- 1State Key Laboratory of Submarine Geoscience, Second Institute of Oceanography, Ministry of Natural Resources, Hangzhou, China
- 2Key Laboratory of System Control and Information Processing, Ministry of Education of China, Institute of Image Processing and Pattern Recognition, Shanghai Jiao Tong University, Shanghai, China
- 3Key Laboratory of Marine Ecosystem Dynamics, Ministry of Natural Resources and Second Institute of Oceanography, Ministry of Natural Resources, Hangzhou, China
- 4School of Oceanography, Shanghai Jiao Tong University, Shanghai, China
Marine invasive Decapoda species have caused huge losses to biodiversity and world fisheries. Early awareness of non-indigenous species (NIS) is critical to prompt response and mitigate impacts. Citizen support has emerged as a valuable tool for the early detection of NIS worldwide. However, the great biodiversity of Decapoda species in global oceans poses challenges for the public to the recognize marine Decapoda species, especially for the uncommon or unfamiliar specimens, which sometimes might be NIS. However, despite the remarkable performance of deep learning (DL) techniques in automated image analysis, there remains a scarcity of professional tools tailored specifically for the image classification of diverse decapods. To tackle this challenge, a web application for automated image classification of marine Decapoda species, termed DecapodAI, was developed by training a fine-tuned Contrastive Language–Image Pretraining model with the images from the World Register of Marine Species. For the test dataset, DecapodAI achieved average accuracies of 0.717 for family, 0.719 for genus, and 0.773 for species. Online service is provided at http://www.csbio.sjtu.edu.cn/bioinf/DecapodAI/. It is expected to promote public participation by alleviating the burden of manually analyzing images and has promising application prospects in exploring and monitoring the biodiversity of decapods in global oceans, including early awareness of NIS.
Introduction
Marine Decapoda species, which are widely found from the coastal zone to the abyssal zone, play vital roles in ecosystems and in world fisheries (Marquez and Idaszkin, 2021; Joyce et al., 2022; Truchet et al., 2023). In 2020, the total capture production of marine Brachyura crabs was estimated to be 290,000 tons (live weight) (FAO, 2022). However, while Decapoda species are essential for ecosystems and fisheries, certain invasive species could negatively impact the ecosystem, leading to the loss of native biodiversity and economy. For instance, the Europe-native green crab (Carcinus maenas) has been introduced to the Pacific and Atlantic coasts of Canada (Jamieson et al., 1998). This invasion has led to significant ecological and economic impacts, including the displacement of the American lobster (Homarus americanus), resulting in commercial losses of $44 to $114 million per year. Furthermore, juvenile C. maenas crabs outcompete juvenile Dungeness crabs (Metacarcinus magister), resulting in the population decline of the latter and losses of over $6 million per year in the British Columbia decapod fishery (Colautti et al., 2006; Griffin et al., 2023). The expansion of C. maenas is also particularly destructive to the native ecosystems, with Canadian bivalve populations exhibiting 5- to 10-fold decrease in the presence of C. maenas (Tan and Beal, 2015; Ens et al., 2022). In Atlantic Canada, C. maenas has caused 50% to 100% declines in biomass of Eelgrass meadows that many estuarine organisms rely on for food and shelter (Matheson et al., 2016; Ens et al., 2022). Early awareness of non-indigenous species (NIS) is crucial to mitigating these losses in biodiversity and economy. Citizen support has emerged as a valuable tool for early detection, playing an impactful role in monitoring NIS worldwide (Giovos et al., 2019; Johnson et al., 2020). To determine whether certain Decapoda specimens are indigenous or not, the first step is to recognize them. However, the order Decapoda encompasses an immense diversity of crustaceans, with approximately 10,000 species having been described, including crabs, shrimps, and crayfishes (Hobbs, 2001; Creed, 2009). The great biodiversity of Decapoda species poses challenges for the citizens. Without considerable domain knowledge, local citizens may struggle to recognize new NIS. This is especially true when the specimen is uncommon or unfamiliar to them. It takes time for the citizens to identify the specimen, such as by comparing the morphological features of the specimen against the atlas to find out the most similar species. Meanwhile, it is costly to educate the citizens to classify the highly diverse Decapoda species in global oceans. Moreover, even for professionals, the domain knowledge should be kept updated along with exploding knowledge on biodiversity, such as discoveries of novel species. Furthermore, there are huge classification tasks in monitoring the marine Decapoda community, such as in assessing the impacts from pollution, overexploitation, and other anthropogenic pressures. The classification of Decapoda specimens by human is labor-intensive and time-consuming. Hence, an efficient automated tool for assisting professional taxonomic identification of Decapoda specimens is urgently needed to tackle these challenges.
Deep learning (DL) techniques have demonstrated remarkable performance in automated image analysis of specific Decapoda species, such as shrimp classification in food production lines (Liu et al., 2019) and gender classification of the Chinese mitten crab (Eriocheir sinensis) (Chen et al., 2023). However, despite the availability of numerous DL open-source packages and AutoML platforms, there remains a scarcity of professional tools tailored specifically for the image classification of diverse decapods. Importantly, users often have to undertake dataset preparation, model training, parameter optimization, model deployment, and even the entire process themselves to implement the automated image classification. Therefore, in this study, we aim to develop an automated image classifier and propose a user-friendly web application, named DecapodAI, which leverages multi-modal DL techniques to facilitate image classification of marine Decapoda species on a global scale.
Method
Theoretically, every decapod species has the potential to become a NIS if it is introduced outside of its natural habitats. Thus, to identify NIS, a dataset collecting images of diverse decapods found within the global oceans is required, which is crucial for creating a robust automated image classifier to enhance the performance of the DL models. Firstly, to construct the dataset, the images of Decapoda species, as well as their taxonomic annotation, were collected from the World Register of Marine Species (WoRMS, https://marinespecies.org/), as WoRMS aims at providing an authoritative and comprehensive list of names of global marine species. After manually screening out noise images (such as maps), a total of 1,541 images, which cover 553 species belonging to 317 genera from 102 families, remained for model training and performance evaluation. Each image was labeled with taxonomic annotation at the family, genus, and species levels, respectively. The resource links for these images and their taxonomic annotations are included in Appendix S1.
Secondly, the DL model based on CLIP (Contrastive Language–Image Pretraining) was selected as the backbone network, which proposed a progressive learning and achieved in-depth advances in training (Dong et al., 2022). For instance, CLIP achieved competitive accuracy without requiring as many training examples as traditional models, such as the original ResNet-50 (Radford et al., 2021). Despite the successful application of DL methods to the image classification of marine species, such as EchoAI using EffiecientNetV2 for marine echinoderms (Zhou et al., 2023) and FishAI utilizing Vision Transformer for marine fish (Yang et al., 2024), the accuracy for species with limited labeled images should be improved. Specifically, for certain decapod species recorded in WoRMS, fewer than 10 taxonomically labeled images are available. To address this issue and enhance performance for these underrepresented species, the multi-modal deep network CLIP_L14 was employed. Details about CLIP_L14 could be found in the following website: https://huggingface.co/openai/clip-vit-large-patch14. This approach leverages both text and visual information, achieving superior accuracy in few-shot learning scenarios. The introduction of fine-tuning CLIP is illustrated in Figure 1. CLIP uses contrastive learning to pre-train on large-scale image–text pairs like images and their descriptions to learn cross-modal semantic representations. The image encoder that extracts image features and the text encoder that extracts text features are jointly trained through contrastive loss, making related images and texts closer in the vector space and irrelevant ones farther away. Combined with fine-tuning techniques, the weights of the early layers of the encoder are frozen, and the model parameters are updated using a small learning rate and task loss function on a dataset for a specific task. The main hyperparameters during model training are shown in Appendix S2.
The CLIP model utilizes text encoding to generate text vector [T1, T2, T3…TN] and image encoding to produce image vector [I1, I2, I3…IN]. Combined with fine-tuning techniques, contrastive loss of matrix is mainly used to train the model. In the testing phase, the most probable labels are chosen for test images.
Thirdly, the CLIP network was trained with 1,060 images at the family, genus, and species levels, respectively, using a batch size of 16, and crafted into the image classifier, termed DecapodAI-Core. DecapodAI was derived from “Decapoda” and “AI.” For each input image, DecapodAI-Core returned recognition results in taxonomic names according to probabilities from the highest to the lowest. Subsequently, the accuracy conventionally measures the proportion of images for which the classification inference matches the expected label. To evaluate the performance of DecapodAI-Core, the average accuracies of the top 1 inference (the ones with the highest probability) were calculated for the test dataset at the family, genus, and species levels, respectively. The detailed accuracy metrics for the test dataset across all families are included in Appendix S3. Similarly, the average accuracies for the inference results with the top 2, 3, 4, and 5 highest probabilities were calculated as previously described (Zhou et al., 2023). Finally, online service is provided openly available at the web application, DecapodAI (http://www.csbio.sjtu.edu.cn/bioinf/DecapodAI/). Once the image is successfully uploaded, it usually takes about seconds for the program to process. The results could be accessed by clicking the hyperlinks when the job is finished, and the results are available for download. While uploading the image, email address could be provided optionally, and the link for the results would also be sent to the email provided. To demonstrate the classification performance of DecapodAI, four images were downloaded from the Global Biodiversity Information Facility (GBIF, https://www.gbif.org) and were used as input images. The resource links for these four images are included in Appendix S4.
Results
The DecapodAI model, trained on the dataset using a fine-tuned CLIP, demonstrates its performance at the family, genus, and species levels through the convergence of the loss function during training, as illustrated in Figure 2.
DecapodAI achieved average top 1 classification accuracies on the test dataset of 0.717 at the family level, 0.719 at the genus level, and 0.773 at the species level, as shown in Figure 3. Accuracy improved from top 1 to top 5: at the family level, it increased from 0.717 to 0.902; at the genus level, it increased from 0.719 to 0.914; and at the species level, it increased from 0.773 to 0.939. These variations in accuracy across different taxonomic levels could be attributed to the diversity of text descriptions and dataset quality. In comparison, EfficientNetV2 achieved lower top 1 classification accuracies on the same test dataset: 0.597 at the family level, 0.561 at the genus level, and 0.576 at the species level. These results are notably lower than those obtained with fine-tuned CLIP.
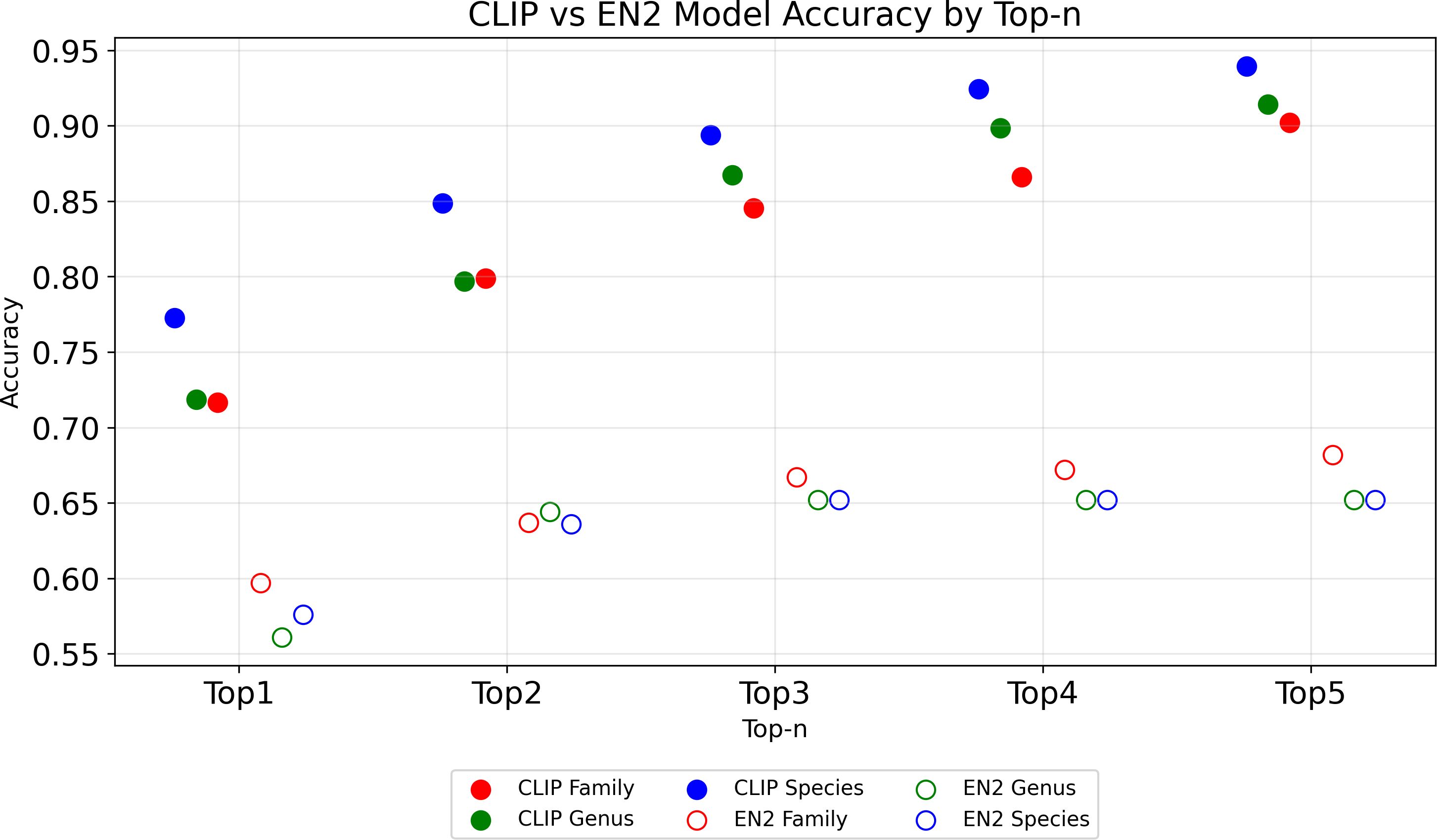
Figure 3. The average accuracy of DecapodAI with CLIP and EfficientNetV2 (EN2) at three taxonomic levels: family, genus, and species.
The accuracy for each family is detailed in Appendix S3, which demonstrates that the DecapodAI model using CLIP outperforms the EfficientNetV2 model, particularly on datasets with a small number of images. As for the invasive species C. maenas in the test dataset, the accuracy reached 1.00. In demonstration of DecapodAI, the images from GBIF were used as input. The top 5 inference results at the species level for each image are shown in Figure 4, along with the probability. Among them, the image of the well-known invasive crab, C. maenas, was correctly classified by DecapodAI. Furthermore, DecapodAI also classified the image of Percnon gibbesi well (Figure 4). P. gibbesi is a native crab species from Eastern Pacific and Tropical Atlantic Ocean (Guillén et al., 2016). Because of its ability to adapt to Mediterranean waters, including its feeding habits, P. gibbesi has spread rapidly and has been proposed to be included in the list of the 100 “Worst Invasives” in the Mediterranean (Puccio et al., 2006; Streftaris and Zenetos, 2006).
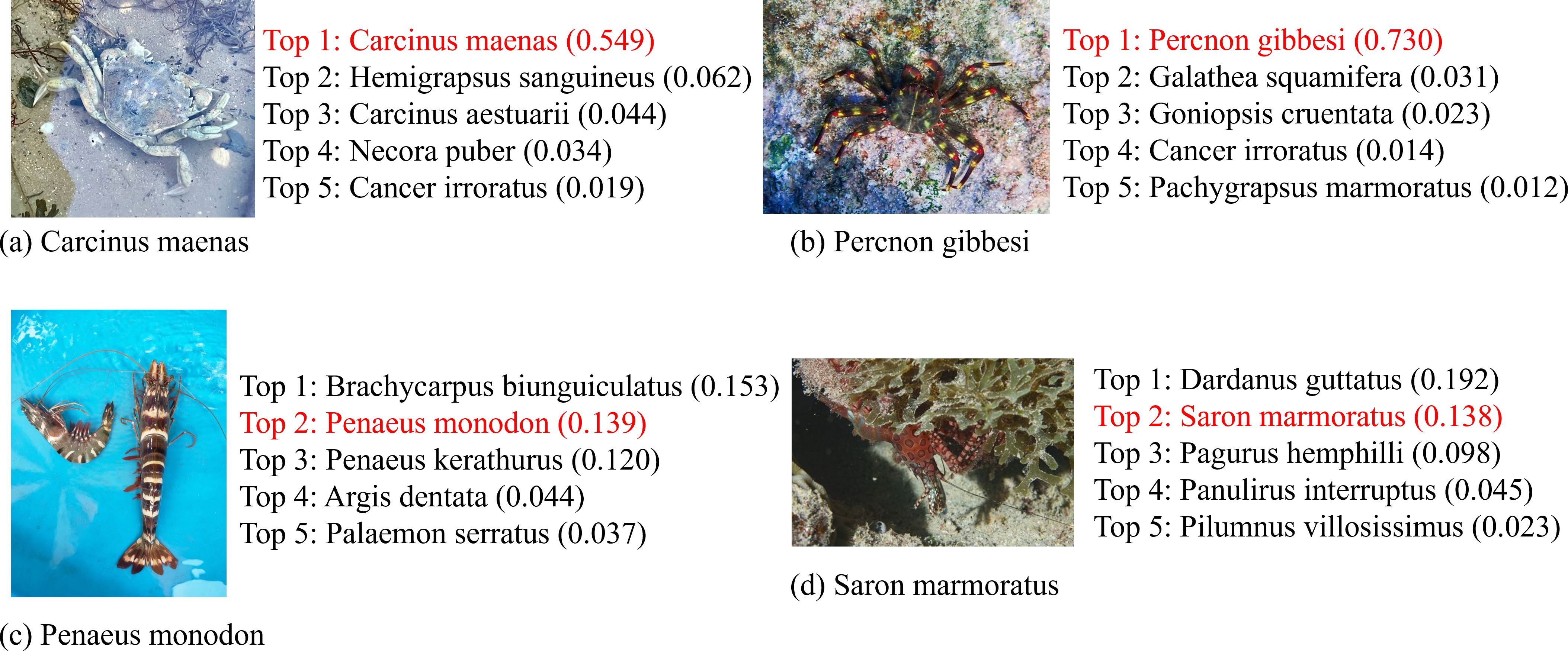
Figure 4. Demonstrations for classification at the species level by DecapodAI. The names in red indicate the matched taxonomic classification derived from the image source.
In addition, the image of the Asian tiger shrimp Penaeus monodon, a NIS in the western north Atlantic and Gulf of Mexico (Fuller et al., 2014), was misidentified as Brachycarpus biunguiculatus at the top 1 inference level (Figure 4). However, DecapodAI correctly classified it at the top 2 level (Figure 4). For another instance, DecapodAI correctly classified the image of Indo-Pacific hippolytid species Saron marmoratus at the top 2 level (Figure 4). This species is among the ornamental pet shrimps in marine aquarium and has been recorded in the Mediterranean Sea from specimens photographed along the Israeli coastline (Rothman et al., 2013).
Discussion
The marine NIS are alarmingly impacting and reshaping the biodiversity in oceans globally. There are numerous documentations on invasive Decapoda species, such as the tiger shrimp P. monodon in almost all the Colombian Caribbean Sea (Aguirre-Pabón et al., 2023) and the blue crab Callinectes sapidus in the Mediterranean Sea (Compa et al., 2023). These invasive Decapoda species not only harm indigenous species but also cause substantial disruptions in biodiversity, posing threats to fisheries. Recognizing the importance of early awareness and timely monitoring of NIS for biodiversity conservation, we have developed DecapodAI, a publicly accessible and easy-to-use web application that bridges these gaps by providing automated image classification for Decapoda species on a global scale. This tool allows users around the world to upload images of the specimens of interest and conduct automated classification without any prior knowledge of taxonomy, machine learning, or programming. For instance, a fisherman or scuba diver could use DecapodAI for the identification of the unfamiliar Decapoda specimens found in bycatches or in photographs. The outputs of classification candidates for the input images offered by DecapodAI could also give clues for further survey, such as taxonomic identification by experts or primer design in environmental DNA (eDNA) analysis based on polymerase chain reaction, which is highly sensitive in detecting NIS. Moreover, the public can take advantage of DecapodAI to raise their knowledge of biodiversity and perception of conservation.
It has to be pointed out that the current version of DecapodAI was developed based on only 1,541 images of 553 species. On average, there are less than three images per species. The baseline version of DecapodAI may not match the accuracy of human experts in fine-grained classification. The performance could be improved in the future as WoRMS updates its image database with more species and images. In addition, staying updated with advances in DL techniques could also enhance the performance of DecapodAI.
In summary, an image classifier based on fine-tuning CLIP with image and text information, DecapodAI, was developed for robustly automated classification of Decapoda species at a global scale. This easy-to-use web application can effectively provide community taxonomic references at three different resolutions (family, genus, and species) for marine decapods of interest. DecapodAI can help alleviate the burden of manually analyzing imagery data and enhance the monitoring efforts, including early awareness of NIS. It is expected to promote public participation and contribute to marine biodiversity conservation practices and has promising application prospects in exploring and monitoring the biodiversity of decapods in global oceans.
Data availability statement
The original contributions presented in the study are included in the article/Supplementary Material. Further inquiries can be directed to the corresponding authors.
Author contributions
PZ: Conceptualization, Data curation, Funding acquisition, Investigation, Methodology, Project administration, Resources, Supervision, Validation, Visualization, Writing – original draft, Writing – review & editing. ZZ: Formal Analysis, Investigation, Methodology, Software, Validation, Visualization, Writing – original draft, Writing – review & editing. CY: Data curation, Formal analysis, Methodology, Validation, Visualization, Writing – original draft, Writing – review & editing. YF: Investigation, Methodology, Software, Validation, Visualization, Writing – review & editing. Y-XB: Writing – original draft, Writing – review & editing. C-SW: Data curation, Funding acquisition, Resources, Writing – review & editing. D-SZ: Data curation, Funding acquisition, Resources, Writing – review & editing. H-BS: Funding acquisition, Resources, Supervision, Writing – review & editing. XP: Funding acquisition, Investigation, Methodology, Project administration, Resources, Software, Supervision, Validation, Writing – original draft, Writing – review & editing.
Funding
The author(s) declare that financial support was received for the research and/or publication of this article. This work was supported by grants from the National Key Research and Development Program of China (2022YFC2804001, 2022YFC2803900, and 2024YFC2814401) and the Oceanic Interdisciplinary Program of Shanghai Jiao Tong University (No. SL2022ZD108).
Conflict of interest
The authors declare that the research was conducted in the absence of any commercial or financial relationships that could be construed as a potential conflict of interest.
Publisher’s note
All claims expressed in this article are solely those of the authors and do not necessarily represent those of their affiliated organizations, or those of the publisher, the editors and the reviewers. Any product that may be evaluated in this article, or claim that may be made by its manufacturer, is not guaranteed or endorsed by the publisher.
Supplementary material
The Supplementary Material for this article can be found online at: https://www.frontiersin.org/articles/10.3389/fmars.2025.1496831/full#supplementary-material
References
Aguirre-Pabón J., Chasqui L., Muñoz E., Narváez-Barandica J. (2023). Multiple origins define the genetic structure of tiger shrimp Penaeus monodon in the Colombian Caribbean Sea. Heliyon 9, e17727. doi: 10.1016/j.heliyon.2023.e17727
Chen X., Zhang Y., Li D., Duan Q. (2023). Chinese mitten crab detection and gender classification method based on GMNet-YOLOv4. Comput. Electron. Agric. 214, 108318. doi: 10.1016/j.compag.2023.108318
Colautti R. I., Bailey S.A., van Overdijk C. D. A., Amundsen K., MacIsaac H. J. (2006). Characterised and projected costs of nonindigenous species in Canada. Biol. Invasions 8, 45–59. doi: 10.1007/s10530-005-0236-y
Compa M., Perelló E., Box A., Colomar V., Pinya S., Sureda A. (2023). Ingestion of microplastics and microfibers by the invasive blue crab Callinectes sapidus (Rathbun 1896) in the Balearic Islands, Spain. Environ. Sci. Pollution Res. 30 (56), 119329–119342. doi: 10.1007/s11356-023-30333-x
Creed R. (2009). “Decapoda,” in Encyclopedia of inland waters. Ed. Likens G. E. (Academic Press, Oxford), 271–279.
Dong X., Jianmin B., Zhang T., Chen D., Gu S., Zhang W., et al. (2022). CLIP itself is a strong fine-tuner: achieving 85.7% and 88.0% Top-1 accuracy with viT-B and viT-L on imageNet. arXiv preprint. doi: 10.48550/arXiv.2212.06138
Ens N. J., Harvey B., Davies M. M., Thomson H. M., Meyers K. J., Yakimishyn J., et al. (2022). The Green Wave: reviewing the environmental impacts of the invasive European green crab (Carcinus maenas) and potential management approaches. Environ. Rev. 30, 306–322. doi: 10.1139/er-2021-0059
FAO (2022). The state of world fisheries and aquaculture 2022 (Rome: Food and Agriculture Organization of the United Nations).
Fuller P., Knott D., Kingsley-Smith P., Morris J., Buckel C., Hunter M., et al. (2014). Invasion of Asian tiger shrimp, Penaeus monodon Fabricius 1798, in the western north Atlantic and Gulf of Mexico. Aquat. Invasions 9, 59–70. doi: 10.3391/ai.2014.9.1.05
Giovos I., Kleitou P., Poursanidis D., Batjakas I., Bernardi G., Crocetta F., et al. (2019). Citizen-science for monitoring marine invasions and stimulating public engagement: a case project from the eastern Mediterranean. Biol. Invasions 21, 3707–3721. doi: 10.1007/s10530-019-02083-w
Griffin R. A., Boyd A., Weinrauch A., Blewett T. A. (2023). Invasive investigation: uptake and transport of l-leucine in the gill epithelium of crustaceans. Conserv. Physiol. 11, coad015. doi: 10.1093/conphys/coad015
Guillén J. E., Santiago J., Triviño A., Soler G., Joaquín M., Gras D. (2016). Assessment of the effects of Percnon gibbesi in taxocenosis decapod crustaceans in the Iberian southeast (Alicante, Spain). Front. Mar. Sci. Conference Abstract: XIX Iberian Symposium on Marine Biology Studies. doi: 10.3389/conf.FMARS.2016.05.00019
Hobbs H. H. (2001). 23 - DECAPODA. Ecology and classification of north american freshwater invertebrates. 2nd ed. Eds. Thorp J. H., Covich A. P. (San Diego: Academic Press), 955–1001.
Jamieson G., D G. E., A A. D., Elner R. W. (1998). Potential ecological implications from the introduction of the European green crab, Carcinus maenas (Linneaus), to British Columbia, Canada, and Washington, USA. J. Natural History 32, 1587–1598. doi: 10.1080/00222939800771121
Johnson B. A., Mader A. D., Dasgupta R., Kumar P. (2020). Citizen science and invasive alien species: An analysis of citizen science initiatives using information and communications technology (ICT) to collect invasive alien species observations. Global Ecol. Conserv. 21, e00812. doi: 10.1016/j.gecco.2019.e00812
Joyce H., Frias J., Kavanagh F., Lynch R., Pagter E., White J., et al. (2022). Plastics, prawns, and patterns: Microplastic loadings in Nephrops norvegicus and surrounding habitat in the North East Atlantic. Sci. Total Environ. 826, 154036. doi: 10.1016/j.scitotenv.2022.154036
Liu Z., Jia X., Xu X. (2019). Study of shrimp recognition methods using smart networks. Comput. Electron. Agric. 165, 104926. doi: 10.1016/j.compag.2019.104926
Marquez F., Idaszkin Y. L. (2021). Crab carapace shape as a biomarker of salt marsh metals pollution. Chemosphere 276, 130195. doi: 10.1016/j.chemosphere.2021.130195
Matheson K., McKenzie C., Rs G., Robichaud D., Bradbury I., Snelgrove P., et al. (2016). Linking eelgrass decline and impacts on associated fish communities to European green crab Carcinus maenas invasion. Marine Ecol. Prog. Ser. 548, 31–45. doi: 10.3354/meps11674
Puccio V., Relini M., Azzurro E., Relini L. O. (2006). Feeding habits of percnon gibbesi (H. Milne edwards 1853) in the sicily strait. Hydrobiologia 557, 79–84. doi: 10.1007/s10750-005-1310-2
Radford A., Kim J., Hallacy C., Ramesh A., Goh G., Agarwal S., et al. (2021). Learning transferable visual models from natural language supervision. In Proceedings of the 38th International Conference on Machine Learning, PMLR 139, 8748–8763.
Rothman S. B. S., Shlagman A., Galil B. S. (2013). Saron marmoratus, an Indo-Pacific marble shrimp (Hippolytidae: Decapoda: Crustacea) in the Mediterranean Sea. Marine Biodiversity Records 6, e129. doi: 10.1017/S1755267213000997
Streftaris N., Zenetos A. (2006). Alien marine species in the mediterranean - the 100 ‘Worst invasives’ and their impact. Mediterranean Marine Sci. 7, 87–118. doi: 10.12681/mms.180
Tan E. B. P., Beal B. F. (2015). Interactions between the invasive European green crab, Carcinus maenas (L.), and juveniles of the soft-shell clam, Mya arenaria L., in eastern Maine, USA. J. Exp. Marine Biol. Ecol. 462, 62–73. doi: 10.1016/j.jembe.2014.10.021
Truchet D. M., Negro C. L., Buzzi N. S., Mora M. C., Marcovecchio J. E. (2023). Assessment of metal contamination in an urbanized estuary (Atlantic Ocean) using crabs as biomonitors: A multiple biomarker approach. Chemosphere 312, 137317. doi: 10.1016/j.chemosphere.2022.137317
Yang C., Zhou P., Wang C.-S., Fu G.-Y., Xu X.-W., Niu Z., et al. (2024). FishAI: Automated hierarchical marine fish image classification with vision transformer. Eng. Rep. 6 (12), e12992. doi: 10.1002/eng2.12992
Keywords: Decapoda, biodiversity, non-indigenous species, invasive species, deep learning, automated image classification
Citation: Zhou P, Zhou Z, Yang C, Fang Y, Bu Y-X, Wang C-S, Zhang D-S, Shen H-B and Pan X (2025) Development of a multi-modal deep-learning-based web application for image classification of marine decapods in conservation practice. Front. Mar. Sci. 12:1496831. doi: 10.3389/fmars.2025.1496831
Received: 15 September 2024; Accepted: 03 April 2025;
Published: 12 May 2025.
Edited by:
Wei Wang, Ocean University of China, ChinaReviewed by:
Zhibin Niu, Tianjin University, ChinaBo Yao, Chinese Academy of Medical Sciences and Peking Union Medical College, China
Copyright © 2025 Zhou, Zhou, Yang, Fang, Bu, Wang, Zhang, Shen and Pan. This is an open-access article distributed under the terms of the Creative Commons Attribution License (CC BY). The use, distribution or reproduction in other forums is permitted, provided the original author(s) and the copyright owner(s) are credited and that the original publication in this journal is cited, in accordance with accepted academic practice. No use, distribution or reproduction is permitted which does not comply with these terms.
*Correspondence: Peng Zhou, emhvdXBlbmdAc2lvLm9yZy5jbg==; Xiaoyong Pan, MjAwOHh5cGFuQHNqdHUuZWR1LmNu