- 1Departement of Communication, Information, Systems and Sensors, Royal Military Academy, Brussels, Belgium
- 2Departement of Mathematics, Royal Military Academy, Brussels, Belgium
Whether it is to initially deploy a network or to restore the connectivity in a partitioned one, the question of the optimal Relay Node (RN) placement arises. This problem is already challenging when considering a static homogeneous network. However, diversity in transmission parameters within the network can induce diversity in transmission ranges, imposing the consideration of heterogeneity in the network. Furthermore, if the nodes are moving, the RN placement scheme must manage a smooth repositioning of the RNs without any large jumps or major restructuring. This paper introduces an effective strategy for deploying the minimum number of RNs in order to restore the connectivity between the nodes of a partitioned heterogeneous network. Through the statistical analysis of results from numerous randomly generated scenarios, the proposed Barycenter-focused Relay nodes placement for Heterogeneous wireless Networks (BRHEN) algorithm is shown to be an improvement on other similar approaches in terms of the number of RNs and the latency. Additionally, BRHEN exhibits stability in the positions and number of RNs when small displacements are applied to the Initial Nodes (INs). This characteristic makes this method suitable for scenarios with moving INs.
1 Introduction
Modern wireless networks are inherently heterogeneous, incorporating diverse types of nodes with varying capabilities, communication ranges, and energy constraints. This heterogeneity introduces significant challenges in maintaining network connectivity, optimizing performance, and ensuring efficient data transmission. A critical aspect of network management in such environments is the strategic placement of Relay Nodes (RNs), which serve to improve connectivity, extend network coverage, and enhance overall reliability.
Effective relay node placement becomes particularly crucial in scenarios where network partitions arise due to node failures, mobility, or energy depletion. Conventional relay placement methods often assume homogeneous network conditions, failing to account for the diverse characteristics of nodes present in real-world deployments. However, in heterogeneous networks—such as those found in Internet of Things (IoT) ecosystems, heterogeneous cellular networks (HetNets), and next-generation wireless communication systems (Parihar et al. 2024a; Parihar et al. 2024b; Swami et al., 2022) — a homogeneous approach to RN placement is insufficient. Instead, adaptive placement strategies that consider node diversity, transmission power, and network topology dynamics are essential for maintaining seamless connectivity and optimizing performance.
Given these challenges, an effective relay node placement method that explicitly accounts for network heterogeneity is essential. Existing approaches often fail to fully address the impact of node diversity and mobility on RN deployment strategies, highlighting the need for more adaptive solutions. In the following, we review the current state of research on relay node placement, by examining the methodologies proposed to handle network heterogeneity, and by identifying key gaps that motivate the need for improved strategies.
A wide range of scenarios requiring RN deployment can be found in the literature. For example, in Ladosz et al. (2018) they are used to reconnect segmented networks or isolated users, as well as to build a relay network for a search and rescue mission in Yanmaz (2021) or in a disaster area in ur Rahman et al. (2018). In Wang et al. (2019), Yang et al. (2016) and Grönkvist et al. (2022), they are also considered to enhance wireless traffic in cellular networks and to enable a higher frequency network in military and tactical situations. Other examples of RN uses can be found in Wu et al. (2017), in which the question of energy efficiency is studied in a scenario in which a mobile node is used to relay the data from several mobile sensing robots; and in Cao et al. (2016) where they discuss a relay selection scheme to improve the end-to-end symbol error rate between two nodes. These RNs can be ground nodes, Unmanned Aerial Vehicles (UAVs) or even Intelligent Reflecting Surfaces (IRSs) as proposed in Swami and Bhatia (2021). Nonetheless, the challenge of optimally placing these relays in order to minimize their number, maximize throughput, and, in the event of a non-static network, minimize RN movements and topology changes persists.
To minimize the number of relays, Yanmaz (2021) used the Steiner Tree Problem with Minimum number of Steiner Points and the Bounded Edge-Length (STP-MSPBEL) method of Lin and Xue (1999), Cheng et al. (2008). Given a set of points and a maximum edge size (line segment between two points), the Steiner Tree Problem (STP) is the placement of the minimum number of additional points so that there is a path from each point to the others via edges. This problem has been shown to be NP-Hard by Lin and Xue (1999). STP-MSPBEL is a well-known approach to solving the STP, with a performance ratio of 5 — this indicates that the result is at most 5 times the optimal solution, or put differently, at most 5 times the minimum number of additional points. Since the development of that method, new approaches have been developed with a lower performance ratio and better results. The best-known scheme with a proved good performance ratio to date is the k-restricted Loss-Contracting Algorithm (k-LCA), which is polynomial in time and has a performance ratio of
These methods consider the network to be homogeneous, i.e., all the nodes have the same range. However, this may not be the case, e.g., in modern scenarios combining emerging technologies such as in (Parihar et al., 2024a, Parihar et al., 2024b; Swami et al., 2022), or in a simple scenario where a Wireless Sensor Network (WSN) is segmented and RNs are deployed to restore the connectivity between the segments as in (Lee and Younis, 2010a; Lee and Younis, 2010b; Lee and Younis, 2010c), the RNs may have a different range than the initial nodes (INs). A third example is a tactical scenario in which some units are limited to handheld radios with a power of 5 W, whil others have a vehicular support and radio with a power of 10 W and one of 20 W. If units are out of range, relays must be deployed to connect the network (see Figure 1). A heterogeneous network with three different ranges is to be considered for the INs, in addition to the relay range.
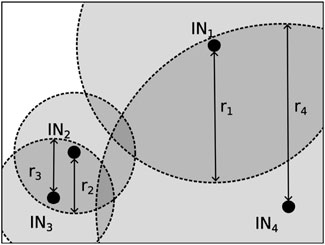
Figure 1. Schematic view of a partitioned heterogeneous network where
Some methods have considered heterogeneity to some extent: MST-1tRN (Minimum spanning tree based approximation algorithm for single-Tiered Relay Node placement) Lloyd and Xue (2007), NAP Liu et al. (2019), and Xie et al. (2020) using the Adaptive Whale Optimization (AWO) method account for transmission range differences between INs and RNs. Going further, Han et al. (2010) and Deyab et al. (2011) introduce heterogeneity in the set of INs and even in the set of RNs for the latter.
The STP is a viewpoint where the nodes remain stationary. A scenario with moving nodes requires dynamic adaptability. A straightforward approach is to take each moment (separated by an arbitrary time
The first major contribution of this paper is a method for a balanced placement of the RN(s) with an associated range
This paper is organized as follows. Section 2 provides a detailed description of the proposed methods and algorithms. The performance is evaluated in Section 3 before discussing the applicability of the BRHEN method in Section 4. The paper ends with the conclusion in Section 5 and the future works in Section 6.
2 The proposed methods
Before we begin to describe and analyze our algorithms, let us state a couple of definitions.
DEFINITION 2.1. A node is a point with an associated range
DEFINITION 2.2. Two nodes are said to be connected or to be neighbors when the distance between them is less than the ranges of both nodes (see Figure 2).
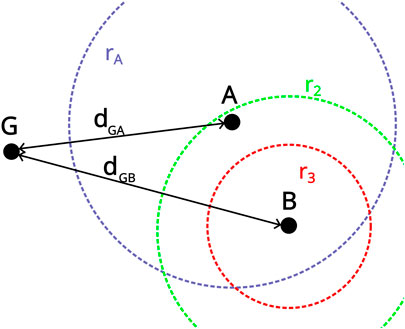
Figure 2. Schematic of two nodes with different ranges illustrated by the dotted circles. If
2.1 Optimized Relay Placement for one heterogeneous link (ORPHe)
A method for placing one or multiple relays with a fixed communication range
The goal is to have a balanced placement. This means that the distance between each successive node is treated in the same way. To do so, we first consider the maximum distance between two successive nodes. It will be equal to the smaller of their ranges. This distance will be reduced by a positive real factor less than or equal to one that depends only on (a) the distance between
In the following, the distance between two points
A method for the case where
Even if it is sufficient to provide the connection between
For our method, and similarly to Han et al. (2010), we define
with
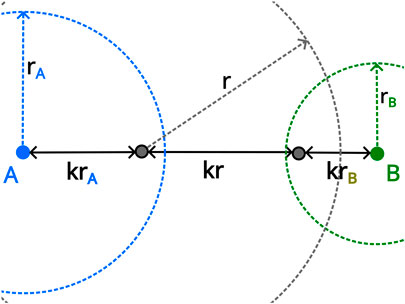
Figure 3. RN (in grey) placement between two INs with different ranges (blue and green) with the ORPHe method. The distance between two nodes is the maximal distance, given by
Equation 1 provides us with an iterative way of positioning RNs to increase Signal-to-Noise Ratio (SNR) across all links, instead of just those between the RNs.
In Algorithm 1, this method is used to realign a set of nodes, or a segment,
2.2 The BRHEN approach
In this section, the concept of segment associated with an IN is used. It refers to the set which includes the IN and all of the relays that are successively placed starting from that node. Two segments will be considered neighbors if at least one node from the first segment is a neighbor of at least one node from the second segment.
The proposed BRHEN approach is a heuristic method for heterogeneous networks that aims to connect all INs using the minimum number of relays. After a first initialization, BRHEN operates in rounds. A round begins with a search for neighbors, followed by a relocation of the current relays to improve link stability if a neighbor is found. If the segment is not connected, the round concludes with the placement of a new relay. Only when the last two unconnected segments see each other as neighbors does the algorithm exit the loop and returns the complete set of nodes. For reference, the pseudocode is displayed in Algorithm 2.
In the Following, the details of these steps will be unfolded. The set of INs will be referred to as
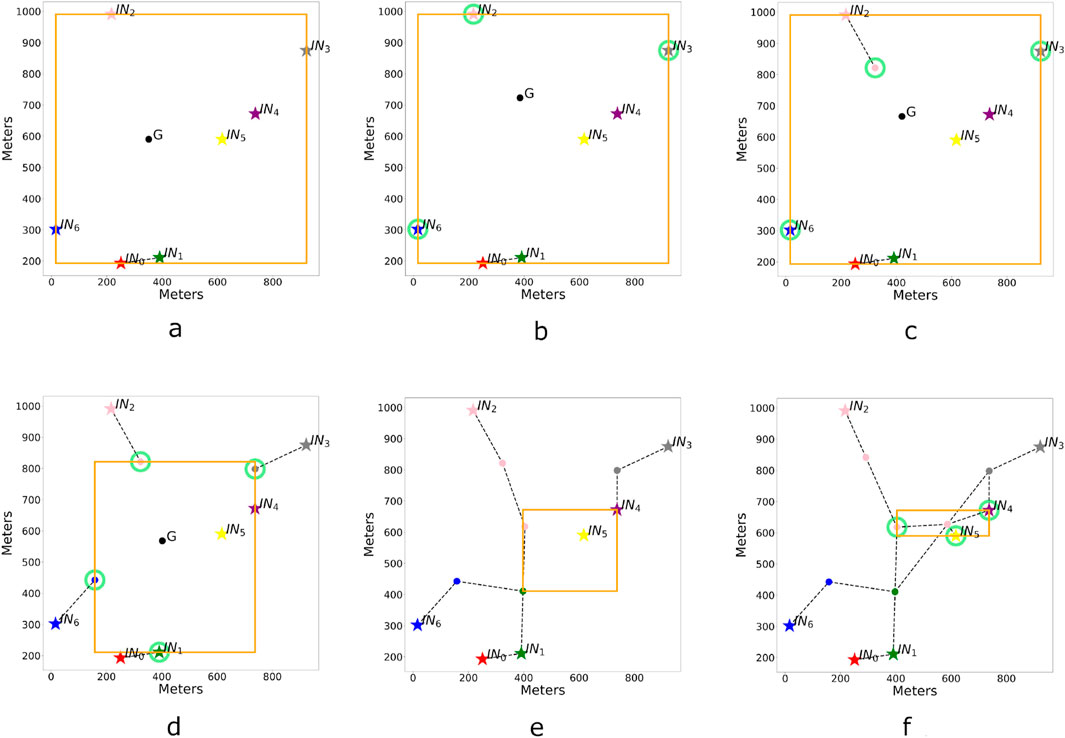
Figure 4. Some steps of the BRHEN algorithm are given above. The dashed lines represent the possibility of connection. And the side of the orange rectangle highlights the considered nodes for relay placement (a) Initialization phase: defining the first BNs.
2.2.1 Initialization
BRHEN assigns a segment with the corresponding index to each initial node, i.e., the segment
The information of the set of
Before using an example for a better understanding of the algorithm, let us first detail some of its key steps.
2.2.2 Key steps
2.2.2.1 Remark
During a round of the BRHEN algorithm, different segments will in turn be the focus as the index
2.2.2.2 Border nodes (BNs) identification
The Border Nodes (BNs) are selected from the nodes that were not previously BNs and belong to an unconnected segment. Within this set of nodes, those having coordinates corresponding to at least one extremum (
2.2.2.3 Barycenter
The BRHEN algorithm uses the (unweighted) barycenter of the eligible nodes to provide a direction towards which the RNs will be placed and to use as reference for selecting the connected segment when necessary. The eligible nodes for the barycenter computation will always be part of
with the sums spanning all the elements to be considered and
2.2.2.4 Finding the neighbors
To determine if a segment is connected to the currently considered segment,
The function that checks for a neighbor in another segment is called
2.2.2.5 Choosing which segment to mark as connected
The simplest approach to determine which segment is marked as connected is to consistently mark the same segment, either the one identified by
The first one occurs when
This strategy provides a way to keep the closest segment to
2.2.2.6 Realignment of the current segment
This part is optional, although it is implemented in order to reduce the distance between the nodes. Using the placement method for one heterogeneous link described above, it is ensured that all the distances between the nodes of the current segment
Here is an example to illustrate how this realignment works in the algorithm. Let
The function called
A similar realignment can be performed on the current segment
2.2.2.7 RN placement
The BRHEN algorithm determines the RN placement direction based on the position of the barycenter of the eligible nodes. The eligible nodes are the
If the distance between the considered node and the barycenter
Otherwise, if
2.2.3 Detailed workings with example
This description of the workings of the BRHEN algorithm uses the scenario presented in Figure 4 as reference.
2.2.3.1 First round
The round starts by identifying the BNs with the
Beginning with the index 0, the search for neighbors of
The next segment is now considered with the index 2,
2.2.3.2 Subsequent rounds
The second round starts with a connected segment and three new nodes as illustrated in Figure 4d. The new BNs are circled in green.
In the third round, only
The fourth round starts with the same set of
2.3 Algorithms analysis
In this subsection, original proofs for the validity of the formulae used in the ORPHe algorithm and the convergence of BRHEN algorithm are given.
2.3.1 Analysis of ORPHe
Property 2.3. The coordinates of a point
with
Proposition 2.4. If
Proof. The proof will be done only for the
Thus, the
with
Remark 2.5. In the following, we will consider the connectivity of the nodes. Since all relays will have the same range
Proposition 2.6. Let
Where
Proof. After placing a node towards
Theorem 2.7. Let
The coordinates can also be expressed using the coordinates of the previous node:
with
Furthermore, all nodes are connected for
Equation 8 give the positions of all the RNs from the position of one of the INs depending on its index, whereas Equation 9 are the iterative formulae that give the position of the subsequent RNs from the previous one (or from the IN for the first RN).
Proof. Firstly, the placement of the
Then, with Equation 5 we can express the coordinates of the node
with
Thus, the distance between the two consecutive nodes
Proposition 2.8. Let us consider the maximum range of each node to be the distance within which a desired channel capacity is ensured and assume two different propagation channels, one being between an IN and a RN and the other one between two RN, with possibly different Path-Loss Exponents (PLEs). Let us also assume that all the RNs have the same range. Finally that the bandwidth used by each node is the same and that we have an Additive White Gaussian Noise (AWGN). Then, the ORPHe placement method is an improvement on a method leaving some distances between nodes to be maximal and is optimal for the special case where the two PLE are identical.
Proof. For an AWGN channel the channel capacity is given by
where
with
Since the idea behind the ranges is that they give the same capacity, substituting
In our case, we have
and we want to reduce the distances between the nodes such that
with
However, from the last equality of Equation 11 and that of Equation 12, we get that
Let us demonstrate that, for the optimal SNR, the equalities are to be conserved when modifying the distances. If they were not, we might have, for example,
The channel capacity of the link between
Because the right-hand side is the result when assuming the equality of the SNR, we can conclude that assuming the equality is optimal.
Three different cases are to be analyzed:
2.3.1.1 Case
Equation 14 becomes
2.3.1.2 Case
Substituting
Given that
since
Comparing the limiting SNR from two previous results to the limiting SNR when nothing is done (Equation 11) gives the following successive inequalities
The first inequality is because
2.3.1.3 Case
Substituting
since
Finally, comparing the limiting SNRs yields
The first inequality is due to
Thus, assuming that the channels have the same LPE for determining a coefficient to reduce the distance between the node as is implicitly done in the ORPHe algorithm, is suboptimal yet enhances, nonetheless, the channel capacity compared to leaving some distances between nodes to be maximal.
2.3.2 Analysis of BRHEN
Property 2.9. The barycenter
In addition, it is equal to an extremum in
Proof. The proof will only be done for the
The lower bound is obtained by substituting all
It is equal if all the
This property guarantees that the new relays placed from the border nodes towards their barycenter remain within the rectangle defined by those nodes. This means that the next border identification, which only takes into account the nodes not considered yet, will have nodes strictly inside the previous rectangle to consider. As a result, the algorithm converges as the rectangles become smaller.
3 Performance evaluation
This section assesses the effectiveness of BRHEN by comparing performance across multiple metrics using simulations. The results show that BRHEN performs better in terms of the number of relays placed when the Initial Nodes (INs) are heterogeneous with respect to the communication ranges and when the relays have yet a different range. The simulations also indicate that BRHEN performs better in terms of average hop count between two INs once the network is established. Therefore, the resulting topography produces a lower data delivery latency. An analysis of the behavior of each method for large-scale networks shows that BRHEN handles large and dense networks better than its counterparts. The data also show that BRHEN is computationally light and that it is stable with regard to the positions and the number of RNs when the INs are randomly displaced by up to 10% of the smallest range. Additionally, in the last two subsections, we studied the effect of the order of consideration of the different segments on the resulting network and the effect of applying the ORPHe method within the BRHEN algorithm.
3.1 Validation simulations
The performance of BRHEN is compared to two baseline approaches. This subsection introduces the simulations setup, the performance metrics and the baseline approaches used for comparisons.
3.1.1 Simulations setup and performance metrics
The simulations consider a partitioned network with INs, with different ranges
• Relative communication ranges of the nodes (
• Number of Initial Nodes
• INs displacement
We assessed the impact of these parameters on the following eight metrics.
• Number of Relay Nodes
• Average Hop count
• Number of rounds
• Mean RN displacement
• Difference between first and best number of RNs
• Smallest SNR improvement
3.1.2 Baseline approaches
• CORP: This algorithm considers a homogeneous set of INs with a communication range
• MST-1tRN (enhanced): The basic idea behind this algorithm is to compute a MST and populate the edges of the MST with the required number of RNs. The MST yields a set of edges connecting the nodes such that there is a path from each node to the others with the minimum total length. In Lloyd and Xue (2007), it is assumed that all nodes have the same range
To ensure that all methods worked under the same initial conditions in the following simulations, cells were used to place the INs. In addition, since CORP works with a unique range, this range must be the smallest; otherwise, some nodes in neighboring cells would not be connected. This is the reason why the size of a cell is defined by
3.2 Comparison of the different methods
In order to test the BRHEN method in a more general way, the scenarios are randomly generated. The INs are placed one by one with a random selection of a unused cell in a square area with a given side length (in number of cells). Each IN is randomly assigned a range
The
In the following results, displayed in Figures 5–10, it should be noted that the great variance in the results is due, to a large extent, to the fact that the scenarios are generated completely randomly. This randomness means that all the INs might be placed as a cluster somewhere in the map or very disparate. Alternatively, every INs could have the smaller (or larger) range. This would influence, for example, the number of RNs of all the methods in a similar way.
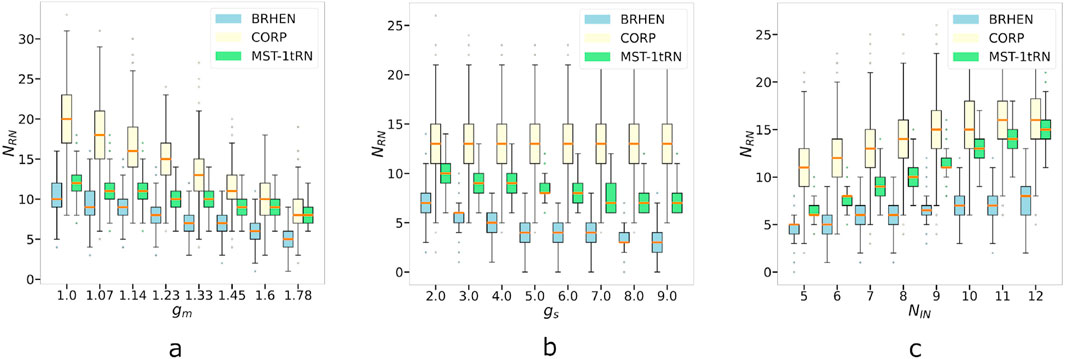
Figure 5. Results comparison regarding the evolution of the number of relays
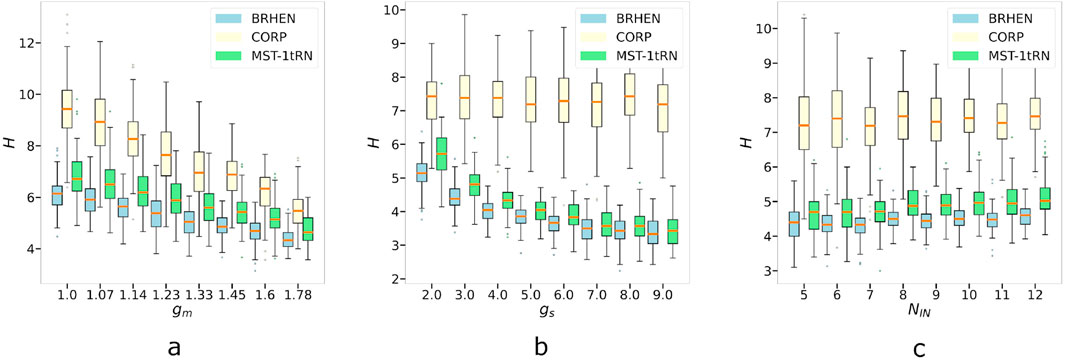
Figure 6. Results comparison regarding the evolution of the average hop count
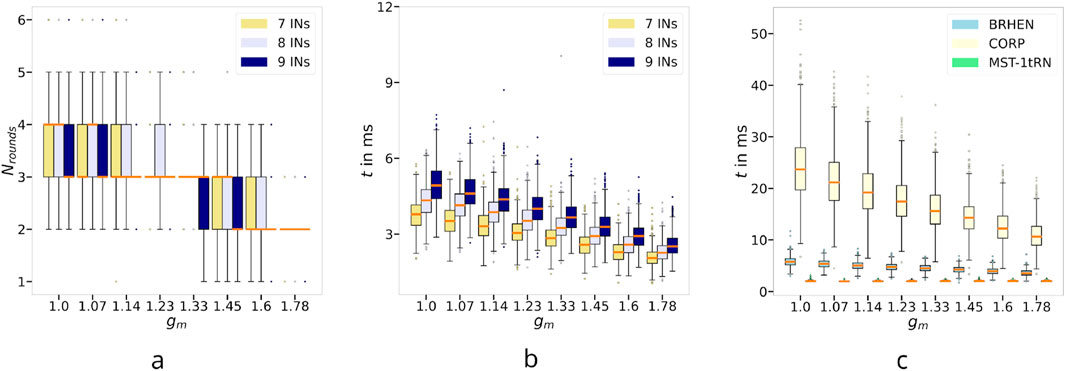
Figure 7. (a) Number of rounds in function of the range for different number of INs. (b) BRHEN computation time in milliseconds (ms) as a function of the range for different number of INs. (c) Comparison of the computing time of BRHEN (blue), CORP (yellow) and MST-1tRN (green).
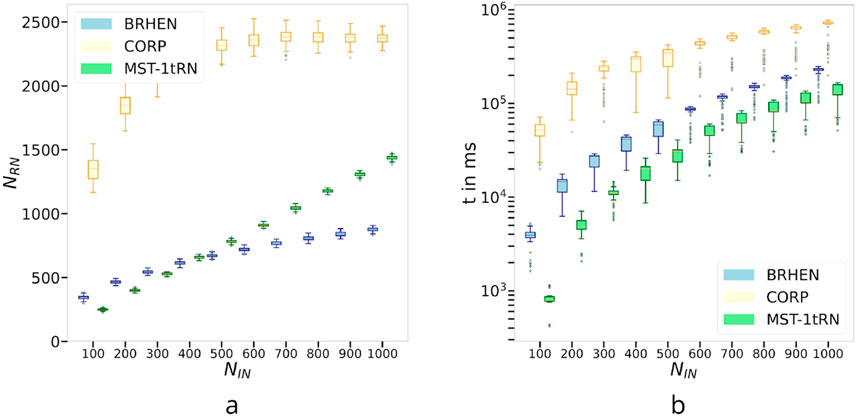
Figure 8. The large-scale behavior of the three considered methods for (a) the number of relay nodes
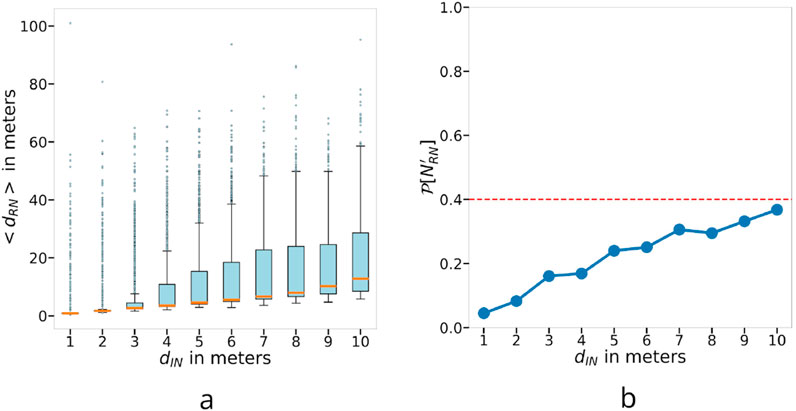
Figure 9. Assessment of the stability of the BRHEN approach. (a) Mean RN displacement in meters as a function of the distance of the IN displacements. (b) Probability that the number of RN
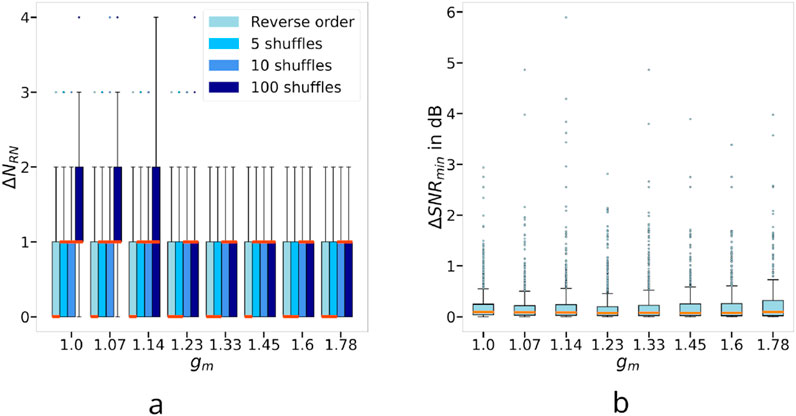
Figure 10. (a) Comparison of the difference in
3.2.1 Number of RNs
3.2.1.1 Behavior for increasing , and
As it is expected with increasing ranges, all the methods see their
3.2.1.2 Behavior for and growing with respect to
Figure 5b shows that
3.2.1.3 Behavior for an increasing number of INs
Finally, Figure 5c depicts the number of RNs required to connect an increasing number of INs. The three methods see their
3.2.2 Average hop count
3.2.2.1 Behavior for increasing , and
Figure 6a shows that the average hop counts follows the same pattern as the number of RNs, which is also expected. The notable difference is that MST-1tRN has a decreasing rate similar to that of BRHEN, which keeps MST-1tRN centered around one additional hop from BRHEN.
3.2.2.2 Behavior for and growing with respect to
It can be seen in Figure 6b that
3.2.2.3 Behavior for an increasing the number of INs
In Figure 6c, the average hop count behaves quite differently from the number of RNs. The results are fairly constant for all the approaches. The results of CORP are again almost double that of BRHEN. MST-1tRN generates a slightly higher average hop count than BRHEN.
3.2.3 Number of rounds
The number of rounds performed by the BRHEN algorithm have been counted for different numbers of INs and as a function of the ranges. Figure 7a shows that BRHEN converges in a few rounds for any number of INs. In addition, the number of rounds is inversely proportional to the ranges of the nodes.
3.2.4 Computation time
Similarly to the number of rounds, the computation times of the BRHEN algorithm have been recorded for different numbers of INs and as a function of the range. In Figure 7b, it can be seen that the computation time decreases with increasing ranges for all INs. It is expected because the number of relays—thus the number calculations for the positions—and the number of rounds decrease with increasing ranges. The higher computation time for larger number of INs is also expected, since the number of relays grows with the number of INs.
For a comparison of the computing time of BRHEN with the other methods, see Figure 7c. It shows that the simplest method, MST-1tRN, is the fastest method. Its time stays mostly constant even if the ranges are growing due to the fact that the heavier computational task is finding the edges of the MST—which does not involve the ranges. BRHEN is heavier than MST-1tRN, but nothing compared to the CORP method, which needs to evaluate 9 cells to select the better one.
3.3 Scalability
Let us now analyze the behavior of the different methods for larger-scale networks. To do so, we multiplied by 10 the dimensions of the square area which gives rise to
The behavior of
The computation time of the three methods behaves similarly as can be observed in Figure 8b. They grow similarly with
3.4 Stability
To manage mobile INs, a RN placement algorithm must produce positions that are stable with respect to the displacements of the INs. In order to assess this stability, for each scenario, the positions of the RNs were compared to the ones of the same scenario after all the INs are moved a certain distance in a randomly chosen direction. The stability is evaluated for different values of the distance, labeled
Two metrics are used for the assessment. The first metric is the average distance between the positions of the initial scenario and the disrupted scenario of each RN—or mean RN displacement. As is easily conceivable, INs moving towards each other or in opposite directions can induce a variation of
For each value of
3.4.1 Results
It can be seen in Figure 9a that the majority of the data spread more as the
Figure 9b gives the probability that
Now, if we add information on the time within which these displacements occur, we obtain a value for the velocity of the INs. The RNs would have to reach their new position in the same amount of time to prevent the network from partitioning momentarily during these displacements. Therefore, we can study the required RN speed from the results presented in Figure 9a. We can see that the median of
In order to give a more concrete example, for the chosen range values of
3.5 Influence of segment consideration order
Upon initiating a scenario, the INs are given a random (or arbitrary) segment number. The BRHEN algorithm uses these numbers to consider the segments in the standard order. However, that order will influence the overall structure of the resulting network, and nothing guarantees that the given order is yielding the best result.
One way to get closer to the best result is to shuffle the segment consideration order a given number of times and take the best of all the outputs. In the following, the output with the least number of RN will be considered the best. We applied this method for 1 shuffle (a simple reversal of the order), 5, 10 and 100 shuffles, using the same parameter
We can see that from a small number of shuffles (1–5) we already get an appreciable probability that one less RN is deployed. For small values of
These results suggest that doing a few runs of the BRHEN algorithm with shuffled segment orderings has a non-negligible probability of resulting in a better RN layout. Since BRHEN is computationally light, it is a reasonable option. However, for relatively large ranges with respect to the area, a representative sample can consist of only a few shuffled runs.
3.6 Use of the ORPHe method and its effects
With Proposition 2.8, we have shown that using the ORPHe method to place RNs between two nodes allowed the overall SNR between the two to be improved. Here we show that when applied as a realignment method within the BHREN algorithm, we obtain a slight SNR improvement within the segment.
To do so, we are using the same simulation setup as in Subsection 3.2 with
with
The data suggest that there is always a slight improvement in SNR in the realigned segment after using ORPHe. The median is around 0.1 dB, but in some isolated cases, it can be several dB, with a maximum improvement obtained of 6 dB. The results are mostly the same for all values of
4 Applicability of the BRHEN algorithm
The BRHEN algorithm is a heuristic method that is suitable for 1-tiered heterogeneous networks. The heterogeneity appears in the form of the variety of ranges it takes into account. Behind the difference in ranges is hidden a difference in emitting power, sensibility, propagation path, or a combination of those. In cases where the line between two nodes is not horizontal (such as between air and ground), the range that should be applied is the length of the path as it appears on the horizontal plane.
For example, the placement of ground stations for a permanent or temporary network. The difference in range here will be due to the difference in emitting power and sensibility. Another example is with UAVs as RNs, they enjoy free space LOS propagation for the links between each other, resulting in a greater range between them than between ground nodes with the same radio setup.
This second use-case can be pushed further into the realm of Non-Terrestrial Networks (NTN). However, using greater ranges will result in the need to take into account the Earth’s curvature. Using a conformal projection, which keeps the angles—thus the direction toward the barycenter—true, will allow the method to stick to the Cartesian formulation. Nevertheless, the distances are distorted along the meridians. Due to this, for large areas that span several degrees in latitude, a more complex formulation must be developed for the different steps of the BRHEN algorithm.
5 Conclusion
This paper investigates the methods allowing to manage the RN placement for heterogeneous networks, focusing on their effectiveness regarding the number of Relay Nodes (RNs) placed, the average hop count for the delay, the computation time, and the stability of the placement when the network is nonstatic.
Two algorithms are proposed: ORPHe (Optimized Relay Placement for one Heterogeneous link) for an enhanced RN placement between two disconnected nodes and BRHEN (Barycenter-focused Relay node placement for HEterogeneous wireless Network). They can manage a heterogeneous set of INs and RNs with a different set of communication properties. In addition, it does not require the definition of cells—which limits the precision of the computed positions.
Whether in terms of number of RNs used or in terms of the average hop count for two INs to communicate, the simulations results show the advantage of the BRHEN method over two other approaches for heterogeneous networks. Moreover, BRHEN is shown to be computationally light and to have a relatively good stability. These are important features for mobile networks, since the positions have to be updated frequently—the higher the velocities, the higher the frequency—and the updated RN positions have to be reasonably close to the previous ones. This is an important feature allowing to keep the integrity of the network during readjustments using mobile RNs with reasonable speed.
6 Future works
As a follow-up, strategies to optimally cope with the displacements of the INs need to be explored. First, study predictive IN movements first and, ultimately, deal with unexpected IN motion. Other future work will focus on the implementation of terrain and signal propagation loss in the placement selection process before conducting measurement during field trials for validation.
Data availability statement
The raw data supporting the conclusions of this article will be made available by the authors, without undue reservation.
Author contributions
EG: Conceptualization, Data curation, Formal Analysis, Investigation, Software, Writing – original draft, Writing – review and editing. VL: Writing – review and editing. BL: Writing – review and editing. MB: Writing – review and editing.
Funding
The author(s) declare that financial support was received for the research and/or publication of this article. This research is funded by the Royal Military Academy of Belgium.
Conflict of interest
The authors declare that the research was conducted in the absence of any commercial or financial relationships that could be construed as a potential conflict of interest.
Generative AI statement
The author(s) declare that no Generative AI was used in the creation of this manuscript.
Publisher’s note
All claims expressed in this article are solely those of the authors and do not necessarily represent those of their affiliated organizations, or those of the publisher, the editors and the reviewers. Any product that may be evaluated in this article, or claim that may be made by its manufacturer, is not guaranteed or endorsed by the publisher.
Footnotes
1The information of the height,
References
Cao, R., Gao, H., Lv, T., Yang, S., and Huang, S. (2016). Phase-rotation-aided relay selection in two-way decode-and-forward relay networks. IEEE Trans. Veh. Technol. 65, 2922–2935. doi:10.1109/TVT.2015.2442622
Cheng, X., Du, D.-Z., Wang, L., and Xu, B. (2008). Relay sensor placement in wireless sensor networks. Wirel. Netw. 14, 347–355. doi:10.1007/s11276-006-0724-8
Deyab, T. M., Baroudi, U., and Selim, S. Z. (2011). “Optimal placement of heterogeneous wireless sensor and relay nodes,” in 2011 7th international wireless communications and mobile computing conference, 65–70. doi:10.1109/IWCMC.2011.5982508
Grönkvist, J., Hansson, A., Hägglund, K., Komulainen, A., and Sköld, M. (2022). Low-altitude uavs for significantly increased data rate in tactical ad hoc networks. Procedia Comput. Sci. 205, 107–116. doi:10.1016/j.procs.2022.09.012
Han, X., Cao, X., Lloyd, E. L., and Shen, C.-C. (2010). Fault-tolerant relay node placement in heterogeneous wireless sensor networks. IEEE Trans. Mob. Comput. 9, 643–656. doi:10.1109/TMC.2009.161
Ladosz, P., Oh, H., and Chen, W.-H. (2018). Trajectory planning for communication relay unmanned aerial vehicles in urban dynamic environments. J. Intelligent Robotic Syst. 89, 7–25. doi:10.1007/s10846-017-0484-y
Lee, S., and Younis, M. (2010a). Optimized relay placement to federate segments in wireless sensor networks. IEEE J. Sel. Areas Commun. 28, 742–752. doi:10.1109/JSAC.2010.100611
Lee, S., and Younis, M. (2010b). “Qos-aware relay node placement for connecting disjoint segments in wireless sensor networks,” in 2010 6th IEEE International Conference on Distributed Computing in Sensor Systems Workshops (DCOSSW), Santa Barbara, CA, USA, 21-23 June 2010, 1–6. doi:10.1109/DCOSSW.2010.5593290
Lee, S., and Younis, M. (2010c). Recovery from multiple simultaneous failures in wireless sensor networks using minimum steiner tree. J. Parallel Distributed Comput. 70, 525–536. doi:10.1016/j.jpdc.2009.12.004
Lin, G., and Xue, G. (1999). Steiner tree problem with minimum number of Steiner points and bounded edge-length. Inf. Process. Lett. 69, 53–57. doi:10.1016/s0020-0190(98)00201-4
Liu, G., Lu, K., and Li, J. (2019). “Approximation algorithm for relay node placement in singled-tiered wireless sensor networks,” in 2019 IEEE 4th International Conference on Advanced Robotics and Mechatronics (ICARM), Toyonaka, Japan, 03-05 July 2019, 162–167. doi:10.1109/ICARM.2019.8834312
Lloyd, E. L., and Xue, G. (2007). Relay node placement in wireless sensor networks. IEEE Trans. Comput. 56, 134–138. doi:10.1109/TC.2007.250629
Parihar, A. S., Baghel, A., Swami, P., and Bhatia, V. (2024a). “On performance of swipt empowered noma-hetnet with non-linear energy harvesting,” in 2024 national conference on communications (NCC), 1–6. doi:10.1109/NCC60321.2024.10485896
Parihar, A. S., Singh, K., Bhatia, V., Li, C.-P., and Duong, T. Q. (2024b). Performance analysis of noma-enabled active ris-aided mimo heterogeneous iot networks with integrated sensing and communication. IEEE Internet Things J. 11, 28137–28152. doi:10.1109/JIOT.2024.3416951
Robins, G., and Zelikovsky, A. (2005). Tighter bounds for graph steiner tree approximation. SIAM J. Discrete Math. 19, 122–134. doi:10.1137/S0895480101393155
Swami, P., and Bhatia, V. (2021). “Impact of distance on outage probability in irs-noma for beyond 5g networks,” in 2021 IEEE 18th Annual Consumer Communications Networking Conference (CCNC), Las Vegas, NV, USA, 09-12 January 2021, 1–2. doi:10.1109/CCNC49032.2021.9369548
Swami, P., Mishra, M. K., Bhatia, V., Ratnarajah, T., and Trivedi, A. (2022). Performance analysis of sub-6 ghz/mmwave noma hybrid-hetnets using partial csi. IEEE Trans. Veh. Technol. 71, 12958–12971. doi:10.1109/TVT.2022.3198144
ur Rahman, S., Kim, G.-H., Cho, Y.-Z., and Khan, A. (2018). Positioning of uavs for throughput maximization in software-defined disaster area uav communication networks. J. Commun. Netw. 20, 452–463. doi:10.1109/JCN.2018.000070
Wang, Z., Duan, L., and Zhang, R. (2019). Adaptive deployment for uav-aided communication networks. IEEE Trans. Wirel. Commun. 18, 4531–4543. doi:10.1109/TWC.2019.2926279
Wu, Y., Zhang, B., Yang, S., Yi, X., and Yang, X. (2017). “Energy-efficient joint communication-motion planning for relay-assisted wireless robot surveillance,” in IEEE INFOCOM 2017 - IEEE Conference on Computer Communications, Atlanta, GA, USA, 01-04 May 2017, 1–9. doi:10.1109/INFOCOM.2017.8057072
Xie, J., Zhang, B., and Zhang, C. (2020). A novel relay node placement and energy efficient routing method for heterogeneous wireless sensor networks. IEEE Access 8, 202439–202444. doi:10.1109/ACCESS.2020.2984495
Yang, S., Xu, X., Alanis, D., Xin Ng, S., and Hanzo, L. (2016). Is the low-complexity mobile-relay-aided ffr-das capable of outperforming the high-complexity comp? IEEE Trans. Veh. Technol. 65, 2154–2169. doi:10.1109/TVT.2015.2416333
Keywords: relay node placement, heterogeneous network, mobile networks, unmanned aerial vehicle, heuristic method, steiner tree
Citation: Guffens E, Le Nir V, Lauwens B and Becquaert M (2025) Heuristic method for relay node placement in heterogeneous wireless network. Front. Commun. Netw. 6:1567560. doi: 10.3389/frcmn.2025.1567560
Received: 27 January 2025; Accepted: 09 April 2025;
Published: 30 April 2025.
Edited by:
Rosdiadee Nordin, Sunway University, MalaysiaReviewed by:
Pragya Swami, Indian Institute of Technology Indore, IndiaAhmad Bazzi, New York University Abu Dhabi, United Arab Emirates
Copyright © 2025 Guffens, Le Nir, Lauwens and Becquaert. This is an open-access article distributed under the terms of the Creative Commons Attribution License (CC BY). The use, distribution or reproduction in other forums is permitted, provided the original author(s) and the copyright owner(s) are credited and that the original publication in this journal is cited, in accordance with accepted academic practice. No use, distribution or reproduction is permitted which does not comply with these terms.
*Correspondence: Eliott Guffens, ZWxpb3R0Lmd1ZmZlbnNAbWlsLmJl