty and so tend to produce wider fitted distributions than maximum likelihood estimates, give more reliable return time estimates (Zeder et al., 2023); however, these require careful specification of the prior parameter distributions.
In some cases, the chosen variable may not be well approximated by the assumed distribution, leading to biases in estimated probabilities. This may occur because the observations arise from different processes and so are drawn from a mixture of distributions; in the case of annual or seasonal maxima, the sampled events may not be far enough in the tail to ensure asymptotic convergence to the GEV (Ben Alaya et al., 2020, 2021) or the block lengths may be too short. While using longer blocks can be a solution, this may not be feasible in short observational datasets. An alternative involves using distributions such as the extended GEV (eGEV, Nascimento et al., 2016). Furthermore, observation-only-based attribution assumes that the observational record is long enough to represent the response to external forcing. This is challenging for extremes at regional to local levels that are known to be affected by high internal variability, such as daily or hourly precipitation maxima or annual temperature minima. Recent work suggests that training statistical models with process-based covariates on Single-Model Initial-condition Large Ensembles (SMILEs) that simulate many realisations of possible extremes under a given forcing scenario (Suarez-Gutierrez et al., 2020; Bevacqua et al., 2023) and applying these models to reanalyses or observations (Zeder and Fischer, 2023) can help overcome this issue. If the response is expected to be spatially homogeneous over the region of interest, an alternative approach is spatial pooling (Tradowsky et al., 2023). However, in the case of spatially heterogeneous variables such as extreme precipitation, simple spatial pooling may not be an appropriate solution (Sun and Lall, 2015; Li et al., 2019). Another approach is to develop approaches that eschew climate models entirely in a data-driven approach using Granger causal inference methods (Ebert-Uphoff and Deng, 2012). This could be done by constructing a suitable statistical model of observations incorporating relevant non-stationary covariates (Risser and Wehner, 2017; Risser et al., 2021, 2024).
A major challenge in recent years has been to combine information from climate models and observations to produce a single robust attribution statement. One approach is to re-fit the same statistical model to observations and climate models and report the weighted mean of the outputs of interest, typically the probability ratio or change in intensity (see Li and Otto, 2022). A Bayesian procedure to combine information from models and observations into a single non-stationary distribution has been proposed (Robin and Ribes, 2020), in which models are used to define the prior distribution, although more work is required to generalise the approach to variables other than temperature. Other observational constraints could also be used (e.g., model weighting; Brunner et al., 2019).
4.2 Attribution of record-shattering extreme events
In recent years, a number of record-shattering extremes (Fischer et al., 2021, 2023) have been observed, for example, the Pacific Northwest heatwave in 2021 (Philip et al., 2022; Bercos-Hickey et al., 2022). Such extremes can result in severe impacts, often stretching adaptation planning (e.g., Silberner, 2021; Tradowsky et al., 2023). Event attribution of such record-shattering extremes is particularly difficult, as many of the statistical challenges listed above are more pronounced, and some additional issues emerge particularly in observation-based methods in which data availability is limited. Many of the statistical approaches discussed above are unconditional, in that they assume that the events of interest (e.g., all annual maxima) are drawn from a single population. When a record-shattering event arises, this may no longer be a defensible assumption. In the most extreme cases, an event may be far outside the distribution in the previously observed record.
In such cases, numerical modelling approaches must be relied upon. Methods have been developed to simulate trajectories of very rare extremes, such as the UNSEEN approach using initialised hindcasts on subseasonal to seasonal timescales (Thompson et al., 2017; Kent et al., 2017; Risbey et al., 2023) and ensemble boosting based on fully coupled models (Fischer et al., 2023; Gessner et al., 2021; Ragone and Bouchet, 2021). Unlike the methods described above, the purpose here is to increase sample size virtually rather than address dynamical uncertainties. This is achieved by initialising a large number of model simulations with very similar dynamical conditions to those observed at or prior to the event of interest. This can then increase the accuracy of any extreme value statistics employed to assess changes in the frequency and/or intensity of the event due to climate change. Figure 5 demonstrates, with the mix of large ensembles and re-initialised conditions, how ensemble boosting resulted in the simulation of five heatwaves more extreme than the Pacific Northwest event of 2021 (see Fischer et al., 2023).
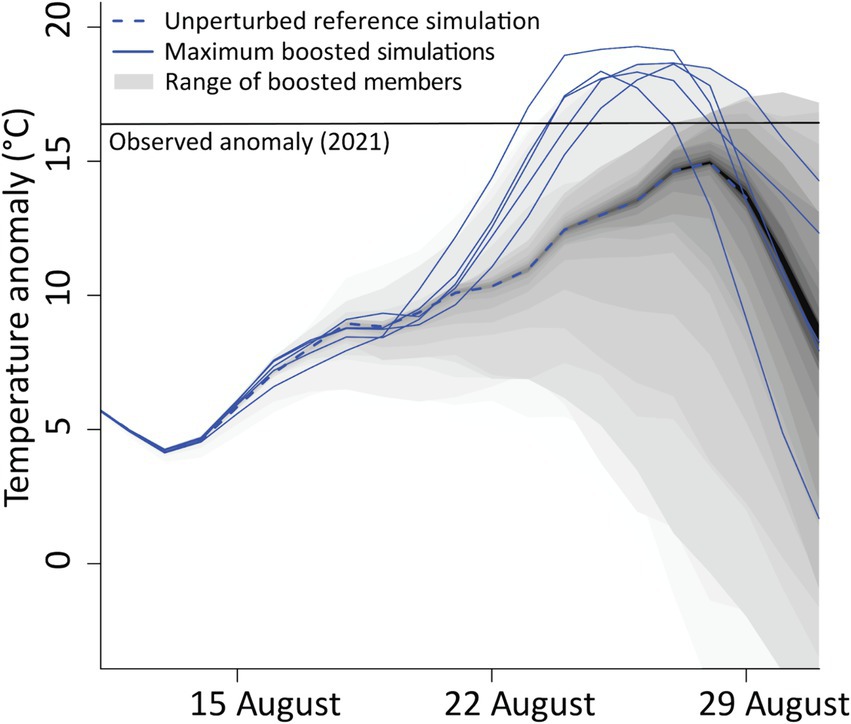
Figure 5. Simulations of 5-day maximum temperature over the Pacific Northwest from the CESM2 climate model with lead times of 5–23 days. Each lead time has a 300-member ensemble, where each member is perturbed by a round-off each in specific humidity. The result is a large ensemble of different background conditions in the lead-up to a heatwave pre-simulated in a transient run by the same model. Five individual members exceed the anomaly observed over the Pacific Northwest in 2021, demonstrating that appropriate samples of record-shattering events can be developed from current dynamical understanding and climate model capabilities. Adapted from Fischer et al. (2023).
Moreover, for the attribution of record-shattering extremes, the availability of SMILES and large ensembles of experiments with prescribed SSTs offer novel opportunities, because due to their large sample sizes, they provide a better sampling of the tails of the distribution in numerous states of plausible climate variability. Additionally, in contrast to observational estimates, they also provide information about future climate states (Deser et al., 2020; Bevacqua et al., 2023). Recent work has also used re-initialised weather forecasts to simulate individual events in a counterfactual climate (Leach et al., 2024), providing detailed information about the effect of climate change on the weather in that particular case. Section 5 delves deeper into the role of physical climate model simulations in attribution.
Many EEA studies deliberately exclude the event in question when fitting a non-stationary distribution, in order to avoid a selection bias (Miralles and Davison, 2023). Including the record-shattering value (e.g., the highlighted observation in Figure 4a) can distort the fit of the distribution, leading to low confidence in estimated return times, which can be sensitive to the most extreme values in the sample (Bercos-Hickey et al., 2022; Philip et al., 2022; Bartusek et al., 2022; McKinnon and Simpson, 2022; Thompson et al., 2023). This problem may be mitigated by using Bayesian parameter estimates, which tend to be less susceptible to single influential observations (Zeder et al., 2023). On the other hand, it has been shown for the case of the 2021 Pacific Northwest heatwave that the observed event is beyond the upper bound of the fitted distribution if the event itself was excluded from the analysis (Bercos-Hickey et al., 2022; Philip et al., 2022). Excluding a record-shattering extreme implies another type of selection and may violate the assumptions in GEV distributions (Miralles and Davison, 2023; Zeder et al., 2023). Alternatively, record-shattering events can be considered as resulting from an implicit “stopping rule”: the event is investigated because the latest value is the first to exceed a threshold of interest, for example, the historic 100-year return level. Accounting for the stopping rule has been shown to reduce biases in return level estimates whilst still including the most extreme events (Barlow et al., 2020; Miralles and Davison, 2023; Vautard et al., 2024).
4.3 Emerging statistical methods—beyond univariate probabilistic attribution
While probabilistic methods for univariate event attribution are relatively well established, some modifications are needed to handle situations where impacts arise from several weather events in combination; these compound events manifest in various forms, encompassing extreme and moderate conditions in multiple variables happening simultaneously or across space and time (Zscheischler et al., 2020). For example, concurrent precipitation and storm surges can lead to widespread flooding in low-lying coastal areas (Bevacqua et al., 2020; Wahl et al., 2015), while compound hot-dry events can exacerbate tree mortality (Hammond et al., 2022). Given that compound events drive many high-impact events (Zscheischler et al., 2020; Bevacqua et al., 2021), attribution statements based on independent univariate exceedances may provide only partial and potentially misleading information about the anthropogenic influence on extreme events. For multivariate events like moist heatwaves or fire weather, analyses have typically relied on collapsing multiple indices into a physically meaningful univariate index and applying univariate statistical methods (see Section 3). Truly multivariate extreme value statistical methods are only just emerging (Cooley et al., 2019), but in some settings (e.g., Zachariah et al., 2023a), multivariate attribution approaches may be used to jointly attribute multiple variables, which may then be combined in impact models; for example, physiological models that require inputs of multiple variables such as temperature, specific humidity, and wind speed (Havenith and Fiala, 2011). Copula-based approaches may also be applied to effectively capture the nature of the relationship between physical hazards and their impacts (e.g., Baldwin et al., 2023). However, a compound event perspective that considers the underlying drivers of a representative univariate index can allow for a better understanding of the climate change effect by quantifying the contribution of the trends of the respective components of the index towards its longer-term changes (van Oldenborgh et al., 2021a; Kimutai et al., 2023a; Bevacqua et al., 2024). Moreover, understanding the dynamics of a compound weather extreme is particularly useful for evaluating climate models in terms of their fitness to conduct an attribution assessment (see Section 5).
Another way to approach compound event attribution is to extend the traditional univariate attribution approach to a multivariate setting, as shown in Figures 4b,c. When impacts are caused by a combination of extreme conditions in two hazard indicators—for example, concurrent hot and dry conditions that caused a crop failure—p0 and p1 can be defined as the probability of concurrent extremes (shaded in red in Figure 4c). Apart from the definition of the hazardous areas, which rely on two hazards rather than one, the framework is identical to the univariate case. While such extensions of the probability ratio framework to a multivariate setting have been proposed (Chiang et al., 2021; Zscheischler and Lehner, 2022), general guidelines and technical tools for compound event attribution are still limited. In particular, these concepts have only been illustrated for simplified cases, and a comprehensive framework that allows the attribution of complex compound event types to anthropogenic climate change is currently lacking.
The most prominent aspects that differentiate univariate and compound event attribution are related to (i) definition of the compound event, (ii) climate model evaluation, and (iii) the sample size required for the assessments. Challenges related to defining spatial and temporal scales become more prominent for compound events, where multiple variables driving the impacts need to be identified. Moreover, once multiple drivers have been identified, it is important to evaluate the ability of climate models to represent these drivers and their dependencies, for instance via simple correlations, copula theory, or multivariate extreme value theory (Villalobos-Herrera et al., 2021; Zscheischler et al., 2021; Zscheischler and Lehner, 2022; Qian et al., 2023). Finally, when taking a probabilistic approach, larger sample sizes are typically required for compound than univariate event attribution (Zscheischler and Lehner, 2022; Bevacqua et al., 2023). A large sample size—for example, from SMILEs—is particularly important for high-dimensional compound events such as multi-year droughts, spatially concurrent precipitation extremes, and multiple co-occurring fire drivers (Bevacqua et al., 2023).
To summarise, all of the methods discussed in Section 4 are affected by uncertainties both in sampling and statistical assumptions; however, as noted by Stott (2016), confidence in the findings of a study can be increased by presenting the results of several complementary methods in combination, along with a discussion of the underlying physical processes. Care must be taken to communicate the event analysed in each case and the caveats associated with each method in order to avoid the impression of contradictory results that can arise from slightly different framings. Rather, emphasis should be placed on the strength of bringing together multiple lines of evidence and how this produces more robust attribution statements than relying on a single analysis.
5 Physical climate models and their application in EEA
5.1 Full probabilistic model simulations for EEA
A broad range of EEA approaches utilise physical climate models in combination with observations and statistical approaches, in particular to manage uncertainties. These models are employed to simulate the class of extreme events of interest in actual (with observed forcings) and counterfactual (with pre-industrial forcings) climate conditions. A widely used approach derives changes in occurrence probabilities following statistical methods in Section 4. This attribution approach, often called the probabilistic or risk-based approach, traditionally employs global climate model ensembles; however, over recent years ensembles of regional climate models (RCMs) have been developed for specific regional areas (e.g., Janes et al., 2019) and also examined in terms of EEA assessments (Stott et al., 2004; Stott et al., 2016; Seneviratne et al., 2021). A complementary conditional approach is based on event storyline simulations that can be implemented in different ways, utilising a range of climate models from global to convection permitting (Trenberth et al., 2015; Shepherd et al., 2018).
Physical climate models are useful tools that simulate many aspects of global climate, including atmospheric circulation and climate change. Climate models simulate many characteristics of observed extreme events in the present climate, as well as plausible patterns of changes. But the attribution of individual, often regional or local, extreme events to driving processes and external forcings requires setting a high bar. Two issues are particularly relevant. Firstly, climate models need to simulate realistic present-day characteristics of the extremes of interest, such as intensity, frequency, duration, and spatial extent. This depends on realistic representations of key processes underlying the event. Climate model biases in representing these processes may hinder the interpretation of results, and location biases may impede the subsequent use in impact attribution. Secondly, climate models should simulate realistic trends, and uncertainties in these trends need to allow for robust attribution statements. This involves both a realistic representation of key processes and the inclusion of all relevant forcings, which fall under the umbrella of fitness-for-purpose (Parker, 2009, 2020; Doblas-Reyes et al., 2021).
Extreme events are caused by an interplay of dynamical factors such as the weather pattern causing the event, thermodynamic factors such as the amount of water vapour available for precipitation, and feedbacks that amplify or moderate the event (Seneviratne et al., 2021). These processes act upon a range of spatial and temporal scales. Depending on the representation of key processes, climate models may not realistically represent all types of extreme events on which attribution assessments are desired. For instance, midlatitude heatwaves are often caused by a large-scale blocking event (an underlying dynamical component), their initial temperature is set by the temperature of the advected air mass (a thermodynamical component), and subsidence, while regional temperature soil moisture feedbacks and land use can amplify local temperatures (Hirsch et al., 2021). While anthropogenic climate change is, via thermodynamical processes, undoubtedly increasing the intensity and frequency of heatwaves, it is more challenging to ascertain the role of dynamical components, particularly in the presence of anthropogenic climate change. One approach that can isolate the role of anthropogenic climate change and other natural drivers is the storyline method, explained in greater detail in Section 5.2. When considering larger-scale mechanisms such as modes of variability, another method is to separate simulations where certain phases are active (e.g., El Nino or La Nina) and compare the event of interest in these simulations to each other and neutral conditions, both under factual and counterfactual climates (Karoly et al., 2016). Another approach is to assess whether a given phase of a mode of variability is a component of the long-term trend of a given class of extreme, which can help in determining the relative role of anthropogenic climate change both in the long-term change as well as the specific event of interest (Kimutai et al., 2023a; Clarke et al., 2024).
Moreover, not all of the dynamical processes of many extreme events are fully understood or realistically simulated (van Oldenborgh et al., 2022). Of particular importance to many extremes is the spatial resolution of the climate models employed. Important meso- and fine-scale dynamical processes are parameterised at coarser resolutions, with the result being a much less realistic simulation of the event. For example, lower-resolution regional climate models parameterise convection, resulting in less structurally defined hurricanes and lower precipitation and wind intensities (Patricola and Wehner, 2018). Figure 6 demonstrates this issue, with the finest resolution (1 km, Figure 6a) resulting in a very realistic hurricane structure in terms of cloud representation, outer rain bands, and the size of structure of the eye, especially when compared to the coarsest resolution presented (27 km; Figure 6c). It is worth noting that all spatial scales represented in Figure 6 are a result of bespoke dynamically downscaling analyses that are generally finer than many readily available climate model simulations, highlighting the need for targeted physical modelling for many types of EEA assessments.
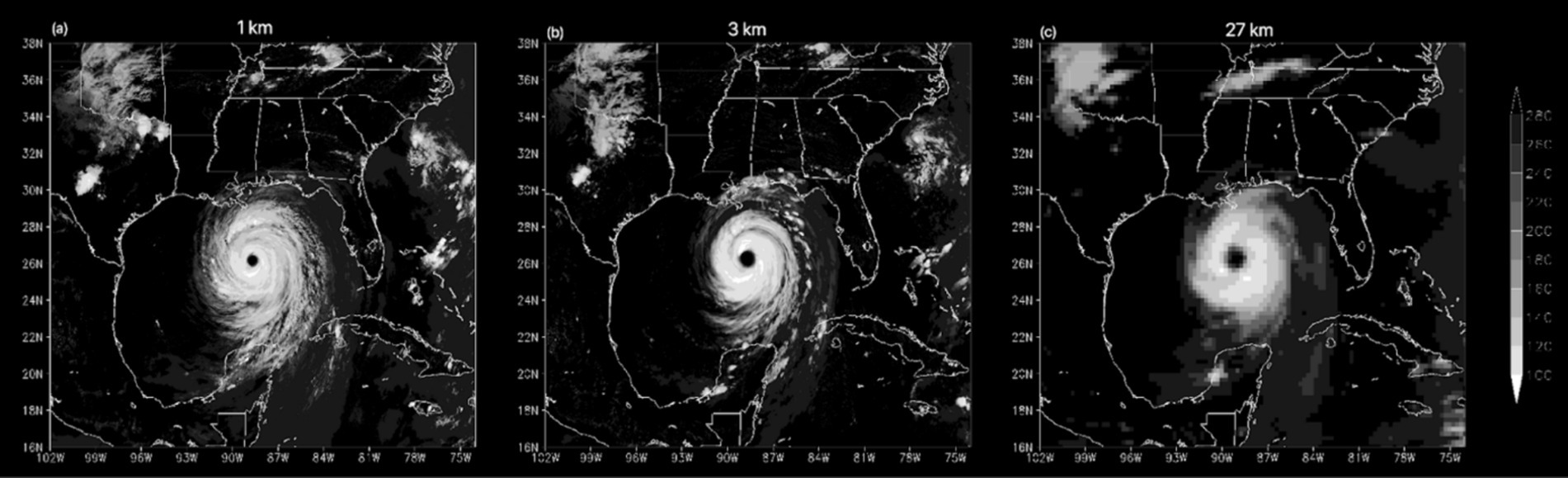
Figure 6. Hurricane Katrina as shown by outgoing longwave radiation (W/m2) at 1800GMT on 28 August 2005 from hindcasts simulated with the Weather Research and Forecasting (WRF) model at (a) 1 km, (b) 3 km, and (c) 27 km resolution. Simulations at 1 km and 3 km resolution did not use a convective parameterisation. Simulations from Patricola and Wehner (2018).
As an example of large-scale circulation biases, Davini and d’Andrea (2020) and Schiemann et al. (2020) demonstrated that global climate models underestimate the number of blocked days in the northern hemisphere summer, a crucial weather pattern causing midlatitude heatwaves. Recent studies also emphasised that global climate model simulations do not represent regional increases in the intensity of extreme heat, owing in large part to lack of change in dynamical weather patterns, such as for the underestimation (resp. overestimation) of the heat extremes trend in Western Europe (resp. Eastern U.S.A.; Patterson, 2023; Vautard et al., 2024, Singh et al., 2023). While these studies did not rule out internal interdecadal variability to explain such differences with exceptional situations in a few regions, it should be kept in mind that potential systematic dynamical biases can significantly alter an accurate regional estimate of changes in return periods.
Misrepresentations of relevant processes also cause biases in the variables of interest for EEA, such as temperature, precipitation, or wind (Eyring et al., 2021; Doblas-Reyes et al., 2021), as well as their dependencies (Zscheischler et al., 2019). These biases may affect not only the magnitude of a variable, but also the simulated location of weather events and temporal distribution (Maraun and Widmann, 2015; Maraun et al., 2021). Location biases are relevant for impact attribution (see Section 6), as the observed local impact cannot be correctly simulated should a climate model simulate the causal event in the wrong location (Mishra et al., 2023). Crucially, biases may depend on the state of the climate system and may thus change from present to future climate (Maraun, 2016). Thus, it is a non-trivial question how biases may be reliably adjusted, for example, by statistical methods, and which adjustments are admissible (Maraun et al., 2017; Doblas-Reyes et al., 2021).
Regarding regional and local processes, it is well known that standard RCMs substantially underestimate the intensities of heavy summer downpours over southern Europe (Ban et al., 2021; Pichelli et al., 2021), and over Southeast Asia have wetter biases than their parent GCMs relative to observations (Nguyen et al., 2022). It is therefore recommended that the representation of key processes is evaluated before proceeding with attribution (van Oldenborgh et al., 2021b). Indeed, in the case of evaluating for certain types of extreme events, such as hurricanes, the simulation of the event itself may be sufficient for passing evaluation, given the complex physics that need to be resolved (i.e., not estimated) on small spatial scales for such an event to realistically appear (Reed et al., 2020).
Regional circulation patterns are important for initiating extreme events, and uncertainties in projecting changes in patterns are substantial (Shepherd, 2014). An example is the polar jet stream and storm tracks in boreal winter (Lee et al., 2021; Harvey et al., 2020) and how these relate to the North Atlantic Oscillation. The dynamical processes that underpin weather extremes during summer tend to be more consistent (Davini and d’Andrea, 2020; Dong et al., 2022; Kang et al., 2023), but the ability of climate models to realistically simulate these processes and their changes has been questioned (Mann, 2018). Thermodynamic changes are directly linked to global mean temperature by well-understood physical processes; therefore, they usually exhibit strong and significant trends and can be attributed to anthropogenic forcing more directly leading to smaller uncertainties (Shepherd, 2014). Examples for such changes are increasing regional temperatures and the resulting increase in heat extremes (Perkins-Kirkpatrick and Gibson, 2017; Seneviratne et al., 2021) and the increasing water vapour content following Clausius–Clapeyron over oceans (Lee et al., 2021). These signals are often so strong that robust attribution statements can still be made, despite uncertain underpinning circulation changes (García-Portela and Maraun, 2023).
Finally, local-scale dynamical feedbacks may amplify or moderate forced changes in extreme events. Land-atmosphere feedbacks influence the severity of heatwaves and severe rainstorms (Seneviratne et al., 2010) and determine the likelihood of concurrent drought-heat events (Zscheischler and Seneviratne, 2017). While climate models can represent the underlying processes, the magnitude of feedbacks is often biased (Dirmeyer et al., 2006; Hall et al., 2008; Sippel et al., 2017) and affects long-term trends (Vogel et al., 2018). As these uncertainties determine the magnitude of the simulated trend, they affect attribution statements about the strength of anthropogenic influences. Moreover, local physical processes and how they are simulated pose particular challenges to the attribution of some types of extreme events, as these processes often operate on sub-grid model scales and are parameterised (e.g., Seneviratne et al., 2021; see Figure 6).
Key for trustworthy attribution statements is the inclusion of all relevant forcings on the climate system. Regional climate trends are not only caused by greenhouse gas emissions and internal climate variability, but also by regional changes in non-greenhouse gas radiative forcings. For instance, a substantial fraction of the increase in summer temperatures over Europe and the Mediterranean has been attributed to decreasing aerosol concentrations over these regions (Dong et al., 2017). These forcings play a role in amplifying heatwave trends, but also for evaporation and thus soil moisture drought (Boé et al., 2020). A further complication for regional individual extreme events is the direct human role in land surface changes, which affect soil moisture, runoff, and temperature extremes when vegetation is managed, changed, or replaced (e.g., Thiery et al., 2020; Cowan et al., 2020). Even radiative forcing by CO2 is not correctly represented in some RCMs, which assume constant CO2 concentrations and impose climatic changes purely via their boundary conditions (Jerez et al., 2018). Regional climate simulations have also been shown to underestimate temperature changes in some regions due to the lack of aerosol-forcing change (Schumacher et al., 2024).
The issues described call for a systematic assessment of which—if any—type of climate model is fit for the purpose of attributing the occurrence of a specific extreme event to anthropogenic forcings (Doblas-Reyes et al., 2021), including assessing whether the model simulates the event for the right physical reasons (Pfahl et al., 2017) and an extension of current model selection guidelines (García-Portela and Maraun, 2023). The evolving use of forecasting systems offers a novel opportunity to evaluate models used in attribution (e.g., Reed et al., 2020). Additionally, new approaches are emerging to better sample rare extreme events and the underlying dynamical set-ups of climate models. These include methods discussed in Section 4, such as UNSEEN, SMILES, and ensemble boosting (Thompson et al., 2017; Risbey et al., 2023; Suarez-Gutierrez et al., 2020; Bevacqua et al., 2023; Fischer et al., 2023).
Managing model uncertainties remains a challenge in EEA. Within the statistical approach of EEA, ensembles of models are considered, together with observations, and both variability and model uncertainties are considered (see, e.g., the WWA protocol, Philip et al., 2020) using inter-model and within-model spread estimates, coupled with a strict model selection. The Bayesian approach also provides a built-in management of uncertainties with ensembles of simulations (see Section 4).
5.2 Conditional model simulations in EEA
As noted in Sections 3 and 4, the sophisticated statistical and physical climate model methods that underpin EEA come with challenges and limitations. This should not deter from using them in attribution assessments, however, combining the most appropriate statistical methods with climate model data can be complex, further hindered by the type of extreme event being attributed (see Section 4) as well as the quality of the observations to detect the event in the first place (Section 2).
Conditional attribution methods following a storyline approach (Shepherd et al., 2018) are a potential solution. Here, attribution assessments may be split into the thermodynamical and dynamical components. The former includes keeping dynamical conditions fixed (Trenberth et al., 2015; Vautard et al., 2016), where the latter, which originated from climate analogue methods (Cattiaux et al., 2010; Vautard et al., 2016; Faranda et al., 2022), estimates changes in the probability of occurrence of a particular weather pattern (e.g., Faranda et al., 2023). Large-member re-initialised climate model ensembles via ensemble boosting (see Section 4.2) have also been explored to develop storylines of exceptionally rare extreme events, allowing for attribution where lead-up dynamical interactions are considered (Fischer et al., 2023; Figure 5). Traditional attribution methods typically concentrate on only thermodynamical components; however, conditional/storyline attribution allows for different types of models to understand how anthropogenic climate change is influencing the resulting extreme event and underpinning mechanisms (e.g., Reed et al., 2020).
The pseudo-global warming approach (Schär et al., 1996; Doblas-Reyes et al., 2021) is an alternative for conditional attribution, where specific physical model simulations are performed, bounded by the dynamical conditions of the event of interest. Fixing the dynamical conditions eliminates large-scale internal variability, such that typically a few simulations of the actual event are necessary to capture regional-scale randomness. Given that only individual events are simulated, these simulations are typically short and computationally efficient, allowing for very high resolution. Inherently, such a method focuses on the (better understood) thermodynamic contribution to such changes. As with analog-based methods, separate model simulations can be used to assess changes in the underlying dynamical conditions to arrive at a more complete understanding of the event (Maraun et al., 2022; Mishra et al., 2023).
Given the potential of conditional attribution to provide dynamically consistent extreme events for accurate statistical estimation and approximation, these methods should be further explored and developed by the wider community in the coming years. Such developments would be of great benefit to anyone interested in attribution beyond climate or academic circles, for example, impact attribution (see Section 6), as the event samples generated are realistic in their physical nature as well as plentiful sample sizes, which are fundamental components towards robust attribution assessments. Moreover, conditional methods relatively easily link to observed impact data. These characteristics make the approach also suitable for application for resource-limited institutions. A challenge for the community during the next decade and beyond is that these simulations are intrinsically event-specific, and therefore also require physical climate expertise.
6 The attribution of impacts of extreme events
Despite the predominant focus of attribution analyses on the anthropogenic signal behind weather extremes, EEA was initially developed to assess the influence of anthropogenic climate change on the impacts that result from them (Allen, 2003). Studies that achieve this by assessing losses (e.g., health, agriculture, infrastructure, or financial) attributable to climate change are known as “impact attribution.” These studies demonstrate more precisely the consequences of climate change for individuals and communities, and can serve as a powerful tool for communication or evidence for evaluating the factual basis of claims made in climate lawsuits and other applications.
Impact attribution brings both challenges and opportunities. Studies require teams with interdisciplinary expertise, collectively addressing impact models with substantial data requirements and climate model uncertainties (Section 5) that may be amplified when combined with modelling impacts. Via a proposed framework, Figure 7 attempts to capture these complexities, highlighting what stages may be public-facing via communication (see Section 7) and what stages happen behind the scenes in an interdisciplinary manner. It is important to note that the omittance of any one of these processes would render an impact attribution assessment less robust, and in some cases, meaningless. Thus, it is imperative that impact attribution is truly interdisciplinary. As impact attribution methods have become more refined, the opportunities these studies bring for conducting societally relevant attribution assessments, specifying event definitions that reflect the processes by which impacts arise (Section 3), and shedding light on the burden of anthropogenic climate change on a greater range of climate-sensitive systems may be realised. In this section, we provide a short overview of existing methods for impact attribution, key challenges in conducting these studies, and the opportunities that this development in attribution brings.
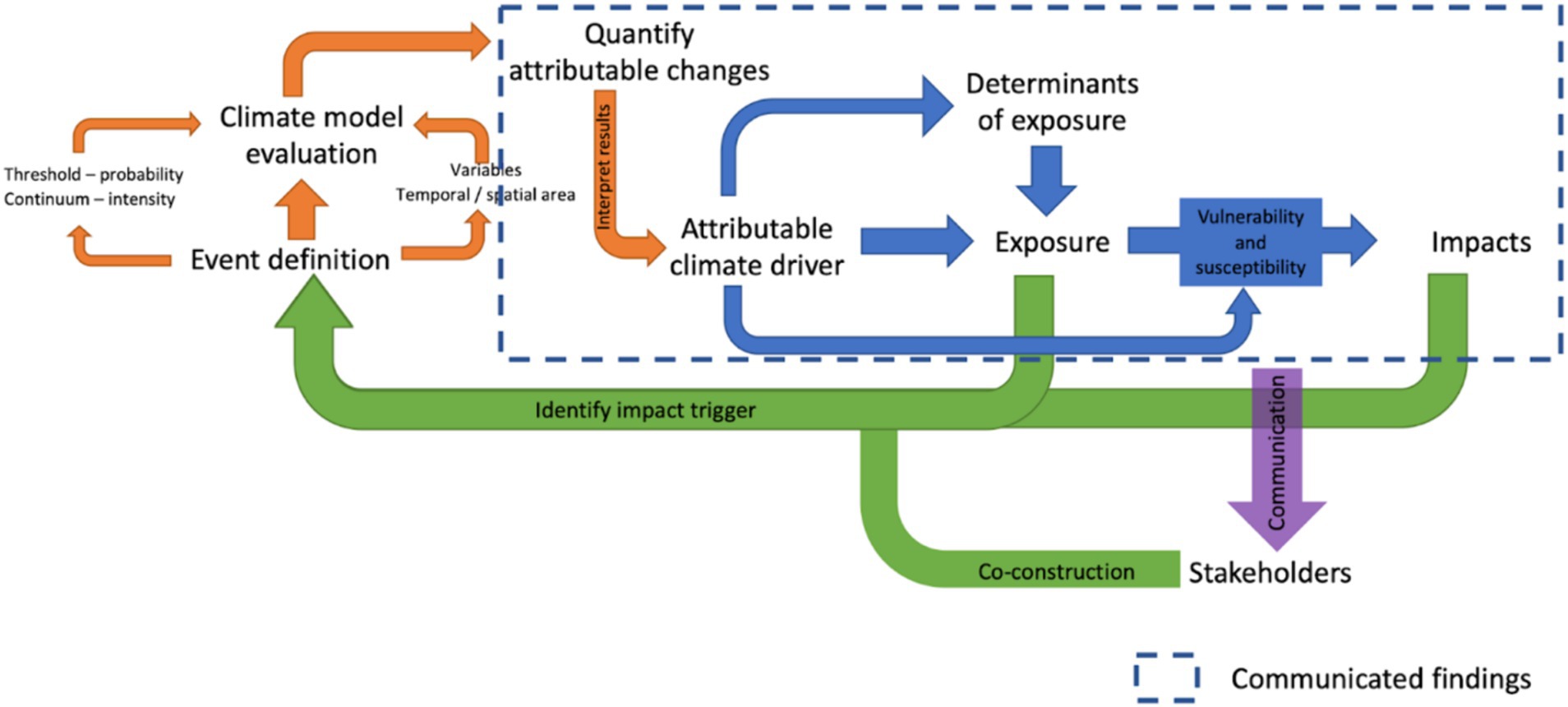
Figure 7. Draft framework for attributing the impacts of extreme events to climate change. Different colours denote the different disciplines required for a successful impact attribution assessment. Should one step not be properly executed, there would be knock-on effects for the rest of the project, rendering the results less reliable and even meaningless in some cases. Note that not all impact attribution assessments would follow this framework specifically, however, would look similar in terms of steps involved and the interweaving of disciplines and expertise.
6.1 Approaches to impact attribution
At present, the types of impacts exacerbated by climate change that have been assessed using formal attribution methods remain limited. Nevertheless, there is a strong theoretical basis indicating that climate change is already causing a wide range of health impacts worldwide (Callaghan et al., 2021). Moreover, data limitations have led to spatial biases in impact attribution analyses already conducted. Whilst observed climatological data challenges play a significant role (Section 2), so does the availability and quality of data from which relevant impacts are derived. This is important since some impact attribution studies combine climate change attribution methods with epidemiological, hydrological, agricultural, and land surface modelling. Collectively, these pioneering impact attribution studies have quantified financial losses from extreme weather events attributable to climate change (Frame et al., 2020a, 2020b) and a range of health (Mitchell, 2016; Vicedo-Cabrera et al., 2021, 2023; Stuart-Smith et al., 2024; Lo et al., 2023), hydrological (Pall et al., 2011; Pietroiusti et al., 2024), and agricultural impacts (Verschuur et al., 2021).
Methods that achieve the initial aims of EEA (Allen, 2003) and assess the impacts attributable to climate change have been developed relatively recently. These attribution analyses have typically been conducted following one of two approaches, which we term “probability-based” and “intensity-based,” respectively. Probability-based analyses typically combine an assessment of the portion of the likelihood of the event that caused certain impacts attributable to climate change (fraction of attributable risk and/or probability ratio, or FAR/PR; see Noy et al., 2024) with the losses that were caused by the hazard, such as heat-related mortality (Mitchell, 2016) or property and infrastructural impacts (Frame et al., 2020a, 2020b; Lott et al., 2021). Intensity-based analyses use relationships between the magnitude of a hazard and the resulting impacts to estimate impacts under contrasting climate conditions (e.g., with and without anthropogenic influence on the climate). Versions of these methods have been applied to quantify heat-related mortality (Vicedo-Cabrera et al., 2021, 2023; Stuart-Smith et al., 2024), burned area changes (Burton et al., 2024), virus outbreaks (Erazo et al., 2024), lake changes (Grant et al., 2021), and net ecosystem productivity (Bastos et al., 2023) attributable to climate change. Others have used hydrological models to ascertain the change in global river flows (Gudmundsson et al., 2021), flooded areas (Wehner and Sampson, 2021), and the area of the European river network facing drought (Bevacqua et al., 2024) under counterfactual climate conditions.
“Probability-based” and “intensity-based” approaches use similar resources and methods to assess different questions: the likelihood of exceeding an impact-relevant hazard threshold, or how the impacts in question would have been different under counterfactual climate conditions. As such, they may provide substantially different estimates of attributable impacts. Given the distinction between the approaches applied, recent work advised that probability-based approaches be reserved for binary impacts (that only occur when conditions exceed a threshold value), and intensity-based approaches for impacts that scale continuously with the hazard magnitude (Perkins-Kirkpatrick et al., 2022; Brown, 2023). Despite the approach, attribution of the impact must usually be performed separately to attribution of the hazard. This is because the impact of a specific hazard is unlikely to be a linear response to hazard itself, and it should not be assumed that the anthropogenic signal behind the hazard is identical to the impact (Perkins-Kirkpatrick et al., 2022). Moreover, FAR and other similar probabilistic approaches should be used with extra caution in impact attribution since FAR assesses the fractional change in an event class (e.g., all heatwaves over a specified domain) and not an individual event (e.g., an observed heatwave), which is the scale on which relevant impacts are typically pertinent (Perkins-Kirkpatrick et al., 2022).
When quantifying impacts that are attributable to climate change, studies seek to determine an event definition that captures the hazards driving the impacts in question. Considerations herein include the meteorological variables included (Lo et al., 2023; Baldwin et al., 2023), spatial, and temporal scale of the event in question (see Section 2). Impact attribution studies may also make use of approaches developed using climate projections. This includes evaluating changes in hazard impacts under storylines of changing climate hazards and land use (see Section 5; Maraun et al., 2022).
6.2 Challenges in extending attribution of meteorological hazards to local impacts
While recent studies have set out methods for quantifying impacts attributable to climate change, various challenges persist. These include (i) the construction of plausible counterfactuals; (ii) quantifying local climate change impacts based on coarse climate model outputs (Fiedler et al., 2021); (iii) limited impact data in some regions; and (iv) attributing multivariate hazards to assess impacts, as well as disagreement in appropriate indices for estimating the effects of climate hazards on impacts (Baldwin et al., 2023).
In common with EEA analyses, the construction of accurate counterfactuals is a challenge for impact attribution because climate models may contain substantial biases (see Section 5). Three different approaches are frequently used. Counterfactuals can be constructed by removing the long-term trend in the observed climate (Mengel et al., 2021), but this approach is unable to disentangle natural variability from forced climate responses. Another approach is a “delta change” method, where the projected trend from climate models is subtracted from observations. Here many different counterfactuals can be created from the different climate models, which allows a better separation between natural variability and the anthropogenic signal (Vicedo-Cabrera et al., 2023; Bevacqua et al., 2024). However, these approaches can lead to unrealistic counterfactual weather conditions, relevant to small-scale and compound events. Finally, climate models can be nudged towards observations (van Garderen et al., 2020) and counterfactuals created by changing boundary conditions (Hamed et al., 2024). For small-scale events such as heavy precipitation, this approach requires the creation of several counterfactuals to account for internal variability.
Secondly, biases in the location of large-to-regional-scale weather phenomena as well as the representation of local processes and feedbacks leading to an impact are additional challenges for accurate impact attribution assessments. To address some of these issues, conditional event attribution approaches such as storyline or forecast-based methods (see Section 5) may be better able to capture the multivariate and evolving nature of the impactful weather conditions than purely statistical approaches (e.g., Mishra et al., 2023).
Thirdly, running impact models may be limited by data constraints and computational needs. Whereas the data need for constructing robust hazard-impact relationships may be substantial, in many locations detailed impact data may not exist, be patchy in time or space, or be unavailable. This constrains the range of studies that can be conducted in attribution research and explains why multi-location studies such as Vicedo-Cabrera et al. (2021) are not truly global. The complexity of accurately measuring local-scale impacts may be further enhanced by changes in the measured impact due to behavioural adaptation (e.g., Ebi et al., 2021) that are difficult to measure and are not commonly accounted for in traditional hazard-impact relationships. Moreover, since impact models are often developed based on empirical relationships, it may be difficult to assess their reliability under counterfactual conditions. Furthermore, running a complex impact model for a suite of climate models can be computationally expensive and potentially prohibitive.
Finally, in the case of impacts that arise due to the combination of multiple meteorological drivers, copula-based multivariate attribution approaches (e.g., Zachariah et al., 2023a) and multivariate indices may yield different results. For instance, different heat stress indicators such as wet-bulb temperature and the universal thermal climate index can provide conflicting assessments. These indices combine different meteorological variables and represent different physiological assumptions. It is therefore essential to understand how well a given index captures the effects of weather on the impacts being assessed (Simpson et al., 2023).
A bottom-up approach may shift the focus of impact attribution studies. It is important to remember that those events with the worst impacts may not be the same as the events that are most extreme meteorologically (van der Wiel et al., 2020) as many other factors may play an important role. To make attribution studies more comprehensive, extending EEA to non-climatic drivers has recently been suggested (Jézéquel et al., 2024).
6.3 Using impact attribution findings
There is growing policy interest in developing workable loss and damage mechanisms, with ongoing efforts in national and international fora. This raises the question of how we can effectively leverage insights from existing attribution research and improve theoretical understandings of how climate change is affecting different phenomena and regional changes to help policymakers. In particular, attribution could assist in determining which losses would be eligible for compensation, on the basis of the extent to which they can be attributed to human influence.
Criteria for determining what counts as an impact of climate change eligible for compensation is primarily a legal or policy decision, and there is no current suggestion that loss and damage funds will be awarded for impacts that have met strict scientific attribution thresholds. However, as mechanisms for loss and damage funding become established, it may be helpful to draw on scientific insight to identify eligible impacts. This is where impact attribution might be useful. Existing scholarship has documented limitations of attribution in this context, including heterogeneous spatial coverage, relatively few impact attribution studies, and limited ability to simulate certain event types (King et al., 2023). Nonetheless, the deadliest weather events in developing nations (agricultural drought, tropical cyclones, floods, and heatwaves) are increasingly amenable to credible attribution analyses using storylines (Noy et al., 2023).
Certain storyline attribution studies of flooding have been combined with census and real estate data attempting to quantify environmental injustice (Smiley et al., 2022) and future exacerbation by climate change (Li et al., 2024). Smiley et al. (2022) found that 30–50% of the properties flooded in Hurricane Harvey would have been untouched without anthropogenic climate change, and that these attributable impacts were predominantly experienced non-White neighbourhoods. It is widely documented that low-income and socially and racially marginalised communities have disproportionate exposure to extreme weather events amplified by climate change. In the case of Hurricane Sandy, areas of New York most affected were those in the lowest quartiles of household income and highest portion of non-White residents (Lieberman-Cribbin et al., 2021). Attribution analyses that directly assess who experiences the additional impacts from changes in events’ intensities attributable to climate change more precisely identify the unequal distribution of the socioeconomic or health impacts of climate change.
Finally, impact attribution is sometimes cited as providing adaptation-relevant information (e.g., Stott et al., 2016). However, research on this topic has questioned this (see, e.g., Osaka and Bellamy, 2020), and other areas of scientific inquiry, such as climate change projections, may provide more directly relevant information for adaptation. This is discussed in more detail in Section 7.
7 Communication of EEA statements
The global increase in attribution studies has been accompanied by an increase in communication of attribution results outside of academia. The development of rapid attribution studies (Philip et al., 2020; Otto et al., 2022) and operational groups leading them, such as World Weather Attribution (WWA; Otto et al., 2022), EWERAM (Tradowsky et al., 2023), and ClimaMeter (Faranda et al., 2022), has led to wider integration of attribution results in general extreme event and climate change news coverage. The press briefings WWA holds after rapid studies are well attended by journalists from different countries, including the country where the event occurred. Together, the journalists generate a wealth of stories in several languages, thus reaching a large general audience. Journalists have noticed a change in extreme weather coverage to include attribution results in the past decade (Osaka et al., 2020). WWA uses its experience in communication over the years to tailor output to different audiences (van Oldenborgh et al., 2021b): (i) a scientific report focusing on technical reproducibility, (ii) a web summary with clear key messages in bullets and a main graphic for the science-literate audience, and (iii) a press release addressing the primary findings in simplified language for the public and media. The interest and media coverage depend on the event impact, regional occurrence, and estimated newsworthiness. Nevertheless, media interest in EEA continues to increase, and journalistic coverage of extreme events includes developing accessible resources such as EEA databases (e.g., https://www.carbonbrief.org/science/extreme-weather/attribution/).
This increase in news coverage and general communication around EEA results poses a few questions related to the purpose of communicating attribution. The main narrative is that EEA could be used to raise awareness, to make climate change more tangible by linking it to something everybody experiences: weather and its extremes (e.g., Stott et al., 2013; Demski et al., 2017; Shepherd et al., 2018; van Oldenborgh et al., 2021b), and ultimately as a tool to promote stronger public engagement with climate change science (Ettinger et al., 2021). An attribution study can also be a scientific substantiation for frequently occurring discussions about the role of climate change post-event.
On the other hand, there are still only a few academic studies analysing the outcome of EEA results on public opinion. Osaka and Bellamy (2020) conducted a survey of stakeholder and citizen perceptions on EEA of the California drought, for which several EEA studies provided seemingly contrasting results on the influence of climate change. They found that when presented with this evidence, laypeople would be drawn to studies in agreement with their pre-existing beliefs. Ettinger et al. (2021) tested the potential of EEA as an engaging tool to communicate climate change in the UK. The participants found EEA to be compelling to connect their personal experience of the weather with climate change. Overall, to better understand the potential of EEA communication, more research is needed, ideally at larger scales and including a variety of demographics (e.g., different social backgrounds, pre-existing climate change beliefs) and assessing how they react to a wider range of EEA case studies.
It is also important to assess the increase in EEA communication may have on the general engagement to fight climate change. One key concern is that EEA results could be instrumentalised to serve the interest of different stakeholders. For example, EEA could accentuate the climatisation of disasters—ascribing all experienced impacts to climate change (Grant et al., 2015)—and obscure other sources of vulnerability and exposure. Similarly, there is importance in communicating when no clear anthropogenic signal is found and the role of various factors (e.g., internal climate variability, model, and methodological shortcomings). Lahsen and Ribot (2022) highlight how attribution results can be used as a way for stakeholders to avoid responsibilities in the wake of disasters that were at least partially caused by bad management. Otto et al. (2022) and Ettinger et al. (2021) argue that EEA results should be accompanied by messages about vulnerability, as disasters are often caused by multiple factors. It is therefore part of the WWA protocol (Philip et al., 2020) to accompany a quantitative assessment of the role of climate change with an in-depth analysis of the role of vulnerability and exposure to the corresponding impacts. Especially for policymakers and humanitarian aid workers, the context of the event in terms of vulnerability and exposure is necessary to increase resilience (van Oldenborgh et al., 2021b). Identifying the role of climate change is particularly challenging when climatic events cause cascading impacts exacerbated by societal responses. Here, determining the role of climate change is challenging but important to better understand climate risk and will be an important challenge in the coming years.
Another challenge surrounds the communication of uncertainty. This message can become complex as studies using different methodologies or data can lead to diverging results for the same event (Otto et al., 2012). Osaka et al. (2020) found that differences in methodological choices are presented as disagreement within the scientific community rather than as answers to different questions surrounding the role of climate change behind the same event. Participants in Ettinger et al. (2021) were against the inclusion of uncertainty in general communications. This issue also relates to an active discussion within the scientific community regarding the best way to present uncertainties and the choice of the null hypothesis (Lloyd and Oreskes, 2018). At the core of this is whether the community prefers to avoid type I errors, false positives, e.g., attributing an event that is not caused by climate change, or type II errors, false negatives, e.g., not attributing an event that is caused by climate change. The current practice, likely inherited from classical statistics, is to avoid type I errors, but there are arguments that this choice goes against the precautionary principle. Consideration of multiple lines of evidence, including physical process reasoning and previous literature alongside the results from the data, can help to provide a rationale, but risks introducing additional uncertainty and unclarity in communication. This problem will become more critical with the rise in attribution of compound events and impacts as results become more complex (Zscheischler and Lehner, 2022).
A crucial point is the communication of changes in probabilities versus changes in intensities. While recently WWA has communicated both where feasible, the media may only broadcast the probability statements as these sound more dramatic, which can result in misleading consequences. The statements for the Pacific Northwest heatwave from 2021 read “Western North American extreme heat virtually impossible without human-caused climate change” and “this heatwave was about 2°C hotter than it would have been if it had occurred at the beginning of the industrial revolution” (Philip et al., 2020). Here the probabilistic statement means that any heatwave in that region exceeding the observed temperature of 49.6°C would have been virtually impossible. In the case of only the probability attribution result being reported, lay people may wrongly infer that without climate change, there would have been no heatwave at all. This is not the case, since the intensity attribution quantifies that climate increased the temperature of the heatwave by 2°C.
Similarly, where results are uncertain, it may be more informative to report the lower or upper bound of an analysis—for example, stating that an event was at least 10% more intense or up to five times more likely—but there is always a risk that this result will be reported or interpreted. A 10% change or 5-fold increase in probability distorts the findings, although many journalists are becoming better at presenting this nuance. WWA guidelines for journalists (Clarke and Otto, 2023) aim to educate journalists in more consistent ways of reporting. Here new ways of communication are needed, and great care is taken to neither exaggerate nor downplay the influence of climate change. This is a key challenge the community must collectively address, such that effective solutions are devised that are communicatively effective and uphold scientific integrity and rigour.
Finally, there are different ways to package EEA results. van Oldenborgh et al. (2021b) summarise key points learnt over the years by WWA: provide several communication media, different levels of details and complexity, tailored to different audiences, and the usefulness of in-person discussions between EEA experts and its intended audience, especially non-scientists. There is still significant work to be done in understanding which type of audience is more responsive to which type of message. Moreover, EEA may be useful in helping lay people to accept climate change science and lowering their carbon footprint. However, again, more research is needed on how to successfully reach relevant groups. There is still some debate about whether quantitative or qualitative results are more engaging. Lewis et al. (2019) argue that quantitative results might not always be the best communication strategy, proposing calibrated language similar to IPCC vocabulary. However, the majority of participants in Ettinger et al. (2021) found quantitative results more compelling. Less traditional communication techniques such as serious games (Parker et al., 2016), or “spinner boards” (Dryden and Morgan, 2020) are other paths to explore. Finally, generative AI could potentially play a role in adjusting the communication about climate change to meet the specific needs and interests of different audiences (Sanders and Hendricks, 2023).
To advance on these topics, more interdisciplinary work with social scientists, in particular from cognitive and communication science, will be needed. A key challenge for the community will be to provide a clearer view on why we want to communicate on attribution studies, to whom, and with which message. Until a greater body of evidence is achieved on how attribution assessments are absorbed by a wide range of audiences, it is difficult to offer practical solutions in how to make such statements more accessible and digestible. A challenge for the wider attribution community over the coming years is to increase this research base, such that evidence-based recommendations to improve attribution communication become clearer and can be implemented across the board.
8 Concluding statements and recommendations
The sophistication and widespread use of EEA have undoubtedly increased since its inception. This article has demonstrated where remarkable advancements have been made but also where significant challenges remain. To highlight recent progress, Figure 8 presents an updated schematic of the scientific understanding and the attribution of various event classes, along with the original figure presented by National Academies of Sciences, Engineering, and Medicine (NASEM) (2016). It is clear that considerable gains have been made in the understanding and attribution of temperature-related extremes, whilst knowledge in other event types has also improved. New event categories have also emerged, e.g., the separation of drought types and tropical cyclone characteristics. In both instances, the scientific understanding behind and attribution of one sub-class is higher than the other. It would be useful to see an updated version of this figure in another decade as EEA continues to progress.
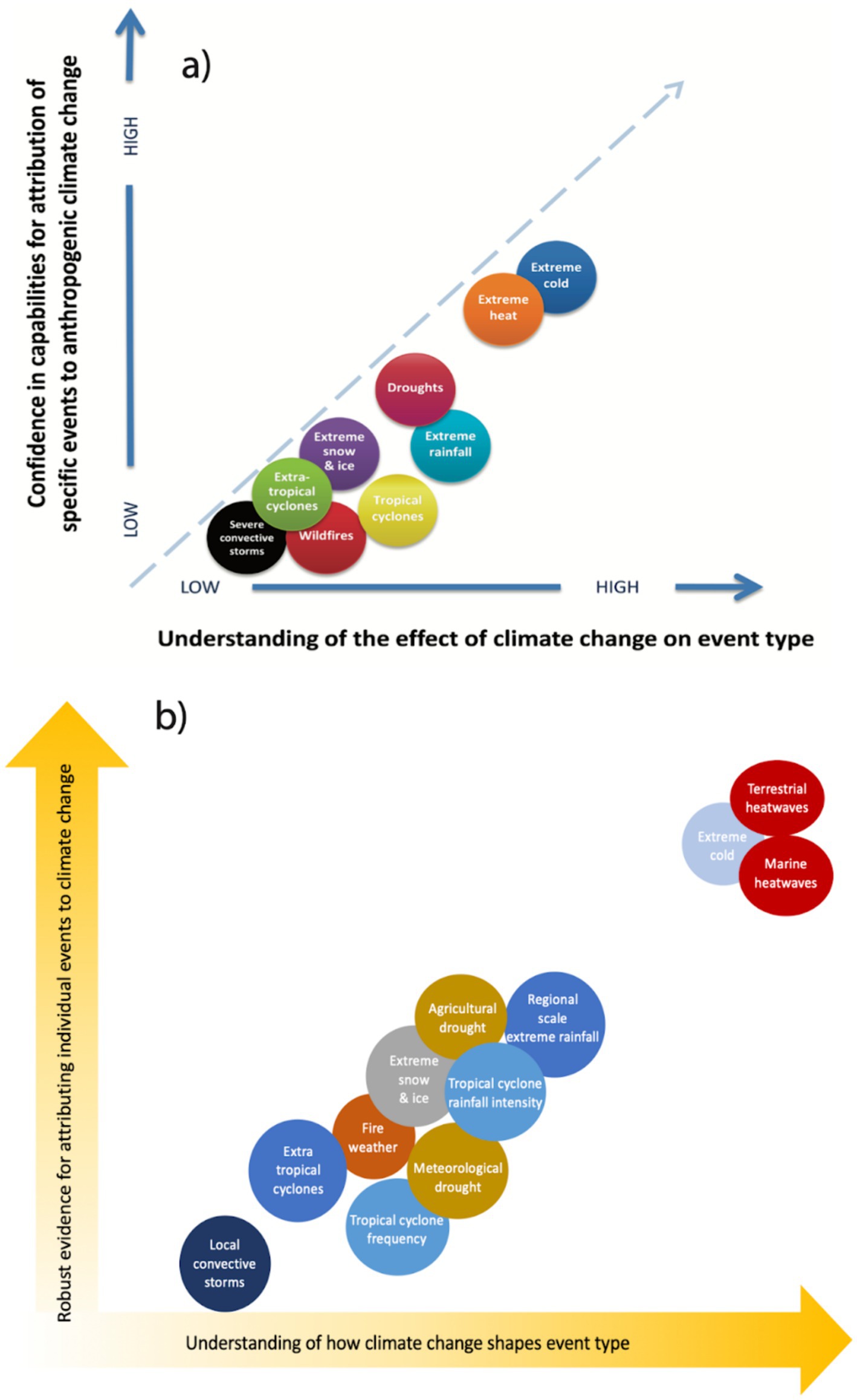
Figure 8. (a) Figure 4.7 from National Academies of Sciences, Engineering, and Medicine (NASEM) (2016), where the understanding of climate change (horizontal axis) on a given extreme event class is considered relative to confidence in the capabilities to attribute a specific event of that class to climate change (vertical axis); (b) an updated version of this schematic, based on assessments made in this article. Figure (a) is reproduced with permission from the National Academies of Sciences, Engineering, and Medicine (NASEM) (2016).
Current challenges in EEA include large gaps in observations both spatially and temporally, inhibiting EEA over large parts of the globe, resulting in inequitable representation of how climate change is influencing high-impact events. This inequity also extends to the capacity of developing nations to perform attribution assessments of local extreme events. While recent initiatives (e.g., WWA) have helped address the inequity of which extreme events are attributed and are attempting to increase local expertise by involving local experts from underrepresented regions, significant issues remain in local capacity, infrastructure, and in-house frameworks necessary for these locations to perform EEA independently. While there have been success stories in providing EEA capacity and infrastructure to some regions (e.g., Tett, 2020), there are also significant challenges in perpetuating engrained imbalances of power historically shaped by colonialism (Dodsworth, 2019). Moreover, as extremely rare, record-shattering and/or compound events become more prominent, traditional statistical and physical model-based attribution methods are challenged well beyond already known limitations. Furthermore, impact attribution and other applications of EEA such as quantifying loss and damage are increasing; however, the underpinning scientific methods are still in their infancy and are highly nuanced, requiring deep, co-ordinated, and ongoing interdisciplinary collaboration. And finally, the popularity of EEA as a communicative tool is steadfast; however, the community has only touched the tip of the iceberg in how to effectively communicate the scientific rigour embedded within EEA assessments. Whilst these challenges may appear insurmountable at first, they can certainly be overcome during the next decade, albeit with significant effort and with consistent interactions with expertise beyond climate science. Based on the findings of this article, we recommend focusing efforts on the following key areas:
1. Increased implementation of EEA studies across all regions, where possible, focusing on lower-income and more vulnerable nations. This requires not only increasing physical observations and corresponding access, as well as using reanalyses where appropriate, but also enhancing working with local experts. Proactive and sustained capacity building that no longer prolongs colonialist approaches and improves the infrastructure, skills, and knowledge required for underrepresented regions to independently perform EEA assessments must also be a priority. Moreover, this will also aid in directly answering any concerns these regions may have regarding EEA, whilst also using their input as to which events/types of events should be studied and their local knowledge on corresponding impacts. In turn, a by-product of increasing observations will allow for event definitions to be more objectively conducted.
2. Protocol in assessing the suitability of physical climate models for EEA. Whilst some basic evaluation is generally performed, a contemporary set of guidelines on how different types of climate models should be used, how to identify individual strengths and limitations, and how to evaluate their reliability for the class of event over the domain of interest is currently lacking.
3. Development of methodologies and best practice guides to account for compound and record-shattering extremes in EEA. As climate change intensifies, so will the occurrence and severity of these types of extremes. Suitable attribution methods must keep up. Although some methods show potential and should be explored further (e.g., storylines, conditional attribution; see Section 4), the understanding of these event types is currently rapidly evolving. This effort comes along with the continued progress of established statistical methods, as reviewed above. This will involve a crucial investment in computational resources to account for both dynamical and statistical uncertainty. Moreover, such resources would need to be shared equally such that the geographic distribution of EEA is improved.
4. Organised and ongoing engagement with impacts and legal communities to strengthen impacts data and develop protocols around impacts attribution and its role in associated applications. Given that the impact of a class of extremes is highly specific to a given field and/or region, deep collaboration with this field and attribution scientists is essential. New roles may emerge which straddle both fields. This will be best achieved by long-term, co-ordinated, and funded research initiatives, so that the underlying climate and impact methods are employed robustly and with high integrity.
5. Significant and ongoing investment in EEA communication. While groups like WWA have been highly successful in conducting and communicating EEA results widely, as a community, we still do not understand how this information is absorbed by different audiences. As a starting point, statements about the same event which appear to be conflicting need to be calibrated so that the public-facing information is complementary and not contradictory. Moreover, many EEA statements continue to be misconstrued by the media. Climate scientists should collaborate with communication experts in developing partnerships and techniques to mitigate this risk in the future.
It is an exciting time for EEA, with the identified challenges presenting new opportunities. EEA is rapidly progressing towards a transdisciplinary field. Thus, for any approach in addressing the identified challenges to be successful, it must occur with ongoing and deep collaborations outside of traditional climate science and attribution.
Data availability statement
The original contributions presented in the study are included in the article/supplementary material, further inquiries can be directed to the corresponding author.
Author contributions
SP-K: Conceptualization, Investigation, Methodology, Project administration, Visualization, Writing – original draft, Writing – review & editing, Supervision, Validation. LA: Conceptualization, Investigation, Project administration, Writing – original draft, Writing – review & editing. AK: Conceptualization, Investigation, Writing – original draft, Writing – review & editing. SK: Investigation, Visualization, Writing – original draft, Writing – review & editing. SP: Conceptualization, Investigation, Validation, Writing – original draft, Writing – review & editing. CB: Conceptualization, Data curation, Investigation, Validation, Visualization, Writing – original draft, Writing – review & editing. DM: Conceptualization, Investigation, Project administration, Validation, Writing – original draft, Writing – review & editing. RS-S: Conceptualization, Investigation, Validation, Visualization, Writing – original draft, Writing – review & editing. AJ: Conceptualization, Investigation, Validation, Visualization, Writing – original draft, Writing – review & editing. EB: Conceptualization, Validation, Writing – original draft, Writing – review & editing. SB: Conceptualization, Writing – original draft, Writing – review & editing. EF: Conceptualization, Investigation, Methodology, Visualization, Writing – original draft, Writing – review & editing. GH: Conceptualization, Investigation, Methodology, Validation, Writing – original draft, Writing – review & editing. JK: Conceptualization, Investigation, Visualization, Writing – original draft, Writing – review & editing. GK: Investigation, Validation, Writing – original draft, Writing – review & editing. KL: Investigation, Validation, Writing – original draft, Writing – review & editing. S-KM: Conceptualization, Investigation, Methodology, Writing – original draft, Writing – review & editing. MN: Conceptualization, Investigation, Writing – original draft, Writing – review & editing. RO: Conceptualization, Investigation, Validation, Writing – original draft, Writing – review & editing. CP: Validation, Visualization, Writing – original draft, Writing – review & editing. IP: Conceptualization, Investigation, Validation, Writing – original draft, Writing – review & editing. AR: Conceptualization, Investigation, Validation, Writing – original draft, Writing – review & editing. TS: Conceptualization, Investigation, Writing – original draft, Writing – review & editing. WT: Conceptualization, Investigation, Validation, Writing – original draft, Writing – review & editing. BT: Conceptualization, Investigation, Validation, Visualization, Writing – original draft, Writing – review & editing. RV: Conceptualization, Validation, Writing – original draft, Writing – review & editing. MW: Conceptualization, Investigation, Visualization, Writing – original draft, Writing – review & editing. JZ: Investigation, Methodology, Validation, Writing – original draft, Writing – review & editing.
Funding
The author(s) declare that financial support was received for the research, authorship, and/or publication of this article. SP-K and LA were funded by Australian Research Council Grant No. CE170100023. AK was funded by Australian Research Council Grant No. CE230100012. EB received funding from the Deutsche Forschungsgemeinschaft (DFG, German Research Foundation) via the Emmy Noether Programme (Grant ID 524780515). CB, EB, JZ, SK, and SP received funding from the European Union’s Horizon 2020 Research and Innovation Programme under Grant Agreement No. 101003469. TS acknowledges support from the National Science Foundation (AGS-2300037) and the National Atmospheric and Oceanic Administration (NA23OAR4310597). CP acknowledges support from the U.S. Department of Energy, Office of Science, Office of Biological and Environmental Research, Earth and Environmental Systems Modeling (EESM) Program, Early Career Research Program Award Number DE‐SC0021109. WT received funding from the European Research Council (ERC) under the European Union’s Horizon Framework Research and Innovation Programme (Grant Agreement No. 101076909; “LACRIMA” Project). KL is funded by the Intra-ACP Climate Services and Related Applications (ClimSA) Programme in Africa, an initiative funded by the European Union’s 11th European Development Fund and implemented by the African Centre of Meteorological Applications for Development through a grant with the African Union Commission as the contracting authority. GH received funding from the European Union’s Horizon 2020 Research and Innovation Programme under the Marie Sklodowska-Curie Grant Agreement No. 860100 (iMIRACLI) and from Natural Environment Research Council (NERC) Project GloSAT (NE/S015698/1). MW was supported by by the Director, Office of Science, Office of Biological and Environmental Research of the U.S. Department of Energy under Contract No. DE340AC02-05CH11231 under the Regional and Global Climate Modeling Program (RGCM) as part of the CASCADE SFA.
Acknowledgments
The authors thank Georgina Harmer from Monash University (Melbourne Australia) for assisting in the design of Figure 1.
Conflict of interest
The authors declare that the research was conducted in the absence of any commercial or financial relationships that could be construed as a potential conflict of interest.
Publisher’s note
All claims expressed in this article are solely those of the authors and do not necessarily represent those of their affiliated organizations, or those of the publisher, the editors and the reviewers. Any product that may be evaluated in this article, or claim that may be made by its manufacturer, is not guaranteed or endorsed by the publisher.
Footnotes
References
Alexander, L. V., Bador, M., Roca, R., Contractor, S., Donat, M. G., and Nguyen, P. L. (2020). Intercomparison of annual precipitation indices and extremes over global land areas from in situ, space-based and reanalysis products. Environ. Res. Lett. 15:055002. doi: 10.1088/1748-9326/AB79E2
Angélil, O., Perkins-Kirkpatrick, S., Alexander, L. V., Stone, D., Donat, M. G., Wehner, M., et al. (2016). Comparing regional precipitation and temperature extremes in climate model and reanalysis products. Weather Clim. Extrem. 13, 35–43. doi: 10.1016/j.wace.2016.07.001
Auld, G., Hegerl, G. C., and Papastathopoulos, I. (2023). Changes in the distribution of annual maximum temperatures in Europe. Adv. Stat. Climatol. Meteorol. Oceanogr. 9, 45–66. doi: 10.5194/ascmo-9-45-2023
Ayugi, B. O., Chung, E. S., Babaousmail, H., and Sian, K. T. C. L. K. (2024). Characterizing the performances of different observational precipitation products and their uncertainties over Africa. Environ. Res. Lett. 19:064009. doi: 10.1088/1748-9326/ad416b
Bador, M., Alexander, L. V., Contractor, S., and Roca, R. (2020). Diverse estimates of annual maxima daily precipitation in 22 state-of-the-art quasi-global land observation datasets. Environ. Res. Lett. 15:035005. doi: 10.1088/1748-9326/AB6A22
Baldwin, J. W., Benmarhnia, T., Ebi, K. L., Jay, O., Lutsko, N. J., and Vanos, J. K. (2023). Humidity’s role in heat-related health outcomes: a heated debate. Environ. Health Perspect. 131:055001. doi: 10.1289/EHP11807
Ban, N., Caillaud, C., Coppola, E., Pichelli, E., Sobolowski, S., Adinolfi, M., et al. (2021). The first multi-model ensemble of regional climate simulations at kilometer-scale resolution, part I: evaluation of precipitation. Clim. Dyn. 57, 275–302. doi: 10.1007/s00382-021-05708-w
Barlow, A. M., Sherlock, C., and Tawn, J. (2020). Inference for extreme values under threshold-based stopping rules. J. R. Stat. Soc. C 69, 765–789. doi: 10.1111/rssc.12420
Bartusek, S., Kornhuber, K., and Ting, M. (2022). 2021 North American heatwave amplified by climate change-driven nonlinear interactions. Nat. Clim. Change 12, 1143–1150. doi: 10.1038/s41558-022-01520-4
Bastos, A., Sippel, S., Frank, D., Mahecha, M. D., Zaehle, S., Zscheischler, J., et al. (2023). A joint framework for studying compound ecoclimatic events. Nat. Rev. Earth Environ. 4, 333–350. doi: 10.1038/s43017-023-00410-3
Ben Alaya, M., Zwiers, F., and Zhang, X. (2020). An evaluation of block-maximum-based estimation of very long return period precipitation extremes with a large ensemble climate simulation. J. Clim. 33, 6957–6970. doi: 10.1175/JCLI-D-19-0011.1
Ben Alaya, M., Zwiers, F., and Zhang, X. (2021). On estimating long period wind speed return levels from annual maxima. Weather Clim. Extrem. 34:100388. doi: 10.1016/j.wace.2021.100388
Bercos-Hickey, E., O’Brien, T. A., Wehner, M. F., Zhang, L., Patricola, C. M., Huang, H., et al. (2022). Anthropogenic contributions to the 2021 Pacific Northwest heatwave. Geophys. Res. Lett. 49:e2022GL099396. doi: 10.1029/2022GL099396
Bevacqua, E., De Michele, C., Manning, C., Couasnon, A., Ribeiro, A. F., Ramos, A. M., et al. (2021). Guidelines for studying diverse types of compound weather and climate events. Earth’s Future 9:e2021EF002340. doi: 10.1029/2021EF002340
Bevacqua, E., Rakovec, O., Schumacher, D. L., Kumar, R., Thober, S., Samaniego, L., et al., (2024). Direct and lagged climate change effects intensified the 2022 European drought. Nat. Geosci.. doi: 10.21203/rs.3.rs-3982665/v1. (accepted)
Bevacqua, E., Suarez-Gutierrez, L., Jézéquel, A., Lehner, F., Vrac, M., Yiou, P., et al. (2023). Advancing research on compound weather and climate events via large ensemble model simulations. Nat. Commun. 14:2145. doi: 10.1038/s41467-023-37847-5
Bevacqua, E., Vousdoukas, M. I., Zappa, G., Hodges, K., Shepherd, T. G., Maraun, D., et al. (2020). More meteorological events that drive compound coastal flooding are projected under climate change. Commun. Earth Environ. 1:p. doi: 10.1038/s43247-020-00044-z
Boé, J., Terray, L., Moine, M. P., Valcke, S., Bellucci, A., Drijfhout, S., et al. (2020). Past long-term summer warming over western Europe in new generation climate models: role of large-scale atmospheric circulation. Environ. Res. Lett. 15:084038. doi: 10.1088/1748-9326/ab8a89
Brown, P. T. (2023). When the fraction of attributable risk does not inform the impact associated with anthropogenic climate change. Clim. Change 176:115. doi: 10.1007/s10584-023-03591-4
Brunet, M., and Jones, P. (2011). Data rescue initiatives: bringing historical climate data into the 21st century. Clim. Res. 47, 29–40. doi: 10.3354/cr00960
Brunner, L., Lorenz, R., Zumwald, M., and Knutti, R. (2019). Quantifying uncertainty in European climate projections using combined performance-independence weighting. Environ. Res. Lett. 14:124010. doi: 10.1088/1748-9326/ab492f
Burton, C., Lampe, S., Kelley, D. I., Thiery, W., Hantson, S., Christidis, N., et al. (2024). Global burned area increasingly explained by climate change. doi: 10.21203/rs.3.rs-3168150/v1 (in review)
Callaghan, M., Schleussner, C. F., Nath, S., Lejeune, Q., Knutson, T. R., Reichstein, M., et al. (2021). Machine-learning-based evidence and attribution mapping of 100,000 climate impact studies. Nat. Clim. Change 11, 966–972. doi: 10.1038/s41558-021-01168-6
Cattiaux, J., and Ribes, A. (2018). Defining single extreme weather events in a climate perspective. Bull. Am. Meteorol. Soc. 99, 1557–1568. doi: 10.1175/BAMS-D-17-0281.1
Cattiaux, J., Vautard, R., Cassou, C., Yiou, P., Masson-Delmotte, V., and Codron, F. (2010). Winter 2010 in Europe: a cold extreme in a warming climate. Geophys. Res. Lett. 37:L20704. doi: 10.1029/2010GL044613
Chiang, F., Greve, P., Mazdiyasni, O., Wada, Y., and Agha Kouchak, A. (2021). A multivariate conditional probability ratio framework for the detection and attribution of compound climate extremes. Geophys. Res. Lett. 48:e2021GL094361. doi: 10.1029/2021GL094361
Clarke, B., Barnes, C., Rodrigues, R., Zachariah, M., Stewart, S., Raju, E., et al. (2024). Climate change, not El Niño, main driver of extreme drought in highly vulnerable Amazon River basin. London: World Weather Attribution, Imperial College London, 43.
Clarke, B., and Otto, F. E. L. (2023). Reporting extreme weather and climate change—guidelines for journalists
Cook, B. I., Smerdon, J. E., Cook, E. R., Williams, A. P., Anchukaitis, K. J., Mankin, J. S., et al. (2022). Megadroughts in the common era and the anthropocene. Nat. Rev. Earth Environ. 3, 741–757. doi: 10.1038/s43017-022-00329-1
Cooley, D., Thibaud, E., Castillo, F., and Wehner, M. F. (2019). A nonparametric method for producing isolines of bivariate exceedance probabilities. Extremes 22, 373–390. doi: 10.1007/s10687-019-00348-0
Cowan, T., Hegerl, G. C., Schurer, A., Tett, S. F. B., Vautard, R., Yiou, P., et al. (2020). Ocean and land forcing of the record-breaking Dust Bowl heatwaves across central United States. Nat. Commun. 11, 2870–2879. doi: 10.1038/s41467-020-16676-w
Davini, P., and d’Andrea, F. (2020). From CMIP3 to CMIP6: northern hemisphere atmospheric blocking simulation in present and future climate. J. Clim. 33, 10021–10038. doi: 10.1175/JCLI-D-19-0862.1
Demski, C., Capstick, S., Pidgeon, N., Sposato, R. G., and Spence, A. (2017). Experience of extreme weather affects climate change mitigation and adaptation responses. Clim. Change 140, 149–164. doi: 10.1007/s10584-016-1837-4
Deser, C., Lehner, F., Rodgers, K. B., Ault, T., Delworth, T. L., DiNezio, P. N., et al. (2020). Insights from earth system model initial-condition large ensembles and future prospects. Nat. Clim. Change 10, 277–286. doi: 10.1038/s41558-020-0731-2
Dirmeyer, P. A., Gao, X., Zhao, M., Guo, Z., Oki, T., and Hanasaki, N. (2006). GSWP-2: multimodel analysis and implications for our perception of the land surface. Bull. Am. Meteorol. Soc. 87, 1381–1398. doi: 10.1175/BAMS-87-10-1381
Doblas-Reyes, F. J., Sörensson, A. A., Almazroui, M., Dosio, A., Gutowski, W. J., Haarsma, R., et al. (2021). “Linking global to regional climate change” in Climate Change 2021—The Physical Science Basis: Working Group I Contribution to the Sixth Assessment Report of the Intergovernmental Panel on Climate Change. eds. V. Masson-Delmotte, P. Zhai, A. Pirani, S. L. Connors, C. Péan, and S. Berger, et al. (Cambridge: Cambridge University Press), 1363–1512.
Dodsworth, S. (2019). “The challenges of making research collaboration in Africa more equitable” in Oxford research encyclopedia of politics.
Dole, R., Hoerling, M., Perlwitz, J., Eischeid, J., Pegion, P., Zhang, T., et al. (2011). Was there a basis for anticipating the 2010 Russian heat wave? Geophys. Res. Lett. 38:L06702. doi: 10.1029/2010GL046582
Donat, M. G., Sillmann, J., Wild, S., Alexander, L. V., Lippmann, T., and Zwiers, F. W. (2014). Consistency of temperature and precipitation extremes across various global gridded in situ and reanalysis datasets. J. Clim. 27, 5019–5035. doi: 10.1175/JCLI-D-13-00405.1
Dong, B., Sutton, R. T., and Shaffrey, L. (2017). Understanding the rapid summer warming and changes in temperature extremes since the mid-1990s over Western Europe. Clim. Dyn. 48, 1537–1554. doi: 10.1007/s00382-016-3158-8
Dong, T., Zhu, X., Deng, R., Ma, Y., and Dong, W. (2022). Detection and attribution of extreme precipitation events over the Asian monsoon region. Weather Clim. Extrem. 38:100497. doi: 10.1016/j.wace.2022.100497
Dryden, R., and Morgan, M. G. (2020). A simple strategy to communicate about climate attribution. Bull. Am. Meteorol. Soc. 101, E949–E953. doi: 10.1175/BAMS-D-19-0174.1
Dunn, R. J., Donat, M. G., and Alexander, L. V. (2022). Comparing extremes indices in recent observational and reanalysis products. Front. Clim. 4:989505. doi: 10.3389/fclim.2022.989505
Ebert-Uphoff, I., and Deng, Y. (2012). Causal discovery for climate research using graphical models. J. Clim. 25, 5648–5665. doi: 10.1175/JCLI-D-11-00387.1
Ebi, K. L., Capon, A., Berry, P., Broderick, C., de Dear, R., Havenith, G., et al. (2021). Hot weather and heat extremes: health risks. Lancet 398, 698–708. doi: 10.1016/S0140-6736(21)01208-3
Erazo, D., Grant, L., Ghisbain, G., Marini, G., Colón-González, F. J., Wint, W., et al. (2024). Contribution of climate change to the spatial expansion of West Nile virus in Europe. Nat. Commun. 15:1196. doi: 10.1038/s41467-024-45290-3
Ettinger, J., Walton, P., Painter, J., Osaka, S., and Otto, F. E. L. (2021). “What’s up with the weather?” public engagement with extreme event attribution in the United Kingdom. Weather Clim. Soc. 13, 341–352. doi: 10.1175/WCAS-D-20-0155.1
Eyring, V., Gillett, N. P., Rao, K. M. A., Barimalala, R., Parrillo, M. B., Bellouin, N., et al. (2021). “Human influence on the climate system” in Climate Change 2021: The Physical Science Basis. Contribution of Working Group I to the Sixth Assessment Report of the Intergovernmental Panel on Climate Change. eds. V. Masson-Delmotte, P. Zhai, A. Pirani, S. L. Connors, C. Péan, and S. Berger, et al. (Cambridge: Cambridge University Press), 423–552.
Faranda, D., Bourdin, S., Ginesta, M., Krouma, M., Noyelle, R., Pons, F., et al. (2022). A climate-change attribution retrospective of some impactful weather extremes of 2021. Weather Clim. Dyn. 3, 1311–1340. doi: 10.5194/wcd-3-1311-2022
Faranda, D., Messori, G., Jezequel, A., Vrac, M., and Yiou, P. (2023). Atmospheric circulation compounds anthropogenic warming and impacts of climate extremes in Europe. Proc. Natl. Acad. Sci. U.S.A. 120:e2214525120. doi: 10.1073/pnas.2214525120
Fiedler, T., Pitman, A. J., Mackenzie, K., Wood, N., Jakob, C., and Perkins-Kirkpatrick, S. E. (2021). Business risk and the emergence of climate analytics. Nat. Clim. Change 11, 87–94. doi: 10.1038/s41558-020-00984-6
Fischer, E. M., Beyerle, U., Bloin-Wibe, L., Gessner, C., Humphrey, V., Lehner, F., et al. (2023). Storylines for unprecedented heatwaves based on ensemble boosting. Nat. Commun. 14:4643. doi: 10.1038/s41467-023-40112-4
Fischer, E. M., Sippel, S., and Knutti, R. (2021). Increasing probability of record-shattering climate extremes. Nat. Clim. Change 11, 689–695. doi: 10.1038/s41558-021-01092-9
Frame, D. J., Rosier, S. M., Noy, I., Harrington, L. J., Carey-Smith, T., Sparrow, S. N., et al. (2020a). Climate change attribution and the economic costs of extreme weather events: a study on damages from extreme rainfall and drought. Clim. Change 162, 781–797. doi: 10.1007/s10584-020-02729-y
Frame, D. J., Wehner, M. F., Noy, I., and Rosier, S. M. (2020b). The economic costs of Hurricane Harvey attributable to climate change. Clim. Change 160, 271–281. doi: 10.1007/s10584-020-02692-8
García-Portela, L., and Maraun, D. (2023). Overstating the effects of anthropogenic climate change? A critical assessment of attribution methods in climate science. Eur. J. Philos. Sci. 13, 17–24. doi: 10.1007/s13194-023-00516-x
Gessner, C., Fischer, E. M., Beyerle, U., and Knutti, R. (2021). Very rare heat extremes: quantifying and understanding using ensemble reinitialization. J. Clim. 34, 1–46. doi: 10.1175/JCLI-D-20-0916.1
Grant, S., Tamason, C. C., and Jensen, P. K. M. (2015). Climatization: a critical perspective of framing disasters as climate change events. Clim. Risk Manag. 10, 27–34. doi: 10.1016/j.crm.2015.09.003
Grant, L., Vanderkelen, I., Gudmundsson, L., Tan, Z., Perroud, M., Stepanenko, V. M., et al. (2021). Attribution of global lake systems change to anthropogenic forcing. Nat. Geosci. 14, 849–854. doi: 10.1038/s41561-021-00833-x
Gudmundsson, L., Boulange, J., Do, H. X., Gosling, S. N., Grillakis, M. G., Koutroulis, A. G., et al. (2021). Globally observed trends in mean and extreme river flow attributed to climate change. Science 371, 1159–1162. doi: 10.1126/science.aba3996
Hall, A., Qu, X., and Neelin, J. D. (2008). Improving predictions of summer climate change in the United States. Geophys. Res. Lett. 35. doi: 10.1029/2007GL032012
Hamed, R., Lesk, C., Shepherd, T., Goulart, H. M., van Garderen, L., van den Hurk, B., et al. (2024). Half of the unprecedented global soybean production failure in 2012 is attributable to climate change. doi: 10.21203/rs.3.rs-3834860/v1
Hammond, W. M., Williams, A. P., Abatzoglou, J. T., Adams, H. D., Klein, T., López, R., et al. (2022). Global field observations of tree die-off reveal hotter-drought fingerprint for Earth’s forests. Nat. Commun. 13:1761. doi: 10.1038/s41467-022-29289-2
Harrington, L. J., Otto, F. E. L., Cowan, T., and Hegerl, G. C. (2019). Circulation analogues and uncertainty in the time-evolution of extreme event probabilities: evidence from the 1947 central European heatwave. Clim. Dyn. 53, 2229–2247. doi: 10.1007/s00382-019-04820-2
Harrison, R. G. (2010). Natural ventilation effects on temperatures within Stevenson screens. Q. J. R. Meteorol. Soc. 136, 253–259. doi: 10.1002/QJ.537
Harvey, B. J., Cook, P., Shaffrey, L. C., and Schiemann, R. (2020). The response of the northern hemisphere storm tracks and jet streams to climate change in the CMIP3, CMIP5, and CMIP6 climate models. J. Geophys. Res. Atmos. 125:e2020JD032701. doi: 10.1029/2020JD032701
Havenith, G., and Fiala, D. (2011). Thermal indices and thermophysiological modelling for heat stress. Compr. Physiol. 6, 255–302. doi: 10.1002/cphy.c140051
Hegerl, G. C., Black, E., Allan, R. P., Ingram, W. J., Polson, D., Trenberth, K. E., et al. (2015). Quantifying changes in the global water cycle. Bull. Am. Meteorol. Soc. 96, 1097–1115. doi: 10.1175/BAMS-D-13-00212.1
Hirsch, A. L., Evans, J. P., Thomas, C., Conroy, B., Hart, M. A., Lipson, M., et al. (2021). Resolving the influence of local flows on urban heat amplification during heatwaves. Environ. Res. Lett. 16:064066. doi: 10.1088/1748-9326/ac0377
Hosseini-Moghari, S. M., and Tang, Q. (2022). Can IMERG data capture the scaling of precipitation extremes with temperature at different time scales? Geophys. Res. Lett. 49:e2021GL096392. doi: 10.1029/2021GL096392
Huggel, C., Wallimann-Helmer, I., Stone, D., and Cramer, W. (2016). Reconciling justice and attribution research to advance climate policy. Nat. Clim. Change 26, 100231–100908. doi: 10.1016/j.wace.2019.100231
Janes, T., McGrath, F., Macadam, I., and Jones, R. (2019). High-resolution climate projections for South Asia to inform climate impacts and adaptation studies in the Ganges-Brahmaputra-Meghna and Mahanadi deltas. Sci. Total Environ. 650, 1499–1520. doi: 10.1016/j.scitotenv.2018.08.376
Jerez, S., López-Romero, J. M., Turco, M., Jiménez-Guerrero, P., Vautard, R., and Montávez, J. P. (2018). Impact of evolving greenhouse gas forcing on the warming signal in regional climate model experiments. Nat. Commun. 9:1304. doi: 10.1038/s41467-018-03527-y
Jézéquel, A., Bastos, A., Wilson, A. M., Ramos, A. M., Shepherd, T. G., Stuart-Smith, R., et al. (2024). Broadening the scope of anthropogenic influence in extreme event attribution. Environ. Res.: Clim. 3:042003. doi: 10.1088/2752-5295/ad7527
Jézéquel, A., Dépoues, V., Guillemot, H., Trolliet, M., Vanderlinden, J.-P., and Yiou, P. (2018). Behind the veil of extreme event attribution. Clim. Change 149, 367–383. doi: 10.1007/s10584-018-2252-9
Junior, F. D. C. V., Zachariah, M., do Vale Silva, T. L., dos Santos, E. P., Coelho, C. A., Alves, L. M., et al. (2024). An attribution study of very intense rainfall events in Eastern Northeast Brazil. Weather Clim. Extrem. 45:100699. doi: 10.1016/j.wace.2024.100699
Kang, J. M., Shaw, T. A., and Sun, L. (2023). Arctic Sea ice loss weakens northern hemisphere summertime storminess but not until the late 21st century. Geophys. Res. Lett. 50:e2022GL102301. doi: 10.1029/2022GL102301
Karmakar, S., and Das, M. K. (2020). On the heat waves in Bangladesh, their trends and associated large-scale tropospheric conditions. J. Eng. Sci. 11, 19–36. doi: 10.3329/jes.v11i1.49544
Karoly, D. J., Black, M. T., Grose, M. R., and King, A. D. (2016). The roles of climate change and El Niño in the record low rainfall in October 2015 in Tasmania, Australia. Bull. Am. Meteorol. Soc. 97, S127–S130. doi: 10.1175/BAMS-D-16-0139.1
Kent, C., Pope, E., Thompson, V., Lewis, K., Scaife, A. A., and Dunstone, N. (2017). Using climate model simulations to assess the current climate risk to maize production. Environ. Res. Lett. 12:054012. doi: 10.1088/1748-9326/aa6cb9
Kimutai, J., Barnes, C., Zachariah, M., Philip, S., Kew, S., Pinto, I., et al. (2023a). Human-induced climate change increased drought severity in horn of Africa. London: Imperial College London.
Kimutai, J., Faka, D., Ayabagabo, P., Barnes, C., Zachariah, M., Pinto, I., et al. (2023b). Limited data prevent assessment of role of climate change in deadly floods affecting highly vulnerable communities around Lake Kivu. London: Grantham Institute, Imperial College London.
King, A. D., Alexander, L. V., and Donat, M. G. (2013). The efficacy of using gridded data to examine extreme rainfall characteristics: a case study from Australia. Int. J. Climatol. 33, 2376–2387. doi: 10.1002/joc.3588
King, A. D., Grose, M. R., Kimutai, J., Pinto, I., and Harrington, L. J. (2023). Event attribution is not ready for a major role in loss and damage. Nat. Clim. Change 13, 415–417. doi: 10.1038/s41558-023-01651-2
Kirchmeier-Young, M. C., Wan, H., Zhang, X., and Seneviratne, S. I. (2019). Importance of framing for extreme event attribution: the role of spatial and temporal scales. Earth’s Future 7, 1192–1204. doi: 10.1029/2019EF001253
Krikken, F., Lehner, F., Haustein, K., Drobyshev, I., and van Oldenborgh, G. J. (2021). Attribution of the role of climate change in the forest fires in Sweden 2018. Nat. Hazards Earth Syst. Sci. 21, 2169–2179. doi: 10.5194/nhess-21-2169-2021
Lahsen, M., and Ribot, J. (2022). Politics of attributing extreme events and disasters to climate change. Wiley Interdiscip. Rev. Clim. Change 13:e750. doi: 10.1002/wcc.750
Leach, N. J., Li, S., Sparrow, S., van Oldenborgh, G. J., Lott, F. C., Weisheimer, A., et al. (2020). Anthropogenic influence on the 2018 summer warm spell in Europe: the impact of different spatio-temporal scales. Bull. Am. Meteorol. Soc. 101, S41–S46. doi: 10.1175/BAMS-D-19-0201.1
Leach, N. J., Roberts, C. D., Aengenheyster, M., Heathcote, D., Mitchell, D. M., Thompson, V., et al. (2024). Heatwave attribution based on reliable operational weather forecasts. Nat. Commun. 15:4530. doi: 10.1038/s41467-024-48280-7
Lee, J.-Y., Marotzke, J., Bala, G., Cao, L., Corti, S., Dunne, J. P., et al. (2021). “Future global climate: scenario-based projections and near-term information” in Climate Change 2021 – The Physical Science Basis: Working Group I Contribution to the Sixth Assessment Report of the Intergovernmental Panel on Climate Change. eds. V. Masson-Delmotte, P. Zhai, A. Pirani, S. L. Connors, C. Péan, and S. Berger, et al. (Cambridge: Cambridge University Press), 553–672.
Lewis, S. C., King, A. D., Perkins-Kirkpatrick, S. E., and Wehner, M. F. (2019). Toward calibrated language for effectively communicating the results of extreme event attribution studies. Earth’s Future 7, 1020–1026. doi: 10.1029/2019EF001273
Li, S., and Otto, F. E. (2022). The role of human-induced climate change in heavy rainfall events such as the one associated with typhoon hagibis. Clim. Change 172:7. doi: 10.1007/s10584-022-03344-9
Li, X., Wehner, M., Judi, D., and Hetland, R. (2024). The influence of climate change on flooding and social inequalities from remnants of Hurricane Ida. Nat. Rev. Earth Environ. doi: 10.21203/rs.3.rs-3470345/v1 (accepted)
Li, C., Zwiers, F., Zhang, X., and Li, G. (2019). How much information is required to well constrain local estimates of future precipitation extremes? Earth’s Future 7, 11–24. doi: 10.1029/2018EF001001
Lieberman-Cribbin, W., Gillezeau, C., Schwartz, R. M., and Taioli, E. (2021). Unequal social vulnerability to hurricane Sandy flood exposure. J. Expo. Sci. Environ. Epidemiol. 31, 804–809. doi: 10.1038/s41370-020-0230-6
Lloyd, E. A., and Oreskes, N. (2018). Climate change attribution: when is it appropriate to accept New methods? Earth’s Future 6, 311–325. doi: 10.1002/2017EF000665
Lloyd, E. A., and Shepherd, T. G. (2021). Climate change attribution and legal contexts: evidence and the role of storylines. Clim. Change 167:28. doi: 10.1007/s10584-021-03177-y
Lo, Y. E., Mitchell, D. M., Buzan, J. R., Zscheischler, J., Schneider, R., Mistry, M. N., et al. (2023). Optimal heat stress metric for modelling heat-related mortality varies from country to country. Int. J. Climatol. 43, 5553–5568. doi: 10.1002/joc.8160
Lott, F. C., Ciavarella, A., Kennedy, J. J., King, A. D., Stott, P. A., Tett, S. F., et al. (2021). Quantifying the contribution of an individual to making extreme weather events more likely. Environ. Res. Lett. 16:104040. doi: 10.1088/1748-9326/abe9e9
Mann, M. E. (2018). “Reconciling climate model/data discrepancies: the case of the ‘Trees That Didn’t Bark’” in Climate modelling (Cham: Palgrave Macmillan), 175–197.
Maraun, D. (2016). Bias correcting climate change simulations—a critical review. Curr. Clim. Change Rep. 2, 211–220. doi: 10.1007/s40641-016-0050-x
Maraun, D., Knevels, R., Mishra, A. N., Truhetz, H., Bevacqua, E., Proske, H., et al. (2022). A severe landslide event in the alpine foreland under possible future climate and land-use changes. Commun. Earth Environ. 3:87. doi: 10.1038/s43247-022-00408-7
Maraun, D., Shepherd, T. G., Widmann, M., Zappa, G., Walton, D., Gutiérrez, J. M., et al. (2017). Towards process-informed bias correction of climate change simulations. Nat. Clim. Change 7, 764–773. doi: 10.1038/nclimate3418
Maraun, D., Truhetz, H., and Schaffer, A. (2021). Regional climate model biases, their dependence on synoptic circulation biases and the potential for bias adjustment: a process-oriented evaluation of the Austrian regional climate projections. J. Geophys. Res. Atmos. 126:e2020JD032824. doi: 10.1029/2020JD032824
Maraun, D., and Widmann, M. (2015). The representation of location by a regional climate model in complex terrain. Hydrol. Earth Syst. Sci. 19, 3449–3456. doi: 10.5194/hess-19-3449-2015
Matthews, T., Wilby, R. L., and Murphy, C. (2019). An emerging tropical cyclone–deadly heat compound hazard. Nat. Clim. Change 9, 602–606. doi: 10.1038/s41558-019-0525-6
McKinnon, K. A., and Simpson, I. R. (2022). How unexpected was the 2021 Pacific northwest heatwave? Geophys. Res. Lett. 49:e2022GL100380. doi: 10.1029/2022GL100380
Mengel, M., Treu, S., Lange, S., and Frieler, K. (2021). ATTRICI v1.1—counterfactual climate for impact attribution. Geosci. Model Dev. 14, 5269–5284. doi: 10.5194/gmd-14-5269-2021
Miralles, O., and Davison, A. C. (2023). Timing and spatial selection bias in rapid extreme event attribution. Weather Clim. Extrem. 41:100584. doi: 10.1016/j.wace.2023.100584
Mishra, A. N., Maraun, D., Knevels, R., Truhetz, H., Brenning, A., and Proske, H. (2023). Climate change amplified the 2009 extreme landslide event in Austria. Clim. Change 176:124. doi: 10.1007/s10584-023-03593-2
Mitchell, D. (2016). Human influences on heat-related health indicators during the 2015 Egyptian heat wave. Bull. Am. Meteorol. Soc. 97, S70–S74. doi: 10.1175/BAMS-D-16-0132.1
Nascimento, F., Bourguignon, M., and Jeremias, L. (2016). Extended generalized extreme value distribution with applications in environmental data. Hacet. J. Math. Stat. 45, 1847–1864. doi: 10.15672/HJMS.20159514081
National Academies of Sciences, Engineering, and Medicine (NASEM) (2016). Attribution of extreme weather events in the context of climate change. Washington, DC: The National Academies Press.
Naveau, P., Hannart, A., and Ribes, A. (2020). Statistical methods for extreme event attribution in climate science. Annu. Rev. Stat. Appl. 7, 89–110. doi: 10.1146/annurev-statistics-031219-041314
Nguyen, P. L., Bador, M., Alexander, L. V., Lane, T. P., and Ngo-Duc, T. (2022). More intense daily precipitation in CORDEX-SEA regional climate models than their forcing global climate models over Southeast Asia. Int. J. Climatol. 42, 6537–6561. doi: 10.1002/joc.7619
Noy, I., Stone, D., and Uher, T. (2024). Extreme events impact attribution: a state of the art. Cell Rep. Sustain. 1:100101. doi: 10.1016/j.crsus.2024.100101
Noy, I., Wehner, M., Stone, D., Rosier, S., Frame, D., Lawal, K. A., et al. (2023). Event attribution is ready to inform loss and damage negotiations. Nat. Clim. Change 13, 1279–1281. doi: 10.1038/s41558-023-01865-4
Osaka, S., and Bellamy, R. (2020). Natural variability or climate change? Stakeholder and citizen perceptions of extreme event attribution. Glob. Environ. Change 62:102070. doi: 10.1016/j.gloenvcha.2020.102070
Osaka, S., Painter, J., Walton, P., and Halperin, A. (2020). Media representation of extreme event attribution: a case study of the 2011–17 California drought. Weather Clim. Soc. 12, 847–862. doi: 10.1175/WCAS-D-19-0050.1
Otto, F. E. L., Kew, S., Philip, S., Stott, P., and van Oldenborgh, G. J. (2022). How to provide useful attribution statements: lessons learned from operationalizing event attribution in Europe. Bull. Am. Meteorol. Soc. 103, S21–S25. doi: 10.1175/BAMS-D-21-0267.1
Otto, F. E. L., Massey, N., van Oldenborgh, G. J., Jones, R. G., and Allen, M. R. (2012). Reconciling two approaches to attribution of the 2010 Russian heat wave. Geophys. Res. Lett. 39:L04702. doi: 10.1029/2011GL050422
Otto, F. E. L., van Oldenborgh, G. J., Eden, J., Stott, P. A., Karoly, D. J., and Allen, M. R. (2016). The attribution question. Nat. Clim. Change 6, 813–816. doi: 10.1038/nclimate3089
Paciorek, C. J., Stone, D. A., and Wehner, M. F. (2018). Quantifying statistical uncertainty in the attribution of human influence on severe weather. Weather Clim. Extrem. 20, 69–80. doi: 10.1016/j.wace.2018.01.002
Pall, P., Aina, T., Stone, D. A., Stott, P. A., Nozawa, T., Hilberts, A. G., et al. (2011). Anthropogenic greenhouse gas contribution to flood risk in England and Wales in autumn 2000. Nature 470, 382–385. doi: 10.1038/nature09762
Parker, W. S. (2009). Confirmation and adequacy-for-purpose in climate modelling. Aristot. Soc. Suppl. Vol. 83, 233–249. doi: 10.1111/j.1467-8349.2009.00180.x
Parker, W. S. (2020). Model evaluation: an adequacy-for-purpose view. Philos. Sci. 87, 457–477. doi: 10.1086/708691
Parker, H. R., Cornforth, R. J., Suarez, P., Allen, M. R., Boyd, E., James, R., et al. (2016). Using a game to engage stakeholders in extreme event attribution science. Int. J. Disaster Risk Sci. 7, 353–365. doi: 10.1007/s13753-016-0105-6
Patricola, C. M., and Wehner, M. F. (2018). Anthropogenic influences on major tropical cyclone events. Nature 563, 339–346. doi: 10.1038/s41586-018-0673-2
Patterson, M. (2023). North-West Europe hottest days are warming twice as fast as mean summer days. Geophys. Res. Lett. 50:e2023GL102757. doi: 10.1029/2023GL102757
Perkins-Kirkpatrick, S. E., and Gibson, P. B. (2017). Changes in regional heatwave characteristics as a function of increasing global temperature. Sci. Rep. 7:12256. doi: 10.1038/s41598-017-12520-2
Perkins-Kirkpatrick, S. E., Stone, D. A., Mitchell, D. M., Rosier, S., King, A. D., Lo, Y. T. E., et al. (2022). On the attribution of the impacts of extreme weather events to anthropogenic climate change. Environ. Res. Lett. 17:024009. doi: 10.1088/1748-9326/ac44c8
Pfahl, S., O’Gorman, P. A., and Fischer, E. M. (2017). Understanding the regional pattern of projected future changes in extreme precipitation. Nat. Clim. Change 7, 423–427. doi: 10.1038/nclimate3287
Philip, S. Y., Kew, S. F., van Oldenborgh, G. J., Anslow, F. S., Seneviratne, S. I., Vautard, R., et al. (2022). Rapid attribution analysis of the extraordinary heat wave on the Pacific coast of the US and Canada in June 2021. Earth Syst. Dynam. 13, 1689–1713. doi: 10.5194/esd-13-1689-2022
Philip, S., Kew, S., van Oldenborgh, G. J., Otto, F., Vautard, R., van der Wiel, K., et al. (2020). A protocol for probabilistic extreme event attribution analyses. Adv. Stat. Clim. Meteorol. Oceanogr. 6, 177–203. doi: 10.5194/ascmo-6-177-2020
Pichelli, E., Coppola, E., Sobolowski, S., Ban, N., Giorgi, F., Stocchi, P., et al. (2021). The first multi-model ensemble of regional climate simulations a kilometer-scale resolution part 2: historical and future simulations of precipitation. Clim. Dyn. 56, 3581–3602. doi: 10.1007/s00382-021-05657-4
Pietroiusti, R., Vanderkelen, I., Otto, F. E. L., Barnes, C., Temple, L., Akurut, M., et al. (2024). Possible role of anthropogenic climate change in the record-breaking 2020 Lake Victoria levels and floods. Earth Syst. Dynam. 15, 225–264. doi: 10.5194/esd-15-225-2024
Qian, C., Ye, Y., Bevacqua, E., and Zscheischler, J. (2023). Human influences on spatially compounding flooding and heatwave events in China and future increasing risks. Weather Clim. Extrem. 42:100616. doi: 10.1016/j.wace.2023.100616
Ragone, F., and Bouchet, F. (2021). Rare event algorithm study of extreme warm summers and heatwaves over Europe. Geophys. Res. Lett. 48:e2020GL091197. doi: 10.1029/2020GL091197
Reed, K. A., Stansfield, A. M., Wehner, M. F., and Zarzycki, C. M. (2020). Forecasted attribution of the human influence on hurricane Florence. Sci. Adv. 6:eaaw 9253. doi: 10.1126/sciadv.aaw9253
Ribes, A., Thao, S., and Cattiaux, J. (2020). Describing the relationship between a weather event and climate change: a new statistical approach. J. Clim. 33, 6297–6314. doi: 10.1175/JCLI-D-19-0217.1
Risbey, J. S., Irving, D. B., Squire, D. T., Matear, R. J., Monselesan, D. P., Pook, M. J., et al. (2023). A large ensemble illustration of how record-shattering heat records can endure. Environ. Res.: Clim. 2:035003. doi: 10.1088/2752-5295/acd714
Risser, M. D., Collins, W. D., Wehner, M. F., O’Brien, T. A., Huang, H., and Ullrich, P. A. (2024). Anthropogenic aerosols mask increases in US rainfall by greenhouse gases. Nat. Commun. 15:1318. doi: 10.1038/s41467-024-45504-8
Risser, M., Collins, W., Wehner, M., O'Brien, T., Paciorek, C., O'Brien, J. P., et al. (2021, 2023). A framework for detection and attribution of regional precipitation change: Application to the United States historical record. Clim. Dyn. 60, 705–741. doi: 10.1007/s00382-022-06321-1
Risser, M. D., and Wehner, M. F. (2017). Attributable human-induced changes in the likelihood and magnitude of the observed extreme precipitation during Hurricane Harvey. Geophys. Res. Lett. 44, 12–457. doi: 10.1002/2017GL075888
Robin, Y., and Ribes, A. (2020). Nonstationary extreme value analysis for event attribution combining climate models and observations. Adv. Stat. Climatol. Meteorol. Oceanogr. 6, 205–221. doi: 10.5194/ascmo-6-205-2020
Sanders, N. E., and Hendricks, R.. (2023). AI could reshape climate communication. Available at: https://eos.org/opinions/ai-could-reshape-climate-communication
Schaller, N., Kay, A. L., Lamb, R., Massey, N. R., van Oldenborgh, G. J., Otto, F. E. L., et al. (2016). The human influence on climate in the winter 2013/2014 floods in southern England. Nat. Clim. Change 6, 627–634. doi: 10.1038/nclimate2927
Schär, C., Frei, C., Lüthi, D., and Davies, H. C. (1996). Surrogate climate-change scenarios for regional climate models. Geophys. Res. Lett. 23, 669–672. doi: 10.1029/96GL00265
Schär, C., Vidale, P. L., Lüthi, D., Frei, C., Häberli, C., Liniger, M. A., et al. (2004). The role of increasing temperature variability in European summer heatwaves. Nature 427, 332–336. doi: 10.1038/nature02300
Schiemann, R., Athanasiadis, P., Barriopedro, D., Doblas-Reyes, F., Lohmann, K., Roberts, M. J., et al. (2020). Northern Hemisphere blocking simulation in current climate models: evaluating progress from the Climate Model Intercomparison Project Phase 5 to 6 and sensitivity to resolution. Weather Clim. Dyn. 1, 277–292. doi: 10.5194/wcd-1-277-2020
Schumacher, D. L., Singh, J., Hauser, M., Fischer, E. M., Wild, M., and Seneviratne, S. I. (2024). Exacerbated summer European warming not captured by climate models neglecting long-term aerosol changes. Commun. Earth Environ. 5:182. doi: 10.1038/s43247-024-01332-8
Seneviratne, S. I., Corti, T., Davin, E. L., Hirschi, M., Jaeger, E. B., Lehner, I., et al. (2010). Investigating soil moisture–climate interactions in a changing climate: a review. Earth Sci. Rev. 99, 125–161. doi: 10.1016/j.earscirev.2010.02.004
Seneviratne, S. I., Zhang, X., Adnan, M., Badi, W., Dereczynski, C., Di Luca, A., et al. (2021). “Weather and climate extreme events in a changing climate” in Climate Change 2021: The Physical Science Basis. Contribution of Working Group I to the Sixth Assessment Report of the Intergovernmental Panel on Climate Change. eds. V. Masson-Delmotte, P. Zhai, A. Pirani, S. L. Connors, C. Péan, and S. Berger, et al. (Cambridge: Cambridge University Press).
Shepherd, T. G. (2014). Atmospheric circulation as a source of uncertainty in climate change projections. Nat. Geosci. 7, 703–708. doi: 10.1038/ngeo2253
Shepherd, T. G., Boyd, E., Calel, R. A., Chapman, S. C., Dessai, S., Dima-West, I. M., et al. (2018). Storylines: an alternative approach to representing uncertainty in physical aspects of climate change. Clim. Change 151, 555–571. doi: 10.1007/s10584-018-2317-9
Silberner, J. (2021). Heat wave causes hundreds of deaths and hospitalisations in Pacific northwest. BMJ 374:n1696. doi: 10.1136/bmj.n1696
Sillmann, J., Croci-Maspoli, M., Kallache, M., and Katz, R. W. (2011). Extreme cold winter temperatures in Europe under the influence of North Atlantic atmospheric blocking. J. Clim. 24, 5899–5913. doi: 10.1175/2011JCLI4075.1
Simpson, C. H., Brousse, O., Ebi, K. L., and Heaviside, C. (2023). Commonly used indices disagree about the effect of moisture on heat stress. npj Clim. Atmos. Sci. 6:78. doi: 10.1038/s41612-023-00408-0
Singh, J., Sippel, S., and Fischer, E. M. (2023). Circulation dampened heat extremes intensification over the Midwest USA and amplified over Western Europe. Commun. Earth Environ. 4:432. doi: 10.1038/s43247-023-01096-7
Sippel, S., Fischer, E. M., Scherrer, S. C., Meinshausen, N., and Knutti, R. (2020). Late 1980s abrupt cold season temperature change in Europe consistent with circulation variability and long-term warming. Environ. Res. Lett. 15:094056. doi: 10.1088/1748-9326/ab86f2
Sippel, S., Forkel, M., Rammig, A., Thonicke, K., Flach, M., Heimann, M., et al. (2017). Contrasting and interacting changes in simulated spring and summer carbon cycle extremes in European ecosystems. Environ. Res. Lett. 12:075006. doi: 10.1088/1748-9326/aa7398
Slater, R., Freychet, N., and Hegerl, G. (2021). Substantial changes in the probability of future annual temperature extremes. Atmos. Sci. Lett. 22:e 1061. doi: 10.1002/asl.1061
Slivinski, L. C., Compo, G. P., Whitaker, J. S., Sardeshmukh, P. D., Giese, B. S., McColl, C., et al. (2019). Towards a more reliable historical reanalysis: improvements for version 3 of the twentieth century reanalysis system. Q. J. R. Meteorol. Soc. 145, 2876–2908. doi: 10.1002/qj.3598
Smiley, K. T., Noy, I., Wehner, M. F., Frame, D., Sampson, C. C., and Wing, O. E. (2022). Social inequalities in climate change-attributed impacts of Hurricane Harvey. Nat. Commun. 13:3418. doi: 10.1038/s41467-022-31056-2
Stott, P. (2016). How climate change affects extreme weather events. Science 352, 1517–1518. doi: 10.1126/science.aaf7271
Stott, P. A., Allen, M., Christidis, N., Dole, R. M., Hoerling, M., Huntingford, C., et al. (2013). “Attribution of weather and climate-related events” in Climate science for serving society. eds. G. Asrar and J. Hurrell (Dordrecht: Springer).
Stott, P. A., Christidis, N., Otto, F. E., Sun, Y., Vanderlinden, J.-P., van Oldenborgh, G. J., et al. (2016). Attribution of extreme weather and climate-related events. Wiley Interdiscip. Rev. Clim. Change 7, 23–41
Stott, P. A., Stone, D. A., and Allen, M. R. (2004). Human contribution to the European heatwave of 2003. Nature 432, 610–614. doi: 10.1038/nature03089
Stuart-Smith, R., Vicedo-Cabrera, A., Li, S., Otto, F., Belesova, K., Haines, A., et al. (2024). Quantifying heat-related mortality attributable to human-induced climate change. doi: 10.21203/rs.3.rs-2702337/v2
Suarez-Gutierrez, L., Müller, W. A., Li, C., and Marotzke, J. (2020). Dynamical and thermodynamical drivers of variability in European summer heat extremes. Clim. Dyn. 54, 4351–4366. doi: 10.1007/s00382-020-05233-2
Sun, X., and Lall, U. (2015). Spatially coherent trends of annual maximum daily precipitation in the United States. Geophys. Res. Lett. 42, 9781–9789. doi: 10.1002/2015GL066483
Sun, Q., Miao, C., Duan, Q., Ashouri, H., Sorooshian, S., and Hsu, K. L. (2018). A review of global precipitation data sets: data sources, estimation, and intercomparisons. Rev. Geophys. 56, 79–107. doi: 10.1002/2017RG000574
Tett, S.. (2020). Attributing Chinese hydrological extreme events attributing Chinese hydrological extreme events. 22nd EGU General Assembly. 19484.
Thiery, W., Visser, A. J., Fischer, E. M., Hauser, M., Hirsch, A. L., Lawrence, D. M., et al. (2020). Warming of hot extremes alleviated by expanding irrigation. Nat. Commun. 11:290. doi: 10.1038/s41467-019-14075-4
Thompson, V., Dunstone, N. J., Scaife, A. A., Smith, D. M., Slingo, J. M., Brown, S., et al. (2017). High risk of unprecedented UK rainfall in the current climate. Nat. Commun. 8, 1–6. doi: 10.1038/s41467-017-00275-3
Thompson, V., Mitchell, D., Hegerl, G. C., Collins, M., Leach, N. J., and Slingo, J. M. (2023). The most at-risk regions in the world for high-impact heatwaves. Nat. Commun. 14, 2152–2158. doi: 10.1038/s41467-023-37554-1
Tradowsky, J. S., Philip, S. Y., Kreienkamp, F., Kew, S. F., Lorenz, P., Arrighi, J., et al. (2023). Attribution of the heavy rainfall events leading to severe flooding in western Europe during July 2021. Clim. Change 176:90. doi: 10.1007/s10584-023-03502-7
Trenberth, K. E., Fasullo, J. T., and Shepherd, T. G. (2015). Attribution of climate extreme events. Nat. Clim. Change 5, 725–730. doi: 10.1038/nclimate2657
Trewin, B. (2010). Exposure, instrumentation, and observing practice effects on land temperature measurements. Wiley Interdiscip. Rev. Clim. Change 1, 490–506. doi: 10.1002/WCC.46
van der Wiel, K., Selten, F. M., Bintanja, R., Blackport, R., and Screen, J. A. (2020). Ensemble climate-impact modelling: extreme impacts from moderate meteorological conditions. Environ. Res. Lett. 15:034050. doi: 10.1088/1748-9326/ab7668
van Garderen, L., Feser, F., and Shepherd, T. G. (2020). A methodology for attributing the role of climate change in extreme events: a global spectrally nudged storyline. Nat. Hazards Earth Syst. Sci. 21, 171–186. doi: 10.5194/nhess-21-171-2021
van Oldenborgh, G. J., Krikken, F., Lewis, S., Leach, N. J., Lehner, F., Saunders, K. R., et al. (2021a). Attribution of the Australian bushfire risk to anthropogenic climate change. Nat. Hazards Earth Syst. Sci. 21, 941–960. doi: 10.5194/nhess-21-941-2021
van Oldenborgh, G. J., van der Wiel, K., Kew, S., Philip, S., Otto, F. E. L., Vautard, R., et al. (2021b). Pathways and pitfalls in extreme event attribution. Clim. Change 166:13. doi: 10.1007/s10584-021-03071-7
van Oldenborgh, G. J., Wehner, M. F., Vautard, R., Otto, F. E., Seneviratne, S. I., Stott, P. A., et al. (2022). Attributing and projecting heatwaves is hard: we can do better. Earth’s Future 10:e2021EF002271. doi: 10.1029/2021EF002271
Vautard, R., Barnes, C., Philip, S., Kew, S., Pinto, I., and Otto, F. E. (2024). Heat extremes linearly shift with global warming, with frequency doubling per decade since 1979. Environ. Res. Lett. 19:094033. doi: 10.1088/1748-9326/ad63be
Vautard, R., Yiou, P., Otto, F., Stott, P., Christidis, N., van Oldenborgh, G. J., et al. (2016). Attribution of human-induced dynamical and thermodynamical contributions in extreme weather events. Environ. Res. Lett. 11:114009. doi: 10.1088/1748-9326/11/11/114009
Verschuur, J., Li, S., Wolski, P., and Otto, F. E. L. (2021). Climate change as a driver of food insecurity in the 2007 Lesotho-South Africa drought. Sci. Rep. 11:3852. doi: 10.1038/s41598-021-83375-x
Vicedo-Cabrera, A. M., de Schrijver, E., Schumacher, D. L., Ragettli, M. S., Fischer, E. M., and Seneviratne, S. I. (2023). The footprint of human-induced climate change on heat-related deaths in the summer of 2022 in Switzerland. Environ. Res. Lett. 18:074037. doi: 10.1088/1748-9326/ace0d0
Vicedo-Cabrera, A. M., Scovronick, N., Sera, F., Royé, D., Schneider, R., Tobias, A., et al. (2021). The burden of heat-related mortality attributable to recent human-induced climate change. Nat. Clim. Change 11, 492–500. doi: 10.1038/s41558-021-01058-x
Villalobos-Herrera, R., Bevacqua, E., Ribeiro, A. F. S., Auld, G., Crocetti, L., Mircheva, B., et al. (2021). Towards a compound-event-oriented climate model evaluation: a decomposition of the underlying biases in multivariate fire and heat stress hazards. Nat. Hazards Earth Syst. Sci. 21, 1867–1885. doi: 10.5194/nhess-21-1867-2021
Viney, N. R., and Bates, B. C. (2004). It never rains on Sunday: the prevalence and implications of untagged multi-day rainfall accumulations in the Australian high quality data set. Int. J. Climatol. 24, 1171–1192. doi: 10.1002/joc.1053
Vogel, M. M., Zscheischler, J., and Seneviratne, S. I. (2018). Varying soil moisture–atmosphere feedbacks explain divergent temperature extremes and precipitation projections in Central Europe. Earth Syst. Dynam. 9, 1107–1125. doi: 10.5194/esd-9-1107-2018
Wahl, T., Jain, S., Bender, J., Meyers, S. D., and Luther, M. E. (2015). Increasing risk of compound flooding from storm surge and rainfall for major US cities. Nat. Clim. Change 5, 1093–1097. doi: 10.1038/nclimate2736
Wehner, M., and Sampson, C. (2021). Attributable human-induced changes in the magnitude of flooding in the Houston, Texas region during Hurricane Harvey. Clim. Change 166:20. doi: 10.1007/s10584-021-03114-z
Wehner, M., Stone, D., Krishnan, H., Achuta Rao, K., and Castillo, F. (2016). The deadly combination of heat and humidity in India and Pakistan in summer 2015. Bull. Am. Meteorol. Soc. 97, S81–S86. doi: 10.1175/BAMS-D-16-0145.1
WMO Unified Data Policy. (2022). Available at: https://library.wmo.int/viewer/58009?medianame=WMO_Unified_Data_Policy_brochure_en_#page=2&viewer=picture&o=&n=0&q=
Yule, E. L., Hegerl, G., Schurer, A., and Hawkins, E. (2023). Using early extremes to place the 2022 UK heat waves into historical context. Atmos. Sci. Lett. 24:e1159. doi: 10.1002/ASL.1159
Zachariah, M., Kumari, S., Mondal, A., Haustein, K., and Otto, F. E. (2023a). Attribution of the 2015 drought in Marathwada, India from a multivariate perspective. Weather Clim. Extrem. 39:100546. doi: 10.1016/j.wace.2022.100546
Zachariah, M., Vautard, R., Chandrasekaran, R., Chaithra, S. T., Kimutai, J., Arulalan, T., et al. (2023b). Extreme humid heat in South Asia in April 2023, largely driven by climate change, detrimental to vulnerable and disadvantaged communities. South Asia: World Weather Attribution.
Zeder, J., and Fischer, E. M. (2023). Quantifying the statistical dependence of mid-latitude heatwave intensity and likelihood on prevalent physical drivers and climate change. Adv. Stat. Climatol. Meteorol. Oceanogr. 9, 83–102. doi: 10.5194/ascmo-9-83-2023
Zeder, J., Sippel, S., Pasche, O. C., Engelke, S., and Fischer, E. M. (2023). The effect of a short observational record on the statistics of temperature extremes. Geophys. Res. Lett. 50:e2023GL104090. doi: 10.1029/2023GL104090
Zhang, L., Risser, M. D., Wehner, M. F., and O’Brien, T. A., (2024). Explaining the unexplainable: leveraging extremal dependence to characterize the 2021 Pacific Northwest heatwave. arXiv. Available at: https://doi.org/10.48550/arXiv.2307.03688. [Epub ahead of preprnt]
Zscheischler, J., Fischer, E. M., and Lange, S. (2019). The effect of univariate bias adjustment on multivariate hazard estimates. Earth Syst. Dynam. 10, 31–43. doi: 10.5194/esd-10-31-2019
Zscheischler, J., and Lehner, F. (2022). Attributing compound events to anthropogenic climate change. Bull. Am. Meteorol. Soc. 103, E936–E953. doi: 10.1175/BAMS-D-21-0116.1
Zscheischler, J., Martius, O., Westra, S., Bevacqua, E., Raymond, C., Horton, R. M., et al. (2020). A typology of compound weather and climate events. Nat. Rev. Earth Environ. 1, 333–347. doi: 10.1038/s43017-020-0060-z
Zscheischler, J., Naveau, P., Martius, O., Engelke, S., and Raible, C. C. (2021). Evaluating the dependence structure of compound precipitation and wind speed extremes. Earth Syst. Dynam. 12, 1–16. doi: 10.5194/esd-12-1-2021
Zscheischler, J., and Seneviratne, S. I. (2017). Dependence of drivers affects risks associated with compound events. Sci. Adv. 3:e1700263. doi: 10.1126/sciadv.1700263
Keywords: attribution, extreme event attribution, climate change, climate models (regional and global), climate observations, impact attribution, climate science communication
Citation: Perkins-Kirkpatrick SE, Alexander LV, King AD, Kew SF, Philip SY, Barnes C, Maraun D, Stuart-Smith RF, Jézéquel A, Bevacqua E, Burgess S, Fischer E, Hegerl GC, Kimutai J, Koren G, Lawal KA, Min S-K, New M, Odoulami RC, Patricola CM, Pinto I, Ribes A, Shaw TA, Thiery W, Trewin B, Vautard R, Wehner M and Zscheischler J (2024) Frontiers in attributing climate extremes and associated impacts. Front. Clim. 6:1455023. doi: 10.3389/fclim.2024.1455023
Edited by:
Swagata Payra, Birla Institute of Technology, Mesra, IndiaReviewed by:
Satyaban B. Ratna, India Meteorological Department, IndiaMohan Kumar Das, National Oceanographic and Maritime Institute (NOAMI), Bangladesh
Abhijit Sarkar, Indian Institute of Tropical Meteorology (IITM), India
Copyright © 2024 Perkins-Kirkpatrick, Alexander, King, Kew, Philip, Barnes, Maraun, Stuart-Smith, Jézéquel, Bevacqua, Burgess, Fischer, Hegerl, Kimutai, Koren, Lawal, Min, New, Odoulami, Patricola, Pinto, Ribes, Shaw, Thiery, Trewin, Vautard, Wehner and Zscheischler. This is an open-access article distributed under the terms of the Creative Commons Attribution License (CC BY). The use, distribution or reproduction in other forums is permitted, provided the original author(s) and the copyright owner(s) are credited and that the original publication in this journal is cited, in accordance with accepted academic practice. No use, distribution or reproduction is permitted which does not comply with these terms.
*Correspondence: Sarah E. Perkins-Kirkpatrick, c2FyYWgua2lya3BhdHJpY2tAYW51LmVkdS5hdQ==