- 1School of Mathematics and Statistics, The University of Melbourne, Parkville, VIC, Australia
- 2Department of Civil, Environmental and Geomatic Engineering, Institute of Environmental Engineering, ETH Zurich, Zurich, Switzerland
- 3Institute of Marine Sciences, University of California, Santa Cruz, Santa Cruz, CA, United States
- 4Department of Ecology and Evolutionary Biology, University of California, Santa Cruz, Santa Cruz, CA, United States
- 5Department of Ecology and Evolutionary Biology, Princeton University, Princeton, NJ, United States
The ability of marine microbes to navigate toward chemical hotspots can determine their nutrient uptake and has the potential to affect the cycling of elements in the ocean. The link between bacterial navigation and nutrient cycling highlights the need to understand how chemotaxis functions in the context of marine microenvironments. Chemotaxis hinges on the stochastic binding/unbinding of molecules with surface receptors, the transduction of this information through an intracellular signaling cascade, and the activation and control of flagellar motors. The intrinsic randomness of these processes is a central challenge that cells must deal with in order to navigate, particularly under dilute conditions where noise and signal are similar in magnitude. Such conditions are ubiquitous in the ocean, where nutrient concentrations are often extremely low and subject to rapid variation in space (e.g., particulate matter, nutrient plumes) and time (e.g., diffusing sources, fluid mixing). Stochastic, biophysical models of chemotaxis have the potential to illuminate how bacteria cope with noise to efficiently navigate in such environments. At the same time, new technologies for experimentation allow for continuous interrogation—from milliseconds through to days—of bacterial responses in custom dynamic nutrient landscapes, providing unprecedented access to the behavior of chemotactic cells in microenvironments engineered to mimic those cells navigate in the wild. These recent theoretical and experimental developments have created an opportunity to derive population-level uptake from single-cell motility characteristics in ways that could inform the next generation of marine biogeochemical cycling models.
1. Introduction
The fine-scale interactions between marine bacteria and both dissolved and particulate organic matter underpin marine biogeochemistry, thereby supporting productivity and influencing carbon storage and sequestration in the planet's oceans (Azam, 1998). It has been historically very difficult to characterize marine environments on the microscales that are most relevant to individual bacteria. Rather, research efforts have typically sampled much larger volumes of water and made comparisons from one sampling site to another (Karsenti et al., 2011; Bork et al., 2015). However, at the length scales relevant to individual microbes, the ocean is an intricate and dynamic landscape of nutrient patches, at times too small to be mixed by turbulence (Kiørboe, 2008; Stocker, 2012). The capacity for microbes to actively navigate these structured environments using chemotaxis can strongly influence their nutrient uptake. Although some work has examined time-dependent chemical profiles (Zhu et al., 2012), past investigations of chemotaxis using Escherichia coli and other model organisms have routinely examined steady chemical gradients strong enough to elicit a discernible chemotactic response (Sneddon et al., 2012; Salek et al., 2019). However, the typical chemical gradients wild marine bacteria encounter are often very weak, ephemeral in nature, and with low background concentrations (Stocker, 2012). Shallow gradients are relevant for marine bacteria because, in general, gradients become weaker as one moves away from the source. Yet, detecting such gradients at distance has tremendous value, because they point toward nutrient sources. Shallow gradients are important precisely because they can be used to navigate to regions in the vicinity of sources where gradients become steep, concentrations are high, and bacteria can acquire resources at a high rate.
Past experiments have typically been limited in duration to the 1–100 s timescales over which gradient-climbing occurs (Stocker, 2015), but the timescales over which bacteria respond to nutrient uptake—through gene regulation and bacterial reproduction—are much longer. Accurately quantifying the influence of microscale dynamics in oceanic nutrient cycling hinges on (1) interrogating bacterial chemotaxis in realistic microenvironments for extended periods of time and (2) developing mathematical models that capture the essential features of chemotaxis in dilute, dynamic conditions. In this Perspective, we discuss recently developed experimental tools and mathematical frameworks for furthering our understanding of bacterial chemotaxis. We examine the various ways in which noise can enhance or degrade the sensitivity of bacterial navigation, and outline priorities for future research.
2. Bridging The Timescales of Motility and Metabolism
Bacterial chemotaxis is one of the most thoroughly understood behaviors in all of biology (Berg, 2008; Endres, 2013). Many experimental and modeling approaches have been used to investigate chemotaxis in fine detail, from early capillary assays (Adler, 1973; Brown and Berg, 1974) to experiments in controlled microfluidic environments (Mao et al., 2003; Englert et al., 2009; Seymour et al., 2010; Son et al., 2015; Salek et al., 2019) and computational modeling of complete biochemical pathways (Morton-Firth et al., 1999; Jiang et al., 2010; Long et al., 2017). Despite the vast literature on bacterial chemotaxis, unanswered questions still remain. In particular, we still know relatively little about the types of microenvironments bacteria navigate in the wild, and how chemotaxis operates and evolves in such environments (Endres, 2013; Wong-Ng et al., 2016).
The distribution of nutrients in the ocean is highly structured, varying strongly with depth, across the globe (Hansell et al., 2009), and with time (Druffel et al., 1996). Moreover, there is tremendous diversity in the types of organic matter available (Riedel and Dittmar, 2014; Benner and Amon, 2015), and substantial exchange of materials between dissolved, particulate, colloidal and gel phases (Kiørboe, 2001; Verdugo, 2012). Although bulk concentrations of dissolved organic carbon (DOC) typically vary between 35 and 70 μM throughout the ocean (Hansell et al., 2009), the vast majority of DOC exists in forms either difficult or impossible to use by bacteria. Low molecular weight, highly labile molecules such as dissolved sugars, amino acids, or nucleotides, have only nanomolar concentrations in the bulk and residence times under 1 day (Keil and Kirchman, 1999). These molecules can have transiently high concentrations in the aftermath of cell lysis, predation, or nutrient exudation by phytoplankton, but they are not components of more long-lived hotspots such as gels or particles (Verdugo, 2012). The majority of labile or semi-labile organic matter has much higher molecular weight, and consists of biological macromolecules such as proteins or large algal polysacharides (Benner and Amon, 2015). Too large to pass through bacterial cell membranes, bacteria degrade these molecules into monomers or oligomers using ecto- and exo-enzymes, allowing for their uptake, but also creating public goods which feed other bacteria or give them information on the location of nutrient hotspots.
Dissolved organic matter can be redistributed through ubiquitous fluid flows in the ocean, but at the length scale relevant to microbial motion—less than approximately 1 mm—molecular diffusion predominantly drives the redistribution of chemical cues (Batchelor, 1959). The structure and dynamics of chemical gradients in the ocean thus emerge from the interplay of DOC release by living organisms, the aggregation and disaggregation of DOC into gels and particles, the degradation and uptake of DOC by heterotrophic bacteria, and fluid turbulence.
Experiments (Blackburn et al., 1998; Stocker et al., 2008; Smriga et al., 2016) and mathematical theory (Hein et al., 2016a; Mora and Nemenman, 2019) have begun to assess the role of dynamic chemical gradients (Taylor and Stocker, 2012), investigating the interaction between timescales for motility and timescales for changes in the chemoattractant landscape. Experiments have revealed that marine bacteria are capable of rapidly responding to leaking diatoms (Smriga et al., 2016), pulses of phytoplankton exudates (Seymour et al., 2009), model marine particles and sinking aggregates (Kiørboe et al., 2002), exemplifying the importance of chemotaxis throughout the microbial food web. However, previous work has typically been limited to one single unsteady source, and to durations of a few minutes or less. While this may be long compared to the timescales for cell motility and reorientation (seconds), it is still much shorter than the timescales over which bacterial metabolism varies (Lambert and Kussell, 2014) and cell division occurs (hours to days) (Kirchman, 2016). Observations over minute-long timescales cannot detect slow modulations or systematic changes in swimming speed or chemotactic abilities, which could in principle, strongly affect the collective cell dynamics. Chemotaxis assays conducted over short timescales do not necessarily represent the dynamics of wild foraging bacteria throughout their lifetime. Beyond determining the chemotactic ability of wild marine bacteria, it will be critical to assess their propensity for performing chemotaxis in realistic environments over extended periods of time.
3. Chemotaxis in Realistic Microenvironments
What constitutes a realistic environment varies wildly between different regions of the ocean, from upwelling of nutrient-rich deep water (Lauro et al., 2009) to the nutrient-poor photic zone; and over time with seasons and bloom conditions (Teeling et al., 2012). The chemical microenvironments are sometimes driven predominantly through the action of physical processes (currents, sunlight) whose effects are known a priori. However, in many cases, the nutrient dynamics are strongly coupled to the microbial population itself. Moreover, organic matter may be dissolved (DOM, Figure 1Ai), due to phytoplankton exudation or sloppy feeding (Jackson, 2012); particulate (POM, Figure 1Aii) in marine snow and precipitates; or a combination of DOM and POM in, for example, oil droplets and phytoplankton lysis events (Smriga et al., 2016) (Figure 1Aiii).
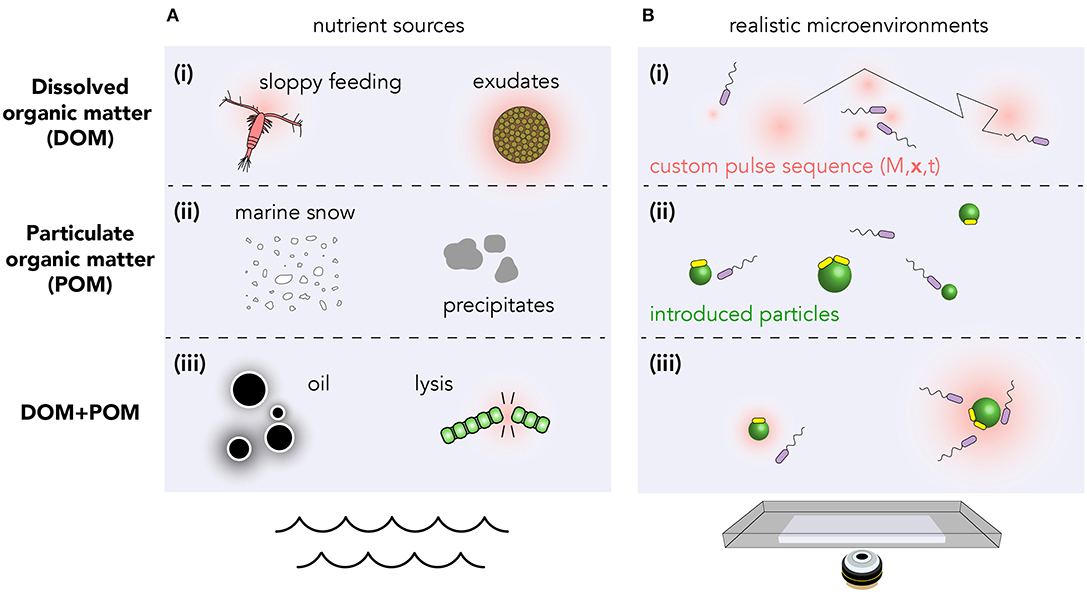
Figure 1. Structured nutrient landscapes found in the ocean can be quantitatively reproduced in laboratory settings using microfluidics, photolysis of caged resources, and discrete nutrient particles. (A) Various biological processes produce resources which may be (i) dissolved, (ii) particulate, or (iii) a combination of both. (B) Realistic microenvironments can be recreated using (i) photolysis of caged compounds to produce custom DOM landscapes (Brumley et al., 2019; Carrara et al., 2020); (ii) spatial arrays of particles (e.g., chitin or alginate) (Datta et al., 2016); or (iii) both. Within these custom arenas, bacterial chemotaxis and population kinetics can be tracked over timescales from milliseconds to days. Transitions between planktonic (purple) and surface-attached bacteria (yellow) can also be quantified (Yawata et al., 2014).
The precise values of the chemical gradients depend on many factors, including the quantity of nutrients released, its molecular diffusivity, the time over which release occurs, the rate of background uptake by bacteria, and any mixing by fluid flows. The phycosphere radius, or distance at which bacteria interact with nutrient pulses, therefore varies from ≲10μm through to several millimeters (Seymour et al., 2017). Sources can vary in duration from seconds to minutes in the case of lysis events (Blackburn et al., 1998), or many hours in the case of continuous leakage.
4. Generating Controlled, Dynamic Chemical Landscapes
A promising method for creating custom dissolved (DOM) nutrient landscapes utilizes the photo-release of caged chemoattractants (McCray and Trentham, 1989; Jasuja et al., 1999; Sagawa et al., 2014; Jikeli et al., 2015). Dissolved organic compounds such as the amino acid glutamate, which naturally occurs in coastal environments and acts as a chemoattractant for marine bacteria (Barbara and Mitchell, 2003; Duursma and Dawson, 2011), can be chemically appended using a “cage” molecule. When bound to the cage, this attractant is undetectable by bacteria. Calibrated exposure to light causes photolysis of the cage, and the precise release of chemoattractant hotspots. Since the illumination can be easily varied in space and time, this method facilitates the creation of custom two-dimensional nutrient landscapes, with exquisite precision (Brumley et al., 2019; Carrara et al., 2020).
Alginate beads (Yawata et al., 2014), chitin particles (Datta et al., 2016) and oil droplets (Desai et al., 2018) can be used to mimic particulate organic matter (POM), investigate bacterial chemotaxis, and study the physics of attachment/detachment dynamics (Figure 1). However, controlled sequential introduction of multiple particles can be difficult to achieve experimentally. Moreover, particulate matter in the ocean tends to sediment, making visualization in realistic environments challenging. Microscopy techniques that continuously follow individual marine snow particles for days at a time (Krishnamurthy et al., 2019) have the potential to provide tremendous insight into the long-time dynamics of bacterial chemotaxis and particle colonization. Reconciling the known resource landscape with bacterial trajectories measured using high throughput techniques (Taute et al., 2015; Waite et al., 2016; Brumley et al., 2019), is key to teasing apart the mechanisms bacteria use to navigate their microenvironment.
5. Noise in Bacterial Chemotaxis
From the stochastic encounters with chemoattractant molecules to the actuation of the flagellar motors, there are many sources of noise that can influence the capacity of microbes to detect and respond to chemical gradients (Sourjik and Wingreen, 2012; Micali and Endres, 2016; Kromer et al., 2018). Individual microbes experience the chemical environment as a sequence of encounters with individual molecules (Berg and Purcell, 1977) (see Figure 2A). The randomness in this sequence is particularly important within marine environments, where resource hotspots may be very small (≲1pmol) (Blackburn et al., 1998; Davis and Benner, 2007) and short-lived (seconds to minutes) (Smriga et al., 2016). The discrete molecular nature of the chemoattractant means that even in a steady, uniform environment, the number of chemoattractant molecules reaching the surface receptors per unit of time will fluctuate (Endres and Wingreen, 2008; Mora and Wingreen, 2010), and may be as small as a few molecules per second (Brumley et al., 2019). Provided that bacteria rely on temporal signaling to perform chemotaxis (Macnab and Koshland, 1972; Segall et al., 1986), a gradient can only ever be defined in an average sense, and requires integration of the arrival sequence over an appropriate timescale, T. If the cell's uncertainty in the gradient estimate is larger in magnitude than the gradient itself, a typical measurement will not be able to resolve the signal. The photo-release of glutamate in sub-picomole quantities—explicitly designed to mimic an individual lysing phytoplankton cell (Blackburn et al., 1998)—was used to assess the role of sensory noise in realistic environments (Brumley et al., 2019). The chemotactic precision of Vibrio ordalii was found to be close to the fundamental limit set by this molecule counting noise. While these results assume sampling through discrete time intervals, it will be important for future work to examine the role of continuous integration of ligand binding events (Mora and Nemenman, 2019), determine exactly how cells average measurements, and ascertain how noise propagates through the chemotaxis networks of different model organisms (Micali and Endres, 2019). Phenotypic variation may also influence the collective chemotactic response of many cells (Frankel et al., 2014; Waite et al., 2018).
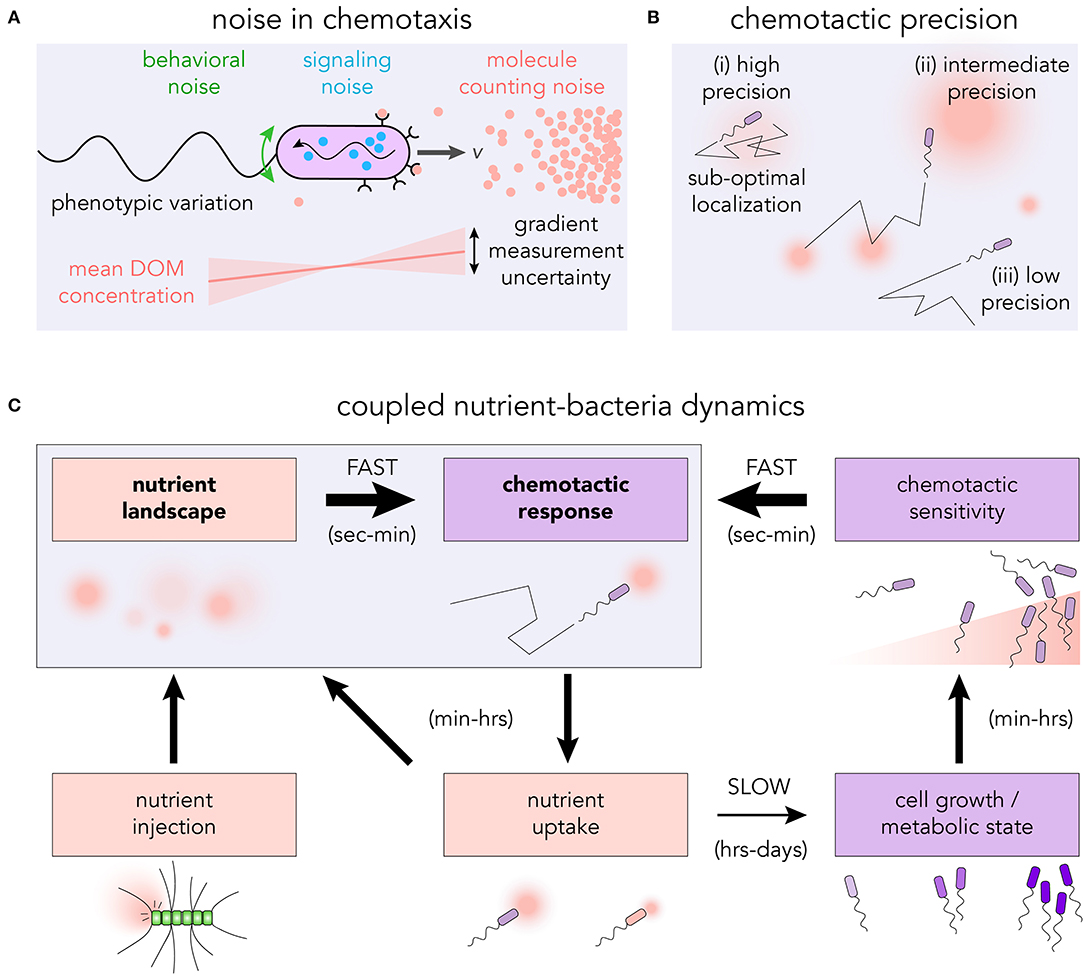
Figure 2. (A) The discrete nature of dissolved chemoattractants introduces molecule counting noise into the bacterium's measurement of the gradient. In conjunction with other sources of noise in the signaling pathway, the flagellar response as well as rotational diffusion, the cell executes an effective chemotactic response. (B) The level of chemotactic precision governs the ability of bacteria to navigate toward, and reside at, regions of high DOM concentration. (i) High chemotactic precision may result in sub-optimal localization at local nutrient maxima; (ii) intermediate precision allows for both exploration and exploitation; and (iii) low precision results in undirected motility. (C) Highly coupled nutrient-bacteria dynamics in chemotactic foraging cycle. The traditional means of studying chemotaxis is to assess the motility response to prescribed nutrient landscapes (seconds to minutes). However, over timescales of hours to days, feedback loops exist which have the capacity to reshape the nutrient landscape and modify the chemotactic response.
Intracellular noise can also influence the capacity of bacteria to respond to chemical gradients (Korobkova et al., 2004) (see Figure 2A). The discrete nature of signaling molecules places limits on a cell's chemotactic ability in a manner similar to extracellular counting noise (Bialek and Setayeshgar, 2005). Suppressing internal noise in biochemical networks generally requires a cell to produce and maintain an increased number of signaling molecules (Lestas et al., 2010; Govern and ten Wolde, 2014). Depending on the dynamics of the chemical environment, the extra cost in noise suppression (Sartori and Tu, 2015) may exceed the additional resources acquired through greater sensitivity. For systems in which the noise frequency is much higher than that of the signal, it may be possible to filter noise (Andrews et al., 2006). However, for realistic marine environments, the timescales over which chemoattractant concentrations vary and signaling molecules fluctuate may be similar. The conflation of these timescales could thwart the ability for cells to successfully filter the intracellular noise.
While it is intuitive that noise in the incoming chemical signal and the internal signaling pathway can degrade chemotactic performance, there are cases in which cells actually appear to benefit from noise (Flores et al., 2012). For bacteria with multiple flagella such as E. coli, stochastic coordination of flagellar motors can reduce the latency below that of an individual motor, which can assist in steep gradients (Sneddon et al., 2012). Random fluctuations in the signal protein CheY-P have also been shown to increase the chemotactic sensitivity of E. coli at the level of the flagellar motors (Hu and Tu, 2013; He et al., 2016). Yet, these particular mechanisms are generally not accessible to marine bacteria, which typically possess one flagellum (Xie et al., 2011) and operate in shallow, ephemeral gradients.
Greater chemotactic abilities do not necessarily lead to enhanced resource acquisition or growth (Ni et al., 2020). The level of bacterial chemotaxis which confers the greatest advantage for individual cells depends on the structure of the chemical microenvironment (Celani and Vergassola, 2010). For bacteria in the vicinity of a single isolated nutrient source, a greater chemotactic precision will generally lead to an enhancement in the nutrient exposure and uptake rate (Smriga et al., 2016). However, in an environment with multiple nutrient sources of different magnitude, a cell which perfectly follows the gradient may become trapped in a locally favorable, but globally sub-optimal position (see Figure 2Bi). Sources of noise which reduce the cell's chemotactic sensitivity may actually improve the overall nutrient acquisition by allowing the cell to more thoroughly explore its environment (see Figure 2Bii). The optimal bacterial behavior will therefore be sensitively linked to the precise spatiotemporal dynamics of the chemical microenvironment (Passino, 2002). Theoretical frameworks linking organismal navigation with the overall resource landscape structure (Hein et al., 2016b; Seymour et al., 2017; Hein and Martin, 2019) provide predictions about the optimal strategies for bacterial foraging, which can be explicitly tested, for example using microfluidic platforms.
6. Bacterial Uptake Kinetics in Structured Microenvironments
Because of fluctuations in nutrient exposure within the structured microenvironments of the ocean, the generation times, and therefore biomass production, of wild bacteria are likely to be fundamentally different from those measured during experiments in homogenous batch culture (Fenchel, 2002; Azam and Malfatti, 2007). In fact, temporal fluctuations can drive distinct growth physiologies and strategies (Yan et al., 2017), even for environments with the same mean nutrient concentration. Bacterial chemotaxis has the capacity to systematically redistribute the cells, and therefore may provide a feedback loop between the microbial population and the resource landscape (Cremer et al., 2019). In cases where there is strong two-way coupling between the bacterial dynamics and the nutrient field—i.e., nutrient field drives chemotaxis, and bacterial consumption shapes nutrient profile—it is important to study the system for an extended period of time (Carrara et al., 2020). This will provide great utility in determining realistic cell growth rates that are often very difficult to measure in situ (Kirchman, 2016).
Chemotaxis, as well as plasticity in physiology and behavioral strategy, can enhance the ability of bacteria to respond to spatial and temporal variation in resources. This, in turn, has the potential to influence ecosystem level processes such as remineralization rates or carbon export fluxes. Rates of these microbially mediated processes exhibit considerable regional variation (Hansell et al., 2012; Mouw et al., 2016), and they also strongly influence the distribution of carbon throughout the oceans and atmosphere (Kwon et al., 2009). Global-scale biogeochemical models rely on highly simplified, phenomenological parameterizations of remineralization of particulate and dissolved organic matter, which prevents them from detecting carbon-cycle feedbacks induced by the ecological dynamics of marine microbes (Mislan et al., 2014). The ability to assess long-term changes in microbial motility presents a unique opportunity for determining the influence of physiological states in bacterial chemotaxis (Jordan et al., 2013; Cremer et al., 2019), which is a key step to understanding how to link microbes and biogeochemistry.
Following early evidence that aspects of E. coli chemotaxis are independent of uptake or metabolism (Adler, 1969), research has largely overlooked the capacity for uptake or physiological states to influence motility. Studies of microbial motion often use highly specific culturing conditions. If the nutrients are too scarce cells may not grow, swim or navigate. Similarly, cell motility and chemotaxis may also be reduced by overly abundant nutrients, since they are not advantageous when resources are plentiful (Ni et al., 2020). Additional work suggests that E. coli (Taylor and Zhulin, 1998), Azospirillum brasilense (Alexandre et al., 2000) and other species of bacteria (Alexandre and Zhulin, 2001) exhibit metabolism-dependent chemotaxis. Bacteria appear to modulate the way they perform chemotaxis—and indeed whether they even swim at all—based on their metabolic state, which presumably helps cells cope with the conditions they experience in natural environments (Ni et al., 2020). Correlations between cellular motility and other copiotrophic traits (Koch, 2001), such as ribosomal copy number, hydrolytic enzymes, and genes for environmental sensing and signaling (Lauro et al., 2009; Roller et al., 2016) hint at the capacity for motile bacteria to rapidly expand their population, to adapt their behavioral and trophic strategies in response to their environment, and to structure local nutrient fields by being the primary degraders of biological macromolecules. The feedback loop between nutrient exposure, swimming speed (Son et al., 2016) and ultimately chemotactic sensitivity (Hein et al., 2016a; Ni et al., 2020) exemplifies the need to interrogate bacteria in realistic environments for extended periods of time, and investigate temporal variations in motility and chemotactic performance (see Figure 2C), within and between successive generations. Since biogeochemical cycling will be driven not only by the spatial distribution of cells with respect to the nutrient field, but also by their metabolic state, modeling both of these processes (Egbert et al., 2010) may prove to be important in predicting nutrient cycling in the ocean.
7. Priorities for Future Research
The capacity to generate controlled, dynamic chemical landscapes, while simultaneously visualizing bacterial responses from milliseconds through to days, represents a uniquely powerful approach to investigating marine microbial processes. An important area for future exploration will be considering multiple interacting species of bacteria and other organisms (Amin et al., 2012; Hol et al., 2016; Raina et al., 2019), where population and community dynamics may depend on the nutrient properties and organismal phenotypes. This will be particularly important in assessing the spatial patterning of metabolic handoffs (Anantharaman et al., 2016), density dependent competition dynamics (Gude et al., 2020), and the role of quorum sensing (Hmelo, 2017).
Investigating the rich interplay between multiple chemoattractants represents another important direction for future research. Except in some simplified domains (Kalinin et al., 2010), bacterial chemotaxis is routinely studied in response to single molecular species. Yet, phytoplankton exudates and other dissolved organic matter exist as complex mixtures of compounds which differ in their abundance, diffusivity and propensity to elicit chemotaxis (Davis and Benner, 2007; Duursma and Dawson, 2011). Emerging analytical methods of characterizing chemical microenvironments, exudation rates and nutrient exchanges (Wessel et al., 2013) will further inform models for microbial motion (Keller and Segel, 1970; Jackson, 1987; Bray et al., 2007; Desai et al., 2019) through to ocean-scale dynamics of microbial populations (Barton et al., 2010; Worden et al., 2015; Kuhn et al., 2019).
Author Contributions
DB wrote the first draft of the manuscript. All authors revised the manuscript and approved it for publication.
Funding
This work was supported by an Australian Research Council Discovery Early Career Researcher Award DE180100911 (to DB), an NSF IOS Grant 1855956 (to AH), NSF grant OCE-1848576 (to SL and GH), NOAA-AWD1005828 (to GH), a Simons Foundation Grant 395890 (to SL and GH), a grant from the Swiss National Science Foundation (315230_176189) (to RS), and a Simons Foundation Grant 542395 (to RS) as part of the Principles of Microbial Ecosystems Collaborative (PriME).
Conflict of Interest
The authors declare that the research was conducted in the absence of any commercial or financial relationships that could be construed as a potential conflict of interest.
References
Adler, J. (1969). Chemoreceptors in bacteria. Science 166, 1588–1597. doi: 10.1126/science.166.3913.1588
Adler, J. (1973). A method for measuring chemotaxis and use of the method to determine optimum conditions for chemotaxis by Escherichia coli. Microbiology 74, 77–91. doi: 10.1099/00221287-74-1-77
Alexandre, G., Greer, S. E., and Zhulin, I. B. (2000). Energy taxis is the dominant behavior in Azospirillum brasilense. J. Bacteriol. 182, 6042–6048. doi: 10.1128/JB.182.21.6042-6048.2000
Alexandre, G., and Zhulin, I. B. (2001). More than one way to sense chemicals. J. Bacteriol. 183, 4681–4686. doi: 10.1128/JB.183.16.4681-4686.2001
Amin, S. A., Parker, M. S., and Armbrust, E. V. (2012). Interactions between diatoms and bacteria. Microbiol. Mol. Biol. Rev. 76, 667–684. doi: 10.1128/MMBR.00007-12
Anantharaman, K., Brown, C. T., Hug, L. A., Sharon, I., Castelle, C. J., Probst, A. J., et al. (2016). Thousands of microbial genomes shed light on interconnected biogeochemical processes in an aquifer system. Nat. Commun. 7:13219. doi: 10.1038/ncomms13219
Andrews, B. W., Yi, T.-M., and Iglesias, P. A. (2006). Optimal noise filtering in the chemotactic response of Escherichia coli. PLoS Comput. Biol. 2:e154. doi: 10.1371/journal.pcbi.0020154
Azam, F. (1998). Microbial control of oceanic carbon flux: the plot thickens. Science 280, 694–696. doi: 10.1126/science.280.5364.694
Azam, F., and Malfatti, F. (2007). Microbial structuring of marine ecosystems. Nat. Rev. Micro 5, 782–791. doi: 10.1038/nrmicro1747
Barbara, G. M., and Mitchell, J. G. (2003). Marine bacterial organisation around point-like sources of amino acids. FEMS Microbiol. Ecol. 43, 99–109. doi: 10.1111/j.1574-6941.2003.tb01049.x
Barton, A. D., Dutkiewicz, S., Flierl, G., Bragg, J., and Follows, M. J. (2010). Patterns of diversity in marine phytoplankton. Science 327, 1509–1511. doi: 10.1126/science.1184961
Batchelor, G. K. (1959). Small-scale variation of convected quantities like temperature in turbulent fluid Part 1. General discussion and the case of small conductivity. J. Fluid Mech. 5, 113–133. doi: 10.1017/S002211205900009X
Benner, R., and Amon, R. M. W. (2015). The size-reactivity continuum of major bioelements in the ocean. Annu. Rev. Mar. Sci. 7, 185–205. doi: 10.1146/annurev-marine-010213-135126
Berg, H. C., and Purcell, E. M. (1977). Physics of chemoreception. Biophys. J. 20, 193–219. doi: 10.1016/S0006-3495(77)85544-6
Bialek, W., and Setayeshgar, S. (2005). Physical limits to biochemical signaling. Proc. Natl. Acad. Sci. U.S.A. 102, 10040–10045. doi: 10.1073/pnas.0504321102
Blackburn, N., Fenchel, T., and Mitchell, J. (1998). Microscale nutrient patches in planktonic habitats shown by chemotactic bacteria. Science 282, 2254–2256. doi: 10.1126/science.282.5397.2254
Bork, P., Bowler, C., de Vargas, C., Gorsky, G., Karsenti, E., and Wincker, P. (2015). Tara Oceans studies plankton at planetary scale. Science 348, 873–873. doi: 10.1126/science.aac5605
Bray, D., Levin, M. D., and Lipkow, K. (2007). The chemotactic behavior of computer-based surrogate bacteria. Curr. Biol. 17, 12–19. doi: 10.1016/j.cub.2006.11.027
Brown, D. A., and Berg, H. C. (1974). Temporal Stimulation of Chemotaxis in Escherichia coli. Proc. Natl. Acad. Sci. U.S.A. 71, 1388–1392. doi: 10.1073/pnas.71.4.1388
Brumley, D. R., Carrara, F., Hein, A. M., Yawata, Y., Levin, S. A., and Stocker, R. (2019). Bacteria push the limits of chemotactic precision to navigate dynamic chemical gradients. Proc. Natl. Acad. Sci. U.S.A. 116, 10792–10797. doi: 10.1073/pnas.1816621116
Carrara, F., Brumley, D. R., Hein, A. M., Yawata, Y., Salek, M. M., Lee, K. S., et al. (2020). Generating controlled, dynamic chemical landscapes to study microbial behavior. J. Visual. Exp. e60589. doi: 10.3791/60589
Celani, A., and Vergassola, M. (2010). Bacterial strategies for chemotaxis response. Proc. Natl. Acad. Sci. U.S.A. 107, 1391–1396. doi: 10.1073/pnas.0909673107
Cremer, J., Honda, T., Tang, Y., Wong-Ng, J., Vergassola, M., and Hwa, T. (2019). Chemotaxis as a navigation strategy to boost range expansion. Nature. 575, 658–663. doi: 10.1038/s41586-019-1733-y
Datta, M. S., Sliwerska, E., Gore, J., Polz, M. F., and Cordero, O. X. (2016). Microbial interactions lead to rapid micro-scale successions on model marine particles. Nat. Commun. 7:11965. doi: 10.1038/ncomms11965
Davis, J., and Benner, R. (2007). Quantitative estimates of labile and semi-labile dissolved organic carbon in the western Arctic Ocean: a molecular approach. Limnol. Oceanogr. 52, 2434–2444. doi: 10.4319/lo.2007.52.6.2434
Desai, N., Shaik, V. A., and Ardekani, A. M. (2018). Hydrodynamics-mediated trapping of micro-swimmers near drops. Soft Matter 14, 264–278. doi: 10.1039/C7SM01615H
Desai, N., Shaik, V. A., and Ardekani, A. M. (2019). Hydrodynamic interaction enhances colonization of sinking nutrient so-urces by motile microorganisms. Front. Microbiol. 10:289. doi: 10.3389/fmicb.2019.00289
Druffel, E. R. M., Bauer, J. E., Williams, P. M., Griffin, S., and Wolgast, D. (1996). Seasonal variability of particulate organic radiocarbon in the northeast Pacific Ocean. J. Geophys. Res. Oceans 101, 20543–20552. doi: 10.1029/96JC01850
Egbert, M. D., Barandiaran, X. E., and Di Paolo, E. A. (2010). A minimal model of metabolism-based chemotaxis. PLoS Comput. Biol. 6:e1001004. doi: 10.1371/journal.pcbi.1001004
Endres, R. G. (2013). Physical Principles in Sensing and Signaling: With an Introduction to Modeling in Biology. Oxford: Oxford University Press.
Endres, R. G., and Wingreen, N. S. (2008). Accuracy of direct gradient sensing by single cells. Proc. Natl. Acad. Sci. U.S.A. 105, 15749–15754. doi: 10.1073/pnas.0804688105
Englert, D. L., Manson, M. D., and Jayaraman, A. (2009). Flow-based microfluidic device for quantifying bacterial chemotaxis in stable, competing gradients. Appl. Environ. Microbiol. 75, 4557–4564. doi: 10.1128/AEM.02952-08
Fenchel, T. (2002). Microbial behavior in a heterogeneous world. Science 296, 1068–1071. doi: 10.1126/science.1070118
Flores, M., Shimizu, T. S., ten Wolde, P. R., and Tostevin, F. (2012). Signaling noise enhances chemotactic drift of E. coli. Phys. Rev. Lett. 109:148101. doi: 10.1103/PhysRevLett.109.148101
Frankel, N. W., Pontius, W., Dufour, Y. S., Long, J., Hernandez-Nunez, L., and Emonet, T. (2014). Adaptability of non-genetic diversity in bacterial chemotaxis. eLife 3:e03526. doi: 10.7554/eLife.03526
Govern, C. C., and ten Wolde, P. R. (2014). Optimal resource allocation in cellular sensing systems. Proc. Natl. Acad. Sci. U.S.A. 111, 17486–17491. doi: 10.1073/pnas.1411524111
Gude, S., Pinçe, E., Taute, K. M., Seinen, A.-B., Shimizu, T. S., and Tans, S. J. (2020). Bacterial coexistence driven by motility and spatial competition. Nature 578, 588–592. doi: 10.1038/s41586-020-2033-2
Hansell, D. A., Carlson, C. A., Repeta, D. J., and Schlitzer, R. (2009). Dissolved organic matter in the ocean: a controversy stimulates new insights. Oceanography 22, 202–211. doi: 10.5670/oceanog.2009.109
Hansell, D. A., Carlson, C. A., and Schlitzer, R. (2012). Net removal of major marine dissolved organic carbon fractions in the subsurface ocean. Glob. Biogeochem. Cycles 26. doi: 10.1029/2011GB004069
He, R., Zhang, R., and Yuan, J. (2016). Noise-induced increase of sensitivity in bacterial chemotaxis. Biophys. J. 111, 430–437. doi: 10.1016/j.bpj.2016.06.013
Hein, A. M., Brumley, D. R., Carrara, F., Stocker, R., and Levin, S. A. (2016a). Physical limits on bacterial navigation in dynamic environments. J. R. Soc. Interfac 13:20150844. doi: 10.1098/rsif.2015.0844
Hein, A. M., Carrara, F., Brumley, D. R., Stocker, R., and Levin, S. A. (2016b). Natural search algorithms as a bridge between organisms, evolution, and ecology. Proc. Natl Acad. Sci. U.S.A. 113, 9413–9420. doi: 10.1073/pnas.1606195113
Hein, A. M., and Martin, B. T. (2019). Information limitation and the dynamics of coupled ecological systems. Nat. Ecol. Evol. 4, 82–90. doi: 10.1038/s41559-019-1008-x
Hmelo, L. R. (2017). Quorum sensing in marine microbial environments. Annu. Rev. Mar. Sci. 9, 257–281. doi: 10.1146/annurev-marine-010816-060656
Hol, F. J. H., Rotem, O., Jurkevitch, E., Dekker, C., and Koster, D. A. (2016). Bacterial predator-prey dynamics in microscale patchy landscapes. Proc. R. Soc. Lond. B Biol. Sci. 283:20152154. doi: 10.1098/rspb.2015.2154
Hu, B., and Tu, Y. (2013). Coordinated switching of bacterial flagellar motors: evidence for direct motor-motor coupling? Phys. Rev. Lett. 110:158703. doi: 10.1103/PhysRevLett.110.158703
Jackson, G. A. (1987). Simulating chemosensory responses of marine microorganisms. Limnol. Oceanogr. 32, 1253–1266. doi: 10.4319/lo.1987.32.6.1253
Jackson, G. A. (2012). Seascapes: the world of aquatic organisms as determined by their particulate natures. J. Exp. Biol. 215, 1017–1030. doi: 10.1242/jeb.059105
Jasuja, R., Keyoung, J., Reid, G. P., Trentham, D. R., and Khan, S. (1999). Chemotactic responses of Escherichia coli to small jumps of photoreleased L-aspartate. Biophys. J. 76, 1706–1719. doi: 10.1016/S0006-3495(99)77329-7
Jiang, L., Ouyang, Q., and Tu, Y. (2010). Quantitative modeling of Escherichia coli chemotactic motion in environments varying in space and time. PLoS Comput. Biol. 6:e1000735. doi: 10.1371/journal.pcbi.1000735
Jikeli, J. F., Alvarez, L., Friedrich, B. M., Wilson, L. G., Pascal, R., Colin, R., et al. (2015). Sperm navigation along helical paths in 3D chemoattractant landscapes. Nat. Commun. 6:7985. doi: 10.1038/ncomms8985
Jordan, D., Kuehn, S., Katifori, E., and Leibler, S. (2013). Behavioral diversity in microbes and low-dimensional phenotypic spaces. Proc. Natl. Acad. Sci. U.S.A. 110, 14018–14023. doi: 10.1073/pnas.1308282110
Kalinin, Y., Neumann, S., Sourjik, V., and Wu, M. (2010). Responses of Escherichia coli bacteria to two opposing chemoattractant gradients depend on the chemoreceptor ratio. J. Bacteriol. 192, 1796–1800. doi: 10.1128/JB.01507-09
Karsenti, E., Acinas, S. G., Bork, P., Bowler, C., De Vargas, C., Raes, J., et al. (2011). A holistic approach to marine eco-systems biology. PLoS Biol. 9:e1001177. doi: 10.1371/journal.pbio.1001177
Keil, R. G., and Kirchman, D. L. (1999). Utilization of dissolved protein and amino acids in the northern sargasso sea. Aquat. Microb. Ecol. 18, 293–300. doi: 10.3354/ame018293
Keller, E. F., and Segel, L. A. (1970). Initiation of slime mold aggregation viewed as an instability. J. Theoret. Biol. 26, 399–415. doi: 10.1016/0022-5193(70)90092-5
Kiørboe, T. (2001). Formation and fate of marine snow: small-scale processes with large-scale implications. Sci. Mar. 65, 57–71. doi: 10.3989/scimar.2001.65s257
Kiørboe, T. (2008). A Mechanistic Approach to Plankton Ecology. Princeton University Press. doi: 10.1515/9780691190310
Kiørboe, T., Grossart, H.-P., Ploug, H., and Tang, K. (2002). Mechanisms and rates of bacterial colonization of sinking aggregates. Appl. Environ. Microbiol. 68, 3996–4006. doi: 10.1128/AEM.68.8.3996-4006.2002
Kirchman, D. L. (2016). Growth rates of microbes in the oceans. Annu. Rev. Mar. Sci. 8, 285–309. doi: 10.1146/annurev-marine-122414-033938
Korobkova, E., Emonet, T., Vilar, J. M. G., Shimizu, T. S., and Cluzel, P. (2004). From molecular noise to behavioural variability in a single bacterium. Nature 428, 574–578. doi: 10.1038/nature02404
Krishnamurthy, D., Li, H., Benoit du Rey, F., Cambournac, P., Larson, A., and Prakash, M. (2019). Scale-free vertical tracking microscopy: towards bridging scales in biological oceanography. bioRxiv 610246. 1–44. doi: 10.1101/610246
Kromer, J. A., Märcker, S., Lange, S., Baier, C., and Friedrich, B. M. (2018). Decision making improves sperm chemotaxis in the presence of noise. PLoS Comput. Biol. 14:e1006109. doi: 10.1371/journal.pcbi.1006109
Kuhn, A. M., Dutkiewicz, S., Jahn, O., Clayton, S., Rynearson, T. A., Mazloff, M. R., et al. (2019). Temporal and spatial scales of correlation in marine phytoplankton communities. J. Geophys. Res. Oceans. 124, 9417–9438. doi: 10.1029/2019JC015331
Kwon, E. Y., Primeau, F., and Sarmiento, J. L. (2009). The impact of remineralization depth on the air-sea carbon balance. Nat. Geosci. 2, 630–635. doi: 10.1038/ngeo612
Lambert, G., and Kussell, E. (2014). Memory and fitness optimization of bacteria under fluctuating environments. PLoS Genet. 10:e1004556. doi: 10.1371/journal.pgen.1004556
Lauro, F. M., McDougald, D., Thomas, T., Williams, T. J., Egan, S., Rice, S., et al. (2009). The genomic basis of trophic strategy in marine bacteria. Proc. Natl. Acad. Sci. U.S.A. 106, 15527–15533. doi: 10.1073/pnas.0903507106
Lestas, I., Vinnicombe, G., and Paulsson, J. (2010). Fundamental limits on the suppression of molecular fluctuations. Nature 467, 174–178. doi: 10.1038/nature09333
Long, J., Zucker, S. W., and Emonet, T. (2017). Feedback between motion and sensation provides nonlinear boost in run-and-tumble navigation. PLoS Comput. Biol. 13:e1005429. doi: 10.1371/journal.pcbi.1005429
Macnab, R. M., and Koshland, D. E. (1972). The gradient-sensing mechanism in bacterial chemotaxis. Proc. Natl Acad. Sci. U.S.A. 69, 2509–2512. doi: 10.1073/pnas.69.9.2509
Mao, H., Cremer, P. S., and Manson, M. D. (2003). A sensitive, versatile microfluidic assay for bacterial chemotaxis. Proc. Natl Acad. Sci. U.S.A. 100, 5449–5454. doi: 10.1073/pnas.0931258100
McCray, J. A., and Trentham, D. R. (1989). Properties and uses of photoreactive caged compounds. Annu. Rev. Biophys. Biophys. Chem. 18, 239–270. doi: 10.1146/annurev.bb.18.060189.001323
Micali, G., and Endres, R. G. (2016). Bacterial chemotaxis: information processing, thermodynamics, and behavior. Curr. Opin. Microbiol. 30, 8–15. doi: 10.1016/j.mib.2015.12.001
Micali, G., and Endres, R. G. (2019). Maximal information transmission is compatible with ultrasensitive biological pathways. Sci. Rep. 9:16898. doi: 10.1038/s41598-019-53273-4
Mislan, K. A. S., Stock, C. A., Dunne, J. P., and Sarmiento, J. L. (2014). Group behavior among model bacteria influences particulate carbon remineralization depths. J. Mar. Res. 72, 183–218. doi: 10.1357/002224014814901985
Mora, T., and Nemenman, I. (2019). Physical limit to concentration sensing in a changing environment. Phys. Rev. Lett. 123:198101. doi: 10.1103/PhysRevLett.123.198101
Mora, T., and Wingreen, N. S. (2010). Limits of sensing temporal concentration changes by single cells. Phys. Rev. Lett. 104:248101. doi: 10.1103/PhysRevLett.104.248101
Morton-Firth, C. J., Shimizu, T. S., and Bray, D. (1999). A free-energy-based stochastic simulation of the tar receptor complex1. J. Mol. Biol. 286, 1059–1074. doi: 10.1006/jmbi.1999.2535
Mouw, C. B., Barnett, A., McKinley, G. A., Gloege, L., and Pilcher, D. (2016). Phytoplankton size impact on export flux in the global ocean. Glob. Biogeochem. Cycles 30, 1542–1562. doi: 10.1002/2015GB005355
Ni, B., Colin, R., Link, H., Endres, R. G., and Sourjik, V. (2020). Growth-rate dependent resource investment in bacterial motile behavior quantitatively follows potential benefit of chemotaxis. Proc. Natl. Acad. Sci. U.S.A. 117, 595–601. doi: 10.1073/pnas.1910849117
Passino, K. M. (2002). Biomimicry of bacterial foraging for distributed optimization and control. IEEE Control Syst. 22, 52–67. doi: 10.1109/MCS.2002.1004010
Raina, J.-B., Fernandez, V., Lambert, B., Stocker, R., and Seymour, J. R. (2019). The role of microbial motility and chemotaxis in symbiosis. Nat. Rev. Microbiol. 17, 284–294. doi: 10.1038/s41579-019-0182-9
Riedel, T., and Dittmar, T. (2014). A method detection limit for the analysis of natural organic matter via Fourier transform ion cyclotron resonance mass spectrometry. Anal. Chem. 86, 8376–8382. doi: 10.1021/ac501946m
Roller, B. R. K., Stoddard, S. F., and Schmidt, T. M. (2016). Exploiting rRNA operon copy number to investigate bacterial reproductive strategies. Nat. Microbiol. 1:16160. doi: 10.1038/nmicrobiol.2016.160
Sagawa, T., Kikuchi, Y., Inoue, Y., Takahashi, H., Muraoka, T., Kinbara, K., et al. (2014). Single-cell E. coli response to an instantaneously applied chemotactic signal. Biophys. J. 107, 730–739. doi: 10.1016/j.bpj.2014.06.017
Salek, M. M., Carrara, F., Fernandez, V., Guasto, J. S., and Stocker, R. (2019). Bacterial chemotaxis in a microfluidic T-maze reveals strong phenotypic heterogeneity in chemotactic sensitivity. Nat. Commun. 10:1877. doi: 10.1038/s41467-019-09521-2
Sartori, P., and Tu, Y. (2015). Free energy cost of reducing noise while maintaining a high sensitivity. Phys. Rev. Lett. 115:118102. doi: 10.1103/PhysRevLett.115.118102
Segall, J. E., Block, S. M., and Berg, H. C. (1986). Temporal comparisons in bacterial chemotaxis. Proc. Natl Acad. Sci. U.S.A. 83, 8987–8991. doi: 10.1073/pnas.83.23.8987
Seymour, J. R., Amin, S. A., Raina, J.-B., and Stocker, R. (2017). Zooming in on the phycosphere: the ecological interface for phytoplankton-bacteria relationships. Nat. Microbiol. 2:17065. doi: 10.1038/nmicrobiol.2017.65
Seymour, J. R., Marcos, and Stocker, R. (2009). Resource patch formation and exploitation throughout the marine microbial food web. Am. Natural. 173, E15–E29. doi: 10.1086/593004
Seymour, J. R., Simó, R., Ahmed, T., and Stocker, R. (2010). Chemoattraction to dimethylsulfoniopropionate throughout the marine microbial food web. Science 329, 342–345. doi: 10.1126/science.1188418
Smriga, S., Fernandez, V. I., Mitchell, J. G., and Stocker, R. (2016). Chemotaxis toward phytoplankton drives organic matter partitioning among marine bacteria. Proc. Natl. Acad. Sci. U.S.A. 113, 1576–1581. doi: 10.1073/pnas.1512307113
Sneddon, M., Pontius, W., and Emonet, T. (2012). Stochastic coordination of multiple actuators reduces latency and improves chemotactic response in bacteria. Proc. Natl. Acad. Sci. U.S.A. 109, 805–810. doi: 10.1073/pnas.1113706109
Son, K., Brumley, D. R., and Stocker, R. (2015). Live from under the lens: exploring microbial motility with dynamic imaging and microfluidics. Nat. Rev. Microbiol. 13, 761–775. doi: 10.1038/nrmicro3567
Son, K., Menolascina, F., and Stocker, R. (2016). Speed-dependent chemotactic precision in marine bacteria. Proc. Natl. Acad. Sci. U.S.A. 113, 8624–8629. doi: 10.1073/pnas.1602307113
Sourjik, V., and Wingreen, N. S. (2012). Responding to chemical gradients: bacterial chemotaxis. Curr. Opin. Cell Biol. 24, 262–268. doi: 10.1016/j.ceb.2011.11.008
Stocker, R. (2012). Marine microbes see a sea of gradients. Science 338, 628–633. doi: 10.1126/science.1208929
Stocker, R. (2015). The 100 a length scale in the microbial ocean. Aquat. Microb. Ecol. 76, 189–194. doi: 10.3354/ame01777
Stocker, R., Seymour, J. R., Samadani, A., Hunt, D. E., and Polz, M. F. (2008). Rapid chemotactic response enables marine bacteria to exploit ephemeral microscale nutrient patches. Proc. Natl. Acad. Sci. U.S.A. 105, 4209–4214. doi: 10.1073/pnas.0709765105
Taute, K. M., Gude, S., Tans, S. J., and Shimizu, T. S. (2015). High-throughput 3D tracking of bacteria on a standard phase contrast microscope. Nat. Commun. 6:8776. doi: 10.1038/ncomms9776
Taylor, B. L., and Zhulin, I. B. (1998). In search of higher energy: metabolism-dependent behaviour in bacteria. Mol. Microbiol. 28, 683–690. doi: 10.1046/j.1365-2958.1998.00835.x
Taylor, J. R., and Stocker, R. (2012). Trade-offs of chemotactic foraging in turbulent water. Science 338, 675–679. doi: 10.1126/science.1219417
Teeling, H., Fuchs, B. M., Becher, D., Klockow, C., Gardebrecht, A., Bennke, C. M., et al. (2012). Substrate-controlled succession of marine bacterioplankton populations induced by a phytoplankton bloom. Science 336, 608–611. doi: 10.1126/science.1218344
Verdugo, P. (2012). Marine microgels. Annu. Rev. Mar. Sci. 4, 375–400. doi: 10.1146/annurev-marine-120709-142759
Waite, A. J., Frankel, N. W., Dufour, Y. S., Johnston, J. F., Long, J., et al. (2016). Non-genetic diversity modulates population performance. Mol. Syst. Biol. 12:895. doi: 10.15252/msb.20167044
Waite, A. J., Frankel, N. W., and Emonet, T. (2018). Behavioral variability and phenotypic diversity in bacterial chemotaxis. Annu. Rev. Biophys. 47, 595–616. doi: 10.1146/annurev-biophys-062215-010954
Wessel, A. K., Hmelo, L., Parsek, M. R., and Whiteley, M. (2013). Going local: technologies for exploring bacterial microenvironments. Nat. Rev. Microbiol. 11, 337–348. doi: 10.1038/nrmicro3010
Wong-Ng, J., Melbinger, A., Celani, A., and Vergassola, M. (2016). The role of adaptation in bacterial speed races. PLoS Comput. Biol. 12:e1004974. doi: 10.1371/journal.pcbi.1004974
Worden, A. Z., Follows, M. J., Giovannoni, S. J., Wilken, S., Zimmerman, A. E., and Keeling, P. J. (2015). Rethinking the marine carbon cycle: Factoring in the multifarious lifestyles of microbes. Science 347:6223. doi: 10.1126/science.1257594
Xie, L., Altindal, T., Chattopadhyay, S., and Wu, X.-L. (2011). Bacterial flagellum as a propeller and as a rudder for efficient chemotaxis. Proc. Natl. Acad. Sci. U.S.A. 108, 2246–2251. doi: 10.1073/pnas.1011953108
Yan, J., Nadell, C. D., and Bassler, B. L. (2017). Environmental fluctuation governs selection for plasticity in biofilm production. ISME J. 11, 1569–1577. doi: 10.1038/ismej.2017.33
Yawata, Y., Cordero, O. X., Menolascina, F., Hehemann, J.-H., Polz, M. F., and Stocker, R. (2014). Competition-dispersal tradeoff ecologically differentiates recently speciated marine bacterioplankton populations. Proc. Natl. Acad. Sci. U.S.A. 111, 5622–5627. doi: 10.1073/pnas.1318943111
Keywords: chemotaxis, bacteria, motility, sensing noise, ocean, microbial ecology, navigation, fluctuations
Citation: Brumley DR, Carrara F, Hein AM, Hagstrom GI, Levin SA and Stocker R (2020) Cutting Through the Noise: Bacterial Chemotaxis in Marine Microenvironments. Front. Mar. Sci. 7:527. doi: 10.3389/fmars.2020.00527
Received: 24 January 2020; Accepted: 09 June 2020;
Published: 24 July 2020.
Edited by:
Aditya R. Nayak, Florida Atlantic University, United StatesReviewed by:
Francesca Malfatti, Istituto Nazionale di Oceanografia e di Geofisica Sperimentale (OGS), ItalyJian Sheng, Texas A&M University Corpus Christi, United States
Navish Wadhwa, Harvard University, United States
Copyright © 2020 Brumley, Carrara, Hein, Hagstrom, Levin and Stocker. This is an open-access article distributed under the terms of the Creative Commons Attribution License (CC BY). The use, distribution or reproduction in other forums is permitted, provided the original author(s) and the copyright owner(s) are credited and that the original publication in this journal is cited, in accordance with accepted academic practice. No use, distribution or reproduction is permitted which does not comply with these terms.
*Correspondence: Douglas R. Brumley, ZC5icnVtbGV5QHVuaW1lbGIuZWR1LmF1